Update README.md
Browse files
README.md
CHANGED
@@ -20,6 +20,8 @@ The model can do one universal classification task: determine whether a hypothes
|
|
20 |
(`entailment` vs. `not_entailment`).
|
21 |
This task format is based on the Natural Language Inference task (NLI).
|
22 |
The task is so universal that any classification task can be reformulated into this task.
|
|
|
|
|
23 |
|
24 |
|
25 |
## Training data
|
@@ -29,12 +31,9 @@ I first created a list of 500+ diverse text classification tasks for 25 professi
|
|
29 |
I then used this as seed data to generate several hundred thousand texts for the different tasks with Mixtral-8x7B-Instruct-v0.1.
|
30 |
The final dataset used is available in the [synthetic_zeroshot_mixtral_v0.1](https://huggingface.co/datasets/MoritzLaurer/synthetic_zeroshot_mixtral_v0.1) dataset
|
31 |
in the subset `mixtral_written_text_for_tasks_v4`. Data curation was done in multiple iterations and I will release more information on this process soon.
|
32 |
-
2. Two commercially-friendly NLI datasets: ([MNLI](https://huggingface.co/datasets/nyu-mll/multi_nli), [FEVER-NLI](https://huggingface.co/datasets/fever).
|
33 |
These datasets were added to increase generalization. Datasets like ANLI were excluded due to their non-commercial license.
|
34 |
|
35 |
-
Note that compared to other NLI models, this model predicts two classes (`entailment` vs. `not_entailment`)
|
36 |
-
as opposed to three classes (entailment/neutral/contradiction)
|
37 |
-
|
38 |
The model was only trained on English data. I will release a multilingual version of this model soon.
|
39 |
For __multilingual use-cases__,
|
40 |
I alternatively recommend machine translating texts to English with libraries like [EasyNMT](https://github.com/UKPLab/EasyNMT).
|
@@ -43,8 +42,7 @@ validation with English data can be easier if you don't speak all languages in y
|
|
43 |
|
44 |
|
45 |
|
46 |
-
|
47 |
-
#### Simple zero-shot classification pipeline
|
48 |
```python
|
49 |
#!pip install transformers[sentencepiece]
|
50 |
from transformers import pipeline
|
@@ -58,10 +56,6 @@ print(output)
|
|
58 |
|
59 |
`multi_label=False` forces the model to decide on only one class. `multi_label=True` enables the model to choose multiple classes.
|
60 |
|
61 |
-
### Details on data and training
|
62 |
-
|
63 |
-
Reproduction code is available here, in the `v2_synthetic_data` directory: https://github.com/MoritzLaurer/zeroshot-classifier/tree/main
|
64 |
-
|
65 |
|
66 |
## Metrics
|
67 |
|
@@ -69,10 +63,6 @@ The model was evaluated on 28 different text classification tasks with the [bala
|
|
69 |
The main reference point is `facebook/bart-large-mnli` which is at the time of writing (27.03.24) the most used commercially-friendly 0-shot classifier.
|
70 |
The different `...zeroshot-v2.0` models were all trained with the same data and the only difference the the underlying foundation model.
|
71 |
|
72 |
-
Note that my `...zeroshot-v1.1` models (e.g. [deberta-v3-base-zeroshot-v1.1-all-33](https://huggingface.co/MoritzLaurer/deberta-v3-base-zeroshot-v1.1-all-33))
|
73 |
-
perform better on these 28 datasets, but they are trained on several datasets with non-commercial licenses.
|
74 |
-
For commercial users, I therefore recommend using the v2.0 model and non-commercial users might get better performance with the v1.1 models.
|
75 |
-
|
76 |
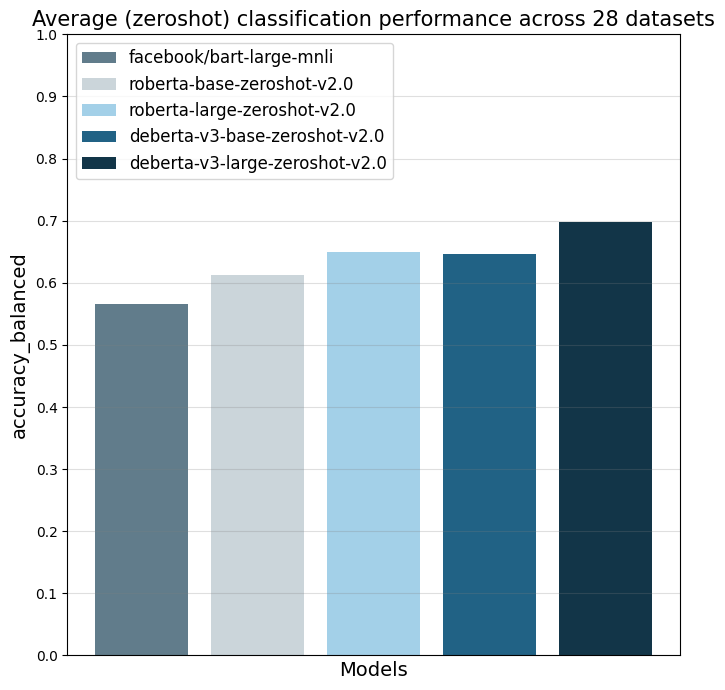
|
77 |
|
78 |
| | facebook/bart-large-mnli | roberta-base-zeroshot-v2.0 | roberta-large-zeroshot-v2.0 | deberta-v3-base-zeroshot-v2.0 | deberta-v3-large-zeroshot-v2.0 |
|
@@ -109,6 +99,21 @@ For commercial users, I therefore recommend using the v2.0 model and non-commerc
|
|
109 |
|
110 |
|
111 |
|
|
|
|
|
|
|
|
|
|
|
|
|
|
|
|
|
|
|
|
|
|
|
|
|
|
|
|
|
|
|
112 |
|
113 |
## Limitations and bias
|
114 |
The model can only do text classification tasks.
|
@@ -149,7 +154,8 @@ If you have questions or ideas for cooperation, contact me at moritz{at}huggingf
|
|
149 |
|
150 |
|
151 |
### Flexible usage and "prompting"
|
152 |
-
You can formulate your own hypotheses by changing the `hypothesis_template` of the zeroshot pipeline.
|
|
|
153 |
|
154 |
```python
|
155 |
from transformers import pipeline
|
|
|
20 |
(`entailment` vs. `not_entailment`).
|
21 |
This task format is based on the Natural Language Inference task (NLI).
|
22 |
The task is so universal that any classification task can be reformulated into this task.
|
23 |
+
Note that compared to other NLI models, this model predicts two classes (`entailment` vs. `not_entailment`)
|
24 |
+
as opposed to three classes (entailment/neutral/contradiction).
|
25 |
|
26 |
|
27 |
## Training data
|
|
|
31 |
I then used this as seed data to generate several hundred thousand texts for the different tasks with Mixtral-8x7B-Instruct-v0.1.
|
32 |
The final dataset used is available in the [synthetic_zeroshot_mixtral_v0.1](https://huggingface.co/datasets/MoritzLaurer/synthetic_zeroshot_mixtral_v0.1) dataset
|
33 |
in the subset `mixtral_written_text_for_tasks_v4`. Data curation was done in multiple iterations and I will release more information on this process soon.
|
34 |
+
2. Two commercially-friendly NLI datasets: ([MNLI](https://huggingface.co/datasets/nyu-mll/multi_nli), [FEVER-NLI](https://huggingface.co/datasets/fever)).
|
35 |
These datasets were added to increase generalization. Datasets like ANLI were excluded due to their non-commercial license.
|
36 |
|
|
|
|
|
|
|
37 |
The model was only trained on English data. I will release a multilingual version of this model soon.
|
38 |
For __multilingual use-cases__,
|
39 |
I alternatively recommend machine translating texts to English with libraries like [EasyNMT](https://github.com/UKPLab/EasyNMT).
|
|
|
42 |
|
43 |
|
44 |
|
45 |
+
## How to use the model
|
|
|
46 |
```python
|
47 |
#!pip install transformers[sentencepiece]
|
48 |
from transformers import pipeline
|
|
|
56 |
|
57 |
`multi_label=False` forces the model to decide on only one class. `multi_label=True` enables the model to choose multiple classes.
|
58 |
|
|
|
|
|
|
|
|
|
59 |
|
60 |
## Metrics
|
61 |
|
|
|
63 |
The main reference point is `facebook/bart-large-mnli` which is at the time of writing (27.03.24) the most used commercially-friendly 0-shot classifier.
|
64 |
The different `...zeroshot-v2.0` models were all trained with the same data and the only difference the the underlying foundation model.
|
65 |
|
|
|
|
|
|
|
|
|
66 |
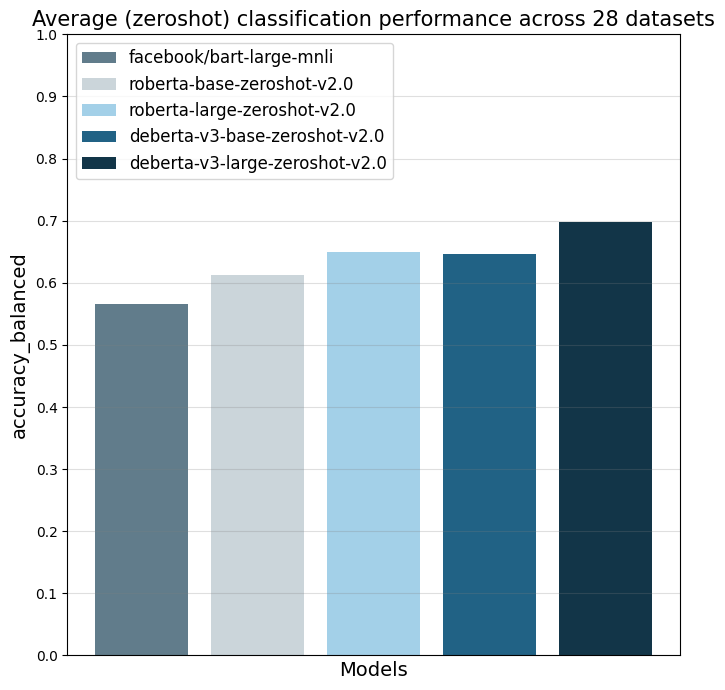
|
67 |
|
68 |
| | facebook/bart-large-mnli | roberta-base-zeroshot-v2.0 | roberta-large-zeroshot-v2.0 | deberta-v3-base-zeroshot-v2.0 | deberta-v3-large-zeroshot-v2.0 |
|
|
|
99 |
|
100 |
|
101 |
|
102 |
+
## When to use which model
|
103 |
+
|
104 |
+
- deberta-v3 vs. roberta: deberta-v3 performs clearly better than roberta, but it is slower.
|
105 |
+
roberta is directly compatible with Hugging Face's production inference TEI containers and flash attention.
|
106 |
+
These containers are a good choice for production use-cases. tl;dr: For accuracy, use a deberta-v3 model.
|
107 |
+
If production inference speed is a concern, you can consider a roberta model (e.g. in a TEI container and [HF Inference Endpoints](https://ui.endpoints.huggingface.co/catalog)).
|
108 |
+
- `zeroshot-v1.1` vs. `zeroshot-v2.0` models: My `zeroshot-v1.1` models (see [Zeroshot Classifier Collection](https://huggingface.co/collections/MoritzLaurer/zeroshot-classifiers-6548b4ff407bb19ff5c3ad6f)))
|
109 |
+
perform better on these 28 datasets, but they are trained on several datasets with non-commercial licenses.
|
110 |
+
For commercial users, I therefore recommend using a v2.0 model and non-commercial users might get better performance with a v1.1 model.
|
111 |
+
|
112 |
+
## Reproduction
|
113 |
+
|
114 |
+
Reproduction code is available here, in the `v2_synthetic_data` directory: https://github.com/MoritzLaurer/zeroshot-classifier/tree/main
|
115 |
+
|
116 |
+
|
117 |
|
118 |
## Limitations and bias
|
119 |
The model can only do text classification tasks.
|
|
|
154 |
|
155 |
|
156 |
### Flexible usage and "prompting"
|
157 |
+
You can formulate your own hypotheses by changing the `hypothesis_template` of the zeroshot pipeline.
|
158 |
+
Similar to "prompt engineering" for LLMs, you can test different formulations of your `hypothesis_template` and verbalized classes to improve performance.
|
159 |
|
160 |
```python
|
161 |
from transformers import pipeline
|