Bridging the Visual Gap: Fine-Tuning Multimodal Models with Knowledge-Adapted Captions
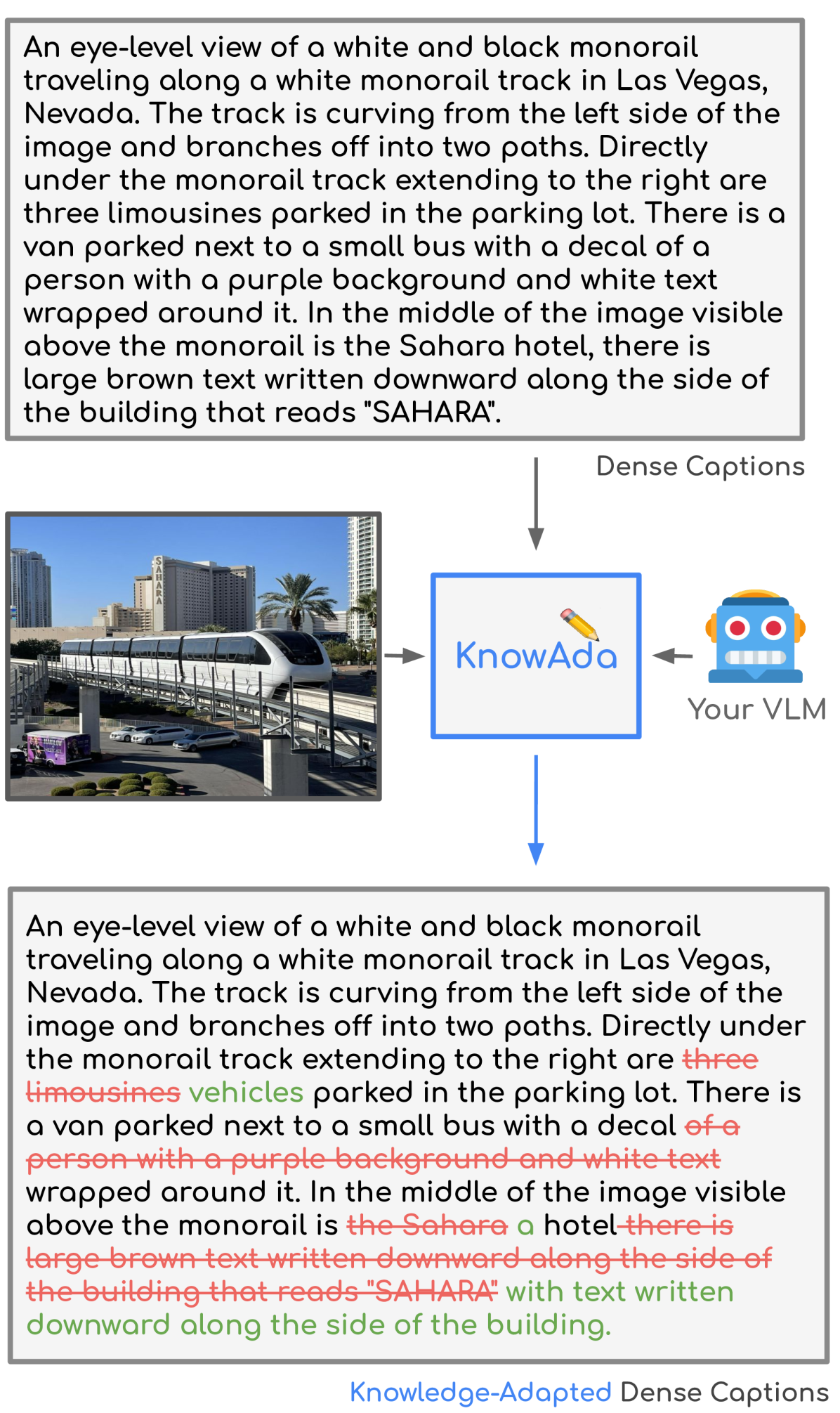
Overview
- Introduces KnowAda, a novel fine-tuning approach for multimodal models.
- Addresses the "visual gap" where existing models struggle with complex visual reasoning.
- Leverages knowledge-adapted captions enriched with external knowledge.
- Demonstrates improved performance on visual question answering (VQA) tasks.
- Shows promise for enhancing multimodal models' reasoning abilities.
Plain English Explanation
KnowAda bridges the gap between visual information and model understanding, boosting performance in complex visual reasoning tasks.
Many current multimodal models, like those explored in Vision-Language Models under Cultural Inclusive Considerations, struggle with tasks requiring deep visual understanding. They often fail to grasp the nuances of an image and how different elements relate to each other. This creates a "visual gap" limiting their effectiveness in tasks like visual question answering (VQA). Existing methods, despite improvements, fall short when the questions require understanding intricate relationships within the image or background knowledge not readily apparent. This gap highlights the need for innovative approaches that enhance the model's understanding of visual content. Similar issues are also found in general language models with respect to Detecting and Mitigating Hallucination in Large Vision-Language Models, highlighting the broader challenges of factual accuracy and coherence in AI.
KnowAda tackles this visual gap by equipping models with "knowledge-adapted captions." Instead of relying solely on basic image descriptions, these captions integrate external knowledge relevant to the scene. Imagine a picture of a historical landmark. A regular caption might simply say "A building." A knowledge-adapted caption would provide context like "The Eiffel Tower, built in 1889 by Gustave Eiffel." This extra information empowers the model to reason more effectively. By providing context and filling in background details, KnowAda helps the model bridge the visual gap and answer complex questions accurately, much like how Directed Domain Fine-tuning: Tailoring Separate Modalities specializes models to specific domains. This approach is similar to giving someone more details before asking a question, leading to more informed and accurate answers, much like how researchers are exploring whether Do More Details Always Introduce More Hallucinations?.
Key Findings
The paper demonstrates that fine-tuning multimodal models with knowledge-adapted captions significantly improves performance on VQA tasks. The enhanced captions provide the model with a richer understanding of the visual content, enabling it to answer questions that require more in-depth reasoning.
Technical Explanation
KnowAda starts by probing existing Vision-Language Models (VLMs) to identify areas where their knowledge is lacking. This analysis informs the creation of knowledge-adapted captions. The captions are generated by combining initial descriptions with relevant external knowledge retrieved using the question as a guide. This process ensures the added information is specifically targeted to enhance the model's ability to answer complex questions. The VLM is then fine-tuned using these enriched captions, enabling it to integrate the provided knowledge into its understanding of the image. The architecture itself is not modified; the improvement comes from the enriched data used for fine-tuning.
This approach leads to improved performance in visual question answering. The model can now leverage the additional context provided by the knowledge-adapted captions to reason more effectively about the image and answer more complex questions. The implications for the field are significant. This work demonstrates a promising way to bridge the visual gap in current multimodal models, paving the way for more sophisticated visual reasoning capabilities in AI.
Critical Analysis
While KnowAda shows promise, it has some limitations. The effectiveness of the approach relies on the quality and relevance of the external knowledge used. Inaccurate or irrelevant knowledge could negatively impact performance. Additionally, the process of generating knowledge-adapted captions might be computationally expensive, particularly for large datasets. Bridging the Visual Gap: Fine-tuning Multimodal Models would benefit from future research exploring more efficient ways to generate and integrate external knowledge. Further investigation is also needed to understand how KnowAda generalizes to other visual reasoning tasks beyond VQA. It's also worth exploring how this approach interacts with techniques designed to mitigate hallucinations, like those described in Detecting and Mitigating Hallucination in Large Vision-Language Models.
Conclusion
KnowAda offers a compelling strategy for enhancing the visual reasoning abilities of multimodal models. By using knowledge-adapted captions, it addresses the visual gap limiting current models. This approach improves performance on complex VQA tasks and opens up exciting avenues for future research. The potential implications extend beyond research, promising more intelligent and capable AI systems in various applications. This could lead to improvements in areas like image search, content understanding, and human-computer interaction. Further research into efficient knowledge integration and generalization to other tasks will be crucial for unlocking the full potential of this approach, as seen in the broader context of multimodal model development, such as Vision-Language Models under Cultural Inclusive Considerations.