html_url
stringlengths 47
49
| title
stringlengths 4
111
| comments
stringlengths 71
20.4k
| body
stringlengths 0
12.9k
β | comment_length_in_words
int64 16
1.61k
| text
stringlengths 100
20.5k
|
---|---|---|---|---|---|
https://github.com/huggingface/datasets/pull/173 | Rm extracted test dirs | Awesome! I guess you might have to add the changes for the MockDLManager now in a different file though because of my last PR - sorry! | All the dummy data used for tests were duplicated. For each dataset, we had one zip file but also its extracted directory. I removed all these directories
Furthermore instead of extracting next to the dummy_data.zip file, we extract in the temp `cached_dir` used for tests, so that all the extracted directories get removed after testing.
Finally there was a bug in the `mock_download_manager` that would let it create directories with invalid names, as in #172. I fixed that by encoding url arguments. I had to rename the dummy data for `scientific_papers` and `cnn_dailymail` (the aws tests don't pass for those 2 in this PR, but they will once aws will be synced, as the local ones do)
Let me know if it sounds good to you @patrickvonplaten . I'm still not entirely familiar with the mock downloader | 26 | text: Rm extracted test dirs
All the dummy data used for tests were duplicated. For each dataset, we had one zip file but also its extracted directory. I removed all these directories
Furthermore instead of extracting next to the dummy_data.zip file, we extract in the temp `cached_dir` used for tests, so that all the extracted directories get removed after testing.
Finally there was a bug in the `mock_download_manager` that would let it create directories with invalid names, as in #172. I fixed that by encoding url arguments. I had to rename the dummy data for `scientific_papers` and `cnn_dailymail` (the aws tests don't pass for those 2 in this PR, but they will once aws will be synced, as the local ones do)
Let me know if it sounds good to you @patrickvonplaten . I'm still not entirely familiar with the mock downloader
Awesome! I guess you might have to add the changes for the MockDLManager now in a different file though because of my last PR - sorry! |
https://github.com/huggingface/datasets/pull/171 | fix squad metric format | One thing for SQuAD is that I wanted to be able to use the SQuAD dataset directly in the metrics and I'm not sure it will be possible with this format.
(maybe it's not really possible in general though) | The format of the squad metric was wrong.
This should fix #143
I tested with
```python3
predictions = [
{'id': '56be4db0acb8001400a502ec', 'prediction_text': 'Denver Broncos'}
]
references = [
{'answers': [{'text': 'Denver Broncos'}], 'id': '56be4db0acb8001400a502ec'}
]
``` | 39 | text: fix squad metric format
The format of the squad metric was wrong.
This should fix #143
I tested with
```python3
predictions = [
{'id': '56be4db0acb8001400a502ec', 'prediction_text': 'Denver Broncos'}
]
references = [
{'answers': [{'text': 'Denver Broncos'}], 'id': '56be4db0acb8001400a502ec'}
]
```
One thing for SQuAD is that I wanted to be able to use the SQuAD dataset directly in the metrics and I'm not sure it will be possible with this format.
(maybe it's not really possible in general though) |
https://github.com/huggingface/datasets/pull/171 | fix squad metric format | This is kinda related to one thing I had in mind which is that we may want to be able to dump our model predictions in a `Dataset` as well so that we don't keep them in memory (and we can export them in a nice format later as well when we will have a serialization formats).
Maybe this is overkill though, I haven't fully wraped my head around this. | The format of the squad metric was wrong.
This should fix #143
I tested with
```python3
predictions = [
{'id': '56be4db0acb8001400a502ec', 'prediction_text': 'Denver Broncos'}
]
references = [
{'answers': [{'text': 'Denver Broncos'}], 'id': '56be4db0acb8001400a502ec'}
]
``` | 70 | text: fix squad metric format
The format of the squad metric was wrong.
This should fix #143
I tested with
```python3
predictions = [
{'id': '56be4db0acb8001400a502ec', 'prediction_text': 'Denver Broncos'}
]
references = [
{'answers': [{'text': 'Denver Broncos'}], 'id': '56be4db0acb8001400a502ec'}
]
```
This is kinda related to one thing I had in mind which is that we may want to be able to dump our model predictions in a `Dataset` as well so that we don't keep them in memory (and we can export them in a nice format later as well when we will have a serialization formats).
Maybe this is overkill though, I haven't fully wraped my head around this. |
https://github.com/huggingface/datasets/pull/171 | fix squad metric format | I'm also perfectly fine with merging this PR in the current state and working on a larger scope later. | The format of the squad metric was wrong.
This should fix #143
I tested with
```python3
predictions = [
{'id': '56be4db0acb8001400a502ec', 'prediction_text': 'Denver Broncos'}
]
references = [
{'answers': [{'text': 'Denver Broncos'}], 'id': '56be4db0acb8001400a502ec'}
]
``` | 19 | text: fix squad metric format
The format of the squad metric was wrong.
This should fix #143
I tested with
```python3
predictions = [
{'id': '56be4db0acb8001400a502ec', 'prediction_text': 'Denver Broncos'}
]
references = [
{'answers': [{'text': 'Denver Broncos'}], 'id': '56be4db0acb8001400a502ec'}
]
```
I'm also perfectly fine with merging this PR in the current state and working on a larger scope later. |
https://github.com/huggingface/datasets/pull/171 | fix squad metric format | This is the format needed to run the official script directly. The format of the squad dataset is different from the input of the metric.
> One thing for SQuAD is that I wanted to be able to use the SQuAD dataset directly in the metrics and I'm not sure it will be possible with this format.
>
> (maybe it's not really possible in general though)
Ok I see. I'll try to use the same format | The format of the squad metric was wrong.
This should fix #143
I tested with
```python3
predictions = [
{'id': '56be4db0acb8001400a502ec', 'prediction_text': 'Denver Broncos'}
]
references = [
{'answers': [{'text': 'Denver Broncos'}], 'id': '56be4db0acb8001400a502ec'}
]
``` | 77 | text: fix squad metric format
The format of the squad metric was wrong.
This should fix #143
I tested with
```python3
predictions = [
{'id': '56be4db0acb8001400a502ec', 'prediction_text': 'Denver Broncos'}
]
references = [
{'answers': [{'text': 'Denver Broncos'}], 'id': '56be4db0acb8001400a502ec'}
]
```
This is the format needed to run the official script directly. The format of the squad dataset is different from the input of the metric.
> One thing for SQuAD is that I wanted to be able to use the SQuAD dataset directly in the metrics and I'm not sure it will be possible with this format.
>
> (maybe it's not really possible in general though)
Ok I see. I'll try to use the same format |
https://github.com/huggingface/datasets/pull/171 | fix squad metric format | Ok with this update I changed the format to fit the squad dataset format.
Now you can do:
```python
squad_dset = nlp.load_dataset("squad")
squad_metric = nlp.load_metric("/Users/quentinlhoest/Desktop/hf/nlp-bis/metrics/squad")
predictions = [
{"id": v["id"], "prediction_text": v["answers"]["text"][0]} # take first possible answer
for v in squad_dset["validation"]
]
squad_metric.compute(predictions, squad_dset["validation"])
``` | The format of the squad metric was wrong.
This should fix #143
I tested with
```python3
predictions = [
{'id': '56be4db0acb8001400a502ec', 'prediction_text': 'Denver Broncos'}
]
references = [
{'answers': [{'text': 'Denver Broncos'}], 'id': '56be4db0acb8001400a502ec'}
]
``` | 45 | text: fix squad metric format
The format of the squad metric was wrong.
This should fix #143
I tested with
```python3
predictions = [
{'id': '56be4db0acb8001400a502ec', 'prediction_text': 'Denver Broncos'}
]
references = [
{'answers': [{'text': 'Denver Broncos'}], 'id': '56be4db0acb8001400a502ec'}
]
```
Ok with this update I changed the format to fit the squad dataset format.
Now you can do:
```python
squad_dset = nlp.load_dataset("squad")
squad_metric = nlp.load_metric("/Users/quentinlhoest/Desktop/hf/nlp-bis/metrics/squad")
predictions = [
{"id": v["id"], "prediction_text": v["answers"]["text"][0]} # take first possible answer
for v in squad_dset["validation"]
]
squad_metric.compute(predictions, squad_dset["validation"])
``` |
https://github.com/huggingface/datasets/pull/169 | Adding Qanta (Quizbowl) Dataset | Hi @EntilZha - sorry for waiting so long until taking action here. We created a new command and a new recipe of how to add dummy_data. Can you maybe rebase to `master` as explained in 7. of https://github.com/huggingface/nlp/blob/master/CONTRIBUTING.md#how-to-contribute-to-nlp and check that your dummy data is correct following the instructions here: https://github.com/huggingface/nlp/blob/master/CONTRIBUTING.md#how-to-add-a-dataset ?
If the tests described in 5. of https://github.com/huggingface/nlp/blob/master/CONTRIBUTING.md#how-to-add-a-dataset pass we can merge the PR :-) | This PR adds the qanta question answering datasets from [Quizbowl: The Case for Incremental Question Answering](https://arxiv.org/abs/1904.04792) and [Trick Me If You Can: Human-in-the-loop Generation of Adversarial Question Answering Examples](https://www.aclweb.org/anthology/Q19-1029/) (adversarial fold)
This partially continues a discussion around fixing dummy data from https://github.com/huggingface/nlp/issues/161
I ran the following code to double check that it works and did some sanity checks on the output. The majority of the code itself is from our `allennlp` version of the dataset reader.
```python
import nlp
# Default is full question
data = nlp.load_dataset('./datasets/qanta')
# Four configs
# Primarily useful for training
data = nlp.load_dataset('./datasets/qanta', 'mode=sentences,char_skip=25')
# Primarily used in evaluation
data = nlp.load_dataset('./datasets/qanta', 'mode=first,char_skip=25')
data = nlp.load_dataset('./datasets/qanta', 'mode=full,char_skip=25')
# Primarily useful in evaluation and "live" play
data = nlp.load_dataset('./datasets/qanta', 'mode=runs,char_skip=25')
``` | 67 | text: Adding Qanta (Quizbowl) Dataset
This PR adds the qanta question answering datasets from [Quizbowl: The Case for Incremental Question Answering](https://arxiv.org/abs/1904.04792) and [Trick Me If You Can: Human-in-the-loop Generation of Adversarial Question Answering Examples](https://www.aclweb.org/anthology/Q19-1029/) (adversarial fold)
This partially continues a discussion around fixing dummy data from https://github.com/huggingface/nlp/issues/161
I ran the following code to double check that it works and did some sanity checks on the output. The majority of the code itself is from our `allennlp` version of the dataset reader.
```python
import nlp
# Default is full question
data = nlp.load_dataset('./datasets/qanta')
# Four configs
# Primarily useful for training
data = nlp.load_dataset('./datasets/qanta', 'mode=sentences,char_skip=25')
# Primarily used in evaluation
data = nlp.load_dataset('./datasets/qanta', 'mode=first,char_skip=25')
data = nlp.load_dataset('./datasets/qanta', 'mode=full,char_skip=25')
# Primarily useful in evaluation and "live" play
data = nlp.load_dataset('./datasets/qanta', 'mode=runs,char_skip=25')
```
Hi @EntilZha - sorry for waiting so long until taking action here. We created a new command and a new recipe of how to add dummy_data. Can you maybe rebase to `master` as explained in 7. of https://github.com/huggingface/nlp/blob/master/CONTRIBUTING.md#how-to-contribute-to-nlp and check that your dummy data is correct following the instructions here: https://github.com/huggingface/nlp/blob/master/CONTRIBUTING.md#how-to-add-a-dataset ?
If the tests described in 5. of https://github.com/huggingface/nlp/blob/master/CONTRIBUTING.md#how-to-add-a-dataset pass we can merge the PR :-) |
https://github.com/huggingface/datasets/pull/169 | Adding Qanta (Quizbowl) Dataset | I updated to the most recent master and followed the steps, but still having the similar error where it can't find the correct file since the path to the directory is given, rather than the individual files within them. This still something wrong about how I'm inputting the data or how the tests are reading it? | This PR adds the qanta question answering datasets from [Quizbowl: The Case for Incremental Question Answering](https://arxiv.org/abs/1904.04792) and [Trick Me If You Can: Human-in-the-loop Generation of Adversarial Question Answering Examples](https://www.aclweb.org/anthology/Q19-1029/) (adversarial fold)
This partially continues a discussion around fixing dummy data from https://github.com/huggingface/nlp/issues/161
I ran the following code to double check that it works and did some sanity checks on the output. The majority of the code itself is from our `allennlp` version of the dataset reader.
```python
import nlp
# Default is full question
data = nlp.load_dataset('./datasets/qanta')
# Four configs
# Primarily useful for training
data = nlp.load_dataset('./datasets/qanta', 'mode=sentences,char_skip=25')
# Primarily used in evaluation
data = nlp.load_dataset('./datasets/qanta', 'mode=first,char_skip=25')
data = nlp.load_dataset('./datasets/qanta', 'mode=full,char_skip=25')
# Primarily useful in evaluation and "live" play
data = nlp.load_dataset('./datasets/qanta', 'mode=runs,char_skip=25')
``` | 56 | text: Adding Qanta (Quizbowl) Dataset
This PR adds the qanta question answering datasets from [Quizbowl: The Case for Incremental Question Answering](https://arxiv.org/abs/1904.04792) and [Trick Me If You Can: Human-in-the-loop Generation of Adversarial Question Answering Examples](https://www.aclweb.org/anthology/Q19-1029/) (adversarial fold)
This partially continues a discussion around fixing dummy data from https://github.com/huggingface/nlp/issues/161
I ran the following code to double check that it works and did some sanity checks on the output. The majority of the code itself is from our `allennlp` version of the dataset reader.
```python
import nlp
# Default is full question
data = nlp.load_dataset('./datasets/qanta')
# Four configs
# Primarily useful for training
data = nlp.load_dataset('./datasets/qanta', 'mode=sentences,char_skip=25')
# Primarily used in evaluation
data = nlp.load_dataset('./datasets/qanta', 'mode=first,char_skip=25')
data = nlp.load_dataset('./datasets/qanta', 'mode=full,char_skip=25')
# Primarily useful in evaluation and "live" play
data = nlp.load_dataset('./datasets/qanta', 'mode=runs,char_skip=25')
```
I updated to the most recent master and followed the steps, but still having the similar error where it can't find the correct file since the path to the directory is given, rather than the individual files within them. This still something wrong about how I'm inputting the data or how the tests are reading it? |
https://github.com/huggingface/datasets/pull/169 | Adding Qanta (Quizbowl) Dataset | It's the dummy_data structure. You actually have to call the dummy data file name `dummy_data` (not .json anything). So there should not be a `dummy_data` folder but for each config only a `dummy_data` which contains your json dummy data. Can you maybe try once more - if it doesn't work I do it for you :-). | This PR adds the qanta question answering datasets from [Quizbowl: The Case for Incremental Question Answering](https://arxiv.org/abs/1904.04792) and [Trick Me If You Can: Human-in-the-loop Generation of Adversarial Question Answering Examples](https://www.aclweb.org/anthology/Q19-1029/) (adversarial fold)
This partially continues a discussion around fixing dummy data from https://github.com/huggingface/nlp/issues/161
I ran the following code to double check that it works and did some sanity checks on the output. The majority of the code itself is from our `allennlp` version of the dataset reader.
```python
import nlp
# Default is full question
data = nlp.load_dataset('./datasets/qanta')
# Four configs
# Primarily useful for training
data = nlp.load_dataset('./datasets/qanta', 'mode=sentences,char_skip=25')
# Primarily used in evaluation
data = nlp.load_dataset('./datasets/qanta', 'mode=first,char_skip=25')
data = nlp.load_dataset('./datasets/qanta', 'mode=full,char_skip=25')
# Primarily useful in evaluation and "live" play
data = nlp.load_dataset('./datasets/qanta', 'mode=runs,char_skip=25')
``` | 56 | text: Adding Qanta (Quizbowl) Dataset
This PR adds the qanta question answering datasets from [Quizbowl: The Case for Incremental Question Answering](https://arxiv.org/abs/1904.04792) and [Trick Me If You Can: Human-in-the-loop Generation of Adversarial Question Answering Examples](https://www.aclweb.org/anthology/Q19-1029/) (adversarial fold)
This partially continues a discussion around fixing dummy data from https://github.com/huggingface/nlp/issues/161
I ran the following code to double check that it works and did some sanity checks on the output. The majority of the code itself is from our `allennlp` version of the dataset reader.
```python
import nlp
# Default is full question
data = nlp.load_dataset('./datasets/qanta')
# Four configs
# Primarily useful for training
data = nlp.load_dataset('./datasets/qanta', 'mode=sentences,char_skip=25')
# Primarily used in evaluation
data = nlp.load_dataset('./datasets/qanta', 'mode=first,char_skip=25')
data = nlp.load_dataset('./datasets/qanta', 'mode=full,char_skip=25')
# Primarily useful in evaluation and "live" play
data = nlp.load_dataset('./datasets/qanta', 'mode=runs,char_skip=25')
```
It's the dummy_data structure. You actually have to call the dummy data file name `dummy_data` (not .json anything). So there should not be a `dummy_data` folder but for each config only a `dummy_data` which contains your json dummy data. Can you maybe try once more - if it doesn't work I do it for you :-). |
https://github.com/huggingface/datasets/pull/169 | Adding Qanta (Quizbowl) Dataset | Would that work if there are multiple files? In my case, I'm including something similar to squad 1.0/2.0 where we have the main dataset + an additional challenge set in different files. Would I have the zip decompress to two files in that case? | This PR adds the qanta question answering datasets from [Quizbowl: The Case for Incremental Question Answering](https://arxiv.org/abs/1904.04792) and [Trick Me If You Can: Human-in-the-loop Generation of Adversarial Question Answering Examples](https://www.aclweb.org/anthology/Q19-1029/) (adversarial fold)
This partially continues a discussion around fixing dummy data from https://github.com/huggingface/nlp/issues/161
I ran the following code to double check that it works and did some sanity checks on the output. The majority of the code itself is from our `allennlp` version of the dataset reader.
```python
import nlp
# Default is full question
data = nlp.load_dataset('./datasets/qanta')
# Four configs
# Primarily useful for training
data = nlp.load_dataset('./datasets/qanta', 'mode=sentences,char_skip=25')
# Primarily used in evaluation
data = nlp.load_dataset('./datasets/qanta', 'mode=first,char_skip=25')
data = nlp.load_dataset('./datasets/qanta', 'mode=full,char_skip=25')
# Primarily useful in evaluation and "live" play
data = nlp.load_dataset('./datasets/qanta', 'mode=runs,char_skip=25')
``` | 44 | text: Adding Qanta (Quizbowl) Dataset
This PR adds the qanta question answering datasets from [Quizbowl: The Case for Incremental Question Answering](https://arxiv.org/abs/1904.04792) and [Trick Me If You Can: Human-in-the-loop Generation of Adversarial Question Answering Examples](https://www.aclweb.org/anthology/Q19-1029/) (adversarial fold)
This partially continues a discussion around fixing dummy data from https://github.com/huggingface/nlp/issues/161
I ran the following code to double check that it works and did some sanity checks on the output. The majority of the code itself is from our `allennlp` version of the dataset reader.
```python
import nlp
# Default is full question
data = nlp.load_dataset('./datasets/qanta')
# Four configs
# Primarily useful for training
data = nlp.load_dataset('./datasets/qanta', 'mode=sentences,char_skip=25')
# Primarily used in evaluation
data = nlp.load_dataset('./datasets/qanta', 'mode=first,char_skip=25')
data = nlp.load_dataset('./datasets/qanta', 'mode=full,char_skip=25')
# Primarily useful in evaluation and "live" play
data = nlp.load_dataset('./datasets/qanta', 'mode=runs,char_skip=25')
```
Would that work if there are multiple files? In my case, I'm including something similar to squad 1.0/2.0 where we have the main dataset + an additional challenge set in different files. Would I have the zip decompress to two files in that case? |
https://github.com/huggingface/datasets/pull/169 | Adding Qanta (Quizbowl) Dataset | This dataset was actually a special case. It helped us improve the dummy data instructions :-), see #195 .Close this PR and merge #194. | This PR adds the qanta question answering datasets from [Quizbowl: The Case for Incremental Question Answering](https://arxiv.org/abs/1904.04792) and [Trick Me If You Can: Human-in-the-loop Generation of Adversarial Question Answering Examples](https://www.aclweb.org/anthology/Q19-1029/) (adversarial fold)
This partially continues a discussion around fixing dummy data from https://github.com/huggingface/nlp/issues/161
I ran the following code to double check that it works and did some sanity checks on the output. The majority of the code itself is from our `allennlp` version of the dataset reader.
```python
import nlp
# Default is full question
data = nlp.load_dataset('./datasets/qanta')
# Four configs
# Primarily useful for training
data = nlp.load_dataset('./datasets/qanta', 'mode=sentences,char_skip=25')
# Primarily used in evaluation
data = nlp.load_dataset('./datasets/qanta', 'mode=first,char_skip=25')
data = nlp.load_dataset('./datasets/qanta', 'mode=full,char_skip=25')
# Primarily useful in evaluation and "live" play
data = nlp.load_dataset('./datasets/qanta', 'mode=runs,char_skip=25')
``` | 24 | text: Adding Qanta (Quizbowl) Dataset
This PR adds the qanta question answering datasets from [Quizbowl: The Case for Incremental Question Answering](https://arxiv.org/abs/1904.04792) and [Trick Me If You Can: Human-in-the-loop Generation of Adversarial Question Answering Examples](https://www.aclweb.org/anthology/Q19-1029/) (adversarial fold)
This partially continues a discussion around fixing dummy data from https://github.com/huggingface/nlp/issues/161
I ran the following code to double check that it works and did some sanity checks on the output. The majority of the code itself is from our `allennlp` version of the dataset reader.
```python
import nlp
# Default is full question
data = nlp.load_dataset('./datasets/qanta')
# Four configs
# Primarily useful for training
data = nlp.load_dataset('./datasets/qanta', 'mode=sentences,char_skip=25')
# Primarily used in evaluation
data = nlp.load_dataset('./datasets/qanta', 'mode=first,char_skip=25')
data = nlp.load_dataset('./datasets/qanta', 'mode=full,char_skip=25')
# Primarily useful in evaluation and "live" play
data = nlp.load_dataset('./datasets/qanta', 'mode=runs,char_skip=25')
```
This dataset was actually a special case. It helped us improve the dummy data instructions :-), see #195 .Close this PR and merge #194. |
https://github.com/huggingface/datasets/pull/152 | Add GLUE config name check | If tests are being added, any guidance on where to add tests would be helpful!
Tagging @thomwolf for review | Fixes #130 by adding a name check to the Glue class | 19 | text: Add GLUE config name check
Fixes #130 by adding a name check to the Glue class
If tests are being added, any guidance on where to add tests would be helpful!
Tagging @thomwolf for review |
https://github.com/huggingface/datasets/pull/152 | Add GLUE config name check | Looks good to me. Is this compatible with the way we are doing tests right now @patrickvonplaten ? | Fixes #130 by adding a name check to the Glue class | 18 | text: Add GLUE config name check
Fixes #130 by adding a name check to the Glue class
Looks good to me. Is this compatible with the way we are doing tests right now @patrickvonplaten ? |
https://github.com/huggingface/datasets/pull/152 | Add GLUE config name check | If the tests pass it should be fine :-)
@Bharat123rox could you check whether the tests pass locally via:
`pytest tests/test_dataset_common.py::LocalDatasetTest::test_load_dataset_glue` | Fixes #130 by adding a name check to the Glue class | 21 | text: Add GLUE config name check
Fixes #130 by adding a name check to the Glue class
If the tests pass it should be fine :-)
@Bharat123rox could you check whether the tests pass locally via:
`pytest tests/test_dataset_common.py::LocalDatasetTest::test_load_dataset_glue` |
https://github.com/huggingface/datasets/pull/152 | Add GLUE config name check | The test fails with an `AssertionError` because the name is not being passed to kwargs, however I'm not sure how to do that, because only the config file is being passed to the tests of all datasets?
I'm guessing this is the corresponding code:
https://github.com/huggingface/nlp/blob/2b3621bb5c78caf02c5a969b8e67fa0c145da4e6/tests/test_dataset_common.py#L141-L143
And these are the logs:
```
___________________ DatasetTest.test_load_dataset_local_glue ___________________
self = <tests.test_dataset_common.DatasetTest testMethod=test_load_dataset_local_glue>
dataset_name = 'glue'
@local
def test_load_dataset_local(self, dataset_name):
# test only first config
if "/" in dataset_name:
logging.info("Skip {} because it is not a canonical dataset")
return
configs = self.dataset_tester.load_all_configs(dataset_name, is_local=True)[:1]
> self.dataset_tester.check_load_dataset(dataset_name, configs, is_local=True)
tests/test_dataset_common.py:200:
_ _ _ _ _ _ _ _ _ _ _ _ _ _ _ _ _ _ _ _ _ _ _ _ _ _ _ _ _ _ _ _ _ _ _ _ _ _ _ _
tests/test_dataset_common.py:74: in check_load_dataset
dataset_builder = dataset_builder_cls(config=config, cache_dir=processed_temp_dir)
_ _ _ _ _ _ _ _ _ _ _ _ _ _ _ _ _ _ _ _ _ _ _ _ _ _ _ _ _ _ _ _ _ _ _ _ _ _ _ _
self = <nlp.datasets.glue.fa7c9f982200144186b2831060b54199cf028e4bbdc5f40acd339ee343342597.glue.Glue object at 0x135c0ea90>
args = ()
kwargs = {'cache_dir': '/var/folders/r6/mnw5ntvn5y72j7d4s1fm273m0000gn/T/tmpa9rpq3tl', 'config': GlueConfig(name='cola', versio...linguistic theory. Each example is a sequence of words annotated\nwith whether it is a grammatical English sentence.')}
def __init__(self, *args, **kwargs):
> assert ('name' in kwargs and kwargs['name'] is not None), "Glue has to be called with a configuration name"
E AssertionError: Glue has to be called with a configuration name
/usr/local/lib/python3.7/site-packages/nlp/datasets/glue/fa7c9f982200144186b2831060b54199cf028e4bbdc5f40acd339ee343342597/glue.py:139: AssertionError
----------------------------- Captured stderr call -----------------------------
INFO:nlp.load:Checking ./datasets/glue/glue.py for additional imports.
INFO:filelock:Lock 5209998288 acquired on ./datasets/glue/glue.py.lock
INFO:nlp.load:Found main folder for dataset ./datasets/glue/glue.py at /usr/local/lib/python3.7/site-packages/nlp/datasets/glue
INFO:nlp.load:Found specific version folder for dataset ./datasets/glue/glue.py at /usr/local/lib/python3.7/site-packages/nlp/datasets/glue/fa7c9f982200144186b2831060b54199cf028e4bbdc5f40acd339ee343342597
INFO:nlp.load:Found script file from ./datasets/glue/glue.py to /usr/local/lib/python3.7/site-packages/nlp/datasets/glue/fa7c9f982200144186b2831060b54199cf028e4bbdc5f40acd339ee343342597/glue.py
INFO:nlp.load:Found dataset infos file from ./datasets/glue/dataset_infos.json to /usr/local/lib/python3.7/site-packages/nlp/datasets/glue/fa7c9f982200144186b2831060b54199cf028e4bbdc5f40acd339ee343342597/dataset_infos.json
INFO:nlp.load:Found metadata file for dataset ./datasets/glue/glue.py at /usr/local/lib/python3.7/site-packages/nlp/datasets/glue/fa7c9f982200144186b2831060b54199cf028e4bbdc5f40acd339ee343342597/glue.json
INFO:filelock:Lock 5209998288 released on ./datasets/glue/glue.py.lock
INFO:nlp.load:Checking ./datasets/glue/glue.py for additional imports.
INFO:filelock:Lock 5196802640 acquired on ./datasets/glue/glue.py.lock
INFO:nlp.load:Found main folder for dataset ./datasets/glue/glue.py at /usr/local/lib/python3.7/site-packages/nlp/datasets/glue
INFO:nlp.load:Found specific version folder for dataset ./datasets/glue/glue.py at /usr/local/lib/python3.7/site-packages/nlp/datasets/glue/fa7c9f982200144186b2831060b54199cf028e4bbdc5f40acd339ee343342597
INFO:nlp.load:Found script file from ./datasets/glue/glue.py to /usr/local/lib/python3.7/site-packages/nlp/datasets/glue/fa7c9f982200144186b2831060b54199cf028e4bbdc5f40acd339ee343342597/glue.py
INFO:nlp.load:Found dataset infos file from ./datasets/glue/dataset_infos.json to /usr/local/lib/python3.7/site-packages/nlp/datasets/glue/fa7c9f982200144186b2831060b54199cf028e4bbdc5f40acd339ee343342597/dataset_infos.json
INFO:nlp.load:Found metadata file for dataset ./datasets/glue/glue.py at /usr/local/lib/python3.7/site-packages/nlp/datasets/glue/fa7c9f982200144186b2831060b54199cf028e4bbdc5f40acd339ee343342597/glue.json
INFO:filelock:Lock 5196802640 released on ./datasets/glue/glue.py.lock
------------------------------ Captured log call -------------------------------
INFO nlp.load:load.py:157 Checking ./datasets/glue/glue.py for additional imports.
INFO filelock:filelock.py:274 Lock 5209998288 acquired on ./datasets/glue/glue.py.lock
INFO nlp.load:load.py:320 Found main folder for dataset ./datasets/glue/glue.py at /usr/local/lib/python3.7/site-packages/nlp/datasets/glue
INFO nlp.load:load.py:333 Found specific version folder for dataset ./datasets/glue/glue.py at /usr/local/lib/python3.7/site-packages/nlp/datasets/glue/fa7c9f982200144186b2831060b54199cf028e4bbdc5f40acd339ee343342597
INFO nlp.load:load.py:346 Found script file from ./datasets/glue/glue.py to /usr/local/lib/python3.7/site-packages/nlp/datasets/glue/fa7c9f982200144186b2831060b54199cf028e4bbdc5f40acd339ee343342597/glue.py
INFO nlp.load:load.py:356 Found dataset infos file from ./datasets/glue/dataset_infos.json to /usr/local/lib/python3.7/site-packages/nlp/datasets/glue/fa7c9f982200144186b2831060b54199cf028e4bbdc5f40acd339ee343342597/dataset_infos.json
INFO nlp.load:load.py:367 Found metadata file for dataset ./datasets/glue/glue.py at /usr/local/lib/python3.7/site-packages/nlp/datasets/glue/fa7c9f982200144186b2831060b54199cf028e4bbdc5f40acd339ee343342597/glue.json
INFO filelock:filelock.py:318 Lock 5209998288 released on ./datasets/glue/glue.py.lock
INFO nlp.load:load.py:157 Checking ./datasets/glue/glue.py for additional imports.
INFO filelock:filelock.py:274 Lock 5196802640 acquired on ./datasets/glue/glue.py.lock
INFO nlp.load:load.py:320 Found main folder for dataset ./datasets/glue/glue.py at /usr/local/lib/python3.7/site-packages/nlp/datasets/glue
INFO nlp.load:load.py:333 Found specific version folder for dataset ./datasets/glue/glue.py at /usr/local/lib/python3.7/site-packages/nlp/datasets/glue/fa7c9f982200144186b2831060b54199cf028e4bbdc5f40acd339ee343342597
INFO nlp.load:load.py:346 Found script file from ./datasets/glue/glue.py to /usr/local/lib/python3.7/site-packages/nlp/datasets/glue/fa7c9f982200144186b2831060b54199cf028e4bbdc5f40acd339ee343342597/glue.py
INFO nlp.load:load.py:356 Found dataset infos file from ./datasets/glue/dataset_infos.json to /usr/local/lib/python3.7/site-packages/nlp/datasets/glue/fa7c9f982200144186b2831060b54199cf028e4bbdc5f40acd339ee343342597/dataset_infos.json
INFO nlp.load:load.py:367 Found metadata file for dataset ./datasets/glue/glue.py at /usr/local/lib/python3.7/site-packages/nlp/datasets/glue/fa7c9f982200144186b2831060b54199cf028e4bbdc5f40acd339ee343342597/glue.json
INFO filelock:filelock.py:318 Lock 5196802640 released on ./datasets/glue/glue.py.lock
``` | Fixes #130 by adding a name check to the Glue class | 511 | text: Add GLUE config name check
Fixes #130 by adding a name check to the Glue class
The test fails with an `AssertionError` because the name is not being passed to kwargs, however I'm not sure how to do that, because only the config file is being passed to the tests of all datasets?
I'm guessing this is the corresponding code:
https://github.com/huggingface/nlp/blob/2b3621bb5c78caf02c5a969b8e67fa0c145da4e6/tests/test_dataset_common.py#L141-L143
And these are the logs:
```
___________________ DatasetTest.test_load_dataset_local_glue ___________________
self = <tests.test_dataset_common.DatasetTest testMethod=test_load_dataset_local_glue>
dataset_name = 'glue'
@local
def test_load_dataset_local(self, dataset_name):
# test only first config
if "/" in dataset_name:
logging.info("Skip {} because it is not a canonical dataset")
return
configs = self.dataset_tester.load_all_configs(dataset_name, is_local=True)[:1]
> self.dataset_tester.check_load_dataset(dataset_name, configs, is_local=True)
tests/test_dataset_common.py:200:
_ _ _ _ _ _ _ _ _ _ _ _ _ _ _ _ _ _ _ _ _ _ _ _ _ _ _ _ _ _ _ _ _ _ _ _ _ _ _ _
tests/test_dataset_common.py:74: in check_load_dataset
dataset_builder = dataset_builder_cls(config=config, cache_dir=processed_temp_dir)
_ _ _ _ _ _ _ _ _ _ _ _ _ _ _ _ _ _ _ _ _ _ _ _ _ _ _ _ _ _ _ _ _ _ _ _ _ _ _ _
self = <nlp.datasets.glue.fa7c9f982200144186b2831060b54199cf028e4bbdc5f40acd339ee343342597.glue.Glue object at 0x135c0ea90>
args = ()
kwargs = {'cache_dir': '/var/folders/r6/mnw5ntvn5y72j7d4s1fm273m0000gn/T/tmpa9rpq3tl', 'config': GlueConfig(name='cola', versio...linguistic theory. Each example is a sequence of words annotated\nwith whether it is a grammatical English sentence.')}
def __init__(self, *args, **kwargs):
> assert ('name' in kwargs and kwargs['name'] is not None), "Glue has to be called with a configuration name"
E AssertionError: Glue has to be called with a configuration name
/usr/local/lib/python3.7/site-packages/nlp/datasets/glue/fa7c9f982200144186b2831060b54199cf028e4bbdc5f40acd339ee343342597/glue.py:139: AssertionError
----------------------------- Captured stderr call -----------------------------
INFO:nlp.load:Checking ./datasets/glue/glue.py for additional imports.
INFO:filelock:Lock 5209998288 acquired on ./datasets/glue/glue.py.lock
INFO:nlp.load:Found main folder for dataset ./datasets/glue/glue.py at /usr/local/lib/python3.7/site-packages/nlp/datasets/glue
INFO:nlp.load:Found specific version folder for dataset ./datasets/glue/glue.py at /usr/local/lib/python3.7/site-packages/nlp/datasets/glue/fa7c9f982200144186b2831060b54199cf028e4bbdc5f40acd339ee343342597
INFO:nlp.load:Found script file from ./datasets/glue/glue.py to /usr/local/lib/python3.7/site-packages/nlp/datasets/glue/fa7c9f982200144186b2831060b54199cf028e4bbdc5f40acd339ee343342597/glue.py
INFO:nlp.load:Found dataset infos file from ./datasets/glue/dataset_infos.json to /usr/local/lib/python3.7/site-packages/nlp/datasets/glue/fa7c9f982200144186b2831060b54199cf028e4bbdc5f40acd339ee343342597/dataset_infos.json
INFO:nlp.load:Found metadata file for dataset ./datasets/glue/glue.py at /usr/local/lib/python3.7/site-packages/nlp/datasets/glue/fa7c9f982200144186b2831060b54199cf028e4bbdc5f40acd339ee343342597/glue.json
INFO:filelock:Lock 5209998288 released on ./datasets/glue/glue.py.lock
INFO:nlp.load:Checking ./datasets/glue/glue.py for additional imports.
INFO:filelock:Lock 5196802640 acquired on ./datasets/glue/glue.py.lock
INFO:nlp.load:Found main folder for dataset ./datasets/glue/glue.py at /usr/local/lib/python3.7/site-packages/nlp/datasets/glue
INFO:nlp.load:Found specific version folder for dataset ./datasets/glue/glue.py at /usr/local/lib/python3.7/site-packages/nlp/datasets/glue/fa7c9f982200144186b2831060b54199cf028e4bbdc5f40acd339ee343342597
INFO:nlp.load:Found script file from ./datasets/glue/glue.py to /usr/local/lib/python3.7/site-packages/nlp/datasets/glue/fa7c9f982200144186b2831060b54199cf028e4bbdc5f40acd339ee343342597/glue.py
INFO:nlp.load:Found dataset infos file from ./datasets/glue/dataset_infos.json to /usr/local/lib/python3.7/site-packages/nlp/datasets/glue/fa7c9f982200144186b2831060b54199cf028e4bbdc5f40acd339ee343342597/dataset_infos.json
INFO:nlp.load:Found metadata file for dataset ./datasets/glue/glue.py at /usr/local/lib/python3.7/site-packages/nlp/datasets/glue/fa7c9f982200144186b2831060b54199cf028e4bbdc5f40acd339ee343342597/glue.json
INFO:filelock:Lock 5196802640 released on ./datasets/glue/glue.py.lock
------------------------------ Captured log call -------------------------------
INFO nlp.load:load.py:157 Checking ./datasets/glue/glue.py for additional imports.
INFO filelock:filelock.py:274 Lock 5209998288 acquired on ./datasets/glue/glue.py.lock
INFO nlp.load:load.py:320 Found main folder for dataset ./datasets/glue/glue.py at /usr/local/lib/python3.7/site-packages/nlp/datasets/glue
INFO nlp.load:load.py:333 Found specific version folder for dataset ./datasets/glue/glue.py at /usr/local/lib/python3.7/site-packages/nlp/datasets/glue/fa7c9f982200144186b2831060b54199cf028e4bbdc5f40acd339ee343342597
INFO nlp.load:load.py:346 Found script file from ./datasets/glue/glue.py to /usr/local/lib/python3.7/site-packages/nlp/datasets/glue/fa7c9f982200144186b2831060b54199cf028e4bbdc5f40acd339ee343342597/glue.py
INFO nlp.load:load.py:356 Found dataset infos file from ./datasets/glue/dataset_infos.json to /usr/local/lib/python3.7/site-packages/nlp/datasets/glue/fa7c9f982200144186b2831060b54199cf028e4bbdc5f40acd339ee343342597/dataset_infos.json
INFO nlp.load:load.py:367 Found metadata file for dataset ./datasets/glue/glue.py at /usr/local/lib/python3.7/site-packages/nlp/datasets/glue/fa7c9f982200144186b2831060b54199cf028e4bbdc5f40acd339ee343342597/glue.json
INFO filelock:filelock.py:318 Lock 5209998288 released on ./datasets/glue/glue.py.lock
INFO nlp.load:load.py:157 Checking ./datasets/glue/glue.py for additional imports.
INFO filelock:filelock.py:274 Lock 5196802640 acquired on ./datasets/glue/glue.py.lock
INFO nlp.load:load.py:320 Found main folder for dataset ./datasets/glue/glue.py at /usr/local/lib/python3.7/site-packages/nlp/datasets/glue
INFO nlp.load:load.py:333 Found specific version folder for dataset ./datasets/glue/glue.py at /usr/local/lib/python3.7/site-packages/nlp/datasets/glue/fa7c9f982200144186b2831060b54199cf028e4bbdc5f40acd339ee343342597
INFO nlp.load:load.py:346 Found script file from ./datasets/glue/glue.py to /usr/local/lib/python3.7/site-packages/nlp/datasets/glue/fa7c9f982200144186b2831060b54199cf028e4bbdc5f40acd339ee343342597/glue.py
INFO nlp.load:load.py:356 Found dataset infos file from ./datasets/glue/dataset_infos.json to /usr/local/lib/python3.7/site-packages/nlp/datasets/glue/fa7c9f982200144186b2831060b54199cf028e4bbdc5f40acd339ee343342597/dataset_infos.json
INFO nlp.load:load.py:367 Found metadata file for dataset ./datasets/glue/glue.py at /usr/local/lib/python3.7/site-packages/nlp/datasets/glue/fa7c9f982200144186b2831060b54199cf028e4bbdc5f40acd339ee343342597/glue.json
INFO filelock:filelock.py:318 Lock 5196802640 released on ./datasets/glue/glue.py.lock
``` |
https://github.com/huggingface/datasets/pull/150 | Add WNUT 17 NER dataset | The PR looks awesome!
Since you have already added a dataset I imagine the tests as described in 5. of https://github.com/huggingface/nlp/blob/master/CONTRIBUTING.md#how-to-add-a-dataset all pass, right @stefan-it ?
I think we are then good to merge this :-) @lhoestq | Hi,
this PR adds the WNUT 17 dataset to `nlp`.
> Emerging and Rare entity recognition
> This shared task focuses on identifying unusual, previously-unseen entities in the context of emerging discussions. Named entities form the basis of many modern approaches to other tasks (like event clustering and summarisation), but recall on them is a real problem in noisy text - even among annotators. This drop tends to be due to novel entities and surface forms. Take for example the tweet βso.. kktny in 30 mins?β - even human experts find entity kktny hard to detect and resolve. This task will evaluate the ability to detect and classify novel, emerging, singleton named entities in noisy text.
>
> The goal of this task is to provide a definition of emerging and of rare entities, and based on that, also datasets for detecting these entities.
More information about the dataset can be found on the [shared task page](https://noisy-text.github.io/2017/emerging-rare-entities.html).
Dataset is taken is taken from their [GitHub repository](https://github.com/leondz/emerging_entities_17), because the data provided in this repository contains minor fixes in the dataset format.
## Usage
Then the WNUT 17 dataset can be used in `nlp` like this:
```python
import nlp
wnut_17 = nlp.load_dataset("./datasets/wnut_17/wnut_17.py")
print(wnut_17)
```
This outputs:
```txt
'train': Dataset(schema: {'id': 'string', 'tokens': 'list<item: string>', 'labels': 'list<item: string>'}, num_rows: 3394)
'validation': Dataset(schema: {'id': 'string', 'tokens': 'list<item: string>', 'labels': 'list<item: string>'}, num_rows: 1009)
'test': Dataset(schema: {'id': 'string', 'tokens': 'list<item: string>', 'labels': 'list<item: string>'}, num_rows: 1287)
```
Number are identical with the ones in [this paper](https://www.ijcai.org/Proceedings/2019/0702.pdf) and are the same as using the `dataset` reader in Flair.
## Features
The following feature format is used to represent a sentence in the WNUT 17 dataset:
| Feature | Example | Description
| ---- | ---- | -----------------
| `id` | `0` | Number (id) of current sentence
| `tokens` | `["AHFA", "extends", "deadline"]` | List of tokens (strings) for a sentence
| `labels` | `["B-group", "O", "O"]` | List of labels (outer span)
The following labels are used in WNUT 17:
```txt
O
B-corporation
I-corporation
B-location
I-location
B-product
I-product
B-person
I-person
B-group
I-group
B-creative-work
I-creative-work
``` | 37 | text: Add WNUT 17 NER dataset
Hi,
this PR adds the WNUT 17 dataset to `nlp`.
> Emerging and Rare entity recognition
> This shared task focuses on identifying unusual, previously-unseen entities in the context of emerging discussions. Named entities form the basis of many modern approaches to other tasks (like event clustering and summarisation), but recall on them is a real problem in noisy text - even among annotators. This drop tends to be due to novel entities and surface forms. Take for example the tweet βso.. kktny in 30 mins?β - even human experts find entity kktny hard to detect and resolve. This task will evaluate the ability to detect and classify novel, emerging, singleton named entities in noisy text.
>
> The goal of this task is to provide a definition of emerging and of rare entities, and based on that, also datasets for detecting these entities.
More information about the dataset can be found on the [shared task page](https://noisy-text.github.io/2017/emerging-rare-entities.html).
Dataset is taken is taken from their [GitHub repository](https://github.com/leondz/emerging_entities_17), because the data provided in this repository contains minor fixes in the dataset format.
## Usage
Then the WNUT 17 dataset can be used in `nlp` like this:
```python
import nlp
wnut_17 = nlp.load_dataset("./datasets/wnut_17/wnut_17.py")
print(wnut_17)
```
This outputs:
```txt
'train': Dataset(schema: {'id': 'string', 'tokens': 'list<item: string>', 'labels': 'list<item: string>'}, num_rows: 3394)
'validation': Dataset(schema: {'id': 'string', 'tokens': 'list<item: string>', 'labels': 'list<item: string>'}, num_rows: 1009)
'test': Dataset(schema: {'id': 'string', 'tokens': 'list<item: string>', 'labels': 'list<item: string>'}, num_rows: 1287)
```
Number are identical with the ones in [this paper](https://www.ijcai.org/Proceedings/2019/0702.pdf) and are the same as using the `dataset` reader in Flair.
## Features
The following feature format is used to represent a sentence in the WNUT 17 dataset:
| Feature | Example | Description
| ---- | ---- | -----------------
| `id` | `0` | Number (id) of current sentence
| `tokens` | `["AHFA", "extends", "deadline"]` | List of tokens (strings) for a sentence
| `labels` | `["B-group", "O", "O"]` | List of labels (outer span)
The following labels are used in WNUT 17:
```txt
O
B-corporation
I-corporation
B-location
I-location
B-product
I-product
B-person
I-person
B-group
I-group
B-creative-work
I-creative-work
```
The PR looks awesome!
Since you have already added a dataset I imagine the tests as described in 5. of https://github.com/huggingface/nlp/blob/master/CONTRIBUTING.md#how-to-add-a-dataset all pass, right @stefan-it ?
I think we are then good to merge this :-) @lhoestq |
https://github.com/huggingface/datasets/pull/150 | Add WNUT 17 NER dataset | Nice !
One thing though: I saw that you copied the `dataset_info.json` (one split info), which is different from the `dataset_infos.json` (split infos of all configs) that we expect.
Could you generate the `dataset_infos.json` file using this command please ?
```
python nlp-cli test datasets/wnut_17 --save_infos --all_configs
``` | Hi,
this PR adds the WNUT 17 dataset to `nlp`.
> Emerging and Rare entity recognition
> This shared task focuses on identifying unusual, previously-unseen entities in the context of emerging discussions. Named entities form the basis of many modern approaches to other tasks (like event clustering and summarisation), but recall on them is a real problem in noisy text - even among annotators. This drop tends to be due to novel entities and surface forms. Take for example the tweet βso.. kktny in 30 mins?β - even human experts find entity kktny hard to detect and resolve. This task will evaluate the ability to detect and classify novel, emerging, singleton named entities in noisy text.
>
> The goal of this task is to provide a definition of emerging and of rare entities, and based on that, also datasets for detecting these entities.
More information about the dataset can be found on the [shared task page](https://noisy-text.github.io/2017/emerging-rare-entities.html).
Dataset is taken is taken from their [GitHub repository](https://github.com/leondz/emerging_entities_17), because the data provided in this repository contains minor fixes in the dataset format.
## Usage
Then the WNUT 17 dataset can be used in `nlp` like this:
```python
import nlp
wnut_17 = nlp.load_dataset("./datasets/wnut_17/wnut_17.py")
print(wnut_17)
```
This outputs:
```txt
'train': Dataset(schema: {'id': 'string', 'tokens': 'list<item: string>', 'labels': 'list<item: string>'}, num_rows: 3394)
'validation': Dataset(schema: {'id': 'string', 'tokens': 'list<item: string>', 'labels': 'list<item: string>'}, num_rows: 1009)
'test': Dataset(schema: {'id': 'string', 'tokens': 'list<item: string>', 'labels': 'list<item: string>'}, num_rows: 1287)
```
Number are identical with the ones in [this paper](https://www.ijcai.org/Proceedings/2019/0702.pdf) and are the same as using the `dataset` reader in Flair.
## Features
The following feature format is used to represent a sentence in the WNUT 17 dataset:
| Feature | Example | Description
| ---- | ---- | -----------------
| `id` | `0` | Number (id) of current sentence
| `tokens` | `["AHFA", "extends", "deadline"]` | List of tokens (strings) for a sentence
| `labels` | `["B-group", "O", "O"]` | List of labels (outer span)
The following labels are used in WNUT 17:
```txt
O
B-corporation
I-corporation
B-location
I-location
B-product
I-product
B-person
I-person
B-group
I-group
B-creative-work
I-creative-work
``` | 48 | text: Add WNUT 17 NER dataset
Hi,
this PR adds the WNUT 17 dataset to `nlp`.
> Emerging and Rare entity recognition
> This shared task focuses on identifying unusual, previously-unseen entities in the context of emerging discussions. Named entities form the basis of many modern approaches to other tasks (like event clustering and summarisation), but recall on them is a real problem in noisy text - even among annotators. This drop tends to be due to novel entities and surface forms. Take for example the tweet βso.. kktny in 30 mins?β - even human experts find entity kktny hard to detect and resolve. This task will evaluate the ability to detect and classify novel, emerging, singleton named entities in noisy text.
>
> The goal of this task is to provide a definition of emerging and of rare entities, and based on that, also datasets for detecting these entities.
More information about the dataset can be found on the [shared task page](https://noisy-text.github.io/2017/emerging-rare-entities.html).
Dataset is taken is taken from their [GitHub repository](https://github.com/leondz/emerging_entities_17), because the data provided in this repository contains minor fixes in the dataset format.
## Usage
Then the WNUT 17 dataset can be used in `nlp` like this:
```python
import nlp
wnut_17 = nlp.load_dataset("./datasets/wnut_17/wnut_17.py")
print(wnut_17)
```
This outputs:
```txt
'train': Dataset(schema: {'id': 'string', 'tokens': 'list<item: string>', 'labels': 'list<item: string>'}, num_rows: 3394)
'validation': Dataset(schema: {'id': 'string', 'tokens': 'list<item: string>', 'labels': 'list<item: string>'}, num_rows: 1009)
'test': Dataset(schema: {'id': 'string', 'tokens': 'list<item: string>', 'labels': 'list<item: string>'}, num_rows: 1287)
```
Number are identical with the ones in [this paper](https://www.ijcai.org/Proceedings/2019/0702.pdf) and are the same as using the `dataset` reader in Flair.
## Features
The following feature format is used to represent a sentence in the WNUT 17 dataset:
| Feature | Example | Description
| ---- | ---- | -----------------
| `id` | `0` | Number (id) of current sentence
| `tokens` | `["AHFA", "extends", "deadline"]` | List of tokens (strings) for a sentence
| `labels` | `["B-group", "O", "O"]` | List of labels (outer span)
The following labels are used in WNUT 17:
```txt
O
B-corporation
I-corporation
B-location
I-location
B-product
I-product
B-person
I-person
B-group
I-group
B-creative-work
I-creative-work
```
Nice !
One thing though: I saw that you copied the `dataset_info.json` (one split info), which is different from the `dataset_infos.json` (split infos of all configs) that we expect.
Could you generate the `dataset_infos.json` file using this command please ?
```
python nlp-cli test datasets/wnut_17 --save_infos --all_configs
``` |
https://github.com/huggingface/datasets/pull/150 | Add WNUT 17 NER dataset | Hi @patrickvonplaten I just rebased onto latest `master` version and executed the commands. All tests passed then :)
@lhoestq thanks for that hint! I've generated and added the `dataset_infos.json` and deleted `dataset_info.json`. | Hi,
this PR adds the WNUT 17 dataset to `nlp`.
> Emerging and Rare entity recognition
> This shared task focuses on identifying unusual, previously-unseen entities in the context of emerging discussions. Named entities form the basis of many modern approaches to other tasks (like event clustering and summarisation), but recall on them is a real problem in noisy text - even among annotators. This drop tends to be due to novel entities and surface forms. Take for example the tweet βso.. kktny in 30 mins?β - even human experts find entity kktny hard to detect and resolve. This task will evaluate the ability to detect and classify novel, emerging, singleton named entities in noisy text.
>
> The goal of this task is to provide a definition of emerging and of rare entities, and based on that, also datasets for detecting these entities.
More information about the dataset can be found on the [shared task page](https://noisy-text.github.io/2017/emerging-rare-entities.html).
Dataset is taken is taken from their [GitHub repository](https://github.com/leondz/emerging_entities_17), because the data provided in this repository contains minor fixes in the dataset format.
## Usage
Then the WNUT 17 dataset can be used in `nlp` like this:
```python
import nlp
wnut_17 = nlp.load_dataset("./datasets/wnut_17/wnut_17.py")
print(wnut_17)
```
This outputs:
```txt
'train': Dataset(schema: {'id': 'string', 'tokens': 'list<item: string>', 'labels': 'list<item: string>'}, num_rows: 3394)
'validation': Dataset(schema: {'id': 'string', 'tokens': 'list<item: string>', 'labels': 'list<item: string>'}, num_rows: 1009)
'test': Dataset(schema: {'id': 'string', 'tokens': 'list<item: string>', 'labels': 'list<item: string>'}, num_rows: 1287)
```
Number are identical with the ones in [this paper](https://www.ijcai.org/Proceedings/2019/0702.pdf) and are the same as using the `dataset` reader in Flair.
## Features
The following feature format is used to represent a sentence in the WNUT 17 dataset:
| Feature | Example | Description
| ---- | ---- | -----------------
| `id` | `0` | Number (id) of current sentence
| `tokens` | `["AHFA", "extends", "deadline"]` | List of tokens (strings) for a sentence
| `labels` | `["B-group", "O", "O"]` | List of labels (outer span)
The following labels are used in WNUT 17:
```txt
O
B-corporation
I-corporation
B-location
I-location
B-product
I-product
B-person
I-person
B-group
I-group
B-creative-work
I-creative-work
``` | 32 | text: Add WNUT 17 NER dataset
Hi,
this PR adds the WNUT 17 dataset to `nlp`.
> Emerging and Rare entity recognition
> This shared task focuses on identifying unusual, previously-unseen entities in the context of emerging discussions. Named entities form the basis of many modern approaches to other tasks (like event clustering and summarisation), but recall on them is a real problem in noisy text - even among annotators. This drop tends to be due to novel entities and surface forms. Take for example the tweet βso.. kktny in 30 mins?β - even human experts find entity kktny hard to detect and resolve. This task will evaluate the ability to detect and classify novel, emerging, singleton named entities in noisy text.
>
> The goal of this task is to provide a definition of emerging and of rare entities, and based on that, also datasets for detecting these entities.
More information about the dataset can be found on the [shared task page](https://noisy-text.github.io/2017/emerging-rare-entities.html).
Dataset is taken is taken from their [GitHub repository](https://github.com/leondz/emerging_entities_17), because the data provided in this repository contains minor fixes in the dataset format.
## Usage
Then the WNUT 17 dataset can be used in `nlp` like this:
```python
import nlp
wnut_17 = nlp.load_dataset("./datasets/wnut_17/wnut_17.py")
print(wnut_17)
```
This outputs:
```txt
'train': Dataset(schema: {'id': 'string', 'tokens': 'list<item: string>', 'labels': 'list<item: string>'}, num_rows: 3394)
'validation': Dataset(schema: {'id': 'string', 'tokens': 'list<item: string>', 'labels': 'list<item: string>'}, num_rows: 1009)
'test': Dataset(schema: {'id': 'string', 'tokens': 'list<item: string>', 'labels': 'list<item: string>'}, num_rows: 1287)
```
Number are identical with the ones in [this paper](https://www.ijcai.org/Proceedings/2019/0702.pdf) and are the same as using the `dataset` reader in Flair.
## Features
The following feature format is used to represent a sentence in the WNUT 17 dataset:
| Feature | Example | Description
| ---- | ---- | -----------------
| `id` | `0` | Number (id) of current sentence
| `tokens` | `["AHFA", "extends", "deadline"]` | List of tokens (strings) for a sentence
| `labels` | `["B-group", "O", "O"]` | List of labels (outer span)
The following labels are used in WNUT 17:
```txt
O
B-corporation
I-corporation
B-location
I-location
B-product
I-product
B-person
I-person
B-group
I-group
B-creative-work
I-creative-work
```
Hi @patrickvonplaten I just rebased onto latest `master` version and executed the commands. All tests passed then :)
@lhoestq thanks for that hint! I've generated and added the `dataset_infos.json` and deleted `dataset_info.json`. |
https://github.com/huggingface/datasets/pull/140 | [Tests] run local tests as default | You are right and I think those are usual best practice :) I'm 100% fine with this^^ | This PR also enables local tests by default
I think it's safer for now to enable both local and aws tests for every commit. The problem currently is that when we do a PR to add a dataset, the dataset is not yet on AWS on therefore not tested on the PR itself. Thus the PR will always be green even if the datasets are not correct. This PR aims at fixing this.
## Suggestion on how to commit to the repo from now on:
Now since the repo is "online", I think we should adopt a couple of best practices:
1) - No direct committing to the repo anymore. Every change should be opened in a PR and be well documented so that we can find it later
2) - Every PR has to be reviewed by at least x people (I guess @thomwolf you should decide here) because we now have to be much more careful when doing changes to the API for backward compatibility, etc...
| 17 | text: [Tests] run local tests as default
This PR also enables local tests by default
I think it's safer for now to enable both local and aws tests for every commit. The problem currently is that when we do a PR to add a dataset, the dataset is not yet on AWS on therefore not tested on the PR itself. Thus the PR will always be green even if the datasets are not correct. This PR aims at fixing this.
## Suggestion on how to commit to the repo from now on:
Now since the repo is "online", I think we should adopt a couple of best practices:
1) - No direct committing to the repo anymore. Every change should be opened in a PR and be well documented so that we can find it later
2) - Every PR has to be reviewed by at least x people (I guess @thomwolf you should decide here) because we now have to be much more careful when doing changes to the API for backward compatibility, etc...
You are right and I think those are usual best practice :) I'm 100% fine with this^^ |
https://github.com/huggingface/datasets/pull/139 | Add GermEval 2014 NER dataset | That's awesome - thanks @stefan-it :-)
Could you maybe rebase to master and check if all dummy data tests are fine. I should have included the local tests directly in the test suite so that all PRs are fully checked: #140 - sorry :D | Hi,
this PR adds the GermEval 2014 NER dataset π
> The GermEval 2014 NER Shared Task builds on a new dataset with German Named Entity annotation [1] with the following properties:
> - The data was sampled from German Wikipedia and News Corpora as a collection of citations.
> - The dataset covers over 31,000 sentences corresponding to over 590,000 tokens.
> - The NER annotation uses the NoSta-D guidelines, which extend the TΓΌbingen Treebank guidelines, using four main NER categories with sub-structure, and annotating embeddings among NEs such as [ORG FC Kickers [LOC Darmstadt]].
Dataset will be downloaded from the [official GermEval 2014 website](https://sites.google.com/site/germeval2014ner/data).
## Dataset format
Here's an example of the dataset format from the original dataset:
```tsv
# http://de.wikipedia.org/wiki/Manfred_Korfmann [2009-10-17]
1 Aufgrund O O
2 seiner O O
3 Initiative O O
4 fand O O
5 2001/2002 O O
6 in O O
7 Stuttgart B-LOC O
8 , O O
9 Braunschweig B-LOC O
10 und O O
11 Bonn B-LOC O
12 eine O O
13 groΓe O O
14 und O O
15 publizistisch O O
16 vielbeachtete O O
17 Troia-Ausstellung B-LOCpart O
18 statt O O
19 , O O
20 β O O
21 Troia B-OTH B-LOC
22 - I-OTH O
23 Traum I-OTH O
24 und I-OTH O
25 Wirklichkeit I-OTH O
26 β O O
27 . O O
```
The sentence is encoded as one token per line (tab separated columns.
The first column contains either a `#`, which signals the source the sentence is cited from and the date it was retrieved, or the token number within the sentence.
The second column contains the token.
Column three and four contain the named entity (in IOB2 scheme).
Outer spans are encoded in the third column, embedded/nested spans in the fourth column.
## Features
I decided to keep most information from the dataset. That means the so called "source" information (where the sentences come from + date information) is also returned for each sentence in the feature vector.
For each sentence in the dataset, one feature vector (`nlp.Features` definition) will be returned:
| Feature | Example | Description
| ---- | ---- | -----------------
| `id` | `0` | Number (id) of current sentence
| `source` | `http://de.wikipedia.org/wiki/Manfred_Korfmann [2009-10-17]` | URL and retrieval date as string
| `tokens` | `["Schwartau", "sagte", ":"]` | List of tokens (strings) for a sentence
| `labels` | `["B-PER", "O", "O"]` | List of labels (outer span)
| `nested-labels` | `["O", "O", "O"]` | List of labels for nested span
## Example
The following command downloads the dataset from the official GermEval 2014 page and pre-processed it:
```bash
python nlp-cli test datasets/germeval_14 --all_configs
```
It then outputs the number for training, development and testset. The training set consists of 24,000 sentences, the development set of 2,200 and the test of 5,100 sentences.
Now it can be imported and used with `nlp`:
```python
import nlp
germeval = nlp.load_dataset("./datasets/germeval_14/germeval_14.py")
assert len(germeval["train"]) == 24000
# Show first sentence of training set:
germeval["train"][0]
``` | 44 | text: Add GermEval 2014 NER dataset
Hi,
this PR adds the GermEval 2014 NER dataset π
> The GermEval 2014 NER Shared Task builds on a new dataset with German Named Entity annotation [1] with the following properties:
> - The data was sampled from German Wikipedia and News Corpora as a collection of citations.
> - The dataset covers over 31,000 sentences corresponding to over 590,000 tokens.
> - The NER annotation uses the NoSta-D guidelines, which extend the TΓΌbingen Treebank guidelines, using four main NER categories with sub-structure, and annotating embeddings among NEs such as [ORG FC Kickers [LOC Darmstadt]].
Dataset will be downloaded from the [official GermEval 2014 website](https://sites.google.com/site/germeval2014ner/data).
## Dataset format
Here's an example of the dataset format from the original dataset:
```tsv
# http://de.wikipedia.org/wiki/Manfred_Korfmann [2009-10-17]
1 Aufgrund O O
2 seiner O O
3 Initiative O O
4 fand O O
5 2001/2002 O O
6 in O O
7 Stuttgart B-LOC O
8 , O O
9 Braunschweig B-LOC O
10 und O O
11 Bonn B-LOC O
12 eine O O
13 groΓe O O
14 und O O
15 publizistisch O O
16 vielbeachtete O O
17 Troia-Ausstellung B-LOCpart O
18 statt O O
19 , O O
20 β O O
21 Troia B-OTH B-LOC
22 - I-OTH O
23 Traum I-OTH O
24 und I-OTH O
25 Wirklichkeit I-OTH O
26 β O O
27 . O O
```
The sentence is encoded as one token per line (tab separated columns.
The first column contains either a `#`, which signals the source the sentence is cited from and the date it was retrieved, or the token number within the sentence.
The second column contains the token.
Column three and four contain the named entity (in IOB2 scheme).
Outer spans are encoded in the third column, embedded/nested spans in the fourth column.
## Features
I decided to keep most information from the dataset. That means the so called "source" information (where the sentences come from + date information) is also returned for each sentence in the feature vector.
For each sentence in the dataset, one feature vector (`nlp.Features` definition) will be returned:
| Feature | Example | Description
| ---- | ---- | -----------------
| `id` | `0` | Number (id) of current sentence
| `source` | `http://de.wikipedia.org/wiki/Manfred_Korfmann [2009-10-17]` | URL and retrieval date as string
| `tokens` | `["Schwartau", "sagte", ":"]` | List of tokens (strings) for a sentence
| `labels` | `["B-PER", "O", "O"]` | List of labels (outer span)
| `nested-labels` | `["O", "O", "O"]` | List of labels for nested span
## Example
The following command downloads the dataset from the official GermEval 2014 page and pre-processed it:
```bash
python nlp-cli test datasets/germeval_14 --all_configs
```
It then outputs the number for training, development and testset. The training set consists of 24,000 sentences, the development set of 2,200 and the test of 5,100 sentences.
Now it can be imported and used with `nlp`:
```python
import nlp
germeval = nlp.load_dataset("./datasets/germeval_14/germeval_14.py")
assert len(germeval["train"]) == 24000
# Show first sentence of training set:
germeval["train"][0]
```
That's awesome - thanks @stefan-it :-)
Could you maybe rebase to master and check if all dummy data tests are fine. I should have included the local tests directly in the test suite so that all PRs are fully checked: #140 - sorry :D |
https://github.com/huggingface/datasets/pull/139 | Add GermEval 2014 NER dataset | @patrickvonplaten Rebased it π
How can it test π€ I used:
```bash
RUN_SLOW=1 RUN_LOCAL=1 pytest tests/test_dataset_common.py::DatasetTest::test_load_real_dataset_local_germeval_14
# and
RUN_SLOW=1 RUN_LOCAL=1 pytest tests/test_dataset_common.py::DatasetTest::test_load_dataset_all_configs_local_germeval_14
```
and the tests still pass :) | Hi,
this PR adds the GermEval 2014 NER dataset π
> The GermEval 2014 NER Shared Task builds on a new dataset with German Named Entity annotation [1] with the following properties:
> - The data was sampled from German Wikipedia and News Corpora as a collection of citations.
> - The dataset covers over 31,000 sentences corresponding to over 590,000 tokens.
> - The NER annotation uses the NoSta-D guidelines, which extend the TΓΌbingen Treebank guidelines, using four main NER categories with sub-structure, and annotating embeddings among NEs such as [ORG FC Kickers [LOC Darmstadt]].
Dataset will be downloaded from the [official GermEval 2014 website](https://sites.google.com/site/germeval2014ner/data).
## Dataset format
Here's an example of the dataset format from the original dataset:
```tsv
# http://de.wikipedia.org/wiki/Manfred_Korfmann [2009-10-17]
1 Aufgrund O O
2 seiner O O
3 Initiative O O
4 fand O O
5 2001/2002 O O
6 in O O
7 Stuttgart B-LOC O
8 , O O
9 Braunschweig B-LOC O
10 und O O
11 Bonn B-LOC O
12 eine O O
13 groΓe O O
14 und O O
15 publizistisch O O
16 vielbeachtete O O
17 Troia-Ausstellung B-LOCpart O
18 statt O O
19 , O O
20 β O O
21 Troia B-OTH B-LOC
22 - I-OTH O
23 Traum I-OTH O
24 und I-OTH O
25 Wirklichkeit I-OTH O
26 β O O
27 . O O
```
The sentence is encoded as one token per line (tab separated columns.
The first column contains either a `#`, which signals the source the sentence is cited from and the date it was retrieved, or the token number within the sentence.
The second column contains the token.
Column three and four contain the named entity (in IOB2 scheme).
Outer spans are encoded in the third column, embedded/nested spans in the fourth column.
## Features
I decided to keep most information from the dataset. That means the so called "source" information (where the sentences come from + date information) is also returned for each sentence in the feature vector.
For each sentence in the dataset, one feature vector (`nlp.Features` definition) will be returned:
| Feature | Example | Description
| ---- | ---- | -----------------
| `id` | `0` | Number (id) of current sentence
| `source` | `http://de.wikipedia.org/wiki/Manfred_Korfmann [2009-10-17]` | URL and retrieval date as string
| `tokens` | `["Schwartau", "sagte", ":"]` | List of tokens (strings) for a sentence
| `labels` | `["B-PER", "O", "O"]` | List of labels (outer span)
| `nested-labels` | `["O", "O", "O"]` | List of labels for nested span
## Example
The following command downloads the dataset from the official GermEval 2014 page and pre-processed it:
```bash
python nlp-cli test datasets/germeval_14 --all_configs
```
It then outputs the number for training, development and testset. The training set consists of 24,000 sentences, the development set of 2,200 and the test of 5,100 sentences.
Now it can be imported and used with `nlp`:
```python
import nlp
germeval = nlp.load_dataset("./datasets/germeval_14/germeval_14.py")
assert len(germeval["train"]) == 24000
# Show first sentence of training set:
germeval["train"][0]
``` | 29 | text: Add GermEval 2014 NER dataset
Hi,
this PR adds the GermEval 2014 NER dataset π
> The GermEval 2014 NER Shared Task builds on a new dataset with German Named Entity annotation [1] with the following properties:
> - The data was sampled from German Wikipedia and News Corpora as a collection of citations.
> - The dataset covers over 31,000 sentences corresponding to over 590,000 tokens.
> - The NER annotation uses the NoSta-D guidelines, which extend the TΓΌbingen Treebank guidelines, using four main NER categories with sub-structure, and annotating embeddings among NEs such as [ORG FC Kickers [LOC Darmstadt]].
Dataset will be downloaded from the [official GermEval 2014 website](https://sites.google.com/site/germeval2014ner/data).
## Dataset format
Here's an example of the dataset format from the original dataset:
```tsv
# http://de.wikipedia.org/wiki/Manfred_Korfmann [2009-10-17]
1 Aufgrund O O
2 seiner O O
3 Initiative O O
4 fand O O
5 2001/2002 O O
6 in O O
7 Stuttgart B-LOC O
8 , O O
9 Braunschweig B-LOC O
10 und O O
11 Bonn B-LOC O
12 eine O O
13 groΓe O O
14 und O O
15 publizistisch O O
16 vielbeachtete O O
17 Troia-Ausstellung B-LOCpart O
18 statt O O
19 , O O
20 β O O
21 Troia B-OTH B-LOC
22 - I-OTH O
23 Traum I-OTH O
24 und I-OTH O
25 Wirklichkeit I-OTH O
26 β O O
27 . O O
```
The sentence is encoded as one token per line (tab separated columns.
The first column contains either a `#`, which signals the source the sentence is cited from and the date it was retrieved, or the token number within the sentence.
The second column contains the token.
Column three and four contain the named entity (in IOB2 scheme).
Outer spans are encoded in the third column, embedded/nested spans in the fourth column.
## Features
I decided to keep most information from the dataset. That means the so called "source" information (where the sentences come from + date information) is also returned for each sentence in the feature vector.
For each sentence in the dataset, one feature vector (`nlp.Features` definition) will be returned:
| Feature | Example | Description
| ---- | ---- | -----------------
| `id` | `0` | Number (id) of current sentence
| `source` | `http://de.wikipedia.org/wiki/Manfred_Korfmann [2009-10-17]` | URL and retrieval date as string
| `tokens` | `["Schwartau", "sagte", ":"]` | List of tokens (strings) for a sentence
| `labels` | `["B-PER", "O", "O"]` | List of labels (outer span)
| `nested-labels` | `["O", "O", "O"]` | List of labels for nested span
## Example
The following command downloads the dataset from the official GermEval 2014 page and pre-processed it:
```bash
python nlp-cli test datasets/germeval_14 --all_configs
```
It then outputs the number for training, development and testset. The training set consists of 24,000 sentences, the development set of 2,200 and the test of 5,100 sentences.
Now it can be imported and used with `nlp`:
```python
import nlp
germeval = nlp.load_dataset("./datasets/germeval_14/germeval_14.py")
assert len(germeval["train"]) == 24000
# Show first sentence of training set:
germeval["train"][0]
```
@patrickvonplaten Rebased it π
How can it test π€ I used:
```bash
RUN_SLOW=1 RUN_LOCAL=1 pytest tests/test_dataset_common.py::DatasetTest::test_load_real_dataset_local_germeval_14
# and
RUN_SLOW=1 RUN_LOCAL=1 pytest tests/test_dataset_common.py::DatasetTest::test_load_dataset_all_configs_local_germeval_14
```
and the tests still pass :) |
https://github.com/huggingface/datasets/pull/139 | Add GermEval 2014 NER dataset | Perfect, if these tests pass that's great - I'll merge the PR then :-) Was it very difficult to create the dummy data structure? | Hi,
this PR adds the GermEval 2014 NER dataset π
> The GermEval 2014 NER Shared Task builds on a new dataset with German Named Entity annotation [1] with the following properties:
> - The data was sampled from German Wikipedia and News Corpora as a collection of citations.
> - The dataset covers over 31,000 sentences corresponding to over 590,000 tokens.
> - The NER annotation uses the NoSta-D guidelines, which extend the TΓΌbingen Treebank guidelines, using four main NER categories with sub-structure, and annotating embeddings among NEs such as [ORG FC Kickers [LOC Darmstadt]].
Dataset will be downloaded from the [official GermEval 2014 website](https://sites.google.com/site/germeval2014ner/data).
## Dataset format
Here's an example of the dataset format from the original dataset:
```tsv
# http://de.wikipedia.org/wiki/Manfred_Korfmann [2009-10-17]
1 Aufgrund O O
2 seiner O O
3 Initiative O O
4 fand O O
5 2001/2002 O O
6 in O O
7 Stuttgart B-LOC O
8 , O O
9 Braunschweig B-LOC O
10 und O O
11 Bonn B-LOC O
12 eine O O
13 groΓe O O
14 und O O
15 publizistisch O O
16 vielbeachtete O O
17 Troia-Ausstellung B-LOCpart O
18 statt O O
19 , O O
20 β O O
21 Troia B-OTH B-LOC
22 - I-OTH O
23 Traum I-OTH O
24 und I-OTH O
25 Wirklichkeit I-OTH O
26 β O O
27 . O O
```
The sentence is encoded as one token per line (tab separated columns.
The first column contains either a `#`, which signals the source the sentence is cited from and the date it was retrieved, or the token number within the sentence.
The second column contains the token.
Column three and four contain the named entity (in IOB2 scheme).
Outer spans are encoded in the third column, embedded/nested spans in the fourth column.
## Features
I decided to keep most information from the dataset. That means the so called "source" information (where the sentences come from + date information) is also returned for each sentence in the feature vector.
For each sentence in the dataset, one feature vector (`nlp.Features` definition) will be returned:
| Feature | Example | Description
| ---- | ---- | -----------------
| `id` | `0` | Number (id) of current sentence
| `source` | `http://de.wikipedia.org/wiki/Manfred_Korfmann [2009-10-17]` | URL and retrieval date as string
| `tokens` | `["Schwartau", "sagte", ":"]` | List of tokens (strings) for a sentence
| `labels` | `["B-PER", "O", "O"]` | List of labels (outer span)
| `nested-labels` | `["O", "O", "O"]` | List of labels for nested span
## Example
The following command downloads the dataset from the official GermEval 2014 page and pre-processed it:
```bash
python nlp-cli test datasets/germeval_14 --all_configs
```
It then outputs the number for training, development and testset. The training set consists of 24,000 sentences, the development set of 2,200 and the test of 5,100 sentences.
Now it can be imported and used with `nlp`:
```python
import nlp
germeval = nlp.load_dataset("./datasets/germeval_14/germeval_14.py")
assert len(germeval["train"]) == 24000
# Show first sentence of training set:
germeval["train"][0]
``` | 24 | text: Add GermEval 2014 NER dataset
Hi,
this PR adds the GermEval 2014 NER dataset π
> The GermEval 2014 NER Shared Task builds on a new dataset with German Named Entity annotation [1] with the following properties:
> - The data was sampled from German Wikipedia and News Corpora as a collection of citations.
> - The dataset covers over 31,000 sentences corresponding to over 590,000 tokens.
> - The NER annotation uses the NoSta-D guidelines, which extend the TΓΌbingen Treebank guidelines, using four main NER categories with sub-structure, and annotating embeddings among NEs such as [ORG FC Kickers [LOC Darmstadt]].
Dataset will be downloaded from the [official GermEval 2014 website](https://sites.google.com/site/germeval2014ner/data).
## Dataset format
Here's an example of the dataset format from the original dataset:
```tsv
# http://de.wikipedia.org/wiki/Manfred_Korfmann [2009-10-17]
1 Aufgrund O O
2 seiner O O
3 Initiative O O
4 fand O O
5 2001/2002 O O
6 in O O
7 Stuttgart B-LOC O
8 , O O
9 Braunschweig B-LOC O
10 und O O
11 Bonn B-LOC O
12 eine O O
13 groΓe O O
14 und O O
15 publizistisch O O
16 vielbeachtete O O
17 Troia-Ausstellung B-LOCpart O
18 statt O O
19 , O O
20 β O O
21 Troia B-OTH B-LOC
22 - I-OTH O
23 Traum I-OTH O
24 und I-OTH O
25 Wirklichkeit I-OTH O
26 β O O
27 . O O
```
The sentence is encoded as one token per line (tab separated columns.
The first column contains either a `#`, which signals the source the sentence is cited from and the date it was retrieved, or the token number within the sentence.
The second column contains the token.
Column three and four contain the named entity (in IOB2 scheme).
Outer spans are encoded in the third column, embedded/nested spans in the fourth column.
## Features
I decided to keep most information from the dataset. That means the so called "source" information (where the sentences come from + date information) is also returned for each sentence in the feature vector.
For each sentence in the dataset, one feature vector (`nlp.Features` definition) will be returned:
| Feature | Example | Description
| ---- | ---- | -----------------
| `id` | `0` | Number (id) of current sentence
| `source` | `http://de.wikipedia.org/wiki/Manfred_Korfmann [2009-10-17]` | URL and retrieval date as string
| `tokens` | `["Schwartau", "sagte", ":"]` | List of tokens (strings) for a sentence
| `labels` | `["B-PER", "O", "O"]` | List of labels (outer span)
| `nested-labels` | `["O", "O", "O"]` | List of labels for nested span
## Example
The following command downloads the dataset from the official GermEval 2014 page and pre-processed it:
```bash
python nlp-cli test datasets/germeval_14 --all_configs
```
It then outputs the number for training, development and testset. The training set consists of 24,000 sentences, the development set of 2,200 and the test of 5,100 sentences.
Now it can be imported and used with `nlp`:
```python
import nlp
germeval = nlp.load_dataset("./datasets/germeval_14/germeval_14.py")
assert len(germeval["train"]) == 24000
# Show first sentence of training set:
germeval["train"][0]
```
Perfect, if these tests pass that's great - I'll merge the PR then :-) Was it very difficult to create the dummy data structure? |
https://github.com/huggingface/datasets/pull/123 | [Tests] Local => aws | For each dataset, If there exist a `dataset_info.json`, then the command `nlp-cli test path/to/my/dataset --al_configs` is successful only if the `dataset_infos.json` is correct. The infos are correct if the size and checksums of the downloaded file are correct, and if the number of examples in each split are correct.
Note: the `test` command is supposed to test the script, that's why it runs the script even if the cached files already exist. Let me know if it's good to you. | ## Change default Test from local => aws
As a default we set` aws=True`, `Local=False`, `slow=False`
### 1. RUN_AWS=1 (default)
This runs 4 tests per dataset script.
a) Does the dataset script have a valid etag / Can it be reached on AWS?
b) Can we load its `builder_class`?
c) Can we load **all** dataset configs?
d) _Most importantly_: Can we load the dataset?
Important - we currently only test the first config of each dataset to reduce test time. Total test time is around 1min20s.
### 2. RUN_LOCAL=1 RUN_AWS=0
***This should be done when debugging dataset scripts of the ./datasets folder***
This only runs 1 test per dataset test, which is equivalent to aws d) - Can we load the dataset from the local `datasets` directory?
### 3. RUN_SLOW=1
We should set up to run these tests maybe 1 time per week ? @thomwolf
The `slow` tests include two more important tests.
e) Can we load the dataset with all possible configs? This test will probably fail at the moment because a lot of dummy data is missing. We should add the dummy data step by step to be sure that all configs work.
f) Test that the actual dataset can be loaded. This will take quite some time to run, but is important to make sure that the "real" data can be loaded. It will also test whether the dataset script has the correct checksums file which is currently not tested with `aws=True`. @lhoestq - is there an easy way to check cheaply whether the `dataset_info.json` is correct for each dataset script? | 80 | text: [Tests] Local => aws
## Change default Test from local => aws
As a default we set` aws=True`, `Local=False`, `slow=False`
### 1. RUN_AWS=1 (default)
This runs 4 tests per dataset script.
a) Does the dataset script have a valid etag / Can it be reached on AWS?
b) Can we load its `builder_class`?
c) Can we load **all** dataset configs?
d) _Most importantly_: Can we load the dataset?
Important - we currently only test the first config of each dataset to reduce test time. Total test time is around 1min20s.
### 2. RUN_LOCAL=1 RUN_AWS=0
***This should be done when debugging dataset scripts of the ./datasets folder***
This only runs 1 test per dataset test, which is equivalent to aws d) - Can we load the dataset from the local `datasets` directory?
### 3. RUN_SLOW=1
We should set up to run these tests maybe 1 time per week ? @thomwolf
The `slow` tests include two more important tests.
e) Can we load the dataset with all possible configs? This test will probably fail at the moment because a lot of dummy data is missing. We should add the dummy data step by step to be sure that all configs work.
f) Test that the actual dataset can be loaded. This will take quite some time to run, but is important to make sure that the "real" data can be loaded. It will also test whether the dataset script has the correct checksums file which is currently not tested with `aws=True`. @lhoestq - is there an easy way to check cheaply whether the `dataset_info.json` is correct for each dataset script?
For each dataset, If there exist a `dataset_info.json`, then the command `nlp-cli test path/to/my/dataset --al_configs` is successful only if the `dataset_infos.json` is correct. The infos are correct if the size and checksums of the downloaded file are correct, and if the number of examples in each split are correct.
Note: the `test` command is supposed to test the script, that's why it runs the script even if the cached files already exist. Let me know if it's good to you. |
https://github.com/huggingface/datasets/pull/123 | [Tests] Local => aws | > For each dataset, If there exist a `dataset_info.json`, then the command `nlp-cli test path/to/my/dataset --al_configs` is successful only if the `dataset_infos.json` is correct. The infos are correct if the size and checksums of the downloaded file are correct, and if the number of examples in each split are correct.
>
> Note: the `test` command is supposed to test the script, that's why it runs the script even if the cached files already exist. Let me know if it's good to you.
Does it have to download the whole data to check if the checksums are correct? I guess so no? | ## Change default Test from local => aws
As a default we set` aws=True`, `Local=False`, `slow=False`
### 1. RUN_AWS=1 (default)
This runs 4 tests per dataset script.
a) Does the dataset script have a valid etag / Can it be reached on AWS?
b) Can we load its `builder_class`?
c) Can we load **all** dataset configs?
d) _Most importantly_: Can we load the dataset?
Important - we currently only test the first config of each dataset to reduce test time. Total test time is around 1min20s.
### 2. RUN_LOCAL=1 RUN_AWS=0
***This should be done when debugging dataset scripts of the ./datasets folder***
This only runs 1 test per dataset test, which is equivalent to aws d) - Can we load the dataset from the local `datasets` directory?
### 3. RUN_SLOW=1
We should set up to run these tests maybe 1 time per week ? @thomwolf
The `slow` tests include two more important tests.
e) Can we load the dataset with all possible configs? This test will probably fail at the moment because a lot of dummy data is missing. We should add the dummy data step by step to be sure that all configs work.
f) Test that the actual dataset can be loaded. This will take quite some time to run, but is important to make sure that the "real" data can be loaded. It will also test whether the dataset script has the correct checksums file which is currently not tested with `aws=True`. @lhoestq - is there an easy way to check cheaply whether the `dataset_info.json` is correct for each dataset script? | 102 | text: [Tests] Local => aws
## Change default Test from local => aws
As a default we set` aws=True`, `Local=False`, `slow=False`
### 1. RUN_AWS=1 (default)
This runs 4 tests per dataset script.
a) Does the dataset script have a valid etag / Can it be reached on AWS?
b) Can we load its `builder_class`?
c) Can we load **all** dataset configs?
d) _Most importantly_: Can we load the dataset?
Important - we currently only test the first config of each dataset to reduce test time. Total test time is around 1min20s.
### 2. RUN_LOCAL=1 RUN_AWS=0
***This should be done when debugging dataset scripts of the ./datasets folder***
This only runs 1 test per dataset test, which is equivalent to aws d) - Can we load the dataset from the local `datasets` directory?
### 3. RUN_SLOW=1
We should set up to run these tests maybe 1 time per week ? @thomwolf
The `slow` tests include two more important tests.
e) Can we load the dataset with all possible configs? This test will probably fail at the moment because a lot of dummy data is missing. We should add the dummy data step by step to be sure that all configs work.
f) Test that the actual dataset can be loaded. This will take quite some time to run, but is important to make sure that the "real" data can be loaded. It will also test whether the dataset script has the correct checksums file which is currently not tested with `aws=True`. @lhoestq - is there an easy way to check cheaply whether the `dataset_info.json` is correct for each dataset script?
> For each dataset, If there exist a `dataset_info.json`, then the command `nlp-cli test path/to/my/dataset --al_configs` is successful only if the `dataset_infos.json` is correct. The infos are correct if the size and checksums of the downloaded file are correct, and if the number of examples in each split are correct.
>
> Note: the `test` command is supposed to test the script, that's why it runs the script even if the cached files already exist. Let me know if it's good to you.
Does it have to download the whole data to check if the checksums are correct? I guess so no? |
https://github.com/huggingface/datasets/pull/123 | [Tests] Local => aws | > > For each dataset, If there exist a `dataset_info.json`, then the command `nlp-cli test path/to/my/dataset --al_configs` is successful only if the `dataset_infos.json` is correct. The infos are correct if the size and checksums of the downloaded file are correct, and if the number of examples in each split are correct.
> > Note: the `test` command is supposed to test the script, that's why it runs the script even if the cached files already exist. Let me know if it's good to you.
>
> Does it have to download the whole data to check if the checksums are correct? I guess so no?
Yes it has to download them all (unless they were already downloaded in which case it just uses the cached downloaded files). | ## Change default Test from local => aws
As a default we set` aws=True`, `Local=False`, `slow=False`
### 1. RUN_AWS=1 (default)
This runs 4 tests per dataset script.
a) Does the dataset script have a valid etag / Can it be reached on AWS?
b) Can we load its `builder_class`?
c) Can we load **all** dataset configs?
d) _Most importantly_: Can we load the dataset?
Important - we currently only test the first config of each dataset to reduce test time. Total test time is around 1min20s.
### 2. RUN_LOCAL=1 RUN_AWS=0
***This should be done when debugging dataset scripts of the ./datasets folder***
This only runs 1 test per dataset test, which is equivalent to aws d) - Can we load the dataset from the local `datasets` directory?
### 3. RUN_SLOW=1
We should set up to run these tests maybe 1 time per week ? @thomwolf
The `slow` tests include two more important tests.
e) Can we load the dataset with all possible configs? This test will probably fail at the moment because a lot of dummy data is missing. We should add the dummy data step by step to be sure that all configs work.
f) Test that the actual dataset can be loaded. This will take quite some time to run, but is important to make sure that the "real" data can be loaded. It will also test whether the dataset script has the correct checksums file which is currently not tested with `aws=True`. @lhoestq - is there an easy way to check cheaply whether the `dataset_info.json` is correct for each dataset script? | 127 | text: [Tests] Local => aws
## Change default Test from local => aws
As a default we set` aws=True`, `Local=False`, `slow=False`
### 1. RUN_AWS=1 (default)
This runs 4 tests per dataset script.
a) Does the dataset script have a valid etag / Can it be reached on AWS?
b) Can we load its `builder_class`?
c) Can we load **all** dataset configs?
d) _Most importantly_: Can we load the dataset?
Important - we currently only test the first config of each dataset to reduce test time. Total test time is around 1min20s.
### 2. RUN_LOCAL=1 RUN_AWS=0
***This should be done when debugging dataset scripts of the ./datasets folder***
This only runs 1 test per dataset test, which is equivalent to aws d) - Can we load the dataset from the local `datasets` directory?
### 3. RUN_SLOW=1
We should set up to run these tests maybe 1 time per week ? @thomwolf
The `slow` tests include two more important tests.
e) Can we load the dataset with all possible configs? This test will probably fail at the moment because a lot of dummy data is missing. We should add the dummy data step by step to be sure that all configs work.
f) Test that the actual dataset can be loaded. This will take quite some time to run, but is important to make sure that the "real" data can be loaded. It will also test whether the dataset script has the correct checksums file which is currently not tested with `aws=True`. @lhoestq - is there an easy way to check cheaply whether the `dataset_info.json` is correct for each dataset script?
> > For each dataset, If there exist a `dataset_info.json`, then the command `nlp-cli test path/to/my/dataset --al_configs` is successful only if the `dataset_infos.json` is correct. The infos are correct if the size and checksums of the downloaded file are correct, and if the number of examples in each split are correct.
> > Note: the `test` command is supposed to test the script, that's why it runs the script even if the cached files already exist. Let me know if it's good to you.
>
> Does it have to download the whole data to check if the checksums are correct? I guess so no?
Yes it has to download them all (unless they were already downloaded in which case it just uses the cached downloaded files). |
https://github.com/huggingface/datasets/pull/106 | Add data dir test command | Nice - I think we can merge this. I will update the checksums for `wikihow` then as well | 18 | text: Add data dir test command
Nice - I think we can merge this. I will update the checksums for `wikihow` then as well |
|
https://github.com/huggingface/datasets/pull/103 | [Manual downloads] add logic proposal for manual downloads and add wikihow | > Wikihow is an example that needs to manually download two files as stated in: https://github.com/mahnazkoupaee/WikiHow-Dataset.
>
> The user can then store these files under a hard-coded name: `wikihowAll.csv` and `wikihowSep.csv` in this case in a directory of his choice, e.g. `~/wikihow/manual_dir`.
>
> The dataset can then be loaded via:
>
> ```python
> import nlp
> nlp.load_dataset("wikihow", data_dir="~/wikihow/manual_dir")
> ```
>
> I added/changed so that there are explicit error messages when using manually downloaded files.
wouldn't be nicer if we can have `manual_dir/wikihow`? | Wikihow is an example that needs to manually download two files as stated in: https://github.com/mahnazkoupaee/WikiHow-Dataset.
The user can then store these files under a hard-coded name: `wikihowAll.csv` and `wikihowSep.csv` in this case in a directory of his choice, e.g. `~/wikihow/manual_dir`.
The dataset can then be loaded via:
```python
import nlp
nlp.load_dataset("wikihow", data_dir="~/wikihow/manual_dir")
```
I added/changed so that there are explicit error messages when using manually downloaded files.
| 87 | text: [Manual downloads] add logic proposal for manual downloads and add wikihow
Wikihow is an example that needs to manually download two files as stated in: https://github.com/mahnazkoupaee/WikiHow-Dataset.
The user can then store these files under a hard-coded name: `wikihowAll.csv` and `wikihowSep.csv` in this case in a directory of his choice, e.g. `~/wikihow/manual_dir`.
The dataset can then be loaded via:
```python
import nlp
nlp.load_dataset("wikihow", data_dir="~/wikihow/manual_dir")
```
I added/changed so that there are explicit error messages when using manually downloaded files.
> Wikihow is an example that needs to manually download two files as stated in: https://github.com/mahnazkoupaee/WikiHow-Dataset.
>
> The user can then store these files under a hard-coded name: `wikihowAll.csv` and `wikihowSep.csv` in this case in a directory of his choice, e.g. `~/wikihow/manual_dir`.
>
> The dataset can then be loaded via:
>
> ```python
> import nlp
> nlp.load_dataset("wikihow", data_dir="~/wikihow/manual_dir")
> ```
>
> I added/changed so that there are explicit error messages when using manually downloaded files.
wouldn't be nicer if we can have `manual_dir/wikihow`? |
https://github.com/huggingface/datasets/pull/103 | [Manual downloads] add logic proposal for manual downloads and add wikihow | > > Wikihow is an example that needs to manually download two files as stated in: https://github.com/mahnazkoupaee/WikiHow-Dataset.
> > The user can then store these files under a hard-coded name: `wikihowAll.csv` and `wikihowSep.csv` in this case in a directory of his choice, e.g. `~/wikihow/manual_dir`.
> > The dataset can then be loaded via:
> > ```python
> > import nlp
> > nlp.load_dataset("wikihow", data_dir="~/wikihow/manual_dir")
> > ```
> >
> >
> > I added/changed so that there are explicit error messages when using manually downloaded files.
>
> wouldn't be nicer if we can have `manual_dir/wikihow`?
Sure, I mean the user can decide whatever he likes best :-) The path one puts in `data_dir` will be used as the path to the manual dir. `nlp.load_dataset("wikihow", data_dir="~/manual_dir/wikihow")` would work as well as any other path ;-) | Wikihow is an example that needs to manually download two files as stated in: https://github.com/mahnazkoupaee/WikiHow-Dataset.
The user can then store these files under a hard-coded name: `wikihowAll.csv` and `wikihowSep.csv` in this case in a directory of his choice, e.g. `~/wikihow/manual_dir`.
The dataset can then be loaded via:
```python
import nlp
nlp.load_dataset("wikihow", data_dir="~/wikihow/manual_dir")
```
I added/changed so that there are explicit error messages when using manually downloaded files.
| 136 | text: [Manual downloads] add logic proposal for manual downloads and add wikihow
Wikihow is an example that needs to manually download two files as stated in: https://github.com/mahnazkoupaee/WikiHow-Dataset.
The user can then store these files under a hard-coded name: `wikihowAll.csv` and `wikihowSep.csv` in this case in a directory of his choice, e.g. `~/wikihow/manual_dir`.
The dataset can then be loaded via:
```python
import nlp
nlp.load_dataset("wikihow", data_dir="~/wikihow/manual_dir")
```
I added/changed so that there are explicit error messages when using manually downloaded files.
> > Wikihow is an example that needs to manually download two files as stated in: https://github.com/mahnazkoupaee/WikiHow-Dataset.
> > The user can then store these files under a hard-coded name: `wikihowAll.csv` and `wikihowSep.csv` in this case in a directory of his choice, e.g. `~/wikihow/manual_dir`.
> > The dataset can then be loaded via:
> > ```python
> > import nlp
> > nlp.load_dataset("wikihow", data_dir="~/wikihow/manual_dir")
> > ```
> >
> >
> > I added/changed so that there are explicit error messages when using manually downloaded files.
>
> wouldn't be nicer if we can have `manual_dir/wikihow`?
Sure, I mean the user can decide whatever he likes best :-) The path one puts in `data_dir` will be used as the path to the manual dir. `nlp.load_dataset("wikihow", data_dir="~/manual_dir/wikihow")` would work as well as any other path ;-) |
https://github.com/huggingface/datasets/pull/102 | Run save infos | Haha that cornell dialogue dataset - that ran for 3h on my computer as well. The `generate_examples` method in this script is one of the most inefficient code samples I've ever seen :D | I replaced the old checksum file with the new `dataset_infos.json` by running the script on almost all the datasets we have. The only one that is still running on my side is the cornell dialog | 33 | text: Run save infos
I replaced the old checksum file with the new `dataset_infos.json` by running the script on almost all the datasets we have. The only one that is still running on my side is the cornell dialog
Haha that cornell dialogue dataset - that ran for 3h on my computer as well. The `generate_examples` method in this script is one of the most inefficient code samples I've ever seen :D |
https://github.com/huggingface/datasets/pull/100 | Add per type scores in seqeval metric | Can you put the kwargs as normal kwargs instead of a dict? (And add them to the kwargs description As well) | This PR add a bit more detail in the seqeval metric. Now the usage and output are:
```python
import nlp
met = nlp.load_metric('metrics/seqeval')
references = [['O', 'O', 'O', 'B-MISC', 'I-MISC', 'I-MISC', 'O'], ['B-PER', 'I-PER', 'O']]
predictions = [['O', 'O', 'B-MISC', 'I-MISC', 'I-MISC', 'I-MISC', 'O'], ['B-PER', 'I-PER', 'O']]
met.compute(predictions, references)
#Output: {'PER': {'precision': 1.0, 'recall': 1.0, 'f1': 1.0, 'number': 1}, 'MISC': {'precision': 0.0, 'recall': 0.0, 'f1': 0, 'number': 1}, 'overall_precision': 0.5, 'overall_recall': 0.5, 'overall_f1': 0.5, 'overall_accuracy': 0.8}
```
It is also possible to compute scores for non IOB notations, POS tagging for example hasn't this kind of notation. Add `suffix` parameter:
```python
import nlp
met = nlp.load_metric('metrics/seqeval')
references = [['O', 'O', 'O', 'MISC', 'MISC', 'MISC', 'O'], ['PER', 'PER', 'O']]
predictions = [['O', 'O', 'MISC', 'MISC', 'MISC', 'MISC', 'O'], ['PER', 'PER', 'O']]
met.compute(predictions, references, metrics_kwargs={"suffix": True})
#Output: {'PER': {'precision': 1.0, 'recall': 1.0, 'f1': 1.0, 'number': 1}, 'MISC': {'precision': 0.0, 'recall': 0.0, 'f1': 0, 'number': 1}, 'overall_precision': 0.5, 'overall_recall': 0.5, 'overall_f1': 0.5, 'overall_accuracy': 0.9}
``` | 21 | text: Add per type scores in seqeval metric
This PR add a bit more detail in the seqeval metric. Now the usage and output are:
```python
import nlp
met = nlp.load_metric('metrics/seqeval')
references = [['O', 'O', 'O', 'B-MISC', 'I-MISC', 'I-MISC', 'O'], ['B-PER', 'I-PER', 'O']]
predictions = [['O', 'O', 'B-MISC', 'I-MISC', 'I-MISC', 'I-MISC', 'O'], ['B-PER', 'I-PER', 'O']]
met.compute(predictions, references)
#Output: {'PER': {'precision': 1.0, 'recall': 1.0, 'f1': 1.0, 'number': 1}, 'MISC': {'precision': 0.0, 'recall': 0.0, 'f1': 0, 'number': 1}, 'overall_precision': 0.5, 'overall_recall': 0.5, 'overall_f1': 0.5, 'overall_accuracy': 0.8}
```
It is also possible to compute scores for non IOB notations, POS tagging for example hasn't this kind of notation. Add `suffix` parameter:
```python
import nlp
met = nlp.load_metric('metrics/seqeval')
references = [['O', 'O', 'O', 'MISC', 'MISC', 'MISC', 'O'], ['PER', 'PER', 'O']]
predictions = [['O', 'O', 'MISC', 'MISC', 'MISC', 'MISC', 'O'], ['PER', 'PER', 'O']]
met.compute(predictions, references, metrics_kwargs={"suffix": True})
#Output: {'PER': {'precision': 1.0, 'recall': 1.0, 'f1': 1.0, 'number': 1}, 'MISC': {'precision': 0.0, 'recall': 0.0, 'f1': 0, 'number': 1}, 'overall_precision': 0.5, 'overall_recall': 0.5, 'overall_f1': 0.5, 'overall_accuracy': 0.9}
```
Can you put the kwargs as normal kwargs instead of a dict? (And add them to the kwargs description As well) |
https://github.com/huggingface/datasets/pull/98 | Webis tl-dr | > Should that rather be in an organization scope, @thomwolf @patrickvonplaten ?
I'm a bit indifferent - both would be fine for me! | Add the Webid TL:DR dataset. | 23 | text: Webis tl-dr
Add the Webid TL:DR dataset.
> Should that rather be in an organization scope, @thomwolf @patrickvonplaten ?
I'm a bit indifferent - both would be fine for me! |
https://github.com/huggingface/datasets/pull/98 | Webis tl-dr | @jplu - if creating the dummy_data is too tedious, I can do it as well :-) | Add the Webid TL:DR dataset. | 16 | text: Webis tl-dr
Add the Webid TL:DR dataset.
@jplu - if creating the dummy_data is too tedious, I can do it as well :-) |
https://github.com/huggingface/datasets/pull/98 | Webis tl-dr | > There is dummy_data here, no ?
Some paths were wrong - the structure is really confusing and the error messages don't really help either - I have to think about how to make this easier to understand!
Hope it was ok that I fiddled with your PR ! | Add the Webid TL:DR dataset. | 49 | text: Webis tl-dr
Add the Webid TL:DR dataset.
> There is dummy_data here, no ?
Some paths were wrong - the structure is really confusing and the error messages don't really help either - I have to think about how to make this easier to understand!
Hope it was ok that I fiddled with your PR ! |
https://github.com/huggingface/datasets/pull/98 | Webis tl-dr | > Some paths were wrong - the structure is really confusing and the error message don't really help either - I have to think about how to make this easier to understand!
Oh ok! I haven't noticed that sorry :(
> Hope it was ok that I fiddled with your PR !
Of course it was ok :) | Add the Webid TL:DR dataset. | 58 | text: Webis tl-dr
Add the Webid TL:DR dataset.
> Some paths were wrong - the structure is really confusing and the error message don't really help either - I have to think about how to make this easier to understand!
Oh ok! I haven't noticed that sorry :(
> Hope it was ok that I fiddled with your PR !
Of course it was ok :) |
https://github.com/huggingface/datasets/pull/98 | Webis tl-dr | @julien-c Looks like what you have in mind?
```python
import nlp
nlp.load_dataset("datasets/webis", "tl_dr")
#Output: Downloading and preparing dataset webis/tl_dr (download: Unknown size, generated: Unknown size, total: Unknown size) to /home/jplu/.cache/huggingface/datasets/webis/tl_dr/1.0.0...
``` | Add the Webid TL:DR dataset. | 31 | text: Webis tl-dr
Add the Webid TL:DR dataset.
@julien-c Looks like what you have in mind?
```python
import nlp
nlp.load_dataset("datasets/webis", "tl_dr")
#Output: Downloading and preparing dataset webis/tl_dr (download: Unknown size, generated: Unknown size, total: Unknown size) to /home/jplu/.cache/huggingface/datasets/webis/tl_dr/1.0.0...
``` |
https://github.com/huggingface/datasets/pull/98 | Webis tl-dr | Merging this for now. Maybe we can see whether to rename it in a different PR @julien-c ?
| Add the Webid TL:DR dataset. | 18 | text: Webis tl-dr
Add the Webid TL:DR dataset.
Merging this for now. Maybe we can see whether to rename it in a different PR @julien-c ?
|
https://github.com/huggingface/datasets/pull/98 | Webis tl-dr | Hi,
Author here of the webis-tldr corpus. Any plans on integrating this dataset into the hub? I remember we could access it in the previous versions of the library. If there is a particular issue that I can help with, do let me know.
Thanks! | Add the Webid TL:DR dataset. | 45 | text: Webis tl-dr
Add the Webid TL:DR dataset.
Hi,
Author here of the webis-tldr corpus. Any plans on integrating this dataset into the hub? I remember we could access it in the previous versions of the library. If there is a particular issue that I can help with, do let me know.
Thanks! |
https://github.com/huggingface/datasets/pull/98 | Webis tl-dr | Hi @shahbazsyed, this dataset _is_ inside the hub but it's namespaced by the organization name `webis`.
You can load it following the steps described in https://huggingface.co/datasets/webis/tl_dr
Here's a Colab showcasing that it works: https://colab.research.google.com/drive/11IrzRVpnMLJZ8_UFFHLR8FhiajjAHRUU?usp=sharing
The reason the code is in S3 and not in this repo is that the dataset is namespaced under the `webis` organization. We don't have a lot of namespaced datasets yet but this should become the main way we add more datasets in the future.
Let us know if that's an issue for you. Thank you! | Add the Webid TL:DR dataset. | 90 | text: Webis tl-dr
Add the Webid TL:DR dataset.
Hi @shahbazsyed, this dataset _is_ inside the hub but it's namespaced by the organization name `webis`.
You can load it following the steps described in https://huggingface.co/datasets/webis/tl_dr
Here's a Colab showcasing that it works: https://colab.research.google.com/drive/11IrzRVpnMLJZ8_UFFHLR8FhiajjAHRUU?usp=sharing
The reason the code is in S3 and not in this repo is that the dataset is namespaced under the `webis` organization. We don't have a lot of namespaced datasets yet but this should become the main way we add more datasets in the future.
Let us know if that's an issue for you. Thank you! |
https://github.com/huggingface/datasets/pull/96 | lm1b | I might have a different version of `isort` than others. It seems like I'm always reordering the imports of others. But isn't really a problem... | Add lm1b dataset. | 25 | text: lm1b
Add lm1b dataset.
I might have a different version of `isort` than others. It seems like I'm always reordering the imports of others. But isn't really a problem... |
https://github.com/huggingface/datasets/pull/95 | Replace checksums files by Dataset infos json | > Ok, really clean!
> I like the logic (not a huge fan of using `_asdict_inner` but it makes sense).
> I think it's a nice improvement!
>
> How should we update the files in the repo? Run a big job on a server or on somebody's computer who has most of the datasets already downloaded?
Maybe we can split the updates among us...IMO most datasets run very quickly.
I think I've downloaded 50 datasets and 80% are loaded in <5min, 15% in <1h and then `wmt` which is still downloading (since 12h).
I deleted my cache because the `wmt` downloads require quite a lot of space, so I only have parts of the `wmt` datasets on my computer.
@mariamabarham I guess you have downloaded most of the datasets no? | ### Better verifications when loading a dataset
I replaced the `urls_checksums` directory that used to contain `checksums.txt` and `cached_sizes.txt`, by a single file `dataset_infos.json`. It's just a dict `config_name` -> `DatasetInfo`.
It simplifies and improves how verifications of checksums and splits sizes are done, as they're all stored in `DatasetInfo` (one per config). Also, having already access to `DatasetInfo` enables to check disk space before running `download_and_prepare` for a given config.
The dataset infos json file is user readable, you can take a look at the squad one that I generated in this PR.
### Renaming
According to these changes, I did some renaming:
`save_checksums` -> `save_infos`
`ignore_checksums` -> `ignore_verifications`
for example, when you are creating a dataset you have to run
```nlp-cli test path/to/my/dataset --save_infos --all_configs```
instead of
```nlp-cli test path/to/my/dataset --save_checksums --all_configs```
### And now, the fun part
We'll have to rerun the `nlp-cli test ... --save_infos --all_configs` for all the datasets
-----------------
feedback appreciated ! | 131 | text: Replace checksums files by Dataset infos json
### Better verifications when loading a dataset
I replaced the `urls_checksums` directory that used to contain `checksums.txt` and `cached_sizes.txt`, by a single file `dataset_infos.json`. It's just a dict `config_name` -> `DatasetInfo`.
It simplifies and improves how verifications of checksums and splits sizes are done, as they're all stored in `DatasetInfo` (one per config). Also, having already access to `DatasetInfo` enables to check disk space before running `download_and_prepare` for a given config.
The dataset infos json file is user readable, you can take a look at the squad one that I generated in this PR.
### Renaming
According to these changes, I did some renaming:
`save_checksums` -> `save_infos`
`ignore_checksums` -> `ignore_verifications`
for example, when you are creating a dataset you have to run
```nlp-cli test path/to/my/dataset --save_infos --all_configs```
instead of
```nlp-cli test path/to/my/dataset --save_checksums --all_configs```
### And now, the fun part
We'll have to rerun the `nlp-cli test ... --save_infos --all_configs` for all the datasets
-----------------
feedback appreciated !
> Ok, really clean!
> I like the logic (not a huge fan of using `_asdict_inner` but it makes sense).
> I think it's a nice improvement!
>
> How should we update the files in the repo? Run a big job on a server or on somebody's computer who has most of the datasets already downloaded?
Maybe we can split the updates among us...IMO most datasets run very quickly.
I think I've downloaded 50 datasets and 80% are loaded in <5min, 15% in <1h and then `wmt` which is still downloading (since 12h).
I deleted my cache because the `wmt` downloads require quite a lot of space, so I only have parts of the `wmt` datasets on my computer.
@mariamabarham I guess you have downloaded most of the datasets no? |
https://github.com/huggingface/datasets/pull/94 | Librispeech | @jplu - I changed this weird archieve - iter method to something simpler. It's only one file to download anyways so I don't see the point of using weird iter methods...It's a huge file though :D 30 million lines of text. Took me quite some time to download :D | Add librispeech dataset and remove some useless content. | 49 | text: Librispeech
Add librispeech dataset and remove some useless content.
@jplu - I changed this weird archieve - iter method to something simpler. It's only one file to download anyways so I don't see the point of using weird iter methods...It's a huge file though :D 30 million lines of text. Took me quite some time to download :D |
https://github.com/huggingface/datasets/pull/88 | Add wiki40b | Looks good to me. I have not really looked too much into the Beam Datasets yet though - so I think you can merge whenever you think is good for Beam datasets :-) | This one is a beam dataset that downloads files using tensorflow.
I tested it on a small config and it works fine | 33 | text: Add wiki40b
This one is a beam dataset that downloads files using tensorflow.
I tested it on a small config and it works fine
Looks good to me. I have not really looked too much into the Beam Datasets yet though - so I think you can merge whenever you think is good for Beam datasets :-) |
https://github.com/huggingface/datasets/pull/80 | Add nbytes + nexamples check | Looks good to me! Should we hard code those numbers in the config classes and make sure that when loading a dataset that the numbers match? | ### Save size and number of examples
Now when you do `save_checksums`, it also create `cached_sizes.txt` right next to the checksum file.
This new file stores the bytes sizes and the number of examples of each split that has been prepared and stored in the cache. Example:
```
# Cached sizes: <full_config_name> <num_bytes> <num_examples>
hansards/house/1.0.0/test 22906629 122290
hansards/house/1.0.0/train 191459584 947969
hansards/senate/1.0.0/test 5711686 25553
hansards/senate/1.0.0/train 40324278 182135
```
### Check processing output
If there is a `caches_sizes.txt`, then each time we run `download_and_prepare` it will make sure that the sizes match. You can set `ignore_checksums=True` if you don't want that to happen.
### Fill Dataset Info
All the split infos and the checksums are now stored correctly in DatasetInfo after `download_and_prepare`
### Check space on disk before running `download_and_prepare`
Check if the space is lower than the sum of the sizes of the files in `checksums.txt` and `cached_files.txt`. This is not ideal though as it considers the files for all configs.
TODO:
A better way to do it would be to have save the `DatasetInfo` instead of the `checksums.txt` and `cached_sizes.txt`, in order to have one file per dataset config (and therefore consider only the sizes of the files for one config and not all of them). It can also be the occasion to factorize all the `download_and_prepare` verifications. Maybe next PR ? | 26 | text: Add nbytes + nexamples check
### Save size and number of examples
Now when you do `save_checksums`, it also create `cached_sizes.txt` right next to the checksum file.
This new file stores the bytes sizes and the number of examples of each split that has been prepared and stored in the cache. Example:
```
# Cached sizes: <full_config_name> <num_bytes> <num_examples>
hansards/house/1.0.0/test 22906629 122290
hansards/house/1.0.0/train 191459584 947969
hansards/senate/1.0.0/test 5711686 25553
hansards/senate/1.0.0/train 40324278 182135
```
### Check processing output
If there is a `caches_sizes.txt`, then each time we run `download_and_prepare` it will make sure that the sizes match. You can set `ignore_checksums=True` if you don't want that to happen.
### Fill Dataset Info
All the split infos and the checksums are now stored correctly in DatasetInfo after `download_and_prepare`
### Check space on disk before running `download_and_prepare`
Check if the space is lower than the sum of the sizes of the files in `checksums.txt` and `cached_files.txt`. This is not ideal though as it considers the files for all configs.
TODO:
A better way to do it would be to have save the `DatasetInfo` instead of the `checksums.txt` and `cached_sizes.txt`, in order to have one file per dataset config (and therefore consider only the sizes of the files for one config and not all of them). It can also be the occasion to factorize all the `download_and_prepare` verifications. Maybe next PR ?
Looks good to me! Should we hard code those numbers in the config classes and make sure that when loading a dataset that the numbers match? |
https://github.com/huggingface/datasets/pull/75 | WIP adding metrics | It's all about my metric stuff so I'll probably merge it unless you want to have a look.
Took the occasion to remove the old doc and requirements.txt | Adding the following metrics as identified by @mariamabarham:
1. BLEU: BiLingual Evaluation Understudy: https://github.com/tensorflow/nmt/blob/master/nmt/scripts/bleu.py, https://github.com/chakki-works/sumeval/blob/master/sumeval/metrics/bleu.py (multilingual)
2. GLEU: Google-BLEU: https://github.com/cnap/gec-ranking/blob/master/scripts/compute_gleu
3. Sacrebleu: https://pypi.org/project/sacrebleu/1.4.8/ (pypi package), https://github.com/mjpost/sacrebleu (github implementation)
4. ROUGE: Recall-Oriented Understudy for Gisting Evaluation: https://github.com/google-research/google-research/tree/master/rouge, https://github.com/chakki-works/sumeval/blob/master/sumeval/metrics/rouge.py (multilingual)
5. Seqeval: https://github.com/chakki-works/seqeval (github implementation), https://pypi.org/project/seqeval/0.0.12/ (pypi package)
6. Coval: coreference evaluation package for the CoNLL and ARRAU datasets https://github.com/ns-moosavi/coval
7. SQuAD v1 evaluation script
8. SQuAD V2 evaluation script: https://worksheets.codalab.org/rest/bundles/0x6b567e1cf2e041ec80d7098f031c5c9e/contents/blob/
9. GLUE
10. XNLI
Not now:
1. Perplexity: https://github.com/allenai/allennlp/blob/master/allennlp/training/metrics/perplexity.py
2. Spearman: https://github.com/allenai/allennlp/blob/master/allennlp/training/metrics/spearman_correlation.py
3. F1_measure: https://github.com/allenai/allennlp/blob/master/allennlp/training/metrics/f1_measure.py
4. Pearson_corelation: https://github.com/allenai/allennlp/blob/master/allennlp/training/metrics/pearson_correlation.py
5. AUC: https://github.com/allenai/allennlp/blob/master/allennlp/training/metrics/auc.py
6. Entropy: https://github.com/allenai/allennlp/blob/master/allennlp/training/metrics/entropy.py | 28 | text: WIP adding metrics
Adding the following metrics as identified by @mariamabarham:
1. BLEU: BiLingual Evaluation Understudy: https://github.com/tensorflow/nmt/blob/master/nmt/scripts/bleu.py, https://github.com/chakki-works/sumeval/blob/master/sumeval/metrics/bleu.py (multilingual)
2. GLEU: Google-BLEU: https://github.com/cnap/gec-ranking/blob/master/scripts/compute_gleu
3. Sacrebleu: https://pypi.org/project/sacrebleu/1.4.8/ (pypi package), https://github.com/mjpost/sacrebleu (github implementation)
4. ROUGE: Recall-Oriented Understudy for Gisting Evaluation: https://github.com/google-research/google-research/tree/master/rouge, https://github.com/chakki-works/sumeval/blob/master/sumeval/metrics/rouge.py (multilingual)
5. Seqeval: https://github.com/chakki-works/seqeval (github implementation), https://pypi.org/project/seqeval/0.0.12/ (pypi package)
6. Coval: coreference evaluation package for the CoNLL and ARRAU datasets https://github.com/ns-moosavi/coval
7. SQuAD v1 evaluation script
8. SQuAD V2 evaluation script: https://worksheets.codalab.org/rest/bundles/0x6b567e1cf2e041ec80d7098f031c5c9e/contents/blob/
9. GLUE
10. XNLI
Not now:
1. Perplexity: https://github.com/allenai/allennlp/blob/master/allennlp/training/metrics/perplexity.py
2. Spearman: https://github.com/allenai/allennlp/blob/master/allennlp/training/metrics/spearman_correlation.py
3. F1_measure: https://github.com/allenai/allennlp/blob/master/allennlp/training/metrics/f1_measure.py
4. Pearson_corelation: https://github.com/allenai/allennlp/blob/master/allennlp/training/metrics/pearson_correlation.py
5. AUC: https://github.com/allenai/allennlp/blob/master/allennlp/training/metrics/auc.py
6. Entropy: https://github.com/allenai/allennlp/blob/master/allennlp/training/metrics/entropy.py
It's all about my metric stuff so I'll probably merge it unless you want to have a look.
Took the occasion to remove the old doc and requirements.txt |
https://github.com/huggingface/datasets/pull/73 | JSON script | The tests for the Wikipedia dataset do not pass anymore with the error:
```
To be able to use this dataset, you need to install the following dependencies ['mwparserfromhell'] using 'pip install mwparserfromhell' for instance'
``` | Add a JSONS script to read JSON datasets from files. | 36 | text: JSON script
Add a JSONS script to read JSON datasets from files.
The tests for the Wikipedia dataset do not pass anymore with the error:
```
To be able to use this dataset, you need to install the following dependencies ['mwparserfromhell'] using 'pip install mwparserfromhell' for instance'
``` |
https://github.com/huggingface/datasets/pull/73 | JSON script | Currently the dummy_data tests are always green because in a PR the dataset is not yet synchronized with aws. This PR fixes this: https://github.com/huggingface/nlp/pull/140 .
Could you test `json` locally or wait until the PR: https://github.com/huggingface/nlp/pull/140 is merged ? :-) | Add a JSONS script to read JSON datasets from files. | 40 | text: JSON script
Add a JSONS script to read JSON datasets from files.
Currently the dummy_data tests are always green because in a PR the dataset is not yet synchronized with aws. This PR fixes this: https://github.com/huggingface/nlp/pull/140 .
Could you test `json` locally or wait until the PR: https://github.com/huggingface/nlp/pull/140 is merged ? :-) |
https://github.com/huggingface/datasets/pull/71 | Fix arrow writer for big datasets using writer_batch_size | After a quick chat with Yacine : the 2Go test may not be sufficient actually, as I'm looking at the size of the array and not the size of the current_rows. If the test doesn't do the job I think I'll remove it and lower the batch size a bit to be sure that it never exceeds 2Go. I'll do more tests later | This PR fixes Yacine's bug.
According to [this](https://github.com/apache/arrow/blob/master/docs/source/cpp/arrays.rst#size-limitations-and-recommendations), it is not recommended to have pyarrow arrays bigger than 2Go.
Therefore I set a default batch size of 100 000 examples per batch. In general it shouldn't exceed 2Go. If it does, I reduce the batch_size on the fly, and I notify the user with a warning. | 63 | text: Fix arrow writer for big datasets using writer_batch_size
This PR fixes Yacine's bug.
According to [this](https://github.com/apache/arrow/blob/master/docs/source/cpp/arrays.rst#size-limitations-and-recommendations), it is not recommended to have pyarrow arrays bigger than 2Go.
Therefore I set a default batch size of 100 000 examples per batch. In general it shouldn't exceed 2Go. If it does, I reduce the batch_size on the fly, and I notify the user with a warning.
After a quick chat with Yacine : the 2Go test may not be sufficient actually, as I'm looking at the size of the array and not the size of the current_rows. If the test doesn't do the job I think I'll remove it and lower the batch size a bit to be sure that it never exceeds 2Go. I'll do more tests later |
https://github.com/huggingface/datasets/pull/70 | adding RACE, QASC, Super_glue and Tiny_shakespear datasets | I think rebasing to master will solve the quality test and the datasets that don't have a testing structure yet because of the manual download - maybe you can put them in `datasets under construction`? Then would also make it easier for me to see how to add tests for them :-) | 52 | text: adding RACE, QASC, Super_glue and Tiny_shakespear datasets
I think rebasing to master will solve the quality test and the datasets that don't have a testing structure yet because of the manual download - maybe you can put them in `datasets under construction`? Then would also make it easier for me to see how to add tests for them :-) |
|
https://github.com/huggingface/datasets/pull/60 | Update to simplify some datasets conversion | Also we should convert `tf.io.gfile.exists` into `os.path.exists` , `tf.io.gfile.listdir`into `os.listdir` and `tf.io.gfile.glob` into `glob.glob` (will need to add `import glob`) | This PR updates the encoding of `Values` like `integers`, `boolean` and `float` to use python casting and avoid having to cast in the dataset scripts, as mentioned here: https://github.com/huggingface/nlp/pull/37#discussion_r420176626
We could also change (not included in this PR yet):
- `supervized_keys` to make them a NamedTuple instead of a dataclass, and
- handle specifically the `Translation` features.
as mentioned here: https://github.com/huggingface/nlp/pull/37#discussion_r421740236
@patrickvonplaten @mariamabarham tell me if you want these two last changes as well. | 20 | text: Update to simplify some datasets conversion
This PR updates the encoding of `Values` like `integers`, `boolean` and `float` to use python casting and avoid having to cast in the dataset scripts, as mentioned here: https://github.com/huggingface/nlp/pull/37#discussion_r420176626
We could also change (not included in this PR yet):
- `supervized_keys` to make them a NamedTuple instead of a dataclass, and
- handle specifically the `Translation` features.
as mentioned here: https://github.com/huggingface/nlp/pull/37#discussion_r421740236
@patrickvonplaten @mariamabarham tell me if you want these two last changes as well.
Also we should convert `tf.io.gfile.exists` into `os.path.exists` , `tf.io.gfile.listdir`into `os.listdir` and `tf.io.gfile.glob` into `glob.glob` (will need to add `import glob`) |
https://github.com/huggingface/datasets/pull/60 | Update to simplify some datasets conversion | > Also we should convert `tf.io.gfile.exists` into `os.path.exists` , `tf.io.gfile.listdir`into `os.listdir` and `tf.io.gfile.glob` into `glob.glob` (will need to add `import glob`)
We should probably open a new PR about this | This PR updates the encoding of `Values` like `integers`, `boolean` and `float` to use python casting and avoid having to cast in the dataset scripts, as mentioned here: https://github.com/huggingface/nlp/pull/37#discussion_r420176626
We could also change (not included in this PR yet):
- `supervized_keys` to make them a NamedTuple instead of a dataclass, and
- handle specifically the `Translation` features.
as mentioned here: https://github.com/huggingface/nlp/pull/37#discussion_r421740236
@patrickvonplaten @mariamabarham tell me if you want these two last changes as well. | 30 | text: Update to simplify some datasets conversion
This PR updates the encoding of `Values` like `integers`, `boolean` and `float` to use python casting and avoid having to cast in the dataset scripts, as mentioned here: https://github.com/huggingface/nlp/pull/37#discussion_r420176626
We could also change (not included in this PR yet):
- `supervized_keys` to make them a NamedTuple instead of a dataclass, and
- handle specifically the `Translation` features.
as mentioned here: https://github.com/huggingface/nlp/pull/37#discussion_r421740236
@patrickvonplaten @mariamabarham tell me if you want these two last changes as well.
> Also we should convert `tf.io.gfile.exists` into `os.path.exists` , `tf.io.gfile.listdir`into `os.listdir` and `tf.io.gfile.glob` into `glob.glob` (will need to add `import glob`)
We should probably open a new PR about this |
https://github.com/huggingface/datasets/pull/60 | Update to simplify some datasets conversion | I think it might be a good idea to both change the supervised keys to a named tuple and also handle the translation features specifically. | This PR updates the encoding of `Values` like `integers`, `boolean` and `float` to use python casting and avoid having to cast in the dataset scripts, as mentioned here: https://github.com/huggingface/nlp/pull/37#discussion_r420176626
We could also change (not included in this PR yet):
- `supervized_keys` to make them a NamedTuple instead of a dataclass, and
- handle specifically the `Translation` features.
as mentioned here: https://github.com/huggingface/nlp/pull/37#discussion_r421740236
@patrickvonplaten @mariamabarham tell me if you want these two last changes as well. | 25 | text: Update to simplify some datasets conversion
This PR updates the encoding of `Values` like `integers`, `boolean` and `float` to use python casting and avoid having to cast in the dataset scripts, as mentioned here: https://github.com/huggingface/nlp/pull/37#discussion_r420176626
We could also change (not included in this PR yet):
- `supervized_keys` to make them a NamedTuple instead of a dataclass, and
- handle specifically the `Translation` features.
as mentioned here: https://github.com/huggingface/nlp/pull/37#discussion_r421740236
@patrickvonplaten @mariamabarham tell me if you want these two last changes as well.
I think it might be a good idea to both change the supervised keys to a named tuple and also handle the translation features specifically. |
https://github.com/huggingface/datasets/pull/60 | Update to simplify some datasets conversion | Just noticed that `pyarrow` apparently does not have a `is_boolean` function. Or do I have the wrong `pyarrow` version? | This PR updates the encoding of `Values` like `integers`, `boolean` and `float` to use python casting and avoid having to cast in the dataset scripts, as mentioned here: https://github.com/huggingface/nlp/pull/37#discussion_r420176626
We could also change (not included in this PR yet):
- `supervized_keys` to make them a NamedTuple instead of a dataclass, and
- handle specifically the `Translation` features.
as mentioned here: https://github.com/huggingface/nlp/pull/37#discussion_r421740236
@patrickvonplaten @mariamabarham tell me if you want these two last changes as well. | 19 | text: Update to simplify some datasets conversion
This PR updates the encoding of `Values` like `integers`, `boolean` and `float` to use python casting and avoid having to cast in the dataset scripts, as mentioned here: https://github.com/huggingface/nlp/pull/37#discussion_r420176626
We could also change (not included in this PR yet):
- `supervized_keys` to make them a NamedTuple instead of a dataclass, and
- handle specifically the `Translation` features.
as mentioned here: https://github.com/huggingface/nlp/pull/37#discussion_r421740236
@patrickvonplaten @mariamabarham tell me if you want these two last changes as well.
Just noticed that `pyarrow` apparently does not have a `is_boolean` function. Or do I have the wrong `pyarrow` version? |
https://github.com/huggingface/datasets/pull/59 | Fix tests | Very weird bug indeed! I think the problem was that when importing `setup_module` we overwrote `pytest's` setup_module function. I think this is the relevant code in pytest: https://github.com/pytest-dev/pytest/blob/9d2eabb397b059b75b746259daeb20ee5588f559/src/_pytest/python.py#L460. | @patrickvonplaten I've broken a bit the tests with #25 while simplifying and re-organizing the `load.py` and `download_manager.py` scripts.
I'm trying to fix them here but I have a weird error, do you think you can have a look?
```bash
(datasets) MacBook-Pro-de-Thomas:datasets thomwolf$ python -m pytest -sv ./tests/test_dataset_common.py::DatasetTest::test_builder_class_snli
============================================================================= test session starts =============================================================================
platform darwin -- Python 3.7.7, pytest-5.4.1, py-1.8.1, pluggy-0.13.1 -- /Users/thomwolf/miniconda2/envs/datasets/bin/python
cachedir: .pytest_cache
rootdir: /Users/thomwolf/Documents/GitHub/datasets
plugins: xdist-1.31.0, forked-1.1.3
collected 1 item
tests/test_dataset_common.py::DatasetTest::test_builder_class_snli ERROR
=================================================================================== ERRORS ====================================================================================
____________________________________________________________ ERROR at setup of DatasetTest.test_builder_class_snli ____________________________________________________________
file_path = <module 'tests.test_dataset_common' from '/Users/thomwolf/Documents/GitHub/datasets/tests/test_dataset_common.py'>
download_config = DownloadConfig(cache_dir=None, force_download=False, resume_download=False, local_files_only=False, proxies=None, user_agent=None, extract_compressed_file=True, force_extract=True)
download_kwargs = {}
def setup_module(file_path: str, download_config: Optional[DownloadConfig] = None, **download_kwargs,) -> DatasetBuilder:
r"""
Download/extract/cache a dataset to add to the lib from a path or url which can be:
- a path to a local directory containing the dataset processing python script
- an url to a S3 directory with a dataset processing python script
Dataset codes are cached inside the lib to allow easy import (avoid ugly sys.path tweaks)
and using cloudpickle (among other things).
Return: tuple of
the unique id associated to the dataset
the local path to the dataset
"""
if download_config is None:
download_config = DownloadConfig(**download_kwargs)
download_config.extract_compressed_file = True
download_config.force_extract = True
> name = list(filter(lambda x: x, file_path.split("/")))[-1] + ".py"
E AttributeError: module 'tests.test_dataset_common' has no attribute 'split'
src/nlp/load.py:169: AttributeError
============================================================================== warnings summary ===============================================================================
/Users/thomwolf/miniconda2/envs/datasets/lib/python3.7/site-packages/tensorflow_core/python/pywrap_tensorflow_internal.py:15
/Users/thomwolf/miniconda2/envs/datasets/lib/python3.7/site-packages/tensorflow_core/python/pywrap_tensorflow_internal.py:15: DeprecationWarning: the imp module is deprecated in favour of importlib; see the module's documentation for alternative uses
import imp
-- Docs: https://docs.pytest.org/en/latest/warnings.html
=========================================================================== short test summary info ===========================================================================
ERROR tests/test_dataset_common.py::DatasetTest::test_builder_class_snli - AttributeError: module 'tests.test_dataset_common' has no attribute 'split'
========================================================================= 1 warning, 1 error in 3.63s =========================================================================
```
| 28 | text: Fix tests
@patrickvonplaten I've broken a bit the tests with #25 while simplifying and re-organizing the `load.py` and `download_manager.py` scripts.
I'm trying to fix them here but I have a weird error, do you think you can have a look?
```bash
(datasets) MacBook-Pro-de-Thomas:datasets thomwolf$ python -m pytest -sv ./tests/test_dataset_common.py::DatasetTest::test_builder_class_snli
============================================================================= test session starts =============================================================================
platform darwin -- Python 3.7.7, pytest-5.4.1, py-1.8.1, pluggy-0.13.1 -- /Users/thomwolf/miniconda2/envs/datasets/bin/python
cachedir: .pytest_cache
rootdir: /Users/thomwolf/Documents/GitHub/datasets
plugins: xdist-1.31.0, forked-1.1.3
collected 1 item
tests/test_dataset_common.py::DatasetTest::test_builder_class_snli ERROR
=================================================================================== ERRORS ====================================================================================
____________________________________________________________ ERROR at setup of DatasetTest.test_builder_class_snli ____________________________________________________________
file_path = <module 'tests.test_dataset_common' from '/Users/thomwolf/Documents/GitHub/datasets/tests/test_dataset_common.py'>
download_config = DownloadConfig(cache_dir=None, force_download=False, resume_download=False, local_files_only=False, proxies=None, user_agent=None, extract_compressed_file=True, force_extract=True)
download_kwargs = {}
def setup_module(file_path: str, download_config: Optional[DownloadConfig] = None, **download_kwargs,) -> DatasetBuilder:
r"""
Download/extract/cache a dataset to add to the lib from a path or url which can be:
- a path to a local directory containing the dataset processing python script
- an url to a S3 directory with a dataset processing python script
Dataset codes are cached inside the lib to allow easy import (avoid ugly sys.path tweaks)
and using cloudpickle (among other things).
Return: tuple of
the unique id associated to the dataset
the local path to the dataset
"""
if download_config is None:
download_config = DownloadConfig(**download_kwargs)
download_config.extract_compressed_file = True
download_config.force_extract = True
> name = list(filter(lambda x: x, file_path.split("/")))[-1] + ".py"
E AttributeError: module 'tests.test_dataset_common' has no attribute 'split'
src/nlp/load.py:169: AttributeError
============================================================================== warnings summary ===============================================================================
/Users/thomwolf/miniconda2/envs/datasets/lib/python3.7/site-packages/tensorflow_core/python/pywrap_tensorflow_internal.py:15
/Users/thomwolf/miniconda2/envs/datasets/lib/python3.7/site-packages/tensorflow_core/python/pywrap_tensorflow_internal.py:15: DeprecationWarning: the imp module is deprecated in favour of importlib; see the module's documentation for alternative uses
import imp
-- Docs: https://docs.pytest.org/en/latest/warnings.html
=========================================================================== short test summary info ===========================================================================
ERROR tests/test_dataset_common.py::DatasetTest::test_builder_class_snli - AttributeError: module 'tests.test_dataset_common' has no attribute 'split'
========================================================================= 1 warning, 1 error in 3.63s =========================================================================
```
Very weird bug indeed! I think the problem was that when importing `setup_module` we overwrote `pytest's` setup_module function. I think this is the relevant code in pytest: https://github.com/pytest-dev/pytest/blob/9d2eabb397b059b75b746259daeb20ee5588f559/src/_pytest/python.py#L460. |
https://github.com/huggingface/datasets/pull/59 | Fix tests | Also PR: #25 introduced some renaming: `DatasetBuilder.builder_config` -> `DatasetBuilder.config` so that we will have to change most of the dataset scripts (Just replace the "builder_config" with "config").
I think the renaming is a good idea and I can do the fix with a bash regex, but will have to re-upload most of the datasets. @thomwolf @mariamabarham
| @patrickvonplaten I've broken a bit the tests with #25 while simplifying and re-organizing the `load.py` and `download_manager.py` scripts.
I'm trying to fix them here but I have a weird error, do you think you can have a look?
```bash
(datasets) MacBook-Pro-de-Thomas:datasets thomwolf$ python -m pytest -sv ./tests/test_dataset_common.py::DatasetTest::test_builder_class_snli
============================================================================= test session starts =============================================================================
platform darwin -- Python 3.7.7, pytest-5.4.1, py-1.8.1, pluggy-0.13.1 -- /Users/thomwolf/miniconda2/envs/datasets/bin/python
cachedir: .pytest_cache
rootdir: /Users/thomwolf/Documents/GitHub/datasets
plugins: xdist-1.31.0, forked-1.1.3
collected 1 item
tests/test_dataset_common.py::DatasetTest::test_builder_class_snli ERROR
=================================================================================== ERRORS ====================================================================================
____________________________________________________________ ERROR at setup of DatasetTest.test_builder_class_snli ____________________________________________________________
file_path = <module 'tests.test_dataset_common' from '/Users/thomwolf/Documents/GitHub/datasets/tests/test_dataset_common.py'>
download_config = DownloadConfig(cache_dir=None, force_download=False, resume_download=False, local_files_only=False, proxies=None, user_agent=None, extract_compressed_file=True, force_extract=True)
download_kwargs = {}
def setup_module(file_path: str, download_config: Optional[DownloadConfig] = None, **download_kwargs,) -> DatasetBuilder:
r"""
Download/extract/cache a dataset to add to the lib from a path or url which can be:
- a path to a local directory containing the dataset processing python script
- an url to a S3 directory with a dataset processing python script
Dataset codes are cached inside the lib to allow easy import (avoid ugly sys.path tweaks)
and using cloudpickle (among other things).
Return: tuple of
the unique id associated to the dataset
the local path to the dataset
"""
if download_config is None:
download_config = DownloadConfig(**download_kwargs)
download_config.extract_compressed_file = True
download_config.force_extract = True
> name = list(filter(lambda x: x, file_path.split("/")))[-1] + ".py"
E AttributeError: module 'tests.test_dataset_common' has no attribute 'split'
src/nlp/load.py:169: AttributeError
============================================================================== warnings summary ===============================================================================
/Users/thomwolf/miniconda2/envs/datasets/lib/python3.7/site-packages/tensorflow_core/python/pywrap_tensorflow_internal.py:15
/Users/thomwolf/miniconda2/envs/datasets/lib/python3.7/site-packages/tensorflow_core/python/pywrap_tensorflow_internal.py:15: DeprecationWarning: the imp module is deprecated in favour of importlib; see the module's documentation for alternative uses
import imp
-- Docs: https://docs.pytest.org/en/latest/warnings.html
=========================================================================== short test summary info ===========================================================================
ERROR tests/test_dataset_common.py::DatasetTest::test_builder_class_snli - AttributeError: module 'tests.test_dataset_common' has no attribute 'split'
========================================================================= 1 warning, 1 error in 3.63s =========================================================================
```
| 56 | text: Fix tests
@patrickvonplaten I've broken a bit the tests with #25 while simplifying and re-organizing the `load.py` and `download_manager.py` scripts.
I'm trying to fix them here but I have a weird error, do you think you can have a look?
```bash
(datasets) MacBook-Pro-de-Thomas:datasets thomwolf$ python -m pytest -sv ./tests/test_dataset_common.py::DatasetTest::test_builder_class_snli
============================================================================= test session starts =============================================================================
platform darwin -- Python 3.7.7, pytest-5.4.1, py-1.8.1, pluggy-0.13.1 -- /Users/thomwolf/miniconda2/envs/datasets/bin/python
cachedir: .pytest_cache
rootdir: /Users/thomwolf/Documents/GitHub/datasets
plugins: xdist-1.31.0, forked-1.1.3
collected 1 item
tests/test_dataset_common.py::DatasetTest::test_builder_class_snli ERROR
=================================================================================== ERRORS ====================================================================================
____________________________________________________________ ERROR at setup of DatasetTest.test_builder_class_snli ____________________________________________________________
file_path = <module 'tests.test_dataset_common' from '/Users/thomwolf/Documents/GitHub/datasets/tests/test_dataset_common.py'>
download_config = DownloadConfig(cache_dir=None, force_download=False, resume_download=False, local_files_only=False, proxies=None, user_agent=None, extract_compressed_file=True, force_extract=True)
download_kwargs = {}
def setup_module(file_path: str, download_config: Optional[DownloadConfig] = None, **download_kwargs,) -> DatasetBuilder:
r"""
Download/extract/cache a dataset to add to the lib from a path or url which can be:
- a path to a local directory containing the dataset processing python script
- an url to a S3 directory with a dataset processing python script
Dataset codes are cached inside the lib to allow easy import (avoid ugly sys.path tweaks)
and using cloudpickle (among other things).
Return: tuple of
the unique id associated to the dataset
the local path to the dataset
"""
if download_config is None:
download_config = DownloadConfig(**download_kwargs)
download_config.extract_compressed_file = True
download_config.force_extract = True
> name = list(filter(lambda x: x, file_path.split("/")))[-1] + ".py"
E AttributeError: module 'tests.test_dataset_common' has no attribute 'split'
src/nlp/load.py:169: AttributeError
============================================================================== warnings summary ===============================================================================
/Users/thomwolf/miniconda2/envs/datasets/lib/python3.7/site-packages/tensorflow_core/python/pywrap_tensorflow_internal.py:15
/Users/thomwolf/miniconda2/envs/datasets/lib/python3.7/site-packages/tensorflow_core/python/pywrap_tensorflow_internal.py:15: DeprecationWarning: the imp module is deprecated in favour of importlib; see the module's documentation for alternative uses
import imp
-- Docs: https://docs.pytest.org/en/latest/warnings.html
=========================================================================== short test summary info ===========================================================================
ERROR tests/test_dataset_common.py::DatasetTest::test_builder_class_snli - AttributeError: module 'tests.test_dataset_common' has no attribute 'split'
========================================================================= 1 warning, 1 error in 3.63s =========================================================================
```
Also PR: #25 introduced some renaming: `DatasetBuilder.builder_config` -> `DatasetBuilder.config` so that we will have to change most of the dataset scripts (Just replace the "builder_config" with "config").
I think the renaming is a good idea and I can do the fix with a bash regex, but will have to re-upload most of the datasets. @thomwolf @mariamabarham
|
https://github.com/huggingface/datasets/pull/59 | Fix tests | > Also PR: #25 introduced some renaming: `DatasetBuilder.builder_config` -> `DatasetBuilder.config` so that we will have to change most of the dataset scripts (Just replace the "builder_config" with "config").
>
> I think the renaming is a good idea and I can do the fix with a bash regex, but will have to re-upload most of the datasets. @thomwolf @mariamabarham
I think if it only needs a re-uploading, we can rename it, `DatasetBuilder.config` is easier and sounds better | @patrickvonplaten I've broken a bit the tests with #25 while simplifying and re-organizing the `load.py` and `download_manager.py` scripts.
I'm trying to fix them here but I have a weird error, do you think you can have a look?
```bash
(datasets) MacBook-Pro-de-Thomas:datasets thomwolf$ python -m pytest -sv ./tests/test_dataset_common.py::DatasetTest::test_builder_class_snli
============================================================================= test session starts =============================================================================
platform darwin -- Python 3.7.7, pytest-5.4.1, py-1.8.1, pluggy-0.13.1 -- /Users/thomwolf/miniconda2/envs/datasets/bin/python
cachedir: .pytest_cache
rootdir: /Users/thomwolf/Documents/GitHub/datasets
plugins: xdist-1.31.0, forked-1.1.3
collected 1 item
tests/test_dataset_common.py::DatasetTest::test_builder_class_snli ERROR
=================================================================================== ERRORS ====================================================================================
____________________________________________________________ ERROR at setup of DatasetTest.test_builder_class_snli ____________________________________________________________
file_path = <module 'tests.test_dataset_common' from '/Users/thomwolf/Documents/GitHub/datasets/tests/test_dataset_common.py'>
download_config = DownloadConfig(cache_dir=None, force_download=False, resume_download=False, local_files_only=False, proxies=None, user_agent=None, extract_compressed_file=True, force_extract=True)
download_kwargs = {}
def setup_module(file_path: str, download_config: Optional[DownloadConfig] = None, **download_kwargs,) -> DatasetBuilder:
r"""
Download/extract/cache a dataset to add to the lib from a path or url which can be:
- a path to a local directory containing the dataset processing python script
- an url to a S3 directory with a dataset processing python script
Dataset codes are cached inside the lib to allow easy import (avoid ugly sys.path tweaks)
and using cloudpickle (among other things).
Return: tuple of
the unique id associated to the dataset
the local path to the dataset
"""
if download_config is None:
download_config = DownloadConfig(**download_kwargs)
download_config.extract_compressed_file = True
download_config.force_extract = True
> name = list(filter(lambda x: x, file_path.split("/")))[-1] + ".py"
E AttributeError: module 'tests.test_dataset_common' has no attribute 'split'
src/nlp/load.py:169: AttributeError
============================================================================== warnings summary ===============================================================================
/Users/thomwolf/miniconda2/envs/datasets/lib/python3.7/site-packages/tensorflow_core/python/pywrap_tensorflow_internal.py:15
/Users/thomwolf/miniconda2/envs/datasets/lib/python3.7/site-packages/tensorflow_core/python/pywrap_tensorflow_internal.py:15: DeprecationWarning: the imp module is deprecated in favour of importlib; see the module's documentation for alternative uses
import imp
-- Docs: https://docs.pytest.org/en/latest/warnings.html
=========================================================================== short test summary info ===========================================================================
ERROR tests/test_dataset_common.py::DatasetTest::test_builder_class_snli - AttributeError: module 'tests.test_dataset_common' has no attribute 'split'
========================================================================= 1 warning, 1 error in 3.63s =========================================================================
```
| 77 | text: Fix tests
@patrickvonplaten I've broken a bit the tests with #25 while simplifying and re-organizing the `load.py` and `download_manager.py` scripts.
I'm trying to fix them here but I have a weird error, do you think you can have a look?
```bash
(datasets) MacBook-Pro-de-Thomas:datasets thomwolf$ python -m pytest -sv ./tests/test_dataset_common.py::DatasetTest::test_builder_class_snli
============================================================================= test session starts =============================================================================
platform darwin -- Python 3.7.7, pytest-5.4.1, py-1.8.1, pluggy-0.13.1 -- /Users/thomwolf/miniconda2/envs/datasets/bin/python
cachedir: .pytest_cache
rootdir: /Users/thomwolf/Documents/GitHub/datasets
plugins: xdist-1.31.0, forked-1.1.3
collected 1 item
tests/test_dataset_common.py::DatasetTest::test_builder_class_snli ERROR
=================================================================================== ERRORS ====================================================================================
____________________________________________________________ ERROR at setup of DatasetTest.test_builder_class_snli ____________________________________________________________
file_path = <module 'tests.test_dataset_common' from '/Users/thomwolf/Documents/GitHub/datasets/tests/test_dataset_common.py'>
download_config = DownloadConfig(cache_dir=None, force_download=False, resume_download=False, local_files_only=False, proxies=None, user_agent=None, extract_compressed_file=True, force_extract=True)
download_kwargs = {}
def setup_module(file_path: str, download_config: Optional[DownloadConfig] = None, **download_kwargs,) -> DatasetBuilder:
r"""
Download/extract/cache a dataset to add to the lib from a path or url which can be:
- a path to a local directory containing the dataset processing python script
- an url to a S3 directory with a dataset processing python script
Dataset codes are cached inside the lib to allow easy import (avoid ugly sys.path tweaks)
and using cloudpickle (among other things).
Return: tuple of
the unique id associated to the dataset
the local path to the dataset
"""
if download_config is None:
download_config = DownloadConfig(**download_kwargs)
download_config.extract_compressed_file = True
download_config.force_extract = True
> name = list(filter(lambda x: x, file_path.split("/")))[-1] + ".py"
E AttributeError: module 'tests.test_dataset_common' has no attribute 'split'
src/nlp/load.py:169: AttributeError
============================================================================== warnings summary ===============================================================================
/Users/thomwolf/miniconda2/envs/datasets/lib/python3.7/site-packages/tensorflow_core/python/pywrap_tensorflow_internal.py:15
/Users/thomwolf/miniconda2/envs/datasets/lib/python3.7/site-packages/tensorflow_core/python/pywrap_tensorflow_internal.py:15: DeprecationWarning: the imp module is deprecated in favour of importlib; see the module's documentation for alternative uses
import imp
-- Docs: https://docs.pytest.org/en/latest/warnings.html
=========================================================================== short test summary info ===========================================================================
ERROR tests/test_dataset_common.py::DatasetTest::test_builder_class_snli - AttributeError: module 'tests.test_dataset_common' has no attribute 'split'
========================================================================= 1 warning, 1 error in 3.63s =========================================================================
```
> Also PR: #25 introduced some renaming: `DatasetBuilder.builder_config` -> `DatasetBuilder.config` so that we will have to change most of the dataset scripts (Just replace the "builder_config" with "config").
>
> I think the renaming is a good idea and I can do the fix with a bash regex, but will have to re-upload most of the datasets. @thomwolf @mariamabarham
I think if it only needs a re-uploading, we can rename it, `DatasetBuilder.config` is easier and sounds better |
https://github.com/huggingface/datasets/pull/57 | Better cached path | I should have read this PR before doing my own: https://github.com/huggingface/nlp/pull/62 :D
will close mine. Looks great :-) | ### Changes:
- The `cached_path` no longer returns None if the file is missing/the url doesn't work. Instead, it can raise `FileNotFoundError` (missing file), `ConnectionError` (no cache and unreachable url) or `ValueError` (parsing error)
- Fix requests to firebase API that doesn't handle HEAD requests...
- Allow custom download in datasets script: it allows to use `tf.io.gfile.copy` for example, to download from google storage. I added an example: the `boolq` script | 18 | text: Better cached path
### Changes:
- The `cached_path` no longer returns None if the file is missing/the url doesn't work. Instead, it can raise `FileNotFoundError` (missing file), `ConnectionError` (no cache and unreachable url) or `ValueError` (parsing error)
- Fix requests to firebase API that doesn't handle HEAD requests...
- Allow custom download in datasets script: it allows to use `tf.io.gfile.copy` for example, to download from google storage. I added an example: the `boolq` script
I should have read this PR before doing my own: https://github.com/huggingface/nlp/pull/62 :D
will close mine. Looks great :-) |
https://github.com/huggingface/datasets/pull/57 | Better cached path | > Awesome, this is really nice!
>
> By the way, we should improve the `cached_path` method of the `transformers` repo similarly, don't you think (@patrickvonplaten in particular).
Yeah, we should do the same in `transformers` I think - will note it down. | ### Changes:
- The `cached_path` no longer returns None if the file is missing/the url doesn't work. Instead, it can raise `FileNotFoundError` (missing file), `ConnectionError` (no cache and unreachable url) or `ValueError` (parsing error)
- Fix requests to firebase API that doesn't handle HEAD requests...
- Allow custom download in datasets script: it allows to use `tf.io.gfile.copy` for example, to download from google storage. I added an example: the `boolq` script | 43 | text: Better cached path
### Changes:
- The `cached_path` no longer returns None if the file is missing/the url doesn't work. Instead, it can raise `FileNotFoundError` (missing file), `ConnectionError` (no cache and unreachable url) or `ValueError` (parsing error)
- Fix requests to firebase API that doesn't handle HEAD requests...
- Allow custom download in datasets script: it allows to use `tf.io.gfile.copy` for example, to download from google storage. I added an example: the `boolq` script
> Awesome, this is really nice!
>
> By the way, we should improve the `cached_path` method of the `transformers` repo similarly, don't you think (@patrickvonplaten in particular).
Yeah, we should do the same in `transformers` I think - will note it down. |
https://github.com/huggingface/datasets/pull/55 | Beam datasets | Right now the changes are a bit hard to read as the one from #25 are also included. You can wait until #25 is merged before looking at the implementation details | # Beam datasets
## Intro
Beam Datasets are using beam pipelines for preprocessing (basically lots of `.map` over objects called PCollections).
The advantage of apache beam is that you can choose which type of runner you want to use to preprocess your data. The main runners are:
- the `DirectRunner` to run the pipeline locally (default). However I encountered memory issues for big datasets (like the french or english wikipedia). Small dataset work fine
- Google Dataflow. I didn't play with it.
- Spark or Flink, two well known data processing frameworks. I tried to use the Spark/Flink local runners provided by apache beam for python and wasn't able to make them work properly though...
## From tfds beam datasets to our own beam datasets
Tensorflow datasets used beam and a complicated pipeline to shard the TFRecords files.
To allow users to download beam datasets and not having to preprocess them, they also allow to download the already preprocessed datasets from their google storage (the beam pipeline doesn't run in that case).
On our side, we replace TFRecords by something else. Arrow or Parquet do the job but I chose Parquet as: 1) there is a builtin apache beam parquet writer that is quite convenient, and 2) reading parquet from the pyarrow library is also simple and effective (there is a mmap option !)
Moreover we don't shard datasets in many many files like tfds (they were doing probably doing that mainly because of the limit of 2Gb per TFRecord file). Therefore we have a simpler pipeline that saves each split into one parquet file. We also removed the utilities to use their google storage (for now maybe ? we'll have to discuss it).
## Main changes
- Added a BeamWriter to save the output of beam pipelines into parquet files and fill dataset infos
- Create a ParquetReader and refactor a bit the arrow_reader.py
\> **With this, we can now try to add beam datasets from tfds**
I already added the wikipedia one, and I will also try to add the Wiki40b dataset
## Test the wikipedia script
You can download and run the beam pipeline for wikipedia (using the `DirectRunner` by default) like this:
```
>>> import nlp
>>> nlp.load("datasets/nlp/wikipedia", dataset_config="20200501.frr")
```
This wikipedia dataset (lang: frr, North Frisian) is a small one (~10Mb), but feel free to try bigger ones (and fill 20Gb of swap memory if you try the english one lol)
## Next
Should we allow to download preprocessed datasets from the tfds google storage ?
Should we try to optimize the beam pipelines to run locally without memory issues ?
Should we try other data processing frameworks for big datasets, like spark ?
## About this PR
It should be merged after #25
-----------------
I'd be happy to have your feedback and your ideas to improve the processing of big datasets like wikipedia :) | 31 | text: Beam datasets
# Beam datasets
## Intro
Beam Datasets are using beam pipelines for preprocessing (basically lots of `.map` over objects called PCollections).
The advantage of apache beam is that you can choose which type of runner you want to use to preprocess your data. The main runners are:
- the `DirectRunner` to run the pipeline locally (default). However I encountered memory issues for big datasets (like the french or english wikipedia). Small dataset work fine
- Google Dataflow. I didn't play with it.
- Spark or Flink, two well known data processing frameworks. I tried to use the Spark/Flink local runners provided by apache beam for python and wasn't able to make them work properly though...
## From tfds beam datasets to our own beam datasets
Tensorflow datasets used beam and a complicated pipeline to shard the TFRecords files.
To allow users to download beam datasets and not having to preprocess them, they also allow to download the already preprocessed datasets from their google storage (the beam pipeline doesn't run in that case).
On our side, we replace TFRecords by something else. Arrow or Parquet do the job but I chose Parquet as: 1) there is a builtin apache beam parquet writer that is quite convenient, and 2) reading parquet from the pyarrow library is also simple and effective (there is a mmap option !)
Moreover we don't shard datasets in many many files like tfds (they were doing probably doing that mainly because of the limit of 2Gb per TFRecord file). Therefore we have a simpler pipeline that saves each split into one parquet file. We also removed the utilities to use their google storage (for now maybe ? we'll have to discuss it).
## Main changes
- Added a BeamWriter to save the output of beam pipelines into parquet files and fill dataset infos
- Create a ParquetReader and refactor a bit the arrow_reader.py
\> **With this, we can now try to add beam datasets from tfds**
I already added the wikipedia one, and I will also try to add the Wiki40b dataset
## Test the wikipedia script
You can download and run the beam pipeline for wikipedia (using the `DirectRunner` by default) like this:
```
>>> import nlp
>>> nlp.load("datasets/nlp/wikipedia", dataset_config="20200501.frr")
```
This wikipedia dataset (lang: frr, North Frisian) is a small one (~10Mb), but feel free to try bigger ones (and fill 20Gb of swap memory if you try the english one lol)
## Next
Should we allow to download preprocessed datasets from the tfds google storage ?
Should we try to optimize the beam pipelines to run locally without memory issues ?
Should we try other data processing frameworks for big datasets, like spark ?
## About this PR
It should be merged after #25
-----------------
I'd be happy to have your feedback and your ideas to improve the processing of big datasets like wikipedia :)
Right now the changes are a bit hard to read as the one from #25 are also included. You can wait until #25 is merged before looking at the implementation details |
https://github.com/huggingface/datasets/pull/55 | Beam datasets | Nice!! I tested it a bit and works quite well. I will do a my review once the #25 will be merged because there are several overlaps.
At least I can share my thoughts on your **Next** section:
1) I don't think it is a good thing to rely on tfds preprocessed datasets uploaded in their online storage, because they might be updated or deleted at any moment by Google and then possibly break our own processing.
2) Improves the pipeline is always a good direction, but in the meantime we might also share the preprocessed dataset in S3 storage. Which might be another way to see 1), instead of downloading Google preprocessed datasets, using our own ones.
3) Apache Beam can be easily integrated in Spark, so I don't see the need to replace Beam by Spark. | # Beam datasets
## Intro
Beam Datasets are using beam pipelines for preprocessing (basically lots of `.map` over objects called PCollections).
The advantage of apache beam is that you can choose which type of runner you want to use to preprocess your data. The main runners are:
- the `DirectRunner` to run the pipeline locally (default). However I encountered memory issues for big datasets (like the french or english wikipedia). Small dataset work fine
- Google Dataflow. I didn't play with it.
- Spark or Flink, two well known data processing frameworks. I tried to use the Spark/Flink local runners provided by apache beam for python and wasn't able to make them work properly though...
## From tfds beam datasets to our own beam datasets
Tensorflow datasets used beam and a complicated pipeline to shard the TFRecords files.
To allow users to download beam datasets and not having to preprocess them, they also allow to download the already preprocessed datasets from their google storage (the beam pipeline doesn't run in that case).
On our side, we replace TFRecords by something else. Arrow or Parquet do the job but I chose Parquet as: 1) there is a builtin apache beam parquet writer that is quite convenient, and 2) reading parquet from the pyarrow library is also simple and effective (there is a mmap option !)
Moreover we don't shard datasets in many many files like tfds (they were doing probably doing that mainly because of the limit of 2Gb per TFRecord file). Therefore we have a simpler pipeline that saves each split into one parquet file. We also removed the utilities to use their google storage (for now maybe ? we'll have to discuss it).
## Main changes
- Added a BeamWriter to save the output of beam pipelines into parquet files and fill dataset infos
- Create a ParquetReader and refactor a bit the arrow_reader.py
\> **With this, we can now try to add beam datasets from tfds**
I already added the wikipedia one, and I will also try to add the Wiki40b dataset
## Test the wikipedia script
You can download and run the beam pipeline for wikipedia (using the `DirectRunner` by default) like this:
```
>>> import nlp
>>> nlp.load("datasets/nlp/wikipedia", dataset_config="20200501.frr")
```
This wikipedia dataset (lang: frr, North Frisian) is a small one (~10Mb), but feel free to try bigger ones (and fill 20Gb of swap memory if you try the english one lol)
## Next
Should we allow to download preprocessed datasets from the tfds google storage ?
Should we try to optimize the beam pipelines to run locally without memory issues ?
Should we try other data processing frameworks for big datasets, like spark ?
## About this PR
It should be merged after #25
-----------------
I'd be happy to have your feedback and your ideas to improve the processing of big datasets like wikipedia :) | 138 | text: Beam datasets
# Beam datasets
## Intro
Beam Datasets are using beam pipelines for preprocessing (basically lots of `.map` over objects called PCollections).
The advantage of apache beam is that you can choose which type of runner you want to use to preprocess your data. The main runners are:
- the `DirectRunner` to run the pipeline locally (default). However I encountered memory issues for big datasets (like the french or english wikipedia). Small dataset work fine
- Google Dataflow. I didn't play with it.
- Spark or Flink, two well known data processing frameworks. I tried to use the Spark/Flink local runners provided by apache beam for python and wasn't able to make them work properly though...
## From tfds beam datasets to our own beam datasets
Tensorflow datasets used beam and a complicated pipeline to shard the TFRecords files.
To allow users to download beam datasets and not having to preprocess them, they also allow to download the already preprocessed datasets from their google storage (the beam pipeline doesn't run in that case).
On our side, we replace TFRecords by something else. Arrow or Parquet do the job but I chose Parquet as: 1) there is a builtin apache beam parquet writer that is quite convenient, and 2) reading parquet from the pyarrow library is also simple and effective (there is a mmap option !)
Moreover we don't shard datasets in many many files like tfds (they were doing probably doing that mainly because of the limit of 2Gb per TFRecord file). Therefore we have a simpler pipeline that saves each split into one parquet file. We also removed the utilities to use their google storage (for now maybe ? we'll have to discuss it).
## Main changes
- Added a BeamWriter to save the output of beam pipelines into parquet files and fill dataset infos
- Create a ParquetReader and refactor a bit the arrow_reader.py
\> **With this, we can now try to add beam datasets from tfds**
I already added the wikipedia one, and I will also try to add the Wiki40b dataset
## Test the wikipedia script
You can download and run the beam pipeline for wikipedia (using the `DirectRunner` by default) like this:
```
>>> import nlp
>>> nlp.load("datasets/nlp/wikipedia", dataset_config="20200501.frr")
```
This wikipedia dataset (lang: frr, North Frisian) is a small one (~10Mb), but feel free to try bigger ones (and fill 20Gb of swap memory if you try the english one lol)
## Next
Should we allow to download preprocessed datasets from the tfds google storage ?
Should we try to optimize the beam pipelines to run locally without memory issues ?
Should we try other data processing frameworks for big datasets, like spark ?
## About this PR
It should be merged after #25
-----------------
I'd be happy to have your feedback and your ideas to improve the processing of big datasets like wikipedia :)
Nice!! I tested it a bit and works quite well. I will do a my review once the #25 will be merged because there are several overlaps.
At least I can share my thoughts on your **Next** section:
1) I don't think it is a good thing to rely on tfds preprocessed datasets uploaded in their online storage, because they might be updated or deleted at any moment by Google and then possibly break our own processing.
2) Improves the pipeline is always a good direction, but in the meantime we might also share the preprocessed dataset in S3 storage. Which might be another way to see 1), instead of downloading Google preprocessed datasets, using our own ones.
3) Apache Beam can be easily integrated in Spark, so I don't see the need to replace Beam by Spark. |
https://github.com/huggingface/datasets/pull/55 | Beam datasets | Ok I've merged #25 so you can rebase or merge if you want.
I fully agree with @jplu notes for the "next section".
Don't hesitate to use some credit on Google Dataflow if you think it would be useful to give it a try. | # Beam datasets
## Intro
Beam Datasets are using beam pipelines for preprocessing (basically lots of `.map` over objects called PCollections).
The advantage of apache beam is that you can choose which type of runner you want to use to preprocess your data. The main runners are:
- the `DirectRunner` to run the pipeline locally (default). However I encountered memory issues for big datasets (like the french or english wikipedia). Small dataset work fine
- Google Dataflow. I didn't play with it.
- Spark or Flink, two well known data processing frameworks. I tried to use the Spark/Flink local runners provided by apache beam for python and wasn't able to make them work properly though...
## From tfds beam datasets to our own beam datasets
Tensorflow datasets used beam and a complicated pipeline to shard the TFRecords files.
To allow users to download beam datasets and not having to preprocess them, they also allow to download the already preprocessed datasets from their google storage (the beam pipeline doesn't run in that case).
On our side, we replace TFRecords by something else. Arrow or Parquet do the job but I chose Parquet as: 1) there is a builtin apache beam parquet writer that is quite convenient, and 2) reading parquet from the pyarrow library is also simple and effective (there is a mmap option !)
Moreover we don't shard datasets in many many files like tfds (they were doing probably doing that mainly because of the limit of 2Gb per TFRecord file). Therefore we have a simpler pipeline that saves each split into one parquet file. We also removed the utilities to use their google storage (for now maybe ? we'll have to discuss it).
## Main changes
- Added a BeamWriter to save the output of beam pipelines into parquet files and fill dataset infos
- Create a ParquetReader and refactor a bit the arrow_reader.py
\> **With this, we can now try to add beam datasets from tfds**
I already added the wikipedia one, and I will also try to add the Wiki40b dataset
## Test the wikipedia script
You can download and run the beam pipeline for wikipedia (using the `DirectRunner` by default) like this:
```
>>> import nlp
>>> nlp.load("datasets/nlp/wikipedia", dataset_config="20200501.frr")
```
This wikipedia dataset (lang: frr, North Frisian) is a small one (~10Mb), but feel free to try bigger ones (and fill 20Gb of swap memory if you try the english one lol)
## Next
Should we allow to download preprocessed datasets from the tfds google storage ?
Should we try to optimize the beam pipelines to run locally without memory issues ?
Should we try other data processing frameworks for big datasets, like spark ?
## About this PR
It should be merged after #25
-----------------
I'd be happy to have your feedback and your ideas to improve the processing of big datasets like wikipedia :) | 44 | text: Beam datasets
# Beam datasets
## Intro
Beam Datasets are using beam pipelines for preprocessing (basically lots of `.map` over objects called PCollections).
The advantage of apache beam is that you can choose which type of runner you want to use to preprocess your data. The main runners are:
- the `DirectRunner` to run the pipeline locally (default). However I encountered memory issues for big datasets (like the french or english wikipedia). Small dataset work fine
- Google Dataflow. I didn't play with it.
- Spark or Flink, two well known data processing frameworks. I tried to use the Spark/Flink local runners provided by apache beam for python and wasn't able to make them work properly though...
## From tfds beam datasets to our own beam datasets
Tensorflow datasets used beam and a complicated pipeline to shard the TFRecords files.
To allow users to download beam datasets and not having to preprocess them, they also allow to download the already preprocessed datasets from their google storage (the beam pipeline doesn't run in that case).
On our side, we replace TFRecords by something else. Arrow or Parquet do the job but I chose Parquet as: 1) there is a builtin apache beam parquet writer that is quite convenient, and 2) reading parquet from the pyarrow library is also simple and effective (there is a mmap option !)
Moreover we don't shard datasets in many many files like tfds (they were doing probably doing that mainly because of the limit of 2Gb per TFRecord file). Therefore we have a simpler pipeline that saves each split into one parquet file. We also removed the utilities to use their google storage (for now maybe ? we'll have to discuss it).
## Main changes
- Added a BeamWriter to save the output of beam pipelines into parquet files and fill dataset infos
- Create a ParquetReader and refactor a bit the arrow_reader.py
\> **With this, we can now try to add beam datasets from tfds**
I already added the wikipedia one, and I will also try to add the Wiki40b dataset
## Test the wikipedia script
You can download and run the beam pipeline for wikipedia (using the `DirectRunner` by default) like this:
```
>>> import nlp
>>> nlp.load("datasets/nlp/wikipedia", dataset_config="20200501.frr")
```
This wikipedia dataset (lang: frr, North Frisian) is a small one (~10Mb), but feel free to try bigger ones (and fill 20Gb of swap memory if you try the english one lol)
## Next
Should we allow to download preprocessed datasets from the tfds google storage ?
Should we try to optimize the beam pipelines to run locally without memory issues ?
Should we try other data processing frameworks for big datasets, like spark ?
## About this PR
It should be merged after #25
-----------------
I'd be happy to have your feedback and your ideas to improve the processing of big datasets like wikipedia :)
Ok I've merged #25 so you can rebase or merge if you want.
I fully agree with @jplu notes for the "next section".
Don't hesitate to use some credit on Google Dataflow if you think it would be useful to give it a try. |
https://github.com/huggingface/datasets/pull/55 | Beam datasets | Pr is ready for review !
New minor changes:
- re-added the csv dataset builder (it was on my branch from #25 but disappeared from master)
- move the csv script and the wikipedia script to "under construction" for now
- some renaming in the `nlp-cli test` command | # Beam datasets
## Intro
Beam Datasets are using beam pipelines for preprocessing (basically lots of `.map` over objects called PCollections).
The advantage of apache beam is that you can choose which type of runner you want to use to preprocess your data. The main runners are:
- the `DirectRunner` to run the pipeline locally (default). However I encountered memory issues for big datasets (like the french or english wikipedia). Small dataset work fine
- Google Dataflow. I didn't play with it.
- Spark or Flink, two well known data processing frameworks. I tried to use the Spark/Flink local runners provided by apache beam for python and wasn't able to make them work properly though...
## From tfds beam datasets to our own beam datasets
Tensorflow datasets used beam and a complicated pipeline to shard the TFRecords files.
To allow users to download beam datasets and not having to preprocess them, they also allow to download the already preprocessed datasets from their google storage (the beam pipeline doesn't run in that case).
On our side, we replace TFRecords by something else. Arrow or Parquet do the job but I chose Parquet as: 1) there is a builtin apache beam parquet writer that is quite convenient, and 2) reading parquet from the pyarrow library is also simple and effective (there is a mmap option !)
Moreover we don't shard datasets in many many files like tfds (they were doing probably doing that mainly because of the limit of 2Gb per TFRecord file). Therefore we have a simpler pipeline that saves each split into one parquet file. We also removed the utilities to use their google storage (for now maybe ? we'll have to discuss it).
## Main changes
- Added a BeamWriter to save the output of beam pipelines into parquet files and fill dataset infos
- Create a ParquetReader and refactor a bit the arrow_reader.py
\> **With this, we can now try to add beam datasets from tfds**
I already added the wikipedia one, and I will also try to add the Wiki40b dataset
## Test the wikipedia script
You can download and run the beam pipeline for wikipedia (using the `DirectRunner` by default) like this:
```
>>> import nlp
>>> nlp.load("datasets/nlp/wikipedia", dataset_config="20200501.frr")
```
This wikipedia dataset (lang: frr, North Frisian) is a small one (~10Mb), but feel free to try bigger ones (and fill 20Gb of swap memory if you try the english one lol)
## Next
Should we allow to download preprocessed datasets from the tfds google storage ?
Should we try to optimize the beam pipelines to run locally without memory issues ?
Should we try other data processing frameworks for big datasets, like spark ?
## About this PR
It should be merged after #25
-----------------
I'd be happy to have your feedback and your ideas to improve the processing of big datasets like wikipedia :) | 48 | text: Beam datasets
# Beam datasets
## Intro
Beam Datasets are using beam pipelines for preprocessing (basically lots of `.map` over objects called PCollections).
The advantage of apache beam is that you can choose which type of runner you want to use to preprocess your data. The main runners are:
- the `DirectRunner` to run the pipeline locally (default). However I encountered memory issues for big datasets (like the french or english wikipedia). Small dataset work fine
- Google Dataflow. I didn't play with it.
- Spark or Flink, two well known data processing frameworks. I tried to use the Spark/Flink local runners provided by apache beam for python and wasn't able to make them work properly though...
## From tfds beam datasets to our own beam datasets
Tensorflow datasets used beam and a complicated pipeline to shard the TFRecords files.
To allow users to download beam datasets and not having to preprocess them, they also allow to download the already preprocessed datasets from their google storage (the beam pipeline doesn't run in that case).
On our side, we replace TFRecords by something else. Arrow or Parquet do the job but I chose Parquet as: 1) there is a builtin apache beam parquet writer that is quite convenient, and 2) reading parquet from the pyarrow library is also simple and effective (there is a mmap option !)
Moreover we don't shard datasets in many many files like tfds (they were doing probably doing that mainly because of the limit of 2Gb per TFRecord file). Therefore we have a simpler pipeline that saves each split into one parquet file. We also removed the utilities to use their google storage (for now maybe ? we'll have to discuss it).
## Main changes
- Added a BeamWriter to save the output of beam pipelines into parquet files and fill dataset infos
- Create a ParquetReader and refactor a bit the arrow_reader.py
\> **With this, we can now try to add beam datasets from tfds**
I already added the wikipedia one, and I will also try to add the Wiki40b dataset
## Test the wikipedia script
You can download and run the beam pipeline for wikipedia (using the `DirectRunner` by default) like this:
```
>>> import nlp
>>> nlp.load("datasets/nlp/wikipedia", dataset_config="20200501.frr")
```
This wikipedia dataset (lang: frr, North Frisian) is a small one (~10Mb), but feel free to try bigger ones (and fill 20Gb of swap memory if you try the english one lol)
## Next
Should we allow to download preprocessed datasets from the tfds google storage ?
Should we try to optimize the beam pipelines to run locally without memory issues ?
Should we try other data processing frameworks for big datasets, like spark ?
## About this PR
It should be merged after #25
-----------------
I'd be happy to have your feedback and your ideas to improve the processing of big datasets like wikipedia :)
Pr is ready for review !
New minor changes:
- re-added the csv dataset builder (it was on my branch from #25 but disappeared from master)
- move the csv script and the wikipedia script to "under construction" for now
- some renaming in the `nlp-cli test` command |
https://github.com/huggingface/datasets/pull/43 | [Checksums] If no configs exist prevent to run over empty list | Whoops I fixed it directly on master before checking that you have done it in this PR. We may close it | `movie_rationales` e.g. has no configs. | 21 | text: [Checksums] If no configs exist prevent to run over empty list
`movie_rationales` e.g. has no configs.
Whoops I fixed it directly on master before checking that you have done it in this PR. We may close it |
https://github.com/huggingface/datasets/pull/43 | [Checksums] If no configs exist prevent to run over empty list | Yeah, I saw :-) But I think we should add this as well since some datasets have an empty list of configs and then as the code is now it would fail.
In this PR, I just make sure that the code jumps in the correct else if "there are no configs" as is the case for some datasets @mariamabarham | `movie_rationales` e.g. has no configs. | 60 | text: [Checksums] If no configs exist prevent to run over empty list
`movie_rationales` e.g. has no configs.
Yeah, I saw :-) But I think we should add this as well since some datasets have an empty list of configs and then as the code is now it would fail.
In this PR, I just make sure that the code jumps in the correct else if "there are no configs" as is the case for some datasets @mariamabarham |
https://github.com/huggingface/datasets/pull/43 | [Checksums] If no configs exist prevent to run over empty list | Sorry, I thought you meant a different commit . Just saw this one: https://github.com/huggingface/nlp/commit/7c644f284e2303b57612a6e7c904fe13906d893f
.
All good then :-) | `movie_rationales` e.g. has no configs. | 19 | text: [Checksums] If no configs exist prevent to run over empty list
`movie_rationales` e.g. has no configs.
Sorry, I thought you meant a different commit . Just saw this one: https://github.com/huggingface/nlp/commit/7c644f284e2303b57612a6e7c904fe13906d893f
.
All good then :-) |
https://github.com/huggingface/datasets/pull/37 | [Datasets ToDo-List] add datasets | Note:
```
nlp-cli test datasets/nlp/<your-dataset-folder> --save_checksums --all_configs
```
directly saves the checksums in the right place, and runs for all the dataset configurations. | ## Description
This PR acts as a dashboard to see which datasets are added to the library and work.
Cicle-ci should always be green so that we can be sure that newly added datasets are functional.
This PR should not be merged.
## Progress
**For the following datasets the test commands**:
```
RUN_SLOW=1 pytest tests/test_dataset_common.py::DatasetTest::test_load_real_dataset_<your-dataset-name>
```
and
```
RUN_SLOW=1 pytest tests/test_dataset_common.py::DatasetTest::test_load_dataset_all_configs_<your-dataset-name>
```
**passes**.
- [x] Squad
- [x] Sentiment140
- [x] XNLI
- [x] Crime_and_Punish
- [x] movie_rationales
- [x] ai2_arc
- [x] anli
- [x] event2Mind
- [x] Fquad
- [x] blimp
- [x] empathetic_dialogues
- [x] cosmos_qa
- [x] xquad
- [x] blog_authorship_corpus
- [x] SNLI
- [x] break_data
- [x] SQuAD v2
- [x] cfq
- [x] eraser_multi_rc
- [x] Glue
- [x] Tydiqa
- [x] wiki_qa
- [x] wikitext
- [x] winogrande
- [x] wiqa
- [x] esnli
- [x] civil_comments
- [x] commonsense_qa
- [x] com_qa
- [x] coqa
- [x] wiki_split
- [x] cos_e
- [x] xcopa
- [x] quarel
- [x] quartz
- [x] squad_it
- [x] quoref
- [x] squad_pt
- [x] cornell_movie_dialog
- [x] SciQ
- [x] Scifact
- [x] hellaswag
- [x] ted_multi (in translate)
- [x] Aeslc (summarization)
- [x] drop
- [x] gap
- [x] hansard
- [x] opinosis
- [x] MLQA
- [x] math_dataset
## How-To-Add a dataset
**Before adding a dataset make sure that your branch is up to date**:
1. `git checkout add_datasets`
2. `git pull`
**Add a dataset via the `convert_dataset.sh` bash script:**
Running `bash convert_dataset.sh <file/to/tfds/datascript.py>` (*e.g.* `bash convert_dataset.sh ../tensorflow-datasets/tensorflow_datasets/text/movie_rationales.py`) will automatically run all the steps mentioned in **Add a dataset manually** below.
Make sure that you run `convert_dataset.sh` from the root folder of `nlp`.
The conversion script should work almost always for step 1): "convert dataset script from tfds to nlp format" and 2) "create checksum file" and step 3) "make style".
It can also sometimes automatically run step 4) "create the correct dummy data from tfds", but this will only work if a) there is either no config name or only one config name and b) the `tfds testing/test_data/fake_example` is in the correct form.
Nevertheless, the script should always be run in the beginning until an error occurs to be more efficient.
If the conversion script does not work or fails at some step, then you can run the steps manually as follows:
**Add a dataset manually**
Make sure you run all of the following commands from the root of your `nlp` git clone.
Also make sure that you changed to this branch:
```
git checkout add_datasets
```
1) the tfds datascript file should be converted to `nlp` style:
```
python nlp-cli convert --tfds_path <path/to/tensorflow_datasets/text/your_dataset_name>.py --nlp_directory datasets/nlp
```
This will convert the tdfs script and create a folder with the correct name.
2) the checksum file should be added. Use the command:
```
python nlp-cli test datasets/nlp/<your-dataset-folder> --save_checksums --all_configs
```
A checksums.txt file should be created in your folder and the structure should look as follows:
squad/
βββ squad.py/
βββ urls_checksums/
...........βββ checksums.txt
Delete the created `*.lock` file afterward - it should not be uploaded to AWS.
3) run black and isort on your newly added datascript files so that they look nice:
```
make style
```
4) the dummy data should be added. For this it might be useful to take a look into the structure of other examples as shown in the PR here and at `<path/to/tensorflow_datasets/testing/test_data/test_data/fake_examples>` whether the same data can be used.
5) the data can be uploaded to AWS using the command
```
aws s3 cp datasets/nlp/<your-dataset-folder> s3://datasets.huggingface.co/nlp/<your-dataset-folder> --recursive
```
6) check whether all works as expected using:
```
RUN_SLOW=1 pytest tests/test_dataset_common.py::DatasetTest::test_load_real_dataset_<your-dataset-name>
```
and
```
RUN_SLOW=1 pytest tests/test_dataset_common.py::DatasetTest::test_load_dataset_all_configs_<your-dataset-name>
```
7) push to this PR and rerun the circle ci workflow to check whether circle ci stays green.
8) Edit this commend and tick off your newly added dataset :-)
## TODO-list
Maybe we can add a TODO-list here for everybody that feels like adding new datasets so that we will not add the same datasets.
Here a link to available datasets: https://docs.google.com/spreadsheets/d/1zOtEqOrnVQwdgkC4nJrTY6d-Av02u0XFzeKAtBM2fUI/edit#gid=0
Patrick:
- [ ] boolq - *weird download link*
- [ ] c4 - *beam dataset* | 23 | text: [Datasets ToDo-List] add datasets
## Description
This PR acts as a dashboard to see which datasets are added to the library and work.
Cicle-ci should always be green so that we can be sure that newly added datasets are functional.
This PR should not be merged.
## Progress
**For the following datasets the test commands**:
```
RUN_SLOW=1 pytest tests/test_dataset_common.py::DatasetTest::test_load_real_dataset_<your-dataset-name>
```
and
```
RUN_SLOW=1 pytest tests/test_dataset_common.py::DatasetTest::test_load_dataset_all_configs_<your-dataset-name>
```
**passes**.
- [x] Squad
- [x] Sentiment140
- [x] XNLI
- [x] Crime_and_Punish
- [x] movie_rationales
- [x] ai2_arc
- [x] anli
- [x] event2Mind
- [x] Fquad
- [x] blimp
- [x] empathetic_dialogues
- [x] cosmos_qa
- [x] xquad
- [x] blog_authorship_corpus
- [x] SNLI
- [x] break_data
- [x] SQuAD v2
- [x] cfq
- [x] eraser_multi_rc
- [x] Glue
- [x] Tydiqa
- [x] wiki_qa
- [x] wikitext
- [x] winogrande
- [x] wiqa
- [x] esnli
- [x] civil_comments
- [x] commonsense_qa
- [x] com_qa
- [x] coqa
- [x] wiki_split
- [x] cos_e
- [x] xcopa
- [x] quarel
- [x] quartz
- [x] squad_it
- [x] quoref
- [x] squad_pt
- [x] cornell_movie_dialog
- [x] SciQ
- [x] Scifact
- [x] hellaswag
- [x] ted_multi (in translate)
- [x] Aeslc (summarization)
- [x] drop
- [x] gap
- [x] hansard
- [x] opinosis
- [x] MLQA
- [x] math_dataset
## How-To-Add a dataset
**Before adding a dataset make sure that your branch is up to date**:
1. `git checkout add_datasets`
2. `git pull`
**Add a dataset via the `convert_dataset.sh` bash script:**
Running `bash convert_dataset.sh <file/to/tfds/datascript.py>` (*e.g.* `bash convert_dataset.sh ../tensorflow-datasets/tensorflow_datasets/text/movie_rationales.py`) will automatically run all the steps mentioned in **Add a dataset manually** below.
Make sure that you run `convert_dataset.sh` from the root folder of `nlp`.
The conversion script should work almost always for step 1): "convert dataset script from tfds to nlp format" and 2) "create checksum file" and step 3) "make style".
It can also sometimes automatically run step 4) "create the correct dummy data from tfds", but this will only work if a) there is either no config name or only one config name and b) the `tfds testing/test_data/fake_example` is in the correct form.
Nevertheless, the script should always be run in the beginning until an error occurs to be more efficient.
If the conversion script does not work or fails at some step, then you can run the steps manually as follows:
**Add a dataset manually**
Make sure you run all of the following commands from the root of your `nlp` git clone.
Also make sure that you changed to this branch:
```
git checkout add_datasets
```
1) the tfds datascript file should be converted to `nlp` style:
```
python nlp-cli convert --tfds_path <path/to/tensorflow_datasets/text/your_dataset_name>.py --nlp_directory datasets/nlp
```
This will convert the tdfs script and create a folder with the correct name.
2) the checksum file should be added. Use the command:
```
python nlp-cli test datasets/nlp/<your-dataset-folder> --save_checksums --all_configs
```
A checksums.txt file should be created in your folder and the structure should look as follows:
squad/
βββ squad.py/
βββ urls_checksums/
...........βββ checksums.txt
Delete the created `*.lock` file afterward - it should not be uploaded to AWS.
3) run black and isort on your newly added datascript files so that they look nice:
```
make style
```
4) the dummy data should be added. For this it might be useful to take a look into the structure of other examples as shown in the PR here and at `<path/to/tensorflow_datasets/testing/test_data/test_data/fake_examples>` whether the same data can be used.
5) the data can be uploaded to AWS using the command
```
aws s3 cp datasets/nlp/<your-dataset-folder> s3://datasets.huggingface.co/nlp/<your-dataset-folder> --recursive
```
6) check whether all works as expected using:
```
RUN_SLOW=1 pytest tests/test_dataset_common.py::DatasetTest::test_load_real_dataset_<your-dataset-name>
```
and
```
RUN_SLOW=1 pytest tests/test_dataset_common.py::DatasetTest::test_load_dataset_all_configs_<your-dataset-name>
```
7) push to this PR and rerun the circle ci workflow to check whether circle ci stays green.
8) Edit this commend and tick off your newly added dataset :-)
## TODO-list
Maybe we can add a TODO-list here for everybody that feels like adding new datasets so that we will not add the same datasets.
Here a link to available datasets: https://docs.google.com/spreadsheets/d/1zOtEqOrnVQwdgkC4nJrTY6d-Av02u0XFzeKAtBM2fUI/edit#gid=0
Patrick:
- [ ] boolq - *weird download link*
- [ ] c4 - *beam dataset*
Note:
```
nlp-cli test datasets/nlp/<your-dataset-folder> --save_checksums --all_configs
```
directly saves the checksums in the right place, and runs for all the dataset configurations. |
https://github.com/huggingface/datasets/pull/37 | [Datasets ToDo-List] add datasets | https://github.com/huggingface/nlp/pull/15 - But it's probably best to checkout into this branch and look how the dummy data strtucture is for `squad` for example. | ## Description
This PR acts as a dashboard to see which datasets are added to the library and work.
Cicle-ci should always be green so that we can be sure that newly added datasets are functional.
This PR should not be merged.
## Progress
**For the following datasets the test commands**:
```
RUN_SLOW=1 pytest tests/test_dataset_common.py::DatasetTest::test_load_real_dataset_<your-dataset-name>
```
and
```
RUN_SLOW=1 pytest tests/test_dataset_common.py::DatasetTest::test_load_dataset_all_configs_<your-dataset-name>
```
**passes**.
- [x] Squad
- [x] Sentiment140
- [x] XNLI
- [x] Crime_and_Punish
- [x] movie_rationales
- [x] ai2_arc
- [x] anli
- [x] event2Mind
- [x] Fquad
- [x] blimp
- [x] empathetic_dialogues
- [x] cosmos_qa
- [x] xquad
- [x] blog_authorship_corpus
- [x] SNLI
- [x] break_data
- [x] SQuAD v2
- [x] cfq
- [x] eraser_multi_rc
- [x] Glue
- [x] Tydiqa
- [x] wiki_qa
- [x] wikitext
- [x] winogrande
- [x] wiqa
- [x] esnli
- [x] civil_comments
- [x] commonsense_qa
- [x] com_qa
- [x] coqa
- [x] wiki_split
- [x] cos_e
- [x] xcopa
- [x] quarel
- [x] quartz
- [x] squad_it
- [x] quoref
- [x] squad_pt
- [x] cornell_movie_dialog
- [x] SciQ
- [x] Scifact
- [x] hellaswag
- [x] ted_multi (in translate)
- [x] Aeslc (summarization)
- [x] drop
- [x] gap
- [x] hansard
- [x] opinosis
- [x] MLQA
- [x] math_dataset
## How-To-Add a dataset
**Before adding a dataset make sure that your branch is up to date**:
1. `git checkout add_datasets`
2. `git pull`
**Add a dataset via the `convert_dataset.sh` bash script:**
Running `bash convert_dataset.sh <file/to/tfds/datascript.py>` (*e.g.* `bash convert_dataset.sh ../tensorflow-datasets/tensorflow_datasets/text/movie_rationales.py`) will automatically run all the steps mentioned in **Add a dataset manually** below.
Make sure that you run `convert_dataset.sh` from the root folder of `nlp`.
The conversion script should work almost always for step 1): "convert dataset script from tfds to nlp format" and 2) "create checksum file" and step 3) "make style".
It can also sometimes automatically run step 4) "create the correct dummy data from tfds", but this will only work if a) there is either no config name or only one config name and b) the `tfds testing/test_data/fake_example` is in the correct form.
Nevertheless, the script should always be run in the beginning until an error occurs to be more efficient.
If the conversion script does not work or fails at some step, then you can run the steps manually as follows:
**Add a dataset manually**
Make sure you run all of the following commands from the root of your `nlp` git clone.
Also make sure that you changed to this branch:
```
git checkout add_datasets
```
1) the tfds datascript file should be converted to `nlp` style:
```
python nlp-cli convert --tfds_path <path/to/tensorflow_datasets/text/your_dataset_name>.py --nlp_directory datasets/nlp
```
This will convert the tdfs script and create a folder with the correct name.
2) the checksum file should be added. Use the command:
```
python nlp-cli test datasets/nlp/<your-dataset-folder> --save_checksums --all_configs
```
A checksums.txt file should be created in your folder and the structure should look as follows:
squad/
βββ squad.py/
βββ urls_checksums/
...........βββ checksums.txt
Delete the created `*.lock` file afterward - it should not be uploaded to AWS.
3) run black and isort on your newly added datascript files so that they look nice:
```
make style
```
4) the dummy data should be added. For this it might be useful to take a look into the structure of other examples as shown in the PR here and at `<path/to/tensorflow_datasets/testing/test_data/test_data/fake_examples>` whether the same data can be used.
5) the data can be uploaded to AWS using the command
```
aws s3 cp datasets/nlp/<your-dataset-folder> s3://datasets.huggingface.co/nlp/<your-dataset-folder> --recursive
```
6) check whether all works as expected using:
```
RUN_SLOW=1 pytest tests/test_dataset_common.py::DatasetTest::test_load_real_dataset_<your-dataset-name>
```
and
```
RUN_SLOW=1 pytest tests/test_dataset_common.py::DatasetTest::test_load_dataset_all_configs_<your-dataset-name>
```
7) push to this PR and rerun the circle ci workflow to check whether circle ci stays green.
8) Edit this commend and tick off your newly added dataset :-)
## TODO-list
Maybe we can add a TODO-list here for everybody that feels like adding new datasets so that we will not add the same datasets.
Here a link to available datasets: https://docs.google.com/spreadsheets/d/1zOtEqOrnVQwdgkC4nJrTY6d-Av02u0XFzeKAtBM2fUI/edit#gid=0
Patrick:
- [ ] boolq - *weird download link*
- [ ] c4 - *beam dataset* | 23 | text: [Datasets ToDo-List] add datasets
## Description
This PR acts as a dashboard to see which datasets are added to the library and work.
Cicle-ci should always be green so that we can be sure that newly added datasets are functional.
This PR should not be merged.
## Progress
**For the following datasets the test commands**:
```
RUN_SLOW=1 pytest tests/test_dataset_common.py::DatasetTest::test_load_real_dataset_<your-dataset-name>
```
and
```
RUN_SLOW=1 pytest tests/test_dataset_common.py::DatasetTest::test_load_dataset_all_configs_<your-dataset-name>
```
**passes**.
- [x] Squad
- [x] Sentiment140
- [x] XNLI
- [x] Crime_and_Punish
- [x] movie_rationales
- [x] ai2_arc
- [x] anli
- [x] event2Mind
- [x] Fquad
- [x] blimp
- [x] empathetic_dialogues
- [x] cosmos_qa
- [x] xquad
- [x] blog_authorship_corpus
- [x] SNLI
- [x] break_data
- [x] SQuAD v2
- [x] cfq
- [x] eraser_multi_rc
- [x] Glue
- [x] Tydiqa
- [x] wiki_qa
- [x] wikitext
- [x] winogrande
- [x] wiqa
- [x] esnli
- [x] civil_comments
- [x] commonsense_qa
- [x] com_qa
- [x] coqa
- [x] wiki_split
- [x] cos_e
- [x] xcopa
- [x] quarel
- [x] quartz
- [x] squad_it
- [x] quoref
- [x] squad_pt
- [x] cornell_movie_dialog
- [x] SciQ
- [x] Scifact
- [x] hellaswag
- [x] ted_multi (in translate)
- [x] Aeslc (summarization)
- [x] drop
- [x] gap
- [x] hansard
- [x] opinosis
- [x] MLQA
- [x] math_dataset
## How-To-Add a dataset
**Before adding a dataset make sure that your branch is up to date**:
1. `git checkout add_datasets`
2. `git pull`
**Add a dataset via the `convert_dataset.sh` bash script:**
Running `bash convert_dataset.sh <file/to/tfds/datascript.py>` (*e.g.* `bash convert_dataset.sh ../tensorflow-datasets/tensorflow_datasets/text/movie_rationales.py`) will automatically run all the steps mentioned in **Add a dataset manually** below.
Make sure that you run `convert_dataset.sh` from the root folder of `nlp`.
The conversion script should work almost always for step 1): "convert dataset script from tfds to nlp format" and 2) "create checksum file" and step 3) "make style".
It can also sometimes automatically run step 4) "create the correct dummy data from tfds", but this will only work if a) there is either no config name or only one config name and b) the `tfds testing/test_data/fake_example` is in the correct form.
Nevertheless, the script should always be run in the beginning until an error occurs to be more efficient.
If the conversion script does not work or fails at some step, then you can run the steps manually as follows:
**Add a dataset manually**
Make sure you run all of the following commands from the root of your `nlp` git clone.
Also make sure that you changed to this branch:
```
git checkout add_datasets
```
1) the tfds datascript file should be converted to `nlp` style:
```
python nlp-cli convert --tfds_path <path/to/tensorflow_datasets/text/your_dataset_name>.py --nlp_directory datasets/nlp
```
This will convert the tdfs script and create a folder with the correct name.
2) the checksum file should be added. Use the command:
```
python nlp-cli test datasets/nlp/<your-dataset-folder> --save_checksums --all_configs
```
A checksums.txt file should be created in your folder and the structure should look as follows:
squad/
βββ squad.py/
βββ urls_checksums/
...........βββ checksums.txt
Delete the created `*.lock` file afterward - it should not be uploaded to AWS.
3) run black and isort on your newly added datascript files so that they look nice:
```
make style
```
4) the dummy data should be added. For this it might be useful to take a look into the structure of other examples as shown in the PR here and at `<path/to/tensorflow_datasets/testing/test_data/test_data/fake_examples>` whether the same data can be used.
5) the data can be uploaded to AWS using the command
```
aws s3 cp datasets/nlp/<your-dataset-folder> s3://datasets.huggingface.co/nlp/<your-dataset-folder> --recursive
```
6) check whether all works as expected using:
```
RUN_SLOW=1 pytest tests/test_dataset_common.py::DatasetTest::test_load_real_dataset_<your-dataset-name>
```
and
```
RUN_SLOW=1 pytest tests/test_dataset_common.py::DatasetTest::test_load_dataset_all_configs_<your-dataset-name>
```
7) push to this PR and rerun the circle ci workflow to check whether circle ci stays green.
8) Edit this commend and tick off your newly added dataset :-)
## TODO-list
Maybe we can add a TODO-list here for everybody that feels like adding new datasets so that we will not add the same datasets.
Here a link to available datasets: https://docs.google.com/spreadsheets/d/1zOtEqOrnVQwdgkC4nJrTY6d-Av02u0XFzeKAtBM2fUI/edit#gid=0
Patrick:
- [ ] boolq - *weird download link*
- [ ] c4 - *beam dataset*
https://github.com/huggingface/nlp/pull/15 - But it's probably best to checkout into this branch and look how the dummy data strtucture is for `squad` for example. |
https://github.com/huggingface/datasets/pull/37 | [Datasets ToDo-List] add datasets | > are lock files supposed to stay ?
Not sure! I think the checksum command creates them, so I just uploaded them as well. | ## Description
This PR acts as a dashboard to see which datasets are added to the library and work.
Cicle-ci should always be green so that we can be sure that newly added datasets are functional.
This PR should not be merged.
## Progress
**For the following datasets the test commands**:
```
RUN_SLOW=1 pytest tests/test_dataset_common.py::DatasetTest::test_load_real_dataset_<your-dataset-name>
```
and
```
RUN_SLOW=1 pytest tests/test_dataset_common.py::DatasetTest::test_load_dataset_all_configs_<your-dataset-name>
```
**passes**.
- [x] Squad
- [x] Sentiment140
- [x] XNLI
- [x] Crime_and_Punish
- [x] movie_rationales
- [x] ai2_arc
- [x] anli
- [x] event2Mind
- [x] Fquad
- [x] blimp
- [x] empathetic_dialogues
- [x] cosmos_qa
- [x] xquad
- [x] blog_authorship_corpus
- [x] SNLI
- [x] break_data
- [x] SQuAD v2
- [x] cfq
- [x] eraser_multi_rc
- [x] Glue
- [x] Tydiqa
- [x] wiki_qa
- [x] wikitext
- [x] winogrande
- [x] wiqa
- [x] esnli
- [x] civil_comments
- [x] commonsense_qa
- [x] com_qa
- [x] coqa
- [x] wiki_split
- [x] cos_e
- [x] xcopa
- [x] quarel
- [x] quartz
- [x] squad_it
- [x] quoref
- [x] squad_pt
- [x] cornell_movie_dialog
- [x] SciQ
- [x] Scifact
- [x] hellaswag
- [x] ted_multi (in translate)
- [x] Aeslc (summarization)
- [x] drop
- [x] gap
- [x] hansard
- [x] opinosis
- [x] MLQA
- [x] math_dataset
## How-To-Add a dataset
**Before adding a dataset make sure that your branch is up to date**:
1. `git checkout add_datasets`
2. `git pull`
**Add a dataset via the `convert_dataset.sh` bash script:**
Running `bash convert_dataset.sh <file/to/tfds/datascript.py>` (*e.g.* `bash convert_dataset.sh ../tensorflow-datasets/tensorflow_datasets/text/movie_rationales.py`) will automatically run all the steps mentioned in **Add a dataset manually** below.
Make sure that you run `convert_dataset.sh` from the root folder of `nlp`.
The conversion script should work almost always for step 1): "convert dataset script from tfds to nlp format" and 2) "create checksum file" and step 3) "make style".
It can also sometimes automatically run step 4) "create the correct dummy data from tfds", but this will only work if a) there is either no config name or only one config name and b) the `tfds testing/test_data/fake_example` is in the correct form.
Nevertheless, the script should always be run in the beginning until an error occurs to be more efficient.
If the conversion script does not work or fails at some step, then you can run the steps manually as follows:
**Add a dataset manually**
Make sure you run all of the following commands from the root of your `nlp` git clone.
Also make sure that you changed to this branch:
```
git checkout add_datasets
```
1) the tfds datascript file should be converted to `nlp` style:
```
python nlp-cli convert --tfds_path <path/to/tensorflow_datasets/text/your_dataset_name>.py --nlp_directory datasets/nlp
```
This will convert the tdfs script and create a folder with the correct name.
2) the checksum file should be added. Use the command:
```
python nlp-cli test datasets/nlp/<your-dataset-folder> --save_checksums --all_configs
```
A checksums.txt file should be created in your folder and the structure should look as follows:
squad/
βββ squad.py/
βββ urls_checksums/
...........βββ checksums.txt
Delete the created `*.lock` file afterward - it should not be uploaded to AWS.
3) run black and isort on your newly added datascript files so that they look nice:
```
make style
```
4) the dummy data should be added. For this it might be useful to take a look into the structure of other examples as shown in the PR here and at `<path/to/tensorflow_datasets/testing/test_data/test_data/fake_examples>` whether the same data can be used.
5) the data can be uploaded to AWS using the command
```
aws s3 cp datasets/nlp/<your-dataset-folder> s3://datasets.huggingface.co/nlp/<your-dataset-folder> --recursive
```
6) check whether all works as expected using:
```
RUN_SLOW=1 pytest tests/test_dataset_common.py::DatasetTest::test_load_real_dataset_<your-dataset-name>
```
and
```
RUN_SLOW=1 pytest tests/test_dataset_common.py::DatasetTest::test_load_dataset_all_configs_<your-dataset-name>
```
7) push to this PR and rerun the circle ci workflow to check whether circle ci stays green.
8) Edit this commend and tick off your newly added dataset :-)
## TODO-list
Maybe we can add a TODO-list here for everybody that feels like adding new datasets so that we will not add the same datasets.
Here a link to available datasets: https://docs.google.com/spreadsheets/d/1zOtEqOrnVQwdgkC4nJrTY6d-Av02u0XFzeKAtBM2fUI/edit#gid=0
Patrick:
- [ ] boolq - *weird download link*
- [ ] c4 - *beam dataset* | 24 | text: [Datasets ToDo-List] add datasets
## Description
This PR acts as a dashboard to see which datasets are added to the library and work.
Cicle-ci should always be green so that we can be sure that newly added datasets are functional.
This PR should not be merged.
## Progress
**For the following datasets the test commands**:
```
RUN_SLOW=1 pytest tests/test_dataset_common.py::DatasetTest::test_load_real_dataset_<your-dataset-name>
```
and
```
RUN_SLOW=1 pytest tests/test_dataset_common.py::DatasetTest::test_load_dataset_all_configs_<your-dataset-name>
```
**passes**.
- [x] Squad
- [x] Sentiment140
- [x] XNLI
- [x] Crime_and_Punish
- [x] movie_rationales
- [x] ai2_arc
- [x] anli
- [x] event2Mind
- [x] Fquad
- [x] blimp
- [x] empathetic_dialogues
- [x] cosmos_qa
- [x] xquad
- [x] blog_authorship_corpus
- [x] SNLI
- [x] break_data
- [x] SQuAD v2
- [x] cfq
- [x] eraser_multi_rc
- [x] Glue
- [x] Tydiqa
- [x] wiki_qa
- [x] wikitext
- [x] winogrande
- [x] wiqa
- [x] esnli
- [x] civil_comments
- [x] commonsense_qa
- [x] com_qa
- [x] coqa
- [x] wiki_split
- [x] cos_e
- [x] xcopa
- [x] quarel
- [x] quartz
- [x] squad_it
- [x] quoref
- [x] squad_pt
- [x] cornell_movie_dialog
- [x] SciQ
- [x] Scifact
- [x] hellaswag
- [x] ted_multi (in translate)
- [x] Aeslc (summarization)
- [x] drop
- [x] gap
- [x] hansard
- [x] opinosis
- [x] MLQA
- [x] math_dataset
## How-To-Add a dataset
**Before adding a dataset make sure that your branch is up to date**:
1. `git checkout add_datasets`
2. `git pull`
**Add a dataset via the `convert_dataset.sh` bash script:**
Running `bash convert_dataset.sh <file/to/tfds/datascript.py>` (*e.g.* `bash convert_dataset.sh ../tensorflow-datasets/tensorflow_datasets/text/movie_rationales.py`) will automatically run all the steps mentioned in **Add a dataset manually** below.
Make sure that you run `convert_dataset.sh` from the root folder of `nlp`.
The conversion script should work almost always for step 1): "convert dataset script from tfds to nlp format" and 2) "create checksum file" and step 3) "make style".
It can also sometimes automatically run step 4) "create the correct dummy data from tfds", but this will only work if a) there is either no config name or only one config name and b) the `tfds testing/test_data/fake_example` is in the correct form.
Nevertheless, the script should always be run in the beginning until an error occurs to be more efficient.
If the conversion script does not work or fails at some step, then you can run the steps manually as follows:
**Add a dataset manually**
Make sure you run all of the following commands from the root of your `nlp` git clone.
Also make sure that you changed to this branch:
```
git checkout add_datasets
```
1) the tfds datascript file should be converted to `nlp` style:
```
python nlp-cli convert --tfds_path <path/to/tensorflow_datasets/text/your_dataset_name>.py --nlp_directory datasets/nlp
```
This will convert the tdfs script and create a folder with the correct name.
2) the checksum file should be added. Use the command:
```
python nlp-cli test datasets/nlp/<your-dataset-folder> --save_checksums --all_configs
```
A checksums.txt file should be created in your folder and the structure should look as follows:
squad/
βββ squad.py/
βββ urls_checksums/
...........βββ checksums.txt
Delete the created `*.lock` file afterward - it should not be uploaded to AWS.
3) run black and isort on your newly added datascript files so that they look nice:
```
make style
```
4) the dummy data should be added. For this it might be useful to take a look into the structure of other examples as shown in the PR here and at `<path/to/tensorflow_datasets/testing/test_data/test_data/fake_examples>` whether the same data can be used.
5) the data can be uploaded to AWS using the command
```
aws s3 cp datasets/nlp/<your-dataset-folder> s3://datasets.huggingface.co/nlp/<your-dataset-folder> --recursive
```
6) check whether all works as expected using:
```
RUN_SLOW=1 pytest tests/test_dataset_common.py::DatasetTest::test_load_real_dataset_<your-dataset-name>
```
and
```
RUN_SLOW=1 pytest tests/test_dataset_common.py::DatasetTest::test_load_dataset_all_configs_<your-dataset-name>
```
7) push to this PR and rerun the circle ci workflow to check whether circle ci stays green.
8) Edit this commend and tick off your newly added dataset :-)
## TODO-list
Maybe we can add a TODO-list here for everybody that feels like adding new datasets so that we will not add the same datasets.
Here a link to available datasets: https://docs.google.com/spreadsheets/d/1zOtEqOrnVQwdgkC4nJrTY6d-Av02u0XFzeKAtBM2fUI/edit#gid=0
Patrick:
- [ ] boolq - *weird download link*
- [ ] c4 - *beam dataset*
> are lock files supposed to stay ?
Not sure! I think the checksum command creates them, so I just uploaded them as well. |
https://github.com/huggingface/datasets/pull/37 | [Datasets ToDo-List] add datasets | We can trash the `lock` file, they are dummy file that are only used to avoid concurrent access when the library is run.
You can read the filelock readme and code, it's a very simple single-file library: https://github.com/benediktschmitt/py-filelock | ## Description
This PR acts as a dashboard to see which datasets are added to the library and work.
Cicle-ci should always be green so that we can be sure that newly added datasets are functional.
This PR should not be merged.
## Progress
**For the following datasets the test commands**:
```
RUN_SLOW=1 pytest tests/test_dataset_common.py::DatasetTest::test_load_real_dataset_<your-dataset-name>
```
and
```
RUN_SLOW=1 pytest tests/test_dataset_common.py::DatasetTest::test_load_dataset_all_configs_<your-dataset-name>
```
**passes**.
- [x] Squad
- [x] Sentiment140
- [x] XNLI
- [x] Crime_and_Punish
- [x] movie_rationales
- [x] ai2_arc
- [x] anli
- [x] event2Mind
- [x] Fquad
- [x] blimp
- [x] empathetic_dialogues
- [x] cosmos_qa
- [x] xquad
- [x] blog_authorship_corpus
- [x] SNLI
- [x] break_data
- [x] SQuAD v2
- [x] cfq
- [x] eraser_multi_rc
- [x] Glue
- [x] Tydiqa
- [x] wiki_qa
- [x] wikitext
- [x] winogrande
- [x] wiqa
- [x] esnli
- [x] civil_comments
- [x] commonsense_qa
- [x] com_qa
- [x] coqa
- [x] wiki_split
- [x] cos_e
- [x] xcopa
- [x] quarel
- [x] quartz
- [x] squad_it
- [x] quoref
- [x] squad_pt
- [x] cornell_movie_dialog
- [x] SciQ
- [x] Scifact
- [x] hellaswag
- [x] ted_multi (in translate)
- [x] Aeslc (summarization)
- [x] drop
- [x] gap
- [x] hansard
- [x] opinosis
- [x] MLQA
- [x] math_dataset
## How-To-Add a dataset
**Before adding a dataset make sure that your branch is up to date**:
1. `git checkout add_datasets`
2. `git pull`
**Add a dataset via the `convert_dataset.sh` bash script:**
Running `bash convert_dataset.sh <file/to/tfds/datascript.py>` (*e.g.* `bash convert_dataset.sh ../tensorflow-datasets/tensorflow_datasets/text/movie_rationales.py`) will automatically run all the steps mentioned in **Add a dataset manually** below.
Make sure that you run `convert_dataset.sh` from the root folder of `nlp`.
The conversion script should work almost always for step 1): "convert dataset script from tfds to nlp format" and 2) "create checksum file" and step 3) "make style".
It can also sometimes automatically run step 4) "create the correct dummy data from tfds", but this will only work if a) there is either no config name or only one config name and b) the `tfds testing/test_data/fake_example` is in the correct form.
Nevertheless, the script should always be run in the beginning until an error occurs to be more efficient.
If the conversion script does not work or fails at some step, then you can run the steps manually as follows:
**Add a dataset manually**
Make sure you run all of the following commands from the root of your `nlp` git clone.
Also make sure that you changed to this branch:
```
git checkout add_datasets
```
1) the tfds datascript file should be converted to `nlp` style:
```
python nlp-cli convert --tfds_path <path/to/tensorflow_datasets/text/your_dataset_name>.py --nlp_directory datasets/nlp
```
This will convert the tdfs script and create a folder with the correct name.
2) the checksum file should be added. Use the command:
```
python nlp-cli test datasets/nlp/<your-dataset-folder> --save_checksums --all_configs
```
A checksums.txt file should be created in your folder and the structure should look as follows:
squad/
βββ squad.py/
βββ urls_checksums/
...........βββ checksums.txt
Delete the created `*.lock` file afterward - it should not be uploaded to AWS.
3) run black and isort on your newly added datascript files so that they look nice:
```
make style
```
4) the dummy data should be added. For this it might be useful to take a look into the structure of other examples as shown in the PR here and at `<path/to/tensorflow_datasets/testing/test_data/test_data/fake_examples>` whether the same data can be used.
5) the data can be uploaded to AWS using the command
```
aws s3 cp datasets/nlp/<your-dataset-folder> s3://datasets.huggingface.co/nlp/<your-dataset-folder> --recursive
```
6) check whether all works as expected using:
```
RUN_SLOW=1 pytest tests/test_dataset_common.py::DatasetTest::test_load_real_dataset_<your-dataset-name>
```
and
```
RUN_SLOW=1 pytest tests/test_dataset_common.py::DatasetTest::test_load_dataset_all_configs_<your-dataset-name>
```
7) push to this PR and rerun the circle ci workflow to check whether circle ci stays green.
8) Edit this commend and tick off your newly added dataset :-)
## TODO-list
Maybe we can add a TODO-list here for everybody that feels like adding new datasets so that we will not add the same datasets.
Here a link to available datasets: https://docs.google.com/spreadsheets/d/1zOtEqOrnVQwdgkC4nJrTY6d-Av02u0XFzeKAtBM2fUI/edit#gid=0
Patrick:
- [ ] boolq - *weird download link*
- [ ] c4 - *beam dataset* | 38 | text: [Datasets ToDo-List] add datasets
## Description
This PR acts as a dashboard to see which datasets are added to the library and work.
Cicle-ci should always be green so that we can be sure that newly added datasets are functional.
This PR should not be merged.
## Progress
**For the following datasets the test commands**:
```
RUN_SLOW=1 pytest tests/test_dataset_common.py::DatasetTest::test_load_real_dataset_<your-dataset-name>
```
and
```
RUN_SLOW=1 pytest tests/test_dataset_common.py::DatasetTest::test_load_dataset_all_configs_<your-dataset-name>
```
**passes**.
- [x] Squad
- [x] Sentiment140
- [x] XNLI
- [x] Crime_and_Punish
- [x] movie_rationales
- [x] ai2_arc
- [x] anli
- [x] event2Mind
- [x] Fquad
- [x] blimp
- [x] empathetic_dialogues
- [x] cosmos_qa
- [x] xquad
- [x] blog_authorship_corpus
- [x] SNLI
- [x] break_data
- [x] SQuAD v2
- [x] cfq
- [x] eraser_multi_rc
- [x] Glue
- [x] Tydiqa
- [x] wiki_qa
- [x] wikitext
- [x] winogrande
- [x] wiqa
- [x] esnli
- [x] civil_comments
- [x] commonsense_qa
- [x] com_qa
- [x] coqa
- [x] wiki_split
- [x] cos_e
- [x] xcopa
- [x] quarel
- [x] quartz
- [x] squad_it
- [x] quoref
- [x] squad_pt
- [x] cornell_movie_dialog
- [x] SciQ
- [x] Scifact
- [x] hellaswag
- [x] ted_multi (in translate)
- [x] Aeslc (summarization)
- [x] drop
- [x] gap
- [x] hansard
- [x] opinosis
- [x] MLQA
- [x] math_dataset
## How-To-Add a dataset
**Before adding a dataset make sure that your branch is up to date**:
1. `git checkout add_datasets`
2. `git pull`
**Add a dataset via the `convert_dataset.sh` bash script:**
Running `bash convert_dataset.sh <file/to/tfds/datascript.py>` (*e.g.* `bash convert_dataset.sh ../tensorflow-datasets/tensorflow_datasets/text/movie_rationales.py`) will automatically run all the steps mentioned in **Add a dataset manually** below.
Make sure that you run `convert_dataset.sh` from the root folder of `nlp`.
The conversion script should work almost always for step 1): "convert dataset script from tfds to nlp format" and 2) "create checksum file" and step 3) "make style".
It can also sometimes automatically run step 4) "create the correct dummy data from tfds", but this will only work if a) there is either no config name or only one config name and b) the `tfds testing/test_data/fake_example` is in the correct form.
Nevertheless, the script should always be run in the beginning until an error occurs to be more efficient.
If the conversion script does not work or fails at some step, then you can run the steps manually as follows:
**Add a dataset manually**
Make sure you run all of the following commands from the root of your `nlp` git clone.
Also make sure that you changed to this branch:
```
git checkout add_datasets
```
1) the tfds datascript file should be converted to `nlp` style:
```
python nlp-cli convert --tfds_path <path/to/tensorflow_datasets/text/your_dataset_name>.py --nlp_directory datasets/nlp
```
This will convert the tdfs script and create a folder with the correct name.
2) the checksum file should be added. Use the command:
```
python nlp-cli test datasets/nlp/<your-dataset-folder> --save_checksums --all_configs
```
A checksums.txt file should be created in your folder and the structure should look as follows:
squad/
βββ squad.py/
βββ urls_checksums/
...........βββ checksums.txt
Delete the created `*.lock` file afterward - it should not be uploaded to AWS.
3) run black and isort on your newly added datascript files so that they look nice:
```
make style
```
4) the dummy data should be added. For this it might be useful to take a look into the structure of other examples as shown in the PR here and at `<path/to/tensorflow_datasets/testing/test_data/test_data/fake_examples>` whether the same data can be used.
5) the data can be uploaded to AWS using the command
```
aws s3 cp datasets/nlp/<your-dataset-folder> s3://datasets.huggingface.co/nlp/<your-dataset-folder> --recursive
```
6) check whether all works as expected using:
```
RUN_SLOW=1 pytest tests/test_dataset_common.py::DatasetTest::test_load_real_dataset_<your-dataset-name>
```
and
```
RUN_SLOW=1 pytest tests/test_dataset_common.py::DatasetTest::test_load_dataset_all_configs_<your-dataset-name>
```
7) push to this PR and rerun the circle ci workflow to check whether circle ci stays green.
8) Edit this commend and tick off your newly added dataset :-)
## TODO-list
Maybe we can add a TODO-list here for everybody that feels like adding new datasets so that we will not add the same datasets.
Here a link to available datasets: https://docs.google.com/spreadsheets/d/1zOtEqOrnVQwdgkC4nJrTY6d-Av02u0XFzeKAtBM2fUI/edit#gid=0
Patrick:
- [ ] boolq - *weird download link*
- [ ] c4 - *beam dataset*
We can trash the `lock` file, they are dummy file that are only used to avoid concurrent access when the library is run.
You can read the filelock readme and code, it's a very simple single-file library: https://github.com/benediktschmitt/py-filelock |
https://github.com/huggingface/datasets/pull/37 | [Datasets ToDo-List] add datasets | The testing design was slightly changed as explained in https://github.com/huggingface/nlp/pull/51 .
If creating the dummy folder is too confusing it helps to upload everything else to AWS, then run the test and check the INFO when testing on how to create the dummy folder structure. | ## Description
This PR acts as a dashboard to see which datasets are added to the library and work.
Cicle-ci should always be green so that we can be sure that newly added datasets are functional.
This PR should not be merged.
## Progress
**For the following datasets the test commands**:
```
RUN_SLOW=1 pytest tests/test_dataset_common.py::DatasetTest::test_load_real_dataset_<your-dataset-name>
```
and
```
RUN_SLOW=1 pytest tests/test_dataset_common.py::DatasetTest::test_load_dataset_all_configs_<your-dataset-name>
```
**passes**.
- [x] Squad
- [x] Sentiment140
- [x] XNLI
- [x] Crime_and_Punish
- [x] movie_rationales
- [x] ai2_arc
- [x] anli
- [x] event2Mind
- [x] Fquad
- [x] blimp
- [x] empathetic_dialogues
- [x] cosmos_qa
- [x] xquad
- [x] blog_authorship_corpus
- [x] SNLI
- [x] break_data
- [x] SQuAD v2
- [x] cfq
- [x] eraser_multi_rc
- [x] Glue
- [x] Tydiqa
- [x] wiki_qa
- [x] wikitext
- [x] winogrande
- [x] wiqa
- [x] esnli
- [x] civil_comments
- [x] commonsense_qa
- [x] com_qa
- [x] coqa
- [x] wiki_split
- [x] cos_e
- [x] xcopa
- [x] quarel
- [x] quartz
- [x] squad_it
- [x] quoref
- [x] squad_pt
- [x] cornell_movie_dialog
- [x] SciQ
- [x] Scifact
- [x] hellaswag
- [x] ted_multi (in translate)
- [x] Aeslc (summarization)
- [x] drop
- [x] gap
- [x] hansard
- [x] opinosis
- [x] MLQA
- [x] math_dataset
## How-To-Add a dataset
**Before adding a dataset make sure that your branch is up to date**:
1. `git checkout add_datasets`
2. `git pull`
**Add a dataset via the `convert_dataset.sh` bash script:**
Running `bash convert_dataset.sh <file/to/tfds/datascript.py>` (*e.g.* `bash convert_dataset.sh ../tensorflow-datasets/tensorflow_datasets/text/movie_rationales.py`) will automatically run all the steps mentioned in **Add a dataset manually** below.
Make sure that you run `convert_dataset.sh` from the root folder of `nlp`.
The conversion script should work almost always for step 1): "convert dataset script from tfds to nlp format" and 2) "create checksum file" and step 3) "make style".
It can also sometimes automatically run step 4) "create the correct dummy data from tfds", but this will only work if a) there is either no config name or only one config name and b) the `tfds testing/test_data/fake_example` is in the correct form.
Nevertheless, the script should always be run in the beginning until an error occurs to be more efficient.
If the conversion script does not work or fails at some step, then you can run the steps manually as follows:
**Add a dataset manually**
Make sure you run all of the following commands from the root of your `nlp` git clone.
Also make sure that you changed to this branch:
```
git checkout add_datasets
```
1) the tfds datascript file should be converted to `nlp` style:
```
python nlp-cli convert --tfds_path <path/to/tensorflow_datasets/text/your_dataset_name>.py --nlp_directory datasets/nlp
```
This will convert the tdfs script and create a folder with the correct name.
2) the checksum file should be added. Use the command:
```
python nlp-cli test datasets/nlp/<your-dataset-folder> --save_checksums --all_configs
```
A checksums.txt file should be created in your folder and the structure should look as follows:
squad/
βββ squad.py/
βββ urls_checksums/
...........βββ checksums.txt
Delete the created `*.lock` file afterward - it should not be uploaded to AWS.
3) run black and isort on your newly added datascript files so that they look nice:
```
make style
```
4) the dummy data should be added. For this it might be useful to take a look into the structure of other examples as shown in the PR here and at `<path/to/tensorflow_datasets/testing/test_data/test_data/fake_examples>` whether the same data can be used.
5) the data can be uploaded to AWS using the command
```
aws s3 cp datasets/nlp/<your-dataset-folder> s3://datasets.huggingface.co/nlp/<your-dataset-folder> --recursive
```
6) check whether all works as expected using:
```
RUN_SLOW=1 pytest tests/test_dataset_common.py::DatasetTest::test_load_real_dataset_<your-dataset-name>
```
and
```
RUN_SLOW=1 pytest tests/test_dataset_common.py::DatasetTest::test_load_dataset_all_configs_<your-dataset-name>
```
7) push to this PR and rerun the circle ci workflow to check whether circle ci stays green.
8) Edit this commend and tick off your newly added dataset :-)
## TODO-list
Maybe we can add a TODO-list here for everybody that feels like adding new datasets so that we will not add the same datasets.
Here a link to available datasets: https://docs.google.com/spreadsheets/d/1zOtEqOrnVQwdgkC4nJrTY6d-Av02u0XFzeKAtBM2fUI/edit#gid=0
Patrick:
- [ ] boolq - *weird download link*
- [ ] c4 - *beam dataset* | 45 | text: [Datasets ToDo-List] add datasets
## Description
This PR acts as a dashboard to see which datasets are added to the library and work.
Cicle-ci should always be green so that we can be sure that newly added datasets are functional.
This PR should not be merged.
## Progress
**For the following datasets the test commands**:
```
RUN_SLOW=1 pytest tests/test_dataset_common.py::DatasetTest::test_load_real_dataset_<your-dataset-name>
```
and
```
RUN_SLOW=1 pytest tests/test_dataset_common.py::DatasetTest::test_load_dataset_all_configs_<your-dataset-name>
```
**passes**.
- [x] Squad
- [x] Sentiment140
- [x] XNLI
- [x] Crime_and_Punish
- [x] movie_rationales
- [x] ai2_arc
- [x] anli
- [x] event2Mind
- [x] Fquad
- [x] blimp
- [x] empathetic_dialogues
- [x] cosmos_qa
- [x] xquad
- [x] blog_authorship_corpus
- [x] SNLI
- [x] break_data
- [x] SQuAD v2
- [x] cfq
- [x] eraser_multi_rc
- [x] Glue
- [x] Tydiqa
- [x] wiki_qa
- [x] wikitext
- [x] winogrande
- [x] wiqa
- [x] esnli
- [x] civil_comments
- [x] commonsense_qa
- [x] com_qa
- [x] coqa
- [x] wiki_split
- [x] cos_e
- [x] xcopa
- [x] quarel
- [x] quartz
- [x] squad_it
- [x] quoref
- [x] squad_pt
- [x] cornell_movie_dialog
- [x] SciQ
- [x] Scifact
- [x] hellaswag
- [x] ted_multi (in translate)
- [x] Aeslc (summarization)
- [x] drop
- [x] gap
- [x] hansard
- [x] opinosis
- [x] MLQA
- [x] math_dataset
## How-To-Add a dataset
**Before adding a dataset make sure that your branch is up to date**:
1. `git checkout add_datasets`
2. `git pull`
**Add a dataset via the `convert_dataset.sh` bash script:**
Running `bash convert_dataset.sh <file/to/tfds/datascript.py>` (*e.g.* `bash convert_dataset.sh ../tensorflow-datasets/tensorflow_datasets/text/movie_rationales.py`) will automatically run all the steps mentioned in **Add a dataset manually** below.
Make sure that you run `convert_dataset.sh` from the root folder of `nlp`.
The conversion script should work almost always for step 1): "convert dataset script from tfds to nlp format" and 2) "create checksum file" and step 3) "make style".
It can also sometimes automatically run step 4) "create the correct dummy data from tfds", but this will only work if a) there is either no config name or only one config name and b) the `tfds testing/test_data/fake_example` is in the correct form.
Nevertheless, the script should always be run in the beginning until an error occurs to be more efficient.
If the conversion script does not work or fails at some step, then you can run the steps manually as follows:
**Add a dataset manually**
Make sure you run all of the following commands from the root of your `nlp` git clone.
Also make sure that you changed to this branch:
```
git checkout add_datasets
```
1) the tfds datascript file should be converted to `nlp` style:
```
python nlp-cli convert --tfds_path <path/to/tensorflow_datasets/text/your_dataset_name>.py --nlp_directory datasets/nlp
```
This will convert the tdfs script and create a folder with the correct name.
2) the checksum file should be added. Use the command:
```
python nlp-cli test datasets/nlp/<your-dataset-folder> --save_checksums --all_configs
```
A checksums.txt file should be created in your folder and the structure should look as follows:
squad/
βββ squad.py/
βββ urls_checksums/
...........βββ checksums.txt
Delete the created `*.lock` file afterward - it should not be uploaded to AWS.
3) run black and isort on your newly added datascript files so that they look nice:
```
make style
```
4) the dummy data should be added. For this it might be useful to take a look into the structure of other examples as shown in the PR here and at `<path/to/tensorflow_datasets/testing/test_data/test_data/fake_examples>` whether the same data can be used.
5) the data can be uploaded to AWS using the command
```
aws s3 cp datasets/nlp/<your-dataset-folder> s3://datasets.huggingface.co/nlp/<your-dataset-folder> --recursive
```
6) check whether all works as expected using:
```
RUN_SLOW=1 pytest tests/test_dataset_common.py::DatasetTest::test_load_real_dataset_<your-dataset-name>
```
and
```
RUN_SLOW=1 pytest tests/test_dataset_common.py::DatasetTest::test_load_dataset_all_configs_<your-dataset-name>
```
7) push to this PR and rerun the circle ci workflow to check whether circle ci stays green.
8) Edit this commend and tick off your newly added dataset :-)
## TODO-list
Maybe we can add a TODO-list here for everybody that feels like adding new datasets so that we will not add the same datasets.
Here a link to available datasets: https://docs.google.com/spreadsheets/d/1zOtEqOrnVQwdgkC4nJrTY6d-Av02u0XFzeKAtBM2fUI/edit#gid=0
Patrick:
- [ ] boolq - *weird download link*
- [ ] c4 - *beam dataset*
The testing design was slightly changed as explained in https://github.com/huggingface/nlp/pull/51 .
If creating the dummy folder is too confusing it helps to upload everything else to AWS, then run the test and check the INFO when testing on how to create the dummy folder structure. |
https://github.com/huggingface/datasets/pull/36 | Metrics - refactoring, adding support for download and distributed metrics | > Really cool, I love it! I would just raise a tiny point, the distributive version of the metrics might not work properly with TF because it is a different way to do, why not to add a "framework" detection and raise warning when TF is used, saying something like "not available yet in TF switch to non distributive metric computation".
>
> What do you think?
Good point @jplu I'm not sure how you should do distributed metrics evaluation for TF.
There is only one python script, right?
Maybe it's just the same as in the not-distributed case? | Refactoring metrics to have a similar loading API than the datasets and improving the import system.
# Import system
The import system has ben upgraded. There are now three types of imports allowed:
1. `library` imports (identified as "absolute imports")
```python
import seqeval
```
=> we'll test all the imports before running the scripts and if one cannot be imported we'll display an error message like this one:
`ImportError: To be able to use this metric/dataset, you need to install the following dependencies ['seqeval'] using 'pip install seqeval' for instance'`
2. `internal` imports (identified as "relative imports")
```python
import .c4_utils
```
=> we'll assume this point to a file in the same directory/S3-directory as the main script and download this file.
2. `external` imports (identified as "relative imports" with a comment starting with `# From:`)
```python
from .nmt_bleu import compute_bleu # From: https://github.com/tensorflow/nmt/blob/master/nmt/scripts/bleu.py
```
=> we'll assume this point to the URL of a python script (if it's a link to a github file, we'll take the raw file automatically).
=> the script is downloaded and renamed to the import name (here above renamed from `bleu.py` to `nmt_bleu.py`). Renaming the file can be necessary if the distant file has the same name as the dataset/metric processing script. If you forgot to rename the distant script and it has the same name as the dataset/metric, you'll have an explicit error message asking to rename the import anyway.
# Hosting metrics
Metrics are hosted on a S3 bucket like the dataset processing scripts.
# Metrics scripts
Metrics scripts have a lot in common with datasets processing scripts. They also have a `metric.info` including citations, descriptions and links to relevant pages.
Metrics have more documentation to supply to ensure they are used well.
Four examples are already included for reference in [./metrics](./metrics): BLEU, ROUGE, SacreBLEU and SeqEVAL.
# Automatic support for distributed/multi-processing metric computation
We've also added support for automatic distributed/multi-processing metric computation (e.g. when using DistributedDataParallel). We leverage our own dataset format for smart caching in this case.
Here is a quick gist of a standard use of metrics (the simplest usage):
```python
import nlp
bleu_metric = nlp.load_metric('bleu')
# If you only have a single iteration, you can easily compute the score like this
predictions = model(inputs)
score = bleu_metric.compute(predictions, references)
# If you have a loop, you can "add" your predictions and references at each iteration instead of having to save them yourself (the metric object store them efficiently for you)
for batch in dataloader:
model_input, targets = batch
predictions = model(model_inputs)
bleu.add(predictions, targets)
score = bleu_metric.compute() # Compute the score from all the stored predictions/references
```
Here is a quick gist of a use in a distributed torch setup (should work for any python multi-process setup actually). It's pretty much identical to the second example above:
```python
import nlp
# You need to give the total number of parallel python processes (num_process) and the id of each process (process_id)
bleu = nlp.load_metric('bleu', process_id=torch.distributed.get_rank(),b num_process=torch.distributed.get_world_size())
for batch in dataloader:
model_input, targets = batch
predictions = model(model_inputs)
bleu.add(predictions, targets)
score = bleu_metric.compute() # Compute the score on the first node by default (can be set to compute on each node as well)
``` | 99 | text: Metrics - refactoring, adding support for download and distributed metrics
Refactoring metrics to have a similar loading API than the datasets and improving the import system.
# Import system
The import system has ben upgraded. There are now three types of imports allowed:
1. `library` imports (identified as "absolute imports")
```python
import seqeval
```
=> we'll test all the imports before running the scripts and if one cannot be imported we'll display an error message like this one:
`ImportError: To be able to use this metric/dataset, you need to install the following dependencies ['seqeval'] using 'pip install seqeval' for instance'`
2. `internal` imports (identified as "relative imports")
```python
import .c4_utils
```
=> we'll assume this point to a file in the same directory/S3-directory as the main script and download this file.
2. `external` imports (identified as "relative imports" with a comment starting with `# From:`)
```python
from .nmt_bleu import compute_bleu # From: https://github.com/tensorflow/nmt/blob/master/nmt/scripts/bleu.py
```
=> we'll assume this point to the URL of a python script (if it's a link to a github file, we'll take the raw file automatically).
=> the script is downloaded and renamed to the import name (here above renamed from `bleu.py` to `nmt_bleu.py`). Renaming the file can be necessary if the distant file has the same name as the dataset/metric processing script. If you forgot to rename the distant script and it has the same name as the dataset/metric, you'll have an explicit error message asking to rename the import anyway.
# Hosting metrics
Metrics are hosted on a S3 bucket like the dataset processing scripts.
# Metrics scripts
Metrics scripts have a lot in common with datasets processing scripts. They also have a `metric.info` including citations, descriptions and links to relevant pages.
Metrics have more documentation to supply to ensure they are used well.
Four examples are already included for reference in [./metrics](./metrics): BLEU, ROUGE, SacreBLEU and SeqEVAL.
# Automatic support for distributed/multi-processing metric computation
We've also added support for automatic distributed/multi-processing metric computation (e.g. when using DistributedDataParallel). We leverage our own dataset format for smart caching in this case.
Here is a quick gist of a standard use of metrics (the simplest usage):
```python
import nlp
bleu_metric = nlp.load_metric('bleu')
# If you only have a single iteration, you can easily compute the score like this
predictions = model(inputs)
score = bleu_metric.compute(predictions, references)
# If you have a loop, you can "add" your predictions and references at each iteration instead of having to save them yourself (the metric object store them efficiently for you)
for batch in dataloader:
model_input, targets = batch
predictions = model(model_inputs)
bleu.add(predictions, targets)
score = bleu_metric.compute() # Compute the score from all the stored predictions/references
```
Here is a quick gist of a use in a distributed torch setup (should work for any python multi-process setup actually). It's pretty much identical to the second example above:
```python
import nlp
# You need to give the total number of parallel python processes (num_process) and the id of each process (process_id)
bleu = nlp.load_metric('bleu', process_id=torch.distributed.get_rank(),b num_process=torch.distributed.get_world_size())
for batch in dataloader:
model_input, targets = batch
predictions = model(model_inputs)
bleu.add(predictions, targets)
score = bleu_metric.compute() # Compute the score on the first node by default (can be set to compute on each node as well)
```
> Really cool, I love it! I would just raise a tiny point, the distributive version of the metrics might not work properly with TF because it is a different way to do, why not to add a "framework" detection and raise warning when TF is used, saying something like "not available yet in TF switch to non distributive metric computation".
>
> What do you think?
Good point @jplu I'm not sure how you should do distributed metrics evaluation for TF.
There is only one python script, right?
Maybe it's just the same as in the not-distributed case? |
https://github.com/huggingface/datasets/pull/36 | Metrics - refactoring, adding support for download and distributed metrics | I think non-distributed case should work in TF for both cases indeed, but this needs to be tested. | Refactoring metrics to have a similar loading API than the datasets and improving the import system.
# Import system
The import system has ben upgraded. There are now three types of imports allowed:
1. `library` imports (identified as "absolute imports")
```python
import seqeval
```
=> we'll test all the imports before running the scripts and if one cannot be imported we'll display an error message like this one:
`ImportError: To be able to use this metric/dataset, you need to install the following dependencies ['seqeval'] using 'pip install seqeval' for instance'`
2. `internal` imports (identified as "relative imports")
```python
import .c4_utils
```
=> we'll assume this point to a file in the same directory/S3-directory as the main script and download this file.
2. `external` imports (identified as "relative imports" with a comment starting with `# From:`)
```python
from .nmt_bleu import compute_bleu # From: https://github.com/tensorflow/nmt/blob/master/nmt/scripts/bleu.py
```
=> we'll assume this point to the URL of a python script (if it's a link to a github file, we'll take the raw file automatically).
=> the script is downloaded and renamed to the import name (here above renamed from `bleu.py` to `nmt_bleu.py`). Renaming the file can be necessary if the distant file has the same name as the dataset/metric processing script. If you forgot to rename the distant script and it has the same name as the dataset/metric, you'll have an explicit error message asking to rename the import anyway.
# Hosting metrics
Metrics are hosted on a S3 bucket like the dataset processing scripts.
# Metrics scripts
Metrics scripts have a lot in common with datasets processing scripts. They also have a `metric.info` including citations, descriptions and links to relevant pages.
Metrics have more documentation to supply to ensure they are used well.
Four examples are already included for reference in [./metrics](./metrics): BLEU, ROUGE, SacreBLEU and SeqEVAL.
# Automatic support for distributed/multi-processing metric computation
We've also added support for automatic distributed/multi-processing metric computation (e.g. when using DistributedDataParallel). We leverage our own dataset format for smart caching in this case.
Here is a quick gist of a standard use of metrics (the simplest usage):
```python
import nlp
bleu_metric = nlp.load_metric('bleu')
# If you only have a single iteration, you can easily compute the score like this
predictions = model(inputs)
score = bleu_metric.compute(predictions, references)
# If you have a loop, you can "add" your predictions and references at each iteration instead of having to save them yourself (the metric object store them efficiently for you)
for batch in dataloader:
model_input, targets = batch
predictions = model(model_inputs)
bleu.add(predictions, targets)
score = bleu_metric.compute() # Compute the score from all the stored predictions/references
```
Here is a quick gist of a use in a distributed torch setup (should work for any python multi-process setup actually). It's pretty much identical to the second example above:
```python
import nlp
# You need to give the total number of parallel python processes (num_process) and the id of each process (process_id)
bleu = nlp.load_metric('bleu', process_id=torch.distributed.get_rank(),b num_process=torch.distributed.get_world_size())
for batch in dataloader:
model_input, targets = batch
predictions = model(model_inputs)
bleu.add(predictions, targets)
score = bleu_metric.compute() # Compute the score on the first node by default (can be set to compute on each node as well)
``` | 18 | text: Metrics - refactoring, adding support for download and distributed metrics
Refactoring metrics to have a similar loading API than the datasets and improving the import system.
# Import system
The import system has ben upgraded. There are now three types of imports allowed:
1. `library` imports (identified as "absolute imports")
```python
import seqeval
```
=> we'll test all the imports before running the scripts and if one cannot be imported we'll display an error message like this one:
`ImportError: To be able to use this metric/dataset, you need to install the following dependencies ['seqeval'] using 'pip install seqeval' for instance'`
2. `internal` imports (identified as "relative imports")
```python
import .c4_utils
```
=> we'll assume this point to a file in the same directory/S3-directory as the main script and download this file.
2. `external` imports (identified as "relative imports" with a comment starting with `# From:`)
```python
from .nmt_bleu import compute_bleu # From: https://github.com/tensorflow/nmt/blob/master/nmt/scripts/bleu.py
```
=> we'll assume this point to the URL of a python script (if it's a link to a github file, we'll take the raw file automatically).
=> the script is downloaded and renamed to the import name (here above renamed from `bleu.py` to `nmt_bleu.py`). Renaming the file can be necessary if the distant file has the same name as the dataset/metric processing script. If you forgot to rename the distant script and it has the same name as the dataset/metric, you'll have an explicit error message asking to rename the import anyway.
# Hosting metrics
Metrics are hosted on a S3 bucket like the dataset processing scripts.
# Metrics scripts
Metrics scripts have a lot in common with datasets processing scripts. They also have a `metric.info` including citations, descriptions and links to relevant pages.
Metrics have more documentation to supply to ensure they are used well.
Four examples are already included for reference in [./metrics](./metrics): BLEU, ROUGE, SacreBLEU and SeqEVAL.
# Automatic support for distributed/multi-processing metric computation
We've also added support for automatic distributed/multi-processing metric computation (e.g. when using DistributedDataParallel). We leverage our own dataset format for smart caching in this case.
Here is a quick gist of a standard use of metrics (the simplest usage):
```python
import nlp
bleu_metric = nlp.load_metric('bleu')
# If you only have a single iteration, you can easily compute the score like this
predictions = model(inputs)
score = bleu_metric.compute(predictions, references)
# If you have a loop, you can "add" your predictions and references at each iteration instead of having to save them yourself (the metric object store them efficiently for you)
for batch in dataloader:
model_input, targets = batch
predictions = model(model_inputs)
bleu.add(predictions, targets)
score = bleu_metric.compute() # Compute the score from all the stored predictions/references
```
Here is a quick gist of a use in a distributed torch setup (should work for any python multi-process setup actually). It's pretty much identical to the second example above:
```python
import nlp
# You need to give the total number of parallel python processes (num_process) and the id of each process (process_id)
bleu = nlp.load_metric('bleu', process_id=torch.distributed.get_rank(),b num_process=torch.distributed.get_world_size())
for batch in dataloader:
model_input, targets = batch
predictions = model(model_inputs)
bleu.add(predictions, targets)
score = bleu_metric.compute() # Compute the score on the first node by default (can be set to compute on each node as well)
```
I think non-distributed case should work in TF for both cases indeed, but this needs to be tested. |
https://github.com/huggingface/datasets/pull/33 | Big cleanup/refactoring for clean serialization | Great! I think when this merged, we can merge sure that Circle Ci stays happy when uploading new datasets. | This PR cleans many base classes to re-build them as `dataclasses`. We can thus use a simple serialization workflow for `DatasetInfo`, including it's `Features` and `SplitDict` based on `dataclasses` `asdict()`.
The resulting code is a lot shorter, can be easily serialized/deserialized, dataset info are human-readable and we can get rid of the `dataclass_json` dependency.
The scripts have breaking changes and the conversion tool is updated.
Example of dataset info in SQuAD script now:
```python
def _info(self):
return nlp.DatasetInfo(
description=_DESCRIPTION,
features=nlp.Features({
"id":
nlp.Value('string'),
"title":
nlp.Value('string'),
"context":
nlp.Value('string'),
"question":
nlp.Value('string'),
"answers":
nlp.Sequence({
"text": nlp.Value('string'),
"answer_start": nlp.Value('int32'),
}),
}),
# No default supervised_keys (as we have to pass both question
# and context as input).
supervised_keys=None,
homepage="https://rajpurkar.github.io/SQuAD-explorer/",
citation=_CITATION,
)
```
Example of serialized dataset info:
```bash
{
"description": "Stanford Question Answering Dataset (SQuAD) is a reading comprehension dataset, consisting of questions posed by crowdworkers on a set of Wikipedia articles, where the answer to every question is a segment of text, or span, from the corresponding reading passage, or the question might be unanswerable.\n",
"citation": "@article{2016arXiv160605250R,\n author = {{Rajpurkar}, Pranav and {Zhang}, Jian and {Lopyrev},\n Konstantin and {Liang}, Percy},\n title = \"{SQuAD: 100,000+ Questions for Machine Comprehension of Text}\",\n journal = {arXiv e-prints},\n year = 2016,\n eid = {arXiv:1606.05250},\n pages = {arXiv:1606.05250},\narchivePrefix = {arXiv},\n eprint = {1606.05250},\n}\n",
"homepage": "https://rajpurkar.github.io/SQuAD-explorer/",
"license": "",
"features": {
"id": {
"dtype": "string",
"_type": "Value"
},
"title": {
"dtype": "string",
"_type": "Value"
},
"context": {
"dtype": "string",
"_type": "Value"
},
"question": {
"dtype": "string",
"_type": "Value"
},
"answers": {
"feature": {
"text": {
"dtype": "string",
"_type": "Value"
},
"answer_start": {
"dtype": "int32",
"_type": "Value"
}
},
"length": -1,
"_type": "Sequence"
}
},
"supervised_keys": null,
"name": "squad",
"version": {
"version_str": "1.0.0",
"description": "New split API (https://tensorflow.org/datasets/splits)",
"nlp_version_to_prepare": null,
"major": 1,
"minor": 0,
"patch": 0
},
"splits": {
"train": {
"name": "train",
"num_bytes": 79426386,
"num_examples": 87599,
"dataset_name": "squad"
},
"validation": {
"name": "validation",
"num_bytes": 10491883,
"num_examples": 10570,
"dataset_name": "squad"
}
},
"size_in_bytes": 0,
"download_size": 35142551,
"download_checksums": []
}
``` | 19 | text: Big cleanup/refactoring for clean serialization
This PR cleans many base classes to re-build them as `dataclasses`. We can thus use a simple serialization workflow for `DatasetInfo`, including it's `Features` and `SplitDict` based on `dataclasses` `asdict()`.
The resulting code is a lot shorter, can be easily serialized/deserialized, dataset info are human-readable and we can get rid of the `dataclass_json` dependency.
The scripts have breaking changes and the conversion tool is updated.
Example of dataset info in SQuAD script now:
```python
def _info(self):
return nlp.DatasetInfo(
description=_DESCRIPTION,
features=nlp.Features({
"id":
nlp.Value('string'),
"title":
nlp.Value('string'),
"context":
nlp.Value('string'),
"question":
nlp.Value('string'),
"answers":
nlp.Sequence({
"text": nlp.Value('string'),
"answer_start": nlp.Value('int32'),
}),
}),
# No default supervised_keys (as we have to pass both question
# and context as input).
supervised_keys=None,
homepage="https://rajpurkar.github.io/SQuAD-explorer/",
citation=_CITATION,
)
```
Example of serialized dataset info:
```bash
{
"description": "Stanford Question Answering Dataset (SQuAD) is a reading comprehension dataset, consisting of questions posed by crowdworkers on a set of Wikipedia articles, where the answer to every question is a segment of text, or span, from the corresponding reading passage, or the question might be unanswerable.\n",
"citation": "@article{2016arXiv160605250R,\n author = {{Rajpurkar}, Pranav and {Zhang}, Jian and {Lopyrev},\n Konstantin and {Liang}, Percy},\n title = \"{SQuAD: 100,000+ Questions for Machine Comprehension of Text}\",\n journal = {arXiv e-prints},\n year = 2016,\n eid = {arXiv:1606.05250},\n pages = {arXiv:1606.05250},\narchivePrefix = {arXiv},\n eprint = {1606.05250},\n}\n",
"homepage": "https://rajpurkar.github.io/SQuAD-explorer/",
"license": "",
"features": {
"id": {
"dtype": "string",
"_type": "Value"
},
"title": {
"dtype": "string",
"_type": "Value"
},
"context": {
"dtype": "string",
"_type": "Value"
},
"question": {
"dtype": "string",
"_type": "Value"
},
"answers": {
"feature": {
"text": {
"dtype": "string",
"_type": "Value"
},
"answer_start": {
"dtype": "int32",
"_type": "Value"
}
},
"length": -1,
"_type": "Sequence"
}
},
"supervised_keys": null,
"name": "squad",
"version": {
"version_str": "1.0.0",
"description": "New split API (https://tensorflow.org/datasets/splits)",
"nlp_version_to_prepare": null,
"major": 1,
"minor": 0,
"patch": 0
},
"splits": {
"train": {
"name": "train",
"num_bytes": 79426386,
"num_examples": 87599,
"dataset_name": "squad"
},
"validation": {
"name": "validation",
"num_bytes": 10491883,
"num_examples": 10570,
"dataset_name": "squad"
}
},
"size_in_bytes": 0,
"download_size": 35142551,
"download_checksums": []
}
```
Great! I think when this merged, we can merge sure that Circle Ci stays happy when uploading new datasets. |
https://github.com/huggingface/datasets/pull/25 | Add script csv datasets | Ok here is a proposal for a more general API and workflow.
# New `ArrowBasedBuilder`
For all the formats that can be directly and efficiently loaded by Arrow (CSV, JSON, Parquet, Arrow), we don't really want to have to go through a conversion to python and back to Arrow. This new builder has a `_generate_tables` method to yield `Arrow.Tables` instead of single examples.
The tables can be directly casted in Arrow so it's not necessary to supply `Features`, they can be deduced from the `Table` column.
# Central role of the `BuilderConfig` to store all the arguments necessary for the Dataset creation.
`BuilderConfig` provide a few defaults fields `name`, `version`, `description`, `data_files` and `data_dir` which can be used to store values necessary for the creation of the dataset. It can be freely extended to store additional information (see the example for `CsvConfig`).
On the contrary, `DatasetInfo` is designed as an organized and delimited information storage class with predefined fields.
`DatasetInfo` now store two names:
- `builder_name`: Name of the builder script used to create the dataset
- `config_name`: Name of the configuration used to create the dataset.
# Refactoring `load()` arguments and all the chain of processing including the `DownloadManager`
`load()` now accept a selection of arguments which are used to update the `BuilderConfig` and some kwargs which are used to handle the download process.
Supplying a `BuilderConfig` as `config` will override the config provided in the dataset. Supplying a `str` will get the associated config from the dataset. Default is to fetch the first config of the dataset.
Giving additional arguments to `load()` will override the arguments in the `BuilderConfig`.
# CSV script
The `csv.py` script is provided as an example, usage is:
```python
bbc = nlp.load('/Users/thomwolf/Documents/GitHub/datasets/datasets/nlp/csv',
name='bbc',
version="1.0.1",
split='train',
data_files={'train': ['/Users/thomwolf/Documents/GitHub/datasets/datasets/dummy_data/csv/test.csv']},
skip_rows=10,
download_mode='force_redownload')
```
# Checksums
We now don't raise an error if the checksum file is not found.
# `DownloadConfig`
We now have a download configuration class to handle all the specific arguments for file caching like proxies, using only local files or user-agents. | This is a PR allowing to create datasets from local CSV files. A usage might be:
```python
import nlp
ds = nlp.load(
path="csv",
name="bbc",
dataset_files={
nlp.Split.TRAIN: ["datasets/dummy_data/csv/train.csv"],
nlp.Split.TEST: [""datasets/dummy_data/csv/test.csv""]
},
csv_kwargs={
"skip_rows": 0,
"delimiter": ",",
"quote_char": "\"",
"header_as_column_names": True
}
)
```
```
Downloading and preparing dataset bbc/1.0.0 (download: Unknown size, generated: Unknown size, total: Unknown size) to /home/jplu/.cache/huggingface/datasets/bbc/1.0.0...
Dataset bbc downloaded and prepared to /home/jplu/.cache/huggingface/datasets/bbc/1.0.0. Subsequent calls will reuse this data.
{'test': Dataset(schema: {'category': 'string', 'text': 'string'}, num_rows: 49), 'train': Dataset(schema: {'category': 'string', 'text': 'string'}, num_rows: 99), 'validation': Dataset(schema: {'category': 'string', 'text': 'string'}, num_rows: 0)}
```
How it is read:
- `path`: the `csv` word means "I want to create a CSV dataset"
- `name`: the name of this dataset is `bbc`
- `dataset_files`: this is a dictionary where each key is the list of files corresponding to the key split.
- `csv_kwargs`: this is the keywords arguments to "explain" how to read the CSV files
* `skip_rows`: number of rows have to be skipped, starting from the beginning of the file
* `delimiter`: which delimiter is used to separate the columns
* `quote_char`: which quote char is used to represent a column where the delimiter appears in one of them
* `header_as_column_names`: will use the first row (header) of the file as name for the features. Otherwise the names will be automatically generated as `f1`, `f2`, etc... Will be applied after the `skip_rows` parameter.
**TODO**: for now the `csv.py` is copied each time we create a new dataset as `ds_name.py`, this behavior will be modified to have only the `csv.py` script copied only once and not for all the CSV datasets. | 337 | text: Add script csv datasets
This is a PR allowing to create datasets from local CSV files. A usage might be:
```python
import nlp
ds = nlp.load(
path="csv",
name="bbc",
dataset_files={
nlp.Split.TRAIN: ["datasets/dummy_data/csv/train.csv"],
nlp.Split.TEST: [""datasets/dummy_data/csv/test.csv""]
},
csv_kwargs={
"skip_rows": 0,
"delimiter": ",",
"quote_char": "\"",
"header_as_column_names": True
}
)
```
```
Downloading and preparing dataset bbc/1.0.0 (download: Unknown size, generated: Unknown size, total: Unknown size) to /home/jplu/.cache/huggingface/datasets/bbc/1.0.0...
Dataset bbc downloaded and prepared to /home/jplu/.cache/huggingface/datasets/bbc/1.0.0. Subsequent calls will reuse this data.
{'test': Dataset(schema: {'category': 'string', 'text': 'string'}, num_rows: 49), 'train': Dataset(schema: {'category': 'string', 'text': 'string'}, num_rows: 99), 'validation': Dataset(schema: {'category': 'string', 'text': 'string'}, num_rows: 0)}
```
How it is read:
- `path`: the `csv` word means "I want to create a CSV dataset"
- `name`: the name of this dataset is `bbc`
- `dataset_files`: this is a dictionary where each key is the list of files corresponding to the key split.
- `csv_kwargs`: this is the keywords arguments to "explain" how to read the CSV files
* `skip_rows`: number of rows have to be skipped, starting from the beginning of the file
* `delimiter`: which delimiter is used to separate the columns
* `quote_char`: which quote char is used to represent a column where the delimiter appears in one of them
* `header_as_column_names`: will use the first row (header) of the file as name for the features. Otherwise the names will be automatically generated as `f1`, `f2`, etc... Will be applied after the `skip_rows` parameter.
**TODO**: for now the `csv.py` is copied each time we create a new dataset as `ds_name.py`, this behavior will be modified to have only the `csv.py` script copied only once and not for all the CSV datasets.
Ok here is a proposal for a more general API and workflow.
# New `ArrowBasedBuilder`
For all the formats that can be directly and efficiently loaded by Arrow (CSV, JSON, Parquet, Arrow), we don't really want to have to go through a conversion to python and back to Arrow. This new builder has a `_generate_tables` method to yield `Arrow.Tables` instead of single examples.
The tables can be directly casted in Arrow so it's not necessary to supply `Features`, they can be deduced from the `Table` column.
# Central role of the `BuilderConfig` to store all the arguments necessary for the Dataset creation.
`BuilderConfig` provide a few defaults fields `name`, `version`, `description`, `data_files` and `data_dir` which can be used to store values necessary for the creation of the dataset. It can be freely extended to store additional information (see the example for `CsvConfig`).
On the contrary, `DatasetInfo` is designed as an organized and delimited information storage class with predefined fields.
`DatasetInfo` now store two names:
- `builder_name`: Name of the builder script used to create the dataset
- `config_name`: Name of the configuration used to create the dataset.
# Refactoring `load()` arguments and all the chain of processing including the `DownloadManager`
`load()` now accept a selection of arguments which are used to update the `BuilderConfig` and some kwargs which are used to handle the download process.
Supplying a `BuilderConfig` as `config` will override the config provided in the dataset. Supplying a `str` will get the associated config from the dataset. Default is to fetch the first config of the dataset.
Giving additional arguments to `load()` will override the arguments in the `BuilderConfig`.
# CSV script
The `csv.py` script is provided as an example, usage is:
```python
bbc = nlp.load('/Users/thomwolf/Documents/GitHub/datasets/datasets/nlp/csv',
name='bbc',
version="1.0.1",
split='train',
data_files={'train': ['/Users/thomwolf/Documents/GitHub/datasets/datasets/dummy_data/csv/test.csv']},
skip_rows=10,
download_mode='force_redownload')
```
# Checksums
We now don't raise an error if the checksum file is not found.
# `DownloadConfig`
We now have a download configuration class to handle all the specific arguments for file caching like proxies, using only local files or user-agents. |
https://github.com/huggingface/datasets/pull/25 | Add script csv datasets | Ok merging this for now.
One general note is that it's a bit hard to handle the `ClassLabel` generally in both `nlp` and `Arrow` since a class label typically need some metadata for the class names. For now, I raise a `NotImplementedError` when an `ArrowBuilder` output a table with a `DictionaryType` is encountered (which could be a simple equivalent for a `ClassLabel` Feature in Arrow tables).
In general and if we need this in the future for some Beam Datasets for instance, I think we should use one of the `metadata` fields in the `Arrow` type or table's schema to store the relation with indices and class names.
So ping me if you meet Beam datasets which uses `ClassLabels` (cc @lhoestq @patrickvonplaten @mariamabarham). | This is a PR allowing to create datasets from local CSV files. A usage might be:
```python
import nlp
ds = nlp.load(
path="csv",
name="bbc",
dataset_files={
nlp.Split.TRAIN: ["datasets/dummy_data/csv/train.csv"],
nlp.Split.TEST: [""datasets/dummy_data/csv/test.csv""]
},
csv_kwargs={
"skip_rows": 0,
"delimiter": ",",
"quote_char": "\"",
"header_as_column_names": True
}
)
```
```
Downloading and preparing dataset bbc/1.0.0 (download: Unknown size, generated: Unknown size, total: Unknown size) to /home/jplu/.cache/huggingface/datasets/bbc/1.0.0...
Dataset bbc downloaded and prepared to /home/jplu/.cache/huggingface/datasets/bbc/1.0.0. Subsequent calls will reuse this data.
{'test': Dataset(schema: {'category': 'string', 'text': 'string'}, num_rows: 49), 'train': Dataset(schema: {'category': 'string', 'text': 'string'}, num_rows: 99), 'validation': Dataset(schema: {'category': 'string', 'text': 'string'}, num_rows: 0)}
```
How it is read:
- `path`: the `csv` word means "I want to create a CSV dataset"
- `name`: the name of this dataset is `bbc`
- `dataset_files`: this is a dictionary where each key is the list of files corresponding to the key split.
- `csv_kwargs`: this is the keywords arguments to "explain" how to read the CSV files
* `skip_rows`: number of rows have to be skipped, starting from the beginning of the file
* `delimiter`: which delimiter is used to separate the columns
* `quote_char`: which quote char is used to represent a column where the delimiter appears in one of them
* `header_as_column_names`: will use the first row (header) of the file as name for the features. Otherwise the names will be automatically generated as `f1`, `f2`, etc... Will be applied after the `skip_rows` parameter.
**TODO**: for now the `csv.py` is copied each time we create a new dataset as `ds_name.py`, this behavior will be modified to have only the `csv.py` script copied only once and not for all the CSV datasets. | 123 | text: Add script csv datasets
This is a PR allowing to create datasets from local CSV files. A usage might be:
```python
import nlp
ds = nlp.load(
path="csv",
name="bbc",
dataset_files={
nlp.Split.TRAIN: ["datasets/dummy_data/csv/train.csv"],
nlp.Split.TEST: [""datasets/dummy_data/csv/test.csv""]
},
csv_kwargs={
"skip_rows": 0,
"delimiter": ",",
"quote_char": "\"",
"header_as_column_names": True
}
)
```
```
Downloading and preparing dataset bbc/1.0.0 (download: Unknown size, generated: Unknown size, total: Unknown size) to /home/jplu/.cache/huggingface/datasets/bbc/1.0.0...
Dataset bbc downloaded and prepared to /home/jplu/.cache/huggingface/datasets/bbc/1.0.0. Subsequent calls will reuse this data.
{'test': Dataset(schema: {'category': 'string', 'text': 'string'}, num_rows: 49), 'train': Dataset(schema: {'category': 'string', 'text': 'string'}, num_rows: 99), 'validation': Dataset(schema: {'category': 'string', 'text': 'string'}, num_rows: 0)}
```
How it is read:
- `path`: the `csv` word means "I want to create a CSV dataset"
- `name`: the name of this dataset is `bbc`
- `dataset_files`: this is a dictionary where each key is the list of files corresponding to the key split.
- `csv_kwargs`: this is the keywords arguments to "explain" how to read the CSV files
* `skip_rows`: number of rows have to be skipped, starting from the beginning of the file
* `delimiter`: which delimiter is used to separate the columns
* `quote_char`: which quote char is used to represent a column where the delimiter appears in one of them
* `header_as_column_names`: will use the first row (header) of the file as name for the features. Otherwise the names will be automatically generated as `f1`, `f2`, etc... Will be applied after the `skip_rows` parameter.
**TODO**: for now the `csv.py` is copied each time we create a new dataset as `ds_name.py`, this behavior will be modified to have only the `csv.py` script copied only once and not for all the CSV datasets.
Ok merging this for now.
One general note is that it's a bit hard to handle the `ClassLabel` generally in both `nlp` and `Arrow` since a class label typically need some metadata for the class names. For now, I raise a `NotImplementedError` when an `ArrowBuilder` output a table with a `DictionaryType` is encountered (which could be a simple equivalent for a `ClassLabel` Feature in Arrow tables).
In general and if we need this in the future for some Beam Datasets for instance, I think we should use one of the `metadata` fields in the `Arrow` type or table's schema to store the relation with indices and class names.
So ping me if you meet Beam datasets which uses `ClassLabels` (cc @lhoestq @patrickvonplaten @mariamabarham). |
https://github.com/huggingface/datasets/pull/24 | Add checksums | Looks good to me :-)
Just would prefer to get rid of the `_DYNAMICALLY_IMPORTED_MODULE` attribute and replace it by a `get_imported_module()` function. Maybe there is something I'm not seeing here though - what do you think? | ### Checksums files
They are stored next to the dataset script in urls_checksums/checksums.txt.
They are used to check the integrity of the datasets downloaded files.
I kept the same format as tensorflow-datasets.
There is one checksums file for all configs.
### Load a dataset
When you do `load("squad")`, it will also download the checksums file and put it next to the script in nlp/datasets/hash/urls_checksums/checksums.txt.
It also verifies that the downloaded files checksums match the expected ones.
You can ignore checksum tests with `load("squad", ignore_checksums=True)` (under the hood it just adds `ignore_checksums=True` in the `DownloadConfig`)
### Test a dataset
There is a new command `nlp-cli test squad` that runs `download_and_prepare` to see if it runs ok, and that verifies that all the checksums match. Allowed arguments are `--name`, `--all_configs`, `--ignore_checksums` and `--register_checksums`.
### Register checksums
1. If the dataset has external dataset files
The command `nlp-cli test squad --register_checksums --all_configs` runs `download_and_prepare` on all configs to see if it runs ok, and it creates the checksums file.
You can also register one config at a time using `--name` instead ; the checksums file will be completed and not overwritten.
If the script is a local script, the checksum file is moved to urls_checksums/checksums.txt next to the local script, to enable the user to upload both the script and the checksums file afterwards with `nlp-cli upload squad`.
2. If the dataset files are all inside the directory of the dataset script
The user can directly do `nlp-cli upload squad --register_checksums`, as there is no need to download anything.
In this case however, all the dataset must be uploaded at once.
--
PS : it doesn't allow to register checksums for canonical datasets, the file has to be added manually on S3 for now (I guess ?)
Also I feel like we must be sure that this processes would not constrain too much any user from uploading its dataset.
Let me know what you think :) | 36 | text: Add checksums
### Checksums files
They are stored next to the dataset script in urls_checksums/checksums.txt.
They are used to check the integrity of the datasets downloaded files.
I kept the same format as tensorflow-datasets.
There is one checksums file for all configs.
### Load a dataset
When you do `load("squad")`, it will also download the checksums file and put it next to the script in nlp/datasets/hash/urls_checksums/checksums.txt.
It also verifies that the downloaded files checksums match the expected ones.
You can ignore checksum tests with `load("squad", ignore_checksums=True)` (under the hood it just adds `ignore_checksums=True` in the `DownloadConfig`)
### Test a dataset
There is a new command `nlp-cli test squad` that runs `download_and_prepare` to see if it runs ok, and that verifies that all the checksums match. Allowed arguments are `--name`, `--all_configs`, `--ignore_checksums` and `--register_checksums`.
### Register checksums
1. If the dataset has external dataset files
The command `nlp-cli test squad --register_checksums --all_configs` runs `download_and_prepare` on all configs to see if it runs ok, and it creates the checksums file.
You can also register one config at a time using `--name` instead ; the checksums file will be completed and not overwritten.
If the script is a local script, the checksum file is moved to urls_checksums/checksums.txt next to the local script, to enable the user to upload both the script and the checksums file afterwards with `nlp-cli upload squad`.
2. If the dataset files are all inside the directory of the dataset script
The user can directly do `nlp-cli upload squad --register_checksums`, as there is no need to download anything.
In this case however, all the dataset must be uploaded at once.
--
PS : it doesn't allow to register checksums for canonical datasets, the file has to be added manually on S3 for now (I guess ?)
Also I feel like we must be sure that this processes would not constrain too much any user from uploading its dataset.
Let me know what you think :)
Looks good to me :-)
Just would prefer to get rid of the `_DYNAMICALLY_IMPORTED_MODULE` attribute and replace it by a `get_imported_module()` function. Maybe there is something I'm not seeing here though - what do you think? |
https://github.com/huggingface/datasets/pull/24 | Add checksums | > * I'm not sure I understand the general organization of checksums. I see we have a checksum folder with potentially several checksum files but I also see that checksum files can potentially contain several checksums. Could you explain a bit more how this is organized?
It should look like this:
squad/
βββ squad.py/
βββ urls_checksums/
...........βββ checksums.txt
In checksums.txt, the format is one line per (url, size, checksum)
I don't have a strong opinion between `urls_checksums/checksums.txt` or directly `checksums.txt` (not inside the `urls_checksums` folder), let me know what you think.
> * Also regarding your comment on checksum files for "canonical" datasets. I understand we can just create these with `nlp-cli test` and then upload them manually to our S3, right?
Yes you're right | ### Checksums files
They are stored next to the dataset script in urls_checksums/checksums.txt.
They are used to check the integrity of the datasets downloaded files.
I kept the same format as tensorflow-datasets.
There is one checksums file for all configs.
### Load a dataset
When you do `load("squad")`, it will also download the checksums file and put it next to the script in nlp/datasets/hash/urls_checksums/checksums.txt.
It also verifies that the downloaded files checksums match the expected ones.
You can ignore checksum tests with `load("squad", ignore_checksums=True)` (under the hood it just adds `ignore_checksums=True` in the `DownloadConfig`)
### Test a dataset
There is a new command `nlp-cli test squad` that runs `download_and_prepare` to see if it runs ok, and that verifies that all the checksums match. Allowed arguments are `--name`, `--all_configs`, `--ignore_checksums` and `--register_checksums`.
### Register checksums
1. If the dataset has external dataset files
The command `nlp-cli test squad --register_checksums --all_configs` runs `download_and_prepare` on all configs to see if it runs ok, and it creates the checksums file.
You can also register one config at a time using `--name` instead ; the checksums file will be completed and not overwritten.
If the script is a local script, the checksum file is moved to urls_checksums/checksums.txt next to the local script, to enable the user to upload both the script and the checksums file afterwards with `nlp-cli upload squad`.
2. If the dataset files are all inside the directory of the dataset script
The user can directly do `nlp-cli upload squad --register_checksums`, as there is no need to download anything.
In this case however, all the dataset must be uploaded at once.
--
PS : it doesn't allow to register checksums for canonical datasets, the file has to be added manually on S3 for now (I guess ?)
Also I feel like we must be sure that this processes would not constrain too much any user from uploading its dataset.
Let me know what you think :) | 125 | text: Add checksums
### Checksums files
They are stored next to the dataset script in urls_checksums/checksums.txt.
They are used to check the integrity of the datasets downloaded files.
I kept the same format as tensorflow-datasets.
There is one checksums file for all configs.
### Load a dataset
When you do `load("squad")`, it will also download the checksums file and put it next to the script in nlp/datasets/hash/urls_checksums/checksums.txt.
It also verifies that the downloaded files checksums match the expected ones.
You can ignore checksum tests with `load("squad", ignore_checksums=True)` (under the hood it just adds `ignore_checksums=True` in the `DownloadConfig`)
### Test a dataset
There is a new command `nlp-cli test squad` that runs `download_and_prepare` to see if it runs ok, and that verifies that all the checksums match. Allowed arguments are `--name`, `--all_configs`, `--ignore_checksums` and `--register_checksums`.
### Register checksums
1. If the dataset has external dataset files
The command `nlp-cli test squad --register_checksums --all_configs` runs `download_and_prepare` on all configs to see if it runs ok, and it creates the checksums file.
You can also register one config at a time using `--name` instead ; the checksums file will be completed and not overwritten.
If the script is a local script, the checksum file is moved to urls_checksums/checksums.txt next to the local script, to enable the user to upload both the script and the checksums file afterwards with `nlp-cli upload squad`.
2. If the dataset files are all inside the directory of the dataset script
The user can directly do `nlp-cli upload squad --register_checksums`, as there is no need to download anything.
In this case however, all the dataset must be uploaded at once.
--
PS : it doesn't allow to register checksums for canonical datasets, the file has to be added manually on S3 for now (I guess ?)
Also I feel like we must be sure that this processes would not constrain too much any user from uploading its dataset.
Let me know what you think :)
> * I'm not sure I understand the general organization of checksums. I see we have a checksum folder with potentially several checksum files but I also see that checksum files can potentially contain several checksums. Could you explain a bit more how this is organized?
It should look like this:
squad/
βββ squad.py/
βββ urls_checksums/
...........βββ checksums.txt
In checksums.txt, the format is one line per (url, size, checksum)
I don't have a strong opinion between `urls_checksums/checksums.txt` or directly `checksums.txt` (not inside the `urls_checksums` folder), let me know what you think.
> * Also regarding your comment on checksum files for "canonical" datasets. I understand we can just create these with `nlp-cli test` and then upload them manually to our S3, right?
Yes you're right |
https://github.com/huggingface/datasets/pull/24 | Add checksums | Update of the commands:
- nlp-cli test \<dataset\> : Run download_and_prepare and verify checksums
* --name \<name\> : run only for the name
* --all_configs : run for all configs
* --save_checksums : instead of verifying checksums, compute and save them
* --ignore_checksums : don't do checksums verification
- nlp-cli upload \<dataset_folder\> : Upload a dataset
* --upload_checksums : compute and upload checksums for uploaded files
TODO:
- don't overwrite checksums files on S3, to let the user upload a dataset in several steps if needed
Question:
- One idea from @patrickvonplaten : shall we upload checksums everytime we upload files ? (and therefore remove the upload_checksums parameter) | ### Checksums files
They are stored next to the dataset script in urls_checksums/checksums.txt.
They are used to check the integrity of the datasets downloaded files.
I kept the same format as tensorflow-datasets.
There is one checksums file for all configs.
### Load a dataset
When you do `load("squad")`, it will also download the checksums file and put it next to the script in nlp/datasets/hash/urls_checksums/checksums.txt.
It also verifies that the downloaded files checksums match the expected ones.
You can ignore checksum tests with `load("squad", ignore_checksums=True)` (under the hood it just adds `ignore_checksums=True` in the `DownloadConfig`)
### Test a dataset
There is a new command `nlp-cli test squad` that runs `download_and_prepare` to see if it runs ok, and that verifies that all the checksums match. Allowed arguments are `--name`, `--all_configs`, `--ignore_checksums` and `--register_checksums`.
### Register checksums
1. If the dataset has external dataset files
The command `nlp-cli test squad --register_checksums --all_configs` runs `download_and_prepare` on all configs to see if it runs ok, and it creates the checksums file.
You can also register one config at a time using `--name` instead ; the checksums file will be completed and not overwritten.
If the script is a local script, the checksum file is moved to urls_checksums/checksums.txt next to the local script, to enable the user to upload both the script and the checksums file afterwards with `nlp-cli upload squad`.
2. If the dataset files are all inside the directory of the dataset script
The user can directly do `nlp-cli upload squad --register_checksums`, as there is no need to download anything.
In this case however, all the dataset must be uploaded at once.
--
PS : it doesn't allow to register checksums for canonical datasets, the file has to be added manually on S3 for now (I guess ?)
Also I feel like we must be sure that this processes would not constrain too much any user from uploading its dataset.
Let me know what you think :) | 108 | text: Add checksums
### Checksums files
They are stored next to the dataset script in urls_checksums/checksums.txt.
They are used to check the integrity of the datasets downloaded files.
I kept the same format as tensorflow-datasets.
There is one checksums file for all configs.
### Load a dataset
When you do `load("squad")`, it will also download the checksums file and put it next to the script in nlp/datasets/hash/urls_checksums/checksums.txt.
It also verifies that the downloaded files checksums match the expected ones.
You can ignore checksum tests with `load("squad", ignore_checksums=True)` (under the hood it just adds `ignore_checksums=True` in the `DownloadConfig`)
### Test a dataset
There is a new command `nlp-cli test squad` that runs `download_and_prepare` to see if it runs ok, and that verifies that all the checksums match. Allowed arguments are `--name`, `--all_configs`, `--ignore_checksums` and `--register_checksums`.
### Register checksums
1. If the dataset has external dataset files
The command `nlp-cli test squad --register_checksums --all_configs` runs `download_and_prepare` on all configs to see if it runs ok, and it creates the checksums file.
You can also register one config at a time using `--name` instead ; the checksums file will be completed and not overwritten.
If the script is a local script, the checksum file is moved to urls_checksums/checksums.txt next to the local script, to enable the user to upload both the script and the checksums file afterwards with `nlp-cli upload squad`.
2. If the dataset files are all inside the directory of the dataset script
The user can directly do `nlp-cli upload squad --register_checksums`, as there is no need to download anything.
In this case however, all the dataset must be uploaded at once.
--
PS : it doesn't allow to register checksums for canonical datasets, the file has to be added manually on S3 for now (I guess ?)
Also I feel like we must be sure that this processes would not constrain too much any user from uploading its dataset.
Let me know what you think :)
Update of the commands:
- nlp-cli test \<dataset\> : Run download_and_prepare and verify checksums
* --name \<name\> : run only for the name
* --all_configs : run for all configs
* --save_checksums : instead of verifying checksums, compute and save them
* --ignore_checksums : don't do checksums verification
- nlp-cli upload \<dataset_folder\> : Upload a dataset
* --upload_checksums : compute and upload checksums for uploaded files
TODO:
- don't overwrite checksums files on S3, to let the user upload a dataset in several steps if needed
Question:
- One idea from @patrickvonplaten : shall we upload checksums everytime we upload files ? (and therefore remove the upload_checksums parameter) |
https://github.com/huggingface/datasets/pull/21 | Cleanup Features - Updating convert command - Fix Download manager | For conflicts, I think the mention hint "This should be modified because it mentions ..." is missing. | This PR makes a number of changes:
# Updating `Features`
Features are a complex mechanism provided in `tfds` to be able to modify a dataset on-the-fly when serializing to disk and when loading from disk.
We don't really need this because (1) it hides too much from the user and (2) our datatype can be directly mapped to Arrow tables on drive so we usually don't need to change the format before/after serialization.
This PR extracts and refactors these features in a single `features.py` files. It still keep a number of features classes for easy compatibility with tfds, namely the `Sequence`, `Tensor`, `ClassLabel` and `Translation` features.
Some more complex features involving a pre-processing on-the-fly during serialization are kept:
- `ClassLabel` which are able to convert from label strings to integers,
- `Translation`which does some check on the languages.
# Updating the `convert` command
We do a few updates here
- following the simplification of the `features` (cf above), conversion are updated
- we also makes it simpler to convert a single file
- some code need to be fixed manually after conversion (e.g. to remove some encoding processing in former tfds `Text` features. We highlight this code with a "git merge conflict" style syntax for easy manual fixing.
# Fix download manager iterator
You kept me up quite late on Tuesday night with this `os.scandir` change @lhoestq ;-)
| 17 | text: Cleanup Features - Updating convert command - Fix Download manager
This PR makes a number of changes:
# Updating `Features`
Features are a complex mechanism provided in `tfds` to be able to modify a dataset on-the-fly when serializing to disk and when loading from disk.
We don't really need this because (1) it hides too much from the user and (2) our datatype can be directly mapped to Arrow tables on drive so we usually don't need to change the format before/after serialization.
This PR extracts and refactors these features in a single `features.py` files. It still keep a number of features classes for easy compatibility with tfds, namely the `Sequence`, `Tensor`, `ClassLabel` and `Translation` features.
Some more complex features involving a pre-processing on-the-fly during serialization are kept:
- `ClassLabel` which are able to convert from label strings to integers,
- `Translation`which does some check on the languages.
# Updating the `convert` command
We do a few updates here
- following the simplification of the `features` (cf above), conversion are updated
- we also makes it simpler to convert a single file
- some code need to be fixed manually after conversion (e.g. to remove some encoding processing in former tfds `Text` features. We highlight this code with a "git merge conflict" style syntax for easy manual fixing.
# Fix download manager iterator
You kept me up quite late on Tuesday night with this `os.scandir` change @lhoestq ;-)
For conflicts, I think the mention hint "This should be modified because it mentions ..." is missing. |
https://github.com/huggingface/datasets/pull/16 | create our own DownloadManager | The new download manager is ready. I removed the old folder and I fixed a few remaining dependencies.
I tested it on squad and a few others from the dataset folder and it works fine.
The only impact of these changes is that it breaks the `download_and_prepare` script that was used to register the checksums when we create a dataset, as the checksum logic is not implemented.
Let me know if you have remarks | I tried to create our own - and way simpler - download manager, by replacing all the complicated stuff with our own `cached_path` solution.
With this implementation, I tried `dataset = nlp.load('squad')` and it seems to work fine.
For the implementation, what I did exactly:
- I copied the old download manager
- I removed all the dependences to the old `download` files
- I replaced all the download + extract calls by calls to `cached_path`
- I removed unused parameters (extract_dir, compute_stats) (maybe compute_stats could be re-added later if we want to compute stats...)
- I left some functions unimplemented for now. We will probably have to implement them because they are used by some datasets scripts (download_kaggle_data, iter_archive) or because we may need them at some point (download_checksums, _record_sizes_checksums)
Let me know if you think that this is going the right direction or if you have remarks.
Note: I didn't write any test yet as I wanted to read your remarks first | 74 | text: create our own DownloadManager
I tried to create our own - and way simpler - download manager, by replacing all the complicated stuff with our own `cached_path` solution.
With this implementation, I tried `dataset = nlp.load('squad')` and it seems to work fine.
For the implementation, what I did exactly:
- I copied the old download manager
- I removed all the dependences to the old `download` files
- I replaced all the download + extract calls by calls to `cached_path`
- I removed unused parameters (extract_dir, compute_stats) (maybe compute_stats could be re-added later if we want to compute stats...)
- I left some functions unimplemented for now. We will probably have to implement them because they are used by some datasets scripts (download_kaggle_data, iter_archive) or because we may need them at some point (download_checksums, _record_sizes_checksums)
Let me know if you think that this is going the right direction or if you have remarks.
Note: I didn't write any test yet as I wanted to read your remarks first
The new download manager is ready. I removed the old folder and I fixed a few remaining dependencies.
I tested it on squad and a few others from the dataset folder and it works fine.
The only impact of these changes is that it breaks the `download_and_prepare` script that was used to register the checksums when we create a dataset, as the checksum logic is not implemented.
Let me know if you have remarks |
https://github.com/huggingface/datasets/pull/16 | create our own DownloadManager | Ok merged it (a bit fast for you to update the copyright, now I see that. but it's ok, we'll do a pass on these doc/copyright before releasing anyway) | I tried to create our own - and way simpler - download manager, by replacing all the complicated stuff with our own `cached_path` solution.
With this implementation, I tried `dataset = nlp.load('squad')` and it seems to work fine.
For the implementation, what I did exactly:
- I copied the old download manager
- I removed all the dependences to the old `download` files
- I replaced all the download + extract calls by calls to `cached_path`
- I removed unused parameters (extract_dir, compute_stats) (maybe compute_stats could be re-added later if we want to compute stats...)
- I left some functions unimplemented for now. We will probably have to implement them because they are used by some datasets scripts (download_kaggle_data, iter_archive) or because we may need them at some point (download_checksums, _record_sizes_checksums)
Let me know if you think that this is going the right direction or if you have remarks.
Note: I didn't write any test yet as I wanted to read your remarks first | 29 | text: create our own DownloadManager
I tried to create our own - and way simpler - download manager, by replacing all the complicated stuff with our own `cached_path` solution.
With this implementation, I tried `dataset = nlp.load('squad')` and it seems to work fine.
For the implementation, what I did exactly:
- I copied the old download manager
- I removed all the dependences to the old `download` files
- I replaced all the download + extract calls by calls to `cached_path`
- I removed unused parameters (extract_dir, compute_stats) (maybe compute_stats could be re-added later if we want to compute stats...)
- I left some functions unimplemented for now. We will probably have to implement them because they are used by some datasets scripts (download_kaggle_data, iter_archive) or because we may need them at some point (download_checksums, _record_sizes_checksums)
Let me know if you think that this is going the right direction or if you have remarks.
Note: I didn't write any test yet as I wanted to read your remarks first
Ok merged it (a bit fast for you to update the copyright, now I see that. but it's ok, we'll do a pass on these doc/copyright before releasing anyway) |
https://github.com/huggingface/datasets/pull/16 | create our own DownloadManager | Actually two additional things here @lhoestq (I merged too fast sorry, let's make a new PR for additional developments):
- I think we can remove some dependencies now (e.g. `promises`) in setup.py, can you have a look?
- also, I think we can remove the boto3 dependency like here: https://github.com/huggingface/transformers/pull/3968 | I tried to create our own - and way simpler - download manager, by replacing all the complicated stuff with our own `cached_path` solution.
With this implementation, I tried `dataset = nlp.load('squad')` and it seems to work fine.
For the implementation, what I did exactly:
- I copied the old download manager
- I removed all the dependences to the old `download` files
- I replaced all the download + extract calls by calls to `cached_path`
- I removed unused parameters (extract_dir, compute_stats) (maybe compute_stats could be re-added later if we want to compute stats...)
- I left some functions unimplemented for now. We will probably have to implement them because they are used by some datasets scripts (download_kaggle_data, iter_archive) or because we may need them at some point (download_checksums, _record_sizes_checksums)
Let me know if you think that this is going the right direction or if you have remarks.
Note: I didn't write any test yet as I wanted to read your remarks first | 50 | text: create our own DownloadManager
I tried to create our own - and way simpler - download manager, by replacing all the complicated stuff with our own `cached_path` solution.
With this implementation, I tried `dataset = nlp.load('squad')` and it seems to work fine.
For the implementation, what I did exactly:
- I copied the old download manager
- I removed all the dependences to the old `download` files
- I replaced all the download + extract calls by calls to `cached_path`
- I removed unused parameters (extract_dir, compute_stats) (maybe compute_stats could be re-added later if we want to compute stats...)
- I left some functions unimplemented for now. We will probably have to implement them because they are used by some datasets scripts (download_kaggle_data, iter_archive) or because we may need them at some point (download_checksums, _record_sizes_checksums)
Let me know if you think that this is going the right direction or if you have remarks.
Note: I didn't write any test yet as I wanted to read your remarks first
Actually two additional things here @lhoestq (I merged too fast sorry, let's make a new PR for additional developments):
- I think we can remove some dependencies now (e.g. `promises`) in setup.py, can you have a look?
- also, I think we can remove the boto3 dependency like here: https://github.com/huggingface/transformers/pull/3968 |
https://github.com/huggingface/datasets/pull/15 | [Tests] General Test Design for all dataset scripts | > I think I'm fine with this.
>
> The alternative would be to host a small subset of the dataset on the S3 together with the testing script. But I think having all (test file creation + actual tests) in one file is actually quite convenient.
>
> Good for me!
>
> One question though, will we have to create one test file for each of the 100+ datasets or could we make some automatic conversion from tfds dataset test files?
I think if we go the way shown in the PR we would have to create one test file for each of the 100+ datasets.
As far as I know the tfds test files all rely on the user having created a special download folder structure in `tensorflow-datasets/tensorflow_datasets/testing/test_data/fake_examples`.
My hypothesis was:
Becasue, we don't want to work with PRs, no `dataset_script` is going to be in the official repo, so no `dataset_script_test` can be in the repo either. Therefore we can also not have any "fake" test folder structure in the repo.
**BUT:** As you mentioned @thom, we could have a fake data structure on AWS. To add a test the user has to upload multiple small test files when uploading his data set script.
So for a cli this could look like:
`python nlp-cli upload <data_set_script> --testfiles <relative path to test file 1> <relative path to test file 2> ...`
or even easier if the user just creates the dataset folder with the script inside and the testing folder structure, then the API could look like:
`python nlp-cli upload <path/to/dataset/folder>`
and the dataset folder would look like
```
squad
- squad.py
- fake_data # this dir would have to have the exact same structure we get when downloading from the official squad data url
```
This way I think we wouldn't even need any test files at all for each dataset script. For special datasets like `c4` or `wikipedia` we could then allow to optionally upload another test script.
We just assume that this is our downloaded `url` and check all functionality from there.
Thinking a bit more about this solution sounds a) much less work and b) even easier for the user.
A small problem I see here though:
1) What do we do when the depending on the config name the downloaded folder structure is very different? I think for each dataset config name we should have one test, which could correspond to one "fake" folder structure on AWS
@thomwolf What do you think? I would actually go for this solution instead now.
@mariamabarham You have written many more tfds dataset scripts and tests than I have - what do you think?
| The general idea is similar to how testing is done in `transformers`. There is one general `test_dataset_common.py` file which has a `DatasetTesterMixin` class. This class implements all of the logic that can be used in a generic way for all dataset classes. The idea is to keep each individual dataset test file as minimal as possible.
In order to test whether the specific data set class can download the data and generate the examples **without** downloading the actual data all the time, a MockDataLoaderManager class is used which receives a `mock_folder_structure_fn` function from each individual dataset test file that create "fake" data and which returns the same folder structure that would have been created when using the real data downloader. | 448 | text: [Tests] General Test Design for all dataset scripts
The general idea is similar to how testing is done in `transformers`. There is one general `test_dataset_common.py` file which has a `DatasetTesterMixin` class. This class implements all of the logic that can be used in a generic way for all dataset classes. The idea is to keep each individual dataset test file as minimal as possible.
In order to test whether the specific data set class can download the data and generate the examples **without** downloading the actual data all the time, a MockDataLoaderManager class is used which receives a `mock_folder_structure_fn` function from each individual dataset test file that create "fake" data and which returns the same folder structure that would have been created when using the real data downloader.
> I think I'm fine with this.
>
> The alternative would be to host a small subset of the dataset on the S3 together with the testing script. But I think having all (test file creation + actual tests) in one file is actually quite convenient.
>
> Good for me!
>
> One question though, will we have to create one test file for each of the 100+ datasets or could we make some automatic conversion from tfds dataset test files?
I think if we go the way shown in the PR we would have to create one test file for each of the 100+ datasets.
As far as I know the tfds test files all rely on the user having created a special download folder structure in `tensorflow-datasets/tensorflow_datasets/testing/test_data/fake_examples`.
My hypothesis was:
Becasue, we don't want to work with PRs, no `dataset_script` is going to be in the official repo, so no `dataset_script_test` can be in the repo either. Therefore we can also not have any "fake" test folder structure in the repo.
**BUT:** As you mentioned @thom, we could have a fake data structure on AWS. To add a test the user has to upload multiple small test files when uploading his data set script.
So for a cli this could look like:
`python nlp-cli upload <data_set_script> --testfiles <relative path to test file 1> <relative path to test file 2> ...`
or even easier if the user just creates the dataset folder with the script inside and the testing folder structure, then the API could look like:
`python nlp-cli upload <path/to/dataset/folder>`
and the dataset folder would look like
```
squad
- squad.py
- fake_data # this dir would have to have the exact same structure we get when downloading from the official squad data url
```
This way I think we wouldn't even need any test files at all for each dataset script. For special datasets like `c4` or `wikipedia` we could then allow to optionally upload another test script.
We just assume that this is our downloaded `url` and check all functionality from there.
Thinking a bit more about this solution sounds a) much less work and b) even easier for the user.
A small problem I see here though:
1) What do we do when the depending on the config name the downloaded folder structure is very different? I think for each dataset config name we should have one test, which could correspond to one "fake" folder structure on AWS
@thomwolf What do you think? I would actually go for this solution instead now.
@mariamabarham You have written many more tfds dataset scripts and tests than I have - what do you think?
|
https://github.com/huggingface/datasets/pull/15 | [Tests] General Test Design for all dataset scripts | Regarding the tfds tests, I don't really see a point in keeping them because:
1) If you provide a fake data structure, IMO there is no need for each dataset to have an individual test file because (I think) most datasets have the same functions `_split_generators` and `_generate_examples` for which you can just test the functionality in a common test file. For special functions like these beam / pipeline functionality you probably need an extra test file. But @mariamabarham I think you have seen more than I have here as well
2) The dataset test design is very much intertwined with the download manager design and contains a lot of code. I would like to seperate the tests into a) tests for downloading in general b) tests for post download data set pre-processing. Since we are going to change the download code anyways quite a lot, my plan was to focus on b) first. | The general idea is similar to how testing is done in `transformers`. There is one general `test_dataset_common.py` file which has a `DatasetTesterMixin` class. This class implements all of the logic that can be used in a generic way for all dataset classes. The idea is to keep each individual dataset test file as minimal as possible.
In order to test whether the specific data set class can download the data and generate the examples **without** downloading the actual data all the time, a MockDataLoaderManager class is used which receives a `mock_folder_structure_fn` function from each individual dataset test file that create "fake" data and which returns the same folder structure that would have been created when using the real data downloader. | 154 | text: [Tests] General Test Design for all dataset scripts
The general idea is similar to how testing is done in `transformers`. There is one general `test_dataset_common.py` file which has a `DatasetTesterMixin` class. This class implements all of the logic that can be used in a generic way for all dataset classes. The idea is to keep each individual dataset test file as minimal as possible.
In order to test whether the specific data set class can download the data and generate the examples **without** downloading the actual data all the time, a MockDataLoaderManager class is used which receives a `mock_folder_structure_fn` function from each individual dataset test file that create "fake" data and which returns the same folder structure that would have been created when using the real data downloader.
Regarding the tfds tests, I don't really see a point in keeping them because:
1) If you provide a fake data structure, IMO there is no need for each dataset to have an individual test file because (I think) most datasets have the same functions `_split_generators` and `_generate_examples` for which you can just test the functionality in a common test file. For special functions like these beam / pipeline functionality you probably need an extra test file. But @mariamabarham I think you have seen more than I have here as well
2) The dataset test design is very much intertwined with the download manager design and contains a lot of code. I would like to seperate the tests into a) tests for downloading in general b) tests for post download data set pre-processing. Since we are going to change the download code anyways quite a lot, my plan was to focus on b) first. |
https://github.com/huggingface/datasets/pull/15 | [Tests] General Test Design for all dataset scripts | I like the idea of having a fake data folder on S3. I have seen datasets with nested compressed files structures that would be tedious to generate with code. And for users it is probably easier to create a fake data folder by taking a subset of the actual data, and then upload it as you said. | The general idea is similar to how testing is done in `transformers`. There is one general `test_dataset_common.py` file which has a `DatasetTesterMixin` class. This class implements all of the logic that can be used in a generic way for all dataset classes. The idea is to keep each individual dataset test file as minimal as possible.
In order to test whether the specific data set class can download the data and generate the examples **without** downloading the actual data all the time, a MockDataLoaderManager class is used which receives a `mock_folder_structure_fn` function from each individual dataset test file that create "fake" data and which returns the same folder structure that would have been created when using the real data downloader. | 57 | text: [Tests] General Test Design for all dataset scripts
The general idea is similar to how testing is done in `transformers`. There is one general `test_dataset_common.py` file which has a `DatasetTesterMixin` class. This class implements all of the logic that can be used in a generic way for all dataset classes. The idea is to keep each individual dataset test file as minimal as possible.
In order to test whether the specific data set class can download the data and generate the examples **without** downloading the actual data all the time, a MockDataLoaderManager class is used which receives a `mock_folder_structure_fn` function from each individual dataset test file that create "fake" data and which returns the same folder structure that would have been created when using the real data downloader.
I like the idea of having a fake data folder on S3. I have seen datasets with nested compressed files structures that would be tedious to generate with code. And for users it is probably easier to create a fake data folder by taking a subset of the actual data, and then upload it as you said. |
https://github.com/huggingface/datasets/pull/15 | [Tests] General Test Design for all dataset scripts | > > I think I'm fine with this.
> > The alternative would be to host a small subset of the dataset on the S3 together with the testing script. But I think having all (test file creation + actual tests) in one file is actually quite convenient.
> > Good for me!
> > One question though, will we have to create one test file for each of the 100+ datasets or could we make some automatic conversion from tfds dataset test files?
>
> I think if we go the way shown in the PR we would have to create one test file for each of the 100+ datasets.
>
> As far as I know the tfds test files all rely on the user having created a special download folder structure in `tensorflow-datasets/tensorflow_datasets/testing/test_data/fake_examples`.
>
> My hypothesis was:
> Becasue, we don't want to work with PRs, no `dataset_script` is going to be in the official repo, so no `dataset_script_test` can be in the repo either. Therefore we can also not have any "fake" test folder structure in the repo.
>
> **BUT:** As you mentioned @thom, we could have a fake data structure on AWS. To add a test the user has to upload multiple small test files when uploading his data set script.
>
> So for a cli this could look like:
> `python nlp-cli upload <data_set_script> --testfiles <relative path to test file 1> <relative path to test file 2> ...`
>
> or even easier if the user just creates the dataset folder with the script inside and the testing folder structure, then the API could look like:
>
> `python nlp-cli upload <path/to/dataset/folder>`
>
> and the dataset folder would look like
>
> ```
> squad
> - squad.py
> - fake_data # this dir would have to have the exact same structure we get when downloading from the official squad data url
> ```
>
> This way I think we wouldn't even need any test files at all for each dataset script. For special datasets like `c4` or `wikipedia` we could then allow to optionally upload another test script.
> We just assume that this is our downloaded `url` and check all functionality from there.
>
> Thinking a bit more about this solution sounds a) much less work and b) even easier for the user.
>
> A small problem I see here though:
>
> 1. What do we do when the depending on the config name the downloaded folder structure is very different? I think for each dataset config name we should have one test, which could correspond to one "fake" folder structure on AWS
>
> @thomwolf What do you think? I would actually go for this solution instead now.
> @mariamabarham You have written many more tfds dataset scripts and tests than I have - what do you think?
I'm agreed with you just one thing, for some dataset like glue or xtreme you may have multiple datasets in it. so I think a good way is to have one main fake folder and a subdirectory for each dataset inside | The general idea is similar to how testing is done in `transformers`. There is one general `test_dataset_common.py` file which has a `DatasetTesterMixin` class. This class implements all of the logic that can be used in a generic way for all dataset classes. The idea is to keep each individual dataset test file as minimal as possible.
In order to test whether the specific data set class can download the data and generate the examples **without** downloading the actual data all the time, a MockDataLoaderManager class is used which receives a `mock_folder_structure_fn` function from each individual dataset test file that create "fake" data and which returns the same folder structure that would have been created when using the real data downloader. | 526 | text: [Tests] General Test Design for all dataset scripts
The general idea is similar to how testing is done in `transformers`. There is one general `test_dataset_common.py` file which has a `DatasetTesterMixin` class. This class implements all of the logic that can be used in a generic way for all dataset classes. The idea is to keep each individual dataset test file as minimal as possible.
In order to test whether the specific data set class can download the data and generate the examples **without** downloading the actual data all the time, a MockDataLoaderManager class is used which receives a `mock_folder_structure_fn` function from each individual dataset test file that create "fake" data and which returns the same folder structure that would have been created when using the real data downloader.
> > I think I'm fine with this.
> > The alternative would be to host a small subset of the dataset on the S3 together with the testing script. But I think having all (test file creation + actual tests) in one file is actually quite convenient.
> > Good for me!
> > One question though, will we have to create one test file for each of the 100+ datasets or could we make some automatic conversion from tfds dataset test files?
>
> I think if we go the way shown in the PR we would have to create one test file for each of the 100+ datasets.
>
> As far as I know the tfds test files all rely on the user having created a special download folder structure in `tensorflow-datasets/tensorflow_datasets/testing/test_data/fake_examples`.
>
> My hypothesis was:
> Becasue, we don't want to work with PRs, no `dataset_script` is going to be in the official repo, so no `dataset_script_test` can be in the repo either. Therefore we can also not have any "fake" test folder structure in the repo.
>
> **BUT:** As you mentioned @thom, we could have a fake data structure on AWS. To add a test the user has to upload multiple small test files when uploading his data set script.
>
> So for a cli this could look like:
> `python nlp-cli upload <data_set_script> --testfiles <relative path to test file 1> <relative path to test file 2> ...`
>
> or even easier if the user just creates the dataset folder with the script inside and the testing folder structure, then the API could look like:
>
> `python nlp-cli upload <path/to/dataset/folder>`
>
> and the dataset folder would look like
>
> ```
> squad
> - squad.py
> - fake_data # this dir would have to have the exact same structure we get when downloading from the official squad data url
> ```
>
> This way I think we wouldn't even need any test files at all for each dataset script. For special datasets like `c4` or `wikipedia` we could then allow to optionally upload another test script.
> We just assume that this is our downloaded `url` and check all functionality from there.
>
> Thinking a bit more about this solution sounds a) much less work and b) even easier for the user.
>
> A small problem I see here though:
>
> 1. What do we do when the depending on the config name the downloaded folder structure is very different? I think for each dataset config name we should have one test, which could correspond to one "fake" folder structure on AWS
>
> @thomwolf What do you think? I would actually go for this solution instead now.
> @mariamabarham You have written many more tfds dataset scripts and tests than I have - what do you think?
I'm agreed with you just one thing, for some dataset like glue or xtreme you may have multiple datasets in it. so I think a good way is to have one main fake folder and a subdirectory for each dataset inside |
https://github.com/huggingface/datasets/pull/15 | [Tests] General Test Design for all dataset scripts | > Regarding the tfds tests, I don't really see a point in keeping them because:
>
> 1. If you provide a fake data structure, IMO there is no need for each dataset to have an individual test file because (I think) most datasets have the same functions `_split_generators` and `_generate_examples` for which you can just test the functionality in a common test file. For special functions like these beam / pipeline functionality you probably need an extra test file. But @mariamabarham I think you have seen more than I have here as well
> 2. The dataset test design is very much intertwined with the download manager design and contains a lot of code. I would like to seperate the tests into a) tests for downloading in general b) tests for post download data set pre-processing. Since we are going to change the download code anyways quite a lot, my plan was to focus on b) first.
For _split_generator, yes. But I'm not sure for _generate_examples because there is lots of things that should be taken into account such as feature names and types, data format (json, jsonl, csv, tsv,..) | The general idea is similar to how testing is done in `transformers`. There is one general `test_dataset_common.py` file which has a `DatasetTesterMixin` class. This class implements all of the logic that can be used in a generic way for all dataset classes. The idea is to keep each individual dataset test file as minimal as possible.
In order to test whether the specific data set class can download the data and generate the examples **without** downloading the actual data all the time, a MockDataLoaderManager class is used which receives a `mock_folder_structure_fn` function from each individual dataset test file that create "fake" data and which returns the same folder structure that would have been created when using the real data downloader. | 191 | text: [Tests] General Test Design for all dataset scripts
The general idea is similar to how testing is done in `transformers`. There is one general `test_dataset_common.py` file which has a `DatasetTesterMixin` class. This class implements all of the logic that can be used in a generic way for all dataset classes. The idea is to keep each individual dataset test file as minimal as possible.
In order to test whether the specific data set class can download the data and generate the examples **without** downloading the actual data all the time, a MockDataLoaderManager class is used which receives a `mock_folder_structure_fn` function from each individual dataset test file that create "fake" data and which returns the same folder structure that would have been created when using the real data downloader.
> Regarding the tfds tests, I don't really see a point in keeping them because:
>
> 1. If you provide a fake data structure, IMO there is no need for each dataset to have an individual test file because (I think) most datasets have the same functions `_split_generators` and `_generate_examples` for which you can just test the functionality in a common test file. For special functions like these beam / pipeline functionality you probably need an extra test file. But @mariamabarham I think you have seen more than I have here as well
> 2. The dataset test design is very much intertwined with the download manager design and contains a lot of code. I would like to seperate the tests into a) tests for downloading in general b) tests for post download data set pre-processing. Since we are going to change the download code anyways quite a lot, my plan was to focus on b) first.
For _split_generator, yes. But I'm not sure for _generate_examples because there is lots of things that should be taken into account such as feature names and types, data format (json, jsonl, csv, tsv,..) |
https://github.com/huggingface/datasets/pull/15 | [Tests] General Test Design for all dataset scripts | Sounds good to me!
When testing, we could thus just override the prefix in the URL inside the download manager to have them point to the test directory on our S3.
Cc @lhoestq | The general idea is similar to how testing is done in `transformers`. There is one general `test_dataset_common.py` file which has a `DatasetTesterMixin` class. This class implements all of the logic that can be used in a generic way for all dataset classes. The idea is to keep each individual dataset test file as minimal as possible.
In order to test whether the specific data set class can download the data and generate the examples **without** downloading the actual data all the time, a MockDataLoaderManager class is used which receives a `mock_folder_structure_fn` function from each individual dataset test file that create "fake" data and which returns the same folder structure that would have been created when using the real data downloader. | 33 | text: [Tests] General Test Design for all dataset scripts
The general idea is similar to how testing is done in `transformers`. There is one general `test_dataset_common.py` file which has a `DatasetTesterMixin` class. This class implements all of the logic that can be used in a generic way for all dataset classes. The idea is to keep each individual dataset test file as minimal as possible.
In order to test whether the specific data set class can download the data and generate the examples **without** downloading the actual data all the time, a MockDataLoaderManager class is used which receives a `mock_folder_structure_fn` function from each individual dataset test file that create "fake" data and which returns the same folder structure that would have been created when using the real data downloader.
Sounds good to me!
When testing, we could thus just override the prefix in the URL inside the download manager to have them point to the test directory on our S3.
Cc @lhoestq |
https://github.com/huggingface/datasets/pull/15 | [Tests] General Test Design for all dataset scripts | Ok, here is a second draft for the testing structure.
I think the big difficulty here is "How can you generate tests on the fly from a given dataset name, *e.g.* `squad`"?
So, this morning I did some research on "parameterized testing" and pure `unittest` or `pytest` didn't work very well.
I found the lib https://github.com/wolever/parameterized, which works very nicely for our use case I think.
@thomwolf - would it be ok to have a dependence on this lib for `nlp`? It seems like a light-weight lib to me.
This lib allows to add a `parameterization` decorator to a `unittest.TestCase` class so that the class can be instantiated for multiple different arguments (which are the dataset names `squad` etc. in our case).
What I like about this lib is that one only has to add the decorator and the each of the parameterized tests are shown, like this:
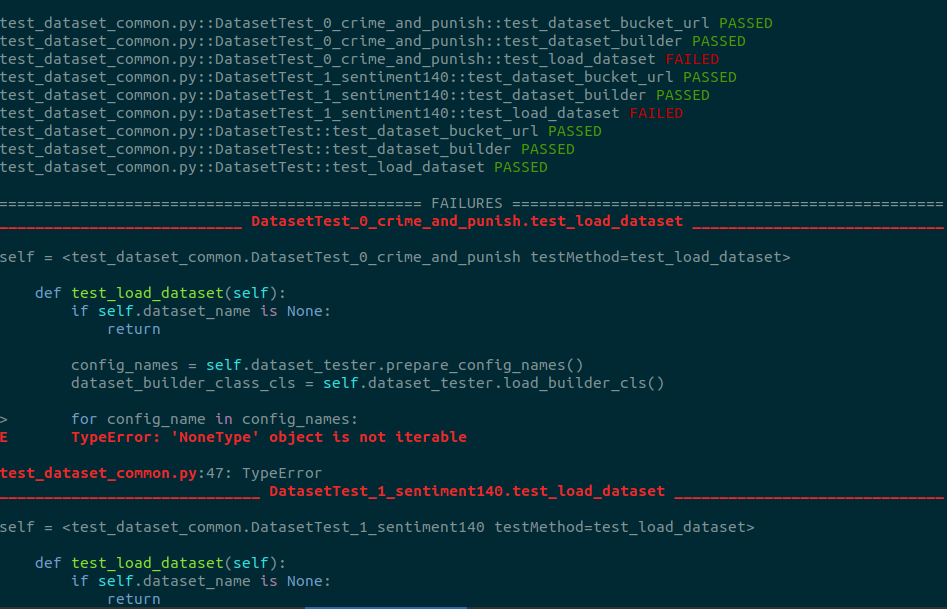
With this structure we would only have to upload the dummy data for each dataset and would not require a specific testing file.
What do you think @thomwolf @mariamabarham @lhoestq ? | The general idea is similar to how testing is done in `transformers`. There is one general `test_dataset_common.py` file which has a `DatasetTesterMixin` class. This class implements all of the logic that can be used in a generic way for all dataset classes. The idea is to keep each individual dataset test file as minimal as possible.
In order to test whether the specific data set class can download the data and generate the examples **without** downloading the actual data all the time, a MockDataLoaderManager class is used which receives a `mock_folder_structure_fn` function from each individual dataset test file that create "fake" data and which returns the same folder structure that would have been created when using the real data downloader. | 183 | text: [Tests] General Test Design for all dataset scripts
The general idea is similar to how testing is done in `transformers`. There is one general `test_dataset_common.py` file which has a `DatasetTesterMixin` class. This class implements all of the logic that can be used in a generic way for all dataset classes. The idea is to keep each individual dataset test file as minimal as possible.
In order to test whether the specific data set class can download the data and generate the examples **without** downloading the actual data all the time, a MockDataLoaderManager class is used which receives a `mock_folder_structure_fn` function from each individual dataset test file that create "fake" data and which returns the same folder structure that would have been created when using the real data downloader.
Ok, here is a second draft for the testing structure.
I think the big difficulty here is "How can you generate tests on the fly from a given dataset name, *e.g.* `squad`"?
So, this morning I did some research on "parameterized testing" and pure `unittest` or `pytest` didn't work very well.
I found the lib https://github.com/wolever/parameterized, which works very nicely for our use case I think.
@thomwolf - would it be ok to have a dependence on this lib for `nlp`? It seems like a light-weight lib to me.
This lib allows to add a `parameterization` decorator to a `unittest.TestCase` class so that the class can be instantiated for multiple different arguments (which are the dataset names `squad` etc. in our case).
What I like about this lib is that one only has to add the decorator and the each of the parameterized tests are shown, like this:
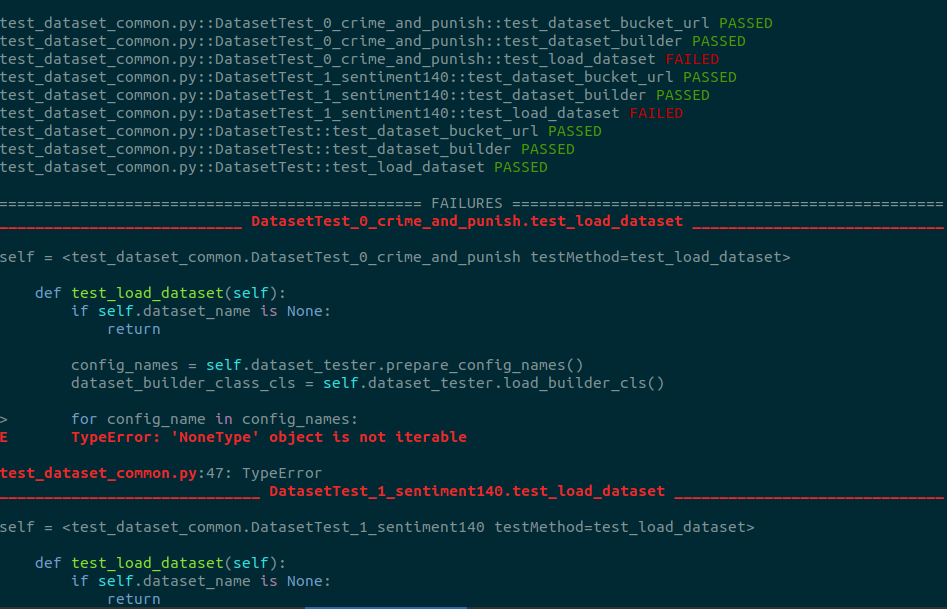
With this structure we would only have to upload the dummy data for each dataset and would not require a specific testing file.
What do you think @thomwolf @mariamabarham @lhoestq ? |
https://github.com/huggingface/datasets/pull/15 | [Tests] General Test Design for all dataset scripts | I think this is a nice solution.
Do you think we could have the `parametrized` dependency in a `[test]` optional installation of `setup.py`? I would really like to keep the dependencies of the standard installation as small as possible. | The general idea is similar to how testing is done in `transformers`. There is one general `test_dataset_common.py` file which has a `DatasetTesterMixin` class. This class implements all of the logic that can be used in a generic way for all dataset classes. The idea is to keep each individual dataset test file as minimal as possible.
In order to test whether the specific data set class can download the data and generate the examples **without** downloading the actual data all the time, a MockDataLoaderManager class is used which receives a `mock_folder_structure_fn` function from each individual dataset test file that create "fake" data and which returns the same folder structure that would have been created when using the real data downloader. | 39 | text: [Tests] General Test Design for all dataset scripts
The general idea is similar to how testing is done in `transformers`. There is one general `test_dataset_common.py` file which has a `DatasetTesterMixin` class. This class implements all of the logic that can be used in a generic way for all dataset classes. The idea is to keep each individual dataset test file as minimal as possible.
In order to test whether the specific data set class can download the data and generate the examples **without** downloading the actual data all the time, a MockDataLoaderManager class is used which receives a `mock_folder_structure_fn` function from each individual dataset test file that create "fake" data and which returns the same folder structure that would have been created when using the real data downloader.
I think this is a nice solution.
Do you think we could have the `parametrized` dependency in a `[test]` optional installation of `setup.py`? I would really like to keep the dependencies of the standard installation as small as possible. |
https://github.com/huggingface/datasets/pull/15 | [Tests] General Test Design for all dataset scripts | > I think this is a nice solution.
>
> Do you think we could have the `parametrized` dependency in a `[test]` optional installation of `setup.py`? I would really like to keep the dependencies of the standard installation as small as possible.
Yes definitely! | The general idea is similar to how testing is done in `transformers`. There is one general `test_dataset_common.py` file which has a `DatasetTesterMixin` class. This class implements all of the logic that can be used in a generic way for all dataset classes. The idea is to keep each individual dataset test file as minimal as possible.
In order to test whether the specific data set class can download the data and generate the examples **without** downloading the actual data all the time, a MockDataLoaderManager class is used which receives a `mock_folder_structure_fn` function from each individual dataset test file that create "fake" data and which returns the same folder structure that would have been created when using the real data downloader. | 44 | text: [Tests] General Test Design for all dataset scripts
The general idea is similar to how testing is done in `transformers`. There is one general `test_dataset_common.py` file which has a `DatasetTesterMixin` class. This class implements all of the logic that can be used in a generic way for all dataset classes. The idea is to keep each individual dataset test file as minimal as possible.
In order to test whether the specific data set class can download the data and generate the examples **without** downloading the actual data all the time, a MockDataLoaderManager class is used which receives a `mock_folder_structure_fn` function from each individual dataset test file that create "fake" data and which returns the same folder structure that would have been created when using the real data downloader.
> I think this is a nice solution.
>
> Do you think we could have the `parametrized` dependency in a `[test]` optional installation of `setup.py`? I would really like to keep the dependencies of the standard installation as small as possible.
Yes definitely! |
https://github.com/huggingface/datasets/pull/15 | [Tests] General Test Design for all dataset scripts | UPDATE:
This test design is ready now. I added dummy data to S3 for the dataests: `squad, crime_and_punish, sentiment140` . The structure can be seen on `https://s3.console.aws.amazon.com/s3/buckets/datasets.huggingface.co/nlp/squad/dummy/?region=us-east-1&tab=overview` for `squad`.
All dummy data files have to be in .zip format and called `dummy_data.zip`. The zip file should thereby have the exact same folder structure one gets from downloading the "real" data url(s).
To show how the .zip file looks like for the added datasets, I added the folder `nlp/datasets/dummy_data` in this PR. I think we can leave for the moment so that people can see better how to add dummy data tests and later delete it like `nlp/datasets/nlp`. | The general idea is similar to how testing is done in `transformers`. There is one general `test_dataset_common.py` file which has a `DatasetTesterMixin` class. This class implements all of the logic that can be used in a generic way for all dataset classes. The idea is to keep each individual dataset test file as minimal as possible.
In order to test whether the specific data set class can download the data and generate the examples **without** downloading the actual data all the time, a MockDataLoaderManager class is used which receives a `mock_folder_structure_fn` function from each individual dataset test file that create "fake" data and which returns the same folder structure that would have been created when using the real data downloader. | 107 | text: [Tests] General Test Design for all dataset scripts
The general idea is similar to how testing is done in `transformers`. There is one general `test_dataset_common.py` file which has a `DatasetTesterMixin` class. This class implements all of the logic that can be used in a generic way for all dataset classes. The idea is to keep each individual dataset test file as minimal as possible.
In order to test whether the specific data set class can download the data and generate the examples **without** downloading the actual data all the time, a MockDataLoaderManager class is used which receives a `mock_folder_structure_fn` function from each individual dataset test file that create "fake" data and which returns the same folder structure that would have been created when using the real data downloader.
UPDATE:
This test design is ready now. I added dummy data to S3 for the dataests: `squad, crime_and_punish, sentiment140` . The structure can be seen on `https://s3.console.aws.amazon.com/s3/buckets/datasets.huggingface.co/nlp/squad/dummy/?region=us-east-1&tab=overview` for `squad`.
All dummy data files have to be in .zip format and called `dummy_data.zip`. The zip file should thereby have the exact same folder structure one gets from downloading the "real" data url(s).
To show how the .zip file looks like for the added datasets, I added the folder `nlp/datasets/dummy_data` in this PR. I think we can leave for the moment so that people can see better how to add dummy data tests and later delete it like `nlp/datasets/nlp`. |
https://github.com/huggingface/datasets/pull/13 | [Make style] | I think this can be quickly reproduced.
I use `black, version 19.10b0`.
When running:
`black nlp/src/arrow_reader.py`
it gives me:
```
error: cannot format /home/patrick/hugging_face/nlp/src/nlp/arrow_reader.py: cannot use --safe with this file; failed to parse source file. AST error message: invalid syntax (<unknown>, line 78)
Oh no! π₯ π π₯
1 file failed to reformat.
```
The line in question is:
https://github.com/huggingface/nlp/blob/6922a16705e61f9e31a365f2606090b84d49241f/src/nlp/arrow_reader.py#L78
What is weird is that the trainer file in `transformers` has more or less the same syntax and black does not fail there:
https://github.com/huggingface/transformers/blob/cb3c2212c79d7ff0a4a4e84c3db48371ecc1c15d/src/transformers/trainer.py#L95
I googled quite a bit about black & typing hints yesterday and didn't find anything useful.
Any ideas @thomwolf @julien-c @LysandreJik ? | Added Makefile and applied make style to all.
make style runs the following code:
```
style:
black --line-length 119 --target-version py35 src
isort --recursive src
```
It's the same code that is run in `transformers`. | 106 | text: [Make style]
Added Makefile and applied make style to all.
make style runs the following code:
```
style:
black --line-length 119 --target-version py35 src
isort --recursive src
```
It's the same code that is run in `transformers`.
I think this can be quickly reproduced.
I use `black, version 19.10b0`.
When running:
`black nlp/src/arrow_reader.py`
it gives me:
```
error: cannot format /home/patrick/hugging_face/nlp/src/nlp/arrow_reader.py: cannot use --safe with this file; failed to parse source file. AST error message: invalid syntax (<unknown>, line 78)
Oh no! π₯ π π₯
1 file failed to reformat.
```
The line in question is:
https://github.com/huggingface/nlp/blob/6922a16705e61f9e31a365f2606090b84d49241f/src/nlp/arrow_reader.py#L78
What is weird is that the trainer file in `transformers` has more or less the same syntax and black does not fail there:
https://github.com/huggingface/transformers/blob/cb3c2212c79d7ff0a4a4e84c3db48371ecc1c15d/src/transformers/trainer.py#L95
I googled quite a bit about black & typing hints yesterday and didn't find anything useful.
Any ideas @thomwolf @julien-c @LysandreJik ? |
https://github.com/huggingface/datasets/pull/13 | [Make style] | > I think this can be quickly reproduced.
> I use `black, version 19.10b0`.
>
> When running:
> `black nlp/src/arrow_reader.py`
> it gives me:
>
> ```
> error: cannot format /home/patrick/hugging_face/nlp/src/nlp/arrow_reader.py: cannot use --safe with this file; failed to parse source file. AST error message: invalid syntax (<unknown>, line 78)
> Oh no! π₯ π π₯
> 1 file failed to reformat.
> ```
>
> The line in question is:
> https://github.com/huggingface/nlp/blob/6922a16705e61f9e31a365f2606090b84d49241f/src/nlp/arrow_reader.py#L78
>
> What is weird is that the trainer file in `transformers` has more or less the same syntax and black does not fail there:
> https://github.com/huggingface/transformers/blob/cb3c2212c79d7ff0a4a4e84c3db48371ecc1c15d/src/transformers/trainer.py#L95
>
> I googled quite a bit about black & typing hints yesterday and didn't find anything useful.
> Any ideas @thomwolf @julien-c @LysandreJik ?
Ok I found the problem. It was the one Julien mentioned and has nothing to do with this line. Black's error message is a bit misleading here, I guess | Added Makefile and applied make style to all.
make style runs the following code:
```
style:
black --line-length 119 --target-version py35 src
isort --recursive src
```
It's the same code that is run in `transformers`. | 156 | text: [Make style]
Added Makefile and applied make style to all.
make style runs the following code:
```
style:
black --line-length 119 --target-version py35 src
isort --recursive src
```
It's the same code that is run in `transformers`.
> I think this can be quickly reproduced.
> I use `black, version 19.10b0`.
>
> When running:
> `black nlp/src/arrow_reader.py`
> it gives me:
>
> ```
> error: cannot format /home/patrick/hugging_face/nlp/src/nlp/arrow_reader.py: cannot use --safe with this file; failed to parse source file. AST error message: invalid syntax (<unknown>, line 78)
> Oh no! π₯ π π₯
> 1 file failed to reformat.
> ```
>
> The line in question is:
> https://github.com/huggingface/nlp/blob/6922a16705e61f9e31a365f2606090b84d49241f/src/nlp/arrow_reader.py#L78
>
> What is weird is that the trainer file in `transformers` has more or less the same syntax and black does not fail there:
> https://github.com/huggingface/transformers/blob/cb3c2212c79d7ff0a4a4e84c3db48371ecc1c15d/src/transformers/trainer.py#L95
>
> I googled quite a bit about black & typing hints yesterday and didn't find anything useful.
> Any ideas @thomwolf @julien-c @LysandreJik ?
Ok I found the problem. It was the one Julien mentioned and has nothing to do with this line. Black's error message is a bit misleading here, I guess |
https://github.com/huggingface/datasets/pull/13 | [Make style] | Ok, just had to remove the python 2 syntax comments `# type`.
Good to merge for me now @thomwolf | Added Makefile and applied make style to all.
make style runs the following code:
```
style:
black --line-length 119 --target-version py35 src
isort --recursive src
```
It's the same code that is run in `transformers`. | 19 | text: [Make style]
Added Makefile and applied make style to all.
make style runs the following code:
```
style:
black --line-length 119 --target-version py35 src
isort --recursive src
```
It's the same code that is run in `transformers`.
Ok, just had to remove the python 2 syntax comments `# type`.
Good to merge for me now @thomwolf |
https://github.com/huggingface/datasets/pull/12 | [Map Function] add assert statement if map function does not return dict or None | Also added to an assert statement that if a dict is returned by function, all values of `dicts` are `lists` | IMO, if a function is provided that is not a print statement (-> returns variable of type `None`) or a function that updates the datasets (-> returns variable of type `dict`), then a `TypeError` should be raised.
Not sure whether you had cases in mind where the user should do something else @thomwolf , but I think a lot of silent errors can be avoided with this assert statement. | 20 | text: [Map Function] add assert statement if map function does not return dict or None
IMO, if a function is provided that is not a print statement (-> returns variable of type `None`) or a function that updates the datasets (-> returns variable of type `dict`), then a `TypeError` should be raised.
Not sure whether you had cases in mind where the user should do something else @thomwolf , but I think a lot of silent errors can be avoided with this assert statement.
Also added to an assert statement that if a dict is returned by function, all values of `dicts` are `lists` |
https://github.com/huggingface/datasets/pull/12 | [Map Function] add assert statement if map function does not return dict or None | Updated the assert statements. Played around with multiple cases and it should be good now IMO. | IMO, if a function is provided that is not a print statement (-> returns variable of type `None`) or a function that updates the datasets (-> returns variable of type `dict`), then a `TypeError` should be raised.
Not sure whether you had cases in mind where the user should do something else @thomwolf , but I think a lot of silent errors can be avoided with this assert statement. | 16 | text: [Map Function] add assert statement if map function does not return dict or None
IMO, if a function is provided that is not a print statement (-> returns variable of type `None`) or a function that updates the datasets (-> returns variable of type `dict`), then a `TypeError` should be raised.
Not sure whether you had cases in mind where the user should do something else @thomwolf , but I think a lot of silent errors can be avoided with this assert statement.
Updated the assert statements. Played around with multiple cases and it should be good now IMO. |