File size: 4,323 Bytes
7c93e9d |
1 2 3 4 5 6 7 8 9 10 11 12 13 14 15 16 17 18 19 20 21 22 23 24 25 26 27 28 29 30 31 32 33 34 35 36 37 38 39 40 41 42 43 44 45 46 47 48 49 50 51 52 53 54 55 56 57 58 59 60 61 62 63 64 65 66 67 68 69 70 71 72 73 74 75 76 77 78 79 80 81 82 83 84 |
# 概述
在微调完成后,我们通过以下方法对数据进行了测试
数据集1:Cosmos QA(包含35.6K个问题的多项选择阅读理解数据集)
▪ 下载地址:https://wilburone.github.io/cosmos/
▪ 测评⽅法:将测试结果上传到 https://leaderboard.allenai.org/cosmosqa/submission/create ◦
数据集2:TrivailQA (基于维基百科和⽹络收集的阅读理解问答数据集)
▪ 下载地址:Download TriviaQA version 1.0 for RC (2.5G)
▪ 测评⽅法:使⽤官⽅仓库中的triviaqa_evaluation.py
(由于官方仓库的测试集形式和本人的不太一样,因此代码上做了专门的修改,不过核心metrics保持不变,仍是Exact完全匹配数与F1参数)
## CosmosQA 实验结果
### 消融研究
| Model | Score | Comment |
|-----------------------|--------|-------------------------------|
| Fewshot MiniCPM | 0.3251 | 原模型,Fewshot |
| Fewshot LoRA MiniCPM | 0.7773 | 微调模型,Fewshot |
| Fewshot CoT LoRA MiniCPM | 0.7790 | 微调模型,Fewshot,思维链 |
| CoT LoRA MiniCPM | 0.8211 | 微调模型,ZeroShot,思维链 |
| ZH LoRA MiniCPM | 0.8215 | 微调模型,ZeroShot,中文提示词|
| LoRA MiniCPM | 0.8291 | 微调模型,ZeroShot|
- 我们可以看到基础的MiniCPM基本不具备阅读理解选择能力,在ZeroShot的情况下根本无法完成任务,即使是在Fewshot中也只比纯随机好一点。
- LoRA微调过后的MiniCPM基本可以实现不错的效果,在测评榜单上的成绩是Top36。
- LoRA微调过的在使用中文提示词的情况下,效果基本只有微小的减损,可以达到Top42。
- 也许是小模型对于提示词和Fewshot的接收能力较低,实验结果,加入Fewshot和CoT,都会让效果得到减损。
### 对比实验
| Model | Score | Comment |
|-------------------|--------|-----------------|
| ChatGPT3.5 | 0.7233 |gpt-3.5-Turbo-0125,FewShot |
| LoRA MiniCPM | 0.8291 |LoRA微调MiniCPM-2b,ZeroShot |
| QLoRA Chatglm3 | 0.8416 |QLoRA微调chatglm3-6b,ZeroShot |
- 我们将微调过后的MiniCPM分别与QLoRA微调的ChatGLM3和ChatGPT3.5进行了对比,事实证明小参数的模型通过指令微调后能够在特定任务上达到超越ChatGPT3.5的效果。
### 实验截图









## TriviaQA 实验结果
实验反复尝试了Fewshot,Zeroshot的LoRA微调MiniCPM-2B,MiniCPM-2B原模型,QLoRA微调ChatGLM3-6B,ChatGLM3-6B,总共八种情况结果都无法得到实际能用的内容。以下是四类模型情况的结果
### MiniCPM-2B LoRA
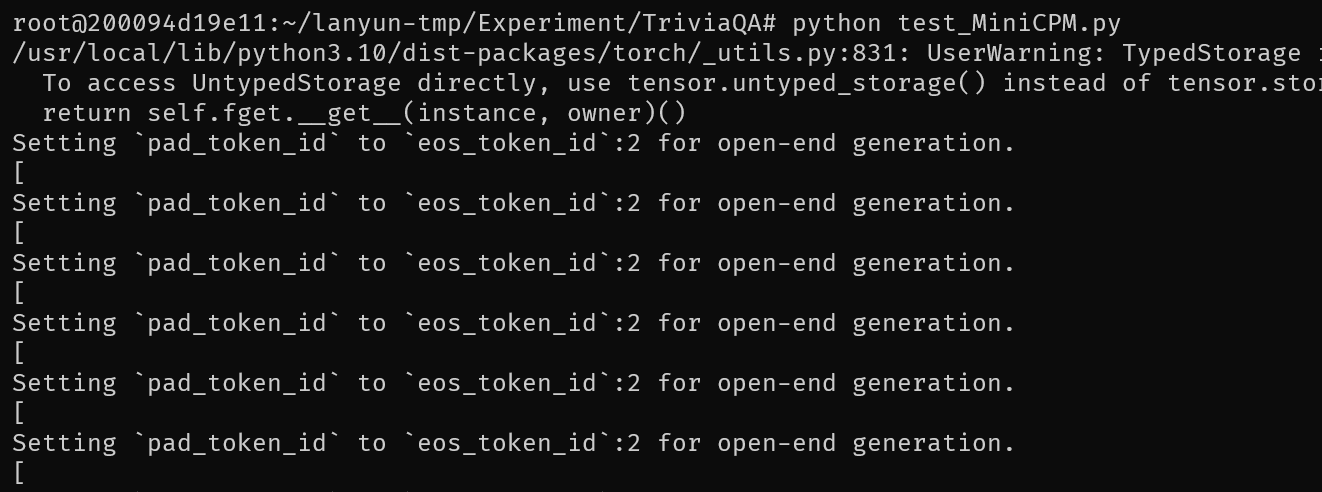
ChatGLM3-6B QLoRA
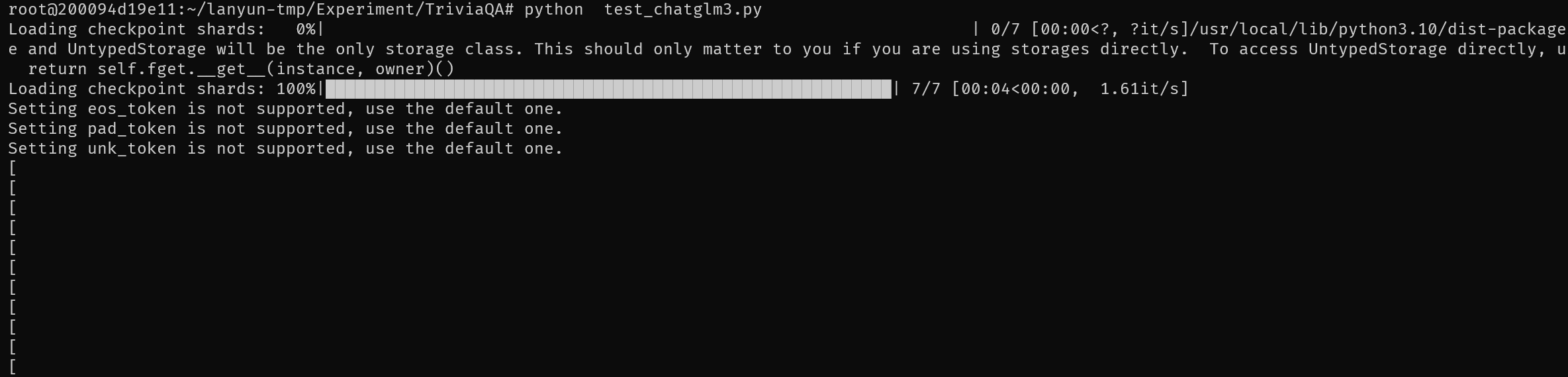
ChatGLM3-6B 原模型
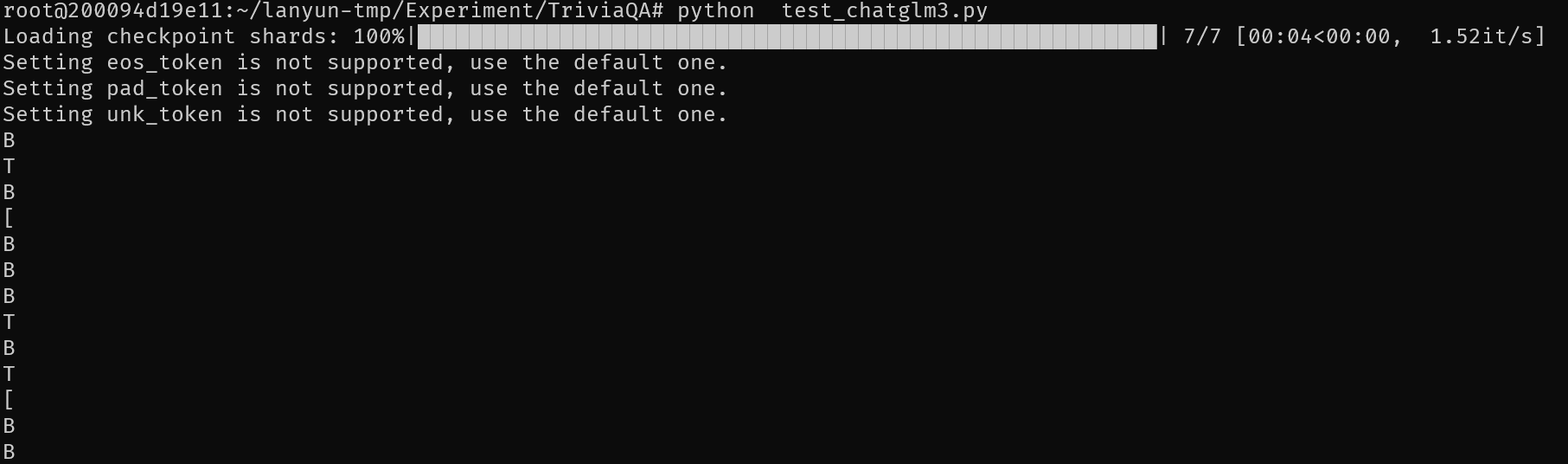
MiniCPM-2B 原模型
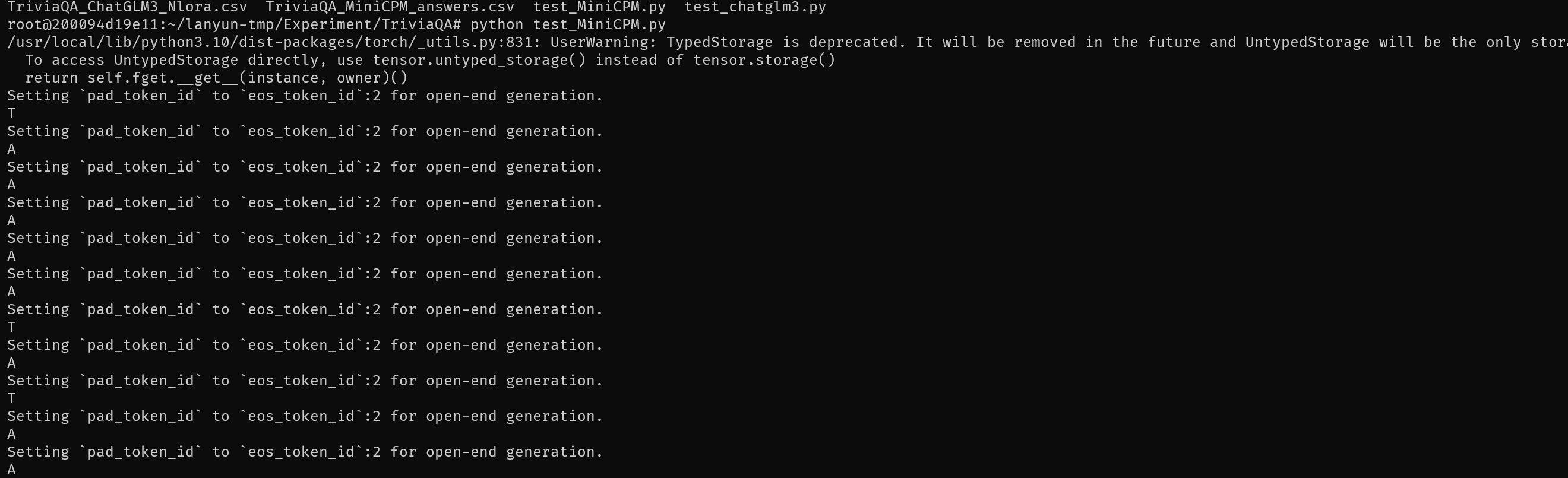
推测是TriviaQA的输入token过多,由于受限于显存大小,我将max_line设置在了512,因此基本无法得到好的效果。
不过本人还是测试了ChatGPT3.5-Turbo的效果,平均F1在0.377,完全匹配率在0.153,1000条的完全错误数只有73个,可以看到在长文阅读理解能力方面还是远超开源小模型的,只是答案不够凝练。
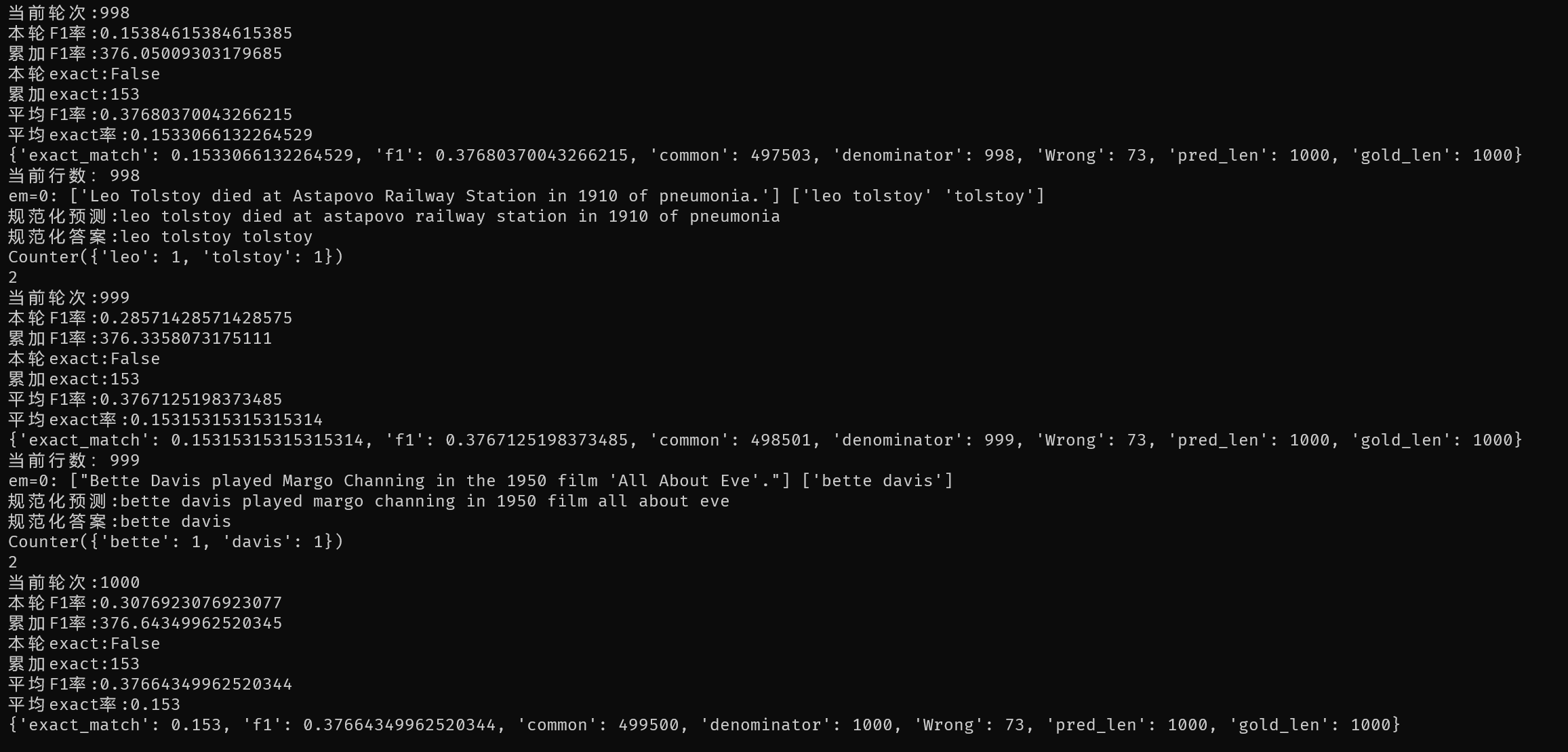
|