Datasets:
Size:
1M<n<10M
ArXiv:
Tags:
programming-language
code
program-synthesis
automatic-code-repair
code-retrieval
code-translation
License:
File size: 9,277 Bytes
b42b91e 1d6da1f b42b91e 6832a65 0c7ebf6 81f0b1d 0c7ebf6 f09b778 0c7ebf6 a211416 0c7ebf6 f09b778 0c7ebf6 56afdcd 0c7ebf6 f09b778 0c7ebf6 f09b778 c9d6bf6 0c7ebf6 aad4f84 0c7ebf6 aad4f84 0c7ebf6 aad4f84 0c7ebf6 aad4f84 0c7ebf6 aad4f84 0c7ebf6 |
1 2 3 4 5 6 7 8 9 10 11 12 13 14 15 16 17 18 19 20 21 22 23 24 25 26 27 28 29 30 31 32 33 34 35 36 37 38 39 40 41 42 43 44 45 46 47 48 49 50 51 52 53 54 55 56 57 58 59 60 61 62 63 64 65 66 67 68 69 70 71 72 73 74 75 76 77 78 79 80 81 82 83 84 85 86 87 88 89 90 91 92 93 94 95 96 97 98 99 100 101 102 103 104 105 106 107 108 109 110 111 112 113 114 115 116 117 118 119 120 121 122 123 124 125 126 127 128 129 130 131 132 133 134 135 136 137 138 139 140 141 142 143 144 145 146 147 148 149 150 151 152 153 154 155 156 157 158 159 160 161 162 163 164 165 166 167 168 169 170 171 172 173 174 175 176 177 178 179 180 181 182 183 184 185 186 187 188 189 190 191 192 193 194 195 196 197 198 199 200 201 202 203 204 |
---
annotations_creators:
- expert-generated
language:
- code
- en
language_creators:
- found
- expert-generated
license:
- cc-by-nc-4.0
multilinguality:
- multilingual
pretty_name: xCodeEval
size_categories:
- 1M<n<10M
- 10M<n<100M
source_datasets:
- original
tags:
- programming-language
- code
- program-synthesis
- automatic-code-repair
- code-retrieval
- code-translation
- code-classification
task_categories:
- translation
- token-classification
- text2text-generation
- text-retrieval
- text-generation
- text-classification
- feature-extraction
- question-answering
---
[github](https://github.com/ntunlp/xCodeEval)
# xCodeEval
[xCodeEval: A Large Scale Multilingual Multitask Benchmark for Code Understanding, Generation, Translation and Retrieval](https://arxiv.org/abs/2303.03004)
We introduce **xCodeEval**, the largest executable multilingual multitask benchmark to date consisting of 25 M document-level coding examples from about 7.5 K unique problems covering up to 17 programming languages with execution-level parallelism. It features a total of seven tasks involving code understanding, generation, translation and retrieval, and it employs an execution-based evaluation. We develop a test-case based multilingual code execution engine, [**ExecEval**](https://github.com/ntunlp/ExecEval) that supports all the programming languages in **xCodeEval**. We also propose a novel data splitting and a data selection schema for balancing data distributions over multiple attributes based on geometric mean and graph-theoretic principle.
This repository contains the sample code and data link for xCodeEval [paper](https://arxiv.org/abs/2303.03004).
# Data Download
Currently this repository supports huggingface [`load_dataset()`](https://huggingface.co/docs/datasets/v1.11.0/package_reference/loading_methods.html#datasets.load_dataset) api. Follow the following example to load dataset for individual examples.
```
import datasets
prog_synthesis_dataset = datasets.load_dataset("NTU-NLP-sg/xCodeEval", "program_synthesis")
code_translation_dataset = datasets.load_dataset("NTU-NLP-sg/xCodeEval", "code_translation")
tag_classification_dataset = datasets.load_dataset("NTU-NLP-sg/xCodeEval", "tag_classification")
apr_dataset = datasets.load_dataset("NTU-NLP-sg/xCodeEval", "apr")
pcode_compilation_dataset = datasets.load_dataset("NTU-NLP-sg/xCodeEval", "code_compilation")
retrieval_code_code_dataset = datasets.load_dataset("NTU-NLP-sg/xCodeEval", "retrieval_code_code")
retrieval_nl_code_dataset = datasets.load_dataset("NTU-NLP-sg/xCodeEval", "retrieval_nl_code")
retrieval_corpus_dataset = datasets.load_dataset("NTU-NLP-sg/xCodeEval", "retrieval_corpus")
```
Data can be also downloaded as a git LFS repo from huggingface.
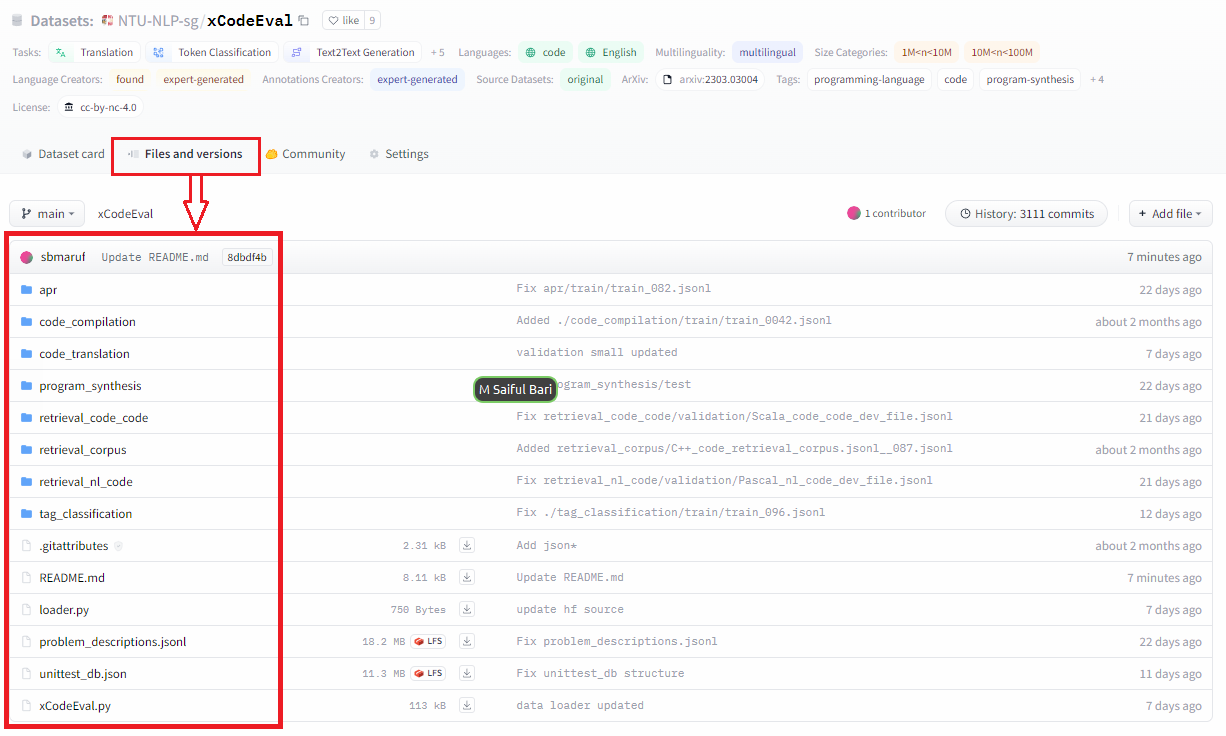
You can download the full data using the following command.
```
GIT_LFS_SKIP_SMUDGE=1 git clone https://huggingface.co/datasets/NTU-NLP-sg/xCodeEval
cd xCodeEval
git lfs pull
```
To download a specific part of the dataset,
```
GIT_LFS_SKIP_SMUDGE=1 git clone https://huggingface.co/datasets/NTU-NLP-sg/xCodeEval
cd xCodeEval
git lfs pull --include "apr/test/*"
```
We propose 7 Tasks.
1. [Tag Classification](https://github.com/ntunlp/xCodeEval/blob/main/apr.md)
2. [Code Compilation](https://github.com/ntunlp/xCodeEval/blob/main/code_compilation.md)
3. [Program Synthesis](https://github.com/ntunlp/xCodeEval/blob/main/program_synthesis.md)
4. [Code Translation](https://github.com/ntunlp/xCodeEval/blob/main/code_translation.md)
5. [Automatic Program Repair](https://github.com/ntunlp/xCodeEval/blob/main/apr.md)
6. [Code-Code Retrieval](https://github.com/ntunlp/xCodeEval/blob/main/retrieval.md)
7. [NL-Code Retrieval](https://github.com/ntunlp/xCodeEval/blob/main/retrieval.md)
# Common Data for different tasks
If you are not using huggingface [`load_dataset()`](https://huggingface.co/docs/datasets/v1.11.0/package_reference/loading_methods.html#datasets.load_dataset) api, you may need to link some data with different tasks.

We have two data files that are required for multiple tasks.
1. `problem_descriptions.jsonl`
2. `unittest_db.json`
You can find these two files in the root directory of the [main](https://huggingface.co/datasets/NTU-NLP-sg/xCodeEval/tree/main) branch of huggingface dataset repository. To avoid data redundancy we didn't include these data with the relevant tasks, rather we add a unique id `src_uid` to retrieve these data.
## Structure of `problem_descriptions.jsonl`
A sample,
```json
{
"description": "There are $$$n$$$ positive integers $$$a_1, a_2, \\dots, a_n$$$. For the one move you can choose any even value $$$c$$$ and divide by two all elements that equal $$$c$$$.For example, if $$$a=[6,8,12,6,3,12]$$$ and you choose $$$c=6$$$, and $$$a$$$ is transformed into $$$a=[3,8,12,3,3,12]$$$ after the move.You need to find the minimal number of moves for transforming $$$a$$$ to an array of only odd integers (each element shouldn't be divisible by $$$2$$$).",
"input_from": "standard input",
"output_to": "standard output",
"time_limit": "3 seconds",
"memory_limit": "256 megabytes",
"input_spec": "The first line of the input contains one integer $$$t$$$ ($$$1 \\le t \\le 10^4$$$) \u2014 the number of test cases in the input. Then $$$t$$$ test cases follow. The first line of a test case contains $$$n$$$ ($$$1 \\le n \\le 2\\cdot10^5$$$) \u2014 the number of integers in the sequence $$$a$$$. The second line contains positive integers $$$a_1, a_2, \\dots, a_n$$$ ($$$1 \\le a_i \\le 10^9$$$). The sum of $$$n$$$ for all test cases in the input doesn't exceed $$$2\\cdot10^5$$$.",
"output_spec": "For $$$t$$$ test cases print the answers in the order of test cases in the input. The answer for the test case is the minimal number of moves needed to make all numbers in the test case odd (i.e. not divisible by $$$2$$$).",
"notes": "NoteIn the first test case of the example, the optimal sequence of moves can be as follows: before making moves $$$a=[40, 6, 40, 3, 20, 1]$$$; choose $$$c=6$$$; now $$$a=[40, 3, 40, 3, 20, 1]$$$; choose $$$c=40$$$; now $$$a=[20, 3, 20, 3, 20, 1]$$$; choose $$$c=20$$$; now $$$a=[10, 3, 10, 3, 10, 1]$$$; choose $$$c=10$$$; now $$$a=[5, 3, 5, 3, 5, 1]$$$ \u2014 all numbers are odd. Thus, all numbers became odd after $$$4$$$ moves. In $$$3$$$ or fewer moves, you cannot make them all odd.",
"sample_inputs": [
"4\n6\n40 6 40 3 20 1\n1\n1024\n4\n2 4 8 16\n3\n3 1 7"
],
"sample_outputs": [
"4\n10\n4\n0"
],
"tags": [
"number theory",
"greedy"
],
"src_uid": "afcd41492158e68095b01ff1e88c3dd4",
"difficulty": 1200,
"created_at": 1576321500
}
```
### Key Definitions
1. `description`: Problem description in textual format, math operations are written in latex.
2. `input_from`: How the program should take the unit test.
3. `output_to`: Where the program should output the result of the unit test.
4. `time_limit`: Time limit to solve the problem.
5. `memory_limit`: Memory limit to solve the problem.
6. `input_spec`: How and in what order the input will be given to the program? It also includes the date range, types, and sizes.
7. `output_spec`: How the outputs should be printed. Most of the time the unit test results are matched with an *exact string match* or *floating point comparison* with a precision boundary.
8. `sample_inputs`: A sample input for the code that is expected to solve the problem described in `description`.
9. `sample_outputs`: The expected output for the `sample_input` that is expected to solve the problem described in `description`.
10. `notes`: Explanation of `sample_inputs` & `sample_outputs`.
11. `tags`: The problem categories.
12. `src_uid`: The unique id of the problem. This ID is referred to in the task data samples instead of putting all this information.
13. `difficulty`: How difficult is it to solve the problem for a human (annotated by an expert human)?
14. `created_at`: The Unix timestamp when the problem was released. Use `datetime` lib in Python to parse it to a human-readable format.
## Structure of `unittest_db.json`
The structure of the `json` file,
```python
unittest_db = {
"db884d679d9cfb1dc4bc511f83beedda" : [
{
"input": "4\r\n3 2 3 2\r\n",
"output": [
"1"
],
},
{
...
},
...
]
"3bc096d8cd3418948d5be6bf297aa9b5":[
...
],
...
}
```
### Key Definitions
1. `unittest_db.json` dict keys i.e., `db884d679d9cfb1dc4bc511f83beedda` are the `src_uid` from `problem_descriptions.jsonl`.
2. `input`: Input of the unit test.
3. `output`: List of expected outputs for the unit test.
# Citation
```
@misc{khan2023xcodeeval,
title={xCodeEval: A Large Scale Multilingual Multitask Benchmark for Code Understanding, Generation, Translation and Retrieval},
author={Mohammad Abdullah Matin Khan and M Saiful Bari and Xuan Long Do and Weishi Wang and Md Rizwan Parvez and Shafiq Joty},
year={2023},
eprint={2303.03004},
archivePrefix={arXiv},
primaryClass={cs.CL}
}
```
|