Datasets:
Tasks:
Question Answering
Sub-tasks:
extractive-qa
Languages:
English
Size:
1K<n<10K
ArXiv:
License:
File size: 21,617 Bytes
9c52d99 6a13b3b 9c52d99 6a13b3b 9c52d99 d3e8ba5 6a3ebf4 63e2429 6a3ebf4 63e2429 6a3ebf4 63e2429 6a3ebf4 63e2429 6a3ebf4 63e2429 6a3ebf4 63e2429 6a3ebf4 9c52d99 1111de4 9c52d99 1111de4 9c52d99 6a3ebf4 |
1 2 3 4 5 6 7 8 9 10 11 12 13 14 15 16 17 18 19 20 21 22 23 24 25 26 27 28 29 30 31 32 33 34 35 36 37 38 39 40 41 42 43 44 45 46 47 48 49 50 51 52 53 54 55 56 57 58 59 60 61 62 63 64 65 66 67 68 69 70 71 72 73 74 75 76 77 78 79 80 81 82 83 84 85 86 87 88 89 90 91 92 93 94 95 96 97 98 99 100 101 102 103 104 105 106 107 108 109 110 111 112 113 114 115 116 117 118 119 120 121 122 123 124 125 126 127 128 129 130 131 132 133 134 135 136 137 138 139 140 141 142 143 144 145 146 147 148 149 150 151 152 153 154 155 156 157 158 159 160 161 162 163 164 165 166 167 168 169 170 171 172 173 174 175 176 177 178 179 180 181 182 183 184 185 186 187 188 189 190 191 192 193 194 195 196 197 198 199 200 201 202 203 204 205 206 207 208 209 210 211 212 213 214 215 216 217 218 219 220 221 222 223 224 225 226 227 228 229 230 231 232 233 234 235 236 237 238 239 240 241 242 243 244 245 246 247 248 249 250 251 252 253 254 255 256 257 258 259 260 261 262 263 264 265 266 267 268 269 270 271 272 273 274 275 276 277 278 279 280 281 282 283 284 285 286 287 288 289 290 291 292 293 294 295 296 297 298 299 300 301 302 303 304 305 306 307 308 309 310 311 312 313 314 315 316 317 318 319 320 321 322 323 324 325 326 327 328 329 330 331 332 333 334 335 336 337 338 339 340 341 342 343 344 345 346 347 348 349 350 351 352 353 354 355 356 357 358 359 360 361 362 363 364 365 366 367 368 369 370 371 372 373 374 375 376 377 378 379 380 381 382 383 384 385 386 387 388 389 390 391 392 393 394 395 396 397 398 399 400 401 402 403 404 405 406 407 408 409 410 411 412 413 414 415 416 417 418 419 420 421 422 423 424 425 426 427 428 429 430 431 432 433 434 435 436 437 438 439 440 441 442 443 444 445 446 447 448 449 450 451 452 453 454 455 456 457 458 459 460 461 462 463 464 465 466 467 468 469 470 471 472 473 474 475 476 477 478 479 480 481 482 483 484 485 486 487 488 489 490 491 492 493 494 495 496 497 498 499 500 501 502 503 504 505 506 507 508 509 510 511 512 513 514 515 516 517 518 519 520 521 522 523 524 525 526 527 528 529 530 531 532 533 534 535 536 537 538 539 540 541 542 543 544 545 546 547 548 549 550 551 552 553 554 555 556 557 558 559 560 561 562 563 564 565 566 567 568 569 570 571 572 573 574 575 576 577 578 579 580 581 582 583 584 585 586 587 588 589 590 591 592 593 594 595 596 597 598 599 600 601 602 603 604 605 606 607 608 609 |
---
annotations_creators:
- expert-generated
language_creators:
- found
language:
- en
license:
- unknown
multilinguality:
- monolingual
size_categories:
- 1K<n<10K
source_datasets:
- original
- extended|yelp_review_full
- extended|other-amazon_reviews_ucsd
- extended|other-tripadvisor_reviews
task_categories:
- question-answering
task_ids:
- extractive-qa
paperswithcode_id: subjqa
pretty_name: subjqa
dataset_info:
- config_name: books
features:
- name: domain
dtype: string
- name: nn_mod
dtype: string
- name: nn_asp
dtype: string
- name: query_mod
dtype: string
- name: query_asp
dtype: string
- name: q_reviews_id
dtype: string
- name: question_subj_level
dtype: int64
- name: ques_subj_score
dtype: float32
- name: is_ques_subjective
dtype: bool
- name: review_id
dtype: string
- name: id
dtype: string
- name: title
dtype: string
- name: context
dtype: string
- name: question
dtype: string
- name: answers
sequence:
- name: text
dtype: string
- name: answer_start
dtype: int32
- name: answer_subj_level
dtype: int64
- name: ans_subj_score
dtype: float32
- name: is_ans_subjective
dtype: bool
splits:
- name: train
num_bytes: 2473128
num_examples: 1314
- name: test
num_bytes: 649413
num_examples: 345
- name: validation
num_bytes: 460214
num_examples: 256
download_size: 11384657
dataset_size: 3582755
- config_name: electronics
features:
- name: domain
dtype: string
- name: nn_mod
dtype: string
- name: nn_asp
dtype: string
- name: query_mod
dtype: string
- name: query_asp
dtype: string
- name: q_reviews_id
dtype: string
- name: question_subj_level
dtype: int64
- name: ques_subj_score
dtype: float32
- name: is_ques_subjective
dtype: bool
- name: review_id
dtype: string
- name: id
dtype: string
- name: title
dtype: string
- name: context
dtype: string
- name: question
dtype: string
- name: answers
sequence:
- name: text
dtype: string
- name: answer_start
dtype: int32
- name: answer_subj_level
dtype: int64
- name: ans_subj_score
dtype: float32
- name: is_ans_subjective
dtype: bool
splits:
- name: train
num_bytes: 2123648
num_examples: 1295
- name: test
num_bytes: 608899
num_examples: 358
- name: validation
num_bytes: 419042
num_examples: 255
download_size: 11384657
dataset_size: 3151589
- config_name: grocery
features:
- name: domain
dtype: string
- name: nn_mod
dtype: string
- name: nn_asp
dtype: string
- name: query_mod
dtype: string
- name: query_asp
dtype: string
- name: q_reviews_id
dtype: string
- name: question_subj_level
dtype: int64
- name: ques_subj_score
dtype: float32
- name: is_ques_subjective
dtype: bool
- name: review_id
dtype: string
- name: id
dtype: string
- name: title
dtype: string
- name: context
dtype: string
- name: question
dtype: string
- name: answers
sequence:
- name: text
dtype: string
- name: answer_start
dtype: int32
- name: answer_subj_level
dtype: int64
- name: ans_subj_score
dtype: float32
- name: is_ans_subjective
dtype: bool
splits:
- name: train
num_bytes: 1317488
num_examples: 1124
- name: test
num_bytes: 721827
num_examples: 591
- name: validation
num_bytes: 254432
num_examples: 218
download_size: 11384657
dataset_size: 2293747
- config_name: movies
features:
- name: domain
dtype: string
- name: nn_mod
dtype: string
- name: nn_asp
dtype: string
- name: query_mod
dtype: string
- name: query_asp
dtype: string
- name: q_reviews_id
dtype: string
- name: question_subj_level
dtype: int64
- name: ques_subj_score
dtype: float32
- name: is_ques_subjective
dtype: bool
- name: review_id
dtype: string
- name: id
dtype: string
- name: title
dtype: string
- name: context
dtype: string
- name: question
dtype: string
- name: answers
sequence:
- name: text
dtype: string
- name: answer_start
dtype: int32
- name: answer_subj_level
dtype: int64
- name: ans_subj_score
dtype: float32
- name: is_ans_subjective
dtype: bool
splits:
- name: train
num_bytes: 2986348
num_examples: 1369
- name: test
num_bytes: 620513
num_examples: 291
- name: validation
num_bytes: 589663
num_examples: 261
download_size: 11384657
dataset_size: 4196524
- config_name: restaurants
features:
- name: domain
dtype: string
- name: nn_mod
dtype: string
- name: nn_asp
dtype: string
- name: query_mod
dtype: string
- name: query_asp
dtype: string
- name: q_reviews_id
dtype: string
- name: question_subj_level
dtype: int64
- name: ques_subj_score
dtype: float32
- name: is_ques_subjective
dtype: bool
- name: review_id
dtype: string
- name: id
dtype: string
- name: title
dtype: string
- name: context
dtype: string
- name: question
dtype: string
- name: answers
sequence:
- name: text
dtype: string
- name: answer_start
dtype: int32
- name: answer_subj_level
dtype: int64
- name: ans_subj_score
dtype: float32
- name: is_ans_subjective
dtype: bool
splits:
- name: train
num_bytes: 1823331
num_examples: 1400
- name: test
num_bytes: 335453
num_examples: 266
- name: validation
num_bytes: 349354
num_examples: 267
download_size: 11384657
dataset_size: 2508138
- config_name: tripadvisor
features:
- name: domain
dtype: string
- name: nn_mod
dtype: string
- name: nn_asp
dtype: string
- name: query_mod
dtype: string
- name: query_asp
dtype: string
- name: q_reviews_id
dtype: string
- name: question_subj_level
dtype: int64
- name: ques_subj_score
dtype: float32
- name: is_ques_subjective
dtype: bool
- name: review_id
dtype: string
- name: id
dtype: string
- name: title
dtype: string
- name: context
dtype: string
- name: question
dtype: string
- name: answers
sequence:
- name: text
dtype: string
- name: answer_start
dtype: int32
- name: answer_subj_level
dtype: int64
- name: ans_subj_score
dtype: float32
- name: is_ans_subjective
dtype: bool
splits:
- name: train
num_bytes: 1575021
num_examples: 1165
- name: test
num_bytes: 689508
num_examples: 512
- name: validation
num_bytes: 312645
num_examples: 230
download_size: 11384657
dataset_size: 2577174
---
# Dataset Card for subjqa
## Table of Contents
- [Dataset Description](#dataset-description)
- [Dataset Summary](#dataset-summary)
- [Supported Tasks and Leaderboards](#supported-tasks-and-leaderboards)
- [Languages](#languages)
- [Dataset Structure](#dataset-structure)
- [Data Instances](#data-instances)
- [Data Fields](#data-fields)
- [Data Splits](#data-splits)
- [Dataset Creation](#dataset-creation)
- [Curation Rationale](#curation-rationale)
- [Source Data](#source-data)
- [Annotations](#annotations)
- [Personal and Sensitive Information](#personal-and-sensitive-information)
- [Considerations for Using the Data](#considerations-for-using-the-data)
- [Social Impact of Dataset](#social-impact-of-dataset)
- [Discussion of Biases](#discussion-of-biases)
- [Other Known Limitations](#other-known-limitations)
- [Additional Information](#additional-information)
- [Dataset Curators](#dataset-curators)
- [Licensing Information](#licensing-information)
- [Citation Information](#citation-information)
- [Contributions](#contributions)
## Dataset Description
- **Repository:** https://github.com/lewtun/SubjQA
- **Paper:** https://arxiv.org/abs/2004.14283
- **Point of Contact:** [Lewis Tunstall](mailto:lewis.c.tunstall@gmail.com)
### Dataset Summary
SubjQA is a question answering dataset that focuses on subjective (as opposed to factual) questions and answers. The dataset consists of roughly **10,000** questions over reviews from 6 different domains: books, movies, grocery, electronics, TripAdvisor (i.e. hotels), and restaurants. Each question is paired with a review and a span is highlighted as the answer to the question (with some questions having no answer). Moreover, both questions and answer spans are assigned a _subjectivity_ label by annotators. Questions such as _"How much does this product weigh?"_ is a factual question (i.e., low subjectivity), while "Is this easy to use?" is a subjective question (i.e., high subjectivity).
In short, SubjQA provides a setting to study how well extractive QA systems perform on finding answer that are less factual and to what extent modeling subjectivity can improve the performance of QA systems.
_Note:_ Much of the information provided on this dataset card is taken from the README provided by the authors in their GitHub repository ([link](https://github.com/megagonlabs/SubjQA)).
To load a domain with `datasets` you can run the following:
```python
from datasets import load_dataset
# other options include: electronics, grocery, movies, restaurants, tripadvisor
dataset = load_dataset("subjqa", "books")
```
### Supported Tasks and Leaderboards
* `question-answering`: The dataset can be used to train a model for extractive question answering, which involves questions whose answer can be identified as a span of text in a review. Success on this task is typically measured by achieving a high Exact Match or F1 score. The BERT model that is first fine-tuned on SQuAD 2.0 and then further fine-tuned on SubjQA achieves the scores shown in the figure below.
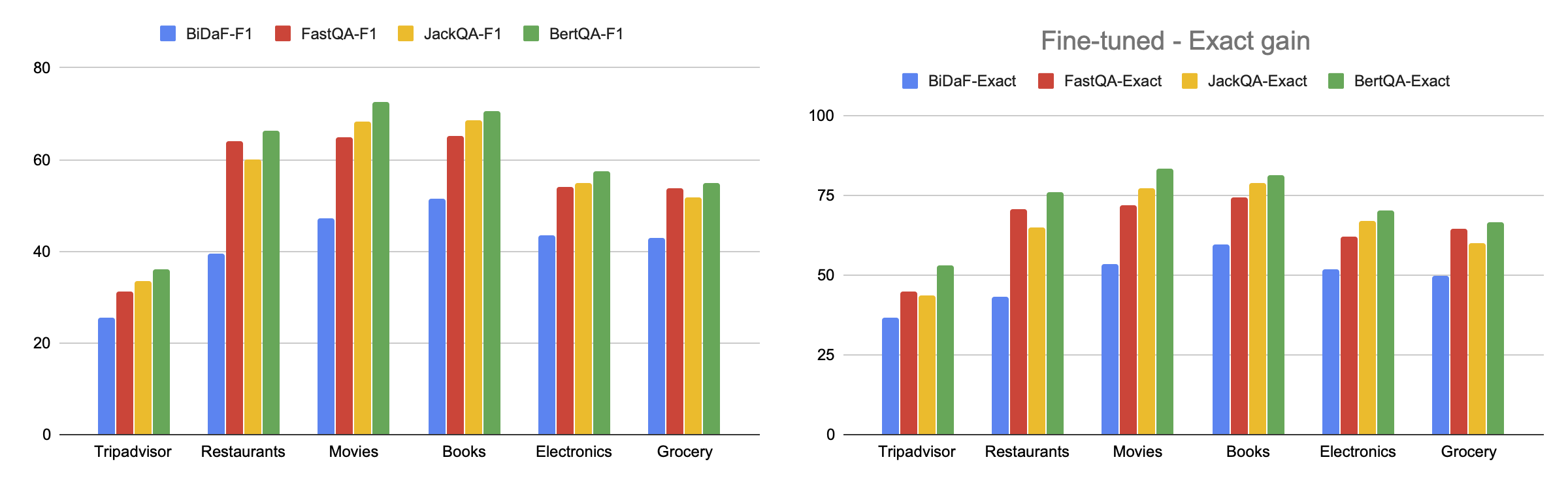
### Languages
The text in the dataset is in English and the associated BCP-47 code is `en`.
## Dataset Structure
### Data Instances
An example from `books` domain is shown below:
```json
{
"answers": {
"ans_subj_score": [1.0],
"answer_start": [324],
"answer_subj_level": [2],
"is_ans_subjective": [true],
"text": ["This is a wonderfully written book"],
},
"context": "While I would not recommend this book to a young reader due to a couple pretty explicate scenes I would recommend it to any adult who just loves a good book. Once I started reading it I could not put it down. I hesitated reading it because I didn't think that the subject matter would be interesting, but I was so wrong. This is a wonderfully written book.",
"domain": "books",
"id": "0255768496a256c5ed7caed9d4e47e4c",
"is_ques_subjective": false,
"nn_asp": "matter",
"nn_mod": "interesting",
"q_reviews_id": "a907837bafe847039c8da374a144bff9",
"query_asp": "part",
"query_mod": "fascinating",
"ques_subj_score": 0.0,
"question": "What are the parts like?",
"question_subj_level": 2,
"review_id": "a7f1a2503eac2580a0ebbc1d24fffca1",
"title": "0002007770",
}
```
### Data Fields
Each domain and split consists of the following columns:
* ```title```: The id of the item/business discussed in the review.
* ```question```: The question (written based on a query opinion).
* ```id```: A unique id assigned to the question-review pair.
* ```q_reviews_id```: A unique id assigned to all question-review pairs with a shared question.
* ```question_subj_level```: The subjectivity level of the question (on a 1 to 5 scale with 1 being the most subjective).
* ```ques_subj_score```: The subjectivity score of the question computed using the [TextBlob](https://textblob.readthedocs.io/en/dev/) package.
* ```context```: The review (that mentions the neighboring opinion).
* ```review_id```: A unique id associated with the review.
* ```answers.text```: The span labeled by annotators as the answer.
* ```answers.answer_start```: The (character-level) start index of the answer span highlighted by annotators.
* ```is_ques_subjective```: A boolean subjectivity label derived from ```question_subj_level``` (i.e., scores below 4 are considered as subjective)
* ```answers.answer_subj_level```: The subjectivity level of the answer span (on a 1 to 5 scale with 1 being the most subjective).
* ```answers.ans_subj_score```: The subjectivity score of the answer span computed usign the [TextBlob](https://textblob.readthedocs.io/en/dev/) package.
* ```answers.is_ans_subjective```: A boolean subjectivity label derived from ```answer_subj_level``` (i.e., scores below 4 are considered as subjective)
* ```domain```: The category/domain of the review (e.g., hotels, books, ...).
* ```nn_mod```: The modifier of the neighboring opinion (which appears in the review).
* ```nn_asp```: The aspect of the neighboring opinion (which appears in the review).
* ```query_mod```: The modifier of the query opinion (around which a question is manually written).
* ```query_asp```: The aspect of the query opinion (around which a question is manually written).
### Data Splits
The question-review pairs from each domain are split into training, development, and test sets. The table below shows the size of the dataset per each domain and split.
| Domain | Train | Dev | Test | Total |
|-------------|-------|-----|------|-------|
| TripAdvisor | 1165 | 230 | 512 | 1686 |
| Restaurants | 1400 | 267 | 266 | 1683 |
| Movies | 1369 | 261 | 291 | 1677 |
| Books | 1314 | 256 | 345 | 1668 |
| Electronics | 1295 | 255 | 358 | 1659 |
| Grocery | 1124 | 218 | 591 | 1725 |
Based on the subjectivity labels provided by annotators, one observes that 73% of the questions and 74% of the answers in the dataset are subjective. This provides a substantial number of subjective QA pairs as well as a reasonable number of factual questions to compare and constrast the performance of QA systems on each type of QA pairs.
Finally, the next table summarizes the average length of the question, the review, and the highlighted answer span for each category.
| Domain | Review Len | Question Len | Answer Len | % answerable |
|-------------|------------|--------------|------------|--------------|
| TripAdvisor | 187.25 | 5.66 | 6.71 | 78.17 |
| Restaurants | 185.40 | 5.44 | 6.67 | 60.72 |
| Movies | 331.56 | 5.59 | 7.32 | 55.69 |
| Books | 285.47 | 5.78 | 7.78 | 52.99 |
| Electronics | 249.44 | 5.56 | 6.98 | 58.89 |
| Grocery | 164.75 | 5.44 | 7.25 | 64.69 |
## Dataset Creation
### Curation Rationale
Most question-answering datasets like SQuAD and Natural Questions focus on answering questions over factual data such as Wikipedia and news articles. However, in domains like e-commerce the questions and answers are often _subjective_, that is, they depend on the personal experience of the users. For example, a customer on Amazon may ask "Is the sound quality any good?", which is more difficult to answer than a factoid question like "What is the capital of Australia?" These considerations motivate the creation of SubjQA as a tool to investigate the relationship between subjectivity and question-answering.
### Source Data
#### Initial Data Collection and Normalization
The SubjQA dataset is constructed based on publicly available review datasets. Specifically, the _movies_, _books_, _electronics_, and _grocery_ categories are constructed using reviews from the [Amazon Review dataset](http://jmcauley.ucsd.edu/data/amazon/links.html). The _TripAdvisor_ category, as the name suggests, is constructed using reviews from TripAdvisor which can be found [here](http://times.cs.uiuc.edu/~wang296/Data/). Finally, the _restaurants_ category is constructed using the [Yelp Dataset](https://www.yelp.com/dataset) which is also publicly available.
The process of constructing SubjQA is discussed in detail in the [paper](https://arxiv.org/abs/2004.14283). In a nutshell, the dataset construction consists of the following steps:
1. First, all _opinions_ expressed in reviews are extracted. In the pipeline, each opinion is modeled as a (_modifier_, _aspect_) pair which is a pair of spans where the former describes the latter. (good, hotel), and (terrible, acting) are a few examples of extracted opinions.
2. Using Matrix Factorization techniques, implication relationships between different expressed opinions are mined. For instance, the system mines that "responsive keys" implies "good keyboard". In our pipeline, we refer to the conclusion of an implication (i.e., "good keyboard" in this examples) as the _query_ opinion, and we refer to the premise (i.e., "responsive keys") as its _neighboring_ opinion.
3. Annotators are then asked to write a question based on _query_ opinions. For instance given "good keyboard" as the query opinion, they might write "Is this keyboard any good?"
4. Each question written based on a _query_ opinion is then paired with a review that mentions its _neighboring_ opinion. In our example, that would be a review that mentions "responsive keys".
5. The question and review pairs are presented to annotators to select the correct answer span, and rate the subjectivity level of the question as well as the subjectivity level of the highlighted answer span.
A visualisation of the data collection pipeline is shown in the image below.
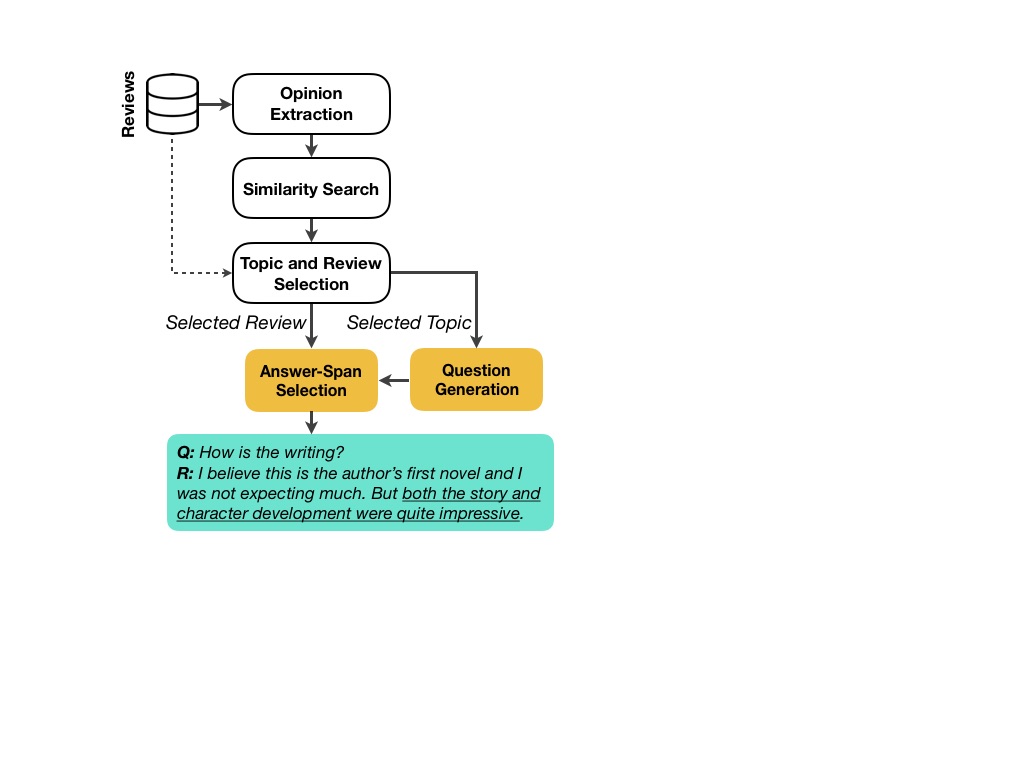
#### Who are the source language producers?
As described above, the source data for SubjQA is customer reviews of products and services on e-commerce websites like Amazon and TripAdvisor.
### Annotations
#### Annotation process
The generation of questions and answer span labels were obtained through the [Appen](https://appen.com/) platform. From the SubjQA paper:
> The platform provides quality control by showing the workers 5 questions at a time, out of which one is labeled by the experts. A worker who fails to maintain 70% accuracy is kicked out by the platform and his judgements are ignored ... To ensure good quality labels, we paid each worker 5 cents per annotation.
The instructions for generating a question are shown in the following figure:
<img width="874" alt="ques_gen" src="https://user-images.githubusercontent.com/26859204/117259092-03d67300-ae4e-11eb-81f2-9077fee1085f.png">
Similarly, the interface for the answer span and subjectivity labelling tasks is shown below:
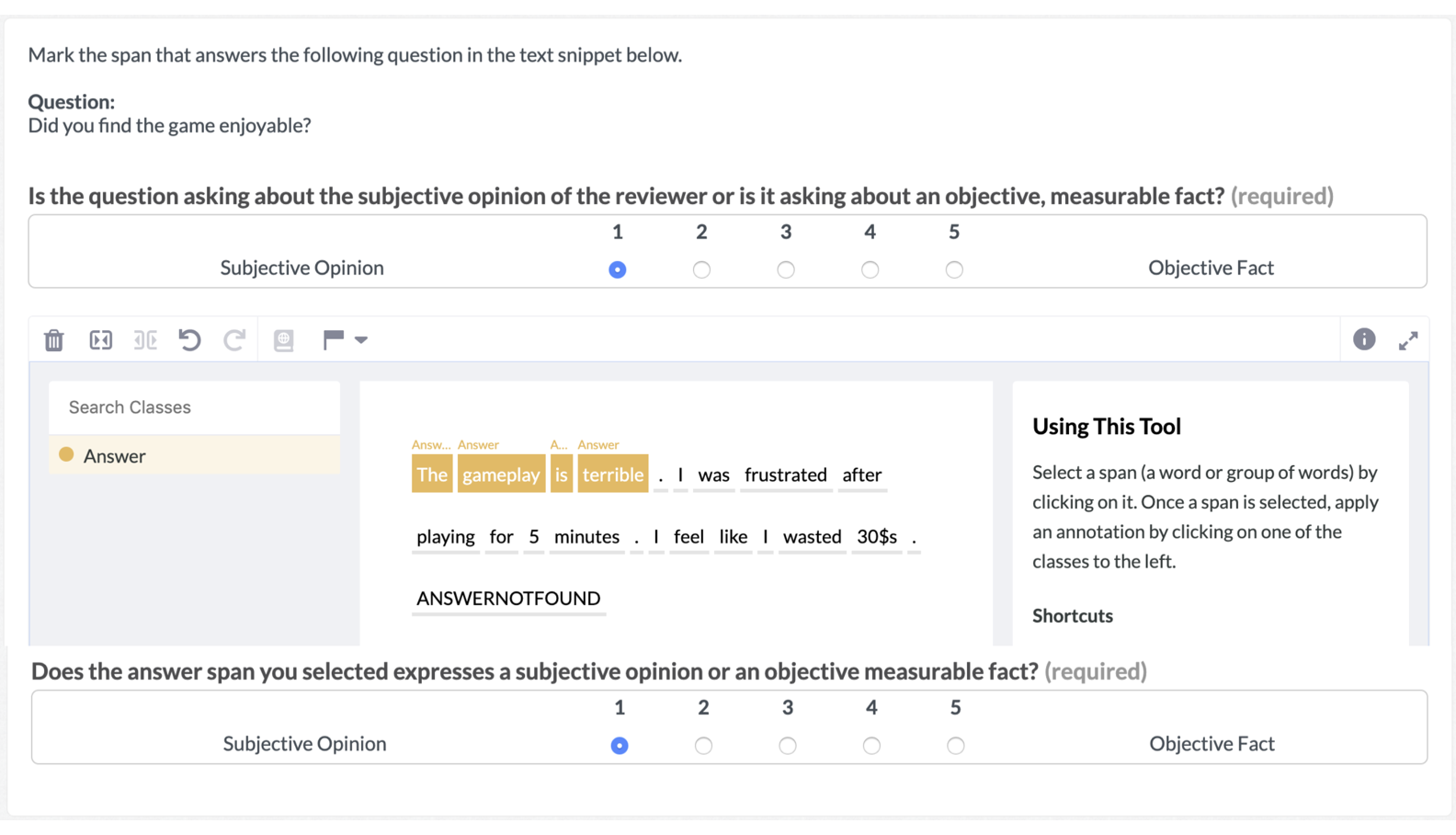
As described in the SubjQA paper, the workers assign subjectivity scores (1-5) to each question and the selected answer span. They can also indicate if a question cannot be answered from the given review.
#### Who are the annotators?
Workers on the Appen platform.
### Personal and Sensitive Information
[Needs More Information]
## Considerations for Using the Data
### Social Impact of Dataset
The SubjQA dataset can be used to develop question-answering systems that can provide better on-demand answers to e-commerce customers who are interested in subjective questions about products and services.
### Discussion of Biases
[Needs More Information]
### Other Known Limitations
[Needs More Information]
## Additional Information
### Dataset Curators
The people involved in creating the SubjQA dataset are the authors of the accompanying paper:
* Johannes Bjerva1, Department of Computer Science, University of Copenhagen, Department of Computer Science, Aalborg University
* Nikita Bhutani, Megagon Labs, Mountain View
* Behzad Golshan, Megagon Labs, Mountain View
* Wang-Chiew Tan, Megagon Labs, Mountain View
* Isabelle Augenstein, Department of Computer Science, University of Copenhagen
### Licensing Information
The SubjQA dataset is provided "as-is", and its creators make no representation as to its accuracy.
The SubjQA dataset is constructed based on the following datasets and thus contains subsets of their data:
* [Amazon Review Dataset](http://jmcauley.ucsd.edu/data/amazon/links.html) from UCSD
* Used for _books_, _movies_, _grocery_, and _electronics_ domains
* [The TripAdvisor Dataset](http://times.cs.uiuc.edu/~wang296/Data/) from UIUC's Database and Information Systems Laboratory
* Used for the _TripAdvisor_ domain
* [The Yelp Dataset](https://www.yelp.com/dataset)
* Used for the _restaurants_ domain
Consequently, the data within each domain of the SubjQA dataset should be considered under the same license as the dataset it was built upon.
### Citation Information
If you are using the dataset, please cite the following in your work:
```
@inproceedings{bjerva20subjqa,
title = "SubjQA: A Dataset for Subjectivity and Review Comprehension",
author = "Bjerva, Johannes and
Bhutani, Nikita and
Golahn, Behzad and
Tan, Wang-Chiew and
Augenstein, Isabelle",
booktitle = "Proceedings of the 2020 Conference on Empirical Methods in Natural Language Processing",
month = November,
year = "2020",
publisher = "Association for Computational Linguistics",
}
```
### Contributions
Thanks to [@lewtun](https://github.com/lewtun) for adding this dataset. |