grimjim/Magnolia-v3-12B
Jim Lai
AI & ML interests
Recent Activity
Organizations
grimjim's activity
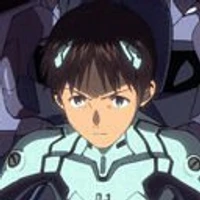
grimjim/Magnolia-v3-12B
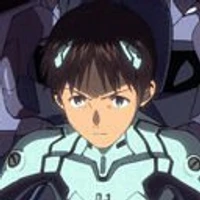
https://github.com/turboderp/exllamav2
meta-llama/Llama-3.2-1B-Instruct
grimjim/llama-3-Nephilim-v3-8B
The proof-of-concept Python script compared a zero-shot creative task of writing a story limited to 500 tokens. Speculative decoding improved performance by approximately one third (e.g., increasing from 31 tokens/sec to 46 tokens/sec) over conventional decoding, and was consistent over a few runs. While not statistically significant, this implies that smaller models aimed at edge computing can serve effectively as draft models in the general case.
It is straightforward to consult literature to affirm that fine-tuning draft models can be a way of inducing behavioral change in target models, in a manner not unlike how samplers can be used to induce changes. I speculate that the impact of a fine-tuned draft model would be on part with a LoRA (Low-Rank Adaptation), as the target model retains veto power. The small size of draft model candidates means that more people can perform local full fine-tuning.
It is intuitively obvious that a distilled model can be used as a draft model for the larger teacher model so long as tokenizers line up; e.g., a distilled 8B model can draft for a 70B teacher model. Perhaps Llama-3.1-SuperNova-Lite 8B could effectively draft for the original Llama-3.1-405B-Instruct model.
arcee-ai/Llama-3.1-SuperNova-Lite
meta-llama/Llama-3.1-405B-Instruct
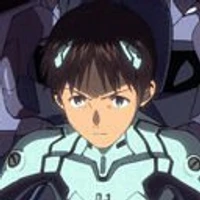
grimjim/Llama-Nephilim-Metamorphosis-v2-8B
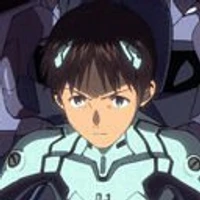
Those look like prefills. Unless you want to train for prefill-specific outputs, it makes sense to remove them.
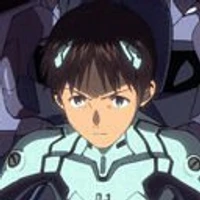
For DPO, I'd stick with what HF recommends, which in their example does not have prompt repetition.
https://huggingface.co/docs/trl/main/en/dpo_trainer
Offhand, for multi-turn data, I'd go with what the LLM "sees" in practice, so prior turns are probably part of the prompt, and "chosen" and "rejected" guide what text generation occurs.
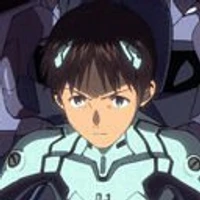
https://arxiv.org/abs/2408.14774
In particular, the observation that "Introducing low quality answers ("shirkers") in 20% of Instruct-SkillMix examples causes performance to plummet..." had me wondering how many ostensibly good datasets out there are in fact populated with a significant number of "shirkers".
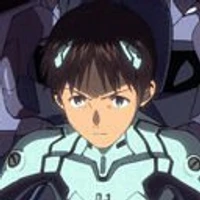
https://arxiv.org/abs/2408.16737
The direct implication is that smaller models could be used to create cost-effective synthetic datasets. And on that note, in the Gemma terms of use, Google explicitly claims no rights on outputs generated from those models, which means one is free to synthgen from the Gemma line. Meta's Llama 3 licence forbids synthetic generation of outputs if used to improve other models. Relevant Mistral, Qwen, and Yi models under the Apache 2.0 license are unrestricted for this purpose.
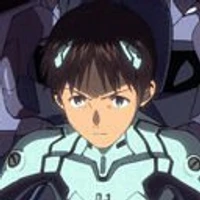
grimjim/Kitsunebi-v1-Gemma2-8k-9B
grimjim/Kitsunebi-v1-Gemma2-8k-9B-GGUF
I opted not to incorporate the UCLA SPPO fine-tune for Gemma2 9B after observing context confusion occur with some frequency during complex scenarios.
Thanks to Axcxept co., ltd. for fine-tuning HODACHI/EZO-Common-9B-gemma-2-it, and to Princeton NLP Group for fine-tuning princeton-nlp/gemma-2-9b-it-SimPO.
AXCXEPT/EZO-Common-9B-gemma-2-it
princeton-nlp/gemma-2-9b-it-SimPO
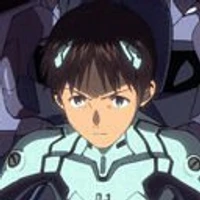
Taking a cue from the paper "The Unreasonable Ineffectiveness of the Deeper Layers" ( https://arxiv.org/abs/2403.17887 ) and PruneMe (https://github.com/arcee-ai/PruneMe), it seems reasonable to target deeper layers identified as more redundant given measured similarity across layers, as the result should be less damaging to models, reducing the need for subsequent fine-tuning. Intuitively, one should expect the resulting intervention layers to be deep but not final. The only uncertainty is if the redundancy successfully encodes refusals, something which is almost certainly model-dependent. This approach only requires the redundancy to be computed once per model, and the result used as a starting point for which layer range to restrict intervention to.
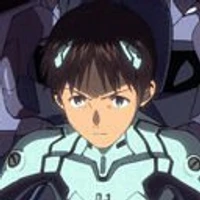
https://arxiv.org/abs/2402.17762
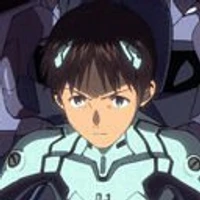
Not the same model, but a related model.
I created what amounted to an abliteration LoRA by contrasting original L3 Instruct against failspy's abliterated L3 Instruct, then applied and merged the L3-derived LoRA on top of original L3.1 Instruct to obtain the final model.
The result appears to outperform mlabonne's reapplication of the abliteration technique directly to L3.1 Instruct.
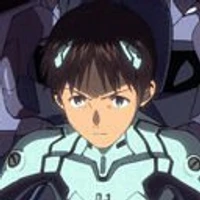
An example use of mergekit to apply the lora is documented at the bottom of the model card as well as in mergekit_config.yaml. Although task_arithmetic was used, a passthrough merge will work as well.
An example of the mergekit LoRA extraction command is at the bottom of the model card for the LoRA:
https://huggingface.co/grimjim/Llama-3-Instruct-abliteration-LoRA-8B
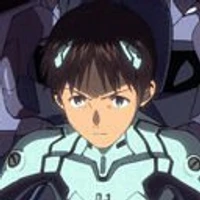
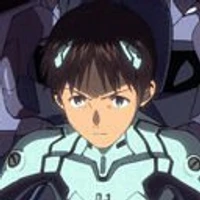
Proof of concept below:
grimjim/Llama-3.1-8B-Instruct-abliterated_via_adapter
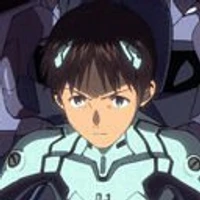
Roleplay is overlooked as a special case of chain-of-thought, where context must be attended to and inferred state of the world and embodied minds must be persisted and evolved along credible narrative lines. LLMs are also being tasked to function as gamemasters. It's a challenging task which points to potential future benchmarks. The fact that the largest commercial LLMs are adept in generating text for roleplay intuitively implies that model intelligence is sufficient so long as it can generalize properly and pay attention to context without becoming confused.
This recent merge of mine composed using 3 academic fine-tunes, none of which were intended for roleplay, has survived the gauntlet of a Reddit post and appears to be a particularly strong 8B model when it comes to roleplay coherence.
grimjim/llama-3-Nephilim-v3-8B (bf16 weights)
grimjim/llama-3-Nephilim-v3-8B-GGUF (select quants)
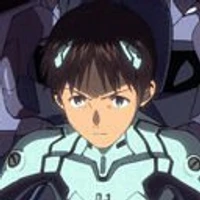
Something odd is happening when merging with the OVA model. It will reduce refusals at medium (0.5-0.6) weight, but at full (1.0) weight against Instruct 8B, the result is incoherent. The LoRA should work, though!
It should also be possible to modify abliteration scripts to instead directly produce a LoRA as output.
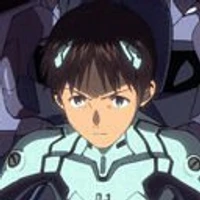
This model is steered to behave opposite to what MopeyMule demonstrated.
Based on the implications of the merge technique, we also propose Orthogonalized Vector Adaptation (OVA). We also extract a LoRA of the counter-refusal abliteration steering vector.
The resulting merger is not a perfect model, but it's a behaviorally interesting model. The model name was inspired by a Philip K. Dick story.
grimjim/Llama-3-Perky-Pat-Instruct-8B
Refusal vector weights ready for use:
grimjim/Llama-3-Instruct-abliteration-OVA-8B
grimjim/Llama-3-Instruct-abliteration-LoRA-8B
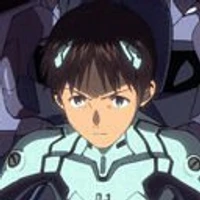
grimjim/Llama-3-Instruct-8B-SPPO-Iter3-SimPO-merge
grimjim/Llama-3-Instruct-8B-SimPO-SPPO-Iter3-merge
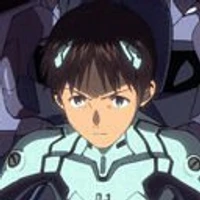
grimjim/kukulemon-v3-soul_mix-32k-7B
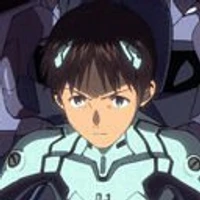
grimjim/kunoichi-lemon-royale-v3-32K-7B