File size: 19,680 Bytes
72644d2 53833c8 72644d2 53833c8 9712961 53833c8 7e52af9 53833c8 f672d78 bedafd6 5b5d33a f672d78 bedafd6 f672d78 bedafd6 f672d78 9712961 2bf0d3a 87fac66 b4823b5 87fac66 83dca2e ed74716 f635c61 ed74716 f635c61 ed74716 0009f1d bedafd6 9712961 de4a37e 53833c8 d8044b7 53833c8 82c4f4d 53833c8 82c4f4d 53833c8 82c4f4d 53833c8 82c4f4d ca7e615 82c4f4d 35dc3d5 82c4f4d ca7e615 82c4f4d 35dc3d5 82c4f4d ca7e615 b4823b5 ca7e615 b4823b5 35dc3d5 b4823b5 ca7e615 b4823b5 35dc3d5 b4823b5 ca7e615 b4823b5 35dc3d5 b4823b5 ca7e615 2006ec5 35dc3d5 cb41537 ca7e615 cb41537 35dc3d5 2006ec5 53833c8 bd771b7 0cc44e2 53833c8 0cc44e2 bd771b7 53833c8 0cc44e2 7e52af9 0cc44e2 ca7e615 9f9cf8b 6d2fbf8 9f9cf8b 64547cd ca7e615 53833c8 |
1 2 3 4 5 6 7 8 9 10 11 12 13 14 15 16 17 18 19 20 21 22 23 24 25 26 27 28 29 30 31 32 33 34 35 36 37 38 39 40 41 42 43 44 45 46 47 48 49 50 51 52 53 54 55 56 57 58 59 60 61 62 63 64 65 66 67 68 69 70 71 72 73 74 75 76 77 78 79 80 81 82 83 84 85 86 87 88 89 90 91 92 93 94 95 96 97 98 99 100 101 102 103 104 105 106 107 108 109 110 111 112 113 114 115 116 117 118 119 120 121 122 123 124 125 126 127 128 129 130 131 132 133 134 135 136 137 138 139 140 141 142 143 144 145 146 147 148 149 150 151 152 153 154 155 156 157 158 159 160 161 162 163 164 165 166 167 168 169 170 171 172 173 174 175 176 177 178 179 180 181 182 183 184 185 186 187 188 189 190 191 192 193 194 195 196 197 198 199 200 201 202 203 204 205 206 207 208 209 210 211 212 213 214 215 216 217 218 219 220 221 222 223 224 225 226 227 228 229 230 231 232 233 234 235 236 237 238 239 240 241 242 243 244 245 246 247 248 249 250 251 252 253 254 |
---
license: apache-2.0
language:
- th
- en
library_name: transformers
pipeline_tag: text-generation
tags:
- openthaigpt
- llama
---
# 🇹🇭 OpenThaiGPT 7b 1.0.0
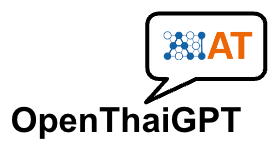
[More Info](https://openthaigpt.aieat.or.th/)
🇹🇭 **OpenThaiGPT 7b Version 1.0.0** is an advanced 7-billion-parameter Thai language chat model based on LLaMA v2 released on April 8, 2024. It has been specifically fine-tuned for Thai instructions and enhanced by incorporating over 10,000 of the most commonly used Thai words into the large language model's (LLM) dictionary, significantly boosting its response speed.
## Highlights
- **Leading-edge Thai language LLM**, setting new benchmarks by achieving the highest average scores across several Thai language exams when compared to all other open-source Thai LLMs.
- **The First 70b Thai opensource LLM**, achieving the higher score on Thai exams than OpenAI GPT 3.5, Google Gemini, and Claude 3 Haiku.
- **Support for extended conversations** across multiple turns.
- Support the use case of **Retrieval Augmented Generation (RAG)** for enriched response generation.
- **Generation speeds increased by tenfold**, thanks to the addition of 10,000 frequently used Thai words to the model's dictionary.
- Pretrained upon a foundation of **more than 65 billion Thai language words** and meticulously fine-tuned with over 1 million Thai instruction examples.
- Capable of understanding and processing **input contexts of up to 4096 Thai words**, allowing for detailed and complex instructions.
## Benchmark by OpenThaiGPT Eval
** Please take a look at ``OTG 7b (April 2024)`` for this model's evaluation result.
| **Exams** | **OTG 7b (Aug 2023)** | **OTG 13b (Dec 2023)** | <b style="color:blue">OTG 7b (April 2024)</b> | **OTG 13b (April 2024)** | **OTG 70b (April 2024)** | **SeaLLM 7b v1** | **SeaLLM 7b v2** | **SeaLion 7b** | **WanchanGLM 7b** | **Sailor-7b-Chat** | **TyphoonGPT 7b Instruct** | **GPT3.5** | **GPT4** | **Gemini Pro** | **Gemini 1.5** | **Claude 3 Haiku** | **Claude 3 Sonnet** | **Claude 3 Opus** |
|----------------------------|-----------------------|------------------------|-------------------------|--------------------------|--------------------------|------------------|------------------|----------------|-------------------|--------------------|----------------------------|------------|----------|----------------|----------------|--------------------|---------------------|-------------------|
| **A-Level** | 17.50% | 34.17% | <b style="color:blue">25.00%</b> | 30.83% | 45.83% | 18.33% | 34.17% | 21.67% | 17.50% | 40.00% | 37.50% | 38.33% | 65.83% | 56.67% | 55.83% | 58.33% | 59.17% | 77.50% |
| **TGAT** | 24.00% | 22.00% | <b style="color:blue">22.00%</b> | 36.00% | 36.00% | 14.00% | 28.00% | 24.00% | 16.00% | 34.00% | 30.00% | 28.00% | 44.00% | 22.00% | 28.00% | 36.00% | 34.00% | 46.00% |
| **TPAT1** | 22.50% | 47.50% | <b style="color:blue">42.50%</b> | 27.50% | 62.50% | 22.50% | 27.50% | 22.50% | 17.50% | 40.00% | 47.50% | 45.00% | 52.50% | 52.50% | 50.00% | 52.50% | 50.00% | 62.50% |
| **thai_investment_consultant_exams** | 8.00% | 28.00% | <b style="color:blue">76.00%</b> | 84.00% | 68.00% | 16.00% | 28.00% | 24.00% | 16.00% | 24.00% | 32.00% | 40.00% | 64.00% | 52.00% | 32.00% | 44.00% | 64.00% | 72.00% |
| **facebook_beleble_tha_200** | 25.00% | 45.00% | <b style="color:blue">34.50%</b> | 39.50% | 70.00% | 13.50% | 51.00% | 27.00% | 24.50% | 63.00% | 51.50% | 50.00% | 72.50% | 65.00% | 74.00% | 63.50% | 77.00% | 90.00% |
| **xcopa_th_200** | 45.00% | 56.50% | <b style="color:blue">49.50%</b> | 51.50% | 74.50% | 26.50% | 47.00% | 51.50% | 48.50% | 68.50% | 65.00% | 64.00% | 82.00% | 68.00% | 74.00% | 64.00% | 80.00% | 86.00% |
| **xnli2.0_th_200** | 33.50% | 34.50% | <b style="color:blue">39.50%</b> | 31.00% | 47.00% | 21.00% | 43.00% | 37.50% | 33.50% | 16.00% | 20.00% | 50.00% | 69.00% | 53.00% | 54.50% | 50.00% | 68.00% | 68.50% |
| **ONET M3** | 17.85% | 38.86% | <b style="color:blue">34.11%</b> | 39.36% | 56.15% | 15.58% | 23.92% | 21.79% | 19.56% | 21.37% | 28.03% | 37.91% | 49.97% | 55.99% | 57.41% | 52.73% | 40.60% | 63.87% |
| **ONET M6** | 21.14% | 28.87% | <b style="color:blue">22.53%</b> | 23.32% | 42.85% | 15.09% | 19.48% | 16.96% | 20.67% | 28.64% | 27.46% | 34.44% | 46.29% | 45.53% | 50.23% | 34.79% | 38.49% | 48.56% |
| **AVERAGE SCORE** | 23.83% | 37.27% | <b style="color:blue;font-size:1.3em">38.40%</b> | 40.33% | 55.87% | 18.06% | 33.56% | 27.44% | 23.75% | 37.28% | 37.67% | 43.07% | 60.68% | 52.30% | 52.89% | 50.65% | 56.81% | 68.32% |
Thai language multiple choice exams, Test on unseen test sets, Zero-shot learning. Benchmark source code and exams information: https://github.com/OpenThaiGPT/openthaigpt_eval
(Updated on: 7 April 2024)
## Licenses
**Source Code**: License Apache Software License 2.0.<br>
**Weight**: Research and **Commercial uses**.<br>
## Sponsors
<img src="https://cdn-uploads.huggingface.co/production/uploads/5fcd9c426d942eaf4d1ebd30/FDC9WYN2iykQbVW1rY4q5.png" width="600px">
## Supports
- Official website: https://openthaigpt.aieat.or.th
- Facebook page: https://web.facebook.com/groups/openthaigpt
- A Discord server for discussion and support [here](https://discord.gg/rUTp6dfVUF)
- E-mail: kobkrit@aieat.or.th
## Prompt Format
Prompt format is based on Llama2 with a small modification (Adding "###" to specify the context part)
```
<s>[INST] <<SYS>
{system_prompt}
<</SYS>>
{human_turn1}###{context_turn1} [/INST]{assistant_turn1}</s><s>{human_turn2}###{context_turn2} [/INST] ...
```
### System prompt:
```
You are a question answering assistant. Answer the question as truthful and helpful as possible คุณคือผู้ช่วยตอบคำถาม จงตอบคำถามอย่างถูกต้องและมีประโยชน์ที่สุด
```
### Examples
#### Single Turn Conversation Example
```
<s>[INST] <<SYS>
You are a question answering assistant. Answer the question as truthful and helpful as possible คุณคือผู้ช่วยตอบคำถาม จงตอบคำถามอย่างถูกต้องและมีประโยชน์ที่สุด
<</SYS>>
สวัสดีครับ [/INST]
```
#### Single Turn Conversation with Context (RAG) Example
```
<s>[INST] <<SYS>
You are a question answering assistant. Answer the question as truthful and helpful as possible คุณคือผู้ช่วยตอบคำถาม จงตอบคำถามอย่างถูกต้องและมีประโยชน์ที่สุด
<</SYS>>
กรุงเทพมีพื้นที่เท่าไร่###กรุงเทพมหานคร เป็นเมืองหลวง นครและมหานครที่มีประชากรมากที่สุดของประเทศไทย กรุงเทพมหานครมีพื้นที่ทั้งหมด 1,568.737 ตร.กม. มีประชากรตามทะเบียนราษฎรกว่า 8 ล้านคน [/INST]
```
#### Multi Turn Conversation Example
##### First turn
```
<s>[INST] <<SYS>
You are a question answering assistant. Answer the question as truthful and helpful as possible คุณคือผู้ช่วยตอบคำถาม จงตอบคำถามอย่างถูกต้องและมีประโยชน์ที่สุด
<</SYS>>
สวัสดีครับ [/INST]
```
##### Second turn
```
<s>[INST] <<SYS>
You are a question answering assistant. Answer the question as truthful and helpful as possible คุณคือผู้ช่วยตอบคำถาม จงตอบคำถามอย่างถูกต้องและมีประโยชน์ที่สุด
<</SYS>>
สวัสดีครับ [/INST]สวัสดีค่ะ มีคำถามอะไร ถามได้เลย</s><s>ขอสูตรทำส้มตำหน่อย [/INST]
```
##### Third turn
```
<s>[INST] <<SYS>
You are a question answering assistant. Answer the question as truthful and helpful as possible คุณคือผู้ช่วยตอบคำถาม จงตอบคำถามอย่างถูกต้องและมีประโยชน์ที่สุด
<</SYS>>
สวัสดีครับ [/INST]สวัสดีค่ะ มีคำถามอะไร ถามได้เลย</s><s>ขอสูตรทำส้มตำหน่อย [/INST]ได้เลยค่ะ ส้มตำเป็นเมนูที่ทำง่ายและอร่อย มาเริ่มกันเลยนะคะ</s><s>เริ่มได้เลย [/INST]
```
##### Fourth turn
```
<s>[INST] <<SYS>
You are a question answering assistant. Answer the question as truthful and helpful as possible คุณคือผู้ช่วยตอบคำถาม จงตอบคำถามอย่างถูกต้องและมีประโยชน์ที่สุด
<</SYS>>
สวัสดีครับ [/INST]สวัสดีค่ะ มีคำถามอะไร ถามได้เลย</s><s>ขอสูตรทำส้มตำหน่อย [/INST]ได้เลยค่ะ ส้มตำเป็นเมนูที่ทำง่ายและอร่อย มาเริ่มกันเลยนะคะ</s><s>เริ่มได้เลย [/INST]
ตำไทยเป็นเมนูที่ใครๆ ก็คุ้นเคย ด้วยรสชาติที่กลมกล่อม หวาน เปรี้ยว เค็ม เผ็ด ครบรส วันนี้เราจะมาทำส้มตำด้วยกันค่ะ สิ่งแรกที่ต้องมีคือเครื่องปรุงดังนี้
- มะละกอดิบ 1 ลูก
- กุ้งแห้ง 1/2 ถ้วย
- กระเทียม 3 กลีบ
- พริกขี้หนู 3 เม็ด
- น้ำตาลปี๊บ 1 ช้อนโต๊ะ
- น้ำปลา 2 ช้อนโต๊ะ
- มะนาว 1 ลูก
- งาขาวคั่ว 1/4 ถ้วย
วิธีทำมีดังนี้ค่ะ
1. เริ่มจากล้างมะละกอให้สะอาด แล้วใช้มีดปอกเปลือก เอาไส้ออก หั่นเป็นเส้นบางๆ เตรียมไว้
2. ตำกุ้งแห้งให้ละเอียด แล้วตักขึ้นพักไว้
3. ใช้ครกหินหรือเครื่องปั่น บดพริกขี้หนูกับกระเทียมให้ละเอียด
4. ใส่กุ้งแห้งที่ตำแล้วลงไปผสม ตามด้วยน้ำตาลปี๊บ น้ำปลา มะนาว และเส้นมะละกอ คลุกเคล้าให้เข้ากัน
5. ชิมรสให้ได้รสหวาน เปรี้ยว เค็ม เผ็ด จากนั้นตักขึ้นเสิร์ฟพร้อมผักสด อาทิ กะหล่ำปลี ถั่วงอก แครอท ผักบุ้ง</s><s>ขอบคุณครับ [/INST]
```
#### Multi Turn Conversation with Context (RAG) Example
```
<s>[INST] <<SYS>
You are a question answering assistant. Answer the question as truthful and helpful as possible คุณคือผู้ช่วยตอบคำถาม จงตอบคำถามอย่างถูกต้องและมีประโยชน์ที่สุด
<</SYS>>
กรุงเทพมีพื้นที่เท่าไร่###กรุงเทพมหานคร เป็นเมืองหลวง นครและมหานครที่มีประชากรมากที่สุดของประเทศไทย กรุงเทพมหานครมีพื้นที่ทั้งหมด 1,568.737 ตร.กม. มีประชากรตามทะเบียนราษฎรกว่า 8 ล้านคน [/INST]
กรุงเทพมหานครมีพื้นที่ทั้งหมด 1,568.737 ตร.กม.</s><s>และประชากรล่ะ [/INST]
```
## How to use
### Huggingface
```python
from transformers import AutoModelForCausalLM, AutoTokenizer
import torch
# Ensure CUDA is available
device = 'cuda' if torch.cuda.is_available() else 'cpu'
print(f"Using device: {device}")
# Init Model
model_path="openthaigpt/openthaigpt-1.0.0-7b-chat"
tokenizer = AutoTokenizer.from_pretrained(model_path, trust_remote_code=True)
model = AutoModelForCausalLM.from_pretrained(model_path, trust_remote_code=True, torch_dtype=torch.float16)
model.to(device)
# Prompt
prompt = "สวัสดีครับ OpenThaiGPT"
llama_prompt = f"<s>[INST] <<SYS>>\nYou are a question answering assistant. Answer the question as truthful and helpful as possible คุณคือผู้ช่วยตอบคำถาม จงตอบคำถามอย่างถูกต้องและมีประโยชน์ที่สุด<</SYS>>\n\n{prompt} [/INST]"
inputs = tokenizer.encode(llama_prompt, return_tensors="pt")
inputs = inputs.to(device)
# Generate
outputs = model.generate(inputs, max_length=512, num_return_sequences=1)
print(tokenizer.decode(outputs[0], skip_special_tokens=True))
```
### vLLM
1. Install VLLM (https://github.com/vllm-project/vllm)
2. Run server
```bash
python -m vllm.entrypoints.api_server --model /path/to/model --tensor-parallel-size num_gpus
```
3. Run inference (CURL example)
```bash
curl --request POST \
--url http://localhost:8000/generate \
--header "Content-Type: application/json" \
--data '{"prompt": "<s>[INST] <<SYS>>\nYou are a question answering assistant. Answer the question as truthful and helpful as possible คุณคือผู้ช่วยตอบคำถาม จงตอบคำถามอย่างถูกต้องและมีประโยชน์ที่สุด\n<</SYS>>\n\nอยากลดความอ้วนต้องทำอย่างไร [/INST]","use_beam_search": false, "temperature": 0.1, "max_tokens": 512, "top_p": 0.75, "top_k": 40, "frequency_penalty": 0.3 "stop": "</s>"}'
```
### LlamaCPP (for GGUF)
1. Build and Install LlamaCPP (LLAMA_CUBLAS=1 is for GPU inference)
```bash
git clone https://github.com/ggerganov/llama.cpp.git \
&& cd llama.cpp \
&& make -j LLAMA_CUBLAS=1 CUDA_DOCKER_ARCH=all
```
2. Run server
```bash
./server -m /path/to/ggml-model-f16.gguf -c 3072 -ngl 81 -ts 1,1 --host 0.0.0.0
```
3. Run inference (CURL example)
```bash
curl --location 'http://localhost:8000/completion' \
--header 'Content-Type: application/json' \
--data '{
"prompt":"<s>[INST] <<SYS>>\nYou are a question answering assistant. Answer the question as truthful and helpful as possible คุณคือผู้ช่วยตอบคำถาม จงตอบคำถามอย่างถูกต้องและมีประโยชน์ที่สุด friendly\n\n<<SYS>>\n\nอยากลดความอ้วนต้องทำอย่างไร [/INST]",
"max_tokens": 512,
"stop":"</s>"
}'
```
### GPU Memory Requirements
| **Number of Parameters** | **FP 16 bits** | **8 bits (Quantized)** | **4 bits (Quantized)** | **Example Graphic Card for 4 bits** |
|------------------|----------------|------------------------|------------------------|---------------------------------------------|
| **7b** | 24 GB | 12 GB | 6 GB | Nvidia RTX 4060 8GB |
| **13b** | 48 GB | 24 GB | 12 GB | Nvidia RTX 4070 16GB |
| **70b** | 192 GB | 96 GB | 48 GB | Nvidia RTX 4090 24GB x 2 cards |
### Authors
* Kobkrit Viriyayudhakorn (kobkrit@aieat.or.th)
* Sumeth Yuenyong (sumeth.yue@mahidol.edu)
* Thaweewat Rugsujarit (thaweewr@scg.com)
* Jillaphat Jaroenkantasima (autsadang41@gmail.com)
* Norapat Buppodom (new@norapat.com)
* Koravich Sangkaew (kwankoravich@gmail.com)
* Peerawat Rojratchadakorn (peerawat.roj@gmail.com)
* Surapon Nonesung (nonesungsurapon@gmail.com)
* Chanon Utupon (chanon.utupon@gmail.com)
* Sadhis Wongprayoon (sadhis.tae@gmail.com)
* Nucharee Thongthungwong (nuchhub@hotmail.com)
* Chawakorn Phiantham (mondcha1507@gmail.com)
* Patteera Triamamornwooth (patt.patteera@gmail.com)
* Nattarika Juntarapaoraya (natt.juntara@gmail.com)
* Kriangkrai Saetan (kraitan.ss21@gmail.com)
* Pitikorn Khlaisamniang (pitikorn32@gmail.com)
<i>Disclaimer: Provided responses are not guaranteed.</i>
|