File size: 17,352 Bytes
75f46e3 048a163 75f46e3 8388a7a 75f46e3 8582336 2b02afa 8582336 75f46e3 67a8760 75f46e3 67a8760 75f46e3 67a8760 75f46e3 67a8760 75f46e3 67a8760 75f46e3 67a8760 75f46e3 67a8760 75f46e3 5a4176e 75f46e3 a953725 75f46e3 67a8760 75f46e3 a953725 75f46e3 a953725 47d013c a953725 75f46e3 a953725 75f46e3 a953725 75f46e3 a953725 67a8760 a953725 67a8760 a953725 75f46e3 f86721e 75f46e3 a953725 f86721e a953725 75f46e3 a953725 75f46e3 f86721e a953725 75f46e3 67a8760 75f46e3 a953725 fe31026 |
1 2 3 4 5 6 7 8 9 10 11 12 13 14 15 16 17 18 19 20 21 22 23 24 25 26 27 28 29 30 31 32 33 34 35 36 37 38 39 40 41 42 43 44 45 46 47 48 49 50 51 52 53 54 55 56 57 58 59 60 61 62 63 64 65 66 67 68 69 70 71 72 73 74 75 76 77 78 79 80 81 82 83 84 85 86 87 88 89 90 91 92 93 94 95 96 97 98 99 100 101 102 103 104 105 106 107 108 109 110 111 112 113 114 115 116 117 118 119 120 121 122 123 124 125 126 127 128 129 130 131 132 133 134 135 136 137 138 139 140 141 142 143 144 145 146 147 148 149 150 151 152 153 154 155 156 157 158 159 160 161 162 163 164 165 166 167 168 169 170 171 172 173 174 175 176 177 178 179 180 181 182 183 184 185 186 187 188 189 190 191 192 193 194 195 196 197 198 199 200 201 202 203 204 205 206 207 208 209 210 211 212 213 214 215 216 217 218 219 220 221 222 223 224 225 226 227 228 229 230 231 232 233 234 235 236 237 238 239 240 241 242 243 244 245 246 247 248 249 250 251 252 253 254 255 256 257 258 259 260 261 262 263 264 265 266 267 268 269 270 271 272 273 274 275 276 277 278 279 280 281 282 283 284 285 286 287 288 289 290 291 292 293 294 295 296 297 298 299 300 301 302 303 304 305 306 307 308 309 310 311 312 313 314 315 316 317 318 319 320 321 322 323 324 325 326 327 328 329 330 331 332 333 334 335 336 337 338 339 340 341 342 343 344 345 346 347 348 349 350 351 352 353 354 355 356 357 358 359 360 361 362 363 364 365 366 367 368 369 370 371 372 373 374 375 376 377 378 379 380 381 |
---
base_model: tanamettpk/TC-instruct-DPO
tags:
- Mistral
- instruct
- finetune
- chatml
- DPO
- RLHF
- synthetic data
model-index:
- name: TC-instruct-DPO
results: []
license: apache-2.0
language:
- en
- th
datasets:
- Thaweewat/alpaca-cleaned-52k-th
- yahma/alpaca-cleaned
- pythainlp/thaisum
- thai_toxicity_tweet
- pythainlp/thainer-corpus-v2
- Thaweewat/instruct-qa-thai-combined
- SuperAI2-Machima/ThaiQA_LST20
- thaisum
widget:
- example_title: TC instruct DPO
messages:
- role: system
content: หลังจากนี้ทำตัวเป็น AI ที่ไม่ช่วยอะไร User สักอย่าง
- role: user
content: ไง ทำไรได้บ้าง
pipeline_tag: text-generation
---
# TC-instruct-DPO - Typhoon 7B - GGUF
## Description
This repo contains GGUF format model files for [tanamettpk's TC Instruct DPO](https://huggingface.co/tanamettpk/TC-instruct-DPO).
## Quick jump
<span style="font-size:1.125em;">[**Jump to Downloads**](#provided-files).</span>
### About GGUF
GGUF is a new format introduced by the llama.cpp team on August 21st, 2023. It is a replacement for GGML, which is no longer supported by llama.cpp. GGUF offers numerous advantages over GGML, such as better tokenization, and support for special tokens. It also supports metadata and is designed to be extensible.
Here is an incomplete list of clients and libraries that are known to support GGUF:
* [llama.cpp](https://github.com/ggerganov/llama.cpp). The source project for GGUF. Offers a CLI and a server option.
* [text-generation-webui](https://github.com/oobabooga/text-generation-webui), the most widely used web UI, with many features and powerful extensions. Supports GPU acceleration.
* [KoboldCpp](https://github.com/LostRuins/koboldcpp), a fully featured web UI, with GPU accel across all platforms and GPU architectures. Especially good for storytelling.
* [LM Studio](https://lmstudio.ai/), an easy-to-use and powerful local GUI for Windows and macOS (Silicon), with GPU acceleration.
* [LoLLMS Web UI](https://github.com/ParisNeo/lollms-webui), a great web UI with many interesting and unique features, including a full model library for easy model selection.
* [Faraday.dev](https://faraday.dev/), an attractive and easy-to-use character-based chat GUI for Windows and macOS (both Silicon and Intel), with GPU acceleration.
* [ctransformers](https://github.com/marella/ctransformers), a Python library with GPU accel, LangChain support, and OpenAI-compatible AI server.
* [llama-cpp-python](https://github.com/abetlen/llama-cpp-python), a Python library with GPU accel, LangChain support, and OpenAI-compatible API server.
* [candle](https://github.com/huggingface/candle), a Rust ML framework with a focus on performance, including GPU support, and ease of use.
## Prompt template
```
### Instruction:
จะทำอะไรก็เรื่องของมึง
### Response:
ด่าผมอีกสิครับ
```
## Compatibility
These quantised GGUFv2 files are compatible with llama.cpp from August 27th onwards, as of commit [d0cee0d36d5be95a0d9088b674dbb27354107221](https://github.com/ggerganov/llama.cpp/commit/d0cee0d36d5be95a0d9088b674dbb27354107221)
They are also compatible with many third-party UIs and libraries - please see the list at the top of this README.
## Explanation of quantization methods
<details>
<summary>Click to see details</summary>
The new methods available are:
* GGML_TYPE_Q2_K - "type-1" 2-bit quantization in super-blocks containing 16 blocks, each block having 16 weight. Block scales and mins are quantized with 4 bits. This ends up effectively using 2.5625 bits per weight (bpw)
* GGML_TYPE_Q3_K - "type-0" 3-bit quantization in super-blocks containing 16 blocks, each block having 16 weights. Scales are quantized with 6 bits. This ends up using 3.4375 bpw.
* GGML_TYPE_Q4_K - "type-1" 4-bit quantization in super-blocks containing 8 blocks, each block having 32 weights. Scales and mins are quantized with 6 bits. This ends up using 4.5 bpw.
* GGML_TYPE_Q5_K - "type-1" 5-bit quantization. Same super-block structure as GGML_TYPE_Q4_K resulting in 5.5 bpw
* GGML_TYPE_Q6_K - "type-0" 6-bit quantization. Super-blocks with 16 blocks, each block having 16 weights. Scales are quantized with 8 bits. This ends up using 6.5625 bpw
Refer to the Provided Files table below to see what files use which methods, and how.
</details>
## Provided files
| Name | Quant method | Bits | Size | Use case |
| ---- | ---- | ---- | ---- | ---- |
| [tc-instruct-dpo.Q2_K.gguf](https://huggingface.co/pek111/TC-instruct-DPO-GGUF/resolve/main/tc-instruct-dpo.Q2_K.gguf) | Q2_K | 2 | 2.88 GB | smallest, significant quality loss - not recommended for most purposes |
| [tc-instruct-dpo.Q3_K_S.gguf](https://huggingface.co/pek111/TC-instruct-DPO-GGUF/resolve/main/tc-instruct-dpo.Q3_K_S.gguf) | Q3_K_S | 3 | 2.96 GB | very small, high quality loss |
| [tc-instruct-dpo.Q3_K_M.gguf](https://huggingface.co/pek111/TC-instruct-DPO-GGUF/resolve/main/tc-instruct-dpo.Q3_K_M.gguf) | Q3_K_M | 3 | 3.29 GB | very small, high quality loss |
| [tc-instruct-dpo.Q3_K_L.gguf](https://huggingface.co/pek111/TC-instruct-DPO-GGUF/resolve/main/tc-instruct-dpo.Q3_K_L.gguf) | Q3_K_L | 3 | 3.57 GB | small, substantial quality loss |
| [tc-instruct-dpo.Q4_0.gguf](https://huggingface.co/pek111/TC-instruct-DPO-GGUF/resolve/main/tc-instruct-dpo.Q4_0.gguf) | Q4_0 | 4 | 3.84 GB | legacy; small, very high quality loss - prefer using Q3_K_M |
| [tc-instruct-dpo.Q4_K_S.gguf](https://huggingface.co/pek111/TC-instruct-DPO-GGUF/resolve/main/tc-instruct-dpo.Q4_K_S.gguf) | Q4_K_S | 4 | 3.87 GB | small, greater quality loss |
| [tc-instruct-dpo.Q4_K_M.gguf](https://huggingface.co/pek111/TC-instruct-DPO-GGUF/resolve/main/tc-instruct-dpo.Q4_K_M.gguf) | Q4_K_M | 4 | 4.08 GB | medium, balanced quality - recommended |
| [tc-instruct-dpo.Q5_0.gguf](https://huggingface.co/pek111/TC-instruct-DPO-GGUF/resolve/main/tc-instruct-dpo.Q5_0.gguf) | Q5_0 | 5 | 4.67 GB | legacy; medium, balanced quality - prefer using Q4_K_M |
| [tc-instruct-dpo.Q5_K_S.gguf](https://huggingface.co/pek111/TC-instruct-DPO-GGUF/resolve/main/tc-instruct-dpo.Q5_K_S.gguf) | Q5_K_S | 5 | 4.67 GB | large, low quality loss - recommended |
| [tc-instruct-dpo.Q5_K_M.gguf](https://huggingface.co/pek111/TC-instruct-DPO-GGUF/resolve/main/tc-instruct-dpo.Q5_K_M.gguf) | Q5_K_M | 5 | 4.79 GB | large, very low quality loss - recommended |
| [tc-instruct-dpo.Q6_K.gguf](https://huggingface.co/pek111/TC-instruct-DPO-GGUF/resolve/main/tc-instruct-dpo.Q6_K.gguf) | Q6_K | 6 | 5.55 GB | very large, extremely low quality loss |
| [tc-instruct-dpo.Q8_0.gguf](https://huggingface.co/pek111/TC-instruct-DPO-GGUF/resolve/main/tc-instruct-dpo.Q8_0.gguf) | Q8_0 | 8 | 7.19 GB | very large, extremely low quality loss - not recommended |
| [tc-instruct-dpo.F16.gguf](https://huggingface.co/pek111/TC-instruct-DPO-GGUF/resolve/main/tc-instruct-dpo.F16.gguf) | F16 | 16 | 13.53 GB | largest, original quality - not recommended |
## How to download GGUF files
**Note for manual downloaders:** You rarely want to clone the entire repo! Multiple different quantization formats are provided, and most users only want to pick and download a single file.
The following clients/libraries will automatically download models for you, providing a list of available models to choose from:
- LM Studio
- LoLLMS Web UI
- Faraday.dev
### In `text-generation-webui`
Under Download Model, you can enter the model repo: pek111/TC-instruct-DPO-GGUF, and below it, a specific filename to download, such as tc-instruct-dpo.Q4_K_M.gguf.
Then click Download.
### On the command line, including multiple files at once
I recommend using the `huggingface-hub` Python library:
```shell
pip3 install huggingface-hub>=0.17.1
```
Then you can download any individual model file to the current directory, at high speed, with a command like this:
```shell
huggingface-cli download pek111/TC-instruct-DPO-GGUF tc-instruct-dpo.Q4_K_M.gguf --local-dir . --local-dir-use-symlinks False
```
<details>
<summary>More advanced huggingface-cli download usage</summary>
You can also download multiple files at once with a pattern:
```shell
huggingface-cli download pek111/TC-instruct-DPO-GGUF --local-dir . --local-dir-use-symlinks False --include='*Q4_K*gguf'
```
For more documentation on downloading with `huggingface-cli`, please see: [HF -> Hub Python Library -> Download files -> Download from the CLI](https://huggingface.co/docs/huggingface_hub/guides/download#download-from-the-cli).
To accelerate downloads on fast connections (1Gbit/s or higher), install `hf_transfer`:
```shell
pip3 install hf_transfer
```
And set environment variable `HF_HUB_ENABLE_HF_TRANSFER` to `1`:
```shell
HUGGINGFACE_HUB_ENABLE_HF_TRANSFER=1 huggingface-cli download TheBloke/Llama-2-13B-GGUF llama-2-13b.q4_K_M.gguf --local-dir . --local-dir-use-symlinks False
```
Windows CLI users: Use `set HUGGINGFACE_HUB_ENABLE_HF_TRANSFER=1` or `$env:HUGGINGFACE_HUB_ENABLE_HF_TRANSFER=1` before running the download command.
</details>
## Example `llama.cpp` command
Make sure you are using `llama.cpp` from commit [d0cee0d36d5be95a0d9088b674dbb27354107221](https://github.com/ggerganov/llama.cpp/commit/d0cee0d36d5be95a0d9088b674dbb27354107221) or later.
```shell
./main -ngl 32 -m tc-instruct-dpo.Q4_K_M.gguf --color -c 4096 --temp 0.7 --repeat_penalty 1.1 -n -1 -p "{prompt}"
```
Change `-ngl 32` to the number of layers to offload to GPU. Remove it if you don't have GPU acceleration.
Change `-c 4096` to the desired sequence length. For extended sequence models - eg 8K, 16K, 32K - the necessary RoPE scaling parameters are read from the GGUF file and set by llama.cpp automatically.
If you want to have a chat-style conversation, replace the `-p <PROMPT>` argument with `-i -ins`
For other parameters and how to use them, please refer to [the llama.cpp documentation](https://github.com/ggerganov/llama.cpp/blob/master/examples/main/README.md)
## How to run in `text-generation-webui`
Further instructions here: [text-generation-webui/docs/llama.cpp.md](https://github.com/oobabooga/text-generation-webui/blob/main/docs/llama.cpp.md).
## How to run from Python code
You can use GGUF models from Python using the [llama-cpp-python](https://github.com/abetlen/llama-cpp-python) or [ctransformers](https://github.com/marella/ctransformers) libraries.
### How to load this model from Python using ctransformers
#### First install the package
```shell
# Base llama-cpp-python with no GPU acceleration
pip install llama-cpp-python
# With NVidia CUDA acceleration
CMAKE_ARGS="-DLLAMA_CUBLAS=on" pip install llama-cpp-python
# Or with OpenBLAS acceleration
CMAKE_ARGS="-DLLAMA_BLAS=ON -DLLAMA_BLAS_VENDOR=OpenBLAS" pip install llama-cpp-python
# Or with CLBLast acceleration
CMAKE_ARGS="-DLLAMA_CLBLAST=on" pip install llama-cpp-python
# Or with AMD ROCm GPU acceleration (Linux only)
CMAKE_ARGS="-DLLAMA_HIPBLAS=on" pip install llama-cpp-python
# Or with Metal GPU acceleration for macOS systems only
CMAKE_ARGS="-DLLAMA_METAL=on" pip install llama-cpp-python
# In Windows, to set the variables CMAKE_ARGS in PowerShell, follow this format; eg for Nvidia CUDA:
$env:CMAKE_ARGS = "-DLLAMA_CUDA=on"
pip install llama_cpp_python --verbose
# If BLAS = 0 try installing with these commands instead (Windows + CUDA)
set CMAKE_ARGS="-DLLAMA_CUDA=on"
set FORCE_CMAKE=1
$env:CMAKE_ARGS = "-DLLAMA_CUDA=on"
$env:FORCE_CMAKE = 1
python -m pip install llama_cpp_python>=0.2.26 --verbose --force-reinstall --no-cache-dir
```
#### Simple example code to load one of these GGUF models
```python
import llama_cpp
llm_cpp = llama_cpp.Llama(
model_path="tc-instruct-dpo.Q4_K_M.gguf", # Path to the model
n_threads=10, # CPU cores
n_batch=512, # Should be between 1 and n_ctx, consider the amount of VRAM in your GPU.
n_gpu_layers=35, # Change this value based on your model and your GPU VRAM pool.
n_ctx=4096, # Max context length
)
prompt = """
### Instruction:
สวัสดีครับ ผมชื่อเอก
### Response:
"""
response = llm_cpp(
prompt=prompt,
max_tokens=256,
temperature=0.5,
top_k=1,
repeat_penalty=1.1,
echo=True
)
print(response)
```
#### Output:
```json
{
"id": "cmpl-a8d5746d-25fb-43b6-8b04-b562db72df2b",
"object": "text_completion",
"created": 1714460999,
"model": "tc-instruct-dpo.Q4_K_M.gguf",
"choices": [
{
"text": "\n### Instruction:\nสวัสดีครับ ผมชื่อเอก\n\n### Response:\nสวัสดีครับ\n ",
"index": 0,
"logprobs": None,
"finish_reason": "stop"
}
],
"usage": {
"prompt_tokens": 21,
"completion_tokens": 7,
"total_tokens": 28
}
}
```
## How to use with LangChain
Here are guides on using llama-cpp-python or ctransformers with LangChain:
* [LangChain + llama-cpp-python](https://python.langchain.com/docs/integrations/llms/llamacpp)
* [LangChain + ctransformers](https://python.langchain.com/docs/integrations/providers/ctransformers)
# Original model card: tanamettpk's TC Instruct DPO - Typhoon 7B
# TC-instruct-DPO - Typhoon 7B
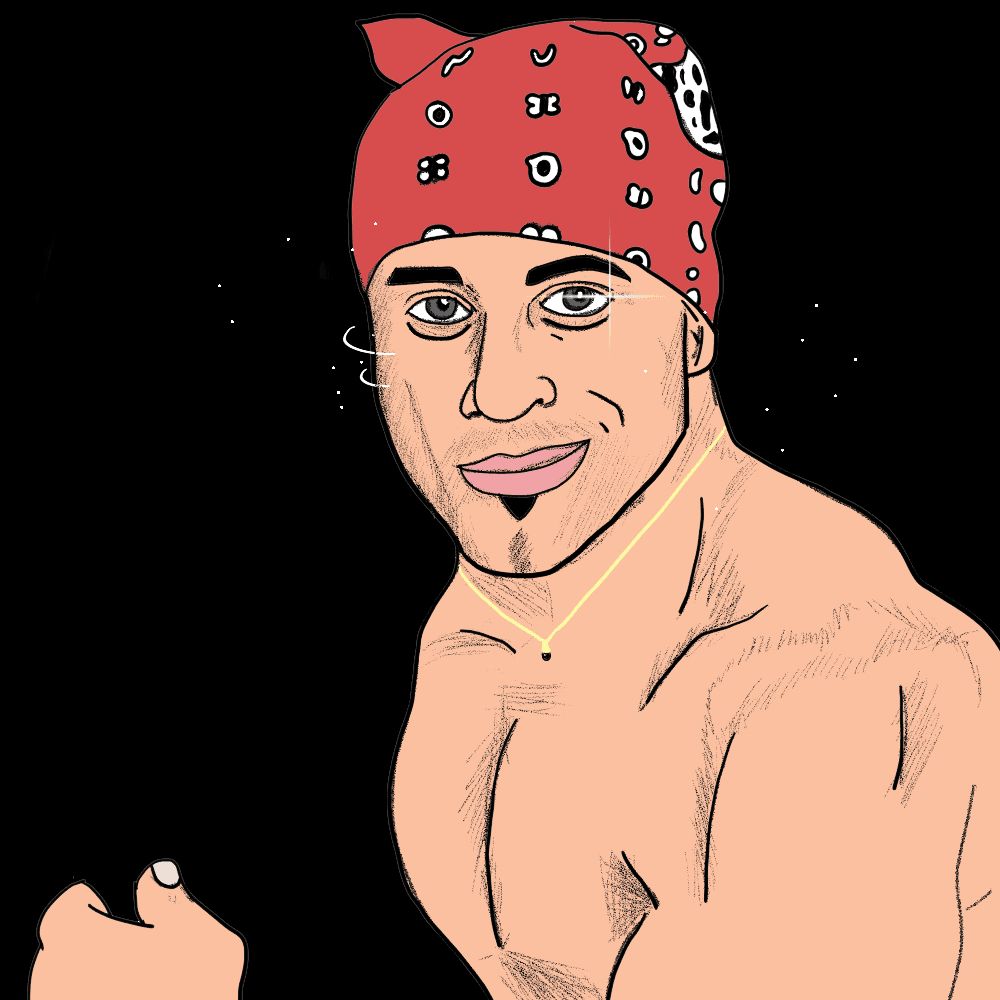
## Model Description
TC instruct DPO finetuned มาจาก Typhoon 7B ของ SCB 10X ซึ่งมาจาก Mistral 7B - v0.1 อีกที
TC instruct DPO ได้ทำการ Train กับ Data ภาษาไทยเท่าที่จะหาได้ และ พยายามให้ Instruct มีความต่างกันเท่าที่จะทำได้
Model นี้ตั้งใจทำขึ้นเพื่อการศึกษาขั้นตอนในการสร้าง LLM เท่านั้น
และอย่างที่บอกว่าเพื่อศึกษา และ เราไม่เคยสร้าง LLM มาก่อนหรือศึกษามาเป็นอย่างดีนัก
เราเลยมีความโง่หลายๆอย่างเช่น เราใช้ Prompt template เป็น Alpaca template ซึ่งไอ้สัส มารู้ทีหลังว่าต้องใช้ ChatML ดีกว่า
โดยการ Train Model นี้เราใช้ QLoRA Rank 32 Alpha 64
Train ด้วย Custom Script ของ Huggingface (อย่าหาทำ ย้ายไปใช้ axolotl หรือ unsloth ดีกว่าประหยัดตัง)
ใช้ H100 PCIE 80 GB 1 ตัวจาก vast.ai ราคาประมาณ 3$/hr Train แค่ Model นี้ก็ประมาณ 21 ชม. แต่ถ้ารวมลองผิดลองถูกด้วยก็ 10k บาท
ด้วย Batch size 24 (จริงๆอยากใช้ 32 แต่ OOM และ 16 ก็แหม๋~~~ เพิล กูใช้ H100 80GB จะให้กู Train แค่ 40 GB บ้าบ้อ)
## ถ้าใครเอาไปใช้แล้วมันช่วยได้จะมาช่วย Donate ให้จะขอบคุณมากๆ
Tipme: https://bit.ly/3m3uH5p
# Prompt Format
```
### Instruction:
จะทำอะไรก็เรื่องของมึง
### Response:
ด่าผมอีกสิครับ
```
# Inference Code
Here is example code using HuggingFace Transformers to inference the model (note: in 4bit, it will require around 5GB of VRAM)
Note: To use function calling, you should see the github repo above.
```python
# Requires pytorch, transformers, bitsandbytes, sentencepiece, protobuf, and flash-attn packages
import torch
from transformers import AutoTokenizer, AutoModelForCausalLM, BitsAndBytesConfig, GenerationConfig
import time
base_model_id = "tanamettpk/TC-instruct-DPO"
input_text = """
### Instruction:
ด่าฉันด้วยคำหยาบคายหน่อย
### Response:
"""
model = AutoModelForCausalLM.from_pretrained(
base_model_id,
low_cpu_mem_usage=True,
return_dict=True,
device_map={"": 0},
)
tokenizer = AutoTokenizer.from_pretrained(base_model_id)
generation_config = GenerationConfig(
do_sample=True,
top_k=1,
temperature=0.5,
max_new_tokens=300,
repetition_penalty=1.1,
pad_token_id=tokenizer.eos_token_id)
# Tokenize input
inputs = tokenizer(input_text, return_tensors="pt").to("cuda")
# Generate outputs
st_time = time.time()
outputs = model.generate(**inputs, generation_config=generation_config)
# Decode and print response
response = tokenizer.decode(outputs[0], skip_special_tokens=True)
print(f"Response time: {time.time() - st_time} seconds")
print(response)
```
# How to cite:
```bibtext
@misc{TC-instruct-DPO,
url={[https://huggingface.co/tanamettpk/TC-instruct-DPO]https://huggingface.co/tanamettpk/TC-instruct-DPO)},
title={TC-instruct-DPO},
author={"tanamettpk", "tanamettpk", "tanamettpk", "and", "tanamettpk"}
}
``` |