--- license: apache-2.0 --- # **m**utual **i**nformation **C**ontrastive **S**entence **E**mbedding (**miCSE**): [](https://arxiv.org/abs/2211.04928) Language model of the pre-print arXiv paper titled: "_**miCSE**: Mutual Information Contrastive Learning for Low-shot Sentence Embeddings_" # Brief Model Description The **miCSE** language model is trained for sentence similarity computation. Training the model imposes alignment between the attention pattern of different views (embeddings of augmentations) during contrastive learning. Learning sentence embeddings with **miCSE** entails enforcing the syntactic consistency across augmented views for every single sentence, making contrastive self-supervised learning more sample efficient. This is achieved by regularizing the attention distribution. Regularizing the attention space enables learning representation in self-supervised fashion even when the _training corpus is comparatively small_. This is particularly interesting for _real-world applications_, where training data is significantly smaller thank Wikipedia. # Intended Use The model intended to be used for encoding sentences or short paragraphs. Given an input text, the model produces a vector embedding, which captures the semantics. The embedding can be used for numerous tasks, e.g., **retrieval**, **clustering** or **sentence similarity** comparison (see example below). Sentence representations correspond to the embedding of the _**[CLS]**_ token. # Training data The model was trained on a random collection of **English** sentences from Wikipedia: [Training data file](https://huggingface.co/datasets/princeton-nlp/datasets-for-simcse/resolve/main/wiki1m_for_simcse.txt) # Model Usage ## Example 1) - Sentence Similarity ```python from transformers import AutoTokenizer, AutoModel import torch.nn as nn tokenizer = AutoTokenizer.from_pretrained("sap-ai-research/miCSE") model = AutoModel.from_pretrained("sap-ai-research/miCSE") # Encoding of sentences in a list with a predefined maximum lengths of tokens (max_length) max_length = 32 sentences = [ "This is a sentence for testing miCSE.", "This is yet another test sentence for the mutual information Contrastive Sentence Embeddings model." ] batch = tokenizer.batch_encode_plus( sentences, return_tensors='pt', padding=True, max_length=max_length, truncation=True ) # Compute the embeddings and keep only the _**[CLS]**_ embedding (the first token) # Get raw embeddings (no gradients) with torch.no_grad(): outputs = model(**batch, output_hidden_states=True, return_dict=True) embeddings = outputs.last_hidden_state[:,0] # Define similarity metric, e.g., cosine similarity sim = nn.CosineSimilarity(dim=-1) # Compute similarity between the **first** and the **second** sentence cos_sim = sim(embeddings.unsqueeze(1), embeddings.unsqueeze(0)) print(f"Distance: {cos_sim[0,1].detach().item()}") ``` ## Example 2) - Clustering ```python from transformers import AutoTokenizer, AutoModel import torch.nn as nn import torch import numpy as np import tqdm from datasets import load_dataset import umap import umap.plot as umap_plot # Determine available hardware if torch.backends.mps.is_available(): device = torch.device("mps") elif torch.cuda.is_available(): device = torch.device("gpu") else: device = torch.device("cpu") # Load tokenizer and model tokenizer = AutoTokenizer.from_pretrained("/Users/d065243/miCSE") model = AutoModel.from_pretrained("/Users/d065243/miCSE") # Load Twitter data for sentiment clustering dataset = load_dataset("tweet_eval", "sentiment") # Compute embeddings of the tweets # set batch size and maxium tweet token length batch_size = 50 max_length = 128 iterations = int(np.floor(len(dataset['train'])/batch_size))*batch_size embedding_stack = [] classes = [] for i in tqdm.notebook.tqdm(range(0,iterations,batch_size)): # create batch batch = tokenizer.batch_encode_plus( dataset['train'][i:i+batch_size]['text'], return_tensors='pt', padding=True, max_length=max_length, truncation=True ).to(device) classes = classes + dataset['train'][i:i+batch_size]['label'] # model inference without gradient with torch.no_grad(): outputs = model(**batch, output_hidden_states=True, return_dict=True) embeddings = outputs.last_hidden_state[:,0] embedding_stack.append( embeddings.cpu().clone() ) embeddings = torch.vstack(embedding_stack) # Cluster embeddings in 2D with UMAP umap_model = umap.UMAP(n_neighbors=250, n_components=2, min_dist=1.0e-9, low_memory=True, angular_rp_forest=True, metric='cosine') umap_model.fit(embeddings) # Plot result umap_plot.points(umap_model, labels = np.array(classes),theme='fire') ``` 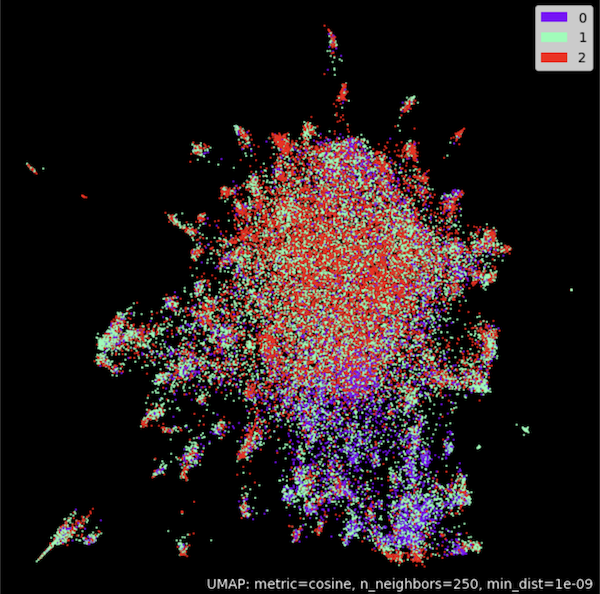 # Benchmark Model results on SentEval Benchmark: ```shell +-------+-------+-------+-------+-------+--------------+-----------------+--------+ | STS12 | STS13 | STS14 | STS15 | STS16 | STSBenchmark | SICKRelatedness | S.Avg. | +-------+-------+-------+-------+-------+--------------+-----------------+--------+ | 71.71 | 83.09 | 75.46 | 83.13 | 80.22 | 79.70 | 73.62 | 78.13 | +-------+-------+-------+-------+-------+--------------+-----------------+--------+ ``` ## Citations If you use this code in your research or want to refer to our work, please cite: ``` @article{Klein2022miCSEMI, title={miCSE: Mutual Information Contrastive Learning for Low-shot Sentence Embeddings}, author={Tassilo Klein and Moin Nabi}, journal={ArXiv}, year={2022}, volume={abs/2211.04928} } ``` #### Authors: - [Tassilo Klein](https://tjklein.github.io/) - [Moin Nabi](https://moinnabi.github.io/)