diff --git a/.gitattributes b/.gitattributes
index c7d9f3332a950355d5a77d85000f05e6f45435ea..24c3f2113486d49620d4caf23938d73dddbd7faa 100644
--- a/.gitattributes
+++ b/.gitattributes
@@ -32,3 +32,8 @@ saved_model/**/* filter=lfs diff=lfs merge=lfs -text
*.zip filter=lfs diff=lfs merge=lfs -text
*.zst filter=lfs diff=lfs merge=lfs -text
*tfevents* filter=lfs diff=lfs merge=lfs -text
+video/4.mp4 filter=lfs diff=lfs merge=lfs -text
+video/5.mp4 filter=lfs diff=lfs merge=lfs -text
+video/9.mp4 filter=lfs diff=lfs merge=lfs -text
+video/bicyclecity.mp4 filter=lfs diff=lfs merge=lfs -text
+video/car.mp4 filter=lfs diff=lfs merge=lfs -text
diff --git a/.github/ISSUE_TEMPLATE/bug.yml b/.github/ISSUE_TEMPLATE/bug.yml
new file mode 100644
index 0000000000000000000000000000000000000000..a83f3d1c1874a13ee356180cb94e0b4a9f28d1f4
--- /dev/null
+++ b/.github/ISSUE_TEMPLATE/bug.yml
@@ -0,0 +1,65 @@
+name: Bug
+# title: " "
+description: Report a Yolov5_StrongSORT_OSNet bug
+labels: [bug]
+body:
+
+ - type: checkboxes
+ attributes:
+ label: Search before asking
+ description: >
+ Please search the [issues](https://github.com/mikel-brostrom/Yolov5_StrongSORT_OSNet/issues) and [discussions](https://github.com/mikel-brostrom/Yolov5_StrongSORT_OSNet/discussions) to see if a similar question already exists.
+ options:
+ - label: >
+ I have searched the Yolov5_StrongSORT_OSNet [issues](https://github.com/mikel-brostrom/Yolov5_StrongSORT_OSNet/issues) and [discussions](https://github.com/mikel-brostrom/Yolov5_StrongSORT_OSNet/discussions) and found no similar questions.
+ required: true
+
+ - type: dropdown
+ attributes:
+ label: Yolov5_StrongSORT_OSNet Component
+ description: |
+ Please select the part of Yolov5_StrongSORT_OSNet where you found the bug.
+ multiple: true
+ options:
+ - "Tracking"
+ - "Evaluation"
+ - "Integrations"
+ - "Other"
+ validations:
+ required: false
+
+ - type: textarea
+ attributes:
+ label: Bug
+ description: Provide console output with error messages and/or screenshots of the bug.
+ placeholder: |
+ 💡 ProTip! Include as much information as possible (screenshots, logs, tracebacks etc.) to receive the most helpful response.
+ validations:
+ required: true
+
+ - type: textarea
+ attributes:
+ label: Environment
+ description: Please specify the software and hardware you used to produce the bug.
+ placeholder: |
+ - YOLO: YOLOv5 🚀 v6.0-67-g60e42e1 torch 1.9.0+cu111 CUDA:0 (A100-SXM4-40GB, 40536MiB)
+ - StrongSORT: osnet_x0_25
+ - OS: Ubuntu 20.04
+ - Python: 3.9.0
+ - ...
+ validations:
+ required: false
+
+ - type: textarea
+ attributes:
+ label: Minimal Reproducible Example
+ description: >
+ When asking a question, people will be better able to provide help if you provide code that they can easily understand and use to **reproduce** the problem.
+ This is referred to by community members as creating a [minimal reproducible example](https://stackoverflow.com/help/minimal-reproducible-example).
+ placeholder: |
+ ```
+ # Code to reproduce your issue here
+ ```
+ validations:
+ required: false
+
diff --git a/.github/ISSUE_TEMPLATE/enhancement.yml b/.github/ISSUE_TEMPLATE/enhancement.yml
new file mode 100644
index 0000000000000000000000000000000000000000..c103580f1b23e825853a2b4ad9d08e6322a34096
--- /dev/null
+++ b/.github/ISSUE_TEMPLATE/enhancement.yml
@@ -0,0 +1,32 @@
+name: Enhancement
+description: Suggest a Yolov5_StrongSORT_OSNet enhancement
+# title: " "
+labels: [enhancement]
+body:
+
+ - type: checkboxes
+ attributes:
+ label: Search before asking
+ description: >
+ Please search the [issues](https://github.com/mikel-brostrom/Yolov5_StrongSORT_OSNet/issues) to see if a similar enhancement request already exists.
+ options:
+ - label: >
+ I have searched the YOLOv5 [issues](https://github.com/mikel-brostrom/Yolov5_StrongSORT_OSNet/issues) and found no similar enhancement requests.
+ required: true
+
+ - type: textarea
+ attributes:
+ label: Description
+ description: A short description of your enhancement.
+ placeholder: |
+ What new enhancement would you like to see in Yolov5_StrongSORT_OSNet?
+ validations:
+ required: true
+
+ - type: textarea
+ attributes:
+ label: Use case
+ description: |
+ Describe the use case of your feature request. It will help us understand and prioritize the feature request.
+ placeholder: |
+ How would this feature be used, and who would use it?
diff --git a/.github/ISSUE_TEMPLATE/question.yml b/.github/ISSUE_TEMPLATE/question.yml
new file mode 100644
index 0000000000000000000000000000000000000000..5da72d5070bf7546227dd4f27e9660c113d4e47e
--- /dev/null
+++ b/.github/ISSUE_TEMPLATE/question.yml
@@ -0,0 +1,24 @@
+name: Question
+description: Ask a Yolov5_StrongSORT_OSNet question
+# title: " "
+labels: [question]
+body:
+
+ - type: checkboxes
+ attributes:
+ label: Search before asking
+ description: >
+ Please search the [issues](https://github.com/mikel-brostrom/Yolov5_StrongSORT_OSNet/issues) to see if a similar question already exists.
+ options:
+ - label: >
+ I have searched the Yolov5_StrongSORT_OSNet [issues](https://github.com/mikel-brostrom/Yolov5_StrongSORT_OSNet/issues) and found no similar bug report.
+ required: true
+
+ - type: textarea
+ attributes:
+ label: Question
+ description: What is your question?
+ placeholder: |
+ 💡 ProTip! Include as much information as possible (screenshots, logs, tracebacks etc.) to receive the most helpful response.
+ validations:
+ required: true
diff --git a/.github/workflows/ci-testing.yml b/.github/workflows/ci-testing.yml
new file mode 100644
index 0000000000000000000000000000000000000000..26af891c2e5426a5aea71b5e7cde6259705a3260
--- /dev/null
+++ b/.github/workflows/ci-testing.yml
@@ -0,0 +1,71 @@
+name: CI CPU testing
+
+on: # https://help.github.com/en/actions/reference/events-that-trigger-workflows
+ push:
+ branches: [master, CIdebug]
+ pull_request:
+ # The branches below must be a subset of the branches above
+ branches: [master, CIdebug]
+
+jobs:
+ cpu-tests:
+
+ runs-on: ${{ matrix.os }}
+ strategy:
+ fail-fast: false
+ matrix:
+ os: [ubuntu-latest, windows-latest] # Error: Container action is only supported on Linux
+ python-version: [3.9]
+ model: ['yolov5s'] # models to test
+
+ # Timeout: https://stackoverflow.com/a/59076067/4521646
+ timeout-minutes: 50
+ steps:
+ - name: Set up Repository
+ uses: actions/checkout@v2
+ with:
+ submodules: recursive
+ - name: Set up Python ${{ matrix.python-version }}
+ uses: actions/setup-python@v2
+ with:
+ python-version: ${{ matrix.python-version }}
+
+ # Note: This uses an internal pip API and may not always work
+ # https://github.com/actions/cache/blob/master/examples.md#multiple-oss-in-a-workflow
+ - name: Get pip cache
+ id: pip-cache
+ run: |
+ python -c "from pip._internal.locations import USER_CACHE_DIR; print('::set-output name=dir::' + USER_CACHE_DIR)"
+ - name: Cache pip
+ uses: actions/cache@v1
+ with:
+ path: ${{ steps.pip-cache.outputs.dir }}
+ key: ${{ runner.os }}-${{ matrix.python-version }}-pip-${{ hashFiles('requirements.txt') }}
+ restore-keys: |
+ ${{ runner.os }}-${{ matrix.python-version }}-pip-
+ - name: Install dependencies
+ run: |
+ python -m pip install --upgrade pip setuptools wheel
+ # ImportError: lap requires numpy, please "pip install numpy".
+ # ImportError: lap requires Cython, please "pip install Cython".
+ pip install numpy Cython
+ pip install -qr requirements.txt -f https://download.pytorch.org/whl/cpu/torch_stable.html
+ pip install requests
+ python --version
+ pip --version
+ pip list
+ cd
+ shell: bash
+ - name: Tests workflow
+ run: |
+ # STRONGSORT
+ python track.py --tracking-method strongsort --yolo-weights ./weights/yolov5n.pt --source yolov5/data/images/bus.jpg
+ # OCSORT
+ python track.py --tracking-method ocsort --yolo-weights ./weights/yolov5n.pt --source yolov5/data/images/bus.jpg
+ # BYTETRACK
+ python track.py --tracking-method bytetrack --yolo-weights ./weights/yolov5n.pt --source yolov5/data/images/bus.jpg
+ # EXPORT
+ python reid_export.py --weights ./weights/osnet_x0_25_msmt17.pt # export deafults to torchscript
+ # STRONGSORT w. EXPORTED REID MODEL
+ python track.py --reid-weights ./weights/osnet_x0_25_msmt17.torchscript --source yolov5/data/images/bus.jpg
+ shell: bash
\ No newline at end of file
diff --git a/.github/workflows/stale.yml b/.github/workflows/stale.yml
new file mode 100644
index 0000000000000000000000000000000000000000..3beec5f982b639a743cc4e48a8b6b9fd48c6d679
--- /dev/null
+++ b/.github/workflows/stale.yml
@@ -0,0 +1,20 @@
+name: Close stale issues
+on:
+ schedule:
+ - cron: "0 0 * * *" # At the end of every day
+
+jobs:
+ stale:
+ runs-on: ubuntu-latest
+ steps:
+ - uses: actions/stale@v3
+ with:
+ repo-token: ${{ secrets.GITHUB_TOKEN }}
+ stale-issue-message: |
+ 👋 Hello, this issue has been automatically marked as stale because it has not had recent activity. Please note it will be closed if no further activity occurs.
+ Feel free to inform us of any other **issues** you discover or **feature requests** that come to mind in the future. Pull Requests (PRs) are also always welcomed!
+ stale-pr-message: 'This issue has been automatically marked as stale because it has not had recent activity. It will be closed if no further activity occurs.'
+ days-before-stale: 30
+ days-before-close: 5
+ exempt-issue-labels: 'documentation,tutorial'
+ operations-per-run: 100 # The maximum number of operations per run, used to control rate limiting.
\ No newline at end of file
diff --git a/.gitignore b/.gitignore
new file mode 100644
index 0000000000000000000000000000000000000000..630998c347acb87e24744c3c9c68c3208a39a71a
--- /dev/null
+++ b/.gitignore
@@ -0,0 +1,11 @@
+.vscode/
+
+# interpreter bytecode
+__pycache__/
+
+# exports
+*_openvino_model
+*.torchscript
+*.pt
+*.onnx
+*.engine
\ No newline at end of file
diff --git a/.gitmodules b/.gitmodules
new file mode 100644
index 0000000000000000000000000000000000000000..e1ba3d9dbc1f3cb0f2204c55c051afc7d4435721
--- /dev/null
+++ b/.gitmodules
@@ -0,0 +1,6 @@
+[submodule "yolov5"]
+ path = yolov5
+ url = https://github.com/ultralytics/yolov5.git
+[submodule "trackers/strong_sort/deep/reid"]
+ path = trackers/strong_sort/deep/reid
+ url = https://github.com/KaiyangZhou/deep-person-reid
diff --git a/.idea/.gitignore b/.idea/.gitignore
new file mode 100644
index 0000000000000000000000000000000000000000..359bb5307e8535ab7d59faf27a7377033291821e
--- /dev/null
+++ b/.idea/.gitignore
@@ -0,0 +1,3 @@
+# 默认忽略的文件
+/shelf/
+/workspace.xml
diff --git a/.idea/inspectionProfiles/Project_Default.xml b/.idea/inspectionProfiles/Project_Default.xml
new file mode 100644
index 0000000000000000000000000000000000000000..ab7e8af7600865ea5c4d29cc75d152456f303194
--- /dev/null
+++ b/.idea/inspectionProfiles/Project_Default.xml
@@ -0,0 +1,26 @@
+
+
+
+
+
+
+
+
+
+
+
\ No newline at end of file
diff --git a/.idea/inspectionProfiles/profiles_settings.xml b/.idea/inspectionProfiles/profiles_settings.xml
new file mode 100644
index 0000000000000000000000000000000000000000..105ce2da2d6447d11dfe32bfb846c3d5b199fc99
--- /dev/null
+++ b/.idea/inspectionProfiles/profiles_settings.xml
@@ -0,0 +1,6 @@
+
+
+
+
+
+
\ No newline at end of file
diff --git a/.idea/misc.xml b/.idea/misc.xml
new file mode 100644
index 0000000000000000000000000000000000000000..e4c68f8253267bed823c757b5e6cc0bb39a426f9
--- /dev/null
+++ b/.idea/misc.xml
@@ -0,0 +1,4 @@
+
+
+
+
\ No newline at end of file
diff --git a/.idea/modules.xml b/.idea/modules.xml
new file mode 100644
index 0000000000000000000000000000000000000000..3fae000cd7a68ab2f3ebb9440ed71b005999de2b
--- /dev/null
+++ b/.idea/modules.xml
@@ -0,0 +1,8 @@
+
+
+
+
+
+
+
+
\ No newline at end of file
diff --git a/.idea/vcs.xml b/.idea/vcs.xml
new file mode 100644
index 0000000000000000000000000000000000000000..015d0c6753bc7d1e7a20e3bda33ae32e9db9db44
--- /dev/null
+++ b/.idea/vcs.xml
@@ -0,0 +1,6 @@
+
+
+
+
+
+
\ No newline at end of file
diff --git a/.idea/workspace.xml b/.idea/workspace.xml
new file mode 100644
index 0000000000000000000000000000000000000000..e1220e5b3dbbea063716c9bc03a740a7ae6158df
--- /dev/null
+++ b/.idea/workspace.xml
@@ -0,0 +1,181 @@
+
+
+
+
+
+
+
+
+
+
+
+
+
+
+
+
+
+
+
+
+
+
+
+
+
+
+
+
+
+
+
+
+
+
+
+
+
+
+
+
+
+
+
+
+
+
+
+
+
+
+
+
+
+
+
+
+
+
+
+
+
+
+
+
+
+
+
+
+
+
+
+
+
+
+
+
+
+
+
+
+
+
+
+
+
+
+
+
+
+
+
+
+
+
+
+
+
+
+
+
+
+
+
+
+
+
+
+
+
+
+
+
+
+
+
+
+
+
+
+
+
+
+
+
+
+
+
+
+
+
+
+
+
+
+
+
+
+
+
+
+
+
+
+
+
+
+
+
+
+
+
+
+
+
+
+
+
+
+
+
+
+
+
+
+
+
+
+
+
+
+
+
+
+ 1684489383896
+
+
+ 1684489383896
+
+
+
+
\ No newline at end of file
diff --git a/.idea/yolov5_tracking-8.0.iml b/.idea/yolov5_tracking-8.0.iml
new file mode 100644
index 0000000000000000000000000000000000000000..714f0689396031a1f9b2b0af71c93e685078689a
--- /dev/null
+++ b/.idea/yolov5_tracking-8.0.iml
@@ -0,0 +1,12 @@
+
+
+
+
+
+
+
+
+
+
+
+
\ No newline at end of file
diff --git a/LICENSE b/LICENSE
new file mode 100644
index 0000000000000000000000000000000000000000..92b370f0e0e1b91cf8baf5d0f78c56a9824c39f1
--- /dev/null
+++ b/LICENSE
@@ -0,0 +1,674 @@
+GNU GENERAL PUBLIC LICENSE
+ Version 3, 29 June 2007
+
+ Copyright (C) 2007 Free Software Foundation, Inc.
+ Everyone is permitted to copy and distribute verbatim copies
+ of this license document, but changing it is not allowed.
+
+ Preamble
+
+ The GNU General Public License is a free, copyleft license for
+software and other kinds of works.
+
+ The licenses for most software and other practical works are designed
+to take away your freedom to share and change the works. By contrast,
+the GNU General Public License is intended to guarantee your freedom to
+share and change all versions of a program--to make sure it remains free
+software for all its users. We, the Free Software Foundation, use the
+GNU General Public License for most of our software; it applies also to
+any other work released this way by its authors. You can apply it to
+your programs, too.
+
+ When we speak of free software, we are referring to freedom, not
+price. Our General Public Licenses are designed to make sure that you
+have the freedom to distribute copies of free software (and charge for
+them if you wish), that you receive source code or can get it if you
+want it, that you can change the software or use pieces of it in new
+free programs, and that you know you can do these things.
+
+ To protect your rights, we need to prevent others from denying you
+these rights or asking you to surrender the rights. Therefore, you have
+certain responsibilities if you distribute copies of the software, or if
+you modify it: responsibilities to respect the freedom of others.
+
+ For example, if you distribute copies of such a program, whether
+gratis or for a fee, you must pass on to the recipients the same
+freedoms that you received. You must make sure that they, too, receive
+or can get the source code. And you must show them these terms so they
+know their rights.
+
+ Developers that use the GNU GPL protect your rights with two steps:
+(1) assert copyright on the software, and (2) offer you this License
+giving you legal permission to copy, distribute and/or modify it.
+
+ For the developers' and authors' protection, the GPL clearly explains
+that there is no warranty for this free software. For both users' and
+authors' sake, the GPL requires that modified versions be marked as
+changed, so that their problems will not be attributed erroneously to
+authors of previous versions.
+
+ Some devices are designed to deny users access to install or run
+modified versions of the software inside them, although the manufacturer
+can do so. This is fundamentally incompatible with the aim of
+protecting users' freedom to change the software. The systematic
+pattern of such abuse occurs in the area of products for individuals to
+use, which is precisely where it is most unacceptable. Therefore, we
+have designed this version of the GPL to prohibit the practice for those
+products. If such problems arise substantially in other domains, we
+stand ready to extend this provision to those domains in future versions
+of the GPL, as needed to protect the freedom of users.
+
+ Finally, every program is threatened constantly by software patents.
+States should not allow patents to restrict development and use of
+software on general-purpose computers, but in those that do, we wish to
+avoid the special danger that patents applied to a free program could
+make it effectively proprietary. To prevent this, the GPL assures that
+patents cannot be used to render the program non-free.
+
+ The precise terms and conditions for copying, distribution and
+modification follow.
+
+ TERMS AND CONDITIONS
+
+ 0. Definitions.
+
+ "This License" refers to version 3 of the GNU General Public License.
+
+ "Copyright" also means copyright-like laws that apply to other kinds of
+works, such as semiconductor masks.
+
+ "The Program" refers to any copyrightable work licensed under this
+License. Each licensee is addressed as "you". "Licensees" and
+"recipients" may be individuals or organizations.
+
+ To "modify" a work means to copy from or adapt all or part of the work
+in a fashion requiring copyright permission, other than the making of an
+exact copy. The resulting work is called a "modified version" of the
+earlier work or a work "based on" the earlier work.
+
+ A "covered work" means either the unmodified Program or a work based
+on the Program.
+
+ To "propagate" a work means to do anything with it that, without
+permission, would make you directly or secondarily liable for
+infringement under applicable copyright law, except executing it on a
+computer or modifying a private copy. Propagation includes copying,
+distribution (with or without modification), making available to the
+public, and in some countries other activities as well.
+
+ To "convey" a work means any kind of propagation that enables other
+parties to make or receive copies. Mere interaction with a user through
+a computer network, with no transfer of a copy, is not conveying.
+
+ An interactive user interface displays "Appropriate Legal Notices"
+to the extent that it includes a convenient and prominently visible
+feature that (1) displays an appropriate copyright notice, and (2)
+tells the user that there is no warranty for the work (except to the
+extent that warranties are provided), that licensees may convey the
+work under this License, and how to view a copy of this License. If
+the interface presents a list of user commands or options, such as a
+menu, a prominent item in the list meets this criterion.
+
+ 1. Source Code.
+
+ The "source code" for a work means the preferred form of the work
+for making modifications to it. "Object code" means any non-source
+form of a work.
+
+ A "Standard Interface" means an interface that either is an official
+standard defined by a recognized standards body, or, in the case of
+interfaces specified for a particular programming language, one that
+is widely used among developers working in that language.
+
+ The "System Libraries" of an executable work include anything, other
+than the work as a whole, that (a) is included in the normal form of
+packaging a Major Component, but which is not part of that Major
+Component, and (b) serves only to enable use of the work with that
+Major Component, or to implement a Standard Interface for which an
+implementation is available to the public in source code form. A
+"Major Component", in this context, means a major essential component
+(kernel, window system, and so on) of the specific operating system
+(if any) on which the executable work runs, or a compiler used to
+produce the work, or an object code interpreter used to run it.
+
+ The "Corresponding Source" for a work in object code form means all
+the source code needed to generate, install, and (for an executable
+work) run the object code and to modify the work, including scripts to
+control those activities. However, it does not include the work's
+System Libraries, or general-purpose tools or generally available free
+programs which are used unmodified in performing those activities but
+which are not part of the work. For example, Corresponding Source
+includes interface definition files associated with source files for
+the work, and the source code for shared libraries and dynamically
+linked subprograms that the work is specifically designed to require,
+such as by intimate data communication or control flow between those
+subprograms and other parts of the work.
+
+ The Corresponding Source need not include anything that users
+can regenerate automatically from other parts of the Corresponding
+Source.
+
+ The Corresponding Source for a work in source code form is that
+same work.
+
+ 2. Basic Permissions.
+
+ All rights granted under this License are granted for the term of
+copyright on the Program, and are irrevocable provided the stated
+conditions are met. This License explicitly affirms your unlimited
+permission to run the unmodified Program. The output from running a
+covered work is covered by this License only if the output, given its
+content, constitutes a covered work. This License acknowledges your
+rights of fair use or other equivalent, as provided by copyright law.
+
+ You may make, run and propagate covered works that you do not
+convey, without conditions so long as your license otherwise remains
+in force. You may convey covered works to others for the sole purpose
+of having them make modifications exclusively for you, or provide you
+with facilities for running those works, provided that you comply with
+the terms of this License in conveying all material for which you do
+not control copyright. Those thus making or running the covered works
+for you must do so exclusively on your behalf, under your direction
+and control, on terms that prohibit them from making any copies of
+your copyrighted material outside their relationship with you.
+
+ Conveying under any other circumstances is permitted solely under
+the conditions stated below. Sublicensing is not allowed; section 10
+makes it unnecessary.
+
+ 3. Protecting Users' Legal Rights From Anti-Circumvention Law.
+
+ No covered work shall be deemed part of an effective technological
+measure under any applicable law fulfilling obligations under article
+11 of the WIPO copyright treaty adopted on 20 December 1996, or
+similar laws prohibiting or restricting circumvention of such
+measures.
+
+ When you convey a covered work, you waive any legal power to forbid
+circumvention of technological measures to the extent such circumvention
+is effected by exercising rights under this License with respect to
+the covered work, and you disclaim any intention to limit operation or
+modification of the work as a means of enforcing, against the work's
+users, your or third parties' legal rights to forbid circumvention of
+technological measures.
+
+ 4. Conveying Verbatim Copies.
+
+ You may convey verbatim copies of the Program's source code as you
+receive it, in any medium, provided that you conspicuously and
+appropriately publish on each copy an appropriate copyright notice;
+keep intact all notices stating that this License and any
+non-permissive terms added in accord with section 7 apply to the code;
+keep intact all notices of the absence of any warranty; and give all
+recipients a copy of this License along with the Program.
+
+ You may charge any price or no price for each copy that you convey,
+and you may offer support or warranty protection for a fee.
+
+ 5. Conveying Modified Source Versions.
+
+ You may convey a work based on the Program, or the modifications to
+produce it from the Program, in the form of source code under the
+terms of section 4, provided that you also meet all of these conditions:
+
+ a) The work must carry prominent notices stating that you modified
+ it, and giving a relevant date.
+
+ b) The work must carry prominent notices stating that it is
+ released under this License and any conditions added under section
+ 7. This requirement modifies the requirement in section 4 to
+ "keep intact all notices".
+
+ c) You must license the entire work, as a whole, under this
+ License to anyone who comes into possession of a copy. This
+ License will therefore apply, along with any applicable section 7
+ additional terms, to the whole of the work, and all its parts,
+ regardless of how they are packaged. This License gives no
+ permission to license the work in any other way, but it does not
+ invalidate such permission if you have separately received it.
+
+ d) If the work has interactive user interfaces, each must display
+ Appropriate Legal Notices; however, if the Program has interactive
+ interfaces that do not display Appropriate Legal Notices, your
+ work need not make them do so.
+
+ A compilation of a covered work with other separate and independent
+works, which are not by their nature extensions of the covered work,
+and which are not combined with it such as to form a larger program,
+in or on a volume of a storage or distribution medium, is called an
+"aggregate" if the compilation and its resulting copyright are not
+used to limit the access or legal rights of the compilation's users
+beyond what the individual works permit. Inclusion of a covered work
+in an aggregate does not cause this License to apply to the other
+parts of the aggregate.
+
+ 6. Conveying Non-Source Forms.
+
+ You may convey a covered work in object code form under the terms
+of sections 4 and 5, provided that you also convey the
+machine-readable Corresponding Source under the terms of this License,
+in one of these ways:
+
+ a) Convey the object code in, or embodied in, a physical product
+ (including a physical distribution medium), accompanied by the
+ Corresponding Source fixed on a durable physical medium
+ customarily used for software interchange.
+
+ b) Convey the object code in, or embodied in, a physical product
+ (including a physical distribution medium), accompanied by a
+ written offer, valid for at least three years and valid for as
+ long as you offer spare parts or customer support for that product
+ model, to give anyone who possesses the object code either (1) a
+ copy of the Corresponding Source for all the software in the
+ product that is covered by this License, on a durable physical
+ medium customarily used for software interchange, for a price no
+ more than your reasonable cost of physically performing this
+ conveying of source, or (2) access to copy the
+ Corresponding Source from a network server at no charge.
+
+ c) Convey individual copies of the object code with a copy of the
+ written offer to provide the Corresponding Source. This
+ alternative is allowed only occasionally and noncommercially, and
+ only if you received the object code with such an offer, in accord
+ with subsection 6b.
+
+ d) Convey the object code by offering access from a designated
+ place (gratis or for a charge), and offer equivalent access to the
+ Corresponding Source in the same way through the same place at no
+ further charge. You need not require recipients to copy the
+ Corresponding Source along with the object code. If the place to
+ copy the object code is a network server, the Corresponding Source
+ may be on a different server (operated by you or a third party)
+ that supports equivalent copying facilities, provided you maintain
+ clear directions next to the object code saying where to find the
+ Corresponding Source. Regardless of what server hosts the
+ Corresponding Source, you remain obligated to ensure that it is
+ available for as long as needed to satisfy these requirements.
+
+ e) Convey the object code using peer-to-peer transmission, provided
+ you inform other peers where the object code and Corresponding
+ Source of the work are being offered to the general public at no
+ charge under subsection 6d.
+
+ A separable portion of the object code, whose source code is excluded
+from the Corresponding Source as a System Library, need not be
+included in conveying the object code work.
+
+ A "User Product" is either (1) a "consumer product", which means any
+tangible personal property which is normally used for personal, family,
+or household purposes, or (2) anything designed or sold for incorporation
+into a dwelling. In determining whether a product is a consumer product,
+doubtful cases shall be resolved in favor of coverage. For a particular
+product received by a particular user, "normally used" refers to a
+typical or common use of that class of product, regardless of the status
+of the particular user or of the way in which the particular user
+actually uses, or expects or is expected to use, the product. A product
+is a consumer product regardless of whether the product has substantial
+commercial, industrial or non-consumer uses, unless such uses represent
+the only significant mode of use of the product.
+
+ "Installation Information" for a User Product means any methods,
+procedures, authorization keys, or other information required to install
+and execute modified versions of a covered work in that User Product from
+a modified version of its Corresponding Source. The information must
+suffice to ensure that the continued functioning of the modified object
+code is in no case prevented or interfered with solely because
+modification has been made.
+
+ If you convey an object code work under this section in, or with, or
+specifically for use in, a User Product, and the conveying occurs as
+part of a transaction in which the right of possession and use of the
+User Product is transferred to the recipient in perpetuity or for a
+fixed term (regardless of how the transaction is characterized), the
+Corresponding Source conveyed under this section must be accompanied
+by the Installation Information. But this requirement does not apply
+if neither you nor any third party retains the ability to install
+modified object code on the User Product (for example, the work has
+been installed in ROM).
+
+ The requirement to provide Installation Information does not include a
+requirement to continue to provide support service, warranty, or updates
+for a work that has been modified or installed by the recipient, or for
+the User Product in which it has been modified or installed. Access to a
+network may be denied when the modification itself materially and
+adversely affects the operation of the network or violates the rules and
+protocols for communication across the network.
+
+ Corresponding Source conveyed, and Installation Information provided,
+in accord with this section must be in a format that is publicly
+documented (and with an implementation available to the public in
+source code form), and must require no special password or key for
+unpacking, reading or copying.
+
+ 7. Additional Terms.
+
+ "Additional permissions" are terms that supplement the terms of this
+License by making exceptions from one or more of its conditions.
+Additional permissions that are applicable to the entire Program shall
+be treated as though they were included in this License, to the extent
+that they are valid under applicable law. If additional permissions
+apply only to part of the Program, that part may be used separately
+under those permissions, but the entire Program remains governed by
+this License without regard to the additional permissions.
+
+ When you convey a copy of a covered work, you may at your option
+remove any additional permissions from that copy, or from any part of
+it. (Additional permissions may be written to require their own
+removal in certain cases when you modify the work.) You may place
+additional permissions on material, added by you to a covered work,
+for which you have or can give appropriate copyright permission.
+
+ Notwithstanding any other provision of this License, for material you
+add to a covered work, you may (if authorized by the copyright holders of
+that material) supplement the terms of this License with terms:
+
+ a) Disclaiming warranty or limiting liability differently from the
+ terms of sections 15 and 16 of this License; or
+
+ b) Requiring preservation of specified reasonable legal notices or
+ author attributions in that material or in the Appropriate Legal
+ Notices displayed by works containing it; or
+
+ c) Prohibiting misrepresentation of the origin of that material, or
+ requiring that modified versions of such material be marked in
+ reasonable ways as different from the original version; or
+
+ d) Limiting the use for publicity purposes of names of licensors or
+ authors of the material; or
+
+ e) Declining to grant rights under trademark law for use of some
+ trade names, trademarks, or service marks; or
+
+ f) Requiring indemnification of licensors and authors of that
+ material by anyone who conveys the material (or modified versions of
+ it) with contractual assumptions of liability to the recipient, for
+ any liability that these contractual assumptions directly impose on
+ those licensors and authors.
+
+ All other non-permissive additional terms are considered "further
+restrictions" within the meaning of section 10. If the Program as you
+received it, or any part of it, contains a notice stating that it is
+governed by this License along with a term that is a further
+restriction, you may remove that term. If a license document contains
+a further restriction but permits relicensing or conveying under this
+License, you may add to a covered work material governed by the terms
+of that license document, provided that the further restriction does
+not survive such relicensing or conveying.
+
+ If you add terms to a covered work in accord with this section, you
+must place, in the relevant source files, a statement of the
+additional terms that apply to those files, or a notice indicating
+where to find the applicable terms.
+
+ Additional terms, permissive or non-permissive, may be stated in the
+form of a separately written license, or stated as exceptions;
+the above requirements apply either way.
+
+ 8. Termination.
+
+ You may not propagate or modify a covered work except as expressly
+provided under this License. Any attempt otherwise to propagate or
+modify it is void, and will automatically terminate your rights under
+this License (including any patent licenses granted under the third
+paragraph of section 11).
+
+ However, if you cease all violation of this License, then your
+license from a particular copyright holder is reinstated (a)
+provisionally, unless and until the copyright holder explicitly and
+finally terminates your license, and (b) permanently, if the copyright
+holder fails to notify you of the violation by some reasonable means
+prior to 60 days after the cessation.
+
+ Moreover, your license from a particular copyright holder is
+reinstated permanently if the copyright holder notifies you of the
+violation by some reasonable means, this is the first time you have
+received notice of violation of this License (for any work) from that
+copyright holder, and you cure the violation prior to 30 days after
+your receipt of the notice.
+
+ Termination of your rights under this section does not terminate the
+licenses of parties who have received copies or rights from you under
+this License. If your rights have been terminated and not permanently
+reinstated, you do not qualify to receive new licenses for the same
+material under section 10.
+
+ 9. Acceptance Not Required for Having Copies.
+
+ You are not required to accept this License in order to receive or
+run a copy of the Program. Ancillary propagation of a covered work
+occurring solely as a consequence of using peer-to-peer transmission
+to receive a copy likewise does not require acceptance. However,
+nothing other than this License grants you permission to propagate or
+modify any covered work. These actions infringe copyright if you do
+not accept this License. Therefore, by modifying or propagating a
+covered work, you indicate your acceptance of this License to do so.
+
+ 10. Automatic Licensing of Downstream Recipients.
+
+ Each time you convey a covered work, the recipient automatically
+receives a license from the original licensors, to run, modify and
+propagate that work, subject to this License. You are not responsible
+for enforcing compliance by third parties with this License.
+
+ An "entity transaction" is a transaction transferring control of an
+organization, or substantially all assets of one, or subdividing an
+organization, or merging organizations. If propagation of a covered
+work results from an entity transaction, each party to that
+transaction who receives a copy of the work also receives whatever
+licenses to the work the party's predecessor in interest had or could
+give under the previous paragraph, plus a right to possession of the
+Corresponding Source of the work from the predecessor in interest, if
+the predecessor has it or can get it with reasonable efforts.
+
+ You may not impose any further restrictions on the exercise of the
+rights granted or affirmed under this License. For example, you may
+not impose a license fee, royalty, or other charge for exercise of
+rights granted under this License, and you may not initiate litigation
+(including a cross-claim or counterclaim in a lawsuit) alleging that
+any patent claim is infringed by making, using, selling, offering for
+sale, or importing the Program or any portion of it.
+
+ 11. Patents.
+
+ A "contributor" is a copyright holder who authorizes use under this
+License of the Program or a work on which the Program is based. The
+work thus licensed is called the contributor's "contributor version".
+
+ A contributor's "essential patent claims" are all patent claims
+owned or controlled by the contributor, whether already acquired or
+hereafter acquired, that would be infringed by some manner, permitted
+by this License, of making, using, or selling its contributor version,
+but do not include claims that would be infringed only as a
+consequence of further modification of the contributor version. For
+purposes of this definition, "control" includes the right to grant
+patent sublicenses in a manner consistent with the requirements of
+this License.
+
+ Each contributor grants you a non-exclusive, worldwide, royalty-free
+patent license under the contributor's essential patent claims, to
+make, use, sell, offer for sale, import and otherwise run, modify and
+propagate the contents of its contributor version.
+
+ In the following three paragraphs, a "patent license" is any express
+agreement or commitment, however denominated, not to enforce a patent
+(such as an express permission to practice a patent or covenant not to
+sue for patent infringement). To "grant" such a patent license to a
+party means to make such an agreement or commitment not to enforce a
+patent against the party.
+
+ If you convey a covered work, knowingly relying on a patent license,
+and the Corresponding Source of the work is not available for anyone
+to copy, free of charge and under the terms of this License, through a
+publicly available network server or other readily accessible means,
+then you must either (1) cause the Corresponding Source to be so
+available, or (2) arrange to deprive yourself of the benefit of the
+patent license for this particular work, or (3) arrange, in a manner
+consistent with the requirements of this License, to extend the patent
+license to downstream recipients. "Knowingly relying" means you have
+actual knowledge that, but for the patent license, your conveying the
+covered work in a country, or your recipient's use of the covered work
+in a country, would infringe one or more identifiable patents in that
+country that you have reason to believe are valid.
+
+ If, pursuant to or in connection with a single transaction or
+arrangement, you convey, or propagate by procuring conveyance of, a
+covered work, and grant a patent license to some of the parties
+receiving the covered work authorizing them to use, propagate, modify
+or convey a specific copy of the covered work, then the patent license
+you grant is automatically extended to all recipients of the covered
+work and works based on it.
+
+ A patent license is "discriminatory" if it does not include within
+the scope of its coverage, prohibits the exercise of, or is
+conditioned on the non-exercise of one or more of the rights that are
+specifically granted under this License. You may not convey a covered
+work if you are a party to an arrangement with a third party that is
+in the business of distributing software, under which you make payment
+to the third party based on the extent of your activity of conveying
+the work, and under which the third party grants, to any of the
+parties who would receive the covered work from you, a discriminatory
+patent license (a) in connection with copies of the covered work
+conveyed by you (or copies made from those copies), or (b) primarily
+for and in connection with specific products or compilations that
+contain the covered work, unless you entered into that arrangement,
+or that patent license was granted, prior to 28 March 2007.
+
+ Nothing in this License shall be construed as excluding or limiting
+any implied license or other defenses to infringement that may
+otherwise be available to you under applicable patent law.
+
+ 12. No Surrender of Others' Freedom.
+
+ If conditions are imposed on you (whether by court order, agreement or
+otherwise) that contradict the conditions of this License, they do not
+excuse you from the conditions of this License. If you cannot convey a
+covered work so as to satisfy simultaneously your obligations under this
+License and any other pertinent obligations, then as a consequence you may
+not convey it at all. For example, if you agree to terms that obligate you
+to collect a royalty for further conveying from those to whom you convey
+the Program, the only way you could satisfy both those terms and this
+License would be to refrain entirely from conveying the Program.
+
+ 13. Use with the GNU Affero General Public License.
+
+ Notwithstanding any other provision of this License, you have
+permission to link or combine any covered work with a work licensed
+under version 3 of the GNU Affero General Public License into a single
+combined work, and to convey the resulting work. The terms of this
+License will continue to apply to the part which is the covered work,
+but the special requirements of the GNU Affero General Public License,
+section 13, concerning interaction through a network will apply to the
+combination as such.
+
+ 14. Revised Versions of this License.
+
+ The Free Software Foundation may publish revised and/or new versions of
+the GNU General Public License from time to time. Such new versions will
+be similar in spirit to the present version, but may differ in detail to
+address new problems or concerns.
+
+ Each version is given a distinguishing version number. If the
+Program specifies that a certain numbered version of the GNU General
+Public License "or any later version" applies to it, you have the
+option of following the terms and conditions either of that numbered
+version or of any later version published by the Free Software
+Foundation. If the Program does not specify a version number of the
+GNU General Public License, you may choose any version ever published
+by the Free Software Foundation.
+
+ If the Program specifies that a proxy can decide which future
+versions of the GNU General Public License can be used, that proxy's
+public statement of acceptance of a version permanently authorizes you
+to choose that version for the Program.
+
+ Later license versions may give you additional or different
+permissions. However, no additional obligations are imposed on any
+author or copyright holder as a result of your choosing to follow a
+later version.
+
+ 15. Disclaimer of Warranty.
+
+ THERE IS NO WARRANTY FOR THE PROGRAM, TO THE EXTENT PERMITTED BY
+APPLICABLE LAW. EXCEPT WHEN OTHERWISE STATED IN WRITING THE COPYRIGHT
+HOLDERS AND/OR OTHER PARTIES PROVIDE THE PROGRAM "AS IS" WITHOUT WARRANTY
+OF ANY KIND, EITHER EXPRESSED OR IMPLIED, INCLUDING, BUT NOT LIMITED TO,
+THE IMPLIED WARRANTIES OF MERCHANTABILITY AND FITNESS FOR A PARTICULAR
+PURPOSE. THE ENTIRE RISK AS TO THE QUALITY AND PERFORMANCE OF THE PROGRAM
+IS WITH YOU. SHOULD THE PROGRAM PROVE DEFECTIVE, YOU ASSUME THE COST OF
+ALL NECESSARY SERVICING, REPAIR OR CORRECTION.
+
+ 16. Limitation of Liability.
+
+ IN NO EVENT UNLESS REQUIRED BY APPLICABLE LAW OR AGREED TO IN WRITING
+WILL ANY COPYRIGHT HOLDER, OR ANY OTHER PARTY WHO MODIFIES AND/OR CONVEYS
+THE PROGRAM AS PERMITTED ABOVE, BE LIABLE TO YOU FOR DAMAGES, INCLUDING ANY
+GENERAL, SPECIAL, INCIDENTAL OR CONSEQUENTIAL DAMAGES ARISING OUT OF THE
+USE OR INABILITY TO USE THE PROGRAM (INCLUDING BUT NOT LIMITED TO LOSS OF
+DATA OR DATA BEING RENDERED INACCURATE OR LOSSES SUSTAINED BY YOU OR THIRD
+PARTIES OR A FAILURE OF THE PROGRAM TO OPERATE WITH ANY OTHER PROGRAMS),
+EVEN IF SUCH HOLDER OR OTHER PARTY HAS BEEN ADVISED OF THE POSSIBILITY OF
+SUCH DAMAGES.
+
+ 17. Interpretation of Sections 15 and 16.
+
+ If the disclaimer of warranty and limitation of liability provided
+above cannot be given local legal effect according to their terms,
+reviewing courts shall apply local law that most closely approximates
+an absolute waiver of all civil liability in connection with the
+Program, unless a warranty or assumption of liability accompanies a
+copy of the Program in return for a fee.
+
+ END OF TERMS AND CONDITIONS
+
+ How to Apply These Terms to Your New Programs
+
+ If you develop a new program, and you want it to be of the greatest
+possible use to the public, the best way to achieve this is to make it
+free software which everyone can redistribute and change under these terms.
+
+ To do so, attach the following notices to the program. It is safest
+to attach them to the start of each source file to most effectively
+state the exclusion of warranty; and each file should have at least
+the "copyright" line and a pointer to where the full notice is found.
+
+
+ Copyright (C)
+
+ This program is free software: you can redistribute it and/or modify
+ it under the terms of the GNU General Public License as published by
+ the Free Software Foundation, either version 3 of the License, or
+ (at your option) any later version.
+
+ This program is distributed in the hope that it will be useful,
+ but WITHOUT ANY WARRANTY; without even the implied warranty of
+ MERCHANTABILITY or FITNESS FOR A PARTICULAR PURPOSE. See the
+ GNU General Public License for more details.
+
+ You should have received a copy of the GNU General Public License
+ along with this program. If not, see .
+
+Also add information on how to contact you by electronic and paper mail.
+
+ If the program does terminal interaction, make it output a short
+notice like this when it starts in an interactive mode:
+
+ Copyright (C)
+ This program comes with ABSOLUTELY NO WARRANTY; for details type `show w'.
+ This is free software, and you are welcome to redistribute it
+ under certain conditions; type `show c' for details.
+
+The hypothetical commands `show w' and `show c' should show the appropriate
+parts of the General Public License. Of course, your program's commands
+might be different; for a GUI interface, you would use an "about box".
+
+ You should also get your employer (if you work as a programmer) or school,
+if any, to sign a "copyright disclaimer" for the program, if necessary.
+For more information on this, and how to apply and follow the GNU GPL, see
+.
+
+ The GNU General Public License does not permit incorporating your program
+into proprietary programs. If your program is a subroutine library, you
+may consider it more useful to permit linking proprietary applications with
+the library. If this is what you want to do, use the GNU Lesser General
+Public License instead of this License. But first, please read
+.
diff --git a/__pycache__/track.cpython-38.pyc b/__pycache__/track.cpython-38.pyc
new file mode 100644
index 0000000000000000000000000000000000000000..c5575cacdcacd323ca4ced84208adcb370c29a41
Binary files /dev/null and b/__pycache__/track.cpython-38.pyc differ
diff --git a/demo.py b/demo.py
new file mode 100644
index 0000000000000000000000000000000000000000..37c03924b48dca84932104ec359f16cd0228ab52
--- /dev/null
+++ b/demo.py
@@ -0,0 +1,98 @@
+import gradio as gr
+import tempfile
+import os
+import track
+import shutil
+from pathlib import Path
+from yolov5 import detect
+from PIL import Image
+
+# 目标检测
+def Detect(image):
+ # 创建临时文件夹
+ temp_path = tempfile.TemporaryDirectory(dir="./")
+ temp_dir = temp_path.name
+ # 临时图片的路径
+ temp_image_path = os.path.join(temp_dir, f"temp.jpg")
+ # 存储临时图片
+ img = Image.fromarray(image)
+ img.save(temp_image_path)
+ # 结果图片的存储目录
+ temp_result_path = os.path.join(temp_dir, "tempresult")
+ # 对临时图片进行检测
+ detect.run(source=temp_image_path, data="test_image/FLIR.yaml", weights="weights/best.pt", project=f'./{temp_dir}',name = 'tempresult', hide_conf=False, conf_thres=0.35)
+ # 结果图片的路径
+ temp_result_path = os.path.join(temp_result_path, os.listdir(temp_result_path)[0])
+ # 读取结果图片
+ result_image = Image.open(temp_result_path).copy()
+ # 删除临时文件夹
+ temp_path.cleanup()
+ return result_image
+
+# 候选图片
+example_image= [
+ "./test_image/video-2SReBn5LtAkL5HMj2-frame-005072-MA7NCLQGoqq9aHaiL.jpg",
+ "./test_image/video-2rsjnZFyGQGeynfbv-frame-003708-6fPQbB7jtibwaYAE7.jpg",
+ "./test_image/video-2SReBn5LtAkL5HMj2-frame-000317-HTgPBFgZyPdwQnNvE.jpg",
+ "./test_image/video-jNQtRj6NGycZDEXpe-frame-002515-J3YntG8ntvZheKK3P.jpg",
+ "./test_image/video-kDDWXrnLSoSdHCZ7S-frame-003063-eaKjPvPskDPjenZ8S.jpg",
+ "./test_image/video-r68Yr9RPWEp5fW2ZF-frame-000333-X6K5iopqbmjKEsSqN.jpg"
+ ]
+
+# 目标追踪
+def Track(video, tracking_method):
+ # 存储临时视频的文件夹
+ temp_dir = "./temp"
+ # 先清空temp文件夹
+ shutil.rmtree("./temp")
+ os.mkdir("./temp")
+ # 获取视频的名字
+ video_name = os.path.basename(video)
+ # 对视频进行检测
+ track.run(source=video, yolo_weights=Path("weights/best2.pt"),reid_weights=Path("weights/osnet_x0_25_msmt17.pt") , project=Path(f'./{temp_dir}'),name = 'tempresult', tracking_method=tracking_method)
+ # 结果视频的路径
+ temp_result_path = os.path.join(f'./{temp_dir}', "tempresult", video_name)
+ # 返回结果视频的路径
+ return temp_result_path
+
+# 候选视频
+example_video= [
+ ["./video/5.mp4", None],
+ ["./video/bicyclecity.mp4", None],
+ ["./video/9.mp4", None],
+ ["./video/8.mp4", None],
+ ["./video/4.mp4", None],
+ ["./video/car.mp4", None],
+ ]
+
+iface_Image = gr.Interface(fn=Detect,
+ inputs=gr.Image(label="上传一张红外图像,仅支持jpg格式"),
+ outputs=gr.Image(label="检测结果"),
+ examples=example_image)
+
+iface_video = gr.Interface(fn=Track,
+ inputs=[gr.Video(label="上传段红外视频,仅支持mp4格式"), gr.Radio(["bytetrack", "strongsort"], label="track methond", info="选择追踪器", value="bytetrack")],
+ outputs=gr.Video(label="追踪结果"),
+ examples=example_video)
+
+demo = gr.TabbedInterface([iface_video, iface_Image], tab_names=["目标追踪", "目标检测"], title="红外目标检测追踪")
+
+demo.launch(share=True)
+
+
+
+
+
+
+
+
+
+
+
+
+
+
+
+
+
+
diff --git a/flagged/log.csv b/flagged/log.csv
new file mode 100644
index 0000000000000000000000000000000000000000..32cc3e54c013e10097dac8da36b11a48da43d60b
--- /dev/null
+++ b/flagged/log.csv
@@ -0,0 +1,2 @@
+text,output,flag,username,timestamp
+sfsda 东风东,SFSDA 东风东,,,2023-05-21 21:03:22.616101
diff --git a/reid_export.py b/reid_export.py
new file mode 100644
index 0000000000000000000000000000000000000000..cde0a70ad822b3c93b217df2fea6231fc6366b7c
--- /dev/null
+++ b/reid_export.py
@@ -0,0 +1,314 @@
+import argparse
+
+import os
+# limit the number of cpus used by high performance libraries
+os.environ["OMP_NUM_THREADS"] = "1"
+os.environ["OPENBLAS_NUM_THREADS"] = "1"
+os.environ["MKL_NUM_THREADS"] = "1"
+os.environ["VECLIB_MAXIMUM_THREADS"] = "1"
+os.environ["NUMEXPR_NUM_THREADS"] = "1"
+
+import sys
+import numpy as np
+from pathlib import Path
+import torch
+import time
+import platform
+import pandas as pd
+import subprocess
+import torch.backends.cudnn as cudnn
+from torch.utils.mobile_optimizer import optimize_for_mobile
+
+FILE = Path(__file__).resolve()
+ROOT = FILE.parents[0] # yolov5 strongsort root directory
+WEIGHTS = ROOT / 'weights'
+
+print(ROOT)
+
+if str(ROOT) not in sys.path:
+ sys.path.append(str(ROOT)) # add ROOT to PATH
+if str(ROOT / 'yolov5') not in sys.path:
+ sys.path.append(str(ROOT / 'yolov5')) # add yolov5 ROOT to PATH
+
+ROOT = Path(os.path.relpath(ROOT, Path.cwd())) # relative
+
+import logging
+from yolov5.utils.torch_utils import select_device
+from yolov5.models.common import DetectMultiBackend
+from yolov5.utils.general import LOGGER, colorstr, check_requirements, check_version
+from trackers.strong_sort.deep.models import build_model
+from trackers.strong_sort.deep.reid_model_factory import get_model_name, load_pretrained_weights
+
+
+def file_size(path):
+ # Return file/dir size (MB)
+ path = Path(path)
+ if path.is_file():
+ return path.stat().st_size / 1E6
+ elif path.is_dir():
+ return sum(f.stat().st_size for f in path.glob('**/*') if f.is_file()) / 1E6
+ else:
+ return 0.0
+
+
+def export_formats():
+ # YOLOv5 export formats
+ x = [
+ ['PyTorch', '-', '.pt', True, True],
+ ['TorchScript', 'torchscript', '.torchscript', True, True],
+ ['ONNX', 'onnx', '.onnx', True, True],
+ ['OpenVINO', 'openvino', '_openvino_model', True, False],
+ ['TensorRT', 'engine', '.engine', False, True],
+ ['TensorFlow Lite', 'tflite', '.tflite', True, False],
+ ]
+ return pd.DataFrame(x, columns=['Format', 'Argument', 'Suffix', 'CPU', 'GPU'])
+
+
+def export_torchscript(model, im, file, optimize, prefix=colorstr('TorchScript:')):
+ # YOLOv5 TorchScript model export
+ try:
+ LOGGER.info(f'\n{prefix} starting export with torch {torch.__version__}...')
+ f = file.with_suffix('.torchscript')
+
+ ts = torch.jit.trace(model, im, strict=False)
+ if optimize: # https://pytorch.org/tutorials/recipes/mobile_interpreter.html
+ optimize_for_mobile(ts)._save_for_lite_interpreter(str(f))
+ else:
+ ts.save(str(f))
+
+ LOGGER.info(f'{prefix} export success, saved as {f} ({file_size(f):.1f} MB)')
+ return f
+ except Exception as e:
+ LOGGER.info(f'{prefix} export failure: {e}')
+
+
+def export_onnx(model, im, file, opset, dynamic, simplify, prefix=colorstr('ONNX:')):
+ # ONNX export
+ try:
+ check_requirements(('onnx',))
+ import onnx
+
+ f = file.with_suffix('.onnx')
+ LOGGER.info(f'\n{prefix} starting export with onnx {onnx.__version__}...')
+
+ if dynamic:
+ dynamic = {'images': {0: 'batch'}} # shape(1,3,640,640)
+ dynamic['output'] = {0: 'batch'} # shape(1,25200,85)
+
+ torch.onnx.export(
+ model.cpu() if dynamic else model, # --dynamic only compatible with cpu
+ im.cpu() if dynamic else im,
+ f,
+ verbose=False,
+ opset_version=opset,
+ do_constant_folding=True,
+ input_names=['images'],
+ output_names=['output'],
+ dynamic_axes=dynamic or None
+ )
+ # Checks
+ model_onnx = onnx.load(f) # load onnx model
+ onnx.checker.check_model(model_onnx) # check onnx model
+ onnx.save(model_onnx, f)
+
+ # Simplify
+ if simplify:
+ try:
+ cuda = torch.cuda.is_available()
+ check_requirements(('onnxruntime-gpu' if cuda else 'onnxruntime', 'onnx-simplifier>=0.4.1'))
+ import onnxsim
+
+ LOGGER.info(f'simplifying with onnx-simplifier {onnxsim.__version__}...')
+ model_onnx, check = onnxsim.simplify(model_onnx)
+ assert check, 'assert check failed'
+ onnx.save(model_onnx, f)
+ except Exception as e:
+ LOGGER.info(f'simplifier failure: {e}')
+ LOGGER.info(f'{prefix} export success, saved as {f} ({file_size(f):.1f} MB)')
+ return f
+ except Exception as e:
+ LOGGER.info(f'export failure: {e}')
+
+
+
+def export_openvino(file, half, prefix=colorstr('OpenVINO:')):
+ # YOLOv5 OpenVINO export
+ check_requirements(('openvino-dev',)) # requires openvino-dev: https://pypi.org/project/openvino-dev/
+ import openvino.inference_engine as ie
+ try:
+ LOGGER.info(f'\n{prefix} starting export with openvino {ie.__version__}...')
+ f = str(file).replace('.pt', f'_openvino_model{os.sep}')
+
+ cmd = f"mo --input_model {file.with_suffix('.onnx')} --output_dir {f} --data_type {'FP16' if half else 'FP32'}"
+ subprocess.check_output(cmd.split()) # export
+ except Exception as e:
+ LOGGER.info(f'export failure: {e}')
+ LOGGER.info(f'{prefix} export success, saved as {f} ({file_size(f):.1f} MB)')
+ return f
+
+
+def export_tflite(file, half, prefix=colorstr('TFLite:')):
+ # YOLOv5 OpenVINO export
+ try:
+ check_requirements(('openvino2tensorflow', 'tensorflow', 'tensorflow_datasets')) # requires openvino-dev: https://pypi.org/project/openvino-dev/
+ import openvino.inference_engine as ie
+ LOGGER.info(f'\n{prefix} starting export with openvino {ie.__version__}...')
+ output = Path(str(file).replace(f'_openvino_model{os.sep}', f'_tflite_model{os.sep}'))
+ modelxml = list(Path(file).glob('*.xml'))[0]
+ cmd = f"openvino2tensorflow \
+ --model_path {modelxml} \
+ --model_output_path {output} \
+ --output_pb \
+ --output_saved_model \
+ --output_no_quant_float32_tflite \
+ --output_dynamic_range_quant_tflite"
+ subprocess.check_output(cmd.split()) # export
+
+ LOGGER.info(f'{prefix} export success, results saved in {output} ({file_size(f):.1f} MB)')
+ return f
+ except Exception as e:
+ LOGGER.info(f'\n{prefix} export failure: {e}')
+
+
+def export_engine(model, im, file, half, dynamic, simplify, workspace=4, verbose=False, prefix=colorstr('TensorRT:')):
+ # YOLOv5 TensorRT export https://developer.nvidia.com/tensorrt
+ try:
+ assert im.device.type != 'cpu', 'export running on CPU but must be on GPU, i.e. `python export.py --device 0`'
+ try:
+ import tensorrt as trt
+ except Exception:
+ if platform.system() == 'Linux':
+ check_requirements(('nvidia-tensorrt',), cmds=('-U --index-url https://pypi.ngc.nvidia.com',))
+ import tensorrt as trt
+
+ if trt.__version__[0] == '7': # TensorRT 7 handling https://github.com/ultralytics/yolov5/issues/6012
+ grid = model.model[-1].anchor_grid
+ model.model[-1].anchor_grid = [a[..., :1, :1, :] for a in grid]
+ export_onnx(model, im, file, 12, dynamic, simplify) # opset 12
+ model.model[-1].anchor_grid = grid
+ else: # TensorRT >= 8
+ check_version(trt.__version__, '8.0.0', hard=True) # require tensorrt>=8.0.0
+ export_onnx(model, im, file, 12, dynamic, simplify) # opset 13
+ onnx = file.with_suffix('.onnx')
+
+ LOGGER.info(f'\n{prefix} starting export with TensorRT {trt.__version__}...')
+ assert onnx.exists(), f'failed to export ONNX file: {onnx}'
+ f = file.with_suffix('.engine') # TensorRT engine file
+ logger = trt.Logger(trt.Logger.INFO)
+ if verbose:
+ logger.min_severity = trt.Logger.Severity.VERBOSE
+
+ builder = trt.Builder(logger)
+ config = builder.create_builder_config()
+ config.max_workspace_size = workspace * 1 << 30
+ # config.set_memory_pool_limit(trt.MemoryPoolType.WORKSPACE, workspace << 30) # fix TRT 8.4 deprecation notice
+
+ flag = (1 << int(trt.NetworkDefinitionCreationFlag.EXPLICIT_BATCH))
+ network = builder.create_network(flag)
+ parser = trt.OnnxParser(network, logger)
+ if not parser.parse_from_file(str(onnx)):
+ raise RuntimeError(f'failed to load ONNX file: {onnx}')
+
+ inputs = [network.get_input(i) for i in range(network.num_inputs)]
+ outputs = [network.get_output(i) for i in range(network.num_outputs)]
+ LOGGER.info(f'{prefix} Network Description:')
+ for inp in inputs:
+ LOGGER.info(f'{prefix}\tinput "{inp.name}" with shape {inp.shape} and dtype {inp.dtype}')
+ for out in outputs:
+ LOGGER.info(f'{prefix}\toutput "{out.name}" with shape {out.shape} and dtype {out.dtype}')
+
+ if dynamic:
+ if im.shape[0] <= 1:
+ LOGGER.warning(f"{prefix}WARNING: --dynamic model requires maximum --batch-size argument")
+ profile = builder.create_optimization_profile()
+ for inp in inputs:
+ profile.set_shape(inp.name, (1, *im.shape[1:]), (max(1, im.shape[0] // 2), *im.shape[1:]), im.shape)
+ config.add_optimization_profile(profile)
+
+ LOGGER.info(f'{prefix} building FP{16 if builder.platform_has_fast_fp16 and half else 32} engine in {f}')
+ if builder.platform_has_fast_fp16 and half:
+ config.set_flag(trt.BuilderFlag.FP16)
+ with builder.build_engine(network, config) as engine, open(f, 'wb') as t:
+ t.write(engine.serialize())
+ LOGGER.info(f'{prefix} export success, saved as {f} ({file_size(f):.1f} MB)')
+ return f
+ except Exception as e:
+ LOGGER.info(f'\n{prefix} export failure: {e}')
+
+
+if __name__ == "__main__":
+
+ parser = argparse.ArgumentParser(description="ReID export")
+ parser.add_argument('--batch-size', type=int, default=1, help='batch size')
+ parser.add_argument('--imgsz', '--img', '--img-size', nargs='+', type=int, default=[256, 128], help='image (h, w)')
+ parser.add_argument('--device', default='cpu', help='cuda device, i.e. 0 or 0,1,2,3 or cpu')
+ parser.add_argument('--optimize', action='store_true', help='TorchScript: optimize for mobile')
+ parser.add_argument('--dynamic', action='store_true', help='ONNX/TF/TensorRT: dynamic axes')
+ parser.add_argument('--simplify', action='store_true', help='ONNX: simplify model')
+ parser.add_argument('--opset', type=int, default=12, help='ONNX: opset version')
+ parser.add_argument('--workspace', type=int, default=4, help='TensorRT: workspace size (GB)')
+ parser.add_argument('--verbose', action='store_true', help='TensorRT: verbose log')
+ parser.add_argument('--weights', nargs='+', type=str, default=WEIGHTS / 'osnet_x0_25_msmt17.pt', help='model.pt path(s)')
+ parser.add_argument('--half', action='store_true', help='FP16 half-precision export')
+ parser.add_argument('--include',
+ nargs='+',
+ default=['torchscript'],
+ help='torchscript, onnx, openvino, engine')
+ args = parser.parse_args()
+
+ t = time.time()
+
+ include = [x.lower() for x in args.include] # to lowercase
+ fmts = tuple(export_formats()['Argument'][1:]) # --include arguments
+ flags = [x in include for x in fmts]
+ assert sum(flags) == len(include), f'ERROR: Invalid --include {include}, valid --include arguments are {fmts}'
+ jit, onnx, openvino, engine, tflite = flags # export booleans
+
+ args.device = select_device(args.device)
+ if args.half:
+ assert args.device.type != 'cpu', '--half only compatible with GPU export, i.e. use --device 0'
+ assert not args.dynamic, '--half not compatible with --dynamic, i.e. use either --half or --dynamic but not both'
+
+ if type(args.weights) is list:
+ args.weights = Path(args.weights[0])
+
+ model = build_model(
+ get_model_name(args.weights),
+ num_classes=1,
+ pretrained=not (args.weights and args.weights.is_file() and args.weights.suffix == '.pt'),
+ use_gpu=args.device
+ ).to(args.device)
+ load_pretrained_weights(model, args.weights)
+ model.eval()
+
+ if args.optimize:
+ assert device.type == 'cpu', '--optimize not compatible with cuda devices, i.e. use --device cpu'
+
+ im = torch.zeros(args.batch_size, 3, args.imgsz[0], args.imgsz[1]).to(args.device) # image size(1,3,640,480) BCHW iDetection
+ for _ in range(2):
+ y = model(im) # dry runs
+ if args.half:
+ im, model = im.half(), model.half() # to FP16
+ shape = tuple((y[0] if isinstance(y, tuple) else y).shape) # model output shape
+ LOGGER.info(f"\n{colorstr('PyTorch:')} starting from {args.weights} with output shape {shape} ({file_size(args.weights):.1f} MB)")
+
+ # Exports
+ f = [''] * len(fmts) # exported filenames
+ if jit:
+ f[0] = export_torchscript(model, im, args.weights, args.optimize) # opset 12
+ if engine: # TensorRT required before ONNX
+ f[1] = export_engine(model, im, args.weights, args.half, args.dynamic, args.simplify, args.workspace, args.verbose)
+ if onnx: # OpenVINO requires ONNX
+ f[2] = export_onnx(model, im, args.weights, args.opset, args.dynamic, args.simplify) # opset 12
+ if openvino:
+ f[3] = export_openvino(args.weights, args.half)
+ if tflite:
+ export_tflite(f, False)
+
+ # Finish
+ f = [str(x) for x in f if x] # filter out '' and None
+ if any(f):
+ LOGGER.info(f'\nExport complete ({time.time() - t:.1f}s)'
+ f"\nResults saved to {colorstr('bold', args.weights.parent.resolve())}"
+ f"\nVisualize: https://netron.app")
+
diff --git a/requirements.txt b/requirements.txt
new file mode 100644
index 0000000000000000000000000000000000000000..ef2ab5f0e2a8557308f5c912708e76cabc6673cc
Binary files /dev/null and b/requirements.txt differ
diff --git a/test.py b/test.py
new file mode 100644
index 0000000000000000000000000000000000000000..69b8866b85fda89b7793f47dd7de08328e45f08f
--- /dev/null
+++ b/test.py
@@ -0,0 +1,17 @@
+import track
+import os
+from pathlib import Path
+
+FILE = Path(__file__).resolve()
+ROOT = FILE.parents[0] # yolov5 strongsort root directory
+WEIGHTS = ROOT / 'weights'
+trackers = "strongsort" # ["strongsort", "bytetrack"]
+path = "./video"
+v = [x for x in os.listdir(path) if x.endswith(".mp4")]
+
+for i in v:
+ # , classes=[0, 1, 2, 3, 5, 7, 9, 11, 10]
+ track.run(source=ROOT / f"video/{i}", tracking_method=trackers, yolo_weights=WEIGHTS / "best2.pt", project=ROOT / f"runs/{trackers}3", name=f"video{i.split('.')[0]}", iou_thres=0.45, conf_thres=0.35, hide_conf=True)
+ print(f"{i}检测完毕")
+
+
diff --git a/test_image/FLIR.yaml b/test_image/FLIR.yaml
new file mode 100644
index 0000000000000000000000000000000000000000..acd784f39519cc08f288d4a3bf9246c80261e860
--- /dev/null
+++ b/test_image/FLIR.yaml
@@ -0,0 +1,29 @@
+train: VOCdevkit\images\train # 8493 images 训练集
+val: VOCdevkit\images\val # 2249 images 验证集
+
+# number of classes 类别数
+nc: 15
+
+
+# Classes
+names:
+ 0: person
+ 1: bike
+ 2: car
+ 3: motor
+ 4: bus
+ 5: truck #6-5
+ 6: light #7-6
+ 7: hydrant #8-7
+ 8: sign # 9-8
+
+
+# 5: train
+# 10: dog
+# 11: skateboard
+# 12: stroller
+# 13: scooter
+# 14: other vehicle
+
+
+
diff --git a/test_image/video-2SReBn5LtAkL5HMj2-frame-000317-HTgPBFgZyPdwQnNvE.jpg b/test_image/video-2SReBn5LtAkL5HMj2-frame-000317-HTgPBFgZyPdwQnNvE.jpg
new file mode 100644
index 0000000000000000000000000000000000000000..d74915ffb61446045d4ac42759830eee44d0b24a
Binary files /dev/null and b/test_image/video-2SReBn5LtAkL5HMj2-frame-000317-HTgPBFgZyPdwQnNvE.jpg differ
diff --git a/test_image/video-2SReBn5LtAkL5HMj2-frame-005072-MA7NCLQGoqq9aHaiL.jpg b/test_image/video-2SReBn5LtAkL5HMj2-frame-005072-MA7NCLQGoqq9aHaiL.jpg
new file mode 100644
index 0000000000000000000000000000000000000000..5af73a413d15670ca59fa7f930743e7f648e6b7e
Binary files /dev/null and b/test_image/video-2SReBn5LtAkL5HMj2-frame-005072-MA7NCLQGoqq9aHaiL.jpg differ
diff --git a/test_image/video-2rsjnZFyGQGeynfbv-frame-003708-6fPQbB7jtibwaYAE7.jpg b/test_image/video-2rsjnZFyGQGeynfbv-frame-003708-6fPQbB7jtibwaYAE7.jpg
new file mode 100644
index 0000000000000000000000000000000000000000..bd78fb9250d9de6428fadf0dcacae86b98aef249
Binary files /dev/null and b/test_image/video-2rsjnZFyGQGeynfbv-frame-003708-6fPQbB7jtibwaYAE7.jpg differ
diff --git a/test_image/video-jNQtRj6NGycZDEXpe-frame-002515-J3YntG8ntvZheKK3P.jpg b/test_image/video-jNQtRj6NGycZDEXpe-frame-002515-J3YntG8ntvZheKK3P.jpg
new file mode 100644
index 0000000000000000000000000000000000000000..58ff1b4d863308255691b838b4dca62625bbcc06
Binary files /dev/null and b/test_image/video-jNQtRj6NGycZDEXpe-frame-002515-J3YntG8ntvZheKK3P.jpg differ
diff --git a/test_image/video-kDDWXrnLSoSdHCZ7S-frame-003063-eaKjPvPskDPjenZ8S.jpg b/test_image/video-kDDWXrnLSoSdHCZ7S-frame-003063-eaKjPvPskDPjenZ8S.jpg
new file mode 100644
index 0000000000000000000000000000000000000000..e0e5a5ba3e6428f488292501489aea2f061a59bf
Binary files /dev/null and b/test_image/video-kDDWXrnLSoSdHCZ7S-frame-003063-eaKjPvPskDPjenZ8S.jpg differ
diff --git a/test_image/video-r68Yr9RPWEp5fW2ZF-frame-000333-X6K5iopqbmjKEsSqN.jpg b/test_image/video-r68Yr9RPWEp5fW2ZF-frame-000333-X6K5iopqbmjKEsSqN.jpg
new file mode 100644
index 0000000000000000000000000000000000000000..e256cc689d7ebb392d5e5b9ea5105b8be0d931d6
Binary files /dev/null and b/test_image/video-r68Yr9RPWEp5fW2ZF-frame-000333-X6K5iopqbmjKEsSqN.jpg differ
diff --git a/track.py b/track.py
new file mode 100644
index 0000000000000000000000000000000000000000..329fdc63e90ca41fc29834f24cf1b0e78eaf8104
--- /dev/null
+++ b/track.py
@@ -0,0 +1,308 @@
+import argparse
+
+import os
+# limit the number of cpus used by high performance libraries
+os.environ["OMP_NUM_THREADS"] = "1"
+os.environ["OPENBLAS_NUM_THREADS"] = "1"
+os.environ["MKL_NUM_THREADS"] = "1"
+os.environ["VECLIB_MAXIMUM_THREADS"] = "1"
+os.environ["NUMEXPR_NUM_THREADS"] = "1"
+
+import sys
+import numpy as np
+from pathlib import Path
+import torch
+import torch.backends.cudnn as cudnn
+
+FILE = Path(__file__).resolve()
+ROOT = FILE.parents[0] # yolov5 strongsort root directory
+WEIGHTS = ROOT / 'weights'
+
+if str(ROOT) not in sys.path:
+ sys.path.append(str(ROOT)) # add ROOT to PATH
+if str(ROOT / 'yolov5') not in sys.path:
+ sys.path.append(str(ROOT / 'yolov5')) # add yolov5 ROOT to PATH
+if str(ROOT / 'trackers' / 'strong_sort') not in sys.path:
+ sys.path.append(str(ROOT / 'trackers' / 'strong_sort')) # add strong_sort ROOT to PATH
+
+ROOT = Path(os.path.relpath(ROOT, Path.cwd())) # relative
+
+import logging
+from yolov5.models.common import DetectMultiBackend
+from yolov5.utils.dataloaders import VID_FORMATS, LoadImages, LoadStreams
+from yolov5.utils.general import (LOGGER, check_img_size, non_max_suppression, scale_boxes, check_requirements, cv2,
+ check_imshow, xyxy2xywh, increment_path, strip_optimizer, colorstr, print_args, check_file)
+from yolov5.utils.torch_utils import select_device, time_sync
+from yolov5.utils.plots import Annotator, colors, save_one_box
+from trackers.multi_tracker_zoo import create_tracker
+
+# remove duplicated stream handler to avoid duplicated logging
+#logging.getLogger().removeHandler(logging.getLogger().handlers[0])
+
+@torch.no_grad()
+def run(
+ source='0',
+ yolo_weights=WEIGHTS / 'yolov5m.pt', # model.pt path(s),
+ reid_weights=WEIGHTS / 'osnet_x0_25_msmt17.pt', # model.pt path,
+ tracking_method='strongsort',
+ imgsz=(640, 640), # inference size (height, width)
+ conf_thres=0.25, # confidence threshold
+ iou_thres=0.45, # NMS IOU threshold
+ max_det=1000, # maximum detections per image
+ device='', # cuda device, i.e. 0 or 0,1,2,3 or cpu
+ show_vid=False, # show results
+ save_txt=False, # save results to *.txt
+ save_conf=False, # save confidences in --save-txt labels
+ save_crop=False, # save cropped prediction boxes
+ save_vid=True, # save confidences in --save-txt labels
+ nosave=False, # do not save images/videos
+ classes=None, # filter by class: --class 0, or --class 0 2 3
+ agnostic_nms=False, # class-agnostic NMS
+ augment=False, # augmented inference
+ visualize=False, # visualize features
+ update=False, # update all models
+ project=ROOT / 'runs/track', # save results to project/name
+ name='exp', # save results to project/name
+ exist_ok=False, # existing project/name ok, do not increment
+ line_thickness=1, # bounding box thickness (pixels)
+ hide_labels=False, # hide labels
+ hide_conf=False, # hide confidences
+ hide_class=False, # hide IDs
+ half=False, # use FP16 half-precision inference
+ dnn=False, # use OpenCV DNN for ONNX inference
+ vid_stride=1, # video frame-rate stride
+):
+ save_txt = True
+ source = str(source)
+ save_img = not nosave and not source.endswith('.txt') # save inference images
+ is_file = Path(source).suffix[1:] in (VID_FORMATS)
+ is_url = source.lower().startswith(('rtsp://', 'rtmp://', 'http://', 'https://'))
+ webcam = source.isnumeric() or source.endswith('.txt') or (is_url and not is_file)
+ if is_url and is_file:
+ source = check_file(source) # download
+
+ # Directories
+ if not isinstance(yolo_weights, list): # single yolo model
+ exp_name = yolo_weights.stem
+ elif type(yolo_weights) is list and len(yolo_weights) == 1: # single models after --yolo_weights
+ exp_name = Path(yolo_weights[0]).stem
+ else: # multiple models after --yolo_weights
+ exp_name = 'ensemble'
+ exp_name = name if name else exp_name + "_" + reid_weights.stem
+ save_dir = increment_path(Path(project) / exp_name, exist_ok=exist_ok) # increment run
+ (save_dir / 'tracks' if save_txt else save_dir).mkdir(parents=True, exist_ok=True) # make dir
+
+ # Load model
+ device = select_device(device)
+ model = DetectMultiBackend(yolo_weights, device=device, dnn=dnn, data=None, fp16=half)
+ stride, names, pt = model.stride, model.names, model.pt
+ imgsz = check_img_size(imgsz, s=stride) # check image size
+
+ # Dataloader
+ if webcam:
+ show_vid = check_imshow()
+ dataset = LoadStreams(source, img_size=imgsz, stride=stride, auto=pt, vid_stride=vid_stride)
+ nr_sources = len(dataset)
+ else:
+ dataset = LoadImages(source, img_size=imgsz, stride=stride, auto=pt)
+ nr_sources = 1
+ vid_path, vid_writer, txt_path = [None] * nr_sources, [None] * nr_sources, [None] * nr_sources
+
+ # Create as many strong sort instances as there are video sources
+ tracker_list = []
+ for i in range(nr_sources):
+ tracker = create_tracker(tracking_method, reid_weights, device, half)
+ tracker_list.append(tracker, )
+ if hasattr(tracker_list[i], 'model'):
+ if hasattr(tracker_list[i].model, 'warmup'):
+ tracker_list[i].model.warmup()
+ outputs = [None] * nr_sources
+
+ # Run tracking
+ #model.warmup(imgsz=(1 if pt else nr_sources, 3, *imgsz)) # warmup
+ dt, seen = [0.0, 0.0, 0.0, 0.0], 0
+ curr_frames, prev_frames = [None] * nr_sources, [None] * nr_sources
+ for frame_idx, (path, im, im0s, vid_cap, s) in enumerate(dataset):
+ t1 = time_sync()
+ im = torch.from_numpy(im).to(device)
+ im = im.half() if half else im.float() # uint8 to fp16/32
+ im /= 255.0 # 0 - 255 to 0.0 - 1.0
+ if len(im.shape) == 3:
+ im = im[None] # expand for batch dim
+ t2 = time_sync()
+ dt[0] += t2 - t1
+
+ # Inference
+ visualize = increment_path(save_dir / Path(path[0]).stem, mkdir=True) if visualize else False
+ pred = model(im, augment=augment, visualize=visualize)
+ t3 = time_sync()
+ dt[1] += t3 - t2
+
+ # Apply NMS
+ pred = non_max_suppression(pred, conf_thres, iou_thres, classes, agnostic_nms, max_det=max_det)
+ dt[2] += time_sync() - t3
+
+ # Process detections
+ for i, det in enumerate(pred): # detections per image
+ seen += 1
+ if webcam: # nr_sources >= 1
+ p, im0, _ = path[i], im0s[i].copy(), dataset.count
+ p = Path(p) # to Path
+ s += f'{i}: '
+ txt_file_name = p.name
+ save_path = str(save_dir / p.name) # im.jpg, vid.mp4, ...
+ else:
+ p, im0, _ = path, im0s.copy(), getattr(dataset, 'frame', 0)
+ p = Path(p) # to Path
+ # video file
+ if source.endswith(VID_FORMATS):
+ txt_file_name = p.stem
+ save_path = str(save_dir / p.name) # im.jpg, vid.mp4, ...
+ # folder with imgs
+ else:
+ txt_file_name = p.parent.name # get folder name containing current img
+ save_path = str(save_dir / p.parent.name) # im.jpg, vid.mp4, ...
+ curr_frames[i] = im0
+
+ txt_path = str(save_dir / 'tracks' / txt_file_name) # im.txt
+ s += '%gx%g ' % im.shape[2:] # print string
+ imc = im0.copy() if save_crop else im0 # for save_crop
+
+ annotator = Annotator(im0, line_width=line_thickness, example=str(names))
+
+ if hasattr(tracker_list[i], 'tracker') and hasattr(tracker_list[i].tracker, 'camera_update'):
+ if prev_frames[i] is not None and curr_frames[i] is not None: # camera motion compensation
+ tracker_list[i].tracker.camera_update(prev_frames[i], curr_frames[i])
+
+ if det is not None and len(det):
+ # Rescale boxes from img_size to im0 size
+ det[:, :4] = scale_boxes(im.shape[2:], det[:, :4], im0.shape).round() # xyxy
+
+ # Print results
+ for c in det[:, -1].unique():
+ n = (det[:, -1] == c).sum() # detections per class
+ s += f"{n} {names[int(c)]}{'s' * (n > 1)}, " # add to string
+
+ # pass detections to strongsort
+ t4 = time_sync()
+ outputs[i] = tracker_list[i].update(det.cpu(), im0)
+ t5 = time_sync()
+ dt[3] += t5 - t4
+
+ # draw boxes for visualization
+ if len(outputs[i]) > 0:
+ for j, (output, conf) in enumerate(zip(outputs[i], det[:, 4])):
+
+ bboxes = output[0:4]
+ id = output[4]
+ cls = output[5]
+
+ if save_txt:
+ # to MOT format
+ bbox_left = output[0]
+ bbox_top = output[1]
+ bbox_w = output[2] - output[0]
+ bbox_h = output[3] - output[1]
+ # Write MOT compliant results to file
+ with open(txt_path + '.txt', 'a') as f:
+ f.write(('%g ' * 10 + '\n') % (frame_idx + 1, id, bbox_left, # MOT format
+ bbox_top, bbox_w, bbox_h, -1, -1, -1, i))
+ save_vid=True
+
+ if save_vid or save_crop or show_vid: # Add bbox to image
+ c = int(cls) # integer class
+ id = int(id) # integer id
+ label = None if hide_labels else (f'{id} {names[c]}' if hide_conf else \
+ (f'{id} {conf:.2f}' if hide_class else f'{id} {names[c]} {conf:.2f}'))
+ annotator.box_label(bboxes, label, color=colors(c, True))
+ if save_crop:
+ txt_file_name = txt_file_name if (isinstance(path, list) and len(path) > 1) else ''
+ save_one_box(bboxes, imc, file=save_dir / 'crops' / txt_file_name / names[c] / f'{id}' / f'{p.stem}.jpg', BGR=True)
+
+ LOGGER.info(f'{s}Done. yolo:({t3 - t2:.3f}s), {tracking_method}:({t5 - t4:.3f}s)')
+
+ else:
+ #strongsort_list[i].increment_ages()
+ LOGGER.info('No detections')
+
+ # Stream results
+ im0 = annotator.result()
+ if show_vid:
+ cv2.imshow(str(p), im0)
+ cv2.waitKey(1) # 1 millisecond
+
+ # Save results (image with detections)
+ if save_vid:
+ if vid_path[i] != save_path: # new video
+ vid_path[i] = save_path
+ if isinstance(vid_writer[i], cv2.VideoWriter):
+ vid_writer[i].release() # release previous video writer
+ if vid_cap: # video
+ fps = vid_cap.get(cv2.CAP_PROP_FPS)
+ w = int(vid_cap.get(cv2.CAP_PROP_FRAME_WIDTH))
+ h = int(vid_cap.get(cv2.CAP_PROP_FRAME_HEIGHT))
+ else: # stream
+ fps, w, h = 30, im0.shape[1], im0.shape[0]
+ save_path = str(Path(save_path).with_suffix('.mp4')) # force *.mp4 suffix on results videos
+ vid_writer[i] = cv2.VideoWriter(save_path, cv2.VideoWriter_fourcc(*'mp4v'), fps, (w, h))
+ vid_writer[i].write(im0)
+
+ prev_frames[i] = curr_frames[i]
+
+ # Print results
+ t = tuple(x / seen * 1E3 for x in dt) # speeds per image
+ LOGGER.info(f'Speed: %.1fms pre-process, %.1fms inference, %.1fms NMS, %.1fms {tracking_method} update per image at shape {(1, 3, *imgsz)}' % t)
+ if save_txt or save_vid:
+ s = f"\n{len(list(save_dir.glob('tracks/*.txt')))} tracks saved to {save_dir / 'tracks'}" if save_txt else ''
+ LOGGER.info(f"Results saved to {colorstr('bold', save_dir)}{s}")
+ if update:
+ strip_optimizer(yolo_weights) # update model (to fix SourceChangeWarning)
+
+
+def parse_opt():
+ parser = argparse.ArgumentParser()
+ parser.add_argument('--yolo-weights', nargs='+', type=Path, default=WEIGHTS / 'best2.pt', help='model.pt path(s)')
+ parser.add_argument('--reid-weights', type=Path, default=WEIGHTS / 'osnet_x0_25_msmt17.pt')
+ parser.add_argument('--tracking-method', type=str, default='bytetrack', help='strongsort, ocsort, bytetrack')
+ parser.add_argument('--source', type=str, default=r'video', help='file/dir/URL/glob, 0 for webcam')
+ parser.add_argument('--imgsz', '--img', '--img-size', nargs='+', type=int, default=[640], help='inference size h,w')
+ parser.add_argument('--conf-thres', type=float, default=0.5, help='confidence threshold')
+ parser.add_argument('--iou-thres', type=float, default=0.5, help='NMS IoU threshold') #0.5
+ parser.add_argument('--max-det', type=int, default=1000, help='maximum detections per image')
+ parser.add_argument('--device', default='', help='cuda device, i.e. 0 or 0,1,2,3 or cpu')
+ parser.add_argument('--show-vid', action='store_true', help='display tracking video results')
+ parser.add_argument('--save-txt', action='store_true', help='save results to *.txt')
+ parser.add_argument('--save-conf', action='store_true', help='save confidences in --save-txt labels')
+ parser.add_argument('--save-crop', action='store_true', help='save cropped prediction boxes')
+ parser.add_argument('--save-vid', action='store_true', help='save video tracking results')
+ parser.add_argument('--nosave', action='store_false', help='do not save images/videos')
+ # class 0 is person, 1 is bycicle, 2 is car... 79 is oven 0 1 2 3 5 7 9 11 10
+ parser.add_argument('--classes', nargs='+', type=int, help='filter by class: --classes 0, or --classes 0 2 3')
+ parser.add_argument('--agnostic-nms', action='store_true', help='class-agnostic NMS')
+ parser.add_argument('--augment', action='store_true', help='augmented inference')
+ parser.add_argument('--visualize', action='store_true', help='visualize features')
+ parser.add_argument('--update', action='store_true', help='update all models')
+ parser.add_argument('--project', default=ROOT / 'runs/track', help='save results to project/name')
+ parser.add_argument('--name', default='exp', help='save results to project/name')
+ parser.add_argument('--exist-ok', action='store_true', help='existing project/name ok, do not increment')
+ parser.add_argument('--line-thickness', default=1, type=int, help='bounding box thickness (pixels)')
+ parser.add_argument('--hide-labels', default=False, action='store_true', help='hide labels')
+ parser.add_argument('--hide-conf', default=True, action='store_true', help='hide confidences')
+ parser.add_argument('--hide-class', default=False, action='store_true', help='hide IDs')
+ parser.add_argument('--half', action='store_true', help='use FP16 half-precision inference')
+ parser.add_argument('--dnn', action='store_true', help='use OpenCV DNN for ONNX inference')
+ parser.add_argument('--vid-stride', type=int, default=1, help='video frame-rate stride')
+ opt = parser.parse_args()
+ opt.imgsz *= 2 if len(opt.imgsz) == 1 else 1 # expand
+ print_args(vars(opt))
+ return opt
+
+
+def main(opt):
+ check_requirements(requirements=ROOT / 'requirements.txt', exclude=('tensorboard', 'thop'))
+ run(**vars(opt))
+
+
+if __name__ == "__main__":
+ opt = parse_opt()
+ main(opt)
diff --git a/trackers/__init__.py b/trackers/__init__.py
new file mode 100644
index 0000000000000000000000000000000000000000..e69de29bb2d1d6434b8b29ae775ad8c2e48c5391
diff --git a/trackers/__pycache__/__init__.cpython-38.pyc b/trackers/__pycache__/__init__.cpython-38.pyc
new file mode 100644
index 0000000000000000000000000000000000000000..d12a52d2a939ba45f2400e42e25c8fff2961b0bf
Binary files /dev/null and b/trackers/__pycache__/__init__.cpython-38.pyc differ
diff --git a/trackers/__pycache__/__init__.cpython-39.pyc b/trackers/__pycache__/__init__.cpython-39.pyc
new file mode 100644
index 0000000000000000000000000000000000000000..39e1286332fadc1fcb5b49addbf9019e74a98ab7
Binary files /dev/null and b/trackers/__pycache__/__init__.cpython-39.pyc differ
diff --git a/trackers/__pycache__/multi_tracker_zoo.cpython-38.pyc b/trackers/__pycache__/multi_tracker_zoo.cpython-38.pyc
new file mode 100644
index 0000000000000000000000000000000000000000..e0788a67f7c8471e4a773956b84e2044fc5e6f26
Binary files /dev/null and b/trackers/__pycache__/multi_tracker_zoo.cpython-38.pyc differ
diff --git a/trackers/__pycache__/multi_tracker_zoo.cpython-39.pyc b/trackers/__pycache__/multi_tracker_zoo.cpython-39.pyc
new file mode 100644
index 0000000000000000000000000000000000000000..043e687a16da7a8a9517435c091b2e9e1279f268
Binary files /dev/null and b/trackers/__pycache__/multi_tracker_zoo.cpython-39.pyc differ
diff --git a/trackers/bytetrack/__pycache__/basetrack.cpython-38.pyc b/trackers/bytetrack/__pycache__/basetrack.cpython-38.pyc
new file mode 100644
index 0000000000000000000000000000000000000000..aac5546ac51893c22ab46d519a2041783ff381fe
Binary files /dev/null and b/trackers/bytetrack/__pycache__/basetrack.cpython-38.pyc differ
diff --git a/trackers/bytetrack/__pycache__/basetrack.cpython-39.pyc b/trackers/bytetrack/__pycache__/basetrack.cpython-39.pyc
new file mode 100644
index 0000000000000000000000000000000000000000..2beb363aafcb96fd5845e0654f1af1e8acfeb48b
Binary files /dev/null and b/trackers/bytetrack/__pycache__/basetrack.cpython-39.pyc differ
diff --git a/trackers/bytetrack/__pycache__/byte_tracker.cpython-38.pyc b/trackers/bytetrack/__pycache__/byte_tracker.cpython-38.pyc
new file mode 100644
index 0000000000000000000000000000000000000000..5719cc59217f7af3ca15bd8e6cb3b1cfcee7d5c0
Binary files /dev/null and b/trackers/bytetrack/__pycache__/byte_tracker.cpython-38.pyc differ
diff --git a/trackers/bytetrack/__pycache__/byte_tracker.cpython-39.pyc b/trackers/bytetrack/__pycache__/byte_tracker.cpython-39.pyc
new file mode 100644
index 0000000000000000000000000000000000000000..4bcdfdd7c7f67e90fc504cfd80a35210dda0611b
Binary files /dev/null and b/trackers/bytetrack/__pycache__/byte_tracker.cpython-39.pyc differ
diff --git a/trackers/bytetrack/__pycache__/kalman_filter.cpython-38.pyc b/trackers/bytetrack/__pycache__/kalman_filter.cpython-38.pyc
new file mode 100644
index 0000000000000000000000000000000000000000..6af0dbca5f7d61a5aa899d1cd7641cb7eb4b35c0
Binary files /dev/null and b/trackers/bytetrack/__pycache__/kalman_filter.cpython-38.pyc differ
diff --git a/trackers/bytetrack/__pycache__/kalman_filter.cpython-39.pyc b/trackers/bytetrack/__pycache__/kalman_filter.cpython-39.pyc
new file mode 100644
index 0000000000000000000000000000000000000000..b0fd5496002c28fdd6d4a78e09249f5b7309a2f6
Binary files /dev/null and b/trackers/bytetrack/__pycache__/kalman_filter.cpython-39.pyc differ
diff --git a/trackers/bytetrack/__pycache__/matching.cpython-38.pyc b/trackers/bytetrack/__pycache__/matching.cpython-38.pyc
new file mode 100644
index 0000000000000000000000000000000000000000..ba5d65d71d4661ae136825e7f91953709b8bea17
Binary files /dev/null and b/trackers/bytetrack/__pycache__/matching.cpython-38.pyc differ
diff --git a/trackers/bytetrack/__pycache__/matching.cpython-39.pyc b/trackers/bytetrack/__pycache__/matching.cpython-39.pyc
new file mode 100644
index 0000000000000000000000000000000000000000..223c3968f5c2fa5574dc9d1bdb0790764f3e34fa
Binary files /dev/null and b/trackers/bytetrack/__pycache__/matching.cpython-39.pyc differ
diff --git a/trackers/bytetrack/basetrack.py b/trackers/bytetrack/basetrack.py
new file mode 100644
index 0000000000000000000000000000000000000000..4fe2233607f6d4ed28b11a0ae6c0303c8ca19098
--- /dev/null
+++ b/trackers/bytetrack/basetrack.py
@@ -0,0 +1,52 @@
+import numpy as np
+from collections import OrderedDict
+
+
+class TrackState(object):
+ New = 0
+ Tracked = 1
+ Lost = 2
+ Removed = 3
+
+
+class BaseTrack(object):
+ _count = 0
+
+ track_id = 0
+ is_activated = False
+ state = TrackState.New
+
+ history = OrderedDict()
+ features = []
+ curr_feature = None
+ score = 0
+ start_frame = 0
+ frame_id = 0
+ time_since_update = 0
+
+ # multi-camera
+ location = (np.inf, np.inf)
+
+ @property
+ def end_frame(self):
+ return self.frame_id
+
+ @staticmethod
+ def next_id():
+ BaseTrack._count += 1
+ return BaseTrack._count
+
+ def activate(self, *args):
+ raise NotImplementedError
+
+ def predict(self):
+ raise NotImplementedError
+
+ def update(self, *args, **kwargs):
+ raise NotImplementedError
+
+ def mark_lost(self):
+ self.state = TrackState.Lost
+
+ def mark_removed(self):
+ self.state = TrackState.Removed
diff --git a/trackers/bytetrack/byte_tracker.py b/trackers/bytetrack/byte_tracker.py
new file mode 100644
index 0000000000000000000000000000000000000000..62cd67f4c202e368d58fdc80a8cc926ee24c7dbb
--- /dev/null
+++ b/trackers/bytetrack/byte_tracker.py
@@ -0,0 +1,353 @@
+import numpy as np
+from collections import deque
+import os
+import os.path as osp
+import copy
+import torch
+import torch.nn.functional as F
+
+from yolov5.utils.general import xywh2xyxy, xyxy2xywh
+
+
+from trackers.bytetrack.kalman_filter import KalmanFilter
+from trackers.bytetrack import matching
+from trackers.bytetrack.basetrack import BaseTrack, TrackState
+
+class STrack(BaseTrack):
+ shared_kalman = KalmanFilter()
+ def __init__(self, tlwh, score, cls):
+
+ # wait activate
+ self._tlwh = np.asarray(tlwh, dtype=np.float)
+ self.kalman_filter = None
+ self.mean, self.covariance = None, None
+ self.is_activated = False
+
+ self.score = score
+ self.tracklet_len = 0
+ self.cls = cls
+
+ def predict(self):
+ mean_state = self.mean.copy()
+ if self.state != TrackState.Tracked:
+ mean_state[7] = 0
+ self.mean, self.covariance = self.kalman_filter.predict(mean_state, self.covariance)
+
+ @staticmethod
+ def multi_predict(stracks):
+ if len(stracks) > 0:
+ multi_mean = np.asarray([st.mean.copy() for st in stracks])
+ multi_covariance = np.asarray([st.covariance for st in stracks])
+ for i, st in enumerate(stracks):
+ if st.state != TrackState.Tracked:
+ multi_mean[i][7] = 0
+ multi_mean, multi_covariance = STrack.shared_kalman.multi_predict(multi_mean, multi_covariance)
+ for i, (mean, cov) in enumerate(zip(multi_mean, multi_covariance)):
+ stracks[i].mean = mean
+ stracks[i].covariance = cov
+
+ def activate(self, kalman_filter, frame_id):
+ """Start a new tracklet"""
+ self.kalman_filter = kalman_filter
+ self.track_id = self.next_id()
+ self.mean, self.covariance = self.kalman_filter.initiate(self.tlwh_to_xyah(self._tlwh))
+
+ self.tracklet_len = 0
+ self.state = TrackState.Tracked
+ if frame_id == 1:
+ self.is_activated = True
+ # self.is_activated = True
+ self.frame_id = frame_id
+ self.start_frame = frame_id
+
+ def re_activate(self, new_track, frame_id, new_id=False):
+ self.mean, self.covariance = self.kalman_filter.update(
+ self.mean, self.covariance, self.tlwh_to_xyah(new_track.tlwh)
+ )
+ self.tracklet_len = 0
+ self.state = TrackState.Tracked
+ self.is_activated = True
+ self.frame_id = frame_id
+ if new_id:
+ self.track_id = self.next_id()
+ self.score = new_track.score
+ self.cls = new_track.cls
+
+ def update(self, new_track, frame_id):
+ """
+ Update a matched track
+ :type new_track: STrack
+ :type frame_id: int
+ :type update_feature: bool
+ :return:
+ """
+ self.frame_id = frame_id
+ self.tracklet_len += 1
+ # self.cls = cls
+
+ new_tlwh = new_track.tlwh
+ self.mean, self.covariance = self.kalman_filter.update(
+ self.mean, self.covariance, self.tlwh_to_xyah(new_tlwh))
+ self.state = TrackState.Tracked
+ self.is_activated = True
+
+ self.score = new_track.score
+
+ @property
+ # @jit(nopython=True)
+ def tlwh(self):
+ """Get current position in bounding box format `(top left x, top left y,
+ width, height)`.
+ """
+ if self.mean is None:
+ return self._tlwh.copy()
+ ret = self.mean[:4].copy()
+ ret[2] *= ret[3]
+ ret[:2] -= ret[2:] / 2
+ return ret
+
+ @property
+ # @jit(nopython=True)
+ def tlbr(self):
+ """Convert bounding box to format `(min x, min y, max x, max y)`, i.e.,
+ `(top left, bottom right)`.
+ """
+ ret = self.tlwh.copy()
+ ret[2:] += ret[:2]
+ return ret
+
+ @staticmethod
+ # @jit(nopython=True)
+ def tlwh_to_xyah(tlwh):
+ """Convert bounding box to format `(center x, center y, aspect ratio,
+ height)`, where the aspect ratio is `width / height`.
+ """
+ ret = np.asarray(tlwh).copy()
+ ret[:2] += ret[2:] / 2
+ ret[2] /= ret[3]
+ return ret
+
+ def to_xyah(self):
+ return self.tlwh_to_xyah(self.tlwh)
+
+ @staticmethod
+ # @jit(nopython=True)
+ def tlbr_to_tlwh(tlbr):
+ ret = np.asarray(tlbr).copy()
+ ret[2:] -= ret[:2]
+ return ret
+
+ @staticmethod
+ # @jit(nopython=True)
+ def tlwh_to_tlbr(tlwh):
+ ret = np.asarray(tlwh).copy()
+ ret[2:] += ret[:2]
+ return ret
+
+ def __repr__(self):
+ return 'OT_{}_({}-{})'.format(self.track_id, self.start_frame, self.end_frame)
+
+
+class BYTETracker(object):
+ def __init__(self, track_thresh=0.45, track_buffer=25, match_thresh=0.8, frame_rate=30):
+ self.tracked_stracks = [] # type: list[STrack]
+ self.lost_stracks = [] # type: list[STrack]
+ self.removed_stracks = [] # type: list[STrack]
+
+ self.frame_id = 0
+ self.track_buffer=track_buffer
+
+ self.track_thresh = track_thresh
+ self.match_thresh = match_thresh
+ # self.det_thresh = track_thresh
+ self.det_thresh = track_thresh + 0.1
+ self.buffer_size = int(frame_rate / 30.0 * track_buffer)
+ self.max_time_lost = self.buffer_size
+ self.kalman_filter = KalmanFilter()
+
+ def update(self, dets, _):
+ self.frame_id += 1
+ activated_starcks = []
+ refind_stracks = []
+ lost_stracks = []
+ removed_stracks = []
+
+ xyxys = dets[:, 0:4]
+ xywh = xyxy2xywh(xyxys)
+ confs = dets[:, 4]
+ clss = dets[:, 5]
+
+ classes = clss.numpy()
+ xyxys = xyxys.numpy()
+ confs = confs.numpy()
+
+ remain_inds = confs > self.track_thresh
+ inds_low = confs > 0.1
+ inds_high = confs < self.track_thresh
+
+ inds_second = np.logical_and(inds_low, inds_high)
+
+ dets_second = xywh[inds_second]
+ dets = xywh[remain_inds]
+
+ scores_keep = confs[remain_inds]
+ scores_second = confs[inds_second]
+
+ clss_keep = classes[remain_inds]
+ clss_second = classes[remain_inds]
+
+
+ if len(dets) > 0:
+ '''Detections'''
+ detections = [STrack(xyxy, s, c) for (xyxy, s, c) in zip(dets, scores_keep, clss_keep)]
+ else:
+ detections = []
+
+ ''' Add newly detected tracklets to tracked_stracks'''
+ unconfirmed = []
+ tracked_stracks = [] # type: list[STrack]
+ for track in self.tracked_stracks:
+ if not track.is_activated:
+ unconfirmed.append(track)
+ else:
+ tracked_stracks.append(track)
+
+ ''' Step 2: First association, with high score detection boxes'''
+ strack_pool = joint_stracks(tracked_stracks, self.lost_stracks)
+ # Predict the current location with KF
+ STrack.multi_predict(strack_pool)
+ dists = matching.iou_distance(strack_pool, detections)
+ #if not self.args.mot20:
+ dists = matching.fuse_score(dists, detections)
+ matches, u_track, u_detection = matching.linear_assignment(dists, thresh=self.match_thresh)
+
+ for itracked, idet in matches:
+ track = strack_pool[itracked]
+ det = detections[idet]
+ if track.state == TrackState.Tracked:
+ track.update(detections[idet], self.frame_id)
+ activated_starcks.append(track)
+ else:
+ track.re_activate(det, self.frame_id, new_id=False)
+ refind_stracks.append(track)
+
+ ''' Step 3: Second association, with low score detection boxes'''
+ # association the untrack to the low score detections
+ if len(dets_second) > 0:
+ '''Detections'''
+ detections_second = [STrack(xywh, s, c) for (xywh, s, c) in zip(dets_second, scores_second, clss_second)]
+ else:
+ detections_second = []
+ r_tracked_stracks = [strack_pool[i] for i in u_track if strack_pool[i].state == TrackState.Tracked]
+ dists = matching.iou_distance(r_tracked_stracks, detections_second)
+ matches, u_track, u_detection_second = matching.linear_assignment(dists, thresh=0.5)
+ for itracked, idet in matches:
+ track = r_tracked_stracks[itracked]
+ det = detections_second[idet]
+ if track.state == TrackState.Tracked:
+ track.update(det, self.frame_id)
+ activated_starcks.append(track)
+ else:
+ track.re_activate(det, self.frame_id, new_id=False)
+ refind_stracks.append(track)
+
+ for it in u_track:
+ track = r_tracked_stracks[it]
+ if not track.state == TrackState.Lost:
+ track.mark_lost()
+ lost_stracks.append(track)
+
+ '''Deal with unconfirmed tracks, usually tracks with only one beginning frame'''
+ detections = [detections[i] for i in u_detection]
+ dists = matching.iou_distance(unconfirmed, detections)
+ #if not self.args.mot20:
+ dists = matching.fuse_score(dists, detections)
+ matches, u_unconfirmed, u_detection = matching.linear_assignment(dists, thresh=0.7)
+ for itracked, idet in matches:
+ unconfirmed[itracked].update(detections[idet], self.frame_id)
+ activated_starcks.append(unconfirmed[itracked])
+ for it in u_unconfirmed:
+ track = unconfirmed[it]
+ track.mark_removed()
+ removed_stracks.append(track)
+
+ """ Step 4: Init new stracks"""
+ for inew in u_detection:
+ track = detections[inew]
+ if track.score < self.det_thresh:
+ continue
+ track.activate(self.kalman_filter, self.frame_id)
+ activated_starcks.append(track)
+ """ Step 5: Update state"""
+ for track in self.lost_stracks:
+ if self.frame_id - track.end_frame > self.max_time_lost:
+ track.mark_removed()
+ removed_stracks.append(track)
+
+ # print('Ramained match {} s'.format(t4-t3))
+
+ self.tracked_stracks = [t for t in self.tracked_stracks if t.state == TrackState.Tracked]
+ self.tracked_stracks = joint_stracks(self.tracked_stracks, activated_starcks)
+ self.tracked_stracks = joint_stracks(self.tracked_stracks, refind_stracks)
+ self.lost_stracks = sub_stracks(self.lost_stracks, self.tracked_stracks)
+ self.lost_stracks.extend(lost_stracks)
+ self.lost_stracks = sub_stracks(self.lost_stracks, self.removed_stracks)
+ self.removed_stracks.extend(removed_stracks)
+ self.tracked_stracks, self.lost_stracks = remove_duplicate_stracks(self.tracked_stracks, self.lost_stracks)
+ # get scores of lost tracks
+ output_stracks = [track for track in self.tracked_stracks if track.is_activated]
+ outputs = []
+ for t in output_stracks:
+ output= []
+ tlwh = t.tlwh
+ tid = t.track_id
+ tlwh = np.expand_dims(tlwh, axis=0)
+ xyxy = xywh2xyxy(tlwh)
+ xyxy = np.squeeze(xyxy, axis=0)
+ output.extend(xyxy)
+ output.append(tid)
+ output.append(t.cls)
+ outputs.append(output)
+
+ return outputs
+
+
+def joint_stracks(tlista, tlistb):
+ exists = {}
+ res = []
+ for t in tlista:
+ exists[t.track_id] = 1
+ res.append(t)
+ for t in tlistb:
+ tid = t.track_id
+ if not exists.get(tid, 0):
+ exists[tid] = 1
+ res.append(t)
+ return res
+
+
+def sub_stracks(tlista, tlistb):
+ stracks = {}
+ for t in tlista:
+ stracks[t.track_id] = t
+ for t in tlistb:
+ tid = t.track_id
+ if stracks.get(tid, 0):
+ del stracks[tid]
+ return list(stracks.values())
+
+
+def remove_duplicate_stracks(stracksa, stracksb):
+ pdist = matching.iou_distance(stracksa, stracksb)
+ pairs = np.where(pdist < 0.15)
+ dupa, dupb = list(), list()
+ for p, q in zip(*pairs):
+ timep = stracksa[p].frame_id - stracksa[p].start_frame
+ timeq = stracksb[q].frame_id - stracksb[q].start_frame
+ if timep > timeq:
+ dupb.append(q)
+ else:
+ dupa.append(p)
+ resa = [t for i, t in enumerate(stracksa) if not i in dupa]
+ resb = [t for i, t in enumerate(stracksb) if not i in dupb]
+ return resa, resb
diff --git a/trackers/bytetrack/kalman_filter.py b/trackers/bytetrack/kalman_filter.py
new file mode 100644
index 0000000000000000000000000000000000000000..deda8a26292b81bc6512a8f6145afabde6c16d7a
--- /dev/null
+++ b/trackers/bytetrack/kalman_filter.py
@@ -0,0 +1,270 @@
+# vim: expandtab:ts=4:sw=4
+import numpy as np
+import scipy.linalg
+
+
+"""
+Table for the 0.95 quantile of the chi-square distribution with N degrees of
+freedom (contains values for N=1, ..., 9). Taken from MATLAB/Octave's chi2inv
+function and used as Mahalanobis gating threshold.
+"""
+chi2inv95 = {
+ 1: 3.8415,
+ 2: 5.9915,
+ 3: 7.8147,
+ 4: 9.4877,
+ 5: 11.070,
+ 6: 12.592,
+ 7: 14.067,
+ 8: 15.507,
+ 9: 16.919}
+
+
+class KalmanFilter(object):
+ """
+ A simple Kalman filter for tracking bounding boxes in image space.
+
+ The 8-dimensional state space
+
+ x, y, a, h, vx, vy, va, vh
+
+ contains the bounding box center position (x, y), aspect ratio a, height h,
+ and their respective velocities.
+
+ Object motion follows a constant velocity model. The bounding box location
+ (x, y, a, h) is taken as direct observation of the state space (linear
+ observation model).
+
+ """
+
+ def __init__(self):
+ ndim, dt = 4, 1.
+
+ # Create Kalman filter model matrices.
+ self._motion_mat = np.eye(2 * ndim, 2 * ndim)
+ for i in range(ndim):
+ self._motion_mat[i, ndim + i] = dt
+ self._update_mat = np.eye(ndim, 2 * ndim)
+
+ # Motion and observation uncertainty are chosen relative to the current
+ # state estimate. These weights control the amount of uncertainty in
+ # the model. This is a bit hacky.
+ self._std_weight_position = 1. / 20
+ self._std_weight_velocity = 1. / 160
+
+ def initiate(self, measurement):
+ """Create track from unassociated measurement.
+
+ Parameters
+ ----------
+ measurement : ndarray
+ Bounding box coordinates (x, y, a, h) with center position (x, y),
+ aspect ratio a, and height h.
+
+ Returns
+ -------
+ (ndarray, ndarray)
+ Returns the mean vector (8 dimensional) and covariance matrix (8x8
+ dimensional) of the new track. Unobserved velocities are initialized
+ to 0 mean.
+
+ """
+ mean_pos = measurement
+ mean_vel = np.zeros_like(mean_pos)
+ mean = np.r_[mean_pos, mean_vel]
+
+ std = [
+ 2 * self._std_weight_position * measurement[3],
+ 2 * self._std_weight_position * measurement[3],
+ 1e-2,
+ 2 * self._std_weight_position * measurement[3],
+ 10 * self._std_weight_velocity * measurement[3],
+ 10 * self._std_weight_velocity * measurement[3],
+ 1e-5,
+ 10 * self._std_weight_velocity * measurement[3]]
+ covariance = np.diag(np.square(std))
+ return mean, covariance
+
+ def predict(self, mean, covariance):
+ """Run Kalman filter prediction step.
+
+ Parameters
+ ----------
+ mean : ndarray
+ The 8 dimensional mean vector of the object state at the previous
+ time step.
+ covariance : ndarray
+ The 8x8 dimensional covariance matrix of the object state at the
+ previous time step.
+
+ Returns
+ -------
+ (ndarray, ndarray)
+ Returns the mean vector and covariance matrix of the predicted
+ state. Unobserved velocities are initialized to 0 mean.
+
+ """
+ std_pos = [
+ self._std_weight_position * mean[3],
+ self._std_weight_position * mean[3],
+ 1e-2,
+ self._std_weight_position * mean[3]]
+ std_vel = [
+ self._std_weight_velocity * mean[3],
+ self._std_weight_velocity * mean[3],
+ 1e-5,
+ self._std_weight_velocity * mean[3]]
+ motion_cov = np.diag(np.square(np.r_[std_pos, std_vel]))
+
+ #mean = np.dot(self._motion_mat, mean)
+ mean = np.dot(mean, self._motion_mat.T)
+ covariance = np.linalg.multi_dot((
+ self._motion_mat, covariance, self._motion_mat.T)) + motion_cov
+
+ return mean, covariance
+
+ def project(self, mean, covariance):
+ """Project state distribution to measurement space.
+
+ Parameters
+ ----------
+ mean : ndarray
+ The state's mean vector (8 dimensional array).
+ covariance : ndarray
+ The state's covariance matrix (8x8 dimensional).
+
+ Returns
+ -------
+ (ndarray, ndarray)
+ Returns the projected mean and covariance matrix of the given state
+ estimate.
+
+ """
+ std = [
+ self._std_weight_position * mean[3],
+ self._std_weight_position * mean[3],
+ 1e-1,
+ self._std_weight_position * mean[3]]
+ innovation_cov = np.diag(np.square(std))
+
+ mean = np.dot(self._update_mat, mean)
+ covariance = np.linalg.multi_dot((
+ self._update_mat, covariance, self._update_mat.T))
+ return mean, covariance + innovation_cov
+
+ def multi_predict(self, mean, covariance):
+ """Run Kalman filter prediction step (Vectorized version).
+ Parameters
+ ----------
+ mean : ndarray
+ The Nx8 dimensional mean matrix of the object states at the previous
+ time step.
+ covariance : ndarray
+ The Nx8x8 dimensional covariance matrics of the object states at the
+ previous time step.
+ Returns
+ -------
+ (ndarray, ndarray)
+ Returns the mean vector and covariance matrix of the predicted
+ state. Unobserved velocities are initialized to 0 mean.
+ """
+ std_pos = [
+ self._std_weight_position * mean[:, 3],
+ self._std_weight_position * mean[:, 3],
+ 1e-2 * np.ones_like(mean[:, 3]),
+ self._std_weight_position * mean[:, 3]]
+ std_vel = [
+ self._std_weight_velocity * mean[:, 3],
+ self._std_weight_velocity * mean[:, 3],
+ 1e-5 * np.ones_like(mean[:, 3]),
+ self._std_weight_velocity * mean[:, 3]]
+ sqr = np.square(np.r_[std_pos, std_vel]).T
+
+ motion_cov = []
+ for i in range(len(mean)):
+ motion_cov.append(np.diag(sqr[i]))
+ motion_cov = np.asarray(motion_cov)
+
+ mean = np.dot(mean, self._motion_mat.T)
+ left = np.dot(self._motion_mat, covariance).transpose((1, 0, 2))
+ covariance = np.dot(left, self._motion_mat.T) + motion_cov
+
+ return mean, covariance
+
+ def update(self, mean, covariance, measurement):
+ """Run Kalman filter correction step.
+
+ Parameters
+ ----------
+ mean : ndarray
+ The predicted state's mean vector (8 dimensional).
+ covariance : ndarray
+ The state's covariance matrix (8x8 dimensional).
+ measurement : ndarray
+ The 4 dimensional measurement vector (x, y, a, h), where (x, y)
+ is the center position, a the aspect ratio, and h the height of the
+ bounding box.
+
+ Returns
+ -------
+ (ndarray, ndarray)
+ Returns the measurement-corrected state distribution.
+
+ """
+ projected_mean, projected_cov = self.project(mean, covariance)
+
+ chol_factor, lower = scipy.linalg.cho_factor(
+ projected_cov, lower=True, check_finite=False)
+ kalman_gain = scipy.linalg.cho_solve(
+ (chol_factor, lower), np.dot(covariance, self._update_mat.T).T,
+ check_finite=False).T
+ innovation = measurement - projected_mean
+
+ new_mean = mean + np.dot(innovation, kalman_gain.T)
+ new_covariance = covariance - np.linalg.multi_dot((
+ kalman_gain, projected_cov, kalman_gain.T))
+ return new_mean, new_covariance
+
+ def gating_distance(self, mean, covariance, measurements,
+ only_position=False, metric='maha'):
+ """Compute gating distance between state distribution and measurements.
+ A suitable distance threshold can be obtained from `chi2inv95`. If
+ `only_position` is False, the chi-square distribution has 4 degrees of
+ freedom, otherwise 2.
+ Parameters
+ ----------
+ mean : ndarray
+ Mean vector over the state distribution (8 dimensional).
+ covariance : ndarray
+ Covariance of the state distribution (8x8 dimensional).
+ measurements : ndarray
+ An Nx4 dimensional matrix of N measurements, each in
+ format (x, y, a, h) where (x, y) is the bounding box center
+ position, a the aspect ratio, and h the height.
+ only_position : Optional[bool]
+ If True, distance computation is done with respect to the bounding
+ box center position only.
+ Returns
+ -------
+ ndarray
+ Returns an array of length N, where the i-th element contains the
+ squared Mahalanobis distance between (mean, covariance) and
+ `measurements[i]`.
+ """
+ mean, covariance = self.project(mean, covariance)
+ if only_position:
+ mean, covariance = mean[:2], covariance[:2, :2]
+ measurements = measurements[:, :2]
+
+ d = measurements - mean
+ if metric == 'gaussian':
+ return np.sum(d * d, axis=1)
+ elif metric == 'maha':
+ cholesky_factor = np.linalg.cholesky(covariance)
+ z = scipy.linalg.solve_triangular(
+ cholesky_factor, d.T, lower=True, check_finite=False,
+ overwrite_b=True)
+ squared_maha = np.sum(z * z, axis=0)
+ return squared_maha
+ else:
+ raise ValueError('invalid distance metric')
\ No newline at end of file
diff --git a/trackers/bytetrack/matching.py b/trackers/bytetrack/matching.py
new file mode 100644
index 0000000000000000000000000000000000000000..9ceb1b12ee62a1f2c64c5c1510ffec8be92f3245
--- /dev/null
+++ b/trackers/bytetrack/matching.py
@@ -0,0 +1,180 @@
+import cv2
+import numpy as np
+import scipy
+import lap
+from scipy.spatial.distance import cdist
+from cython_bbox import bbox_overlaps as bbox_ious
+from trackers.bytetrack import kalman_filter
+import time
+
+def merge_matches(m1, m2, shape):
+ O,P,Q = shape
+ m1 = np.asarray(m1)
+ m2 = np.asarray(m2)
+
+ M1 = scipy.sparse.coo_matrix((np.ones(len(m1)), (m1[:, 0], m1[:, 1])), shape=(O, P))
+ M2 = scipy.sparse.coo_matrix((np.ones(len(m2)), (m2[:, 0], m2[:, 1])), shape=(P, Q))
+
+ mask = M1*M2
+ match = mask.nonzero()
+ match = list(zip(match[0], match[1]))
+ unmatched_O = tuple(set(range(O)) - set([i for i, j in match]))
+ unmatched_Q = tuple(set(range(Q)) - set([j for i, j in match]))
+
+ return match, unmatched_O, unmatched_Q
+
+
+def _indices_to_matches(cost_matrix, indices, thresh):
+ matched_cost = cost_matrix[tuple(zip(*indices))]
+ matched_mask = (matched_cost <= thresh)
+
+ matches = indices[matched_mask]
+ unmatched_a = tuple(set(range(cost_matrix.shape[0])) - set(matches[:, 0]))
+ unmatched_b = tuple(set(range(cost_matrix.shape[1])) - set(matches[:, 1]))
+
+ return matches, unmatched_a, unmatched_b
+
+
+def linear_assignment(cost_matrix, thresh):
+ if cost_matrix.size == 0:
+ return np.empty((0, 2), dtype=int), tuple(range(cost_matrix.shape[0])), tuple(range(cost_matrix.shape[1]))
+ matches, unmatched_a, unmatched_b = [], [], []
+ cost, x, y = lap.lapjv(cost_matrix, extend_cost=True, cost_limit=thresh)
+ for ix, mx in enumerate(x):
+ if mx >= 0:
+ matches.append([ix, mx])
+ unmatched_a = np.where(x < 0)[0]
+ unmatched_b = np.where(y < 0)[0]
+ matches = np.asarray(matches)
+ return matches, unmatched_a, unmatched_b
+
+
+def ious(atlbrs, btlbrs):
+ """
+ Compute cost based on IoU
+ :type atlbrs: list[tlbr] | np.ndarray
+ :type atlbrs: list[tlbr] | np.ndarray
+
+ :rtype ious np.ndarray
+ """
+ ious = np.zeros((len(atlbrs), len(btlbrs)), dtype=np.float64)
+ if ious.size == 0:
+ return ious
+
+ ious = bbox_ious(
+ np.ascontiguousarray(atlbrs, dtype=np.float64),
+ np.ascontiguousarray(btlbrs, dtype=np.float64)
+ )
+
+ return ious
+
+
+def iou_distance(atracks, btracks):
+ """
+ Compute cost based on IoU
+ :type atracks: list[STrack]
+ :type btracks: list[STrack]
+
+ :rtype cost_matrix np.ndarray
+ """
+
+ if (len(atracks)>0 and isinstance(atracks[0], np.ndarray)) or (len(btracks) > 0 and isinstance(btracks[0], np.ndarray)):
+ atlbrs = atracks
+ btlbrs = btracks
+ else:
+ atlbrs = [track.tlbr for track in atracks]
+ btlbrs = [track.tlbr for track in btracks]
+ _ious = ious(atlbrs, btlbrs)
+ cost_matrix = 1 - _ious
+
+ return cost_matrix
+
+def v_iou_distance(atracks, btracks):
+ """
+ Compute cost based on IoU
+ :type atracks: list[STrack]
+ :type btracks: list[STrack]
+
+ :rtype cost_matrix np.ndarray
+ """
+
+ if (len(atracks)>0 and isinstance(atracks[0], np.ndarray)) or (len(btracks) > 0 and isinstance(btracks[0], np.ndarray)):
+ atlbrs = atracks
+ btlbrs = btracks
+ else:
+ atlbrs = [track.tlwh_to_tlbr(track.pred_bbox) for track in atracks]
+ btlbrs = [track.tlwh_to_tlbr(track.pred_bbox) for track in btracks]
+ _ious = ious(atlbrs, btlbrs)
+ cost_matrix = 1 - _ious
+
+ return cost_matrix
+
+def embedding_distance(tracks, detections, metric='cosine'):
+ """
+ :param tracks: list[STrack]
+ :param detections: list[BaseTrack]
+ :param metric:
+ :return: cost_matrix np.ndarray
+ """
+
+ cost_matrix = np.zeros((len(tracks), len(detections)), dtype=np.float64)
+ if cost_matrix.size == 0:
+ return cost_matrix
+ det_features = np.asarray([track.curr_feat for track in detections], dtype=np.float64)
+ #for i, track in enumerate(tracks):
+ #cost_matrix[i, :] = np.maximum(0.0, cdist(track.smooth_feat.reshape(1,-1), det_features, metric))
+ track_features = np.asarray([track.smooth_feat for track in tracks], dtype=np.float64)
+ cost_matrix = np.maximum(0.0, cdist(track_features, det_features, metric)) # Nomalized features
+ return cost_matrix
+
+
+def gate_cost_matrix(kf, cost_matrix, tracks, detections, only_position=False):
+ if cost_matrix.size == 0:
+ return cost_matrix
+ gating_dim = 2 if only_position else 4
+ gating_threshold = kalman_filter.chi2inv95[gating_dim]
+ measurements = np.asarray([det.to_xyah() for det in detections])
+ for row, track in enumerate(tracks):
+ gating_distance = kf.gating_distance(
+ track.mean, track.covariance, measurements, only_position)
+ cost_matrix[row, gating_distance > gating_threshold] = np.inf
+ return cost_matrix
+
+
+def fuse_motion(kf, cost_matrix, tracks, detections, only_position=False, lambda_=0.98):
+ if cost_matrix.size == 0:
+ return cost_matrix
+ gating_dim = 2 if only_position else 4
+ gating_threshold = kalman_filter.chi2inv95[gating_dim]
+ measurements = np.asarray([det.to_xyah() for det in detections])
+ for row, track in enumerate(tracks):
+ gating_distance = kf.gating_distance(
+ track.mean, track.covariance, measurements, only_position, metric='maha')
+ cost_matrix[row, gating_distance > gating_threshold] = np.inf
+ cost_matrix[row] = lambda_ * cost_matrix[row] + (1 - lambda_) * gating_distance
+ return cost_matrix
+
+
+def fuse_iou(cost_matrix, tracks, detections):
+ if cost_matrix.size == 0:
+ return cost_matrix
+ reid_sim = 1 - cost_matrix
+ iou_dist = iou_distance(tracks, detections)
+ iou_sim = 1 - iou_dist
+ fuse_sim = reid_sim * (1 + iou_sim) / 2
+ det_scores = np.array([det.score for det in detections])
+ det_scores = np.expand_dims(det_scores, axis=0).repeat(cost_matrix.shape[0], axis=0)
+ #fuse_sim = fuse_sim * (1 + det_scores) / 2
+ fuse_cost = 1 - fuse_sim
+ return fuse_cost
+
+
+def fuse_score(cost_matrix, detections):
+ if cost_matrix.size == 0:
+ return cost_matrix
+ iou_sim = 1 - cost_matrix
+ det_scores = np.array([det.score for det in detections])
+ det_scores = np.expand_dims(det_scores, axis=0).repeat(cost_matrix.shape[0], axis=0)
+ fuse_sim = iou_sim * det_scores
+ fuse_cost = 1 - fuse_sim
+ return fuse_cost
\ No newline at end of file
diff --git a/trackers/multi_tracker_zoo.py b/trackers/multi_tracker_zoo.py
new file mode 100644
index 0000000000000000000000000000000000000000..c04c845ce94e9b2edcc51835fe8542a04663ac1d
--- /dev/null
+++ b/trackers/multi_tracker_zoo.py
@@ -0,0 +1,44 @@
+from trackers.strong_sort.utils.parser import get_config
+from trackers.strong_sort.strong_sort import StrongSORT
+from trackers.ocsort.ocsort import OCSort
+from trackers.bytetrack.byte_tracker import BYTETracker
+
+
+def create_tracker(tracker_type, appearance_descriptor_weights, device, half):
+ if tracker_type == 'strongsort':
+ # initialize StrongSORT
+ cfg = get_config()
+ cfg.merge_from_file('trackers/strong_sort/configs/strong_sort.yaml')
+
+ strongsort = StrongSORT(
+ appearance_descriptor_weights,
+ device,
+ half,
+ max_dist=cfg.STRONGSORT.MAX_DIST,
+ max_iou_distance=cfg.STRONGSORT.MAX_IOU_DISTANCE,
+ max_age=cfg.STRONGSORT.MAX_AGE,
+ n_init=cfg.STRONGSORT.N_INIT,
+ nn_budget=cfg.STRONGSORT.NN_BUDGET,
+ mc_lambda=cfg.STRONGSORT.MC_LAMBDA,
+ ema_alpha=cfg.STRONGSORT.EMA_ALPHA,
+
+ )
+ return strongsort
+ elif tracker_type == 'ocsort':
+ ocsort = OCSort(
+ det_thresh=0.45,
+ iou_threshold=0.2,
+ use_byte=False
+ )
+ return ocsort
+ elif tracker_type == 'bytetrack':
+ bytetracker = BYTETracker(
+ track_thresh=0.6,
+ track_buffer=30,
+ match_thresh=0.8,
+ frame_rate=30
+ )
+ return bytetracker
+ else:
+ print('No such tracker')
+ exit()
\ No newline at end of file
diff --git a/trackers/ocsort/__pycache__/association.cpython-38.pyc b/trackers/ocsort/__pycache__/association.cpython-38.pyc
new file mode 100644
index 0000000000000000000000000000000000000000..ffaae52bc51360be55ad2f89c914fa7b9d229851
Binary files /dev/null and b/trackers/ocsort/__pycache__/association.cpython-38.pyc differ
diff --git a/trackers/ocsort/__pycache__/association.cpython-39.pyc b/trackers/ocsort/__pycache__/association.cpython-39.pyc
new file mode 100644
index 0000000000000000000000000000000000000000..4ad7a8450e7b2b3c757a113749d6f5e73b4f8564
Binary files /dev/null and b/trackers/ocsort/__pycache__/association.cpython-39.pyc differ
diff --git a/trackers/ocsort/__pycache__/kalmanfilter.cpython-38.pyc b/trackers/ocsort/__pycache__/kalmanfilter.cpython-38.pyc
new file mode 100644
index 0000000000000000000000000000000000000000..7750a315d6ccba98b296450202c0b265ac0887ec
Binary files /dev/null and b/trackers/ocsort/__pycache__/kalmanfilter.cpython-38.pyc differ
diff --git a/trackers/ocsort/__pycache__/ocsort.cpython-38.pyc b/trackers/ocsort/__pycache__/ocsort.cpython-38.pyc
new file mode 100644
index 0000000000000000000000000000000000000000..97082d0ee455d20cca808cc8f6a27cbc63961e26
Binary files /dev/null and b/trackers/ocsort/__pycache__/ocsort.cpython-38.pyc differ
diff --git a/trackers/ocsort/__pycache__/ocsort.cpython-39.pyc b/trackers/ocsort/__pycache__/ocsort.cpython-39.pyc
new file mode 100644
index 0000000000000000000000000000000000000000..36e848504cf1116739fdf4f10bd89a7148214c4d
Binary files /dev/null and b/trackers/ocsort/__pycache__/ocsort.cpython-39.pyc differ
diff --git a/trackers/ocsort/association.py b/trackers/ocsort/association.py
new file mode 100644
index 0000000000000000000000000000000000000000..1b034197ef83f3e872d60564442b8059177df240
--- /dev/null
+++ b/trackers/ocsort/association.py
@@ -0,0 +1,377 @@
+import os
+import numpy as np
+
+
+def iou_batch(bboxes1, bboxes2):
+ """
+ From SORT: Computes IOU between two bboxes in the form [x1,y1,x2,y2]
+ """
+ bboxes2 = np.expand_dims(bboxes2, 0)
+ bboxes1 = np.expand_dims(bboxes1, 1)
+
+ xx1 = np.maximum(bboxes1[..., 0], bboxes2[..., 0])
+ yy1 = np.maximum(bboxes1[..., 1], bboxes2[..., 1])
+ xx2 = np.minimum(bboxes1[..., 2], bboxes2[..., 2])
+ yy2 = np.minimum(bboxes1[..., 3], bboxes2[..., 3])
+ w = np.maximum(0., xx2 - xx1)
+ h = np.maximum(0., yy2 - yy1)
+ wh = w * h
+ o = wh / ((bboxes1[..., 2] - bboxes1[..., 0]) * (bboxes1[..., 3] - bboxes1[..., 1])
+ + (bboxes2[..., 2] - bboxes2[..., 0]) * (bboxes2[..., 3] - bboxes2[..., 1]) - wh)
+ return(o)
+
+
+def giou_batch(bboxes1, bboxes2):
+ """
+ :param bbox_p: predict of bbox(N,4)(x1,y1,x2,y2)
+ :param bbox_g: groundtruth of bbox(N,4)(x1,y1,x2,y2)
+ :return:
+ """
+ # for details should go to https://arxiv.org/pdf/1902.09630.pdf
+ # ensure predict's bbox form
+ bboxes2 = np.expand_dims(bboxes2, 0)
+ bboxes1 = np.expand_dims(bboxes1, 1)
+
+ xx1 = np.maximum(bboxes1[..., 0], bboxes2[..., 0])
+ yy1 = np.maximum(bboxes1[..., 1], bboxes2[..., 1])
+ xx2 = np.minimum(bboxes1[..., 2], bboxes2[..., 2])
+ yy2 = np.minimum(bboxes1[..., 3], bboxes2[..., 3])
+ w = np.maximum(0., xx2 - xx1)
+ h = np.maximum(0., yy2 - yy1)
+ wh = w * h
+ iou = wh / ((bboxes1[..., 2] - bboxes1[..., 0]) * (bboxes1[..., 3] - bboxes1[..., 1])
+ + (bboxes2[..., 2] - bboxes2[..., 0]) * (bboxes2[..., 3] - bboxes2[..., 1]) - wh)
+
+ xxc1 = np.minimum(bboxes1[..., 0], bboxes2[..., 0])
+ yyc1 = np.minimum(bboxes1[..., 1], bboxes2[..., 1])
+ xxc2 = np.maximum(bboxes1[..., 2], bboxes2[..., 2])
+ yyc2 = np.maximum(bboxes1[..., 3], bboxes2[..., 3])
+ wc = xxc2 - xxc1
+ hc = yyc2 - yyc1
+ assert((wc > 0).all() and (hc > 0).all())
+ area_enclose = wc * hc
+ giou = iou - (area_enclose - wh) / area_enclose
+ giou = (giou + 1.)/2.0 # resize from (-1,1) to (0,1)
+ return giou
+
+
+def diou_batch(bboxes1, bboxes2):
+ """
+ :param bbox_p: predict of bbox(N,4)(x1,y1,x2,y2)
+ :param bbox_g: groundtruth of bbox(N,4)(x1,y1,x2,y2)
+ :return:
+ """
+ # for details should go to https://arxiv.org/pdf/1902.09630.pdf
+ # ensure predict's bbox form
+ bboxes2 = np.expand_dims(bboxes2, 0)
+ bboxes1 = np.expand_dims(bboxes1, 1)
+
+ # calculate the intersection box
+ xx1 = np.maximum(bboxes1[..., 0], bboxes2[..., 0])
+ yy1 = np.maximum(bboxes1[..., 1], bboxes2[..., 1])
+ xx2 = np.minimum(bboxes1[..., 2], bboxes2[..., 2])
+ yy2 = np.minimum(bboxes1[..., 3], bboxes2[..., 3])
+ w = np.maximum(0., xx2 - xx1)
+ h = np.maximum(0., yy2 - yy1)
+ wh = w * h
+ iou = wh / ((bboxes1[..., 2] - bboxes1[..., 0]) * (bboxes1[..., 3] - bboxes1[..., 1])
+ + (bboxes2[..., 2] - bboxes2[..., 0]) * (bboxes2[..., 3] - bboxes2[..., 1]) - wh)
+
+ centerx1 = (bboxes1[..., 0] + bboxes1[..., 2]) / 2.0
+ centery1 = (bboxes1[..., 1] + bboxes1[..., 3]) / 2.0
+ centerx2 = (bboxes2[..., 0] + bboxes2[..., 2]) / 2.0
+ centery2 = (bboxes2[..., 1] + bboxes2[..., 3]) / 2.0
+
+ inner_diag = (centerx1 - centerx2) ** 2 + (centery1 - centery2) ** 2
+
+ xxc1 = np.minimum(bboxes1[..., 0], bboxes2[..., 0])
+ yyc1 = np.minimum(bboxes1[..., 1], bboxes2[..., 1])
+ xxc2 = np.maximum(bboxes1[..., 2], bboxes2[..., 2])
+ yyc2 = np.maximum(bboxes1[..., 3], bboxes2[..., 3])
+
+ outer_diag = (xxc2 - xxc1) ** 2 + (yyc2 - yyc1) ** 2
+ diou = iou - inner_diag / outer_diag
+
+ return (diou + 1) / 2.0 # resize from (-1,1) to (0,1)
+
+def ciou_batch(bboxes1, bboxes2):
+ """
+ :param bbox_p: predict of bbox(N,4)(x1,y1,x2,y2)
+ :param bbox_g: groundtruth of bbox(N,4)(x1,y1,x2,y2)
+ :return:
+ """
+ # for details should go to https://arxiv.org/pdf/1902.09630.pdf
+ # ensure predict's bbox form
+ bboxes2 = np.expand_dims(bboxes2, 0)
+ bboxes1 = np.expand_dims(bboxes1, 1)
+
+ # calculate the intersection box
+ xx1 = np.maximum(bboxes1[..., 0], bboxes2[..., 0])
+ yy1 = np.maximum(bboxes1[..., 1], bboxes2[..., 1])
+ xx2 = np.minimum(bboxes1[..., 2], bboxes2[..., 2])
+ yy2 = np.minimum(bboxes1[..., 3], bboxes2[..., 3])
+ w = np.maximum(0., xx2 - xx1)
+ h = np.maximum(0., yy2 - yy1)
+ wh = w * h
+ iou = wh / ((bboxes1[..., 2] - bboxes1[..., 0]) * (bboxes1[..., 3] - bboxes1[..., 1])
+ + (bboxes2[..., 2] - bboxes2[..., 0]) * (bboxes2[..., 3] - bboxes2[..., 1]) - wh)
+
+ centerx1 = (bboxes1[..., 0] + bboxes1[..., 2]) / 2.0
+ centery1 = (bboxes1[..., 1] + bboxes1[..., 3]) / 2.0
+ centerx2 = (bboxes2[..., 0] + bboxes2[..., 2]) / 2.0
+ centery2 = (bboxes2[..., 1] + bboxes2[..., 3]) / 2.0
+
+ inner_diag = (centerx1 - centerx2) ** 2 + (centery1 - centery2) ** 2
+
+ xxc1 = np.minimum(bboxes1[..., 0], bboxes2[..., 0])
+ yyc1 = np.minimum(bboxes1[..., 1], bboxes2[..., 1])
+ xxc2 = np.maximum(bboxes1[..., 2], bboxes2[..., 2])
+ yyc2 = np.maximum(bboxes1[..., 3], bboxes2[..., 3])
+
+ outer_diag = (xxc2 - xxc1) ** 2 + (yyc2 - yyc1) ** 2
+
+ w1 = bboxes1[..., 2] - bboxes1[..., 0]
+ h1 = bboxes1[..., 3] - bboxes1[..., 1]
+ w2 = bboxes2[..., 2] - bboxes2[..., 0]
+ h2 = bboxes2[..., 3] - bboxes2[..., 1]
+
+ # prevent dividing over zero. add one pixel shift
+ h2 = h2 + 1.
+ h1 = h1 + 1.
+ arctan = np.arctan(w2/h2) - np.arctan(w1/h1)
+ v = (4 / (np.pi ** 2)) * (arctan ** 2)
+ S = 1 - iou
+ alpha = v / (S+v)
+ ciou = iou - inner_diag / outer_diag - alpha * v
+
+ return (ciou + 1) / 2.0 # resize from (-1,1) to (0,1)
+
+
+def ct_dist(bboxes1, bboxes2):
+ """
+ Measure the center distance between two sets of bounding boxes,
+ this is a coarse implementation, we don't recommend using it only
+ for association, which can be unstable and sensitive to frame rate
+ and object speed.
+ """
+ bboxes2 = np.expand_dims(bboxes2, 0)
+ bboxes1 = np.expand_dims(bboxes1, 1)
+
+ centerx1 = (bboxes1[..., 0] + bboxes1[..., 2]) / 2.0
+ centery1 = (bboxes1[..., 1] + bboxes1[..., 3]) / 2.0
+ centerx2 = (bboxes2[..., 0] + bboxes2[..., 2]) / 2.0
+ centery2 = (bboxes2[..., 1] + bboxes2[..., 3]) / 2.0
+
+ ct_dist2 = (centerx1 - centerx2) ** 2 + (centery1 - centery2) ** 2
+
+ ct_dist = np.sqrt(ct_dist2)
+
+ # The linear rescaling is a naive version and needs more study
+ ct_dist = ct_dist / ct_dist.max()
+ return ct_dist.max() - ct_dist # resize to (0,1)
+
+
+
+def speed_direction_batch(dets, tracks):
+ tracks = tracks[..., np.newaxis]
+ CX1, CY1 = (dets[:,0] + dets[:,2])/2.0, (dets[:,1]+dets[:,3])/2.0
+ CX2, CY2 = (tracks[:,0] + tracks[:,2]) /2.0, (tracks[:,1]+tracks[:,3])/2.0
+ dx = CX1 - CX2
+ dy = CY1 - CY2
+ norm = np.sqrt(dx**2 + dy**2) + 1e-6
+ dx = dx / norm
+ dy = dy / norm
+ return dy, dx # size: num_track x num_det
+
+
+def linear_assignment(cost_matrix):
+ try:
+ import lap
+ _, x, y = lap.lapjv(cost_matrix, extend_cost=True)
+ return np.array([[y[i],i] for i in x if i >= 0]) #
+ except ImportError:
+ from scipy.optimize import linear_sum_assignment
+ x, y = linear_sum_assignment(cost_matrix)
+ return np.array(list(zip(x, y)))
+
+
+def associate_detections_to_trackers(detections,trackers,iou_threshold = 0.3):
+ """
+ Assigns detections to tracked object (both represented as bounding boxes)
+ Returns 3 lists of matches, unmatched_detections and unmatched_trackers
+ """
+ if(len(trackers)==0):
+ return np.empty((0,2),dtype=int), np.arange(len(detections)), np.empty((0,5),dtype=int)
+
+ iou_matrix = iou_batch(detections, trackers)
+
+ if min(iou_matrix.shape) > 0:
+ a = (iou_matrix > iou_threshold).astype(np.int32)
+ if a.sum(1).max() == 1 and a.sum(0).max() == 1:
+ matched_indices = np.stack(np.where(a), axis=1)
+ else:
+ matched_indices = linear_assignment(-iou_matrix)
+ else:
+ matched_indices = np.empty(shape=(0,2))
+
+ unmatched_detections = []
+ for d, det in enumerate(detections):
+ if(d not in matched_indices[:,0]):
+ unmatched_detections.append(d)
+ unmatched_trackers = []
+ for t, trk in enumerate(trackers):
+ if(t not in matched_indices[:,1]):
+ unmatched_trackers.append(t)
+
+ #filter out matched with low IOU
+ matches = []
+ for m in matched_indices:
+ if(iou_matrix[m[0], m[1]] 0:
+ a = (iou_matrix > iou_threshold).astype(np.int32)
+ if a.sum(1).max() == 1 and a.sum(0).max() == 1:
+ matched_indices = np.stack(np.where(a), axis=1)
+ else:
+ matched_indices = linear_assignment(-(iou_matrix+angle_diff_cost))
+ else:
+ matched_indices = np.empty(shape=(0,2))
+
+ unmatched_detections = []
+ for d, det in enumerate(detections):
+ if(d not in matched_indices[:,0]):
+ unmatched_detections.append(d)
+ unmatched_trackers = []
+ for t, trk in enumerate(trackers):
+ if(t not in matched_indices[:,1]):
+ unmatched_trackers.append(t)
+
+ # filter out matched with low IOU
+ matches = []
+ for m in matched_indices:
+ if(iou_matrix[m[0], m[1]] 0:
+ a = (iou_matrix > iou_threshold).astype(np.int32)
+ if a.sum(1).max() == 1 and a.sum(0).max() == 1:
+ matched_indices = np.stack(np.where(a), axis=1)
+ else:
+ matched_indices = linear_assignment(cost_matrix)
+ else:
+ matched_indices = np.empty(shape=(0,2))
+
+ unmatched_detections = []
+ for d, det in enumerate(detections):
+ if(d not in matched_indices[:,0]):
+ unmatched_detections.append(d)
+ unmatched_trackers = []
+ for t, trk in enumerate(trackers):
+ if(t not in matched_indices[:,1]):
+ unmatched_trackers.append(t)
+
+ #filter out matched with low IOU
+ matches = []
+ for m in matched_indices:
+ if(iou_matrix[m[0], m[1]]update cycle. The
+predict step, implemented with the method or function predict(),
+uses the state transition matrix F to predict the state in the next
+time period (epoch). The state is stored as a gaussian (x, P), where
+x is the state (column) vector, and P is its covariance. Covariance
+matrix Q specifies the process covariance. In Bayesian terms, this
+prediction is called the *prior*, which you can think of colloquially
+as the estimate prior to incorporating the measurement.
+The update step, implemented with the method or function `update()`,
+incorporates the measurement z with covariance R, into the state
+estimate (x, P). The class stores the system uncertainty in S,
+the innovation (residual between prediction and measurement in
+measurement space) in y, and the Kalman gain in k. The procedural
+form returns these variables to you. In Bayesian terms this computes
+the *posterior* - the estimate after the information from the
+measurement is incorporated.
+Whether you use the OO form or procedural form is up to you. If
+matrices such as H, R, and F are changing each epoch, you'll probably
+opt to use the procedural form. If they are unchanging, the OO
+form is perhaps easier to use since you won't need to keep track
+of these matrices. This is especially useful if you are implementing
+banks of filters or comparing various KF designs for performance;
+a trivial coding bug could lead to using the wrong sets of matrices.
+This module also offers an implementation of the RTS smoother, and
+other helper functions, such as log likelihood computations.
+The Saver class allows you to easily save the state of the
+KalmanFilter class after every update
+This module expects NumPy arrays for all values that expect
+arrays, although in a few cases, particularly method parameters,
+it will accept types that convert to NumPy arrays, such as lists
+of lists. These exceptions are documented in the method or function.
+Examples
+--------
+The following example constructs a constant velocity kinematic
+filter, filters noisy data, and plots the results. It also demonstrates
+using the Saver class to save the state of the filter at each epoch.
+.. code-block:: Python
+ import matplotlib.pyplot as plt
+ import numpy as np
+ from filterpy.kalman import KalmanFilter
+ from filterpy.common import Q_discrete_white_noise, Saver
+ r_std, q_std = 2., 0.003
+ cv = KalmanFilter(dim_x=2, dim_z=1)
+ cv.x = np.array([[0., 1.]]) # position, velocity
+ cv.F = np.array([[1, dt],[ [0, 1]])
+ cv.R = np.array([[r_std^^2]])
+ f.H = np.array([[1., 0.]])
+ f.P = np.diag([.1^^2, .03^^2)
+ f.Q = Q_discrete_white_noise(2, dt, q_std**2)
+ saver = Saver(cv)
+ for z in range(100):
+ cv.predict()
+ cv.update([z + randn() * r_std])
+ saver.save() # save the filter's state
+ saver.to_array()
+ plt.plot(saver.x[:, 0])
+ # plot all of the priors
+ plt.plot(saver.x_prior[:, 0])
+ # plot mahalanobis distance
+ plt.figure()
+ plt.plot(saver.mahalanobis)
+This code implements the same filter using the procedural form
+ x = np.array([[0., 1.]]) # position, velocity
+ F = np.array([[1, dt],[ [0, 1]])
+ R = np.array([[r_std^^2]])
+ H = np.array([[1., 0.]])
+ P = np.diag([.1^^2, .03^^2)
+ Q = Q_discrete_white_noise(2, dt, q_std**2)
+ for z in range(100):
+ x, P = predict(x, P, F=F, Q=Q)
+ x, P = update(x, P, z=[z + randn() * r_std], R=R, H=H)
+ xs.append(x[0, 0])
+ plt.plot(xs)
+For more examples see the test subdirectory, or refer to the
+book cited below. In it I both teach Kalman filtering from basic
+principles, and teach the use of this library in great detail.
+FilterPy library.
+http://github.com/rlabbe/filterpy
+Documentation at:
+https://filterpy.readthedocs.org
+Supporting book at:
+https://github.com/rlabbe/Kalman-and-Bayesian-Filters-in-Python
+This is licensed under an MIT license. See the readme.MD file
+for more information.
+Copyright 2014-2018 Roger R Labbe Jr.
+"""
+
+from __future__ import absolute_import, division
+
+from copy import deepcopy
+from math import log, exp, sqrt
+import sys
+import numpy as np
+from numpy import dot, zeros, eye, isscalar, shape
+import numpy.linalg as linalg
+from filterpy.stats import logpdf
+from filterpy.common import pretty_str, reshape_z
+
+
+class KalmanFilterNew(object):
+ """ Implements a Kalman filter. You are responsible for setting the
+ various state variables to reasonable values; the defaults will
+ not give you a functional filter.
+ For now the best documentation is my free book Kalman and Bayesian
+ Filters in Python [2]_. The test files in this directory also give you a
+ basic idea of use, albeit without much description.
+ In brief, you will first construct this object, specifying the size of
+ the state vector with dim_x and the size of the measurement vector that
+ you will be using with dim_z. These are mostly used to perform size checks
+ when you assign values to the various matrices. For example, if you
+ specified dim_z=2 and then try to assign a 3x3 matrix to R (the
+ measurement noise matrix you will get an assert exception because R
+ should be 2x2. (If for whatever reason you need to alter the size of
+ things midstream just use the underscore version of the matrices to
+ assign directly: your_filter._R = a_3x3_matrix.)
+ After construction the filter will have default matrices created for you,
+ but you must specify the values for each. It’s usually easiest to just
+ overwrite them rather than assign to each element yourself. This will be
+ clearer in the example below. All are of type numpy.array.
+ Examples
+ --------
+ Here is a filter that tracks position and velocity using a sensor that only
+ reads position.
+ First construct the object with the required dimensionality. Here the state
+ (`dim_x`) has 2 coefficients (position and velocity), and the measurement
+ (`dim_z`) has one. In FilterPy `x` is the state, `z` is the measurement.
+ .. code::
+ from filterpy.kalman import KalmanFilter
+ f = KalmanFilter (dim_x=2, dim_z=1)
+ Assign the initial value for the state (position and velocity). You can do this
+ with a two dimensional array like so:
+ .. code::
+ f.x = np.array([[2.], # position
+ [0.]]) # velocity
+ or just use a one dimensional array, which I prefer doing.
+ .. code::
+ f.x = np.array([2., 0.])
+ Define the state transition matrix:
+ .. code::
+ f.F = np.array([[1.,1.],
+ [0.,1.]])
+ Define the measurement function. Here we need to convert a position-velocity
+ vector into just a position vector, so we use:
+ .. code::
+ f.H = np.array([[1., 0.]])
+ Define the state's covariance matrix P.
+ .. code::
+ f.P = np.array([[1000., 0.],
+ [ 0., 1000.] ])
+ Now assign the measurement noise. Here the dimension is 1x1, so I can
+ use a scalar
+ .. code::
+ f.R = 5
+ I could have done this instead:
+ .. code::
+ f.R = np.array([[5.]])
+ Note that this must be a 2 dimensional array.
+ Finally, I will assign the process noise. Here I will take advantage of
+ another FilterPy library function:
+ .. code::
+ from filterpy.common import Q_discrete_white_noise
+ f.Q = Q_discrete_white_noise(dim=2, dt=0.1, var=0.13)
+ Now just perform the standard predict/update loop:
+ .. code::
+ while some_condition_is_true:
+ z = get_sensor_reading()
+ f.predict()
+ f.update(z)
+ do_something_with_estimate (f.x)
+ **Procedural Form**
+ This module also contains stand alone functions to perform Kalman filtering.
+ Use these if you are not a fan of objects.
+ **Example**
+ .. code::
+ while True:
+ z, R = read_sensor()
+ x, P = predict(x, P, F, Q)
+ x, P = update(x, P, z, R, H)
+ See my book Kalman and Bayesian Filters in Python [2]_.
+ You will have to set the following attributes after constructing this
+ object for the filter to perform properly. Please note that there are
+ various checks in place to ensure that you have made everything the
+ 'correct' size. However, it is possible to provide incorrectly sized
+ arrays such that the linear algebra can not perform an operation.
+ It can also fail silently - you can end up with matrices of a size that
+ allows the linear algebra to work, but are the wrong shape for the problem
+ you are trying to solve.
+ Parameters
+ ----------
+ dim_x : int
+ Number of state variables for the Kalman filter. For example, if
+ you are tracking the position and velocity of an object in two
+ dimensions, dim_x would be 4.
+ This is used to set the default size of P, Q, and u
+ dim_z : int
+ Number of of measurement inputs. For example, if the sensor
+ provides you with position in (x,y), dim_z would be 2.
+ dim_u : int (optional)
+ size of the control input, if it is being used.
+ Default value of 0 indicates it is not used.
+ compute_log_likelihood : bool (default = True)
+ Computes log likelihood by default, but this can be a slow
+ computation, so if you never use it you can turn this computation
+ off.
+ Attributes
+ ----------
+ x : numpy.array(dim_x, 1)
+ Current state estimate. Any call to update() or predict() updates
+ this variable.
+ P : numpy.array(dim_x, dim_x)
+ Current state covariance matrix. Any call to update() or predict()
+ updates this variable.
+ x_prior : numpy.array(dim_x, 1)
+ Prior (predicted) state estimate. The *_prior and *_post attributes
+ are for convenience; they store the prior and posterior of the
+ current epoch. Read Only.
+ P_prior : numpy.array(dim_x, dim_x)
+ Prior (predicted) state covariance matrix. Read Only.
+ x_post : numpy.array(dim_x, 1)
+ Posterior (updated) state estimate. Read Only.
+ P_post : numpy.array(dim_x, dim_x)
+ Posterior (updated) state covariance matrix. Read Only.
+ z : numpy.array
+ Last measurement used in update(). Read only.
+ R : numpy.array(dim_z, dim_z)
+ Measurement noise covariance matrix. Also known as the
+ observation covariance.
+ Q : numpy.array(dim_x, dim_x)
+ Process noise covariance matrix. Also known as the transition
+ covariance.
+ F : numpy.array()
+ State Transition matrix. Also known as `A` in some formulation.
+ H : numpy.array(dim_z, dim_x)
+ Measurement function. Also known as the observation matrix, or as `C`.
+ y : numpy.array
+ Residual of the update step. Read only.
+ K : numpy.array(dim_x, dim_z)
+ Kalman gain of the update step. Read only.
+ S : numpy.array
+ System uncertainty (P projected to measurement space). Read only.
+ SI : numpy.array
+ Inverse system uncertainty. Read only.
+ log_likelihood : float
+ log-likelihood of the last measurement. Read only.
+ likelihood : float
+ likelihood of last measurement. Read only.
+ Computed from the log-likelihood. The log-likelihood can be very
+ small, meaning a large negative value such as -28000. Taking the
+ exp() of that results in 0.0, which can break typical algorithms
+ which multiply by this value, so by default we always return a
+ number >= sys.float_info.min.
+ mahalanobis : float
+ mahalanobis distance of the innovation. Read only.
+ inv : function, default numpy.linalg.inv
+ If you prefer another inverse function, such as the Moore-Penrose
+ pseudo inverse, set it to that instead: kf.inv = np.linalg.pinv
+ This is only used to invert self.S. If you know it is diagonal, you
+ might choose to set it to filterpy.common.inv_diagonal, which is
+ several times faster than numpy.linalg.inv for diagonal matrices.
+ alpha : float
+ Fading memory setting. 1.0 gives the normal Kalman filter, and
+ values slightly larger than 1.0 (such as 1.02) give a fading
+ memory effect - previous measurements have less influence on the
+ filter's estimates. This formulation of the Fading memory filter
+ (there are many) is due to Dan Simon [1]_.
+ References
+ ----------
+ .. [1] Dan Simon. "Optimal State Estimation." John Wiley & Sons.
+ p. 208-212. (2006)
+ .. [2] Roger Labbe. "Kalman and Bayesian Filters in Python"
+ https://github.com/rlabbe/Kalman-and-Bayesian-Filters-in-Python
+ """
+
+ def __init__(self, dim_x, dim_z, dim_u=0):
+ if dim_x < 1:
+ raise ValueError('dim_x must be 1 or greater')
+ if dim_z < 1:
+ raise ValueError('dim_z must be 1 or greater')
+ if dim_u < 0:
+ raise ValueError('dim_u must be 0 or greater')
+
+ self.dim_x = dim_x
+ self.dim_z = dim_z
+ self.dim_u = dim_u
+
+ self.x = zeros((dim_x, 1)) # state
+ self.P = eye(dim_x) # uncertainty covariance
+ self.Q = eye(dim_x) # process uncertainty
+ self.B = None # control transition matrix
+ self.F = eye(dim_x) # state transition matrix
+ self.H = zeros((dim_z, dim_x)) # measurement function
+ self.R = eye(dim_z) # measurement uncertainty
+ self._alpha_sq = 1. # fading memory control
+ self.M = np.zeros((dim_x, dim_z)) # process-measurement cross correlation
+ self.z = np.array([[None]*self.dim_z]).T
+
+ # gain and residual are computed during the innovation step. We
+ # save them so that in case you want to inspect them for various
+ # purposes
+ self.K = np.zeros((dim_x, dim_z)) # kalman gain
+ self.y = zeros((dim_z, 1))
+ self.S = np.zeros((dim_z, dim_z)) # system uncertainty
+ self.SI = np.zeros((dim_z, dim_z)) # inverse system uncertainty
+
+ # identity matrix. Do not alter this.
+ self._I = np.eye(dim_x)
+
+ # these will always be a copy of x,P after predict() is called
+ self.x_prior = self.x.copy()
+ self.P_prior = self.P.copy()
+
+ # these will always be a copy of x,P after update() is called
+ self.x_post = self.x.copy()
+ self.P_post = self.P.copy()
+
+ # Only computed only if requested via property
+ self._log_likelihood = log(sys.float_info.min)
+ self._likelihood = sys.float_info.min
+ self._mahalanobis = None
+
+ # keep all observations
+ self.history_obs = []
+
+ self.inv = np.linalg.inv
+
+ self.attr_saved = None
+ self.observed = False
+
+
+ def predict(self, u=None, B=None, F=None, Q=None):
+ """
+ Predict next state (prior) using the Kalman filter state propagation
+ equations.
+ Parameters
+ ----------
+ u : np.array, default 0
+ Optional control vector.
+ B : np.array(dim_x, dim_u), or None
+ Optional control transition matrix; a value of None
+ will cause the filter to use `self.B`.
+ F : np.array(dim_x, dim_x), or None
+ Optional state transition matrix; a value of None
+ will cause the filter to use `self.F`.
+ Q : np.array(dim_x, dim_x), scalar, or None
+ Optional process noise matrix; a value of None will cause the
+ filter to use `self.Q`.
+ """
+
+ if B is None:
+ B = self.B
+ if F is None:
+ F = self.F
+ if Q is None:
+ Q = self.Q
+ elif isscalar(Q):
+ Q = eye(self.dim_x) * Q
+
+
+ # x = Fx + Bu
+ if B is not None and u is not None:
+ self.x = dot(F, self.x) + dot(B, u)
+ else:
+ self.x = dot(F, self.x)
+
+ # P = FPF' + Q
+ self.P = self._alpha_sq * dot(dot(F, self.P), F.T) + Q
+
+ # save prior
+ self.x_prior = self.x.copy()
+ self.P_prior = self.P.copy()
+
+
+
+ def freeze(self):
+ """
+ Save the parameters before non-observation forward
+ """
+ self.attr_saved = deepcopy(self.__dict__)
+
+
+ def unfreeze(self):
+ if self.attr_saved is not None:
+ new_history = deepcopy(self.history_obs)
+ self.__dict__ = self.attr_saved
+ # self.history_obs = new_history
+ self.history_obs = self.history_obs[:-1]
+ occur = [int(d is None) for d in new_history]
+ indices = np.where(np.array(occur)==0)[0]
+ index1 = indices[-2]
+ index2 = indices[-1]
+ box1 = new_history[index1]
+ x1, y1, s1, r1 = box1
+ w1 = np.sqrt(s1 * r1)
+ h1 = np.sqrt(s1 / r1)
+ box2 = new_history[index2]
+ x2, y2, s2, r2 = box2
+ w2 = np.sqrt(s2 * r2)
+ h2 = np.sqrt(s2 / r2)
+ time_gap = index2 - index1
+ dx = (x2-x1)/time_gap
+ dy = (y2-y1)/time_gap
+ dw = (w2-w1)/time_gap
+ dh = (h2-h1)/time_gap
+ for i in range(index2 - index1):
+ """
+ The default virtual trajectory generation is by linear
+ motion (constant speed hypothesis), you could modify this
+ part to implement your own.
+ """
+ x = x1 + (i+1) * dx
+ y = y1 + (i+1) * dy
+ w = w1 + (i+1) * dw
+ h = h1 + (i+1) * dh
+ s = w * h
+ r = w / float(h)
+ new_box = np.array([x, y, s, r]).reshape((4, 1))
+ """
+ I still use predict-update loop here to refresh the parameters,
+ but this can be faster by directly modifying the internal parameters
+ as suggested in the paper. I keep this naive but slow way for
+ easy read and understanding
+ """
+ self.update(new_box)
+ if not i == (index2-index1-1):
+ self.predict()
+
+
+ def update(self, z, R=None, H=None):
+ """
+ Add a new measurement (z) to the Kalman filter.
+ If z is None, nothing is computed. However, x_post and P_post are
+ updated with the prior (x_prior, P_prior), and self.z is set to None.
+ Parameters
+ ----------
+ z : (dim_z, 1): array_like
+ measurement for this update. z can be a scalar if dim_z is 1,
+ otherwise it must be convertible to a column vector.
+ If you pass in a value of H, z must be a column vector the
+ of the correct size.
+ R : np.array, scalar, or None
+ Optionally provide R to override the measurement noise for this
+ one call, otherwise self.R will be used.
+ H : np.array, or None
+ Optionally provide H to override the measurement function for this
+ one call, otherwise self.H will be used.
+ """
+
+ # set to None to force recompute
+ self._log_likelihood = None
+ self._likelihood = None
+ self._mahalanobis = None
+
+ # append the observation
+ self.history_obs.append(z)
+
+ if z is None:
+ if self.observed:
+ """
+ Got no observation so freeze the current parameters for future
+ potential online smoothing.
+ """
+ self.freeze()
+ self.observed = False
+ self.z = np.array([[None]*self.dim_z]).T
+ self.x_post = self.x.copy()
+ self.P_post = self.P.copy()
+ self.y = zeros((self.dim_z, 1))
+ return
+
+ # self.observed = True
+ if not self.observed:
+ """
+ Get observation, use online smoothing to re-update parameters
+ """
+ self.unfreeze()
+ self.observed = True
+
+ if R is None:
+ R = self.R
+ elif isscalar(R):
+ R = eye(self.dim_z) * R
+
+ if H is None:
+ z = reshape_z(z, self.dim_z, self.x.ndim)
+ H = self.H
+
+ # y = z - Hx
+ # error (residual) between measurement and prediction
+ self.y = z - dot(H, self.x)
+
+ # common subexpression for speed
+ PHT = dot(self.P, H.T)
+
+ # S = HPH' + R
+ # project system uncertainty into measurement space
+ self.S = dot(H, PHT) + R
+ self.SI = self.inv(self.S)
+ # K = PH'inv(S)
+ # map system uncertainty into kalman gain
+ self.K = dot(PHT, self.SI)
+
+ # x = x + Ky
+ # predict new x with residual scaled by the kalman gain
+ self.x = self.x + dot(self.K, self.y)
+
+ # P = (I-KH)P(I-KH)' + KRK'
+ # This is more numerically stable
+ # and works for non-optimal K vs the equation
+ # P = (I-KH)P usually seen in the literature.
+
+ I_KH = self._I - dot(self.K, H)
+ self.P = dot(dot(I_KH, self.P), I_KH.T) + dot(dot(self.K, R), self.K.T)
+
+ # save measurement and posterior state
+ self.z = deepcopy(z)
+ self.x_post = self.x.copy()
+ self.P_post = self.P.copy()
+
+ def predict_steadystate(self, u=0, B=None):
+ """
+ Predict state (prior) using the Kalman filter state propagation
+ equations. Only x is updated, P is left unchanged. See
+ update_steadstate() for a longer explanation of when to use this
+ method.
+ Parameters
+ ----------
+ u : np.array
+ Optional control vector. If non-zero, it is multiplied by B
+ to create the control input into the system.
+ B : np.array(dim_x, dim_u), or None
+ Optional control transition matrix; a value of None
+ will cause the filter to use `self.B`.
+ """
+
+ if B is None:
+ B = self.B
+
+ # x = Fx + Bu
+ if B is not None:
+ self.x = dot(self.F, self.x) + dot(B, u)
+ else:
+ self.x = dot(self.F, self.x)
+
+ # save prior
+ self.x_prior = self.x.copy()
+ self.P_prior = self.P.copy()
+
+ def update_steadystate(self, z):
+ """
+ Add a new measurement (z) to the Kalman filter without recomputing
+ the Kalman gain K, the state covariance P, or the system
+ uncertainty S.
+ You can use this for LTI systems since the Kalman gain and covariance
+ converge to a fixed value. Precompute these and assign them explicitly,
+ or run the Kalman filter using the normal predict()/update(0 cycle
+ until they converge.
+ The main advantage of this call is speed. We do significantly less
+ computation, notably avoiding a costly matrix inversion.
+ Use in conjunction with predict_steadystate(), otherwise P will grow
+ without bound.
+ Parameters
+ ----------
+ z : (dim_z, 1): array_like
+ measurement for this update. z can be a scalar if dim_z is 1,
+ otherwise it must be convertible to a column vector.
+ Examples
+ --------
+ >>> cv = kinematic_kf(dim=3, order=2) # 3D const velocity filter
+ >>> # let filter converge on representative data, then save k and P
+ >>> for i in range(100):
+ >>> cv.predict()
+ >>> cv.update([i, i, i])
+ >>> saved_k = np.copy(cv.K)
+ >>> saved_P = np.copy(cv.P)
+ later on:
+ >>> cv = kinematic_kf(dim=3, order=2) # 3D const velocity filter
+ >>> cv.K = np.copy(saved_K)
+ >>> cv.P = np.copy(saved_P)
+ >>> for i in range(100):
+ >>> cv.predict_steadystate()
+ >>> cv.update_steadystate([i, i, i])
+ """
+
+ # set to None to force recompute
+ self._log_likelihood = None
+ self._likelihood = None
+ self._mahalanobis = None
+
+ if z is None:
+ self.z = np.array([[None]*self.dim_z]).T
+ self.x_post = self.x.copy()
+ self.P_post = self.P.copy()
+ self.y = zeros((self.dim_z, 1))
+ return
+
+ z = reshape_z(z, self.dim_z, self.x.ndim)
+
+ # y = z - Hx
+ # error (residual) between measurement and prediction
+ self.y = z - dot(self.H, self.x)
+
+ # x = x + Ky
+ # predict new x with residual scaled by the kalman gain
+ self.x = self.x + dot(self.K, self.y)
+
+ self.z = deepcopy(z)
+ self.x_post = self.x.copy()
+ self.P_post = self.P.copy()
+
+ # set to None to force recompute
+ self._log_likelihood = None
+ self._likelihood = None
+ self._mahalanobis = None
+
+ def update_correlated(self, z, R=None, H=None):
+ """ Add a new measurement (z) to the Kalman filter assuming that
+ process noise and measurement noise are correlated as defined in
+ the `self.M` matrix.
+ A partial derivation can be found in [1]
+ If z is None, nothing is changed.
+ Parameters
+ ----------
+ z : (dim_z, 1): array_like
+ measurement for this update. z can be a scalar if dim_z is 1,
+ otherwise it must be convertible to a column vector.
+ R : np.array, scalar, or None
+ Optionally provide R to override the measurement noise for this
+ one call, otherwise self.R will be used.
+ H : np.array, or None
+ Optionally provide H to override the measurement function for this
+ one call, otherwise self.H will be used.
+ References
+ ----------
+ .. [1] Bulut, Y. (2011). Applied Kalman filter theory (Doctoral dissertation, Northeastern University).
+ http://people.duke.edu/~hpgavin/SystemID/References/Balut-KalmanFilter-PhD-NEU-2011.pdf
+ """
+
+ # set to None to force recompute
+ self._log_likelihood = None
+ self._likelihood = None
+ self._mahalanobis = None
+
+ if z is None:
+ self.z = np.array([[None]*self.dim_z]).T
+ self.x_post = self.x.copy()
+ self.P_post = self.P.copy()
+ self.y = zeros((self.dim_z, 1))
+ return
+
+ if R is None:
+ R = self.R
+ elif isscalar(R):
+ R = eye(self.dim_z) * R
+
+ # rename for readability and a tiny extra bit of speed
+ if H is None:
+ z = reshape_z(z, self.dim_z, self.x.ndim)
+ H = self.H
+
+ # handle special case: if z is in form [[z]] but x is not a column
+ # vector dimensions will not match
+ if self.x.ndim == 1 and shape(z) == (1, 1):
+ z = z[0]
+
+ if shape(z) == (): # is it scalar, e.g. z=3 or z=np.array(3)
+ z = np.asarray([z])
+
+ # y = z - Hx
+ # error (residual) between measurement and prediction
+ self.y = z - dot(H, self.x)
+
+ # common subexpression for speed
+ PHT = dot(self.P, H.T)
+
+ # project system uncertainty into measurement space
+ self.S = dot(H, PHT) + dot(H, self.M) + dot(self.M.T, H.T) + R
+ self.SI = self.inv(self.S)
+
+ # K = PH'inv(S)
+ # map system uncertainty into kalman gain
+ self.K = dot(PHT + self.M, self.SI)
+
+ # x = x + Ky
+ # predict new x with residual scaled by the kalman gain
+ self.x = self.x + dot(self.K, self.y)
+ self.P = self.P - dot(self.K, dot(H, self.P) + self.M.T)
+
+ self.z = deepcopy(z)
+ self.x_post = self.x.copy()
+ self.P_post = self.P.copy()
+
+ def batch_filter(self, zs, Fs=None, Qs=None, Hs=None,
+ Rs=None, Bs=None, us=None, update_first=False,
+ saver=None):
+ """ Batch processes a sequences of measurements.
+ Parameters
+ ----------
+ zs : list-like
+ list of measurements at each time step `self.dt`. Missing
+ measurements must be represented by `None`.
+ Fs : None, list-like, default=None
+ optional value or list of values to use for the state transition
+ matrix F.
+ If Fs is None then self.F is used for all epochs.
+ Otherwise it must contain a list-like list of F's, one for
+ each epoch. This allows you to have varying F per epoch.
+ Qs : None, np.array or list-like, default=None
+ optional value or list of values to use for the process error
+ covariance Q.
+ If Qs is None then self.Q is used for all epochs.
+ Otherwise it must contain a list-like list of Q's, one for
+ each epoch. This allows you to have varying Q per epoch.
+ Hs : None, np.array or list-like, default=None
+ optional list of values to use for the measurement matrix H.
+ If Hs is None then self.H is used for all epochs.
+ If Hs contains a single matrix, then it is used as H for all
+ epochs.
+ Otherwise it must contain a list-like list of H's, one for
+ each epoch. This allows you to have varying H per epoch.
+ Rs : None, np.array or list-like, default=None
+ optional list of values to use for the measurement error
+ covariance R.
+ If Rs is None then self.R is used for all epochs.
+ Otherwise it must contain a list-like list of R's, one for
+ each epoch. This allows you to have varying R per epoch.
+ Bs : None, np.array or list-like, default=None
+ optional list of values to use for the control transition matrix B.
+ If Bs is None then self.B is used for all epochs.
+ Otherwise it must contain a list-like list of B's, one for
+ each epoch. This allows you to have varying B per epoch.
+ us : None, np.array or list-like, default=None
+ optional list of values to use for the control input vector;
+ If us is None then None is used for all epochs (equivalent to 0,
+ or no control input).
+ Otherwise it must contain a list-like list of u's, one for
+ each epoch.
+ update_first : bool, optional, default=False
+ controls whether the order of operations is update followed by
+ predict, or predict followed by update. Default is predict->update.
+ saver : filterpy.common.Saver, optional
+ filterpy.common.Saver object. If provided, saver.save() will be
+ called after every epoch
+ Returns
+ -------
+ means : np.array((n,dim_x,1))
+ array of the state for each time step after the update. Each entry
+ is an np.array. In other words `means[k,:]` is the state at step
+ `k`.
+ covariance : np.array((n,dim_x,dim_x))
+ array of the covariances for each time step after the update.
+ In other words `covariance[k,:,:]` is the covariance at step `k`.
+ means_predictions : np.array((n,dim_x,1))
+ array of the state for each time step after the predictions. Each
+ entry is an np.array. In other words `means[k,:]` is the state at
+ step `k`.
+ covariance_predictions : np.array((n,dim_x,dim_x))
+ array of the covariances for each time step after the prediction.
+ In other words `covariance[k,:,:]` is the covariance at step `k`.
+ Examples
+ --------
+ .. code-block:: Python
+ # this example demonstrates tracking a measurement where the time
+ # between measurement varies, as stored in dts. This requires
+ # that F be recomputed for each epoch. The output is then smoothed
+ # with an RTS smoother.
+ zs = [t + random.randn()*4 for t in range (40)]
+ Fs = [np.array([[1., dt], [0, 1]] for dt in dts]
+ (mu, cov, _, _) = kf.batch_filter(zs, Fs=Fs)
+ (xs, Ps, Ks, Pps) = kf.rts_smoother(mu, cov, Fs=Fs)
+ """
+
+ #pylint: disable=too-many-statements
+ n = np.size(zs, 0)
+ if Fs is None:
+ Fs = [self.F] * n
+ if Qs is None:
+ Qs = [self.Q] * n
+ if Hs is None:
+ Hs = [self.H] * n
+ if Rs is None:
+ Rs = [self.R] * n
+ if Bs is None:
+ Bs = [self.B] * n
+ if us is None:
+ us = [0] * n
+
+ # mean estimates from Kalman Filter
+ if self.x.ndim == 1:
+ means = zeros((n, self.dim_x))
+ means_p = zeros((n, self.dim_x))
+ else:
+ means = zeros((n, self.dim_x, 1))
+ means_p = zeros((n, self.dim_x, 1))
+
+ # state covariances from Kalman Filter
+ covariances = zeros((n, self.dim_x, self.dim_x))
+ covariances_p = zeros((n, self.dim_x, self.dim_x))
+
+ if update_first:
+ for i, (z, F, Q, H, R, B, u) in enumerate(zip(zs, Fs, Qs, Hs, Rs, Bs, us)):
+
+ self.update(z, R=R, H=H)
+ means[i, :] = self.x
+ covariances[i, :, :] = self.P
+
+ self.predict(u=u, B=B, F=F, Q=Q)
+ means_p[i, :] = self.x
+ covariances_p[i, :, :] = self.P
+
+ if saver is not None:
+ saver.save()
+ else:
+ for i, (z, F, Q, H, R, B, u) in enumerate(zip(zs, Fs, Qs, Hs, Rs, Bs, us)):
+
+ self.predict(u=u, B=B, F=F, Q=Q)
+ means_p[i, :] = self.x
+ covariances_p[i, :, :] = self.P
+
+ self.update(z, R=R, H=H)
+ means[i, :] = self.x
+ covariances[i, :, :] = self.P
+
+ if saver is not None:
+ saver.save()
+
+ return (means, covariances, means_p, covariances_p)
+
+ def rts_smoother(self, Xs, Ps, Fs=None, Qs=None, inv=np.linalg.inv):
+ """
+ Runs the Rauch-Tung-Striebel Kalman smoother on a set of
+ means and covariances computed by a Kalman filter. The usual input
+ would come from the output of `KalmanFilter.batch_filter()`.
+ Parameters
+ ----------
+ Xs : numpy.array
+ array of the means (state variable x) of the output of a Kalman
+ filter.
+ Ps : numpy.array
+ array of the covariances of the output of a kalman filter.
+ Fs : list-like collection of numpy.array, optional
+ State transition matrix of the Kalman filter at each time step.
+ Optional, if not provided the filter's self.F will be used
+ Qs : list-like collection of numpy.array, optional
+ Process noise of the Kalman filter at each time step. Optional,
+ if not provided the filter's self.Q will be used
+ inv : function, default numpy.linalg.inv
+ If you prefer another inverse function, such as the Moore-Penrose
+ pseudo inverse, set it to that instead: kf.inv = np.linalg.pinv
+ Returns
+ -------
+ x : numpy.ndarray
+ smoothed means
+ P : numpy.ndarray
+ smoothed state covariances
+ K : numpy.ndarray
+ smoother gain at each step
+ Pp : numpy.ndarray
+ Predicted state covariances
+ Examples
+ --------
+ .. code-block:: Python
+ zs = [t + random.randn()*4 for t in range (40)]
+ (mu, cov, _, _) = kalman.batch_filter(zs)
+ (x, P, K, Pp) = rts_smoother(mu, cov, kf.F, kf.Q)
+ """
+
+ if len(Xs) != len(Ps):
+ raise ValueError('length of Xs and Ps must be the same')
+
+ n = Xs.shape[0]
+ dim_x = Xs.shape[1]
+
+ if Fs is None:
+ Fs = [self.F] * n
+ if Qs is None:
+ Qs = [self.Q] * n
+
+ # smoother gain
+ K = zeros((n, dim_x, dim_x))
+
+ x, P, Pp = Xs.copy(), Ps.copy(), Ps.copy()
+ for k in range(n-2, -1, -1):
+ Pp[k] = dot(dot(Fs[k+1], P[k]), Fs[k+1].T) + Qs[k+1]
+
+ #pylint: disable=bad-whitespace
+ K[k] = dot(dot(P[k], Fs[k+1].T), inv(Pp[k]))
+ x[k] += dot(K[k], x[k+1] - dot(Fs[k+1], x[k]))
+ P[k] += dot(dot(K[k], P[k+1] - Pp[k]), K[k].T)
+
+ return (x, P, K, Pp)
+
+ def get_prediction(self, u=None, B=None, F=None, Q=None):
+ """
+ Predict next state (prior) using the Kalman filter state propagation
+ equations and returns it without modifying the object.
+ Parameters
+ ----------
+ u : np.array, default 0
+ Optional control vector.
+ B : np.array(dim_x, dim_u), or None
+ Optional control transition matrix; a value of None
+ will cause the filter to use `self.B`.
+ F : np.array(dim_x, dim_x), or None
+ Optional state transition matrix; a value of None
+ will cause the filter to use `self.F`.
+ Q : np.array(dim_x, dim_x), scalar, or None
+ Optional process noise matrix; a value of None will cause the
+ filter to use `self.Q`.
+ Returns
+ -------
+ (x, P) : tuple
+ State vector and covariance array of the prediction.
+ """
+
+ if B is None:
+ B = self.B
+ if F is None:
+ F = self.F
+ if Q is None:
+ Q = self.Q
+ elif isscalar(Q):
+ Q = eye(self.dim_x) * Q
+
+ # x = Fx + Bu
+ if B is not None and u is not None:
+ x = dot(F, self.x) + dot(B, u)
+ else:
+ x = dot(F, self.x)
+
+ # P = FPF' + Q
+ P = self._alpha_sq * dot(dot(F, self.P), F.T) + Q
+
+ return x, P
+
+ def get_update(self, z=None):
+ """
+ Computes the new estimate based on measurement `z` and returns it
+ without altering the state of the filter.
+ Parameters
+ ----------
+ z : (dim_z, 1): array_like
+ measurement for this update. z can be a scalar if dim_z is 1,
+ otherwise it must be convertible to a column vector.
+ Returns
+ -------
+ (x, P) : tuple
+ State vector and covariance array of the update.
+ """
+
+ if z is None:
+ return self.x, self.P
+ z = reshape_z(z, self.dim_z, self.x.ndim)
+
+ R = self.R
+ H = self.H
+ P = self.P
+ x = self.x
+
+ # error (residual) between measurement and prediction
+ y = z - dot(H, x)
+
+ # common subexpression for speed
+ PHT = dot(P, H.T)
+
+ # project system uncertainty into measurement space
+ S = dot(H, PHT) + R
+
+ # map system uncertainty into kalman gain
+ K = dot(PHT, self.inv(S))
+
+ # predict new x with residual scaled by the kalman gain
+ x = x + dot(K, y)
+
+ # P = (I-KH)P(I-KH)' + KRK'
+ I_KH = self._I - dot(K, H)
+ P = dot(dot(I_KH, P), I_KH.T) + dot(dot(K, R), K.T)
+
+ return x, P
+
+ def residual_of(self, z):
+ """
+ Returns the residual for the given measurement (z). Does not alter
+ the state of the filter.
+ """
+ z = reshape_z(z, self.dim_z, self.x.ndim)
+ return z - dot(self.H, self.x_prior)
+
+ def measurement_of_state(self, x):
+ """
+ Helper function that converts a state into a measurement.
+ Parameters
+ ----------
+ x : np.array
+ kalman state vector
+ Returns
+ -------
+ z : (dim_z, 1): array_like
+ measurement for this update. z can be a scalar if dim_z is 1,
+ otherwise it must be convertible to a column vector.
+ """
+
+ return dot(self.H, x)
+
+ @property
+ def log_likelihood(self):
+ """
+ log-likelihood of the last measurement.
+ """
+ if self._log_likelihood is None:
+ self._log_likelihood = logpdf(x=self.y, cov=self.S)
+ return self._log_likelihood
+
+ @property
+ def likelihood(self):
+ """
+ Computed from the log-likelihood. The log-likelihood can be very
+ small, meaning a large negative value such as -28000. Taking the
+ exp() of that results in 0.0, which can break typical algorithms
+ which multiply by this value, so by default we always return a
+ number >= sys.float_info.min.
+ """
+ if self._likelihood is None:
+ self._likelihood = exp(self.log_likelihood)
+ if self._likelihood == 0:
+ self._likelihood = sys.float_info.min
+ return self._likelihood
+
+ @property
+ def mahalanobis(self):
+ """"
+ Mahalanobis distance of measurement. E.g. 3 means measurement
+ was 3 standard deviations away from the predicted value.
+ Returns
+ -------
+ mahalanobis : float
+ """
+ if self._mahalanobis is None:
+ self._mahalanobis = sqrt(float(dot(dot(self.y.T, self.SI), self.y)))
+ return self._mahalanobis
+
+ @property
+ def alpha(self):
+ """
+ Fading memory setting. 1.0 gives the normal Kalman filter, and
+ values slightly larger than 1.0 (such as 1.02) give a fading
+ memory effect - previous measurements have less influence on the
+ filter's estimates. This formulation of the Fading memory filter
+ (there are many) is due to Dan Simon [1]_.
+ """
+ return self._alpha_sq**.5
+
+ def log_likelihood_of(self, z):
+ """
+ log likelihood of the measurement `z`. This should only be called
+ after a call to update(). Calling after predict() will yield an
+ incorrect result."""
+
+ if z is None:
+ return log(sys.float_info.min)
+ return logpdf(z, dot(self.H, self.x), self.S)
+
+ @alpha.setter
+ def alpha(self, value):
+ if not np.isscalar(value) or value < 1:
+ raise ValueError('alpha must be a float greater than 1')
+
+ self._alpha_sq = value**2
+
+ def __repr__(self):
+ return '\n'.join([
+ 'KalmanFilter object',
+ pretty_str('dim_x', self.dim_x),
+ pretty_str('dim_z', self.dim_z),
+ pretty_str('dim_u', self.dim_u),
+ pretty_str('x', self.x),
+ pretty_str('P', self.P),
+ pretty_str('x_prior', self.x_prior),
+ pretty_str('P_prior', self.P_prior),
+ pretty_str('x_post', self.x_post),
+ pretty_str('P_post', self.P_post),
+ pretty_str('F', self.F),
+ pretty_str('Q', self.Q),
+ pretty_str('R', self.R),
+ pretty_str('H', self.H),
+ pretty_str('K', self.K),
+ pretty_str('y', self.y),
+ pretty_str('S', self.S),
+ pretty_str('SI', self.SI),
+ pretty_str('M', self.M),
+ pretty_str('B', self.B),
+ pretty_str('z', self.z),
+ pretty_str('log-likelihood', self.log_likelihood),
+ pretty_str('likelihood', self.likelihood),
+ pretty_str('mahalanobis', self.mahalanobis),
+ pretty_str('alpha', self.alpha),
+ pretty_str('inv', self.inv)
+ ])
+
+ def test_matrix_dimensions(self, z=None, H=None, R=None, F=None, Q=None):
+ """
+ Performs a series of asserts to check that the size of everything
+ is what it should be. This can help you debug problems in your design.
+ If you pass in H, R, F, Q those will be used instead of this object's
+ value for those matrices.
+ Testing `z` (the measurement) is problamatic. x is a vector, and can be
+ implemented as either a 1D array or as a nx1 column vector. Thus Hx
+ can be of different shapes. Then, if Hx is a single value, it can
+ be either a 1D array or 2D vector. If either is true, z can reasonably
+ be a scalar (either '3' or np.array('3') are scalars under this
+ definition), a 1D, 1 element array, or a 2D, 1 element array. You are
+ allowed to pass in any combination that works.
+ """
+
+ if H is None:
+ H = self.H
+ if R is None:
+ R = self.R
+ if F is None:
+ F = self.F
+ if Q is None:
+ Q = self.Q
+ x = self.x
+ P = self.P
+
+ assert x.ndim == 1 or x.ndim == 2, \
+ "x must have one or two dimensions, but has {}".format(x.ndim)
+
+ if x.ndim == 1:
+ assert x.shape[0] == self.dim_x, \
+ "Shape of x must be ({},{}), but is {}".format(
+ self.dim_x, 1, x.shape)
+ else:
+ assert x.shape == (self.dim_x, 1), \
+ "Shape of x must be ({},{}), but is {}".format(
+ self.dim_x, 1, x.shape)
+
+ assert P.shape == (self.dim_x, self.dim_x), \
+ "Shape of P must be ({},{}), but is {}".format(
+ self.dim_x, self.dim_x, P.shape)
+
+ assert Q.shape == (self.dim_x, self.dim_x), \
+ "Shape of Q must be ({},{}), but is {}".format(
+ self.dim_x, self.dim_x, P.shape)
+
+ assert F.shape == (self.dim_x, self.dim_x), \
+ "Shape of F must be ({},{}), but is {}".format(
+ self.dim_x, self.dim_x, F.shape)
+
+ assert np.ndim(H) == 2, \
+ "Shape of H must be (dim_z, {}), but is {}".format(
+ P.shape[0], shape(H))
+
+ assert H.shape[1] == P.shape[0], \
+ "Shape of H must be (dim_z, {}), but is {}".format(
+ P.shape[0], H.shape)
+
+ # shape of R must be the same as HPH'
+ hph_shape = (H.shape[0], H.shape[0])
+ r_shape = shape(R)
+
+ if H.shape[0] == 1:
+ # r can be scalar, 1D, or 2D in this case
+ assert r_shape in [(), (1,), (1, 1)], \
+ "R must be scalar or one element array, but is shaped {}".format(
+ r_shape)
+ else:
+ assert r_shape == hph_shape, \
+ "shape of R should be {} but it is {}".format(hph_shape, r_shape)
+
+
+ if z is not None:
+ z_shape = shape(z)
+ else:
+ z_shape = (self.dim_z, 1)
+
+ # H@x must have shape of z
+ Hx = dot(H, x)
+
+ if z_shape == (): # scalar or np.array(scalar)
+ assert Hx.ndim == 1 or shape(Hx) == (1, 1), \
+ "shape of z should be {}, not {} for the given H".format(
+ shape(Hx), z_shape)
+
+ elif shape(Hx) == (1,):
+ assert z_shape[0] == 1, 'Shape of z must be {} for the given H'.format(shape(Hx))
+
+ else:
+ assert (z_shape == shape(Hx) or
+ (len(z_shape) == 1 and shape(Hx) == (z_shape[0], 1))), \
+ "shape of z should be {}, not {} for the given H".format(
+ shape(Hx), z_shape)
+
+ if np.ndim(Hx) > 1 and shape(Hx) != (1, 1):
+ assert shape(Hx) == z_shape, \
+ 'shape of z should be {} for the given H, but it is {}'.format(
+ shape(Hx), z_shape)
+
+
+def update(x, P, z, R, H=None, return_all=False):
+ """
+ Add a new measurement (z) to the Kalman filter. If z is None, nothing
+ is changed.
+ This can handle either the multidimensional or unidimensional case. If
+ all parameters are floats instead of arrays the filter will still work,
+ and return floats for x, P as the result.
+ update(1, 2, 1, 1, 1) # univariate
+ update(x, P, 1
+ Parameters
+ ----------
+ x : numpy.array(dim_x, 1), or float
+ State estimate vector
+ P : numpy.array(dim_x, dim_x), or float
+ Covariance matrix
+ z : (dim_z, 1): array_like
+ measurement for this update. z can be a scalar if dim_z is 1,
+ otherwise it must be convertible to a column vector.
+ R : numpy.array(dim_z, dim_z), or float
+ Measurement noise matrix
+ H : numpy.array(dim_x, dim_x), or float, optional
+ Measurement function. If not provided, a value of 1 is assumed.
+ return_all : bool, default False
+ If true, y, K, S, and log_likelihood are returned, otherwise
+ only x and P are returned.
+ Returns
+ -------
+ x : numpy.array
+ Posterior state estimate vector
+ P : numpy.array
+ Posterior covariance matrix
+ y : numpy.array or scalar
+ Residua. Difference between measurement and state in measurement space
+ K : numpy.array
+ Kalman gain
+ S : numpy.array
+ System uncertainty in measurement space
+ log_likelihood : float
+ log likelihood of the measurement
+ """
+
+ #pylint: disable=bare-except
+
+ if z is None:
+ if return_all:
+ return x, P, None, None, None, None
+ return x, P
+
+ if H is None:
+ H = np.array([1])
+
+ if np.isscalar(H):
+ H = np.array([H])
+
+ Hx = np.atleast_1d(dot(H, x))
+ z = reshape_z(z, Hx.shape[0], x.ndim)
+
+ # error (residual) between measurement and prediction
+ y = z - Hx
+
+ # project system uncertainty into measurement space
+ S = dot(dot(H, P), H.T) + R
+
+
+ # map system uncertainty into kalman gain
+ try:
+ K = dot(dot(P, H.T), linalg.inv(S))
+ except:
+ # can't invert a 1D array, annoyingly
+ K = dot(dot(P, H.T), 1./S)
+
+
+ # predict new x with residual scaled by the kalman gain
+ x = x + dot(K, y)
+
+ # P = (I-KH)P(I-KH)' + KRK'
+ KH = dot(K, H)
+
+ try:
+ I_KH = np.eye(KH.shape[0]) - KH
+ except:
+ I_KH = np.array([1 - KH])
+ P = dot(dot(I_KH, P), I_KH.T) + dot(dot(K, R), K.T)
+
+
+ if return_all:
+ # compute log likelihood
+ log_likelihood = logpdf(z, dot(H, x), S)
+ return x, P, y, K, S, log_likelihood
+ return x, P
+
+
+def update_steadystate(x, z, K, H=None):
+ """
+ Add a new measurement (z) to the Kalman filter. If z is None, nothing
+ is changed.
+ Parameters
+ ----------
+ x : numpy.array(dim_x, 1), or float
+ State estimate vector
+ z : (dim_z, 1): array_like
+ measurement for this update. z can be a scalar if dim_z is 1,
+ otherwise it must be convertible to a column vector.
+ K : numpy.array, or float
+ Kalman gain matrix
+ H : numpy.array(dim_x, dim_x), or float, optional
+ Measurement function. If not provided, a value of 1 is assumed.
+ Returns
+ -------
+ x : numpy.array
+ Posterior state estimate vector
+ Examples
+ --------
+ This can handle either the multidimensional or unidimensional case. If
+ all parameters are floats instead of arrays the filter will still work,
+ and return floats for x, P as the result.
+ >>> update_steadystate(1, 2, 1) # univariate
+ >>> update_steadystate(x, P, z, H)
+ """
+
+
+ if z is None:
+ return x
+
+ if H is None:
+ H = np.array([1])
+
+ if np.isscalar(H):
+ H = np.array([H])
+
+ Hx = np.atleast_1d(dot(H, x))
+ z = reshape_z(z, Hx.shape[0], x.ndim)
+
+ # error (residual) between measurement and prediction
+ y = z - Hx
+
+ # estimate new x with residual scaled by the kalman gain
+ return x + dot(K, y)
+
+
+def predict(x, P, F=1, Q=0, u=0, B=1, alpha=1.):
+ """
+ Predict next state (prior) using the Kalman filter state propagation
+ equations.
+ Parameters
+ ----------
+ x : numpy.array
+ State estimate vector
+ P : numpy.array
+ Covariance matrix
+ F : numpy.array()
+ State Transition matrix
+ Q : numpy.array, Optional
+ Process noise matrix
+ u : numpy.array, Optional, default 0.
+ Control vector. If non-zero, it is multiplied by B
+ to create the control input into the system.
+ B : numpy.array, optional, default 0.
+ Control transition matrix.
+ alpha : float, Optional, default=1.0
+ Fading memory setting. 1.0 gives the normal Kalman filter, and
+ values slightly larger than 1.0 (such as 1.02) give a fading
+ memory effect - previous measurements have less influence on the
+ filter's estimates. This formulation of the Fading memory filter
+ (there are many) is due to Dan Simon
+ Returns
+ -------
+ x : numpy.array
+ Prior state estimate vector
+ P : numpy.array
+ Prior covariance matrix
+ """
+
+ if np.isscalar(F):
+ F = np.array(F)
+ x = dot(F, x) + dot(B, u)
+ P = (alpha * alpha) * dot(dot(F, P), F.T) + Q
+
+ return x, P
+
+
+def predict_steadystate(x, F=1, u=0, B=1):
+ """
+ Predict next state (prior) using the Kalman filter state propagation
+ equations. This steady state form only computes x, assuming that the
+ covariance is constant.
+ Parameters
+ ----------
+ x : numpy.array
+ State estimate vector
+ P : numpy.array
+ Covariance matrix
+ F : numpy.array()
+ State Transition matrix
+ u : numpy.array, Optional, default 0.
+ Control vector. If non-zero, it is multiplied by B
+ to create the control input into the system.
+ B : numpy.array, optional, default 0.
+ Control transition matrix.
+ Returns
+ -------
+ x : numpy.array
+ Prior state estimate vector
+ """
+
+ if np.isscalar(F):
+ F = np.array(F)
+ x = dot(F, x) + dot(B, u)
+
+ return x
+
+
+
+def batch_filter(x, P, zs, Fs, Qs, Hs, Rs, Bs=None, us=None,
+ update_first=False, saver=None):
+ """
+ Batch processes a sequences of measurements.
+ Parameters
+ ----------
+ zs : list-like
+ list of measurements at each time step. Missing measurements must be
+ represented by None.
+ Fs : list-like
+ list of values to use for the state transition matrix matrix.
+ Qs : list-like
+ list of values to use for the process error
+ covariance.
+ Hs : list-like
+ list of values to use for the measurement matrix.
+ Rs : list-like
+ list of values to use for the measurement error
+ covariance.
+ Bs : list-like, optional
+ list of values to use for the control transition matrix;
+ a value of None in any position will cause the filter
+ to use `self.B` for that time step.
+ us : list-like, optional
+ list of values to use for the control input vector;
+ a value of None in any position will cause the filter to use
+ 0 for that time step.
+ update_first : bool, optional
+ controls whether the order of operations is update followed by
+ predict, or predict followed by update. Default is predict->update.
+ saver : filterpy.common.Saver, optional
+ filterpy.common.Saver object. If provided, saver.save() will be
+ called after every epoch
+ Returns
+ -------
+ means : np.array((n,dim_x,1))
+ array of the state for each time step after the update. Each entry
+ is an np.array. In other words `means[k,:]` is the state at step
+ `k`.
+ covariance : np.array((n,dim_x,dim_x))
+ array of the covariances for each time step after the update.
+ In other words `covariance[k,:,:]` is the covariance at step `k`.
+ means_predictions : np.array((n,dim_x,1))
+ array of the state for each time step after the predictions. Each
+ entry is an np.array. In other words `means[k,:]` is the state at
+ step `k`.
+ covariance_predictions : np.array((n,dim_x,dim_x))
+ array of the covariances for each time step after the prediction.
+ In other words `covariance[k,:,:]` is the covariance at step `k`.
+ Examples
+ --------
+ .. code-block:: Python
+ zs = [t + random.randn()*4 for t in range (40)]
+ Fs = [kf.F for t in range (40)]
+ Hs = [kf.H for t in range (40)]
+ (mu, cov, _, _) = kf.batch_filter(zs, Rs=R_list, Fs=Fs, Hs=Hs, Qs=None,
+ Bs=None, us=None, update_first=False)
+ (xs, Ps, Ks, Pps) = kf.rts_smoother(mu, cov, Fs=Fs, Qs=None)
+ """
+
+ n = np.size(zs, 0)
+ dim_x = x.shape[0]
+
+ # mean estimates from Kalman Filter
+ if x.ndim == 1:
+ means = zeros((n, dim_x))
+ means_p = zeros((n, dim_x))
+ else:
+ means = zeros((n, dim_x, 1))
+ means_p = zeros((n, dim_x, 1))
+
+ # state covariances from Kalman Filter
+ covariances = zeros((n, dim_x, dim_x))
+ covariances_p = zeros((n, dim_x, dim_x))
+
+ if us is None:
+ us = [0.] * n
+ Bs = [0.] * n
+
+ if update_first:
+ for i, (z, F, Q, H, R, B, u) in enumerate(zip(zs, Fs, Qs, Hs, Rs, Bs, us)):
+
+ x, P = update(x, P, z, R=R, H=H)
+ means[i, :] = x
+ covariances[i, :, :] = P
+
+ x, P = predict(x, P, u=u, B=B, F=F, Q=Q)
+ means_p[i, :] = x
+ covariances_p[i, :, :] = P
+ if saver is not None:
+ saver.save()
+ else:
+ for i, (z, F, Q, H, R, B, u) in enumerate(zip(zs, Fs, Qs, Hs, Rs, Bs, us)):
+
+ x, P = predict(x, P, u=u, B=B, F=F, Q=Q)
+ means_p[i, :] = x
+ covariances_p[i, :, :] = P
+
+ x, P = update(x, P, z, R=R, H=H)
+ means[i, :] = x
+ covariances[i, :, :] = P
+ if saver is not None:
+ saver.save()
+
+ return (means, covariances, means_p, covariances_p)
+
+
+
+def rts_smoother(Xs, Ps, Fs, Qs):
+ """
+ Runs the Rauch-Tung-Striebel Kalman smoother on a set of
+ means and covariances computed by a Kalman filter. The usual input
+ would come from the output of `KalmanFilter.batch_filter()`.
+ Parameters
+ ----------
+ Xs : numpy.array
+ array of the means (state variable x) of the output of a Kalman
+ filter.
+ Ps : numpy.array
+ array of the covariances of the output of a kalman filter.
+ Fs : list-like collection of numpy.array
+ State transition matrix of the Kalman filter at each time step.
+ Qs : list-like collection of numpy.array, optional
+ Process noise of the Kalman filter at each time step.
+ Returns
+ -------
+ x : numpy.ndarray
+ smoothed means
+ P : numpy.ndarray
+ smoothed state covariances
+ K : numpy.ndarray
+ smoother gain at each step
+ pP : numpy.ndarray
+ predicted state covariances
+ Examples
+ --------
+ .. code-block:: Python
+ zs = [t + random.randn()*4 for t in range (40)]
+ (mu, cov, _, _) = kalman.batch_filter(zs)
+ (x, P, K, pP) = rts_smoother(mu, cov, kf.F, kf.Q)
+ """
+
+ if len(Xs) != len(Ps):
+ raise ValueError('length of Xs and Ps must be the same')
+
+ n = Xs.shape[0]
+ dim_x = Xs.shape[1]
+
+ # smoother gain
+ K = zeros((n, dim_x, dim_x))
+ x, P, pP = Xs.copy(), Ps.copy(), Ps.copy()
+
+ for k in range(n-2, -1, -1):
+ pP[k] = dot(dot(Fs[k], P[k]), Fs[k].T) + Qs[k]
+
+ #pylint: disable=bad-whitespace
+ K[k] = dot(dot(P[k], Fs[k].T), linalg.inv(pP[k]))
+ x[k] += dot(K[k], x[k+1] - dot(Fs[k], x[k]))
+ P[k] += dot(dot(K[k], P[k+1] - pP[k]), K[k].T)
+
+ return (x, P, K, pP)
\ No newline at end of file
diff --git a/trackers/ocsort/ocsort.py b/trackers/ocsort/ocsort.py
new file mode 100644
index 0000000000000000000000000000000000000000..11ea1fc60c1254ae6b58fd30027a5a2c4872c548
--- /dev/null
+++ b/trackers/ocsort/ocsort.py
@@ -0,0 +1,327 @@
+"""
+ This script is adopted from the SORT script by Alex Bewley alex@bewley.ai
+"""
+from __future__ import print_function
+
+import numpy as np
+from .association import *
+from yolov5.utils.general import xywh2xyxy
+
+
+def k_previous_obs(observations, cur_age, k):
+ if len(observations) == 0:
+ return [-1, -1, -1, -1, -1]
+ for i in range(k):
+ dt = k - i
+ if cur_age - dt in observations:
+ return observations[cur_age-dt]
+ max_age = max(observations.keys())
+ return observations[max_age]
+
+
+def convert_bbox_to_z(bbox):
+ """
+ Takes a bounding box in the form [x1,y1,x2,y2] and returns z in the form
+ [x,y,s,r] where x,y is the centre of the box and s is the scale/area and r is
+ the aspect ratio
+ """
+ w = bbox[2] - bbox[0]
+ h = bbox[3] - bbox[1]
+ x = bbox[0] + w/2.
+ y = bbox[1] + h/2.
+ s = w * h # scale is just area
+ r = w / float(h+1e-6)
+ return np.array([x, y, s, r]).reshape((4, 1))
+
+
+def convert_x_to_bbox(x, score=None):
+ """
+ Takes a bounding box in the centre form [x,y,s,r] and returns it in the form
+ [x1,y1,x2,y2] where x1,y1 is the top left and x2,y2 is the bottom right
+ """
+ w = np.sqrt(x[2] * x[3])
+ h = x[2] / w
+ if(score == None):
+ return np.array([x[0]-w/2., x[1]-h/2., x[0]+w/2., x[1]+h/2.]).reshape((1, 4))
+ else:
+ return np.array([x[0]-w/2., x[1]-h/2., x[0]+w/2., x[1]+h/2., score]).reshape((1, 5))
+
+
+def speed_direction(bbox1, bbox2):
+ cx1, cy1 = (bbox1[0]+bbox1[2]) / 2.0, (bbox1[1]+bbox1[3])/2.0
+ cx2, cy2 = (bbox2[0]+bbox2[2]) / 2.0, (bbox2[1]+bbox2[3])/2.0
+ speed = np.array([cy2-cy1, cx2-cx1])
+ norm = np.sqrt((cy2-cy1)**2 + (cx2-cx1)**2) + 1e-6
+ return speed / norm
+
+
+class KalmanBoxTracker(object):
+ """
+ This class represents the internal state of individual tracked objects observed as bbox.
+ """
+ count = 0
+
+ def __init__(self, bbox, cls, delta_t=3, orig=False):
+ """
+ Initialises a tracker using initial bounding box.
+
+ """
+ # define constant velocity model
+ if not orig:
+ from .kalmanfilter import KalmanFilterNew as KalmanFilter
+ self.kf = KalmanFilter(dim_x=7, dim_z=4)
+ else:
+ from filterpy.kalman import KalmanFilter
+ self.kf = KalmanFilter(dim_x=7, dim_z=4)
+ self.kf.F = np.array([[1, 0, 0, 0, 1, 0, 0], [0, 1, 0, 0, 0, 1, 0], [0, 0, 1, 0, 0, 0, 1], [
+ 0, 0, 0, 1, 0, 0, 0], [0, 0, 0, 0, 1, 0, 0], [0, 0, 0, 0, 0, 1, 0], [0, 0, 0, 0, 0, 0, 1]])
+ self.kf.H = np.array([[1, 0, 0, 0, 0, 0, 0], [0, 1, 0, 0, 0, 0, 0],
+ [0, 0, 1, 0, 0, 0, 0], [0, 0, 0, 1, 0, 0, 0]])
+
+ self.kf.R[2:, 2:] *= 10.
+ self.kf.P[4:, 4:] *= 1000. # give high uncertainty to the unobservable initial velocities
+ self.kf.P *= 10.
+ self.kf.Q[-1, -1] *= 0.01
+ self.kf.Q[4:, 4:] *= 0.01
+
+ self.kf.x[:4] = convert_bbox_to_z(bbox)
+ self.time_since_update = 0
+ self.id = KalmanBoxTracker.count
+ KalmanBoxTracker.count += 1
+ self.history = []
+ self.hits = 0
+ self.hit_streak = 0
+ self.age = 0
+ #self.conf = conf
+ self.cls = cls
+ """
+ NOTE: [-1,-1,-1,-1,-1] is a compromising placeholder for non-observation status, the same for the return of
+ function k_previous_obs. It is ugly and I do not like it. But to support generate observation array in a
+ fast and unified way, which you would see below k_observations = np.array([k_previous_obs(...]]), let's bear it for now.
+ """
+ self.last_observation = np.array([-1, -1, -1, -1, -1]) # placeholder
+ self.observations = dict()
+ self.history_observations = []
+ self.velocity = None
+ self.delta_t = delta_t
+
+ def update(self, bbox, cls):
+ """
+ Updates the state vector with observed bbox.
+ """
+
+ if bbox is not None:
+ self.cls = cls
+ if self.last_observation.sum() >= 0: # no previous observation
+ previous_box = None
+ for i in range(self.delta_t):
+ dt = self.delta_t - i
+ if self.age - dt in self.observations:
+ previous_box = self.observations[self.age-dt]
+ break
+ if previous_box is None:
+ previous_box = self.last_observation
+ """
+ Estimate the track speed direction with observations \Delta t steps away
+ """
+ self.velocity = speed_direction(previous_box, bbox)
+
+ """
+ Insert new observations. This is a ugly way to maintain both self.observations
+ and self.history_observations. Bear it for the moment.
+ """
+ self.last_observation = bbox
+ self.observations[self.age] = bbox
+ self.history_observations.append(bbox)
+
+ self.time_since_update = 0
+ self.history = []
+ self.hits += 1
+ self.hit_streak += 1
+ self.kf.update(convert_bbox_to_z(bbox))
+ else:
+ self.kf.update(bbox)
+
+ def predict(self):
+ """
+ Advances the state vector and returns the predicted bounding box estimate.
+ """
+ if((self.kf.x[6]+self.kf.x[2]) <= 0):
+ self.kf.x[6] *= 0.0
+
+ self.kf.predict()
+ self.age += 1
+ if(self.time_since_update > 0):
+ self.hit_streak = 0
+ self.time_since_update += 1
+ self.history.append(convert_x_to_bbox(self.kf.x))
+ return self.history[-1]
+
+ def get_state(self):
+ """
+ Returns the current bounding box estimate.
+ """
+ return convert_x_to_bbox(self.kf.x)
+
+
+"""
+ We support multiple ways for association cost calculation, by default
+ we use IoU. GIoU may have better performance in some situations. We note
+ that we hardly normalize the cost by all methods to (0,1) which may not be
+ the best practice.
+"""
+ASSO_FUNCS = { "iou": iou_batch,
+ "giou": giou_batch,
+ "ciou": ciou_batch,
+ "diou": diou_batch,
+ "ct_dist": ct_dist}
+
+
+class OCSort(object):
+ def __init__(self, det_thresh, max_age=30, min_hits=3,
+ iou_threshold=0.3, delta_t=3, asso_func="iou", inertia=0.2, use_byte=False):
+ """
+ Sets key parameters for SORT
+ """
+ self.max_age = max_age
+ self.min_hits = min_hits
+ self.iou_threshold = iou_threshold
+ self.trackers = []
+ self.frame_count = 0
+ self.det_thresh = det_thresh
+ self.delta_t = delta_t
+ self.asso_func = ASSO_FUNCS[asso_func]
+ self.inertia = inertia
+ self.use_byte = use_byte
+ KalmanBoxTracker.count = 0
+
+ def update(self, dets, _):
+ """
+ Params:
+ dets - a numpy array of detections in the format [[x1,y1,x2,y2,score],[x1,y1,x2,y2,score],...]
+ Requires: this method must be called once for each frame even with empty detections (use np.empty((0, 5)) for frames without detections).
+ Returns the a similar array, where the last column is the object ID.
+ NOTE: The number of objects returned may differ from the number of detections provided.
+ """
+
+ self.frame_count += 1
+
+ xyxys = dets[:, 0:4]
+ confs = dets[:, 4]
+ clss = dets[:, 5]
+
+ classes = clss.numpy()
+ xyxys = xyxys.numpy()
+ confs = confs.numpy()
+
+ output_results = np.column_stack((xyxys, confs, classes))
+
+ inds_low = confs > 0.1
+ inds_high = confs < self.det_thresh
+ inds_second = np.logical_and(inds_low, inds_high) # self.det_thresh > score > 0.1, for second matching
+ dets_second = output_results[inds_second] # detections for second matching
+ remain_inds = confs > self.det_thresh
+ dets = output_results[remain_inds]
+
+ # get predicted locations from existing trackers.
+ trks = np.zeros((len(self.trackers), 5))
+ to_del = []
+ ret = []
+ for t, trk in enumerate(trks):
+ pos = self.trackers[t].predict()[0]
+ trk[:] = [pos[0], pos[1], pos[2], pos[3], 0]
+ if np.any(np.isnan(pos)):
+ to_del.append(t)
+ trks = np.ma.compress_rows(np.ma.masked_invalid(trks))
+ for t in reversed(to_del):
+ self.trackers.pop(t)
+
+ velocities = np.array(
+ [trk.velocity if trk.velocity is not None else np.array((0, 0)) for trk in self.trackers])
+ last_boxes = np.array([trk.last_observation for trk in self.trackers])
+ k_observations = np.array(
+ [k_previous_obs(trk.observations, trk.age, self.delta_t) for trk in self.trackers])
+
+ """
+ First round of association
+ """
+ matched, unmatched_dets, unmatched_trks = associate(
+ dets, trks, self.iou_threshold, velocities, k_observations, self.inertia)
+ for m in matched:
+ self.trackers[m[1]].update(dets[m[0], :5], dets[m[0], 5])
+
+ """
+ Second round of associaton by OCR
+ """
+ # BYTE association
+ if self.use_byte and len(dets_second) > 0 and unmatched_trks.shape[0] > 0:
+ u_trks = trks[unmatched_trks]
+ iou_left = self.asso_func(dets_second, u_trks) # iou between low score detections and unmatched tracks
+ iou_left = np.array(iou_left)
+ if iou_left.max() > self.iou_threshold:
+ """
+ NOTE: by using a lower threshold, e.g., self.iou_threshold - 0.1, you may
+ get a higher performance especially on MOT17/MOT20 datasets. But we keep it
+ uniform here for simplicity
+ """
+ matched_indices = linear_assignment(-iou_left)
+ to_remove_trk_indices = []
+ for m in matched_indices:
+ det_ind, trk_ind = m[0], unmatched_trks[m[1]]
+ if iou_left[m[0], m[1]] < self.iou_threshold:
+ continue
+ self.trackers[trk_ind].update(dets_second[det_ind, :5], dets_second[det_ind, 5])
+ to_remove_trk_indices.append(trk_ind)
+ unmatched_trks = np.setdiff1d(unmatched_trks, np.array(to_remove_trk_indices))
+
+ if unmatched_dets.shape[0] > 0 and unmatched_trks.shape[0] > 0:
+ left_dets = dets[unmatched_dets]
+ left_trks = last_boxes[unmatched_trks]
+ iou_left = self.asso_func(left_dets, left_trks)
+ iou_left = np.array(iou_left)
+ if iou_left.max() > self.iou_threshold:
+ """
+ NOTE: by using a lower threshold, e.g., self.iou_threshold - 0.1, you may
+ get a higher performance especially on MOT17/MOT20 datasets. But we keep it
+ uniform here for simplicity
+ """
+ rematched_indices = linear_assignment(-iou_left)
+ to_remove_det_indices = []
+ to_remove_trk_indices = []
+ for m in rematched_indices:
+ det_ind, trk_ind = unmatched_dets[m[0]], unmatched_trks[m[1]]
+ if iou_left[m[0], m[1]] < self.iou_threshold:
+ continue
+ self.trackers[trk_ind].update(dets[det_ind, :5], dets[det_ind, 5])
+ to_remove_det_indices.append(det_ind)
+ to_remove_trk_indices.append(trk_ind)
+ unmatched_dets = np.setdiff1d(unmatched_dets, np.array(to_remove_det_indices))
+ unmatched_trks = np.setdiff1d(unmatched_trks, np.array(to_remove_trk_indices))
+
+ for m in unmatched_trks:
+ self.trackers[m].update(None, None)
+
+ # create and initialise new trackers for unmatched detections
+ for i in unmatched_dets:
+ trk = KalmanBoxTracker(dets[i, :5], dets[i, 5], delta_t=self.delta_t)
+ self.trackers.append(trk)
+ i = len(self.trackers)
+ for trk in reversed(self.trackers):
+ if trk.last_observation.sum() < 0:
+ d = trk.get_state()[0]
+ else:
+ """
+ this is optional to use the recent observation or the kalman filter prediction,
+ we didn't notice significant difference here
+ """
+ d = trk.last_observation[:4]
+ if (trk.time_since_update < 1) and (trk.hit_streak >= self.min_hits or self.frame_count <= self.min_hits):
+ # +1 as MOT benchmark requires positive
+ ret.append(np.concatenate((d, [trk.id+1], [trk.cls])).reshape(1, -1))
+ i -= 1
+ # remove dead tracklet
+ if(trk.time_since_update > self.max_age):
+ self.trackers.pop(i)
+ if(len(ret) > 0):
+ return np.concatenate(ret)
+ return np.empty((0, 5))
diff --git a/trackers/strong_sort/.gitignore b/trackers/strong_sort/.gitignore
new file mode 100644
index 0000000000000000000000000000000000000000..37ed2f4dc4a1ca945a0d807274bfe2f6cc7e2fec
--- /dev/null
+++ b/trackers/strong_sort/.gitignore
@@ -0,0 +1,13 @@
+# Folders
+__pycache__/
+build/
+*.egg-info
+
+
+# Files
+*.weights
+*.t7
+*.mp4
+*.avi
+*.so
+*.txt
diff --git a/trackers/strong_sort/__init__.py b/trackers/strong_sort/__init__.py
new file mode 100644
index 0000000000000000000000000000000000000000..e69de29bb2d1d6434b8b29ae775ad8c2e48c5391
diff --git a/trackers/strong_sort/__pycache__/__init__.cpython-38.pyc b/trackers/strong_sort/__pycache__/__init__.cpython-38.pyc
new file mode 100644
index 0000000000000000000000000000000000000000..de436e35f3cc799d768dec33235069ec2aac2884
Binary files /dev/null and b/trackers/strong_sort/__pycache__/__init__.cpython-38.pyc differ
diff --git a/trackers/strong_sort/__pycache__/__init__.cpython-39.pyc b/trackers/strong_sort/__pycache__/__init__.cpython-39.pyc
new file mode 100644
index 0000000000000000000000000000000000000000..6b93fffedff6b5dc91e154ef97e24b3c2b0e4873
Binary files /dev/null and b/trackers/strong_sort/__pycache__/__init__.cpython-39.pyc differ
diff --git a/trackers/strong_sort/__pycache__/reid_multibackend.cpython-38.pyc b/trackers/strong_sort/__pycache__/reid_multibackend.cpython-38.pyc
new file mode 100644
index 0000000000000000000000000000000000000000..910287d0b582401b5ed1535fb97ae9e09aa1d802
Binary files /dev/null and b/trackers/strong_sort/__pycache__/reid_multibackend.cpython-38.pyc differ
diff --git a/trackers/strong_sort/__pycache__/reid_multibackend.cpython-39.pyc b/trackers/strong_sort/__pycache__/reid_multibackend.cpython-39.pyc
new file mode 100644
index 0000000000000000000000000000000000000000..0c0cb8ca141854c794db95178b667d7af65a1d77
Binary files /dev/null and b/trackers/strong_sort/__pycache__/reid_multibackend.cpython-39.pyc differ
diff --git a/trackers/strong_sort/__pycache__/strong_sort.cpython-38.pyc b/trackers/strong_sort/__pycache__/strong_sort.cpython-38.pyc
new file mode 100644
index 0000000000000000000000000000000000000000..51a4a5f2064a1cd205de602406e178cd00027bb6
Binary files /dev/null and b/trackers/strong_sort/__pycache__/strong_sort.cpython-38.pyc differ
diff --git a/trackers/strong_sort/__pycache__/strong_sort.cpython-39.pyc b/trackers/strong_sort/__pycache__/strong_sort.cpython-39.pyc
new file mode 100644
index 0000000000000000000000000000000000000000..326b27081fae5983bcd5b479393c8b63ff3bd71e
Binary files /dev/null and b/trackers/strong_sort/__pycache__/strong_sort.cpython-39.pyc differ
diff --git a/trackers/strong_sort/configs/strong_sort.yaml b/trackers/strong_sort/configs/strong_sort.yaml
new file mode 100644
index 0000000000000000000000000000000000000000..8f36793254bef8396e25a7a9d099be4eeb20fde2
--- /dev/null
+++ b/trackers/strong_sort/configs/strong_sort.yaml
@@ -0,0 +1,10 @@
+STRONGSORT:
+ ECC: True # activate camera motion compensation
+ MC_LAMBDA: 0.995 # matching with both appearance (1 - MC_LAMBDA) and motion cost
+ EMA_ALPHA: 0.9 # updates appearance state in an exponential moving average manner
+ MAX_DIST: 0.2 # The matching threshold. Samples with larger distance are considered an invalid match
+ MAX_IOU_DISTANCE: 0.7 # Gating threshold. Associations with cost larger than this value are disregarded.
+ MAX_AGE: 30 # Maximum number of missed misses before a track is deleted
+ N_INIT: 3 # Number of frames that a track remains in initialization phase
+ NN_BUDGET: 100 # Maximum size of the appearance descriptors gallery
+
diff --git a/trackers/strong_sort/deep/__pycache__/reid_model_factory.cpython-38.pyc b/trackers/strong_sort/deep/__pycache__/reid_model_factory.cpython-38.pyc
new file mode 100644
index 0000000000000000000000000000000000000000..220a40f0d931bd1e165efe1ff1a63bbb0068f696
Binary files /dev/null and b/trackers/strong_sort/deep/__pycache__/reid_model_factory.cpython-38.pyc differ
diff --git a/trackers/strong_sort/deep/__pycache__/reid_model_factory.cpython-39.pyc b/trackers/strong_sort/deep/__pycache__/reid_model_factory.cpython-39.pyc
new file mode 100644
index 0000000000000000000000000000000000000000..8602f7df559a3c4766227c0cfecc56ef2634c057
Binary files /dev/null and b/trackers/strong_sort/deep/__pycache__/reid_model_factory.cpython-39.pyc differ
diff --git a/trackers/strong_sort/deep/checkpoint/.gitkeep b/trackers/strong_sort/deep/checkpoint/.gitkeep
new file mode 100644
index 0000000000000000000000000000000000000000..e69de29bb2d1d6434b8b29ae775ad8c2e48c5391
diff --git a/trackers/strong_sort/deep/checkpoint/osnet_x0_25_market1501.pth b/trackers/strong_sort/deep/checkpoint/osnet_x0_25_market1501.pth
new file mode 100644
index 0000000000000000000000000000000000000000..96a5eaab697ac1ab680bd22489e6382aa98c9abf
--- /dev/null
+++ b/trackers/strong_sort/deep/checkpoint/osnet_x0_25_market1501.pth
@@ -0,0 +1,3 @@
+version https://git-lfs.github.com/spec/v1
+oid sha256:c0ff09177a21417a19bc73bbf5af4eb5d2b097c2074ed35e67819ee6cd93612c
+size 2462783
diff --git a/trackers/strong_sort/deep/checkpoint/osnet_x0_25_msmt17.pth b/trackers/strong_sort/deep/checkpoint/osnet_x0_25_msmt17.pth
new file mode 100644
index 0000000000000000000000000000000000000000..4b839e7f4d067187a884fadf586af23e56b054d9
--- /dev/null
+++ b/trackers/strong_sort/deep/checkpoint/osnet_x0_25_msmt17.pth
@@ -0,0 +1,3 @@
+version https://git-lfs.github.com/spec/v1
+oid sha256:6f57607fed9f502b9efed546108132ee715df5a5b6e6932c6269bacb47f59f99
+size 3057863
diff --git a/trackers/strong_sort/deep/checkpoint/osnet_x1_0_msmt17.pth b/trackers/strong_sort/deep/checkpoint/osnet_x1_0_msmt17.pth
new file mode 100644
index 0000000000000000000000000000000000000000..30fd65885298f53ddf5f42cfef052ac988a648f8
--- /dev/null
+++ b/trackers/strong_sort/deep/checkpoint/osnet_x1_0_msmt17.pth
@@ -0,0 +1,3 @@
+version https://git-lfs.github.com/spec/v1
+oid sha256:b7d73dc67c016fd044e4027ff856019496392a7aca8fa0ed56d862a1632c1cf2
+size 10994685
diff --git a/trackers/strong_sort/deep/models/__init__.py b/trackers/strong_sort/deep/models/__init__.py
new file mode 100644
index 0000000000000000000000000000000000000000..3c60ba6f59ca7fa5ff9f3c6a4dcef1357c353dde
--- /dev/null
+++ b/trackers/strong_sort/deep/models/__init__.py
@@ -0,0 +1,122 @@
+from __future__ import absolute_import
+import torch
+
+from .pcb import *
+from .mlfn import *
+from .hacnn import *
+from .osnet import *
+from .senet import *
+from .mudeep import *
+from .nasnet import *
+from .resnet import *
+from .densenet import *
+from .xception import *
+from .osnet_ain import *
+from .resnetmid import *
+from .shufflenet import *
+from .squeezenet import *
+from .inceptionv4 import *
+from .mobilenetv2 import *
+from .resnet_ibn_a import *
+from .resnet_ibn_b import *
+from .shufflenetv2 import *
+from .inceptionresnetv2 import *
+
+__model_factory = {
+ # image classification models
+ 'resnet18': resnet18,
+ 'resnet34': resnet34,
+ 'resnet50': resnet50,
+ 'resnet101': resnet101,
+ 'resnet152': resnet152,
+ 'resnext50_32x4d': resnext50_32x4d,
+ 'resnext101_32x8d': resnext101_32x8d,
+ 'resnet50_fc512': resnet50_fc512,
+ 'se_resnet50': se_resnet50,
+ 'se_resnet50_fc512': se_resnet50_fc512,
+ 'se_resnet101': se_resnet101,
+ 'se_resnext50_32x4d': se_resnext50_32x4d,
+ 'se_resnext101_32x4d': se_resnext101_32x4d,
+ 'densenet121': densenet121,
+ 'densenet169': densenet169,
+ 'densenet201': densenet201,
+ 'densenet161': densenet161,
+ 'densenet121_fc512': densenet121_fc512,
+ 'inceptionresnetv2': inceptionresnetv2,
+ 'inceptionv4': inceptionv4,
+ 'xception': xception,
+ 'resnet50_ibn_a': resnet50_ibn_a,
+ 'resnet50_ibn_b': resnet50_ibn_b,
+ # lightweight models
+ 'nasnsetmobile': nasnetamobile,
+ 'mobilenetv2_x1_0': mobilenetv2_x1_0,
+ 'mobilenetv2_x1_4': mobilenetv2_x1_4,
+ 'shufflenet': shufflenet,
+ 'squeezenet1_0': squeezenet1_0,
+ 'squeezenet1_0_fc512': squeezenet1_0_fc512,
+ 'squeezenet1_1': squeezenet1_1,
+ 'shufflenet_v2_x0_5': shufflenet_v2_x0_5,
+ 'shufflenet_v2_x1_0': shufflenet_v2_x1_0,
+ 'shufflenet_v2_x1_5': shufflenet_v2_x1_5,
+ 'shufflenet_v2_x2_0': shufflenet_v2_x2_0,
+ # reid-specific models
+ 'mudeep': MuDeep,
+ 'resnet50mid': resnet50mid,
+ 'hacnn': HACNN,
+ 'pcb_p6': pcb_p6,
+ 'pcb_p4': pcb_p4,
+ 'mlfn': mlfn,
+ 'osnet_x1_0': osnet_x1_0,
+ 'osnet_x0_75': osnet_x0_75,
+ 'osnet_x0_5': osnet_x0_5,
+ 'osnet_x0_25': osnet_x0_25,
+ 'osnet_ibn_x1_0': osnet_ibn_x1_0,
+ 'osnet_ain_x1_0': osnet_ain_x1_0,
+ 'osnet_ain_x0_75': osnet_ain_x0_75,
+ 'osnet_ain_x0_5': osnet_ain_x0_5,
+ 'osnet_ain_x0_25': osnet_ain_x0_25
+}
+
+
+def show_avai_models():
+ """Displays available models.
+
+ Examples::
+ >>> from torchreid import models
+ >>> models.show_avai_models()
+ """
+ print(list(__model_factory.keys()))
+
+
+def build_model(
+ name, num_classes, loss='softmax', pretrained=True, use_gpu=True
+):
+ """A function wrapper for building a model.
+
+ Args:
+ name (str): model name.
+ num_classes (int): number of training identities.
+ loss (str, optional): loss function to optimize the model. Currently
+ supports "softmax" and "triplet". Default is "softmax".
+ pretrained (bool, optional): whether to load ImageNet-pretrained weights.
+ Default is True.
+ use_gpu (bool, optional): whether to use gpu. Default is True.
+
+ Returns:
+ nn.Module
+
+ Examples::
+ >>> from torchreid import models
+ >>> model = models.build_model('resnet50', 751, loss='softmax')
+ """
+ avai_models = list(__model_factory.keys())
+ if name not in avai_models:
+ raise KeyError(
+ 'Unknown model: {}. Must be one of {}'.format(name, avai_models)
+ )
+ return __model_factory[name](
+ num_classes=num_classes,
+ loss=loss,
+ pretrained=pretrained,
+ use_gpu=use_gpu
+ )
diff --git a/trackers/strong_sort/deep/models/__pycache__/__init__.cpython-38.pyc b/trackers/strong_sort/deep/models/__pycache__/__init__.cpython-38.pyc
new file mode 100644
index 0000000000000000000000000000000000000000..3238cc3816bea69fe7ec4bb91277c2a1d3ca8741
Binary files /dev/null and b/trackers/strong_sort/deep/models/__pycache__/__init__.cpython-38.pyc differ
diff --git a/trackers/strong_sort/deep/models/__pycache__/__init__.cpython-39.pyc b/trackers/strong_sort/deep/models/__pycache__/__init__.cpython-39.pyc
new file mode 100644
index 0000000000000000000000000000000000000000..1b28463a718b7e31a45a61a89cfbaf0fa834e814
Binary files /dev/null and b/trackers/strong_sort/deep/models/__pycache__/__init__.cpython-39.pyc differ
diff --git a/trackers/strong_sort/deep/models/__pycache__/densenet.cpython-38.pyc b/trackers/strong_sort/deep/models/__pycache__/densenet.cpython-38.pyc
new file mode 100644
index 0000000000000000000000000000000000000000..f36ca0839f64346948ccc137800ceac6678e4e85
Binary files /dev/null and b/trackers/strong_sort/deep/models/__pycache__/densenet.cpython-38.pyc differ
diff --git a/trackers/strong_sort/deep/models/__pycache__/densenet.cpython-39.pyc b/trackers/strong_sort/deep/models/__pycache__/densenet.cpython-39.pyc
new file mode 100644
index 0000000000000000000000000000000000000000..8e629efb21cf604c95ec5f3e7e7be1ac193a85f4
Binary files /dev/null and b/trackers/strong_sort/deep/models/__pycache__/densenet.cpython-39.pyc differ
diff --git a/trackers/strong_sort/deep/models/__pycache__/hacnn.cpython-38.pyc b/trackers/strong_sort/deep/models/__pycache__/hacnn.cpython-38.pyc
new file mode 100644
index 0000000000000000000000000000000000000000..ddfdc67cfe3dadbba7010a42bb3f7ce065506211
Binary files /dev/null and b/trackers/strong_sort/deep/models/__pycache__/hacnn.cpython-38.pyc differ
diff --git a/trackers/strong_sort/deep/models/__pycache__/hacnn.cpython-39.pyc b/trackers/strong_sort/deep/models/__pycache__/hacnn.cpython-39.pyc
new file mode 100644
index 0000000000000000000000000000000000000000..9b705f089afbc997c106ab8ba8ba9018571b6c8a
Binary files /dev/null and b/trackers/strong_sort/deep/models/__pycache__/hacnn.cpython-39.pyc differ
diff --git a/trackers/strong_sort/deep/models/__pycache__/inceptionresnetv2.cpython-38.pyc b/trackers/strong_sort/deep/models/__pycache__/inceptionresnetv2.cpython-38.pyc
new file mode 100644
index 0000000000000000000000000000000000000000..cf506c4658620d22caaacaf9a1d71f648a6a5abb
Binary files /dev/null and b/trackers/strong_sort/deep/models/__pycache__/inceptionresnetv2.cpython-38.pyc differ
diff --git a/trackers/strong_sort/deep/models/__pycache__/inceptionresnetv2.cpython-39.pyc b/trackers/strong_sort/deep/models/__pycache__/inceptionresnetv2.cpython-39.pyc
new file mode 100644
index 0000000000000000000000000000000000000000..59385dc53ef9704f66cab68cb4cc74fcf4431d55
Binary files /dev/null and b/trackers/strong_sort/deep/models/__pycache__/inceptionresnetv2.cpython-39.pyc differ
diff --git a/trackers/strong_sort/deep/models/__pycache__/inceptionv4.cpython-38.pyc b/trackers/strong_sort/deep/models/__pycache__/inceptionv4.cpython-38.pyc
new file mode 100644
index 0000000000000000000000000000000000000000..9c93ad1ff1baead05b6a457acda08db09b52dbeb
Binary files /dev/null and b/trackers/strong_sort/deep/models/__pycache__/inceptionv4.cpython-38.pyc differ
diff --git a/trackers/strong_sort/deep/models/__pycache__/inceptionv4.cpython-39.pyc b/trackers/strong_sort/deep/models/__pycache__/inceptionv4.cpython-39.pyc
new file mode 100644
index 0000000000000000000000000000000000000000..ebeb9ef7eeb267dbd4b0ffc6488347a7d078c469
Binary files /dev/null and b/trackers/strong_sort/deep/models/__pycache__/inceptionv4.cpython-39.pyc differ
diff --git a/trackers/strong_sort/deep/models/__pycache__/mlfn.cpython-38.pyc b/trackers/strong_sort/deep/models/__pycache__/mlfn.cpython-38.pyc
new file mode 100644
index 0000000000000000000000000000000000000000..814552a3461f8a32bf6665a1f56053585903b0c1
Binary files /dev/null and b/trackers/strong_sort/deep/models/__pycache__/mlfn.cpython-38.pyc differ
diff --git a/trackers/strong_sort/deep/models/__pycache__/mlfn.cpython-39.pyc b/trackers/strong_sort/deep/models/__pycache__/mlfn.cpython-39.pyc
new file mode 100644
index 0000000000000000000000000000000000000000..82d6d2c33282a8b0ff357436fb7bae42161c402a
Binary files /dev/null and b/trackers/strong_sort/deep/models/__pycache__/mlfn.cpython-39.pyc differ
diff --git a/trackers/strong_sort/deep/models/__pycache__/mobilenetv2.cpython-38.pyc b/trackers/strong_sort/deep/models/__pycache__/mobilenetv2.cpython-38.pyc
new file mode 100644
index 0000000000000000000000000000000000000000..1f793ae4568e49b3d52f95c03891703569727b54
Binary files /dev/null and b/trackers/strong_sort/deep/models/__pycache__/mobilenetv2.cpython-38.pyc differ
diff --git a/trackers/strong_sort/deep/models/__pycache__/mobilenetv2.cpython-39.pyc b/trackers/strong_sort/deep/models/__pycache__/mobilenetv2.cpython-39.pyc
new file mode 100644
index 0000000000000000000000000000000000000000..1d26c63f1dcc605f30531fd27a617965e582a8e0
Binary files /dev/null and b/trackers/strong_sort/deep/models/__pycache__/mobilenetv2.cpython-39.pyc differ
diff --git a/trackers/strong_sort/deep/models/__pycache__/mudeep.cpython-38.pyc b/trackers/strong_sort/deep/models/__pycache__/mudeep.cpython-38.pyc
new file mode 100644
index 0000000000000000000000000000000000000000..66e6cc7c240cb1caaff967eda43318b766eb6d6d
Binary files /dev/null and b/trackers/strong_sort/deep/models/__pycache__/mudeep.cpython-38.pyc differ
diff --git a/trackers/strong_sort/deep/models/__pycache__/mudeep.cpython-39.pyc b/trackers/strong_sort/deep/models/__pycache__/mudeep.cpython-39.pyc
new file mode 100644
index 0000000000000000000000000000000000000000..ac271083965578850cdff1584c1c852256e9cf1b
Binary files /dev/null and b/trackers/strong_sort/deep/models/__pycache__/mudeep.cpython-39.pyc differ
diff --git a/trackers/strong_sort/deep/models/__pycache__/nasnet.cpython-38.pyc b/trackers/strong_sort/deep/models/__pycache__/nasnet.cpython-38.pyc
new file mode 100644
index 0000000000000000000000000000000000000000..fbde2d5aca97f016558635a724b8a9fb15ec7d4b
Binary files /dev/null and b/trackers/strong_sort/deep/models/__pycache__/nasnet.cpython-38.pyc differ
diff --git a/trackers/strong_sort/deep/models/__pycache__/nasnet.cpython-39.pyc b/trackers/strong_sort/deep/models/__pycache__/nasnet.cpython-39.pyc
new file mode 100644
index 0000000000000000000000000000000000000000..985a1fc07ea8caa295af04fca813073fb20c73b2
Binary files /dev/null and b/trackers/strong_sort/deep/models/__pycache__/nasnet.cpython-39.pyc differ
diff --git a/trackers/strong_sort/deep/models/__pycache__/osnet.cpython-38.pyc b/trackers/strong_sort/deep/models/__pycache__/osnet.cpython-38.pyc
new file mode 100644
index 0000000000000000000000000000000000000000..23af84990421f83e80ba37e01244f45244bc8356
Binary files /dev/null and b/trackers/strong_sort/deep/models/__pycache__/osnet.cpython-38.pyc differ
diff --git a/trackers/strong_sort/deep/models/__pycache__/osnet.cpython-39.pyc b/trackers/strong_sort/deep/models/__pycache__/osnet.cpython-39.pyc
new file mode 100644
index 0000000000000000000000000000000000000000..0d91d3128cdc06a87f3a4403ac760329a9619025
Binary files /dev/null and b/trackers/strong_sort/deep/models/__pycache__/osnet.cpython-39.pyc differ
diff --git a/trackers/strong_sort/deep/models/__pycache__/osnet_ain.cpython-38.pyc b/trackers/strong_sort/deep/models/__pycache__/osnet_ain.cpython-38.pyc
new file mode 100644
index 0000000000000000000000000000000000000000..f1545a29c0e9c8d05f571b015f9fb9f794dc6278
Binary files /dev/null and b/trackers/strong_sort/deep/models/__pycache__/osnet_ain.cpython-38.pyc differ
diff --git a/trackers/strong_sort/deep/models/__pycache__/osnet_ain.cpython-39.pyc b/trackers/strong_sort/deep/models/__pycache__/osnet_ain.cpython-39.pyc
new file mode 100644
index 0000000000000000000000000000000000000000..11948409244ea31d2430df57f7e7c8dd6a228514
Binary files /dev/null and b/trackers/strong_sort/deep/models/__pycache__/osnet_ain.cpython-39.pyc differ
diff --git a/trackers/strong_sort/deep/models/__pycache__/pcb.cpython-38.pyc b/trackers/strong_sort/deep/models/__pycache__/pcb.cpython-38.pyc
new file mode 100644
index 0000000000000000000000000000000000000000..37aaf193ad37c6985f60bc767a30f78f425041cf
Binary files /dev/null and b/trackers/strong_sort/deep/models/__pycache__/pcb.cpython-38.pyc differ
diff --git a/trackers/strong_sort/deep/models/__pycache__/pcb.cpython-39.pyc b/trackers/strong_sort/deep/models/__pycache__/pcb.cpython-39.pyc
new file mode 100644
index 0000000000000000000000000000000000000000..07ac3eb48e52e581ff1cddfd32a721e4eb0305c3
Binary files /dev/null and b/trackers/strong_sort/deep/models/__pycache__/pcb.cpython-39.pyc differ
diff --git a/trackers/strong_sort/deep/models/__pycache__/resnet.cpython-38.pyc b/trackers/strong_sort/deep/models/__pycache__/resnet.cpython-38.pyc
new file mode 100644
index 0000000000000000000000000000000000000000..4b57ff48cd05f4c9fc528df641d3d1581887369d
Binary files /dev/null and b/trackers/strong_sort/deep/models/__pycache__/resnet.cpython-38.pyc differ
diff --git a/trackers/strong_sort/deep/models/__pycache__/resnet.cpython-39.pyc b/trackers/strong_sort/deep/models/__pycache__/resnet.cpython-39.pyc
new file mode 100644
index 0000000000000000000000000000000000000000..02868fc45a3abd87fcd873ef1cbfee4c0ff9f038
Binary files /dev/null and b/trackers/strong_sort/deep/models/__pycache__/resnet.cpython-39.pyc differ
diff --git a/trackers/strong_sort/deep/models/__pycache__/resnet_ibn_a.cpython-38.pyc b/trackers/strong_sort/deep/models/__pycache__/resnet_ibn_a.cpython-38.pyc
new file mode 100644
index 0000000000000000000000000000000000000000..8756134f23f627e50bd405c01300579c7323bf5e
Binary files /dev/null and b/trackers/strong_sort/deep/models/__pycache__/resnet_ibn_a.cpython-38.pyc differ
diff --git a/trackers/strong_sort/deep/models/__pycache__/resnet_ibn_a.cpython-39.pyc b/trackers/strong_sort/deep/models/__pycache__/resnet_ibn_a.cpython-39.pyc
new file mode 100644
index 0000000000000000000000000000000000000000..48d73bdc01d54853a828806510387fb396d94bd3
Binary files /dev/null and b/trackers/strong_sort/deep/models/__pycache__/resnet_ibn_a.cpython-39.pyc differ
diff --git a/trackers/strong_sort/deep/models/__pycache__/resnet_ibn_b.cpython-38.pyc b/trackers/strong_sort/deep/models/__pycache__/resnet_ibn_b.cpython-38.pyc
new file mode 100644
index 0000000000000000000000000000000000000000..3555b6e773ea53c132723d7c77ead45c64cd0767
Binary files /dev/null and b/trackers/strong_sort/deep/models/__pycache__/resnet_ibn_b.cpython-38.pyc differ
diff --git a/trackers/strong_sort/deep/models/__pycache__/resnet_ibn_b.cpython-39.pyc b/trackers/strong_sort/deep/models/__pycache__/resnet_ibn_b.cpython-39.pyc
new file mode 100644
index 0000000000000000000000000000000000000000..08749f4e1ed1bbd719c16133dd3ed3963516015c
Binary files /dev/null and b/trackers/strong_sort/deep/models/__pycache__/resnet_ibn_b.cpython-39.pyc differ
diff --git a/trackers/strong_sort/deep/models/__pycache__/resnetmid.cpython-38.pyc b/trackers/strong_sort/deep/models/__pycache__/resnetmid.cpython-38.pyc
new file mode 100644
index 0000000000000000000000000000000000000000..628b04f3de2acddbf5d2db3b30aa9f23f2fe31c9
Binary files /dev/null and b/trackers/strong_sort/deep/models/__pycache__/resnetmid.cpython-38.pyc differ
diff --git a/trackers/strong_sort/deep/models/__pycache__/resnetmid.cpython-39.pyc b/trackers/strong_sort/deep/models/__pycache__/resnetmid.cpython-39.pyc
new file mode 100644
index 0000000000000000000000000000000000000000..1abd6784f64dfc7ffbd433a26d489eac0a4a4b2c
Binary files /dev/null and b/trackers/strong_sort/deep/models/__pycache__/resnetmid.cpython-39.pyc differ
diff --git a/trackers/strong_sort/deep/models/__pycache__/senet.cpython-38.pyc b/trackers/strong_sort/deep/models/__pycache__/senet.cpython-38.pyc
new file mode 100644
index 0000000000000000000000000000000000000000..788ec600f66ffd7201bb291be45f4bfdef59ed0b
Binary files /dev/null and b/trackers/strong_sort/deep/models/__pycache__/senet.cpython-38.pyc differ
diff --git a/trackers/strong_sort/deep/models/__pycache__/senet.cpython-39.pyc b/trackers/strong_sort/deep/models/__pycache__/senet.cpython-39.pyc
new file mode 100644
index 0000000000000000000000000000000000000000..83d3f999eae6b734c143623343414b725ab8477d
Binary files /dev/null and b/trackers/strong_sort/deep/models/__pycache__/senet.cpython-39.pyc differ
diff --git a/trackers/strong_sort/deep/models/__pycache__/shufflenet.cpython-38.pyc b/trackers/strong_sort/deep/models/__pycache__/shufflenet.cpython-38.pyc
new file mode 100644
index 0000000000000000000000000000000000000000..2dae67497f8fde42a6c0fdd810bf91f72e6c0872
Binary files /dev/null and b/trackers/strong_sort/deep/models/__pycache__/shufflenet.cpython-38.pyc differ
diff --git a/trackers/strong_sort/deep/models/__pycache__/shufflenet.cpython-39.pyc b/trackers/strong_sort/deep/models/__pycache__/shufflenet.cpython-39.pyc
new file mode 100644
index 0000000000000000000000000000000000000000..060d1824b66139acfc322d9420bf852824a6d419
Binary files /dev/null and b/trackers/strong_sort/deep/models/__pycache__/shufflenet.cpython-39.pyc differ
diff --git a/trackers/strong_sort/deep/models/__pycache__/shufflenetv2.cpython-38.pyc b/trackers/strong_sort/deep/models/__pycache__/shufflenetv2.cpython-38.pyc
new file mode 100644
index 0000000000000000000000000000000000000000..36a40cb0378b39ca135b95c442201c024ebd81ae
Binary files /dev/null and b/trackers/strong_sort/deep/models/__pycache__/shufflenetv2.cpython-38.pyc differ
diff --git a/trackers/strong_sort/deep/models/__pycache__/shufflenetv2.cpython-39.pyc b/trackers/strong_sort/deep/models/__pycache__/shufflenetv2.cpython-39.pyc
new file mode 100644
index 0000000000000000000000000000000000000000..f1bcd806ca38baf1b57fbf5534d43f0403a1b047
Binary files /dev/null and b/trackers/strong_sort/deep/models/__pycache__/shufflenetv2.cpython-39.pyc differ
diff --git a/trackers/strong_sort/deep/models/__pycache__/squeezenet.cpython-38.pyc b/trackers/strong_sort/deep/models/__pycache__/squeezenet.cpython-38.pyc
new file mode 100644
index 0000000000000000000000000000000000000000..73a4ccc0a2d14fd716c8eda71af009b1afc79ac3
Binary files /dev/null and b/trackers/strong_sort/deep/models/__pycache__/squeezenet.cpython-38.pyc differ
diff --git a/trackers/strong_sort/deep/models/__pycache__/squeezenet.cpython-39.pyc b/trackers/strong_sort/deep/models/__pycache__/squeezenet.cpython-39.pyc
new file mode 100644
index 0000000000000000000000000000000000000000..1f1f31e7b1ae49eb4a5e4c1dc0cdbf34c511d8b2
Binary files /dev/null and b/trackers/strong_sort/deep/models/__pycache__/squeezenet.cpython-39.pyc differ
diff --git a/trackers/strong_sort/deep/models/__pycache__/xception.cpython-38.pyc b/trackers/strong_sort/deep/models/__pycache__/xception.cpython-38.pyc
new file mode 100644
index 0000000000000000000000000000000000000000..dc321a2a4e47d9b007635bea7696885b23b91e7a
Binary files /dev/null and b/trackers/strong_sort/deep/models/__pycache__/xception.cpython-38.pyc differ
diff --git a/trackers/strong_sort/deep/models/__pycache__/xception.cpython-39.pyc b/trackers/strong_sort/deep/models/__pycache__/xception.cpython-39.pyc
new file mode 100644
index 0000000000000000000000000000000000000000..b5c1fe9fa589d78a23df04c5ca12e5c942593203
Binary files /dev/null and b/trackers/strong_sort/deep/models/__pycache__/xception.cpython-39.pyc differ
diff --git a/trackers/strong_sort/deep/models/densenet.py b/trackers/strong_sort/deep/models/densenet.py
new file mode 100644
index 0000000000000000000000000000000000000000..a1d9b7ef85a79cbc4c4e8a81840935531df636b8
--- /dev/null
+++ b/trackers/strong_sort/deep/models/densenet.py
@@ -0,0 +1,380 @@
+"""
+Code source: https://github.com/pytorch/vision
+"""
+from __future__ import division, absolute_import
+import re
+from collections import OrderedDict
+import torch
+import torch.nn as nn
+from torch.nn import functional as F
+from torch.utils import model_zoo
+
+__all__ = [
+ 'densenet121', 'densenet169', 'densenet201', 'densenet161',
+ 'densenet121_fc512'
+]
+
+model_urls = {
+ 'densenet121':
+ 'https://download.pytorch.org/models/densenet121-a639ec97.pth',
+ 'densenet169':
+ 'https://download.pytorch.org/models/densenet169-b2777c0a.pth',
+ 'densenet201':
+ 'https://download.pytorch.org/models/densenet201-c1103571.pth',
+ 'densenet161':
+ 'https://download.pytorch.org/models/densenet161-8d451a50.pth',
+}
+
+
+class _DenseLayer(nn.Sequential):
+
+ def __init__(self, num_input_features, growth_rate, bn_size, drop_rate):
+ super(_DenseLayer, self).__init__()
+ self.add_module('norm1', nn.BatchNorm2d(num_input_features)),
+ self.add_module('relu1', nn.ReLU(inplace=True)),
+ self.add_module(
+ 'conv1',
+ nn.Conv2d(
+ num_input_features,
+ bn_size * growth_rate,
+ kernel_size=1,
+ stride=1,
+ bias=False
+ )
+ ),
+ self.add_module('norm2', nn.BatchNorm2d(bn_size * growth_rate)),
+ self.add_module('relu2', nn.ReLU(inplace=True)),
+ self.add_module(
+ 'conv2',
+ nn.Conv2d(
+ bn_size * growth_rate,
+ growth_rate,
+ kernel_size=3,
+ stride=1,
+ padding=1,
+ bias=False
+ )
+ ),
+ self.drop_rate = drop_rate
+
+ def forward(self, x):
+ new_features = super(_DenseLayer, self).forward(x)
+ if self.drop_rate > 0:
+ new_features = F.dropout(
+ new_features, p=self.drop_rate, training=self.training
+ )
+ return torch.cat([x, new_features], 1)
+
+
+class _DenseBlock(nn.Sequential):
+
+ def __init__(
+ self, num_layers, num_input_features, bn_size, growth_rate, drop_rate
+ ):
+ super(_DenseBlock, self).__init__()
+ for i in range(num_layers):
+ layer = _DenseLayer(
+ num_input_features + i*growth_rate, growth_rate, bn_size,
+ drop_rate
+ )
+ self.add_module('denselayer%d' % (i+1), layer)
+
+
+class _Transition(nn.Sequential):
+
+ def __init__(self, num_input_features, num_output_features):
+ super(_Transition, self).__init__()
+ self.add_module('norm', nn.BatchNorm2d(num_input_features))
+ self.add_module('relu', nn.ReLU(inplace=True))
+ self.add_module(
+ 'conv',
+ nn.Conv2d(
+ num_input_features,
+ num_output_features,
+ kernel_size=1,
+ stride=1,
+ bias=False
+ )
+ )
+ self.add_module('pool', nn.AvgPool2d(kernel_size=2, stride=2))
+
+
+class DenseNet(nn.Module):
+ """Densely connected network.
+
+ Reference:
+ Huang et al. Densely Connected Convolutional Networks. CVPR 2017.
+
+ Public keys:
+ - ``densenet121``: DenseNet121.
+ - ``densenet169``: DenseNet169.
+ - ``densenet201``: DenseNet201.
+ - ``densenet161``: DenseNet161.
+ - ``densenet121_fc512``: DenseNet121 + FC.
+ """
+
+ def __init__(
+ self,
+ num_classes,
+ loss,
+ growth_rate=32,
+ block_config=(6, 12, 24, 16),
+ num_init_features=64,
+ bn_size=4,
+ drop_rate=0,
+ fc_dims=None,
+ dropout_p=None,
+ **kwargs
+ ):
+
+ super(DenseNet, self).__init__()
+ self.loss = loss
+
+ # First convolution
+ self.features = nn.Sequential(
+ OrderedDict(
+ [
+ (
+ 'conv0',
+ nn.Conv2d(
+ 3,
+ num_init_features,
+ kernel_size=7,
+ stride=2,
+ padding=3,
+ bias=False
+ )
+ ),
+ ('norm0', nn.BatchNorm2d(num_init_features)),
+ ('relu0', nn.ReLU(inplace=True)),
+ (
+ 'pool0',
+ nn.MaxPool2d(kernel_size=3, stride=2, padding=1)
+ ),
+ ]
+ )
+ )
+
+ # Each denseblock
+ num_features = num_init_features
+ for i, num_layers in enumerate(block_config):
+ block = _DenseBlock(
+ num_layers=num_layers,
+ num_input_features=num_features,
+ bn_size=bn_size,
+ growth_rate=growth_rate,
+ drop_rate=drop_rate
+ )
+ self.features.add_module('denseblock%d' % (i+1), block)
+ num_features = num_features + num_layers*growth_rate
+ if i != len(block_config) - 1:
+ trans = _Transition(
+ num_input_features=num_features,
+ num_output_features=num_features // 2
+ )
+ self.features.add_module('transition%d' % (i+1), trans)
+ num_features = num_features // 2
+
+ # Final batch norm
+ self.features.add_module('norm5', nn.BatchNorm2d(num_features))
+
+ self.global_avgpool = nn.AdaptiveAvgPool2d(1)
+ self.feature_dim = num_features
+ self.fc = self._construct_fc_layer(fc_dims, num_features, dropout_p)
+
+ # Linear layer
+ self.classifier = nn.Linear(self.feature_dim, num_classes)
+
+ self._init_params()
+
+ def _construct_fc_layer(self, fc_dims, input_dim, dropout_p=None):
+ """Constructs fully connected layer.
+
+ Args:
+ fc_dims (list or tuple): dimensions of fc layers, if None, no fc layers are constructed
+ input_dim (int): input dimension
+ dropout_p (float): dropout probability, if None, dropout is unused
+ """
+ if fc_dims is None:
+ self.feature_dim = input_dim
+ return None
+
+ assert isinstance(
+ fc_dims, (list, tuple)
+ ), 'fc_dims must be either list or tuple, but got {}'.format(
+ type(fc_dims)
+ )
+
+ layers = []
+ for dim in fc_dims:
+ layers.append(nn.Linear(input_dim, dim))
+ layers.append(nn.BatchNorm1d(dim))
+ layers.append(nn.ReLU(inplace=True))
+ if dropout_p is not None:
+ layers.append(nn.Dropout(p=dropout_p))
+ input_dim = dim
+
+ self.feature_dim = fc_dims[-1]
+
+ return nn.Sequential(*layers)
+
+ def _init_params(self):
+ for m in self.modules():
+ if isinstance(m, nn.Conv2d):
+ nn.init.kaiming_normal_(
+ m.weight, mode='fan_out', nonlinearity='relu'
+ )
+ if m.bias is not None:
+ nn.init.constant_(m.bias, 0)
+ elif isinstance(m, nn.BatchNorm2d):
+ nn.init.constant_(m.weight, 1)
+ nn.init.constant_(m.bias, 0)
+ elif isinstance(m, nn.BatchNorm1d):
+ nn.init.constant_(m.weight, 1)
+ nn.init.constant_(m.bias, 0)
+ elif isinstance(m, nn.Linear):
+ nn.init.normal_(m.weight, 0, 0.01)
+ if m.bias is not None:
+ nn.init.constant_(m.bias, 0)
+
+ def forward(self, x):
+ f = self.features(x)
+ f = F.relu(f, inplace=True)
+ v = self.global_avgpool(f)
+ v = v.view(v.size(0), -1)
+
+ if self.fc is not None:
+ v = self.fc(v)
+
+ if not self.training:
+ return v
+
+ y = self.classifier(v)
+
+ if self.loss == 'softmax':
+ return y
+ elif self.loss == 'triplet':
+ return y, v
+ else:
+ raise KeyError('Unsupported loss: {}'.format(self.loss))
+
+
+def init_pretrained_weights(model, model_url):
+ """Initializes model with pretrained weights.
+
+ Layers that don't match with pretrained layers in name or size are kept unchanged.
+ """
+ pretrain_dict = model_zoo.load_url(model_url)
+
+ # '.'s are no longer allowed in module names, but pervious _DenseLayer
+ # has keys 'norm.1', 'relu.1', 'conv.1', 'norm.2', 'relu.2', 'conv.2'.
+ # They are also in the checkpoints in model_urls. This pattern is used
+ # to find such keys.
+ pattern = re.compile(
+ r'^(.*denselayer\d+\.(?:norm|relu|conv))\.((?:[12])\.(?:weight|bias|running_mean|running_var))$'
+ )
+ for key in list(pretrain_dict.keys()):
+ res = pattern.match(key)
+ if res:
+ new_key = res.group(1) + res.group(2)
+ pretrain_dict[new_key] = pretrain_dict[key]
+ del pretrain_dict[key]
+
+ model_dict = model.state_dict()
+ pretrain_dict = {
+ k: v
+ for k, v in pretrain_dict.items()
+ if k in model_dict and model_dict[k].size() == v.size()
+ }
+ model_dict.update(pretrain_dict)
+ model.load_state_dict(model_dict)
+
+
+"""
+Dense network configurations:
+--
+densenet121: num_init_features=64, growth_rate=32, block_config=(6, 12, 24, 16)
+densenet169: num_init_features=64, growth_rate=32, block_config=(6, 12, 32, 32)
+densenet201: num_init_features=64, growth_rate=32, block_config=(6, 12, 48, 32)
+densenet161: num_init_features=96, growth_rate=48, block_config=(6, 12, 36, 24)
+"""
+
+
+def densenet121(num_classes, loss='softmax', pretrained=True, **kwargs):
+ model = DenseNet(
+ num_classes=num_classes,
+ loss=loss,
+ num_init_features=64,
+ growth_rate=32,
+ block_config=(6, 12, 24, 16),
+ fc_dims=None,
+ dropout_p=None,
+ **kwargs
+ )
+ if pretrained:
+ init_pretrained_weights(model, model_urls['densenet121'])
+ return model
+
+
+def densenet169(num_classes, loss='softmax', pretrained=True, **kwargs):
+ model = DenseNet(
+ num_classes=num_classes,
+ loss=loss,
+ num_init_features=64,
+ growth_rate=32,
+ block_config=(6, 12, 32, 32),
+ fc_dims=None,
+ dropout_p=None,
+ **kwargs
+ )
+ if pretrained:
+ init_pretrained_weights(model, model_urls['densenet169'])
+ return model
+
+
+def densenet201(num_classes, loss='softmax', pretrained=True, **kwargs):
+ model = DenseNet(
+ num_classes=num_classes,
+ loss=loss,
+ num_init_features=64,
+ growth_rate=32,
+ block_config=(6, 12, 48, 32),
+ fc_dims=None,
+ dropout_p=None,
+ **kwargs
+ )
+ if pretrained:
+ init_pretrained_weights(model, model_urls['densenet201'])
+ return model
+
+
+def densenet161(num_classes, loss='softmax', pretrained=True, **kwargs):
+ model = DenseNet(
+ num_classes=num_classes,
+ loss=loss,
+ num_init_features=96,
+ growth_rate=48,
+ block_config=(6, 12, 36, 24),
+ fc_dims=None,
+ dropout_p=None,
+ **kwargs
+ )
+ if pretrained:
+ init_pretrained_weights(model, model_urls['densenet161'])
+ return model
+
+
+def densenet121_fc512(num_classes, loss='softmax', pretrained=True, **kwargs):
+ model = DenseNet(
+ num_classes=num_classes,
+ loss=loss,
+ num_init_features=64,
+ growth_rate=32,
+ block_config=(6, 12, 24, 16),
+ fc_dims=[512],
+ dropout_p=None,
+ **kwargs
+ )
+ if pretrained:
+ init_pretrained_weights(model, model_urls['densenet121'])
+ return model
diff --git a/trackers/strong_sort/deep/models/hacnn.py b/trackers/strong_sort/deep/models/hacnn.py
new file mode 100644
index 0000000000000000000000000000000000000000..f21cc82f42fe181317f9a0d89cdede95699f45a9
--- /dev/null
+++ b/trackers/strong_sort/deep/models/hacnn.py
@@ -0,0 +1,414 @@
+from __future__ import division, absolute_import
+import torch
+from torch import nn
+from torch.nn import functional as F
+
+__all__ = ['HACNN']
+
+
+class ConvBlock(nn.Module):
+ """Basic convolutional block.
+
+ convolution + batch normalization + relu.
+
+ Args:
+ in_c (int): number of input channels.
+ out_c (int): number of output channels.
+ k (int or tuple): kernel size.
+ s (int or tuple): stride.
+ p (int or tuple): padding.
+ """
+
+ def __init__(self, in_c, out_c, k, s=1, p=0):
+ super(ConvBlock, self).__init__()
+ self.conv = nn.Conv2d(in_c, out_c, k, stride=s, padding=p)
+ self.bn = nn.BatchNorm2d(out_c)
+
+ def forward(self, x):
+ return F.relu(self.bn(self.conv(x)))
+
+
+class InceptionA(nn.Module):
+
+ def __init__(self, in_channels, out_channels):
+ super(InceptionA, self).__init__()
+ mid_channels = out_channels // 4
+
+ self.stream1 = nn.Sequential(
+ ConvBlock(in_channels, mid_channels, 1),
+ ConvBlock(mid_channels, mid_channels, 3, p=1),
+ )
+ self.stream2 = nn.Sequential(
+ ConvBlock(in_channels, mid_channels, 1),
+ ConvBlock(mid_channels, mid_channels, 3, p=1),
+ )
+ self.stream3 = nn.Sequential(
+ ConvBlock(in_channels, mid_channels, 1),
+ ConvBlock(mid_channels, mid_channels, 3, p=1),
+ )
+ self.stream4 = nn.Sequential(
+ nn.AvgPool2d(3, stride=1, padding=1),
+ ConvBlock(in_channels, mid_channels, 1),
+ )
+
+ def forward(self, x):
+ s1 = self.stream1(x)
+ s2 = self.stream2(x)
+ s3 = self.stream3(x)
+ s4 = self.stream4(x)
+ y = torch.cat([s1, s2, s3, s4], dim=1)
+ return y
+
+
+class InceptionB(nn.Module):
+
+ def __init__(self, in_channels, out_channels):
+ super(InceptionB, self).__init__()
+ mid_channels = out_channels // 4
+
+ self.stream1 = nn.Sequential(
+ ConvBlock(in_channels, mid_channels, 1),
+ ConvBlock(mid_channels, mid_channels, 3, s=2, p=1),
+ )
+ self.stream2 = nn.Sequential(
+ ConvBlock(in_channels, mid_channels, 1),
+ ConvBlock(mid_channels, mid_channels, 3, p=1),
+ ConvBlock(mid_channels, mid_channels, 3, s=2, p=1),
+ )
+ self.stream3 = nn.Sequential(
+ nn.MaxPool2d(3, stride=2, padding=1),
+ ConvBlock(in_channels, mid_channels * 2, 1),
+ )
+
+ def forward(self, x):
+ s1 = self.stream1(x)
+ s2 = self.stream2(x)
+ s3 = self.stream3(x)
+ y = torch.cat([s1, s2, s3], dim=1)
+ return y
+
+
+class SpatialAttn(nn.Module):
+ """Spatial Attention (Sec. 3.1.I.1)"""
+
+ def __init__(self):
+ super(SpatialAttn, self).__init__()
+ self.conv1 = ConvBlock(1, 1, 3, s=2, p=1)
+ self.conv2 = ConvBlock(1, 1, 1)
+
+ def forward(self, x):
+ # global cross-channel averaging
+ x = x.mean(1, keepdim=True)
+ # 3-by-3 conv
+ x = self.conv1(x)
+ # bilinear resizing
+ x = F.upsample(
+ x, (x.size(2) * 2, x.size(3) * 2),
+ mode='bilinear',
+ align_corners=True
+ )
+ # scaling conv
+ x = self.conv2(x)
+ return x
+
+
+class ChannelAttn(nn.Module):
+ """Channel Attention (Sec. 3.1.I.2)"""
+
+ def __init__(self, in_channels, reduction_rate=16):
+ super(ChannelAttn, self).__init__()
+ assert in_channels % reduction_rate == 0
+ self.conv1 = ConvBlock(in_channels, in_channels // reduction_rate, 1)
+ self.conv2 = ConvBlock(in_channels // reduction_rate, in_channels, 1)
+
+ def forward(self, x):
+ # squeeze operation (global average pooling)
+ x = F.avg_pool2d(x, x.size()[2:])
+ # excitation operation (2 conv layers)
+ x = self.conv1(x)
+ x = self.conv2(x)
+ return x
+
+
+class SoftAttn(nn.Module):
+ """Soft Attention (Sec. 3.1.I)
+
+ Aim: Spatial Attention + Channel Attention
+
+ Output: attention maps with shape identical to input.
+ """
+
+ def __init__(self, in_channels):
+ super(SoftAttn, self).__init__()
+ self.spatial_attn = SpatialAttn()
+ self.channel_attn = ChannelAttn(in_channels)
+ self.conv = ConvBlock(in_channels, in_channels, 1)
+
+ def forward(self, x):
+ y_spatial = self.spatial_attn(x)
+ y_channel = self.channel_attn(x)
+ y = y_spatial * y_channel
+ y = torch.sigmoid(self.conv(y))
+ return y
+
+
+class HardAttn(nn.Module):
+ """Hard Attention (Sec. 3.1.II)"""
+
+ def __init__(self, in_channels):
+ super(HardAttn, self).__init__()
+ self.fc = nn.Linear(in_channels, 4 * 2)
+ self.init_params()
+
+ def init_params(self):
+ self.fc.weight.data.zero_()
+ self.fc.bias.data.copy_(
+ torch.tensor(
+ [0, -0.75, 0, -0.25, 0, 0.25, 0, 0.75], dtype=torch.float
+ )
+ )
+
+ def forward(self, x):
+ # squeeze operation (global average pooling)
+ x = F.avg_pool2d(x, x.size()[2:]).view(x.size(0), x.size(1))
+ # predict transformation parameters
+ theta = torch.tanh(self.fc(x))
+ theta = theta.view(-1, 4, 2)
+ return theta
+
+
+class HarmAttn(nn.Module):
+ """Harmonious Attention (Sec. 3.1)"""
+
+ def __init__(self, in_channels):
+ super(HarmAttn, self).__init__()
+ self.soft_attn = SoftAttn(in_channels)
+ self.hard_attn = HardAttn(in_channels)
+
+ def forward(self, x):
+ y_soft_attn = self.soft_attn(x)
+ theta = self.hard_attn(x)
+ return y_soft_attn, theta
+
+
+class HACNN(nn.Module):
+ """Harmonious Attention Convolutional Neural Network.
+
+ Reference:
+ Li et al. Harmonious Attention Network for Person Re-identification. CVPR 2018.
+
+ Public keys:
+ - ``hacnn``: HACNN.
+ """
+
+ # Args:
+ # num_classes (int): number of classes to predict
+ # nchannels (list): number of channels AFTER concatenation
+ # feat_dim (int): feature dimension for a single stream
+ # learn_region (bool): whether to learn region features (i.e. local branch)
+
+ def __init__(
+ self,
+ num_classes,
+ loss='softmax',
+ nchannels=[128, 256, 384],
+ feat_dim=512,
+ learn_region=True,
+ use_gpu=True,
+ **kwargs
+ ):
+ super(HACNN, self).__init__()
+ self.loss = loss
+ self.learn_region = learn_region
+ self.use_gpu = use_gpu
+
+ self.conv = ConvBlock(3, 32, 3, s=2, p=1)
+
+ # Construct Inception + HarmAttn blocks
+ # ============== Block 1 ==============
+ self.inception1 = nn.Sequential(
+ InceptionA(32, nchannels[0]),
+ InceptionB(nchannels[0], nchannels[0]),
+ )
+ self.ha1 = HarmAttn(nchannels[0])
+
+ # ============== Block 2 ==============
+ self.inception2 = nn.Sequential(
+ InceptionA(nchannels[0], nchannels[1]),
+ InceptionB(nchannels[1], nchannels[1]),
+ )
+ self.ha2 = HarmAttn(nchannels[1])
+
+ # ============== Block 3 ==============
+ self.inception3 = nn.Sequential(
+ InceptionA(nchannels[1], nchannels[2]),
+ InceptionB(nchannels[2], nchannels[2]),
+ )
+ self.ha3 = HarmAttn(nchannels[2])
+
+ self.fc_global = nn.Sequential(
+ nn.Linear(nchannels[2], feat_dim),
+ nn.BatchNorm1d(feat_dim),
+ nn.ReLU(),
+ )
+ self.classifier_global = nn.Linear(feat_dim, num_classes)
+
+ if self.learn_region:
+ self.init_scale_factors()
+ self.local_conv1 = InceptionB(32, nchannels[0])
+ self.local_conv2 = InceptionB(nchannels[0], nchannels[1])
+ self.local_conv3 = InceptionB(nchannels[1], nchannels[2])
+ self.fc_local = nn.Sequential(
+ nn.Linear(nchannels[2] * 4, feat_dim),
+ nn.BatchNorm1d(feat_dim),
+ nn.ReLU(),
+ )
+ self.classifier_local = nn.Linear(feat_dim, num_classes)
+ self.feat_dim = feat_dim * 2
+ else:
+ self.feat_dim = feat_dim
+
+ def init_scale_factors(self):
+ # initialize scale factors (s_w, s_h) for four regions
+ self.scale_factors = []
+ self.scale_factors.append(
+ torch.tensor([[1, 0], [0, 0.25]], dtype=torch.float)
+ )
+ self.scale_factors.append(
+ torch.tensor([[1, 0], [0, 0.25]], dtype=torch.float)
+ )
+ self.scale_factors.append(
+ torch.tensor([[1, 0], [0, 0.25]], dtype=torch.float)
+ )
+ self.scale_factors.append(
+ torch.tensor([[1, 0], [0, 0.25]], dtype=torch.float)
+ )
+
+ def stn(self, x, theta):
+ """Performs spatial transform
+
+ x: (batch, channel, height, width)
+ theta: (batch, 2, 3)
+ """
+ grid = F.affine_grid(theta, x.size())
+ x = F.grid_sample(x, grid)
+ return x
+
+ def transform_theta(self, theta_i, region_idx):
+ """Transforms theta to include (s_w, s_h), resulting in (batch, 2, 3)"""
+ scale_factors = self.scale_factors[region_idx]
+ theta = torch.zeros(theta_i.size(0), 2, 3)
+ theta[:, :, :2] = scale_factors
+ theta[:, :, -1] = theta_i
+ if self.use_gpu:
+ theta = theta.cuda()
+ return theta
+
+ def forward(self, x):
+ assert x.size(2) == 160 and x.size(3) == 64, \
+ 'Input size does not match, expected (160, 64) but got ({}, {})'.format(x.size(2), x.size(3))
+ x = self.conv(x)
+
+ # ============== Block 1 ==============
+ # global branch
+ x1 = self.inception1(x)
+ x1_attn, x1_theta = self.ha1(x1)
+ x1_out = x1 * x1_attn
+ # local branch
+ if self.learn_region:
+ x1_local_list = []
+ for region_idx in range(4):
+ x1_theta_i = x1_theta[:, region_idx, :]
+ x1_theta_i = self.transform_theta(x1_theta_i, region_idx)
+ x1_trans_i = self.stn(x, x1_theta_i)
+ x1_trans_i = F.upsample(
+ x1_trans_i, (24, 28), mode='bilinear', align_corners=True
+ )
+ x1_local_i = self.local_conv1(x1_trans_i)
+ x1_local_list.append(x1_local_i)
+
+ # ============== Block 2 ==============
+ # Block 2
+ # global branch
+ x2 = self.inception2(x1_out)
+ x2_attn, x2_theta = self.ha2(x2)
+ x2_out = x2 * x2_attn
+ # local branch
+ if self.learn_region:
+ x2_local_list = []
+ for region_idx in range(4):
+ x2_theta_i = x2_theta[:, region_idx, :]
+ x2_theta_i = self.transform_theta(x2_theta_i, region_idx)
+ x2_trans_i = self.stn(x1_out, x2_theta_i)
+ x2_trans_i = F.upsample(
+ x2_trans_i, (12, 14), mode='bilinear', align_corners=True
+ )
+ x2_local_i = x2_trans_i + x1_local_list[region_idx]
+ x2_local_i = self.local_conv2(x2_local_i)
+ x2_local_list.append(x2_local_i)
+
+ # ============== Block 3 ==============
+ # Block 3
+ # global branch
+ x3 = self.inception3(x2_out)
+ x3_attn, x3_theta = self.ha3(x3)
+ x3_out = x3 * x3_attn
+ # local branch
+ if self.learn_region:
+ x3_local_list = []
+ for region_idx in range(4):
+ x3_theta_i = x3_theta[:, region_idx, :]
+ x3_theta_i = self.transform_theta(x3_theta_i, region_idx)
+ x3_trans_i = self.stn(x2_out, x3_theta_i)
+ x3_trans_i = F.upsample(
+ x3_trans_i, (6, 7), mode='bilinear', align_corners=True
+ )
+ x3_local_i = x3_trans_i + x2_local_list[region_idx]
+ x3_local_i = self.local_conv3(x3_local_i)
+ x3_local_list.append(x3_local_i)
+
+ # ============== Feature generation ==============
+ # global branch
+ x_global = F.avg_pool2d(x3_out,
+ x3_out.size()[2:]
+ ).view(x3_out.size(0), x3_out.size(1))
+ x_global = self.fc_global(x_global)
+ # local branch
+ if self.learn_region:
+ x_local_list = []
+ for region_idx in range(4):
+ x_local_i = x3_local_list[region_idx]
+ x_local_i = F.avg_pool2d(x_local_i,
+ x_local_i.size()[2:]
+ ).view(x_local_i.size(0), -1)
+ x_local_list.append(x_local_i)
+ x_local = torch.cat(x_local_list, 1)
+ x_local = self.fc_local(x_local)
+
+ if not self.training:
+ # l2 normalization before concatenation
+ if self.learn_region:
+ x_global = x_global / x_global.norm(p=2, dim=1, keepdim=True)
+ x_local = x_local / x_local.norm(p=2, dim=1, keepdim=True)
+ return torch.cat([x_global, x_local], 1)
+ else:
+ return x_global
+
+ prelogits_global = self.classifier_global(x_global)
+ if self.learn_region:
+ prelogits_local = self.classifier_local(x_local)
+
+ if self.loss == 'softmax':
+ if self.learn_region:
+ return (prelogits_global, prelogits_local)
+ else:
+ return prelogits_global
+
+ elif self.loss == 'triplet':
+ if self.learn_region:
+ return (prelogits_global, prelogits_local), (x_global, x_local)
+ else:
+ return prelogits_global, x_global
+
+ else:
+ raise KeyError("Unsupported loss: {}".format(self.loss))
diff --git a/trackers/strong_sort/deep/models/inceptionresnetv2.py b/trackers/strong_sort/deep/models/inceptionresnetv2.py
new file mode 100644
index 0000000000000000000000000000000000000000..03e40348425a2b1bc73e6f336efae8e5525cc45c
--- /dev/null
+++ b/trackers/strong_sort/deep/models/inceptionresnetv2.py
@@ -0,0 +1,361 @@
+"""
+Code imported from https://github.com/Cadene/pretrained-models.pytorch
+"""
+from __future__ import division, absolute_import
+import torch
+import torch.nn as nn
+import torch.utils.model_zoo as model_zoo
+
+__all__ = ['inceptionresnetv2']
+
+pretrained_settings = {
+ 'inceptionresnetv2': {
+ 'imagenet': {
+ 'url':
+ 'http://data.lip6.fr/cadene/pretrainedmodels/inceptionresnetv2-520b38e4.pth',
+ 'input_space': 'RGB',
+ 'input_size': [3, 299, 299],
+ 'input_range': [0, 1],
+ 'mean': [0.5, 0.5, 0.5],
+ 'std': [0.5, 0.5, 0.5],
+ 'num_classes': 1000
+ },
+ 'imagenet+background': {
+ 'url':
+ 'http://data.lip6.fr/cadene/pretrainedmodels/inceptionresnetv2-520b38e4.pth',
+ 'input_space': 'RGB',
+ 'input_size': [3, 299, 299],
+ 'input_range': [0, 1],
+ 'mean': [0.5, 0.5, 0.5],
+ 'std': [0.5, 0.5, 0.5],
+ 'num_classes': 1001
+ }
+ }
+}
+
+
+class BasicConv2d(nn.Module):
+
+ def __init__(self, in_planes, out_planes, kernel_size, stride, padding=0):
+ super(BasicConv2d, self).__init__()
+ self.conv = nn.Conv2d(
+ in_planes,
+ out_planes,
+ kernel_size=kernel_size,
+ stride=stride,
+ padding=padding,
+ bias=False
+ ) # verify bias false
+ self.bn = nn.BatchNorm2d(
+ out_planes,
+ eps=0.001, # value found in tensorflow
+ momentum=0.1, # default pytorch value
+ affine=True
+ )
+ self.relu = nn.ReLU(inplace=False)
+
+ def forward(self, x):
+ x = self.conv(x)
+ x = self.bn(x)
+ x = self.relu(x)
+ return x
+
+
+class Mixed_5b(nn.Module):
+
+ def __init__(self):
+ super(Mixed_5b, self).__init__()
+
+ self.branch0 = BasicConv2d(192, 96, kernel_size=1, stride=1)
+
+ self.branch1 = nn.Sequential(
+ BasicConv2d(192, 48, kernel_size=1, stride=1),
+ BasicConv2d(48, 64, kernel_size=5, stride=1, padding=2)
+ )
+
+ self.branch2 = nn.Sequential(
+ BasicConv2d(192, 64, kernel_size=1, stride=1),
+ BasicConv2d(64, 96, kernel_size=3, stride=1, padding=1),
+ BasicConv2d(96, 96, kernel_size=3, stride=1, padding=1)
+ )
+
+ self.branch3 = nn.Sequential(
+ nn.AvgPool2d(3, stride=1, padding=1, count_include_pad=False),
+ BasicConv2d(192, 64, kernel_size=1, stride=1)
+ )
+
+ def forward(self, x):
+ x0 = self.branch0(x)
+ x1 = self.branch1(x)
+ x2 = self.branch2(x)
+ x3 = self.branch3(x)
+ out = torch.cat((x0, x1, x2, x3), 1)
+ return out
+
+
+class Block35(nn.Module):
+
+ def __init__(self, scale=1.0):
+ super(Block35, self).__init__()
+
+ self.scale = scale
+
+ self.branch0 = BasicConv2d(320, 32, kernel_size=1, stride=1)
+
+ self.branch1 = nn.Sequential(
+ BasicConv2d(320, 32, kernel_size=1, stride=1),
+ BasicConv2d(32, 32, kernel_size=3, stride=1, padding=1)
+ )
+
+ self.branch2 = nn.Sequential(
+ BasicConv2d(320, 32, kernel_size=1, stride=1),
+ BasicConv2d(32, 48, kernel_size=3, stride=1, padding=1),
+ BasicConv2d(48, 64, kernel_size=3, stride=1, padding=1)
+ )
+
+ self.conv2d = nn.Conv2d(128, 320, kernel_size=1, stride=1)
+ self.relu = nn.ReLU(inplace=False)
+
+ def forward(self, x):
+ x0 = self.branch0(x)
+ x1 = self.branch1(x)
+ x2 = self.branch2(x)
+ out = torch.cat((x0, x1, x2), 1)
+ out = self.conv2d(out)
+ out = out * self.scale + x
+ out = self.relu(out)
+ return out
+
+
+class Mixed_6a(nn.Module):
+
+ def __init__(self):
+ super(Mixed_6a, self).__init__()
+
+ self.branch0 = BasicConv2d(320, 384, kernel_size=3, stride=2)
+
+ self.branch1 = nn.Sequential(
+ BasicConv2d(320, 256, kernel_size=1, stride=1),
+ BasicConv2d(256, 256, kernel_size=3, stride=1, padding=1),
+ BasicConv2d(256, 384, kernel_size=3, stride=2)
+ )
+
+ self.branch2 = nn.MaxPool2d(3, stride=2)
+
+ def forward(self, x):
+ x0 = self.branch0(x)
+ x1 = self.branch1(x)
+ x2 = self.branch2(x)
+ out = torch.cat((x0, x1, x2), 1)
+ return out
+
+
+class Block17(nn.Module):
+
+ def __init__(self, scale=1.0):
+ super(Block17, self).__init__()
+
+ self.scale = scale
+
+ self.branch0 = BasicConv2d(1088, 192, kernel_size=1, stride=1)
+
+ self.branch1 = nn.Sequential(
+ BasicConv2d(1088, 128, kernel_size=1, stride=1),
+ BasicConv2d(
+ 128, 160, kernel_size=(1, 7), stride=1, padding=(0, 3)
+ ),
+ BasicConv2d(
+ 160, 192, kernel_size=(7, 1), stride=1, padding=(3, 0)
+ )
+ )
+
+ self.conv2d = nn.Conv2d(384, 1088, kernel_size=1, stride=1)
+ self.relu = nn.ReLU(inplace=False)
+
+ def forward(self, x):
+ x0 = self.branch0(x)
+ x1 = self.branch1(x)
+ out = torch.cat((x0, x1), 1)
+ out = self.conv2d(out)
+ out = out * self.scale + x
+ out = self.relu(out)
+ return out
+
+
+class Mixed_7a(nn.Module):
+
+ def __init__(self):
+ super(Mixed_7a, self).__init__()
+
+ self.branch0 = nn.Sequential(
+ BasicConv2d(1088, 256, kernel_size=1, stride=1),
+ BasicConv2d(256, 384, kernel_size=3, stride=2)
+ )
+
+ self.branch1 = nn.Sequential(
+ BasicConv2d(1088, 256, kernel_size=1, stride=1),
+ BasicConv2d(256, 288, kernel_size=3, stride=2)
+ )
+
+ self.branch2 = nn.Sequential(
+ BasicConv2d(1088, 256, kernel_size=1, stride=1),
+ BasicConv2d(256, 288, kernel_size=3, stride=1, padding=1),
+ BasicConv2d(288, 320, kernel_size=3, stride=2)
+ )
+
+ self.branch3 = nn.MaxPool2d(3, stride=2)
+
+ def forward(self, x):
+ x0 = self.branch0(x)
+ x1 = self.branch1(x)
+ x2 = self.branch2(x)
+ x3 = self.branch3(x)
+ out = torch.cat((x0, x1, x2, x3), 1)
+ return out
+
+
+class Block8(nn.Module):
+
+ def __init__(self, scale=1.0, noReLU=False):
+ super(Block8, self).__init__()
+
+ self.scale = scale
+ self.noReLU = noReLU
+
+ self.branch0 = BasicConv2d(2080, 192, kernel_size=1, stride=1)
+
+ self.branch1 = nn.Sequential(
+ BasicConv2d(2080, 192, kernel_size=1, stride=1),
+ BasicConv2d(
+ 192, 224, kernel_size=(1, 3), stride=1, padding=(0, 1)
+ ),
+ BasicConv2d(
+ 224, 256, kernel_size=(3, 1), stride=1, padding=(1, 0)
+ )
+ )
+
+ self.conv2d = nn.Conv2d(448, 2080, kernel_size=1, stride=1)
+ if not self.noReLU:
+ self.relu = nn.ReLU(inplace=False)
+
+ def forward(self, x):
+ x0 = self.branch0(x)
+ x1 = self.branch1(x)
+ out = torch.cat((x0, x1), 1)
+ out = self.conv2d(out)
+ out = out * self.scale + x
+ if not self.noReLU:
+ out = self.relu(out)
+ return out
+
+
+# ----------------
+# Model Definition
+# ----------------
+class InceptionResNetV2(nn.Module):
+ """Inception-ResNet-V2.
+
+ Reference:
+ Szegedy et al. Inception-v4, Inception-ResNet and the Impact of Residual
+ Connections on Learning. AAAI 2017.
+
+ Public keys:
+ - ``inceptionresnetv2``: Inception-ResNet-V2.
+ """
+
+ def __init__(self, num_classes, loss='softmax', **kwargs):
+ super(InceptionResNetV2, self).__init__()
+ self.loss = loss
+
+ # Modules
+ self.conv2d_1a = BasicConv2d(3, 32, kernel_size=3, stride=2)
+ self.conv2d_2a = BasicConv2d(32, 32, kernel_size=3, stride=1)
+ self.conv2d_2b = BasicConv2d(
+ 32, 64, kernel_size=3, stride=1, padding=1
+ )
+ self.maxpool_3a = nn.MaxPool2d(3, stride=2)
+ self.conv2d_3b = BasicConv2d(64, 80, kernel_size=1, stride=1)
+ self.conv2d_4a = BasicConv2d(80, 192, kernel_size=3, stride=1)
+ self.maxpool_5a = nn.MaxPool2d(3, stride=2)
+ self.mixed_5b = Mixed_5b()
+ self.repeat = nn.Sequential(
+ Block35(scale=0.17), Block35(scale=0.17), Block35(scale=0.17),
+ Block35(scale=0.17), Block35(scale=0.17), Block35(scale=0.17),
+ Block35(scale=0.17), Block35(scale=0.17), Block35(scale=0.17),
+ Block35(scale=0.17)
+ )
+ self.mixed_6a = Mixed_6a()
+ self.repeat_1 = nn.Sequential(
+ Block17(scale=0.10), Block17(scale=0.10), Block17(scale=0.10),
+ Block17(scale=0.10), Block17(scale=0.10), Block17(scale=0.10),
+ Block17(scale=0.10), Block17(scale=0.10), Block17(scale=0.10),
+ Block17(scale=0.10), Block17(scale=0.10), Block17(scale=0.10),
+ Block17(scale=0.10), Block17(scale=0.10), Block17(scale=0.10),
+ Block17(scale=0.10), Block17(scale=0.10), Block17(scale=0.10),
+ Block17(scale=0.10), Block17(scale=0.10)
+ )
+ self.mixed_7a = Mixed_7a()
+ self.repeat_2 = nn.Sequential(
+ Block8(scale=0.20), Block8(scale=0.20), Block8(scale=0.20),
+ Block8(scale=0.20), Block8(scale=0.20), Block8(scale=0.20),
+ Block8(scale=0.20), Block8(scale=0.20), Block8(scale=0.20)
+ )
+
+ self.block8 = Block8(noReLU=True)
+ self.conv2d_7b = BasicConv2d(2080, 1536, kernel_size=1, stride=1)
+ self.global_avgpool = nn.AdaptiveAvgPool2d(1)
+ self.classifier = nn.Linear(1536, num_classes)
+
+ def load_imagenet_weights(self):
+ settings = pretrained_settings['inceptionresnetv2']['imagenet']
+ pretrain_dict = model_zoo.load_url(settings['url'])
+ model_dict = self.state_dict()
+ pretrain_dict = {
+ k: v
+ for k, v in pretrain_dict.items()
+ if k in model_dict and model_dict[k].size() == v.size()
+ }
+ model_dict.update(pretrain_dict)
+ self.load_state_dict(model_dict)
+
+ def featuremaps(self, x):
+ x = self.conv2d_1a(x)
+ x = self.conv2d_2a(x)
+ x = self.conv2d_2b(x)
+ x = self.maxpool_3a(x)
+ x = self.conv2d_3b(x)
+ x = self.conv2d_4a(x)
+ x = self.maxpool_5a(x)
+ x = self.mixed_5b(x)
+ x = self.repeat(x)
+ x = self.mixed_6a(x)
+ x = self.repeat_1(x)
+ x = self.mixed_7a(x)
+ x = self.repeat_2(x)
+ x = self.block8(x)
+ x = self.conv2d_7b(x)
+ return x
+
+ def forward(self, x):
+ f = self.featuremaps(x)
+ v = self.global_avgpool(f)
+ v = v.view(v.size(0), -1)
+
+ if not self.training:
+ return v
+
+ y = self.classifier(v)
+
+ if self.loss == 'softmax':
+ return y
+ elif self.loss == 'triplet':
+ return y, v
+ else:
+ raise KeyError('Unsupported loss: {}'.format(self.loss))
+
+
+def inceptionresnetv2(num_classes, loss='softmax', pretrained=True, **kwargs):
+ model = InceptionResNetV2(num_classes=num_classes, loss=loss, **kwargs)
+ if pretrained:
+ model.load_imagenet_weights()
+ return model
diff --git a/trackers/strong_sort/deep/models/inceptionv4.py b/trackers/strong_sort/deep/models/inceptionv4.py
new file mode 100644
index 0000000000000000000000000000000000000000..b14916f140712298866c943ebdb4ebad67d72fc4
--- /dev/null
+++ b/trackers/strong_sort/deep/models/inceptionv4.py
@@ -0,0 +1,381 @@
+from __future__ import division, absolute_import
+import torch
+import torch.nn as nn
+import torch.utils.model_zoo as model_zoo
+
+__all__ = ['inceptionv4']
+"""
+Code imported from https://github.com/Cadene/pretrained-models.pytorch
+"""
+
+pretrained_settings = {
+ 'inceptionv4': {
+ 'imagenet': {
+ 'url':
+ 'http://data.lip6.fr/cadene/pretrainedmodels/inceptionv4-8e4777a0.pth',
+ 'input_space': 'RGB',
+ 'input_size': [3, 299, 299],
+ 'input_range': [0, 1],
+ 'mean': [0.5, 0.5, 0.5],
+ 'std': [0.5, 0.5, 0.5],
+ 'num_classes': 1000
+ },
+ 'imagenet+background': {
+ 'url':
+ 'http://data.lip6.fr/cadene/pretrainedmodels/inceptionv4-8e4777a0.pth',
+ 'input_space': 'RGB',
+ 'input_size': [3, 299, 299],
+ 'input_range': [0, 1],
+ 'mean': [0.5, 0.5, 0.5],
+ 'std': [0.5, 0.5, 0.5],
+ 'num_classes': 1001
+ }
+ }
+}
+
+
+class BasicConv2d(nn.Module):
+
+ def __init__(self, in_planes, out_planes, kernel_size, stride, padding=0):
+ super(BasicConv2d, self).__init__()
+ self.conv = nn.Conv2d(
+ in_planes,
+ out_planes,
+ kernel_size=kernel_size,
+ stride=stride,
+ padding=padding,
+ bias=False
+ ) # verify bias false
+ self.bn = nn.BatchNorm2d(
+ out_planes,
+ eps=0.001, # value found in tensorflow
+ momentum=0.1, # default pytorch value
+ affine=True
+ )
+ self.relu = nn.ReLU(inplace=True)
+
+ def forward(self, x):
+ x = self.conv(x)
+ x = self.bn(x)
+ x = self.relu(x)
+ return x
+
+
+class Mixed_3a(nn.Module):
+
+ def __init__(self):
+ super(Mixed_3a, self).__init__()
+ self.maxpool = nn.MaxPool2d(3, stride=2)
+ self.conv = BasicConv2d(64, 96, kernel_size=3, stride=2)
+
+ def forward(self, x):
+ x0 = self.maxpool(x)
+ x1 = self.conv(x)
+ out = torch.cat((x0, x1), 1)
+ return out
+
+
+class Mixed_4a(nn.Module):
+
+ def __init__(self):
+ super(Mixed_4a, self).__init__()
+
+ self.branch0 = nn.Sequential(
+ BasicConv2d(160, 64, kernel_size=1, stride=1),
+ BasicConv2d(64, 96, kernel_size=3, stride=1)
+ )
+
+ self.branch1 = nn.Sequential(
+ BasicConv2d(160, 64, kernel_size=1, stride=1),
+ BasicConv2d(64, 64, kernel_size=(1, 7), stride=1, padding=(0, 3)),
+ BasicConv2d(64, 64, kernel_size=(7, 1), stride=1, padding=(3, 0)),
+ BasicConv2d(64, 96, kernel_size=(3, 3), stride=1)
+ )
+
+ def forward(self, x):
+ x0 = self.branch0(x)
+ x1 = self.branch1(x)
+ out = torch.cat((x0, x1), 1)
+ return out
+
+
+class Mixed_5a(nn.Module):
+
+ def __init__(self):
+ super(Mixed_5a, self).__init__()
+ self.conv = BasicConv2d(192, 192, kernel_size=3, stride=2)
+ self.maxpool = nn.MaxPool2d(3, stride=2)
+
+ def forward(self, x):
+ x0 = self.conv(x)
+ x1 = self.maxpool(x)
+ out = torch.cat((x0, x1), 1)
+ return out
+
+
+class Inception_A(nn.Module):
+
+ def __init__(self):
+ super(Inception_A, self).__init__()
+ self.branch0 = BasicConv2d(384, 96, kernel_size=1, stride=1)
+
+ self.branch1 = nn.Sequential(
+ BasicConv2d(384, 64, kernel_size=1, stride=1),
+ BasicConv2d(64, 96, kernel_size=3, stride=1, padding=1)
+ )
+
+ self.branch2 = nn.Sequential(
+ BasicConv2d(384, 64, kernel_size=1, stride=1),
+ BasicConv2d(64, 96, kernel_size=3, stride=1, padding=1),
+ BasicConv2d(96, 96, kernel_size=3, stride=1, padding=1)
+ )
+
+ self.branch3 = nn.Sequential(
+ nn.AvgPool2d(3, stride=1, padding=1, count_include_pad=False),
+ BasicConv2d(384, 96, kernel_size=1, stride=1)
+ )
+
+ def forward(self, x):
+ x0 = self.branch0(x)
+ x1 = self.branch1(x)
+ x2 = self.branch2(x)
+ x3 = self.branch3(x)
+ out = torch.cat((x0, x1, x2, x3), 1)
+ return out
+
+
+class Reduction_A(nn.Module):
+
+ def __init__(self):
+ super(Reduction_A, self).__init__()
+ self.branch0 = BasicConv2d(384, 384, kernel_size=3, stride=2)
+
+ self.branch1 = nn.Sequential(
+ BasicConv2d(384, 192, kernel_size=1, stride=1),
+ BasicConv2d(192, 224, kernel_size=3, stride=1, padding=1),
+ BasicConv2d(224, 256, kernel_size=3, stride=2)
+ )
+
+ self.branch2 = nn.MaxPool2d(3, stride=2)
+
+ def forward(self, x):
+ x0 = self.branch0(x)
+ x1 = self.branch1(x)
+ x2 = self.branch2(x)
+ out = torch.cat((x0, x1, x2), 1)
+ return out
+
+
+class Inception_B(nn.Module):
+
+ def __init__(self):
+ super(Inception_B, self).__init__()
+ self.branch0 = BasicConv2d(1024, 384, kernel_size=1, stride=1)
+
+ self.branch1 = nn.Sequential(
+ BasicConv2d(1024, 192, kernel_size=1, stride=1),
+ BasicConv2d(
+ 192, 224, kernel_size=(1, 7), stride=1, padding=(0, 3)
+ ),
+ BasicConv2d(
+ 224, 256, kernel_size=(7, 1), stride=1, padding=(3, 0)
+ )
+ )
+
+ self.branch2 = nn.Sequential(
+ BasicConv2d(1024, 192, kernel_size=1, stride=1),
+ BasicConv2d(
+ 192, 192, kernel_size=(7, 1), stride=1, padding=(3, 0)
+ ),
+ BasicConv2d(
+ 192, 224, kernel_size=(1, 7), stride=1, padding=(0, 3)
+ ),
+ BasicConv2d(
+ 224, 224, kernel_size=(7, 1), stride=1, padding=(3, 0)
+ ),
+ BasicConv2d(
+ 224, 256, kernel_size=(1, 7), stride=1, padding=(0, 3)
+ )
+ )
+
+ self.branch3 = nn.Sequential(
+ nn.AvgPool2d(3, stride=1, padding=1, count_include_pad=False),
+ BasicConv2d(1024, 128, kernel_size=1, stride=1)
+ )
+
+ def forward(self, x):
+ x0 = self.branch0(x)
+ x1 = self.branch1(x)
+ x2 = self.branch2(x)
+ x3 = self.branch3(x)
+ out = torch.cat((x0, x1, x2, x3), 1)
+ return out
+
+
+class Reduction_B(nn.Module):
+
+ def __init__(self):
+ super(Reduction_B, self).__init__()
+
+ self.branch0 = nn.Sequential(
+ BasicConv2d(1024, 192, kernel_size=1, stride=1),
+ BasicConv2d(192, 192, kernel_size=3, stride=2)
+ )
+
+ self.branch1 = nn.Sequential(
+ BasicConv2d(1024, 256, kernel_size=1, stride=1),
+ BasicConv2d(
+ 256, 256, kernel_size=(1, 7), stride=1, padding=(0, 3)
+ ),
+ BasicConv2d(
+ 256, 320, kernel_size=(7, 1), stride=1, padding=(3, 0)
+ ), BasicConv2d(320, 320, kernel_size=3, stride=2)
+ )
+
+ self.branch2 = nn.MaxPool2d(3, stride=2)
+
+ def forward(self, x):
+ x0 = self.branch0(x)
+ x1 = self.branch1(x)
+ x2 = self.branch2(x)
+ out = torch.cat((x0, x1, x2), 1)
+ return out
+
+
+class Inception_C(nn.Module):
+
+ def __init__(self):
+ super(Inception_C, self).__init__()
+
+ self.branch0 = BasicConv2d(1536, 256, kernel_size=1, stride=1)
+
+ self.branch1_0 = BasicConv2d(1536, 384, kernel_size=1, stride=1)
+ self.branch1_1a = BasicConv2d(
+ 384, 256, kernel_size=(1, 3), stride=1, padding=(0, 1)
+ )
+ self.branch1_1b = BasicConv2d(
+ 384, 256, kernel_size=(3, 1), stride=1, padding=(1, 0)
+ )
+
+ self.branch2_0 = BasicConv2d(1536, 384, kernel_size=1, stride=1)
+ self.branch2_1 = BasicConv2d(
+ 384, 448, kernel_size=(3, 1), stride=1, padding=(1, 0)
+ )
+ self.branch2_2 = BasicConv2d(
+ 448, 512, kernel_size=(1, 3), stride=1, padding=(0, 1)
+ )
+ self.branch2_3a = BasicConv2d(
+ 512, 256, kernel_size=(1, 3), stride=1, padding=(0, 1)
+ )
+ self.branch2_3b = BasicConv2d(
+ 512, 256, kernel_size=(3, 1), stride=1, padding=(1, 0)
+ )
+
+ self.branch3 = nn.Sequential(
+ nn.AvgPool2d(3, stride=1, padding=1, count_include_pad=False),
+ BasicConv2d(1536, 256, kernel_size=1, stride=1)
+ )
+
+ def forward(self, x):
+ x0 = self.branch0(x)
+
+ x1_0 = self.branch1_0(x)
+ x1_1a = self.branch1_1a(x1_0)
+ x1_1b = self.branch1_1b(x1_0)
+ x1 = torch.cat((x1_1a, x1_1b), 1)
+
+ x2_0 = self.branch2_0(x)
+ x2_1 = self.branch2_1(x2_0)
+ x2_2 = self.branch2_2(x2_1)
+ x2_3a = self.branch2_3a(x2_2)
+ x2_3b = self.branch2_3b(x2_2)
+ x2 = torch.cat((x2_3a, x2_3b), 1)
+
+ x3 = self.branch3(x)
+
+ out = torch.cat((x0, x1, x2, x3), 1)
+ return out
+
+
+class InceptionV4(nn.Module):
+ """Inception-v4.
+
+ Reference:
+ Szegedy et al. Inception-v4, Inception-ResNet and the Impact of Residual
+ Connections on Learning. AAAI 2017.
+
+ Public keys:
+ - ``inceptionv4``: InceptionV4.
+ """
+
+ def __init__(self, num_classes, loss, **kwargs):
+ super(InceptionV4, self).__init__()
+ self.loss = loss
+
+ self.features = nn.Sequential(
+ BasicConv2d(3, 32, kernel_size=3, stride=2),
+ BasicConv2d(32, 32, kernel_size=3, stride=1),
+ BasicConv2d(32, 64, kernel_size=3, stride=1, padding=1),
+ Mixed_3a(),
+ Mixed_4a(),
+ Mixed_5a(),
+ Inception_A(),
+ Inception_A(),
+ Inception_A(),
+ Inception_A(),
+ Reduction_A(), # Mixed_6a
+ Inception_B(),
+ Inception_B(),
+ Inception_B(),
+ Inception_B(),
+ Inception_B(),
+ Inception_B(),
+ Inception_B(),
+ Reduction_B(), # Mixed_7a
+ Inception_C(),
+ Inception_C(),
+ Inception_C()
+ )
+ self.global_avgpool = nn.AdaptiveAvgPool2d(1)
+ self.classifier = nn.Linear(1536, num_classes)
+
+ def forward(self, x):
+ f = self.features(x)
+ v = self.global_avgpool(f)
+ v = v.view(v.size(0), -1)
+
+ if not self.training:
+ return v
+
+ y = self.classifier(v)
+
+ if self.loss == 'softmax':
+ return y
+ elif self.loss == 'triplet':
+ return y, v
+ else:
+ raise KeyError('Unsupported loss: {}'.format(self.loss))
+
+
+def init_pretrained_weights(model, model_url):
+ """Initializes model with pretrained weights.
+
+ Layers that don't match with pretrained layers in name or size are kept unchanged.
+ """
+ pretrain_dict = model_zoo.load_url(model_url)
+ model_dict = model.state_dict()
+ pretrain_dict = {
+ k: v
+ for k, v in pretrain_dict.items()
+ if k in model_dict and model_dict[k].size() == v.size()
+ }
+ model_dict.update(pretrain_dict)
+ model.load_state_dict(model_dict)
+
+
+def inceptionv4(num_classes, loss='softmax', pretrained=True, **kwargs):
+ model = InceptionV4(num_classes, loss, **kwargs)
+ if pretrained:
+ model_url = pretrained_settings['inceptionv4']['imagenet']['url']
+ init_pretrained_weights(model, model_url)
+ return model
diff --git a/trackers/strong_sort/deep/models/mlfn.py b/trackers/strong_sort/deep/models/mlfn.py
new file mode 100644
index 0000000000000000000000000000000000000000..ac7e126b073db6a710fc41e62624127ca91ec131
--- /dev/null
+++ b/trackers/strong_sort/deep/models/mlfn.py
@@ -0,0 +1,269 @@
+from __future__ import division, absolute_import
+import torch
+import torch.utils.model_zoo as model_zoo
+from torch import nn
+from torch.nn import functional as F
+
+__all__ = ['mlfn']
+
+model_urls = {
+ # training epoch = 5, top1 = 51.6
+ 'imagenet':
+ 'https://mega.nz/#!YHxAhaxC!yu9E6zWl0x5zscSouTdbZu8gdFFytDdl-RAdD2DEfpk',
+}
+
+
+class MLFNBlock(nn.Module):
+
+ def __init__(
+ self, in_channels, out_channels, stride, fsm_channels, groups=32
+ ):
+ super(MLFNBlock, self).__init__()
+ self.groups = groups
+ mid_channels = out_channels // 2
+
+ # Factor Modules
+ self.fm_conv1 = nn.Conv2d(in_channels, mid_channels, 1, bias=False)
+ self.fm_bn1 = nn.BatchNorm2d(mid_channels)
+ self.fm_conv2 = nn.Conv2d(
+ mid_channels,
+ mid_channels,
+ 3,
+ stride=stride,
+ padding=1,
+ bias=False,
+ groups=self.groups
+ )
+ self.fm_bn2 = nn.BatchNorm2d(mid_channels)
+ self.fm_conv3 = nn.Conv2d(mid_channels, out_channels, 1, bias=False)
+ self.fm_bn3 = nn.BatchNorm2d(out_channels)
+
+ # Factor Selection Module
+ self.fsm = nn.Sequential(
+ nn.AdaptiveAvgPool2d(1),
+ nn.Conv2d(in_channels, fsm_channels[0], 1),
+ nn.BatchNorm2d(fsm_channels[0]),
+ nn.ReLU(inplace=True),
+ nn.Conv2d(fsm_channels[0], fsm_channels[1], 1),
+ nn.BatchNorm2d(fsm_channels[1]),
+ nn.ReLU(inplace=True),
+ nn.Conv2d(fsm_channels[1], self.groups, 1),
+ nn.BatchNorm2d(self.groups),
+ nn.Sigmoid(),
+ )
+
+ self.downsample = None
+ if in_channels != out_channels or stride > 1:
+ self.downsample = nn.Sequential(
+ nn.Conv2d(
+ in_channels, out_channels, 1, stride=stride, bias=False
+ ),
+ nn.BatchNorm2d(out_channels),
+ )
+
+ def forward(self, x):
+ residual = x
+ s = self.fsm(x)
+
+ # reduce dimension
+ x = self.fm_conv1(x)
+ x = self.fm_bn1(x)
+ x = F.relu(x, inplace=True)
+
+ # group convolution
+ x = self.fm_conv2(x)
+ x = self.fm_bn2(x)
+ x = F.relu(x, inplace=True)
+
+ # factor selection
+ b, c = x.size(0), x.size(1)
+ n = c // self.groups
+ ss = s.repeat(1, n, 1, 1) # from (b, g, 1, 1) to (b, g*n=c, 1, 1)
+ ss = ss.view(b, n, self.groups, 1, 1)
+ ss = ss.permute(0, 2, 1, 3, 4).contiguous()
+ ss = ss.view(b, c, 1, 1)
+ x = ss * x
+
+ # recover dimension
+ x = self.fm_conv3(x)
+ x = self.fm_bn3(x)
+ x = F.relu(x, inplace=True)
+
+ if self.downsample is not None:
+ residual = self.downsample(residual)
+
+ return F.relu(residual + x, inplace=True), s
+
+
+class MLFN(nn.Module):
+ """Multi-Level Factorisation Net.
+
+ Reference:
+ Chang et al. Multi-Level Factorisation Net for
+ Person Re-Identification. CVPR 2018.
+
+ Public keys:
+ - ``mlfn``: MLFN (Multi-Level Factorisation Net).
+ """
+
+ def __init__(
+ self,
+ num_classes,
+ loss='softmax',
+ groups=32,
+ channels=[64, 256, 512, 1024, 2048],
+ embed_dim=1024,
+ **kwargs
+ ):
+ super(MLFN, self).__init__()
+ self.loss = loss
+ self.groups = groups
+
+ # first convolutional layer
+ self.conv1 = nn.Conv2d(3, channels[0], 7, stride=2, padding=3)
+ self.bn1 = nn.BatchNorm2d(channels[0])
+ self.maxpool = nn.MaxPool2d(3, stride=2, padding=1)
+
+ # main body
+ self.feature = nn.ModuleList(
+ [
+ # layer 1-3
+ MLFNBlock(channels[0], channels[1], 1, [128, 64], self.groups),
+ MLFNBlock(channels[1], channels[1], 1, [128, 64], self.groups),
+ MLFNBlock(channels[1], channels[1], 1, [128, 64], self.groups),
+ # layer 4-7
+ MLFNBlock(
+ channels[1], channels[2], 2, [256, 128], self.groups
+ ),
+ MLFNBlock(
+ channels[2], channels[2], 1, [256, 128], self.groups
+ ),
+ MLFNBlock(
+ channels[2], channels[2], 1, [256, 128], self.groups
+ ),
+ MLFNBlock(
+ channels[2], channels[2], 1, [256, 128], self.groups
+ ),
+ # layer 8-13
+ MLFNBlock(
+ channels[2], channels[3], 2, [512, 128], self.groups
+ ),
+ MLFNBlock(
+ channels[3], channels[3], 1, [512, 128], self.groups
+ ),
+ MLFNBlock(
+ channels[3], channels[3], 1, [512, 128], self.groups
+ ),
+ MLFNBlock(
+ channels[3], channels[3], 1, [512, 128], self.groups
+ ),
+ MLFNBlock(
+ channels[3], channels[3], 1, [512, 128], self.groups
+ ),
+ MLFNBlock(
+ channels[3], channels[3], 1, [512, 128], self.groups
+ ),
+ # layer 14-16
+ MLFNBlock(
+ channels[3], channels[4], 2, [512, 128], self.groups
+ ),
+ MLFNBlock(
+ channels[4], channels[4], 1, [512, 128], self.groups
+ ),
+ MLFNBlock(
+ channels[4], channels[4], 1, [512, 128], self.groups
+ ),
+ ]
+ )
+ self.global_avgpool = nn.AdaptiveAvgPool2d(1)
+
+ # projection functions
+ self.fc_x = nn.Sequential(
+ nn.Conv2d(channels[4], embed_dim, 1, bias=False),
+ nn.BatchNorm2d(embed_dim),
+ nn.ReLU(inplace=True),
+ )
+ self.fc_s = nn.Sequential(
+ nn.Conv2d(self.groups * 16, embed_dim, 1, bias=False),
+ nn.BatchNorm2d(embed_dim),
+ nn.ReLU(inplace=True),
+ )
+
+ self.classifier = nn.Linear(embed_dim, num_classes)
+
+ self.init_params()
+
+ def init_params(self):
+ for m in self.modules():
+ if isinstance(m, nn.Conv2d):
+ nn.init.kaiming_normal_(
+ m.weight, mode='fan_out', nonlinearity='relu'
+ )
+ if m.bias is not None:
+ nn.init.constant_(m.bias, 0)
+ elif isinstance(m, nn.BatchNorm2d):
+ nn.init.constant_(m.weight, 1)
+ nn.init.constant_(m.bias, 0)
+ elif isinstance(m, nn.Linear):
+ nn.init.normal_(m.weight, 0, 0.01)
+ if m.bias is not None:
+ nn.init.constant_(m.bias, 0)
+
+ def forward(self, x):
+ x = self.conv1(x)
+ x = self.bn1(x)
+ x = F.relu(x, inplace=True)
+ x = self.maxpool(x)
+
+ s_hat = []
+ for block in self.feature:
+ x, s = block(x)
+ s_hat.append(s)
+ s_hat = torch.cat(s_hat, 1)
+
+ x = self.global_avgpool(x)
+ x = self.fc_x(x)
+ s_hat = self.fc_s(s_hat)
+
+ v = (x+s_hat) * 0.5
+ v = v.view(v.size(0), -1)
+
+ if not self.training:
+ return v
+
+ y = self.classifier(v)
+
+ if self.loss == 'softmax':
+ return y
+ elif self.loss == 'triplet':
+ return y, v
+ else:
+ raise KeyError('Unsupported loss: {}'.format(self.loss))
+
+
+def init_pretrained_weights(model, model_url):
+ """Initializes model with pretrained weights.
+
+ Layers that don't match with pretrained layers in name or size are kept unchanged.
+ """
+ pretrain_dict = model_zoo.load_url(model_url)
+ model_dict = model.state_dict()
+ pretrain_dict = {
+ k: v
+ for k, v in pretrain_dict.items()
+ if k in model_dict and model_dict[k].size() == v.size()
+ }
+ model_dict.update(pretrain_dict)
+ model.load_state_dict(model_dict)
+
+
+def mlfn(num_classes, loss='softmax', pretrained=True, **kwargs):
+ model = MLFN(num_classes, loss, **kwargs)
+ if pretrained:
+ # init_pretrained_weights(model, model_urls['imagenet'])
+ import warnings
+ warnings.warn(
+ 'The imagenet pretrained weights need to be manually downloaded from {}'
+ .format(model_urls['imagenet'])
+ )
+ return model
diff --git a/trackers/strong_sort/deep/models/mobilenetv2.py b/trackers/strong_sort/deep/models/mobilenetv2.py
new file mode 100644
index 0000000000000000000000000000000000000000..c451ef84e726ebc8d4c8e47253f335494eb801c9
--- /dev/null
+++ b/trackers/strong_sort/deep/models/mobilenetv2.py
@@ -0,0 +1,274 @@
+from __future__ import division, absolute_import
+import torch.utils.model_zoo as model_zoo
+from torch import nn
+from torch.nn import functional as F
+
+__all__ = ['mobilenetv2_x1_0', 'mobilenetv2_x1_4']
+
+model_urls = {
+ # 1.0: top-1 71.3
+ 'mobilenetv2_x1_0':
+ 'https://mega.nz/#!NKp2wAIA!1NH1pbNzY_M2hVk_hdsxNM1NUOWvvGPHhaNr-fASF6c',
+ # 1.4: top-1 73.9
+ 'mobilenetv2_x1_4':
+ 'https://mega.nz/#!RGhgEIwS!xN2s2ZdyqI6vQ3EwgmRXLEW3khr9tpXg96G9SUJugGk',
+}
+
+
+class ConvBlock(nn.Module):
+ """Basic convolutional block.
+
+ convolution (bias discarded) + batch normalization + relu6.
+
+ Args:
+ in_c (int): number of input channels.
+ out_c (int): number of output channels.
+ k (int or tuple): kernel size.
+ s (int or tuple): stride.
+ p (int or tuple): padding.
+ g (int): number of blocked connections from input channels
+ to output channels (default: 1).
+ """
+
+ def __init__(self, in_c, out_c, k, s=1, p=0, g=1):
+ super(ConvBlock, self).__init__()
+ self.conv = nn.Conv2d(
+ in_c, out_c, k, stride=s, padding=p, bias=False, groups=g
+ )
+ self.bn = nn.BatchNorm2d(out_c)
+
+ def forward(self, x):
+ return F.relu6(self.bn(self.conv(x)))
+
+
+class Bottleneck(nn.Module):
+
+ def __init__(self, in_channels, out_channels, expansion_factor, stride=1):
+ super(Bottleneck, self).__init__()
+ mid_channels = in_channels * expansion_factor
+ self.use_residual = stride == 1 and in_channels == out_channels
+ self.conv1 = ConvBlock(in_channels, mid_channels, 1)
+ self.dwconv2 = ConvBlock(
+ mid_channels, mid_channels, 3, stride, 1, g=mid_channels
+ )
+ self.conv3 = nn.Sequential(
+ nn.Conv2d(mid_channels, out_channels, 1, bias=False),
+ nn.BatchNorm2d(out_channels),
+ )
+
+ def forward(self, x):
+ m = self.conv1(x)
+ m = self.dwconv2(m)
+ m = self.conv3(m)
+ if self.use_residual:
+ return x + m
+ else:
+ return m
+
+
+class MobileNetV2(nn.Module):
+ """MobileNetV2.
+
+ Reference:
+ Sandler et al. MobileNetV2: Inverted Residuals and
+ Linear Bottlenecks. CVPR 2018.
+
+ Public keys:
+ - ``mobilenetv2_x1_0``: MobileNetV2 x1.0.
+ - ``mobilenetv2_x1_4``: MobileNetV2 x1.4.
+ """
+
+ def __init__(
+ self,
+ num_classes,
+ width_mult=1,
+ loss='softmax',
+ fc_dims=None,
+ dropout_p=None,
+ **kwargs
+ ):
+ super(MobileNetV2, self).__init__()
+ self.loss = loss
+ self.in_channels = int(32 * width_mult)
+ self.feature_dim = int(1280 * width_mult) if width_mult > 1 else 1280
+
+ # construct layers
+ self.conv1 = ConvBlock(3, self.in_channels, 3, s=2, p=1)
+ self.conv2 = self._make_layer(
+ Bottleneck, 1, int(16 * width_mult), 1, 1
+ )
+ self.conv3 = self._make_layer(
+ Bottleneck, 6, int(24 * width_mult), 2, 2
+ )
+ self.conv4 = self._make_layer(
+ Bottleneck, 6, int(32 * width_mult), 3, 2
+ )
+ self.conv5 = self._make_layer(
+ Bottleneck, 6, int(64 * width_mult), 4, 2
+ )
+ self.conv6 = self._make_layer(
+ Bottleneck, 6, int(96 * width_mult), 3, 1
+ )
+ self.conv7 = self._make_layer(
+ Bottleneck, 6, int(160 * width_mult), 3, 2
+ )
+ self.conv8 = self._make_layer(
+ Bottleneck, 6, int(320 * width_mult), 1, 1
+ )
+ self.conv9 = ConvBlock(self.in_channels, self.feature_dim, 1)
+
+ self.global_avgpool = nn.AdaptiveAvgPool2d(1)
+ self.fc = self._construct_fc_layer(
+ fc_dims, self.feature_dim, dropout_p
+ )
+ self.classifier = nn.Linear(self.feature_dim, num_classes)
+
+ self._init_params()
+
+ def _make_layer(self, block, t, c, n, s):
+ # t: expansion factor
+ # c: output channels
+ # n: number of blocks
+ # s: stride for first layer
+ layers = []
+ layers.append(block(self.in_channels, c, t, s))
+ self.in_channels = c
+ for i in range(1, n):
+ layers.append(block(self.in_channels, c, t))
+ return nn.Sequential(*layers)
+
+ def _construct_fc_layer(self, fc_dims, input_dim, dropout_p=None):
+ """Constructs fully connected layer.
+
+ Args:
+ fc_dims (list or tuple): dimensions of fc layers, if None, no fc layers are constructed
+ input_dim (int): input dimension
+ dropout_p (float): dropout probability, if None, dropout is unused
+ """
+ if fc_dims is None:
+ self.feature_dim = input_dim
+ return None
+
+ assert isinstance(
+ fc_dims, (list, tuple)
+ ), 'fc_dims must be either list or tuple, but got {}'.format(
+ type(fc_dims)
+ )
+
+ layers = []
+ for dim in fc_dims:
+ layers.append(nn.Linear(input_dim, dim))
+ layers.append(nn.BatchNorm1d(dim))
+ layers.append(nn.ReLU(inplace=True))
+ if dropout_p is not None:
+ layers.append(nn.Dropout(p=dropout_p))
+ input_dim = dim
+
+ self.feature_dim = fc_dims[-1]
+
+ return nn.Sequential(*layers)
+
+ def _init_params(self):
+ for m in self.modules():
+ if isinstance(m, nn.Conv2d):
+ nn.init.kaiming_normal_(
+ m.weight, mode='fan_out', nonlinearity='relu'
+ )
+ if m.bias is not None:
+ nn.init.constant_(m.bias, 0)
+ elif isinstance(m, nn.BatchNorm2d):
+ nn.init.constant_(m.weight, 1)
+ nn.init.constant_(m.bias, 0)
+ elif isinstance(m, nn.BatchNorm1d):
+ nn.init.constant_(m.weight, 1)
+ nn.init.constant_(m.bias, 0)
+ elif isinstance(m, nn.Linear):
+ nn.init.normal_(m.weight, 0, 0.01)
+ if m.bias is not None:
+ nn.init.constant_(m.bias, 0)
+
+ def featuremaps(self, x):
+ x = self.conv1(x)
+ x = self.conv2(x)
+ x = self.conv3(x)
+ x = self.conv4(x)
+ x = self.conv5(x)
+ x = self.conv6(x)
+ x = self.conv7(x)
+ x = self.conv8(x)
+ x = self.conv9(x)
+ return x
+
+ def forward(self, x):
+ f = self.featuremaps(x)
+ v = self.global_avgpool(f)
+ v = v.view(v.size(0), -1)
+
+ if self.fc is not None:
+ v = self.fc(v)
+
+ if not self.training:
+ return v
+
+ y = self.classifier(v)
+
+ if self.loss == 'softmax':
+ return y
+ elif self.loss == 'triplet':
+ return y, v
+ else:
+ raise KeyError("Unsupported loss: {}".format(self.loss))
+
+
+def init_pretrained_weights(model, model_url):
+ """Initializes model with pretrained weights.
+
+ Layers that don't match with pretrained layers in name or size are kept unchanged.
+ """
+ pretrain_dict = model_zoo.load_url(model_url)
+ model_dict = model.state_dict()
+ pretrain_dict = {
+ k: v
+ for k, v in pretrain_dict.items()
+ if k in model_dict and model_dict[k].size() == v.size()
+ }
+ model_dict.update(pretrain_dict)
+ model.load_state_dict(model_dict)
+
+
+def mobilenetv2_x1_0(num_classes, loss, pretrained=True, **kwargs):
+ model = MobileNetV2(
+ num_classes,
+ loss=loss,
+ width_mult=1,
+ fc_dims=None,
+ dropout_p=None,
+ **kwargs
+ )
+ if pretrained:
+ # init_pretrained_weights(model, model_urls['mobilenetv2_x1_0'])
+ import warnings
+ warnings.warn(
+ 'The imagenet pretrained weights need to be manually downloaded from {}'
+ .format(model_urls['mobilenetv2_x1_0'])
+ )
+ return model
+
+
+def mobilenetv2_x1_4(num_classes, loss, pretrained=True, **kwargs):
+ model = MobileNetV2(
+ num_classes,
+ loss=loss,
+ width_mult=1.4,
+ fc_dims=None,
+ dropout_p=None,
+ **kwargs
+ )
+ if pretrained:
+ # init_pretrained_weights(model, model_urls['mobilenetv2_x1_4'])
+ import warnings
+ warnings.warn(
+ 'The imagenet pretrained weights need to be manually downloaded from {}'
+ .format(model_urls['mobilenetv2_x1_4'])
+ )
+ return model
diff --git a/trackers/strong_sort/deep/models/mudeep.py b/trackers/strong_sort/deep/models/mudeep.py
new file mode 100644
index 0000000000000000000000000000000000000000..ddbca675b69fcf38523d8687d8c7b279ededd8d1
--- /dev/null
+++ b/trackers/strong_sort/deep/models/mudeep.py
@@ -0,0 +1,206 @@
+from __future__ import division, absolute_import
+import torch
+from torch import nn
+from torch.nn import functional as F
+
+__all__ = ['MuDeep']
+
+
+class ConvBlock(nn.Module):
+ """Basic convolutional block.
+
+ convolution + batch normalization + relu.
+
+ Args:
+ in_c (int): number of input channels.
+ out_c (int): number of output channels.
+ k (int or tuple): kernel size.
+ s (int or tuple): stride.
+ p (int or tuple): padding.
+ """
+
+ def __init__(self, in_c, out_c, k, s, p):
+ super(ConvBlock, self).__init__()
+ self.conv = nn.Conv2d(in_c, out_c, k, stride=s, padding=p)
+ self.bn = nn.BatchNorm2d(out_c)
+
+ def forward(self, x):
+ return F.relu(self.bn(self.conv(x)))
+
+
+class ConvLayers(nn.Module):
+ """Preprocessing layers."""
+
+ def __init__(self):
+ super(ConvLayers, self).__init__()
+ self.conv1 = ConvBlock(3, 48, k=3, s=1, p=1)
+ self.conv2 = ConvBlock(48, 96, k=3, s=1, p=1)
+ self.maxpool = nn.MaxPool2d(kernel_size=3, stride=2, padding=1)
+
+ def forward(self, x):
+ x = self.conv1(x)
+ x = self.conv2(x)
+ x = self.maxpool(x)
+ return x
+
+
+class MultiScaleA(nn.Module):
+ """Multi-scale stream layer A (Sec.3.1)"""
+
+ def __init__(self):
+ super(MultiScaleA, self).__init__()
+ self.stream1 = nn.Sequential(
+ ConvBlock(96, 96, k=1, s=1, p=0),
+ ConvBlock(96, 24, k=3, s=1, p=1),
+ )
+ self.stream2 = nn.Sequential(
+ nn.AvgPool2d(kernel_size=3, stride=1, padding=1),
+ ConvBlock(96, 24, k=1, s=1, p=0),
+ )
+ self.stream3 = ConvBlock(96, 24, k=1, s=1, p=0)
+ self.stream4 = nn.Sequential(
+ ConvBlock(96, 16, k=1, s=1, p=0),
+ ConvBlock(16, 24, k=3, s=1, p=1),
+ ConvBlock(24, 24, k=3, s=1, p=1),
+ )
+
+ def forward(self, x):
+ s1 = self.stream1(x)
+ s2 = self.stream2(x)
+ s3 = self.stream3(x)
+ s4 = self.stream4(x)
+ y = torch.cat([s1, s2, s3, s4], dim=1)
+ return y
+
+
+class Reduction(nn.Module):
+ """Reduction layer (Sec.3.1)"""
+
+ def __init__(self):
+ super(Reduction, self).__init__()
+ self.stream1 = nn.MaxPool2d(kernel_size=3, stride=2, padding=1)
+ self.stream2 = ConvBlock(96, 96, k=3, s=2, p=1)
+ self.stream3 = nn.Sequential(
+ ConvBlock(96, 48, k=1, s=1, p=0),
+ ConvBlock(48, 56, k=3, s=1, p=1),
+ ConvBlock(56, 64, k=3, s=2, p=1),
+ )
+
+ def forward(self, x):
+ s1 = self.stream1(x)
+ s2 = self.stream2(x)
+ s3 = self.stream3(x)
+ y = torch.cat([s1, s2, s3], dim=1)
+ return y
+
+
+class MultiScaleB(nn.Module):
+ """Multi-scale stream layer B (Sec.3.1)"""
+
+ def __init__(self):
+ super(MultiScaleB, self).__init__()
+ self.stream1 = nn.Sequential(
+ nn.AvgPool2d(kernel_size=3, stride=1, padding=1),
+ ConvBlock(256, 256, k=1, s=1, p=0),
+ )
+ self.stream2 = nn.Sequential(
+ ConvBlock(256, 64, k=1, s=1, p=0),
+ ConvBlock(64, 128, k=(1, 3), s=1, p=(0, 1)),
+ ConvBlock(128, 256, k=(3, 1), s=1, p=(1, 0)),
+ )
+ self.stream3 = ConvBlock(256, 256, k=1, s=1, p=0)
+ self.stream4 = nn.Sequential(
+ ConvBlock(256, 64, k=1, s=1, p=0),
+ ConvBlock(64, 64, k=(1, 3), s=1, p=(0, 1)),
+ ConvBlock(64, 128, k=(3, 1), s=1, p=(1, 0)),
+ ConvBlock(128, 128, k=(1, 3), s=1, p=(0, 1)),
+ ConvBlock(128, 256, k=(3, 1), s=1, p=(1, 0)),
+ )
+
+ def forward(self, x):
+ s1 = self.stream1(x)
+ s2 = self.stream2(x)
+ s3 = self.stream3(x)
+ s4 = self.stream4(x)
+ return s1, s2, s3, s4
+
+
+class Fusion(nn.Module):
+ """Saliency-based learning fusion layer (Sec.3.2)"""
+
+ def __init__(self):
+ super(Fusion, self).__init__()
+ self.a1 = nn.Parameter(torch.rand(1, 256, 1, 1))
+ self.a2 = nn.Parameter(torch.rand(1, 256, 1, 1))
+ self.a3 = nn.Parameter(torch.rand(1, 256, 1, 1))
+ self.a4 = nn.Parameter(torch.rand(1, 256, 1, 1))
+
+ # We add an average pooling layer to reduce the spatial dimension
+ # of feature maps, which differs from the original paper.
+ self.avgpool = nn.AvgPool2d(kernel_size=4, stride=4, padding=0)
+
+ def forward(self, x1, x2, x3, x4):
+ s1 = self.a1.expand_as(x1) * x1
+ s2 = self.a2.expand_as(x2) * x2
+ s3 = self.a3.expand_as(x3) * x3
+ s4 = self.a4.expand_as(x4) * x4
+ y = self.avgpool(s1 + s2 + s3 + s4)
+ return y
+
+
+class MuDeep(nn.Module):
+ """Multiscale deep neural network.
+
+ Reference:
+ Qian et al. Multi-scale Deep Learning Architectures
+ for Person Re-identification. ICCV 2017.
+
+ Public keys:
+ - ``mudeep``: Multiscale deep neural network.
+ """
+
+ def __init__(self, num_classes, loss='softmax', **kwargs):
+ super(MuDeep, self).__init__()
+ self.loss = loss
+
+ self.block1 = ConvLayers()
+ self.block2 = MultiScaleA()
+ self.block3 = Reduction()
+ self.block4 = MultiScaleB()
+ self.block5 = Fusion()
+
+ # Due to this fully connected layer, input image has to be fixed
+ # in shape, i.e. (3, 256, 128), such that the last convolutional feature
+ # maps are of shape (256, 16, 8). If input shape is changed,
+ # the input dimension of this layer has to be changed accordingly.
+ self.fc = nn.Sequential(
+ nn.Linear(256 * 16 * 8, 4096),
+ nn.BatchNorm1d(4096),
+ nn.ReLU(),
+ )
+ self.classifier = nn.Linear(4096, num_classes)
+ self.feat_dim = 4096
+
+ def featuremaps(self, x):
+ x = self.block1(x)
+ x = self.block2(x)
+ x = self.block3(x)
+ x = self.block4(x)
+ x = self.block5(*x)
+ return x
+
+ def forward(self, x):
+ x = self.featuremaps(x)
+ x = x.view(x.size(0), -1)
+ x = self.fc(x)
+ y = self.classifier(x)
+
+ if not self.training:
+ return x
+
+ if self.loss == 'softmax':
+ return y
+ elif self.loss == 'triplet':
+ return y, x
+ else:
+ raise KeyError('Unsupported loss: {}'.format(self.loss))
diff --git a/trackers/strong_sort/deep/models/nasnet.py b/trackers/strong_sort/deep/models/nasnet.py
new file mode 100644
index 0000000000000000000000000000000000000000..b1f31def5515c3ba464c86cde471328b50c55b14
--- /dev/null
+++ b/trackers/strong_sort/deep/models/nasnet.py
@@ -0,0 +1,1131 @@
+from __future__ import division, absolute_import
+import torch
+import torch.nn as nn
+import torch.nn.functional as F
+import torch.utils.model_zoo as model_zoo
+
+__all__ = ['nasnetamobile']
+"""
+NASNet Mobile
+Thanks to Anastasiia (https://github.com/DagnyT) for the great help, support and motivation!
+
+
+------------------------------------------------------------------------------------
+ Architecture | Top-1 Acc | Top-5 Acc | Multiply-Adds | Params (M)
+------------------------------------------------------------------------------------
+| NASNet-A (4 @ 1056) | 74.08% | 91.74% | 564 M | 5.3 |
+------------------------------------------------------------------------------------
+# References:
+ - [Learning Transferable Architectures for Scalable Image Recognition]
+ (https://arxiv.org/abs/1707.07012)
+"""
+"""
+Code imported from https://github.com/Cadene/pretrained-models.pytorch
+"""
+
+pretrained_settings = {
+ 'nasnetamobile': {
+ 'imagenet': {
+ # 'url': 'https://github.com/veronikayurchuk/pretrained-models.pytorch/releases/download/v1.0/nasnetmobile-7e03cead.pth.tar',
+ 'url':
+ 'http://data.lip6.fr/cadene/pretrainedmodels/nasnetamobile-7e03cead.pth',
+ 'input_space': 'RGB',
+ 'input_size': [3, 224, 224], # resize 256
+ 'input_range': [0, 1],
+ 'mean': [0.5, 0.5, 0.5],
+ 'std': [0.5, 0.5, 0.5],
+ 'num_classes': 1000
+ },
+ # 'imagenet+background': {
+ # # 'url': 'http://data.lip6.fr/cadene/pretrainedmodels/nasnetalarge-a1897284.pth',
+ # 'input_space': 'RGB',
+ # 'input_size': [3, 224, 224], # resize 256
+ # 'input_range': [0, 1],
+ # 'mean': [0.5, 0.5, 0.5],
+ # 'std': [0.5, 0.5, 0.5],
+ # 'num_classes': 1001
+ # }
+ }
+}
+
+
+class MaxPoolPad(nn.Module):
+
+ def __init__(self):
+ super(MaxPoolPad, self).__init__()
+ self.pad = nn.ZeroPad2d((1, 0, 1, 0))
+ self.pool = nn.MaxPool2d(3, stride=2, padding=1)
+
+ def forward(self, x):
+ x = self.pad(x)
+ x = self.pool(x)
+ x = x[:, :, 1:, 1:].contiguous()
+ return x
+
+
+class AvgPoolPad(nn.Module):
+
+ def __init__(self, stride=2, padding=1):
+ super(AvgPoolPad, self).__init__()
+ self.pad = nn.ZeroPad2d((1, 0, 1, 0))
+ self.pool = nn.AvgPool2d(
+ 3, stride=stride, padding=padding, count_include_pad=False
+ )
+
+ def forward(self, x):
+ x = self.pad(x)
+ x = self.pool(x)
+ x = x[:, :, 1:, 1:].contiguous()
+ return x
+
+
+class SeparableConv2d(nn.Module):
+
+ def __init__(
+ self,
+ in_channels,
+ out_channels,
+ dw_kernel,
+ dw_stride,
+ dw_padding,
+ bias=False
+ ):
+ super(SeparableConv2d, self).__init__()
+ self.depthwise_conv2d = nn.Conv2d(
+ in_channels,
+ in_channels,
+ dw_kernel,
+ stride=dw_stride,
+ padding=dw_padding,
+ bias=bias,
+ groups=in_channels
+ )
+ self.pointwise_conv2d = nn.Conv2d(
+ in_channels, out_channels, 1, stride=1, bias=bias
+ )
+
+ def forward(self, x):
+ x = self.depthwise_conv2d(x)
+ x = self.pointwise_conv2d(x)
+ return x
+
+
+class BranchSeparables(nn.Module):
+
+ def __init__(
+ self,
+ in_channels,
+ out_channels,
+ kernel_size,
+ stride,
+ padding,
+ name=None,
+ bias=False
+ ):
+ super(BranchSeparables, self).__init__()
+ self.relu = nn.ReLU()
+ self.separable_1 = SeparableConv2d(
+ in_channels, in_channels, kernel_size, stride, padding, bias=bias
+ )
+ self.bn_sep_1 = nn.BatchNorm2d(
+ in_channels, eps=0.001, momentum=0.1, affine=True
+ )
+ self.relu1 = nn.ReLU()
+ self.separable_2 = SeparableConv2d(
+ in_channels, out_channels, kernel_size, 1, padding, bias=bias
+ )
+ self.bn_sep_2 = nn.BatchNorm2d(
+ out_channels, eps=0.001, momentum=0.1, affine=True
+ )
+ self.name = name
+
+ def forward(self, x):
+ x = self.relu(x)
+ if self.name == 'specific':
+ x = nn.ZeroPad2d((1, 0, 1, 0))(x)
+ x = self.separable_1(x)
+ if self.name == 'specific':
+ x = x[:, :, 1:, 1:].contiguous()
+
+ x = self.bn_sep_1(x)
+ x = self.relu1(x)
+ x = self.separable_2(x)
+ x = self.bn_sep_2(x)
+ return x
+
+
+class BranchSeparablesStem(nn.Module):
+
+ def __init__(
+ self,
+ in_channels,
+ out_channels,
+ kernel_size,
+ stride,
+ padding,
+ bias=False
+ ):
+ super(BranchSeparablesStem, self).__init__()
+ self.relu = nn.ReLU()
+ self.separable_1 = SeparableConv2d(
+ in_channels, out_channels, kernel_size, stride, padding, bias=bias
+ )
+ self.bn_sep_1 = nn.BatchNorm2d(
+ out_channels, eps=0.001, momentum=0.1, affine=True
+ )
+ self.relu1 = nn.ReLU()
+ self.separable_2 = SeparableConv2d(
+ out_channels, out_channels, kernel_size, 1, padding, bias=bias
+ )
+ self.bn_sep_2 = nn.BatchNorm2d(
+ out_channels, eps=0.001, momentum=0.1, affine=True
+ )
+
+ def forward(self, x):
+ x = self.relu(x)
+ x = self.separable_1(x)
+ x = self.bn_sep_1(x)
+ x = self.relu1(x)
+ x = self.separable_2(x)
+ x = self.bn_sep_2(x)
+ return x
+
+
+class BranchSeparablesReduction(BranchSeparables):
+
+ def __init__(
+ self,
+ in_channels,
+ out_channels,
+ kernel_size,
+ stride,
+ padding,
+ z_padding=1,
+ bias=False
+ ):
+ BranchSeparables.__init__(
+ self, in_channels, out_channels, kernel_size, stride, padding, bias
+ )
+ self.padding = nn.ZeroPad2d((z_padding, 0, z_padding, 0))
+
+ def forward(self, x):
+ x = self.relu(x)
+ x = self.padding(x)
+ x = self.separable_1(x)
+ x = x[:, :, 1:, 1:].contiguous()
+ x = self.bn_sep_1(x)
+ x = self.relu1(x)
+ x = self.separable_2(x)
+ x = self.bn_sep_2(x)
+ return x
+
+
+class CellStem0(nn.Module):
+
+ def __init__(self, stem_filters, num_filters=42):
+ super(CellStem0, self).__init__()
+ self.num_filters = num_filters
+ self.stem_filters = stem_filters
+ self.conv_1x1 = nn.Sequential()
+ self.conv_1x1.add_module('relu', nn.ReLU())
+ self.conv_1x1.add_module(
+ 'conv',
+ nn.Conv2d(
+ self.stem_filters, self.num_filters, 1, stride=1, bias=False
+ )
+ )
+ self.conv_1x1.add_module(
+ 'bn',
+ nn.BatchNorm2d(
+ self.num_filters, eps=0.001, momentum=0.1, affine=True
+ )
+ )
+
+ self.comb_iter_0_left = BranchSeparables(
+ self.num_filters, self.num_filters, 5, 2, 2
+ )
+ self.comb_iter_0_right = BranchSeparablesStem(
+ self.stem_filters, self.num_filters, 7, 2, 3, bias=False
+ )
+
+ self.comb_iter_1_left = nn.MaxPool2d(3, stride=2, padding=1)
+ self.comb_iter_1_right = BranchSeparablesStem(
+ self.stem_filters, self.num_filters, 7, 2, 3, bias=False
+ )
+
+ self.comb_iter_2_left = nn.AvgPool2d(
+ 3, stride=2, padding=1, count_include_pad=False
+ )
+ self.comb_iter_2_right = BranchSeparablesStem(
+ self.stem_filters, self.num_filters, 5, 2, 2, bias=False
+ )
+
+ self.comb_iter_3_right = nn.AvgPool2d(
+ 3, stride=1, padding=1, count_include_pad=False
+ )
+
+ self.comb_iter_4_left = BranchSeparables(
+ self.num_filters, self.num_filters, 3, 1, 1, bias=False
+ )
+ self.comb_iter_4_right = nn.MaxPool2d(3, stride=2, padding=1)
+
+ def forward(self, x):
+ x1 = self.conv_1x1(x)
+
+ x_comb_iter_0_left = self.comb_iter_0_left(x1)
+ x_comb_iter_0_right = self.comb_iter_0_right(x)
+ x_comb_iter_0 = x_comb_iter_0_left + x_comb_iter_0_right
+
+ x_comb_iter_1_left = self.comb_iter_1_left(x1)
+ x_comb_iter_1_right = self.comb_iter_1_right(x)
+ x_comb_iter_1 = x_comb_iter_1_left + x_comb_iter_1_right
+
+ x_comb_iter_2_left = self.comb_iter_2_left(x1)
+ x_comb_iter_2_right = self.comb_iter_2_right(x)
+ x_comb_iter_2 = x_comb_iter_2_left + x_comb_iter_2_right
+
+ x_comb_iter_3_right = self.comb_iter_3_right(x_comb_iter_0)
+ x_comb_iter_3 = x_comb_iter_3_right + x_comb_iter_1
+
+ x_comb_iter_4_left = self.comb_iter_4_left(x_comb_iter_0)
+ x_comb_iter_4_right = self.comb_iter_4_right(x1)
+ x_comb_iter_4 = x_comb_iter_4_left + x_comb_iter_4_right
+
+ x_out = torch.cat(
+ [x_comb_iter_1, x_comb_iter_2, x_comb_iter_3, x_comb_iter_4], 1
+ )
+ return x_out
+
+
+class CellStem1(nn.Module):
+
+ def __init__(self, stem_filters, num_filters):
+ super(CellStem1, self).__init__()
+ self.num_filters = num_filters
+ self.stem_filters = stem_filters
+ self.conv_1x1 = nn.Sequential()
+ self.conv_1x1.add_module('relu', nn.ReLU())
+ self.conv_1x1.add_module(
+ 'conv',
+ nn.Conv2d(
+ 2 * self.num_filters,
+ self.num_filters,
+ 1,
+ stride=1,
+ bias=False
+ )
+ )
+ self.conv_1x1.add_module(
+ 'bn',
+ nn.BatchNorm2d(
+ self.num_filters, eps=0.001, momentum=0.1, affine=True
+ )
+ )
+
+ self.relu = nn.ReLU()
+ self.path_1 = nn.Sequential()
+ self.path_1.add_module(
+ 'avgpool', nn.AvgPool2d(1, stride=2, count_include_pad=False)
+ )
+ self.path_1.add_module(
+ 'conv',
+ nn.Conv2d(
+ self.stem_filters,
+ self.num_filters // 2,
+ 1,
+ stride=1,
+ bias=False
+ )
+ )
+ self.path_2 = nn.ModuleList()
+ self.path_2.add_module('pad', nn.ZeroPad2d((0, 1, 0, 1)))
+ self.path_2.add_module(
+ 'avgpool', nn.AvgPool2d(1, stride=2, count_include_pad=False)
+ )
+ self.path_2.add_module(
+ 'conv',
+ nn.Conv2d(
+ self.stem_filters,
+ self.num_filters // 2,
+ 1,
+ stride=1,
+ bias=False
+ )
+ )
+
+ self.final_path_bn = nn.BatchNorm2d(
+ self.num_filters, eps=0.001, momentum=0.1, affine=True
+ )
+
+ self.comb_iter_0_left = BranchSeparables(
+ self.num_filters,
+ self.num_filters,
+ 5,
+ 2,
+ 2,
+ name='specific',
+ bias=False
+ )
+ self.comb_iter_0_right = BranchSeparables(
+ self.num_filters,
+ self.num_filters,
+ 7,
+ 2,
+ 3,
+ name='specific',
+ bias=False
+ )
+
+ # self.comb_iter_1_left = nn.MaxPool2d(3, stride=2, padding=1)
+ self.comb_iter_1_left = MaxPoolPad()
+ self.comb_iter_1_right = BranchSeparables(
+ self.num_filters,
+ self.num_filters,
+ 7,
+ 2,
+ 3,
+ name='specific',
+ bias=False
+ )
+
+ # self.comb_iter_2_left = nn.AvgPool2d(3, stride=2, padding=1, count_include_pad=False)
+ self.comb_iter_2_left = AvgPoolPad()
+ self.comb_iter_2_right = BranchSeparables(
+ self.num_filters,
+ self.num_filters,
+ 5,
+ 2,
+ 2,
+ name='specific',
+ bias=False
+ )
+
+ self.comb_iter_3_right = nn.AvgPool2d(
+ 3, stride=1, padding=1, count_include_pad=False
+ )
+
+ self.comb_iter_4_left = BranchSeparables(
+ self.num_filters,
+ self.num_filters,
+ 3,
+ 1,
+ 1,
+ name='specific',
+ bias=False
+ )
+ # self.comb_iter_4_right = nn.MaxPool2d(3, stride=2, padding=1)
+ self.comb_iter_4_right = MaxPoolPad()
+
+ def forward(self, x_conv0, x_stem_0):
+ x_left = self.conv_1x1(x_stem_0)
+
+ x_relu = self.relu(x_conv0)
+ # path 1
+ x_path1 = self.path_1(x_relu)
+ # path 2
+ x_path2 = self.path_2.pad(x_relu)
+ x_path2 = x_path2[:, :, 1:, 1:]
+ x_path2 = self.path_2.avgpool(x_path2)
+ x_path2 = self.path_2.conv(x_path2)
+ # final path
+ x_right = self.final_path_bn(torch.cat([x_path1, x_path2], 1))
+
+ x_comb_iter_0_left = self.comb_iter_0_left(x_left)
+ x_comb_iter_0_right = self.comb_iter_0_right(x_right)
+ x_comb_iter_0 = x_comb_iter_0_left + x_comb_iter_0_right
+
+ x_comb_iter_1_left = self.comb_iter_1_left(x_left)
+ x_comb_iter_1_right = self.comb_iter_1_right(x_right)
+ x_comb_iter_1 = x_comb_iter_1_left + x_comb_iter_1_right
+
+ x_comb_iter_2_left = self.comb_iter_2_left(x_left)
+ x_comb_iter_2_right = self.comb_iter_2_right(x_right)
+ x_comb_iter_2 = x_comb_iter_2_left + x_comb_iter_2_right
+
+ x_comb_iter_3_right = self.comb_iter_3_right(x_comb_iter_0)
+ x_comb_iter_3 = x_comb_iter_3_right + x_comb_iter_1
+
+ x_comb_iter_4_left = self.comb_iter_4_left(x_comb_iter_0)
+ x_comb_iter_4_right = self.comb_iter_4_right(x_left)
+ x_comb_iter_4 = x_comb_iter_4_left + x_comb_iter_4_right
+
+ x_out = torch.cat(
+ [x_comb_iter_1, x_comb_iter_2, x_comb_iter_3, x_comb_iter_4], 1
+ )
+ return x_out
+
+
+class FirstCell(nn.Module):
+
+ def __init__(
+ self, in_channels_left, out_channels_left, in_channels_right,
+ out_channels_right
+ ):
+ super(FirstCell, self).__init__()
+ self.conv_1x1 = nn.Sequential()
+ self.conv_1x1.add_module('relu', nn.ReLU())
+ self.conv_1x1.add_module(
+ 'conv',
+ nn.Conv2d(
+ in_channels_right, out_channels_right, 1, stride=1, bias=False
+ )
+ )
+ self.conv_1x1.add_module(
+ 'bn',
+ nn.BatchNorm2d(
+ out_channels_right, eps=0.001, momentum=0.1, affine=True
+ )
+ )
+
+ self.relu = nn.ReLU()
+ self.path_1 = nn.Sequential()
+ self.path_1.add_module(
+ 'avgpool', nn.AvgPool2d(1, stride=2, count_include_pad=False)
+ )
+ self.path_1.add_module(
+ 'conv',
+ nn.Conv2d(
+ in_channels_left, out_channels_left, 1, stride=1, bias=False
+ )
+ )
+ self.path_2 = nn.ModuleList()
+ self.path_2.add_module('pad', nn.ZeroPad2d((0, 1, 0, 1)))
+ self.path_2.add_module(
+ 'avgpool', nn.AvgPool2d(1, stride=2, count_include_pad=False)
+ )
+ self.path_2.add_module(
+ 'conv',
+ nn.Conv2d(
+ in_channels_left, out_channels_left, 1, stride=1, bias=False
+ )
+ )
+
+ self.final_path_bn = nn.BatchNorm2d(
+ out_channels_left * 2, eps=0.001, momentum=0.1, affine=True
+ )
+
+ self.comb_iter_0_left = BranchSeparables(
+ out_channels_right, out_channels_right, 5, 1, 2, bias=False
+ )
+ self.comb_iter_0_right = BranchSeparables(
+ out_channels_right, out_channels_right, 3, 1, 1, bias=False
+ )
+
+ self.comb_iter_1_left = BranchSeparables(
+ out_channels_right, out_channels_right, 5, 1, 2, bias=False
+ )
+ self.comb_iter_1_right = BranchSeparables(
+ out_channels_right, out_channels_right, 3, 1, 1, bias=False
+ )
+
+ self.comb_iter_2_left = nn.AvgPool2d(
+ 3, stride=1, padding=1, count_include_pad=False
+ )
+
+ self.comb_iter_3_left = nn.AvgPool2d(
+ 3, stride=1, padding=1, count_include_pad=False
+ )
+ self.comb_iter_3_right = nn.AvgPool2d(
+ 3, stride=1, padding=1, count_include_pad=False
+ )
+
+ self.comb_iter_4_left = BranchSeparables(
+ out_channels_right, out_channels_right, 3, 1, 1, bias=False
+ )
+
+ def forward(self, x, x_prev):
+ x_relu = self.relu(x_prev)
+ # path 1
+ x_path1 = self.path_1(x_relu)
+ # path 2
+ x_path2 = self.path_2.pad(x_relu)
+ x_path2 = x_path2[:, :, 1:, 1:]
+ x_path2 = self.path_2.avgpool(x_path2)
+ x_path2 = self.path_2.conv(x_path2)
+ # final path
+ x_left = self.final_path_bn(torch.cat([x_path1, x_path2], 1))
+
+ x_right = self.conv_1x1(x)
+
+ x_comb_iter_0_left = self.comb_iter_0_left(x_right)
+ x_comb_iter_0_right = self.comb_iter_0_right(x_left)
+ x_comb_iter_0 = x_comb_iter_0_left + x_comb_iter_0_right
+
+ x_comb_iter_1_left = self.comb_iter_1_left(x_left)
+ x_comb_iter_1_right = self.comb_iter_1_right(x_left)
+ x_comb_iter_1 = x_comb_iter_1_left + x_comb_iter_1_right
+
+ x_comb_iter_2_left = self.comb_iter_2_left(x_right)
+ x_comb_iter_2 = x_comb_iter_2_left + x_left
+
+ x_comb_iter_3_left = self.comb_iter_3_left(x_left)
+ x_comb_iter_3_right = self.comb_iter_3_right(x_left)
+ x_comb_iter_3 = x_comb_iter_3_left + x_comb_iter_3_right
+
+ x_comb_iter_4_left = self.comb_iter_4_left(x_right)
+ x_comb_iter_4 = x_comb_iter_4_left + x_right
+
+ x_out = torch.cat(
+ [
+ x_left, x_comb_iter_0, x_comb_iter_1, x_comb_iter_2,
+ x_comb_iter_3, x_comb_iter_4
+ ], 1
+ )
+ return x_out
+
+
+class NormalCell(nn.Module):
+
+ def __init__(
+ self, in_channels_left, out_channels_left, in_channels_right,
+ out_channels_right
+ ):
+ super(NormalCell, self).__init__()
+ self.conv_prev_1x1 = nn.Sequential()
+ self.conv_prev_1x1.add_module('relu', nn.ReLU())
+ self.conv_prev_1x1.add_module(
+ 'conv',
+ nn.Conv2d(
+ in_channels_left, out_channels_left, 1, stride=1, bias=False
+ )
+ )
+ self.conv_prev_1x1.add_module(
+ 'bn',
+ nn.BatchNorm2d(
+ out_channels_left, eps=0.001, momentum=0.1, affine=True
+ )
+ )
+
+ self.conv_1x1 = nn.Sequential()
+ self.conv_1x1.add_module('relu', nn.ReLU())
+ self.conv_1x1.add_module(
+ 'conv',
+ nn.Conv2d(
+ in_channels_right, out_channels_right, 1, stride=1, bias=False
+ )
+ )
+ self.conv_1x1.add_module(
+ 'bn',
+ nn.BatchNorm2d(
+ out_channels_right, eps=0.001, momentum=0.1, affine=True
+ )
+ )
+
+ self.comb_iter_0_left = BranchSeparables(
+ out_channels_right, out_channels_right, 5, 1, 2, bias=False
+ )
+ self.comb_iter_0_right = BranchSeparables(
+ out_channels_left, out_channels_left, 3, 1, 1, bias=False
+ )
+
+ self.comb_iter_1_left = BranchSeparables(
+ out_channels_left, out_channels_left, 5, 1, 2, bias=False
+ )
+ self.comb_iter_1_right = BranchSeparables(
+ out_channels_left, out_channels_left, 3, 1, 1, bias=False
+ )
+
+ self.comb_iter_2_left = nn.AvgPool2d(
+ 3, stride=1, padding=1, count_include_pad=False
+ )
+
+ self.comb_iter_3_left = nn.AvgPool2d(
+ 3, stride=1, padding=1, count_include_pad=False
+ )
+ self.comb_iter_3_right = nn.AvgPool2d(
+ 3, stride=1, padding=1, count_include_pad=False
+ )
+
+ self.comb_iter_4_left = BranchSeparables(
+ out_channels_right, out_channels_right, 3, 1, 1, bias=False
+ )
+
+ def forward(self, x, x_prev):
+ x_left = self.conv_prev_1x1(x_prev)
+ x_right = self.conv_1x1(x)
+
+ x_comb_iter_0_left = self.comb_iter_0_left(x_right)
+ x_comb_iter_0_right = self.comb_iter_0_right(x_left)
+ x_comb_iter_0 = x_comb_iter_0_left + x_comb_iter_0_right
+
+ x_comb_iter_1_left = self.comb_iter_1_left(x_left)
+ x_comb_iter_1_right = self.comb_iter_1_right(x_left)
+ x_comb_iter_1 = x_comb_iter_1_left + x_comb_iter_1_right
+
+ x_comb_iter_2_left = self.comb_iter_2_left(x_right)
+ x_comb_iter_2 = x_comb_iter_2_left + x_left
+
+ x_comb_iter_3_left = self.comb_iter_3_left(x_left)
+ x_comb_iter_3_right = self.comb_iter_3_right(x_left)
+ x_comb_iter_3 = x_comb_iter_3_left + x_comb_iter_3_right
+
+ x_comb_iter_4_left = self.comb_iter_4_left(x_right)
+ x_comb_iter_4 = x_comb_iter_4_left + x_right
+
+ x_out = torch.cat(
+ [
+ x_left, x_comb_iter_0, x_comb_iter_1, x_comb_iter_2,
+ x_comb_iter_3, x_comb_iter_4
+ ], 1
+ )
+ return x_out
+
+
+class ReductionCell0(nn.Module):
+
+ def __init__(
+ self, in_channels_left, out_channels_left, in_channels_right,
+ out_channels_right
+ ):
+ super(ReductionCell0, self).__init__()
+ self.conv_prev_1x1 = nn.Sequential()
+ self.conv_prev_1x1.add_module('relu', nn.ReLU())
+ self.conv_prev_1x1.add_module(
+ 'conv',
+ nn.Conv2d(
+ in_channels_left, out_channels_left, 1, stride=1, bias=False
+ )
+ )
+ self.conv_prev_1x1.add_module(
+ 'bn',
+ nn.BatchNorm2d(
+ out_channels_left, eps=0.001, momentum=0.1, affine=True
+ )
+ )
+
+ self.conv_1x1 = nn.Sequential()
+ self.conv_1x1.add_module('relu', nn.ReLU())
+ self.conv_1x1.add_module(
+ 'conv',
+ nn.Conv2d(
+ in_channels_right, out_channels_right, 1, stride=1, bias=False
+ )
+ )
+ self.conv_1x1.add_module(
+ 'bn',
+ nn.BatchNorm2d(
+ out_channels_right, eps=0.001, momentum=0.1, affine=True
+ )
+ )
+
+ self.comb_iter_0_left = BranchSeparablesReduction(
+ out_channels_right, out_channels_right, 5, 2, 2, bias=False
+ )
+ self.comb_iter_0_right = BranchSeparablesReduction(
+ out_channels_right, out_channels_right, 7, 2, 3, bias=False
+ )
+
+ self.comb_iter_1_left = MaxPoolPad()
+ self.comb_iter_1_right = BranchSeparablesReduction(
+ out_channels_right, out_channels_right, 7, 2, 3, bias=False
+ )
+
+ self.comb_iter_2_left = AvgPoolPad()
+ self.comb_iter_2_right = BranchSeparablesReduction(
+ out_channels_right, out_channels_right, 5, 2, 2, bias=False
+ )
+
+ self.comb_iter_3_right = nn.AvgPool2d(
+ 3, stride=1, padding=1, count_include_pad=False
+ )
+
+ self.comb_iter_4_left = BranchSeparablesReduction(
+ out_channels_right, out_channels_right, 3, 1, 1, bias=False
+ )
+ self.comb_iter_4_right = MaxPoolPad()
+
+ def forward(self, x, x_prev):
+ x_left = self.conv_prev_1x1(x_prev)
+ x_right = self.conv_1x1(x)
+
+ x_comb_iter_0_left = self.comb_iter_0_left(x_right)
+ x_comb_iter_0_right = self.comb_iter_0_right(x_left)
+ x_comb_iter_0 = x_comb_iter_0_left + x_comb_iter_0_right
+
+ x_comb_iter_1_left = self.comb_iter_1_left(x_right)
+ x_comb_iter_1_right = self.comb_iter_1_right(x_left)
+ x_comb_iter_1 = x_comb_iter_1_left + x_comb_iter_1_right
+
+ x_comb_iter_2_left = self.comb_iter_2_left(x_right)
+ x_comb_iter_2_right = self.comb_iter_2_right(x_left)
+ x_comb_iter_2 = x_comb_iter_2_left + x_comb_iter_2_right
+
+ x_comb_iter_3_right = self.comb_iter_3_right(x_comb_iter_0)
+ x_comb_iter_3 = x_comb_iter_3_right + x_comb_iter_1
+
+ x_comb_iter_4_left = self.comb_iter_4_left(x_comb_iter_0)
+ x_comb_iter_4_right = self.comb_iter_4_right(x_right)
+ x_comb_iter_4 = x_comb_iter_4_left + x_comb_iter_4_right
+
+ x_out = torch.cat(
+ [x_comb_iter_1, x_comb_iter_2, x_comb_iter_3, x_comb_iter_4], 1
+ )
+ return x_out
+
+
+class ReductionCell1(nn.Module):
+
+ def __init__(
+ self, in_channels_left, out_channels_left, in_channels_right,
+ out_channels_right
+ ):
+ super(ReductionCell1, self).__init__()
+ self.conv_prev_1x1 = nn.Sequential()
+ self.conv_prev_1x1.add_module('relu', nn.ReLU())
+ self.conv_prev_1x1.add_module(
+ 'conv',
+ nn.Conv2d(
+ in_channels_left, out_channels_left, 1, stride=1, bias=False
+ )
+ )
+ self.conv_prev_1x1.add_module(
+ 'bn',
+ nn.BatchNorm2d(
+ out_channels_left, eps=0.001, momentum=0.1, affine=True
+ )
+ )
+
+ self.conv_1x1 = nn.Sequential()
+ self.conv_1x1.add_module('relu', nn.ReLU())
+ self.conv_1x1.add_module(
+ 'conv',
+ nn.Conv2d(
+ in_channels_right, out_channels_right, 1, stride=1, bias=False
+ )
+ )
+ self.conv_1x1.add_module(
+ 'bn',
+ nn.BatchNorm2d(
+ out_channels_right, eps=0.001, momentum=0.1, affine=True
+ )
+ )
+
+ self.comb_iter_0_left = BranchSeparables(
+ out_channels_right,
+ out_channels_right,
+ 5,
+ 2,
+ 2,
+ name='specific',
+ bias=False
+ )
+ self.comb_iter_0_right = BranchSeparables(
+ out_channels_right,
+ out_channels_right,
+ 7,
+ 2,
+ 3,
+ name='specific',
+ bias=False
+ )
+
+ # self.comb_iter_1_left = nn.MaxPool2d(3, stride=2, padding=1)
+ self.comb_iter_1_left = MaxPoolPad()
+ self.comb_iter_1_right = BranchSeparables(
+ out_channels_right,
+ out_channels_right,
+ 7,
+ 2,
+ 3,
+ name='specific',
+ bias=False
+ )
+
+ # self.comb_iter_2_left = nn.AvgPool2d(3, stride=2, padding=1, count_include_pad=False)
+ self.comb_iter_2_left = AvgPoolPad()
+ self.comb_iter_2_right = BranchSeparables(
+ out_channels_right,
+ out_channels_right,
+ 5,
+ 2,
+ 2,
+ name='specific',
+ bias=False
+ )
+
+ self.comb_iter_3_right = nn.AvgPool2d(
+ 3, stride=1, padding=1, count_include_pad=False
+ )
+
+ self.comb_iter_4_left = BranchSeparables(
+ out_channels_right,
+ out_channels_right,
+ 3,
+ 1,
+ 1,
+ name='specific',
+ bias=False
+ )
+ # self.comb_iter_4_right = nn.MaxPool2d(3, stride=2, padding=1)
+ self.comb_iter_4_right = MaxPoolPad()
+
+ def forward(self, x, x_prev):
+ x_left = self.conv_prev_1x1(x_prev)
+ x_right = self.conv_1x1(x)
+
+ x_comb_iter_0_left = self.comb_iter_0_left(x_right)
+ x_comb_iter_0_right = self.comb_iter_0_right(x_left)
+ x_comb_iter_0 = x_comb_iter_0_left + x_comb_iter_0_right
+
+ x_comb_iter_1_left = self.comb_iter_1_left(x_right)
+ x_comb_iter_1_right = self.comb_iter_1_right(x_left)
+ x_comb_iter_1 = x_comb_iter_1_left + x_comb_iter_1_right
+
+ x_comb_iter_2_left = self.comb_iter_2_left(x_right)
+ x_comb_iter_2_right = self.comb_iter_2_right(x_left)
+ x_comb_iter_2 = x_comb_iter_2_left + x_comb_iter_2_right
+
+ x_comb_iter_3_right = self.comb_iter_3_right(x_comb_iter_0)
+ x_comb_iter_3 = x_comb_iter_3_right + x_comb_iter_1
+
+ x_comb_iter_4_left = self.comb_iter_4_left(x_comb_iter_0)
+ x_comb_iter_4_right = self.comb_iter_4_right(x_right)
+ x_comb_iter_4 = x_comb_iter_4_left + x_comb_iter_4_right
+
+ x_out = torch.cat(
+ [x_comb_iter_1, x_comb_iter_2, x_comb_iter_3, x_comb_iter_4], 1
+ )
+ return x_out
+
+
+class NASNetAMobile(nn.Module):
+ """Neural Architecture Search (NAS).
+
+ Reference:
+ Zoph et al. Learning Transferable Architectures
+ for Scalable Image Recognition. CVPR 2018.
+
+ Public keys:
+ - ``nasnetamobile``: NASNet-A Mobile.
+ """
+
+ def __init__(
+ self,
+ num_classes,
+ loss,
+ stem_filters=32,
+ penultimate_filters=1056,
+ filters_multiplier=2,
+ **kwargs
+ ):
+ super(NASNetAMobile, self).__init__()
+ self.stem_filters = stem_filters
+ self.penultimate_filters = penultimate_filters
+ self.filters_multiplier = filters_multiplier
+ self.loss = loss
+
+ filters = self.penultimate_filters // 24
+ # 24 is default value for the architecture
+
+ self.conv0 = nn.Sequential()
+ self.conv0.add_module(
+ 'conv',
+ nn.Conv2d(
+ in_channels=3,
+ out_channels=self.stem_filters,
+ kernel_size=3,
+ padding=0,
+ stride=2,
+ bias=False
+ )
+ )
+ self.conv0.add_module(
+ 'bn',
+ nn.BatchNorm2d(
+ self.stem_filters, eps=0.001, momentum=0.1, affine=True
+ )
+ )
+
+ self.cell_stem_0 = CellStem0(
+ self.stem_filters, num_filters=filters // (filters_multiplier**2)
+ )
+ self.cell_stem_1 = CellStem1(
+ self.stem_filters, num_filters=filters // filters_multiplier
+ )
+
+ self.cell_0 = FirstCell(
+ in_channels_left=filters,
+ out_channels_left=filters // 2, # 1, 0.5
+ in_channels_right=2 * filters,
+ out_channels_right=filters
+ ) # 2, 1
+ self.cell_1 = NormalCell(
+ in_channels_left=2 * filters,
+ out_channels_left=filters, # 2, 1
+ in_channels_right=6 * filters,
+ out_channels_right=filters
+ ) # 6, 1
+ self.cell_2 = NormalCell(
+ in_channels_left=6 * filters,
+ out_channels_left=filters, # 6, 1
+ in_channels_right=6 * filters,
+ out_channels_right=filters
+ ) # 6, 1
+ self.cell_3 = NormalCell(
+ in_channels_left=6 * filters,
+ out_channels_left=filters, # 6, 1
+ in_channels_right=6 * filters,
+ out_channels_right=filters
+ ) # 6, 1
+
+ self.reduction_cell_0 = ReductionCell0(
+ in_channels_left=6 * filters,
+ out_channels_left=2 * filters, # 6, 2
+ in_channels_right=6 * filters,
+ out_channels_right=2 * filters
+ ) # 6, 2
+
+ self.cell_6 = FirstCell(
+ in_channels_left=6 * filters,
+ out_channels_left=filters, # 6, 1
+ in_channels_right=8 * filters,
+ out_channels_right=2 * filters
+ ) # 8, 2
+ self.cell_7 = NormalCell(
+ in_channels_left=8 * filters,
+ out_channels_left=2 * filters, # 8, 2
+ in_channels_right=12 * filters,
+ out_channels_right=2 * filters
+ ) # 12, 2
+ self.cell_8 = NormalCell(
+ in_channels_left=12 * filters,
+ out_channels_left=2 * filters, # 12, 2
+ in_channels_right=12 * filters,
+ out_channels_right=2 * filters
+ ) # 12, 2
+ self.cell_9 = NormalCell(
+ in_channels_left=12 * filters,
+ out_channels_left=2 * filters, # 12, 2
+ in_channels_right=12 * filters,
+ out_channels_right=2 * filters
+ ) # 12, 2
+
+ self.reduction_cell_1 = ReductionCell1(
+ in_channels_left=12 * filters,
+ out_channels_left=4 * filters, # 12, 4
+ in_channels_right=12 * filters,
+ out_channels_right=4 * filters
+ ) # 12, 4
+
+ self.cell_12 = FirstCell(
+ in_channels_left=12 * filters,
+ out_channels_left=2 * filters, # 12, 2
+ in_channels_right=16 * filters,
+ out_channels_right=4 * filters
+ ) # 16, 4
+ self.cell_13 = NormalCell(
+ in_channels_left=16 * filters,
+ out_channels_left=4 * filters, # 16, 4
+ in_channels_right=24 * filters,
+ out_channels_right=4 * filters
+ ) # 24, 4
+ self.cell_14 = NormalCell(
+ in_channels_left=24 * filters,
+ out_channels_left=4 * filters, # 24, 4
+ in_channels_right=24 * filters,
+ out_channels_right=4 * filters
+ ) # 24, 4
+ self.cell_15 = NormalCell(
+ in_channels_left=24 * filters,
+ out_channels_left=4 * filters, # 24, 4
+ in_channels_right=24 * filters,
+ out_channels_right=4 * filters
+ ) # 24, 4
+
+ self.relu = nn.ReLU()
+ self.dropout = nn.Dropout()
+ self.classifier = nn.Linear(24 * filters, num_classes)
+
+ self._init_params()
+
+ def _init_params(self):
+ for m in self.modules():
+ if isinstance(m, nn.Conv2d):
+ nn.init.kaiming_normal_(
+ m.weight, mode='fan_out', nonlinearity='relu'
+ )
+ if m.bias is not None:
+ nn.init.constant_(m.bias, 0)
+ elif isinstance(m, nn.BatchNorm2d):
+ nn.init.constant_(m.weight, 1)
+ nn.init.constant_(m.bias, 0)
+ elif isinstance(m, nn.BatchNorm1d):
+ nn.init.constant_(m.weight, 1)
+ nn.init.constant_(m.bias, 0)
+ elif isinstance(m, nn.Linear):
+ nn.init.normal_(m.weight, 0, 0.01)
+ if m.bias is not None:
+ nn.init.constant_(m.bias, 0)
+
+ def features(self, input):
+ x_conv0 = self.conv0(input)
+ x_stem_0 = self.cell_stem_0(x_conv0)
+ x_stem_1 = self.cell_stem_1(x_conv0, x_stem_0)
+
+ x_cell_0 = self.cell_0(x_stem_1, x_stem_0)
+ x_cell_1 = self.cell_1(x_cell_0, x_stem_1)
+ x_cell_2 = self.cell_2(x_cell_1, x_cell_0)
+ x_cell_3 = self.cell_3(x_cell_2, x_cell_1)
+
+ x_reduction_cell_0 = self.reduction_cell_0(x_cell_3, x_cell_2)
+
+ x_cell_6 = self.cell_6(x_reduction_cell_0, x_cell_3)
+ x_cell_7 = self.cell_7(x_cell_6, x_reduction_cell_0)
+ x_cell_8 = self.cell_8(x_cell_7, x_cell_6)
+ x_cell_9 = self.cell_9(x_cell_8, x_cell_7)
+
+ x_reduction_cell_1 = self.reduction_cell_1(x_cell_9, x_cell_8)
+
+ x_cell_12 = self.cell_12(x_reduction_cell_1, x_cell_9)
+ x_cell_13 = self.cell_13(x_cell_12, x_reduction_cell_1)
+ x_cell_14 = self.cell_14(x_cell_13, x_cell_12)
+ x_cell_15 = self.cell_15(x_cell_14, x_cell_13)
+
+ x_cell_15 = self.relu(x_cell_15)
+ x_cell_15 = F.avg_pool2d(
+ x_cell_15,
+ x_cell_15.size()[2:]
+ ) # global average pool
+ x_cell_15 = x_cell_15.view(x_cell_15.size(0), -1)
+ x_cell_15 = self.dropout(x_cell_15)
+
+ return x_cell_15
+
+ def forward(self, input):
+ v = self.features(input)
+
+ if not self.training:
+ return v
+
+ y = self.classifier(v)
+
+ if self.loss == 'softmax':
+ return y
+ elif self.loss == 'triplet':
+ return y, v
+ else:
+ raise KeyError('Unsupported loss: {}'.format(self.loss))
+
+
+def init_pretrained_weights(model, model_url):
+ """Initializes model with pretrained weights.
+
+ Layers that don't match with pretrained layers in name or size are kept unchanged.
+ """
+ pretrain_dict = model_zoo.load_url(model_url)
+ model_dict = model.state_dict()
+ pretrain_dict = {
+ k: v
+ for k, v in pretrain_dict.items()
+ if k in model_dict and model_dict[k].size() == v.size()
+ }
+ model_dict.update(pretrain_dict)
+ model.load_state_dict(model_dict)
+
+
+def nasnetamobile(num_classes, loss='softmax', pretrained=True, **kwargs):
+ model = NASNetAMobile(num_classes, loss, **kwargs)
+ if pretrained:
+ model_url = pretrained_settings['nasnetamobile']['imagenet']['url']
+ init_pretrained_weights(model, model_url)
+ return model
diff --git a/trackers/strong_sort/deep/models/osnet.py b/trackers/strong_sort/deep/models/osnet.py
new file mode 100644
index 0000000000000000000000000000000000000000..b77388f13289f050da2bf2bdebd40ab4fce6f976
--- /dev/null
+++ b/trackers/strong_sort/deep/models/osnet.py
@@ -0,0 +1,598 @@
+from __future__ import division, absolute_import
+import warnings
+import torch
+from torch import nn
+from torch.nn import functional as F
+
+__all__ = [
+ 'osnet_x1_0', 'osnet_x0_75', 'osnet_x0_5', 'osnet_x0_25', 'osnet_ibn_x1_0'
+]
+
+pretrained_urls = {
+ 'osnet_x1_0':
+ 'https://drive.google.com/uc?id=1LaG1EJpHrxdAxKnSCJ_i0u-nbxSAeiFY',
+ 'osnet_x0_75':
+ 'https://drive.google.com/uc?id=1uwA9fElHOk3ZogwbeY5GkLI6QPTX70Hq',
+ 'osnet_x0_5':
+ 'https://drive.google.com/uc?id=16DGLbZukvVYgINws8u8deSaOqjybZ83i',
+ 'osnet_x0_25':
+ 'https://drive.google.com/uc?id=1rb8UN5ZzPKRc_xvtHlyDh-cSz88YX9hs',
+ 'osnet_ibn_x1_0':
+ 'https://drive.google.com/uc?id=1sr90V6irlYYDd4_4ISU2iruoRG8J__6l'
+}
+
+
+##########
+# Basic layers
+##########
+class ConvLayer(nn.Module):
+ """Convolution layer (conv + bn + relu)."""
+
+ def __init__(
+ self,
+ in_channels,
+ out_channels,
+ kernel_size,
+ stride=1,
+ padding=0,
+ groups=1,
+ IN=False
+ ):
+ super(ConvLayer, self).__init__()
+ self.conv = nn.Conv2d(
+ in_channels,
+ out_channels,
+ kernel_size,
+ stride=stride,
+ padding=padding,
+ bias=False,
+ groups=groups
+ )
+ if IN:
+ self.bn = nn.InstanceNorm2d(out_channels, affine=True)
+ else:
+ self.bn = nn.BatchNorm2d(out_channels)
+ self.relu = nn.ReLU(inplace=True)
+
+ def forward(self, x):
+ x = self.conv(x)
+ x = self.bn(x)
+ x = self.relu(x)
+ return x
+
+
+class Conv1x1(nn.Module):
+ """1x1 convolution + bn + relu."""
+
+ def __init__(self, in_channels, out_channels, stride=1, groups=1):
+ super(Conv1x1, self).__init__()
+ self.conv = nn.Conv2d(
+ in_channels,
+ out_channels,
+ 1,
+ stride=stride,
+ padding=0,
+ bias=False,
+ groups=groups
+ )
+ self.bn = nn.BatchNorm2d(out_channels)
+ self.relu = nn.ReLU(inplace=True)
+
+ def forward(self, x):
+ x = self.conv(x)
+ x = self.bn(x)
+ x = self.relu(x)
+ return x
+
+
+class Conv1x1Linear(nn.Module):
+ """1x1 convolution + bn (w/o non-linearity)."""
+
+ def __init__(self, in_channels, out_channels, stride=1):
+ super(Conv1x1Linear, self).__init__()
+ self.conv = nn.Conv2d(
+ in_channels, out_channels, 1, stride=stride, padding=0, bias=False
+ )
+ self.bn = nn.BatchNorm2d(out_channels)
+
+ def forward(self, x):
+ x = self.conv(x)
+ x = self.bn(x)
+ return x
+
+
+class Conv3x3(nn.Module):
+ """3x3 convolution + bn + relu."""
+
+ def __init__(self, in_channels, out_channels, stride=1, groups=1):
+ super(Conv3x3, self).__init__()
+ self.conv = nn.Conv2d(
+ in_channels,
+ out_channels,
+ 3,
+ stride=stride,
+ padding=1,
+ bias=False,
+ groups=groups
+ )
+ self.bn = nn.BatchNorm2d(out_channels)
+ self.relu = nn.ReLU(inplace=True)
+
+ def forward(self, x):
+ x = self.conv(x)
+ x = self.bn(x)
+ x = self.relu(x)
+ return x
+
+
+class LightConv3x3(nn.Module):
+ """Lightweight 3x3 convolution.
+
+ 1x1 (linear) + dw 3x3 (nonlinear).
+ """
+
+ def __init__(self, in_channels, out_channels):
+ super(LightConv3x3, self).__init__()
+ self.conv1 = nn.Conv2d(
+ in_channels, out_channels, 1, stride=1, padding=0, bias=False
+ )
+ self.conv2 = nn.Conv2d(
+ out_channels,
+ out_channels,
+ 3,
+ stride=1,
+ padding=1,
+ bias=False,
+ groups=out_channels
+ )
+ self.bn = nn.BatchNorm2d(out_channels)
+ self.relu = nn.ReLU(inplace=True)
+
+ def forward(self, x):
+ x = self.conv1(x)
+ x = self.conv2(x)
+ x = self.bn(x)
+ x = self.relu(x)
+ return x
+
+
+##########
+# Building blocks for omni-scale feature learning
+##########
+class ChannelGate(nn.Module):
+ """A mini-network that generates channel-wise gates conditioned on input tensor."""
+
+ def __init__(
+ self,
+ in_channels,
+ num_gates=None,
+ return_gates=False,
+ gate_activation='sigmoid',
+ reduction=16,
+ layer_norm=False
+ ):
+ super(ChannelGate, self).__init__()
+ if num_gates is None:
+ num_gates = in_channels
+ self.return_gates = return_gates
+ self.global_avgpool = nn.AdaptiveAvgPool2d(1)
+ self.fc1 = nn.Conv2d(
+ in_channels,
+ in_channels // reduction,
+ kernel_size=1,
+ bias=True,
+ padding=0
+ )
+ self.norm1 = None
+ if layer_norm:
+ self.norm1 = nn.LayerNorm((in_channels // reduction, 1, 1))
+ self.relu = nn.ReLU(inplace=True)
+ self.fc2 = nn.Conv2d(
+ in_channels // reduction,
+ num_gates,
+ kernel_size=1,
+ bias=True,
+ padding=0
+ )
+ if gate_activation == 'sigmoid':
+ self.gate_activation = nn.Sigmoid()
+ elif gate_activation == 'relu':
+ self.gate_activation = nn.ReLU(inplace=True)
+ elif gate_activation == 'linear':
+ self.gate_activation = None
+ else:
+ raise RuntimeError(
+ "Unknown gate activation: {}".format(gate_activation)
+ )
+
+ def forward(self, x):
+ input = x
+ x = self.global_avgpool(x)
+ x = self.fc1(x)
+ if self.norm1 is not None:
+ x = self.norm1(x)
+ x = self.relu(x)
+ x = self.fc2(x)
+ if self.gate_activation is not None:
+ x = self.gate_activation(x)
+ if self.return_gates:
+ return x
+ return input * x
+
+
+class OSBlock(nn.Module):
+ """Omni-scale feature learning block."""
+
+ def __init__(
+ self,
+ in_channels,
+ out_channels,
+ IN=False,
+ bottleneck_reduction=4,
+ **kwargs
+ ):
+ super(OSBlock, self).__init__()
+ mid_channels = out_channels // bottleneck_reduction
+ self.conv1 = Conv1x1(in_channels, mid_channels)
+ self.conv2a = LightConv3x3(mid_channels, mid_channels)
+ self.conv2b = nn.Sequential(
+ LightConv3x3(mid_channels, mid_channels),
+ LightConv3x3(mid_channels, mid_channels),
+ )
+ self.conv2c = nn.Sequential(
+ LightConv3x3(mid_channels, mid_channels),
+ LightConv3x3(mid_channels, mid_channels),
+ LightConv3x3(mid_channels, mid_channels),
+ )
+ self.conv2d = nn.Sequential(
+ LightConv3x3(mid_channels, mid_channels),
+ LightConv3x3(mid_channels, mid_channels),
+ LightConv3x3(mid_channels, mid_channels),
+ LightConv3x3(mid_channels, mid_channels),
+ )
+ self.gate = ChannelGate(mid_channels)
+ self.conv3 = Conv1x1Linear(mid_channels, out_channels)
+ self.downsample = None
+ if in_channels != out_channels:
+ self.downsample = Conv1x1Linear(in_channels, out_channels)
+ self.IN = None
+ if IN:
+ self.IN = nn.InstanceNorm2d(out_channels, affine=True)
+
+ def forward(self, x):
+ identity = x
+ x1 = self.conv1(x)
+ x2a = self.conv2a(x1)
+ x2b = self.conv2b(x1)
+ x2c = self.conv2c(x1)
+ x2d = self.conv2d(x1)
+ x2 = self.gate(x2a) + self.gate(x2b) + self.gate(x2c) + self.gate(x2d)
+ x3 = self.conv3(x2)
+ if self.downsample is not None:
+ identity = self.downsample(identity)
+ out = x3 + identity
+ if self.IN is not None:
+ out = self.IN(out)
+ return F.relu(out)
+
+
+##########
+# Network architecture
+##########
+class OSNet(nn.Module):
+ """Omni-Scale Network.
+
+ Reference:
+ - Zhou et al. Omni-Scale Feature Learning for Person Re-Identification. ICCV, 2019.
+ - Zhou et al. Learning Generalisable Omni-Scale Representations
+ for Person Re-Identification. TPAMI, 2021.
+ """
+
+ def __init__(
+ self,
+ num_classes,
+ blocks,
+ layers,
+ channels,
+ feature_dim=512,
+ loss='softmax',
+ IN=False,
+ **kwargs
+ ):
+ super(OSNet, self).__init__()
+ num_blocks = len(blocks)
+ assert num_blocks == len(layers)
+ assert num_blocks == len(channels) - 1
+ self.loss = loss
+ self.feature_dim = feature_dim
+
+ # convolutional backbone
+ self.conv1 = ConvLayer(3, channels[0], 7, stride=2, padding=3, IN=IN)
+ self.maxpool = nn.MaxPool2d(3, stride=2, padding=1)
+ self.conv2 = self._make_layer(
+ blocks[0],
+ layers[0],
+ channels[0],
+ channels[1],
+ reduce_spatial_size=True,
+ IN=IN
+ )
+ self.conv3 = self._make_layer(
+ blocks[1],
+ layers[1],
+ channels[1],
+ channels[2],
+ reduce_spatial_size=True
+ )
+ self.conv4 = self._make_layer(
+ blocks[2],
+ layers[2],
+ channels[2],
+ channels[3],
+ reduce_spatial_size=False
+ )
+ self.conv5 = Conv1x1(channels[3], channels[3])
+ self.global_avgpool = nn.AdaptiveAvgPool2d(1)
+ # fully connected layer
+ self.fc = self._construct_fc_layer(
+ self.feature_dim, channels[3], dropout_p=None
+ )
+ # identity classification layer
+ self.classifier = nn.Linear(self.feature_dim, num_classes)
+
+ self._init_params()
+
+ def _make_layer(
+ self,
+ block,
+ layer,
+ in_channels,
+ out_channels,
+ reduce_spatial_size,
+ IN=False
+ ):
+ layers = []
+
+ layers.append(block(in_channels, out_channels, IN=IN))
+ for i in range(1, layer):
+ layers.append(block(out_channels, out_channels, IN=IN))
+
+ if reduce_spatial_size:
+ layers.append(
+ nn.Sequential(
+ Conv1x1(out_channels, out_channels),
+ nn.AvgPool2d(2, stride=2)
+ )
+ )
+
+ return nn.Sequential(*layers)
+
+ def _construct_fc_layer(self, fc_dims, input_dim, dropout_p=None):
+ if fc_dims is None or fc_dims < 0:
+ self.feature_dim = input_dim
+ return None
+
+ if isinstance(fc_dims, int):
+ fc_dims = [fc_dims]
+
+ layers = []
+ for dim in fc_dims:
+ layers.append(nn.Linear(input_dim, dim))
+ layers.append(nn.BatchNorm1d(dim))
+ layers.append(nn.ReLU(inplace=True))
+ if dropout_p is not None:
+ layers.append(nn.Dropout(p=dropout_p))
+ input_dim = dim
+
+ self.feature_dim = fc_dims[-1]
+
+ return nn.Sequential(*layers)
+
+ def _init_params(self):
+ for m in self.modules():
+ if isinstance(m, nn.Conv2d):
+ nn.init.kaiming_normal_(
+ m.weight, mode='fan_out', nonlinearity='relu'
+ )
+ if m.bias is not None:
+ nn.init.constant_(m.bias, 0)
+
+ elif isinstance(m, nn.BatchNorm2d):
+ nn.init.constant_(m.weight, 1)
+ nn.init.constant_(m.bias, 0)
+
+ elif isinstance(m, nn.BatchNorm1d):
+ nn.init.constant_(m.weight, 1)
+ nn.init.constant_(m.bias, 0)
+
+ elif isinstance(m, nn.Linear):
+ nn.init.normal_(m.weight, 0, 0.01)
+ if m.bias is not None:
+ nn.init.constant_(m.bias, 0)
+
+ def featuremaps(self, x):
+ x = self.conv1(x)
+ x = self.maxpool(x)
+ x = self.conv2(x)
+ x = self.conv3(x)
+ x = self.conv4(x)
+ x = self.conv5(x)
+ return x
+
+ def forward(self, x, return_featuremaps=False):
+ x = self.featuremaps(x)
+ if return_featuremaps:
+ return x
+ v = self.global_avgpool(x)
+ v = v.view(v.size(0), -1)
+ if self.fc is not None:
+ v = self.fc(v)
+ if not self.training:
+ return v
+ y = self.classifier(v)
+ if self.loss == 'softmax':
+ return y
+ elif self.loss == 'triplet':
+ return y, v
+ else:
+ raise KeyError("Unsupported loss: {}".format(self.loss))
+
+
+def init_pretrained_weights(model, key=''):
+ """Initializes model with pretrained weights.
+
+ Layers that don't match with pretrained layers in name or size are kept unchanged.
+ """
+ import os
+ import errno
+ import gdown
+ from collections import OrderedDict
+
+ def _get_torch_home():
+ ENV_TORCH_HOME = 'TORCH_HOME'
+ ENV_XDG_CACHE_HOME = 'XDG_CACHE_HOME'
+ DEFAULT_CACHE_DIR = '~/.cache'
+ torch_home = os.path.expanduser(
+ os.getenv(
+ ENV_TORCH_HOME,
+ os.path.join(
+ os.getenv(ENV_XDG_CACHE_HOME, DEFAULT_CACHE_DIR), 'torch'
+ )
+ )
+ )
+ return torch_home
+
+ torch_home = _get_torch_home()
+ model_dir = os.path.join(torch_home, 'checkpoints')
+ try:
+ os.makedirs(model_dir)
+ except OSError as e:
+ if e.errno == errno.EEXIST:
+ # Directory already exists, ignore.
+ pass
+ else:
+ # Unexpected OSError, re-raise.
+ raise
+ filename = key + '_imagenet.pth'
+ cached_file = os.path.join(model_dir, filename)
+
+ if not os.path.exists(cached_file):
+ gdown.download(pretrained_urls[key], cached_file, quiet=False)
+
+ state_dict = torch.load(cached_file)
+ model_dict = model.state_dict()
+ new_state_dict = OrderedDict()
+ matched_layers, discarded_layers = [], []
+
+ for k, v in state_dict.items():
+ if k.startswith('module.'):
+ k = k[7:] # discard module.
+
+ if k in model_dict and model_dict[k].size() == v.size():
+ new_state_dict[k] = v
+ matched_layers.append(k)
+ else:
+ discarded_layers.append(k)
+
+ model_dict.update(new_state_dict)
+ model.load_state_dict(model_dict)
+
+ if len(matched_layers) == 0:
+ warnings.warn(
+ 'The pretrained weights from "{}" cannot be loaded, '
+ 'please check the key names manually '
+ '(** ignored and continue **)'.format(cached_file)
+ )
+ else:
+ print(
+ 'Successfully loaded imagenet pretrained weights from "{}"'.
+ format(cached_file)
+ )
+ if len(discarded_layers) > 0:
+ print(
+ '** The following layers are discarded '
+ 'due to unmatched keys or layer size: {}'.
+ format(discarded_layers)
+ )
+
+
+##########
+# Instantiation
+##########
+def osnet_x1_0(num_classes=1000, pretrained=True, loss='softmax', **kwargs):
+ # standard size (width x1.0)
+ model = OSNet(
+ num_classes,
+ blocks=[OSBlock, OSBlock, OSBlock],
+ layers=[2, 2, 2],
+ channels=[64, 256, 384, 512],
+ loss=loss,
+ **kwargs
+ )
+ if pretrained:
+ init_pretrained_weights(model, key='osnet_x1_0')
+ return model
+
+
+def osnet_x0_75(num_classes=1000, pretrained=True, loss='softmax', **kwargs):
+ # medium size (width x0.75)
+ model = OSNet(
+ num_classes,
+ blocks=[OSBlock, OSBlock, OSBlock],
+ layers=[2, 2, 2],
+ channels=[48, 192, 288, 384],
+ loss=loss,
+ **kwargs
+ )
+ if pretrained:
+ init_pretrained_weights(model, key='osnet_x0_75')
+ return model
+
+
+def osnet_x0_5(num_classes=1000, pretrained=True, loss='softmax', **kwargs):
+ # tiny size (width x0.5)
+ model = OSNet(
+ num_classes,
+ blocks=[OSBlock, OSBlock, OSBlock],
+ layers=[2, 2, 2],
+ channels=[32, 128, 192, 256],
+ loss=loss,
+ **kwargs
+ )
+ if pretrained:
+ init_pretrained_weights(model, key='osnet_x0_5')
+ return model
+
+
+def osnet_x0_25(num_classes=1000, pretrained=True, loss='softmax', **kwargs):
+ # very tiny size (width x0.25)
+ model = OSNet(
+ num_classes,
+ blocks=[OSBlock, OSBlock, OSBlock],
+ layers=[2, 2, 2],
+ channels=[16, 64, 96, 128],
+ loss=loss,
+ **kwargs
+ )
+ if pretrained:
+ init_pretrained_weights(model, key='osnet_x0_25')
+ return model
+
+
+def osnet_ibn_x1_0(
+ num_classes=1000, pretrained=True, loss='softmax', **kwargs
+):
+ # standard size (width x1.0) + IBN layer
+ # Ref: Pan et al. Two at Once: Enhancing Learning and Generalization Capacities via IBN-Net. ECCV, 2018.
+ model = OSNet(
+ num_classes,
+ blocks=[OSBlock, OSBlock, OSBlock],
+ layers=[2, 2, 2],
+ channels=[64, 256, 384, 512],
+ loss=loss,
+ IN=True,
+ **kwargs
+ )
+ if pretrained:
+ init_pretrained_weights(model, key='osnet_ibn_x1_0')
+ return model
diff --git a/trackers/strong_sort/deep/models/osnet_ain.py b/trackers/strong_sort/deep/models/osnet_ain.py
new file mode 100644
index 0000000000000000000000000000000000000000..3f9f7bd0704502401d499fd2bfdb802522b99efe
--- /dev/null
+++ b/trackers/strong_sort/deep/models/osnet_ain.py
@@ -0,0 +1,609 @@
+from __future__ import division, absolute_import
+import warnings
+import torch
+from torch import nn
+from torch.nn import functional as F
+
+__all__ = [
+ 'osnet_ain_x1_0', 'osnet_ain_x0_75', 'osnet_ain_x0_5', 'osnet_ain_x0_25'
+]
+
+pretrained_urls = {
+ 'osnet_ain_x1_0':
+ 'https://drive.google.com/uc?id=1-CaioD9NaqbHK_kzSMW8VE4_3KcsRjEo',
+ 'osnet_ain_x0_75':
+ 'https://drive.google.com/uc?id=1apy0hpsMypqstfencdH-jKIUEFOW4xoM',
+ 'osnet_ain_x0_5':
+ 'https://drive.google.com/uc?id=1KusKvEYyKGDTUBVRxRiz55G31wkihB6l',
+ 'osnet_ain_x0_25':
+ 'https://drive.google.com/uc?id=1SxQt2AvmEcgWNhaRb2xC4rP6ZwVDP0Wt'
+}
+
+
+##########
+# Basic layers
+##########
+class ConvLayer(nn.Module):
+ """Convolution layer (conv + bn + relu)."""
+
+ def __init__(
+ self,
+ in_channels,
+ out_channels,
+ kernel_size,
+ stride=1,
+ padding=0,
+ groups=1,
+ IN=False
+ ):
+ super(ConvLayer, self).__init__()
+ self.conv = nn.Conv2d(
+ in_channels,
+ out_channels,
+ kernel_size,
+ stride=stride,
+ padding=padding,
+ bias=False,
+ groups=groups
+ )
+ if IN:
+ self.bn = nn.InstanceNorm2d(out_channels, affine=True)
+ else:
+ self.bn = nn.BatchNorm2d(out_channels)
+ self.relu = nn.ReLU()
+
+ def forward(self, x):
+ x = self.conv(x)
+ x = self.bn(x)
+ return self.relu(x)
+
+
+class Conv1x1(nn.Module):
+ """1x1 convolution + bn + relu."""
+
+ def __init__(self, in_channels, out_channels, stride=1, groups=1):
+ super(Conv1x1, self).__init__()
+ self.conv = nn.Conv2d(
+ in_channels,
+ out_channels,
+ 1,
+ stride=stride,
+ padding=0,
+ bias=False,
+ groups=groups
+ )
+ self.bn = nn.BatchNorm2d(out_channels)
+ self.relu = nn.ReLU()
+
+ def forward(self, x):
+ x = self.conv(x)
+ x = self.bn(x)
+ return self.relu(x)
+
+
+class Conv1x1Linear(nn.Module):
+ """1x1 convolution + bn (w/o non-linearity)."""
+
+ def __init__(self, in_channels, out_channels, stride=1, bn=True):
+ super(Conv1x1Linear, self).__init__()
+ self.conv = nn.Conv2d(
+ in_channels, out_channels, 1, stride=stride, padding=0, bias=False
+ )
+ self.bn = None
+ if bn:
+ self.bn = nn.BatchNorm2d(out_channels)
+
+ def forward(self, x):
+ x = self.conv(x)
+ if self.bn is not None:
+ x = self.bn(x)
+ return x
+
+
+class Conv3x3(nn.Module):
+ """3x3 convolution + bn + relu."""
+
+ def __init__(self, in_channels, out_channels, stride=1, groups=1):
+ super(Conv3x3, self).__init__()
+ self.conv = nn.Conv2d(
+ in_channels,
+ out_channels,
+ 3,
+ stride=stride,
+ padding=1,
+ bias=False,
+ groups=groups
+ )
+ self.bn = nn.BatchNorm2d(out_channels)
+ self.relu = nn.ReLU()
+
+ def forward(self, x):
+ x = self.conv(x)
+ x = self.bn(x)
+ return self.relu(x)
+
+
+class LightConv3x3(nn.Module):
+ """Lightweight 3x3 convolution.
+
+ 1x1 (linear) + dw 3x3 (nonlinear).
+ """
+
+ def __init__(self, in_channels, out_channels):
+ super(LightConv3x3, self).__init__()
+ self.conv1 = nn.Conv2d(
+ in_channels, out_channels, 1, stride=1, padding=0, bias=False
+ )
+ self.conv2 = nn.Conv2d(
+ out_channels,
+ out_channels,
+ 3,
+ stride=1,
+ padding=1,
+ bias=False,
+ groups=out_channels
+ )
+ self.bn = nn.BatchNorm2d(out_channels)
+ self.relu = nn.ReLU()
+
+ def forward(self, x):
+ x = self.conv1(x)
+ x = self.conv2(x)
+ x = self.bn(x)
+ return self.relu(x)
+
+
+class LightConvStream(nn.Module):
+ """Lightweight convolution stream."""
+
+ def __init__(self, in_channels, out_channels, depth):
+ super(LightConvStream, self).__init__()
+ assert depth >= 1, 'depth must be equal to or larger than 1, but got {}'.format(
+ depth
+ )
+ layers = []
+ layers += [LightConv3x3(in_channels, out_channels)]
+ for i in range(depth - 1):
+ layers += [LightConv3x3(out_channels, out_channels)]
+ self.layers = nn.Sequential(*layers)
+
+ def forward(self, x):
+ return self.layers(x)
+
+
+##########
+# Building blocks for omni-scale feature learning
+##########
+class ChannelGate(nn.Module):
+ """A mini-network that generates channel-wise gates conditioned on input tensor."""
+
+ def __init__(
+ self,
+ in_channels,
+ num_gates=None,
+ return_gates=False,
+ gate_activation='sigmoid',
+ reduction=16,
+ layer_norm=False
+ ):
+ super(ChannelGate, self).__init__()
+ if num_gates is None:
+ num_gates = in_channels
+ self.return_gates = return_gates
+ self.global_avgpool = nn.AdaptiveAvgPool2d(1)
+ self.fc1 = nn.Conv2d(
+ in_channels,
+ in_channels // reduction,
+ kernel_size=1,
+ bias=True,
+ padding=0
+ )
+ self.norm1 = None
+ if layer_norm:
+ self.norm1 = nn.LayerNorm((in_channels // reduction, 1, 1))
+ self.relu = nn.ReLU()
+ self.fc2 = nn.Conv2d(
+ in_channels // reduction,
+ num_gates,
+ kernel_size=1,
+ bias=True,
+ padding=0
+ )
+ if gate_activation == 'sigmoid':
+ self.gate_activation = nn.Sigmoid()
+ elif gate_activation == 'relu':
+ self.gate_activation = nn.ReLU()
+ elif gate_activation == 'linear':
+ self.gate_activation = None
+ else:
+ raise RuntimeError(
+ "Unknown gate activation: {}".format(gate_activation)
+ )
+
+ def forward(self, x):
+ input = x
+ x = self.global_avgpool(x)
+ x = self.fc1(x)
+ if self.norm1 is not None:
+ x = self.norm1(x)
+ x = self.relu(x)
+ x = self.fc2(x)
+ if self.gate_activation is not None:
+ x = self.gate_activation(x)
+ if self.return_gates:
+ return x
+ return input * x
+
+
+class OSBlock(nn.Module):
+ """Omni-scale feature learning block."""
+
+ def __init__(self, in_channels, out_channels, reduction=4, T=4, **kwargs):
+ super(OSBlock, self).__init__()
+ assert T >= 1
+ assert out_channels >= reduction and out_channels % reduction == 0
+ mid_channels = out_channels // reduction
+
+ self.conv1 = Conv1x1(in_channels, mid_channels)
+ self.conv2 = nn.ModuleList()
+ for t in range(1, T + 1):
+ self.conv2 += [LightConvStream(mid_channels, mid_channels, t)]
+ self.gate = ChannelGate(mid_channels)
+ self.conv3 = Conv1x1Linear(mid_channels, out_channels)
+ self.downsample = None
+ if in_channels != out_channels:
+ self.downsample = Conv1x1Linear(in_channels, out_channels)
+
+ def forward(self, x):
+ identity = x
+ x1 = self.conv1(x)
+ x2 = 0
+ for conv2_t in self.conv2:
+ x2_t = conv2_t(x1)
+ x2 = x2 + self.gate(x2_t)
+ x3 = self.conv3(x2)
+ if self.downsample is not None:
+ identity = self.downsample(identity)
+ out = x3 + identity
+ return F.relu(out)
+
+
+class OSBlockINin(nn.Module):
+ """Omni-scale feature learning block with instance normalization."""
+
+ def __init__(self, in_channels, out_channels, reduction=4, T=4, **kwargs):
+ super(OSBlockINin, self).__init__()
+ assert T >= 1
+ assert out_channels >= reduction and out_channels % reduction == 0
+ mid_channels = out_channels // reduction
+
+ self.conv1 = Conv1x1(in_channels, mid_channels)
+ self.conv2 = nn.ModuleList()
+ for t in range(1, T + 1):
+ self.conv2 += [LightConvStream(mid_channels, mid_channels, t)]
+ self.gate = ChannelGate(mid_channels)
+ self.conv3 = Conv1x1Linear(mid_channels, out_channels, bn=False)
+ self.downsample = None
+ if in_channels != out_channels:
+ self.downsample = Conv1x1Linear(in_channels, out_channels)
+ self.IN = nn.InstanceNorm2d(out_channels, affine=True)
+
+ def forward(self, x):
+ identity = x
+ x1 = self.conv1(x)
+ x2 = 0
+ for conv2_t in self.conv2:
+ x2_t = conv2_t(x1)
+ x2 = x2 + self.gate(x2_t)
+ x3 = self.conv3(x2)
+ x3 = self.IN(x3) # IN inside residual
+ if self.downsample is not None:
+ identity = self.downsample(identity)
+ out = x3 + identity
+ return F.relu(out)
+
+
+##########
+# Network architecture
+##########
+class OSNet(nn.Module):
+ """Omni-Scale Network.
+
+ Reference:
+ - Zhou et al. Omni-Scale Feature Learning for Person Re-Identification. ICCV, 2019.
+ - Zhou et al. Learning Generalisable Omni-Scale Representations
+ for Person Re-Identification. TPAMI, 2021.
+ """
+
+ def __init__(
+ self,
+ num_classes,
+ blocks,
+ layers,
+ channels,
+ feature_dim=512,
+ loss='softmax',
+ conv1_IN=False,
+ **kwargs
+ ):
+ super(OSNet, self).__init__()
+ num_blocks = len(blocks)
+ assert num_blocks == len(layers)
+ assert num_blocks == len(channels) - 1
+ self.loss = loss
+ self.feature_dim = feature_dim
+
+ # convolutional backbone
+ self.conv1 = ConvLayer(
+ 3, channels[0], 7, stride=2, padding=3, IN=conv1_IN
+ )
+ self.maxpool = nn.MaxPool2d(3, stride=2, padding=1)
+ self.conv2 = self._make_layer(
+ blocks[0], layers[0], channels[0], channels[1]
+ )
+ self.pool2 = nn.Sequential(
+ Conv1x1(channels[1], channels[1]), nn.AvgPool2d(2, stride=2)
+ )
+ self.conv3 = self._make_layer(
+ blocks[1], layers[1], channels[1], channels[2]
+ )
+ self.pool3 = nn.Sequential(
+ Conv1x1(channels[2], channels[2]), nn.AvgPool2d(2, stride=2)
+ )
+ self.conv4 = self._make_layer(
+ blocks[2], layers[2], channels[2], channels[3]
+ )
+ self.conv5 = Conv1x1(channels[3], channels[3])
+ self.global_avgpool = nn.AdaptiveAvgPool2d(1)
+ # fully connected layer
+ self.fc = self._construct_fc_layer(
+ self.feature_dim, channels[3], dropout_p=None
+ )
+ # identity classification layer
+ self.classifier = nn.Linear(self.feature_dim, num_classes)
+
+ self._init_params()
+
+ def _make_layer(self, blocks, layer, in_channels, out_channels):
+ layers = []
+ layers += [blocks[0](in_channels, out_channels)]
+ for i in range(1, len(blocks)):
+ layers += [blocks[i](out_channels, out_channels)]
+ return nn.Sequential(*layers)
+
+ def _construct_fc_layer(self, fc_dims, input_dim, dropout_p=None):
+ if fc_dims is None or fc_dims < 0:
+ self.feature_dim = input_dim
+ return None
+
+ if isinstance(fc_dims, int):
+ fc_dims = [fc_dims]
+
+ layers = []
+ for dim in fc_dims:
+ layers.append(nn.Linear(input_dim, dim))
+ layers.append(nn.BatchNorm1d(dim))
+ layers.append(nn.ReLU())
+ if dropout_p is not None:
+ layers.append(nn.Dropout(p=dropout_p))
+ input_dim = dim
+
+ self.feature_dim = fc_dims[-1]
+
+ return nn.Sequential(*layers)
+
+ def _init_params(self):
+ for m in self.modules():
+ if isinstance(m, nn.Conv2d):
+ nn.init.kaiming_normal_(
+ m.weight, mode='fan_out', nonlinearity='relu'
+ )
+ if m.bias is not None:
+ nn.init.constant_(m.bias, 0)
+
+ elif isinstance(m, nn.BatchNorm2d):
+ nn.init.constant_(m.weight, 1)
+ nn.init.constant_(m.bias, 0)
+
+ elif isinstance(m, nn.BatchNorm1d):
+ nn.init.constant_(m.weight, 1)
+ nn.init.constant_(m.bias, 0)
+
+ elif isinstance(m, nn.InstanceNorm2d):
+ nn.init.constant_(m.weight, 1)
+ nn.init.constant_(m.bias, 0)
+
+ elif isinstance(m, nn.Linear):
+ nn.init.normal_(m.weight, 0, 0.01)
+ if m.bias is not None:
+ nn.init.constant_(m.bias, 0)
+
+ def featuremaps(self, x):
+ x = self.conv1(x)
+ x = self.maxpool(x)
+ x = self.conv2(x)
+ x = self.pool2(x)
+ x = self.conv3(x)
+ x = self.pool3(x)
+ x = self.conv4(x)
+ x = self.conv5(x)
+ return x
+
+ def forward(self, x, return_featuremaps=False):
+ x = self.featuremaps(x)
+ if return_featuremaps:
+ return x
+ v = self.global_avgpool(x)
+ v = v.view(v.size(0), -1)
+ if self.fc is not None:
+ v = self.fc(v)
+ if not self.training:
+ return v
+ y = self.classifier(v)
+ if self.loss == 'softmax':
+ return y
+ elif self.loss == 'triplet':
+ return y, v
+ else:
+ raise KeyError("Unsupported loss: {}".format(self.loss))
+
+
+def init_pretrained_weights(model, key=''):
+ """Initializes model with pretrained weights.
+
+ Layers that don't match with pretrained layers in name or size are kept unchanged.
+ """
+ import os
+ import errno
+ import gdown
+ from collections import OrderedDict
+
+ def _get_torch_home():
+ ENV_TORCH_HOME = 'TORCH_HOME'
+ ENV_XDG_CACHE_HOME = 'XDG_CACHE_HOME'
+ DEFAULT_CACHE_DIR = '~/.cache'
+ torch_home = os.path.expanduser(
+ os.getenv(
+ ENV_TORCH_HOME,
+ os.path.join(
+ os.getenv(ENV_XDG_CACHE_HOME, DEFAULT_CACHE_DIR), 'torch'
+ )
+ )
+ )
+ return torch_home
+
+ torch_home = _get_torch_home()
+ model_dir = os.path.join(torch_home, 'checkpoints')
+ try:
+ os.makedirs(model_dir)
+ except OSError as e:
+ if e.errno == errno.EEXIST:
+ # Directory already exists, ignore.
+ pass
+ else:
+ # Unexpected OSError, re-raise.
+ raise
+ filename = key + '_imagenet.pth'
+ cached_file = os.path.join(model_dir, filename)
+
+ if not os.path.exists(cached_file):
+ gdown.download(pretrained_urls[key], cached_file, quiet=False)
+
+ state_dict = torch.load(cached_file)
+ model_dict = model.state_dict()
+ new_state_dict = OrderedDict()
+ matched_layers, discarded_layers = [], []
+
+ for k, v in state_dict.items():
+ if k.startswith('module.'):
+ k = k[7:] # discard module.
+
+ if k in model_dict and model_dict[k].size() == v.size():
+ new_state_dict[k] = v
+ matched_layers.append(k)
+ else:
+ discarded_layers.append(k)
+
+ model_dict.update(new_state_dict)
+ model.load_state_dict(model_dict)
+
+ if len(matched_layers) == 0:
+ warnings.warn(
+ 'The pretrained weights from "{}" cannot be loaded, '
+ 'please check the key names manually '
+ '(** ignored and continue **)'.format(cached_file)
+ )
+ else:
+ print(
+ 'Successfully loaded imagenet pretrained weights from "{}"'.
+ format(cached_file)
+ )
+ if len(discarded_layers) > 0:
+ print(
+ '** The following layers are discarded '
+ 'due to unmatched keys or layer size: {}'.
+ format(discarded_layers)
+ )
+
+
+##########
+# Instantiation
+##########
+def osnet_ain_x1_0(
+ num_classes=1000, pretrained=True, loss='softmax', **kwargs
+):
+ model = OSNet(
+ num_classes,
+ blocks=[
+ [OSBlockINin, OSBlockINin], [OSBlock, OSBlockINin],
+ [OSBlockINin, OSBlock]
+ ],
+ layers=[2, 2, 2],
+ channels=[64, 256, 384, 512],
+ loss=loss,
+ conv1_IN=True,
+ **kwargs
+ )
+ if pretrained:
+ init_pretrained_weights(model, key='osnet_ain_x1_0')
+ return model
+
+
+def osnet_ain_x0_75(
+ num_classes=1000, pretrained=True, loss='softmax', **kwargs
+):
+ model = OSNet(
+ num_classes,
+ blocks=[
+ [OSBlockINin, OSBlockINin], [OSBlock, OSBlockINin],
+ [OSBlockINin, OSBlock]
+ ],
+ layers=[2, 2, 2],
+ channels=[48, 192, 288, 384],
+ loss=loss,
+ conv1_IN=True,
+ **kwargs
+ )
+ if pretrained:
+ init_pretrained_weights(model, key='osnet_ain_x0_75')
+ return model
+
+
+def osnet_ain_x0_5(
+ num_classes=1000, pretrained=True, loss='softmax', **kwargs
+):
+ model = OSNet(
+ num_classes,
+ blocks=[
+ [OSBlockINin, OSBlockINin], [OSBlock, OSBlockINin],
+ [OSBlockINin, OSBlock]
+ ],
+ layers=[2, 2, 2],
+ channels=[32, 128, 192, 256],
+ loss=loss,
+ conv1_IN=True,
+ **kwargs
+ )
+ if pretrained:
+ init_pretrained_weights(model, key='osnet_ain_x0_5')
+ return model
+
+
+def osnet_ain_x0_25(
+ num_classes=1000, pretrained=True, loss='softmax', **kwargs
+):
+ model = OSNet(
+ num_classes,
+ blocks=[
+ [OSBlockINin, OSBlockINin], [OSBlock, OSBlockINin],
+ [OSBlockINin, OSBlock]
+ ],
+ layers=[2, 2, 2],
+ channels=[16, 64, 96, 128],
+ loss=loss,
+ conv1_IN=True,
+ **kwargs
+ )
+ if pretrained:
+ init_pretrained_weights(model, key='osnet_ain_x0_25')
+ return model
diff --git a/trackers/strong_sort/deep/models/pcb.py b/trackers/strong_sort/deep/models/pcb.py
new file mode 100644
index 0000000000000000000000000000000000000000..92c74148763a600ed331bb0e361588fbf3b09189
--- /dev/null
+++ b/trackers/strong_sort/deep/models/pcb.py
@@ -0,0 +1,314 @@
+from __future__ import division, absolute_import
+import torch.utils.model_zoo as model_zoo
+from torch import nn
+from torch.nn import functional as F
+
+__all__ = ['pcb_p6', 'pcb_p4']
+
+model_urls = {
+ 'resnet18': 'https://download.pytorch.org/models/resnet18-5c106cde.pth',
+ 'resnet34': 'https://download.pytorch.org/models/resnet34-333f7ec4.pth',
+ 'resnet50': 'https://download.pytorch.org/models/resnet50-19c8e357.pth',
+ 'resnet101': 'https://download.pytorch.org/models/resnet101-5d3b4d8f.pth',
+ 'resnet152': 'https://download.pytorch.org/models/resnet152-b121ed2d.pth',
+}
+
+
+def conv3x3(in_planes, out_planes, stride=1):
+ """3x3 convolution with padding"""
+ return nn.Conv2d(
+ in_planes,
+ out_planes,
+ kernel_size=3,
+ stride=stride,
+ padding=1,
+ bias=False
+ )
+
+
+class BasicBlock(nn.Module):
+ expansion = 1
+
+ def __init__(self, inplanes, planes, stride=1, downsample=None):
+ super(BasicBlock, self).__init__()
+ self.conv1 = conv3x3(inplanes, planes, stride)
+ self.bn1 = nn.BatchNorm2d(planes)
+ self.relu = nn.ReLU(inplace=True)
+ self.conv2 = conv3x3(planes, planes)
+ self.bn2 = nn.BatchNorm2d(planes)
+ self.downsample = downsample
+ self.stride = stride
+
+ def forward(self, x):
+ residual = x
+
+ out = self.conv1(x)
+ out = self.bn1(out)
+ out = self.relu(out)
+
+ out = self.conv2(out)
+ out = self.bn2(out)
+
+ if self.downsample is not None:
+ residual = self.downsample(x)
+
+ out += residual
+ out = self.relu(out)
+
+ return out
+
+
+class Bottleneck(nn.Module):
+ expansion = 4
+
+ def __init__(self, inplanes, planes, stride=1, downsample=None):
+ super(Bottleneck, self).__init__()
+ self.conv1 = nn.Conv2d(inplanes, planes, kernel_size=1, bias=False)
+ self.bn1 = nn.BatchNorm2d(planes)
+ self.conv2 = nn.Conv2d(
+ planes,
+ planes,
+ kernel_size=3,
+ stride=stride,
+ padding=1,
+ bias=False
+ )
+ self.bn2 = nn.BatchNorm2d(planes)
+ self.conv3 = nn.Conv2d(
+ planes, planes * self.expansion, kernel_size=1, bias=False
+ )
+ self.bn3 = nn.BatchNorm2d(planes * self.expansion)
+ self.relu = nn.ReLU(inplace=True)
+ self.downsample = downsample
+ self.stride = stride
+
+ def forward(self, x):
+ residual = x
+
+ out = self.conv1(x)
+ out = self.bn1(out)
+ out = self.relu(out)
+
+ out = self.conv2(out)
+ out = self.bn2(out)
+ out = self.relu(out)
+
+ out = self.conv3(out)
+ out = self.bn3(out)
+
+ if self.downsample is not None:
+ residual = self.downsample(x)
+
+ out += residual
+ out = self.relu(out)
+
+ return out
+
+
+class DimReduceLayer(nn.Module):
+
+ def __init__(self, in_channels, out_channels, nonlinear):
+ super(DimReduceLayer, self).__init__()
+ layers = []
+ layers.append(
+ nn.Conv2d(
+ in_channels, out_channels, 1, stride=1, padding=0, bias=False
+ )
+ )
+ layers.append(nn.BatchNorm2d(out_channels))
+
+ if nonlinear == 'relu':
+ layers.append(nn.ReLU(inplace=True))
+ elif nonlinear == 'leakyrelu':
+ layers.append(nn.LeakyReLU(0.1))
+
+ self.layers = nn.Sequential(*layers)
+
+ def forward(self, x):
+ return self.layers(x)
+
+
+class PCB(nn.Module):
+ """Part-based Convolutional Baseline.
+
+ Reference:
+ Sun et al. Beyond Part Models: Person Retrieval with Refined
+ Part Pooling (and A Strong Convolutional Baseline). ECCV 2018.
+
+ Public keys:
+ - ``pcb_p4``: PCB with 4-part strips.
+ - ``pcb_p6``: PCB with 6-part strips.
+ """
+
+ def __init__(
+ self,
+ num_classes,
+ loss,
+ block,
+ layers,
+ parts=6,
+ reduced_dim=256,
+ nonlinear='relu',
+ **kwargs
+ ):
+ self.inplanes = 64
+ super(PCB, self).__init__()
+ self.loss = loss
+ self.parts = parts
+ self.feature_dim = 512 * block.expansion
+
+ # backbone network
+ self.conv1 = nn.Conv2d(
+ 3, 64, kernel_size=7, stride=2, padding=3, bias=False
+ )
+ self.bn1 = nn.BatchNorm2d(64)
+ self.relu = nn.ReLU(inplace=True)
+ self.maxpool = nn.MaxPool2d(kernel_size=3, stride=2, padding=1)
+ self.layer1 = self._make_layer(block, 64, layers[0])
+ self.layer2 = self._make_layer(block, 128, layers[1], stride=2)
+ self.layer3 = self._make_layer(block, 256, layers[2], stride=2)
+ self.layer4 = self._make_layer(block, 512, layers[3], stride=1)
+
+ # pcb layers
+ self.parts_avgpool = nn.AdaptiveAvgPool2d((self.parts, 1))
+ self.dropout = nn.Dropout(p=0.5)
+ self.conv5 = DimReduceLayer(
+ 512 * block.expansion, reduced_dim, nonlinear=nonlinear
+ )
+ self.feature_dim = reduced_dim
+ self.classifier = nn.ModuleList(
+ [
+ nn.Linear(self.feature_dim, num_classes)
+ for _ in range(self.parts)
+ ]
+ )
+
+ self._init_params()
+
+ def _make_layer(self, block, planes, blocks, stride=1):
+ downsample = None
+ if stride != 1 or self.inplanes != planes * block.expansion:
+ downsample = nn.Sequential(
+ nn.Conv2d(
+ self.inplanes,
+ planes * block.expansion,
+ kernel_size=1,
+ stride=stride,
+ bias=False
+ ),
+ nn.BatchNorm2d(planes * block.expansion),
+ )
+
+ layers = []
+ layers.append(block(self.inplanes, planes, stride, downsample))
+ self.inplanes = planes * block.expansion
+ for i in range(1, blocks):
+ layers.append(block(self.inplanes, planes))
+
+ return nn.Sequential(*layers)
+
+ def _init_params(self):
+ for m in self.modules():
+ if isinstance(m, nn.Conv2d):
+ nn.init.kaiming_normal_(
+ m.weight, mode='fan_out', nonlinearity='relu'
+ )
+ if m.bias is not None:
+ nn.init.constant_(m.bias, 0)
+ elif isinstance(m, nn.BatchNorm2d):
+ nn.init.constant_(m.weight, 1)
+ nn.init.constant_(m.bias, 0)
+ elif isinstance(m, nn.BatchNorm1d):
+ nn.init.constant_(m.weight, 1)
+ nn.init.constant_(m.bias, 0)
+ elif isinstance(m, nn.Linear):
+ nn.init.normal_(m.weight, 0, 0.01)
+ if m.bias is not None:
+ nn.init.constant_(m.bias, 0)
+
+ def featuremaps(self, x):
+ x = self.conv1(x)
+ x = self.bn1(x)
+ x = self.relu(x)
+ x = self.maxpool(x)
+ x = self.layer1(x)
+ x = self.layer2(x)
+ x = self.layer3(x)
+ x = self.layer4(x)
+ return x
+
+ def forward(self, x):
+ f = self.featuremaps(x)
+ v_g = self.parts_avgpool(f)
+
+ if not self.training:
+ v_g = F.normalize(v_g, p=2, dim=1)
+ return v_g.view(v_g.size(0), -1)
+
+ v_g = self.dropout(v_g)
+ v_h = self.conv5(v_g)
+
+ y = []
+ for i in range(self.parts):
+ v_h_i = v_h[:, :, i, :]
+ v_h_i = v_h_i.view(v_h_i.size(0), -1)
+ y_i = self.classifier[i](v_h_i)
+ y.append(y_i)
+
+ if self.loss == 'softmax':
+ return y
+ elif self.loss == 'triplet':
+ v_g = F.normalize(v_g, p=2, dim=1)
+ return y, v_g.view(v_g.size(0), -1)
+ else:
+ raise KeyError('Unsupported loss: {}'.format(self.loss))
+
+
+def init_pretrained_weights(model, model_url):
+ """Initializes model with pretrained weights.
+
+ Layers that don't match with pretrained layers in name or size are kept unchanged.
+ """
+ pretrain_dict = model_zoo.load_url(model_url)
+ model_dict = model.state_dict()
+ pretrain_dict = {
+ k: v
+ for k, v in pretrain_dict.items()
+ if k in model_dict and model_dict[k].size() == v.size()
+ }
+ model_dict.update(pretrain_dict)
+ model.load_state_dict(model_dict)
+
+
+def pcb_p6(num_classes, loss='softmax', pretrained=True, **kwargs):
+ model = PCB(
+ num_classes=num_classes,
+ loss=loss,
+ block=Bottleneck,
+ layers=[3, 4, 6, 3],
+ last_stride=1,
+ parts=6,
+ reduced_dim=256,
+ nonlinear='relu',
+ **kwargs
+ )
+ if pretrained:
+ init_pretrained_weights(model, model_urls['resnet50'])
+ return model
+
+
+def pcb_p4(num_classes, loss='softmax', pretrained=True, **kwargs):
+ model = PCB(
+ num_classes=num_classes,
+ loss=loss,
+ block=Bottleneck,
+ layers=[3, 4, 6, 3],
+ last_stride=1,
+ parts=4,
+ reduced_dim=256,
+ nonlinear='relu',
+ **kwargs
+ )
+ if pretrained:
+ init_pretrained_weights(model, model_urls['resnet50'])
+ return model
diff --git a/trackers/strong_sort/deep/models/resnet.py b/trackers/strong_sort/deep/models/resnet.py
new file mode 100644
index 0000000000000000000000000000000000000000..63d7f43ff43373d28c45de3930da0bdbee817b61
--- /dev/null
+++ b/trackers/strong_sort/deep/models/resnet.py
@@ -0,0 +1,530 @@
+"""
+Code source: https://github.com/pytorch/vision
+"""
+from __future__ import division, absolute_import
+import torch.utils.model_zoo as model_zoo
+from torch import nn
+
+__all__ = [
+ 'resnet18', 'resnet34', 'resnet50', 'resnet101', 'resnet152',
+ 'resnext50_32x4d', 'resnext101_32x8d', 'resnet50_fc512'
+]
+
+model_urls = {
+ 'resnet18':
+ 'https://download.pytorch.org/models/resnet18-5c106cde.pth',
+ 'resnet34':
+ 'https://download.pytorch.org/models/resnet34-333f7ec4.pth',
+ 'resnet50':
+ 'https://download.pytorch.org/models/resnet50-19c8e357.pth',
+ 'resnet101':
+ 'https://download.pytorch.org/models/resnet101-5d3b4d8f.pth',
+ 'resnet152':
+ 'https://download.pytorch.org/models/resnet152-b121ed2d.pth',
+ 'resnext50_32x4d':
+ 'https://download.pytorch.org/models/resnext50_32x4d-7cdf4587.pth',
+ 'resnext101_32x8d':
+ 'https://download.pytorch.org/models/resnext101_32x8d-8ba56ff5.pth',
+}
+
+
+def conv3x3(in_planes, out_planes, stride=1, groups=1, dilation=1):
+ """3x3 convolution with padding"""
+ return nn.Conv2d(
+ in_planes,
+ out_planes,
+ kernel_size=3,
+ stride=stride,
+ padding=dilation,
+ groups=groups,
+ bias=False,
+ dilation=dilation
+ )
+
+
+def conv1x1(in_planes, out_planes, stride=1):
+ """1x1 convolution"""
+ return nn.Conv2d(
+ in_planes, out_planes, kernel_size=1, stride=stride, bias=False
+ )
+
+
+class BasicBlock(nn.Module):
+ expansion = 1
+
+ def __init__(
+ self,
+ inplanes,
+ planes,
+ stride=1,
+ downsample=None,
+ groups=1,
+ base_width=64,
+ dilation=1,
+ norm_layer=None
+ ):
+ super(BasicBlock, self).__init__()
+ if norm_layer is None:
+ norm_layer = nn.BatchNorm2d
+ if groups != 1 or base_width != 64:
+ raise ValueError(
+ 'BasicBlock only supports groups=1 and base_width=64'
+ )
+ if dilation > 1:
+ raise NotImplementedError(
+ "Dilation > 1 not supported in BasicBlock"
+ )
+ # Both self.conv1 and self.downsample layers downsample the input when stride != 1
+ self.conv1 = conv3x3(inplanes, planes, stride)
+ self.bn1 = norm_layer(planes)
+ self.relu = nn.ReLU(inplace=True)
+ self.conv2 = conv3x3(planes, planes)
+ self.bn2 = norm_layer(planes)
+ self.downsample = downsample
+ self.stride = stride
+
+ def forward(self, x):
+ identity = x
+
+ out = self.conv1(x)
+ out = self.bn1(out)
+ out = self.relu(out)
+
+ out = self.conv2(out)
+ out = self.bn2(out)
+
+ if self.downsample is not None:
+ identity = self.downsample(x)
+
+ out += identity
+ out = self.relu(out)
+
+ return out
+
+
+class Bottleneck(nn.Module):
+ expansion = 4
+
+ def __init__(
+ self,
+ inplanes,
+ planes,
+ stride=1,
+ downsample=None,
+ groups=1,
+ base_width=64,
+ dilation=1,
+ norm_layer=None
+ ):
+ super(Bottleneck, self).__init__()
+ if norm_layer is None:
+ norm_layer = nn.BatchNorm2d
+ width = int(planes * (base_width/64.)) * groups
+ # Both self.conv2 and self.downsample layers downsample the input when stride != 1
+ self.conv1 = conv1x1(inplanes, width)
+ self.bn1 = norm_layer(width)
+ self.conv2 = conv3x3(width, width, stride, groups, dilation)
+ self.bn2 = norm_layer(width)
+ self.conv3 = conv1x1(width, planes * self.expansion)
+ self.bn3 = norm_layer(planes * self.expansion)
+ self.relu = nn.ReLU(inplace=True)
+ self.downsample = downsample
+ self.stride = stride
+
+ def forward(self, x):
+ identity = x
+
+ out = self.conv1(x)
+ out = self.bn1(out)
+ out = self.relu(out)
+
+ out = self.conv2(out)
+ out = self.bn2(out)
+ out = self.relu(out)
+
+ out = self.conv3(out)
+ out = self.bn3(out)
+
+ if self.downsample is not None:
+ identity = self.downsample(x)
+
+ out += identity
+ out = self.relu(out)
+
+ return out
+
+
+class ResNet(nn.Module):
+ """Residual network.
+
+ Reference:
+ - He et al. Deep Residual Learning for Image Recognition. CVPR 2016.
+ - Xie et al. Aggregated Residual Transformations for Deep Neural Networks. CVPR 2017.
+
+ Public keys:
+ - ``resnet18``: ResNet18.
+ - ``resnet34``: ResNet34.
+ - ``resnet50``: ResNet50.
+ - ``resnet101``: ResNet101.
+ - ``resnet152``: ResNet152.
+ - ``resnext50_32x4d``: ResNeXt50.
+ - ``resnext101_32x8d``: ResNeXt101.
+ - ``resnet50_fc512``: ResNet50 + FC.
+ """
+
+ def __init__(
+ self,
+ num_classes,
+ loss,
+ block,
+ layers,
+ zero_init_residual=False,
+ groups=1,
+ width_per_group=64,
+ replace_stride_with_dilation=None,
+ norm_layer=None,
+ last_stride=2,
+ fc_dims=None,
+ dropout_p=None,
+ **kwargs
+ ):
+ super(ResNet, self).__init__()
+ if norm_layer is None:
+ norm_layer = nn.BatchNorm2d
+ self._norm_layer = norm_layer
+ self.loss = loss
+ self.feature_dim = 512 * block.expansion
+ self.inplanes = 64
+ self.dilation = 1
+ if replace_stride_with_dilation is None:
+ # each element in the tuple indicates if we should replace
+ # the 2x2 stride with a dilated convolution instead
+ replace_stride_with_dilation = [False, False, False]
+ if len(replace_stride_with_dilation) != 3:
+ raise ValueError(
+ "replace_stride_with_dilation should be None "
+ "or a 3-element tuple, got {}".
+ format(replace_stride_with_dilation)
+ )
+ self.groups = groups
+ self.base_width = width_per_group
+ self.conv1 = nn.Conv2d(
+ 3, self.inplanes, kernel_size=7, stride=2, padding=3, bias=False
+ )
+ self.bn1 = norm_layer(self.inplanes)
+ self.relu = nn.ReLU(inplace=True)
+ self.maxpool = nn.MaxPool2d(kernel_size=3, stride=2, padding=1)
+ self.layer1 = self._make_layer(block, 64, layers[0])
+ self.layer2 = self._make_layer(
+ block,
+ 128,
+ layers[1],
+ stride=2,
+ dilate=replace_stride_with_dilation[0]
+ )
+ self.layer3 = self._make_layer(
+ block,
+ 256,
+ layers[2],
+ stride=2,
+ dilate=replace_stride_with_dilation[1]
+ )
+ self.layer4 = self._make_layer(
+ block,
+ 512,
+ layers[3],
+ stride=last_stride,
+ dilate=replace_stride_with_dilation[2]
+ )
+ self.global_avgpool = nn.AdaptiveAvgPool2d((1, 1))
+ self.fc = self._construct_fc_layer(
+ fc_dims, 512 * block.expansion, dropout_p
+ )
+ self.classifier = nn.Linear(self.feature_dim, num_classes)
+
+ self._init_params()
+
+ # Zero-initialize the last BN in each residual branch,
+ # so that the residual branch starts with zeros, and each residual block behaves like an identity.
+ # This improves the model by 0.2~0.3% according to https://arxiv.org/abs/1706.02677
+ if zero_init_residual:
+ for m in self.modules():
+ if isinstance(m, Bottleneck):
+ nn.init.constant_(m.bn3.weight, 0)
+ elif isinstance(m, BasicBlock):
+ nn.init.constant_(m.bn2.weight, 0)
+
+ def _make_layer(self, block, planes, blocks, stride=1, dilate=False):
+ norm_layer = self._norm_layer
+ downsample = None
+ previous_dilation = self.dilation
+ if dilate:
+ self.dilation *= stride
+ stride = 1
+ if stride != 1 or self.inplanes != planes * block.expansion:
+ downsample = nn.Sequential(
+ conv1x1(self.inplanes, planes * block.expansion, stride),
+ norm_layer(planes * block.expansion),
+ )
+
+ layers = []
+ layers.append(
+ block(
+ self.inplanes, planes, stride, downsample, self.groups,
+ self.base_width, previous_dilation, norm_layer
+ )
+ )
+ self.inplanes = planes * block.expansion
+ for _ in range(1, blocks):
+ layers.append(
+ block(
+ self.inplanes,
+ planes,
+ groups=self.groups,
+ base_width=self.base_width,
+ dilation=self.dilation,
+ norm_layer=norm_layer
+ )
+ )
+
+ return nn.Sequential(*layers)
+
+ def _construct_fc_layer(self, fc_dims, input_dim, dropout_p=None):
+ """Constructs fully connected layer
+
+ Args:
+ fc_dims (list or tuple): dimensions of fc layers, if None, no fc layers are constructed
+ input_dim (int): input dimension
+ dropout_p (float): dropout probability, if None, dropout is unused
+ """
+ if fc_dims is None:
+ self.feature_dim = input_dim
+ return None
+
+ assert isinstance(
+ fc_dims, (list, tuple)
+ ), 'fc_dims must be either list or tuple, but got {}'.format(
+ type(fc_dims)
+ )
+
+ layers = []
+ for dim in fc_dims:
+ layers.append(nn.Linear(input_dim, dim))
+ layers.append(nn.BatchNorm1d(dim))
+ layers.append(nn.ReLU(inplace=True))
+ if dropout_p is not None:
+ layers.append(nn.Dropout(p=dropout_p))
+ input_dim = dim
+
+ self.feature_dim = fc_dims[-1]
+
+ return nn.Sequential(*layers)
+
+ def _init_params(self):
+ for m in self.modules():
+ if isinstance(m, nn.Conv2d):
+ nn.init.kaiming_normal_(
+ m.weight, mode='fan_out', nonlinearity='relu'
+ )
+ if m.bias is not None:
+ nn.init.constant_(m.bias, 0)
+ elif isinstance(m, nn.BatchNorm2d):
+ nn.init.constant_(m.weight, 1)
+ nn.init.constant_(m.bias, 0)
+ elif isinstance(m, nn.BatchNorm1d):
+ nn.init.constant_(m.weight, 1)
+ nn.init.constant_(m.bias, 0)
+ elif isinstance(m, nn.Linear):
+ nn.init.normal_(m.weight, 0, 0.01)
+ if m.bias is not None:
+ nn.init.constant_(m.bias, 0)
+
+ def featuremaps(self, x):
+ x = self.conv1(x)
+ x = self.bn1(x)
+ x = self.relu(x)
+ x = self.maxpool(x)
+ x = self.layer1(x)
+ x = self.layer2(x)
+ x = self.layer3(x)
+ x = self.layer4(x)
+ return x
+
+ def forward(self, x):
+ f = self.featuremaps(x)
+ v = self.global_avgpool(f)
+ v = v.view(v.size(0), -1)
+
+ if self.fc is not None:
+ v = self.fc(v)
+
+ if not self.training:
+ return v
+
+ y = self.classifier(v)
+
+ if self.loss == 'softmax':
+ return y
+ elif self.loss == 'triplet':
+ return y, v
+ else:
+ raise KeyError("Unsupported loss: {}".format(self.loss))
+
+
+def init_pretrained_weights(model, model_url):
+ """Initializes model with pretrained weights.
+
+ Layers that don't match with pretrained layers in name or size are kept unchanged.
+ """
+ pretrain_dict = model_zoo.load_url(model_url)
+ model_dict = model.state_dict()
+ pretrain_dict = {
+ k: v
+ for k, v in pretrain_dict.items()
+ if k in model_dict and model_dict[k].size() == v.size()
+ }
+ model_dict.update(pretrain_dict)
+ model.load_state_dict(model_dict)
+
+
+"""ResNet"""
+
+
+def resnet18(num_classes, loss='softmax', pretrained=True, **kwargs):
+ model = ResNet(
+ num_classes=num_classes,
+ loss=loss,
+ block=BasicBlock,
+ layers=[2, 2, 2, 2],
+ last_stride=2,
+ fc_dims=None,
+ dropout_p=None,
+ **kwargs
+ )
+ if pretrained:
+ init_pretrained_weights(model, model_urls['resnet18'])
+ return model
+
+
+def resnet34(num_classes, loss='softmax', pretrained=True, **kwargs):
+ model = ResNet(
+ num_classes=num_classes,
+ loss=loss,
+ block=BasicBlock,
+ layers=[3, 4, 6, 3],
+ last_stride=2,
+ fc_dims=None,
+ dropout_p=None,
+ **kwargs
+ )
+ if pretrained:
+ init_pretrained_weights(model, model_urls['resnet34'])
+ return model
+
+
+def resnet50(num_classes, loss='softmax', pretrained=True, **kwargs):
+ model = ResNet(
+ num_classes=num_classes,
+ loss=loss,
+ block=Bottleneck,
+ layers=[3, 4, 6, 3],
+ last_stride=2,
+ fc_dims=None,
+ dropout_p=None,
+ **kwargs
+ )
+ if pretrained:
+ init_pretrained_weights(model, model_urls['resnet50'])
+ return model
+
+
+def resnet101(num_classes, loss='softmax', pretrained=True, **kwargs):
+ model = ResNet(
+ num_classes=num_classes,
+ loss=loss,
+ block=Bottleneck,
+ layers=[3, 4, 23, 3],
+ last_stride=2,
+ fc_dims=None,
+ dropout_p=None,
+ **kwargs
+ )
+ if pretrained:
+ init_pretrained_weights(model, model_urls['resnet101'])
+ return model
+
+
+def resnet152(num_classes, loss='softmax', pretrained=True, **kwargs):
+ model = ResNet(
+ num_classes=num_classes,
+ loss=loss,
+ block=Bottleneck,
+ layers=[3, 8, 36, 3],
+ last_stride=2,
+ fc_dims=None,
+ dropout_p=None,
+ **kwargs
+ )
+ if pretrained:
+ init_pretrained_weights(model, model_urls['resnet152'])
+ return model
+
+
+"""ResNeXt"""
+
+
+def resnext50_32x4d(num_classes, loss='softmax', pretrained=True, **kwargs):
+ model = ResNet(
+ num_classes=num_classes,
+ loss=loss,
+ block=Bottleneck,
+ layers=[3, 4, 6, 3],
+ last_stride=2,
+ fc_dims=None,
+ dropout_p=None,
+ groups=32,
+ width_per_group=4,
+ **kwargs
+ )
+ if pretrained:
+ init_pretrained_weights(model, model_urls['resnext50_32x4d'])
+ return model
+
+
+def resnext101_32x8d(num_classes, loss='softmax', pretrained=True, **kwargs):
+ model = ResNet(
+ num_classes=num_classes,
+ loss=loss,
+ block=Bottleneck,
+ layers=[3, 4, 23, 3],
+ last_stride=2,
+ fc_dims=None,
+ dropout_p=None,
+ groups=32,
+ width_per_group=8,
+ **kwargs
+ )
+ if pretrained:
+ init_pretrained_weights(model, model_urls['resnext101_32x8d'])
+ return model
+
+
+"""
+ResNet + FC
+"""
+
+
+def resnet50_fc512(num_classes, loss='softmax', pretrained=True, **kwargs):
+ model = ResNet(
+ num_classes=num_classes,
+ loss=loss,
+ block=Bottleneck,
+ layers=[3, 4, 6, 3],
+ last_stride=1,
+ fc_dims=[512],
+ dropout_p=None,
+ **kwargs
+ )
+ if pretrained:
+ init_pretrained_weights(model, model_urls['resnet50'])
+ return model
diff --git a/trackers/strong_sort/deep/models/resnet_ibn_a.py b/trackers/strong_sort/deep/models/resnet_ibn_a.py
new file mode 100644
index 0000000000000000000000000000000000000000..d198e7c9e361c40d25bc7eb1f352b971596ee124
--- /dev/null
+++ b/trackers/strong_sort/deep/models/resnet_ibn_a.py
@@ -0,0 +1,289 @@
+"""
+Credit to https://github.com/XingangPan/IBN-Net.
+"""
+from __future__ import division, absolute_import
+import math
+import torch
+import torch.nn as nn
+import torch.utils.model_zoo as model_zoo
+
+__all__ = ['resnet50_ibn_a']
+
+model_urls = {
+ 'resnet50': 'https://download.pytorch.org/models/resnet50-19c8e357.pth',
+ 'resnet101': 'https://download.pytorch.org/models/resnet101-5d3b4d8f.pth',
+ 'resnet152': 'https://download.pytorch.org/models/resnet152-b121ed2d.pth',
+}
+
+
+def conv3x3(in_planes, out_planes, stride=1):
+ "3x3 convolution with padding"
+ return nn.Conv2d(
+ in_planes,
+ out_planes,
+ kernel_size=3,
+ stride=stride,
+ padding=1,
+ bias=False
+ )
+
+
+class BasicBlock(nn.Module):
+ expansion = 1
+
+ def __init__(self, inplanes, planes, stride=1, downsample=None):
+ super(BasicBlock, self).__init__()
+ self.conv1 = conv3x3(inplanes, planes, stride)
+ self.bn1 = nn.BatchNorm2d(planes)
+ self.relu = nn.ReLU(inplace=True)
+ self.conv2 = conv3x3(planes, planes)
+ self.bn2 = nn.BatchNorm2d(planes)
+ self.downsample = downsample
+ self.stride = stride
+
+ def forward(self, x):
+ residual = x
+
+ out = self.conv1(x)
+ out = self.bn1(out)
+ out = self.relu(out)
+
+ out = self.conv2(out)
+ out = self.bn2(out)
+
+ if self.downsample is not None:
+ residual = self.downsample(x)
+
+ out += residual
+ out = self.relu(out)
+
+ return out
+
+
+class IBN(nn.Module):
+
+ def __init__(self, planes):
+ super(IBN, self).__init__()
+ half1 = int(planes / 2)
+ self.half = half1
+ half2 = planes - half1
+ self.IN = nn.InstanceNorm2d(half1, affine=True)
+ self.BN = nn.BatchNorm2d(half2)
+
+ def forward(self, x):
+ split = torch.split(x, self.half, 1)
+ out1 = self.IN(split[0].contiguous())
+ out2 = self.BN(split[1].contiguous())
+ out = torch.cat((out1, out2), 1)
+ return out
+
+
+class Bottleneck(nn.Module):
+ expansion = 4
+
+ def __init__(self, inplanes, planes, ibn=False, stride=1, downsample=None):
+ super(Bottleneck, self).__init__()
+ self.conv1 = nn.Conv2d(inplanes, planes, kernel_size=1, bias=False)
+ if ibn:
+ self.bn1 = IBN(planes)
+ else:
+ self.bn1 = nn.BatchNorm2d(planes)
+ self.conv2 = nn.Conv2d(
+ planes,
+ planes,
+ kernel_size=3,
+ stride=stride,
+ padding=1,
+ bias=False
+ )
+ self.bn2 = nn.BatchNorm2d(planes)
+ self.conv3 = nn.Conv2d(
+ planes, planes * self.expansion, kernel_size=1, bias=False
+ )
+ self.bn3 = nn.BatchNorm2d(planes * self.expansion)
+ self.relu = nn.ReLU(inplace=True)
+ self.downsample = downsample
+ self.stride = stride
+
+ def forward(self, x):
+ residual = x
+
+ out = self.conv1(x)
+ out = self.bn1(out)
+ out = self.relu(out)
+
+ out = self.conv2(out)
+ out = self.bn2(out)
+ out = self.relu(out)
+
+ out = self.conv3(out)
+ out = self.bn3(out)
+
+ if self.downsample is not None:
+ residual = self.downsample(x)
+
+ out += residual
+ out = self.relu(out)
+
+ return out
+
+
+class ResNet(nn.Module):
+ """Residual network + IBN layer.
+
+ Reference:
+ - He et al. Deep Residual Learning for Image Recognition. CVPR 2016.
+ - Pan et al. Two at Once: Enhancing Learning and Generalization
+ Capacities via IBN-Net. ECCV 2018.
+ """
+
+ def __init__(
+ self,
+ block,
+ layers,
+ num_classes=1000,
+ loss='softmax',
+ fc_dims=None,
+ dropout_p=None,
+ **kwargs
+ ):
+ scale = 64
+ self.inplanes = scale
+ super(ResNet, self).__init__()
+ self.loss = loss
+ self.feature_dim = scale * 8 * block.expansion
+
+ self.conv1 = nn.Conv2d(
+ 3, scale, kernel_size=7, stride=2, padding=3, bias=False
+ )
+ self.bn1 = nn.BatchNorm2d(scale)
+ self.relu = nn.ReLU(inplace=True)
+ self.maxpool = nn.MaxPool2d(kernel_size=3, stride=2, padding=1)
+ self.layer1 = self._make_layer(block, scale, layers[0])
+ self.layer2 = self._make_layer(block, scale * 2, layers[1], stride=2)
+ self.layer3 = self._make_layer(block, scale * 4, layers[2], stride=2)
+ self.layer4 = self._make_layer(block, scale * 8, layers[3], stride=2)
+ self.avgpool = nn.AdaptiveAvgPool2d((1, 1))
+ self.fc = self._construct_fc_layer(
+ fc_dims, scale * 8 * block.expansion, dropout_p
+ )
+ self.classifier = nn.Linear(self.feature_dim, num_classes)
+
+ for m in self.modules():
+ if isinstance(m, nn.Conv2d):
+ n = m.kernel_size[0] * m.kernel_size[1] * m.out_channels
+ m.weight.data.normal_(0, math.sqrt(2. / n))
+ elif isinstance(m, nn.BatchNorm2d):
+ m.weight.data.fill_(1)
+ m.bias.data.zero_()
+ elif isinstance(m, nn.InstanceNorm2d):
+ m.weight.data.fill_(1)
+ m.bias.data.zero_()
+
+ def _make_layer(self, block, planes, blocks, stride=1):
+ downsample = None
+ if stride != 1 or self.inplanes != planes * block.expansion:
+ downsample = nn.Sequential(
+ nn.Conv2d(
+ self.inplanes,
+ planes * block.expansion,
+ kernel_size=1,
+ stride=stride,
+ bias=False
+ ),
+ nn.BatchNorm2d(planes * block.expansion),
+ )
+
+ layers = []
+ ibn = True
+ if planes == 512:
+ ibn = False
+ layers.append(block(self.inplanes, planes, ibn, stride, downsample))
+ self.inplanes = planes * block.expansion
+ for i in range(1, blocks):
+ layers.append(block(self.inplanes, planes, ibn))
+
+ return nn.Sequential(*layers)
+
+ def _construct_fc_layer(self, fc_dims, input_dim, dropout_p=None):
+ """Constructs fully connected layer
+
+ Args:
+ fc_dims (list or tuple): dimensions of fc layers, if None, no fc layers are constructed
+ input_dim (int): input dimension
+ dropout_p (float): dropout probability, if None, dropout is unused
+ """
+ if fc_dims is None:
+ self.feature_dim = input_dim
+ return None
+
+ assert isinstance(
+ fc_dims, (list, tuple)
+ ), 'fc_dims must be either list or tuple, but got {}'.format(
+ type(fc_dims)
+ )
+
+ layers = []
+ for dim in fc_dims:
+ layers.append(nn.Linear(input_dim, dim))
+ layers.append(nn.BatchNorm1d(dim))
+ layers.append(nn.ReLU(inplace=True))
+ if dropout_p is not None:
+ layers.append(nn.Dropout(p=dropout_p))
+ input_dim = dim
+
+ self.feature_dim = fc_dims[-1]
+
+ return nn.Sequential(*layers)
+
+ def featuremaps(self, x):
+ x = self.conv1(x)
+ x = self.bn1(x)
+ x = self.relu(x)
+ x = self.maxpool(x)
+ x = self.layer1(x)
+ x = self.layer2(x)
+ x = self.layer3(x)
+ x = self.layer4(x)
+ return x
+
+ def forward(self, x):
+ f = self.featuremaps(x)
+ v = self.avgpool(f)
+ v = v.view(v.size(0), -1)
+ if self.fc is not None:
+ v = self.fc(v)
+ if not self.training:
+ return v
+ y = self.classifier(v)
+ if self.loss == 'softmax':
+ return y
+ elif self.loss == 'triplet':
+ return y, v
+ else:
+ raise KeyError("Unsupported loss: {}".format(self.loss))
+
+
+def init_pretrained_weights(model, model_url):
+ """Initializes model with pretrained weights.
+
+ Layers that don't match with pretrained layers in name or size are kept unchanged.
+ """
+ pretrain_dict = model_zoo.load_url(model_url)
+ model_dict = model.state_dict()
+ pretrain_dict = {
+ k: v
+ for k, v in pretrain_dict.items()
+ if k in model_dict and model_dict[k].size() == v.size()
+ }
+ model_dict.update(pretrain_dict)
+ model.load_state_dict(model_dict)
+
+
+def resnet50_ibn_a(num_classes, loss='softmax', pretrained=False, **kwargs):
+ model = ResNet(
+ Bottleneck, [3, 4, 6, 3], num_classes=num_classes, loss=loss, **kwargs
+ )
+ if pretrained:
+ init_pretrained_weights(model, model_urls['resnet50'])
+ return model
diff --git a/trackers/strong_sort/deep/models/resnet_ibn_b.py b/trackers/strong_sort/deep/models/resnet_ibn_b.py
new file mode 100644
index 0000000000000000000000000000000000000000..9881cc7d64e97a74bab35e6145197d6d740689ad
--- /dev/null
+++ b/trackers/strong_sort/deep/models/resnet_ibn_b.py
@@ -0,0 +1,274 @@
+"""
+Credit to https://github.com/XingangPan/IBN-Net.
+"""
+from __future__ import division, absolute_import
+import math
+import torch.nn as nn
+import torch.utils.model_zoo as model_zoo
+
+__all__ = ['resnet50_ibn_b']
+
+model_urls = {
+ 'resnet50': 'https://download.pytorch.org/models/resnet50-19c8e357.pth',
+ 'resnet101': 'https://download.pytorch.org/models/resnet101-5d3b4d8f.pth',
+ 'resnet152': 'https://download.pytorch.org/models/resnet152-b121ed2d.pth',
+}
+
+
+def conv3x3(in_planes, out_planes, stride=1):
+ "3x3 convolution with padding"
+ return nn.Conv2d(
+ in_planes,
+ out_planes,
+ kernel_size=3,
+ stride=stride,
+ padding=1,
+ bias=False
+ )
+
+
+class BasicBlock(nn.Module):
+ expansion = 1
+
+ def __init__(self, inplanes, planes, stride=1, downsample=None):
+ super(BasicBlock, self).__init__()
+ self.conv1 = conv3x3(inplanes, planes, stride)
+ self.bn1 = nn.BatchNorm2d(planes)
+ self.relu = nn.ReLU(inplace=True)
+ self.conv2 = conv3x3(planes, planes)
+ self.bn2 = nn.BatchNorm2d(planes)
+ self.downsample = downsample
+ self.stride = stride
+
+ def forward(self, x):
+ residual = x
+
+ out = self.conv1(x)
+ out = self.bn1(out)
+ out = self.relu(out)
+
+ out = self.conv2(out)
+ out = self.bn2(out)
+
+ if self.downsample is not None:
+ residual = self.downsample(x)
+
+ out += residual
+ out = self.relu(out)
+
+ return out
+
+
+class Bottleneck(nn.Module):
+ expansion = 4
+
+ def __init__(self, inplanes, planes, stride=1, downsample=None, IN=False):
+ super(Bottleneck, self).__init__()
+ self.conv1 = nn.Conv2d(inplanes, planes, kernel_size=1, bias=False)
+ self.bn1 = nn.BatchNorm2d(planes)
+ self.conv2 = nn.Conv2d(
+ planes,
+ planes,
+ kernel_size=3,
+ stride=stride,
+ padding=1,
+ bias=False
+ )
+ self.bn2 = nn.BatchNorm2d(planes)
+ self.conv3 = nn.Conv2d(
+ planes, planes * self.expansion, kernel_size=1, bias=False
+ )
+ self.bn3 = nn.BatchNorm2d(planes * self.expansion)
+ self.IN = None
+ if IN:
+ self.IN = nn.InstanceNorm2d(planes * 4, affine=True)
+ self.relu = nn.ReLU(inplace=True)
+ self.downsample = downsample
+ self.stride = stride
+
+ def forward(self, x):
+ residual = x
+
+ out = self.conv1(x)
+ out = self.bn1(out)
+ out = self.relu(out)
+
+ out = self.conv2(out)
+ out = self.bn2(out)
+ out = self.relu(out)
+
+ out = self.conv3(out)
+ out = self.bn3(out)
+
+ if self.downsample is not None:
+ residual = self.downsample(x)
+
+ out += residual
+ if self.IN is not None:
+ out = self.IN(out)
+ out = self.relu(out)
+
+ return out
+
+
+class ResNet(nn.Module):
+ """Residual network + IBN layer.
+
+ Reference:
+ - He et al. Deep Residual Learning for Image Recognition. CVPR 2016.
+ - Pan et al. Two at Once: Enhancing Learning and Generalization
+ Capacities via IBN-Net. ECCV 2018.
+ """
+
+ def __init__(
+ self,
+ block,
+ layers,
+ num_classes=1000,
+ loss='softmax',
+ fc_dims=None,
+ dropout_p=None,
+ **kwargs
+ ):
+ scale = 64
+ self.inplanes = scale
+ super(ResNet, self).__init__()
+ self.loss = loss
+ self.feature_dim = scale * 8 * block.expansion
+
+ self.conv1 = nn.Conv2d(
+ 3, scale, kernel_size=7, stride=2, padding=3, bias=False
+ )
+ self.bn1 = nn.InstanceNorm2d(scale, affine=True)
+ self.relu = nn.ReLU(inplace=True)
+ self.maxpool = nn.MaxPool2d(kernel_size=3, stride=2, padding=1)
+ self.layer1 = self._make_layer(
+ block, scale, layers[0], stride=1, IN=True
+ )
+ self.layer2 = self._make_layer(
+ block, scale * 2, layers[1], stride=2, IN=True
+ )
+ self.layer3 = self._make_layer(block, scale * 4, layers[2], stride=2)
+ self.layer4 = self._make_layer(block, scale * 8, layers[3], stride=2)
+ self.avgpool = nn.AdaptiveAvgPool2d((1, 1))
+ self.fc = self._construct_fc_layer(
+ fc_dims, scale * 8 * block.expansion, dropout_p
+ )
+ self.classifier = nn.Linear(self.feature_dim, num_classes)
+
+ for m in self.modules():
+ if isinstance(m, nn.Conv2d):
+ n = m.kernel_size[0] * m.kernel_size[1] * m.out_channels
+ m.weight.data.normal_(0, math.sqrt(2. / n))
+ elif isinstance(m, nn.BatchNorm2d):
+ m.weight.data.fill_(1)
+ m.bias.data.zero_()
+ elif isinstance(m, nn.InstanceNorm2d):
+ m.weight.data.fill_(1)
+ m.bias.data.zero_()
+
+ def _make_layer(self, block, planes, blocks, stride=1, IN=False):
+ downsample = None
+ if stride != 1 or self.inplanes != planes * block.expansion:
+ downsample = nn.Sequential(
+ nn.Conv2d(
+ self.inplanes,
+ planes * block.expansion,
+ kernel_size=1,
+ stride=stride,
+ bias=False
+ ),
+ nn.BatchNorm2d(planes * block.expansion),
+ )
+
+ layers = []
+ layers.append(block(self.inplanes, planes, stride, downsample))
+ self.inplanes = planes * block.expansion
+ for i in range(1, blocks - 1):
+ layers.append(block(self.inplanes, planes))
+ layers.append(block(self.inplanes, planes, IN=IN))
+
+ return nn.Sequential(*layers)
+
+ def _construct_fc_layer(self, fc_dims, input_dim, dropout_p=None):
+ """Constructs fully connected layer
+
+ Args:
+ fc_dims (list or tuple): dimensions of fc layers, if None, no fc layers are constructed
+ input_dim (int): input dimension
+ dropout_p (float): dropout probability, if None, dropout is unused
+ """
+ if fc_dims is None:
+ self.feature_dim = input_dim
+ return None
+
+ assert isinstance(
+ fc_dims, (list, tuple)
+ ), 'fc_dims must be either list or tuple, but got {}'.format(
+ type(fc_dims)
+ )
+
+ layers = []
+ for dim in fc_dims:
+ layers.append(nn.Linear(input_dim, dim))
+ layers.append(nn.BatchNorm1d(dim))
+ layers.append(nn.ReLU(inplace=True))
+ if dropout_p is not None:
+ layers.append(nn.Dropout(p=dropout_p))
+ input_dim = dim
+
+ self.feature_dim = fc_dims[-1]
+
+ return nn.Sequential(*layers)
+
+ def featuremaps(self, x):
+ x = self.conv1(x)
+ x = self.bn1(x)
+ x = self.relu(x)
+ x = self.maxpool(x)
+ x = self.layer1(x)
+ x = self.layer2(x)
+ x = self.layer3(x)
+ x = self.layer4(x)
+ return x
+
+ def forward(self, x):
+ f = self.featuremaps(x)
+ v = self.avgpool(f)
+ v = v.view(v.size(0), -1)
+ if self.fc is not None:
+ v = self.fc(v)
+ if not self.training:
+ return v
+ y = self.classifier(v)
+ if self.loss == 'softmax':
+ return y
+ elif self.loss == 'triplet':
+ return y, v
+ else:
+ raise KeyError("Unsupported loss: {}".format(self.loss))
+
+
+def init_pretrained_weights(model, model_url):
+ """Initializes model with pretrained weights.
+
+ Layers that don't match with pretrained layers in name or size are kept unchanged.
+ """
+ pretrain_dict = model_zoo.load_url(model_url)
+ model_dict = model.state_dict()
+ pretrain_dict = {
+ k: v
+ for k, v in pretrain_dict.items()
+ if k in model_dict and model_dict[k].size() == v.size()
+ }
+ model_dict.update(pretrain_dict)
+ model.load_state_dict(model_dict)
+
+
+def resnet50_ibn_b(num_classes, loss='softmax', pretrained=False, **kwargs):
+ model = ResNet(
+ Bottleneck, [3, 4, 6, 3], num_classes=num_classes, loss=loss, **kwargs
+ )
+ if pretrained:
+ init_pretrained_weights(model, model_urls['resnet50'])
+ return model
diff --git a/trackers/strong_sort/deep/models/resnetmid.py b/trackers/strong_sort/deep/models/resnetmid.py
new file mode 100644
index 0000000000000000000000000000000000000000..017f6c62653535a7b04566227d893cb4dfa2a34c
--- /dev/null
+++ b/trackers/strong_sort/deep/models/resnetmid.py
@@ -0,0 +1,307 @@
+from __future__ import division, absolute_import
+import torch
+import torch.utils.model_zoo as model_zoo
+from torch import nn
+
+__all__ = ['resnet50mid']
+
+model_urls = {
+ 'resnet18': 'https://download.pytorch.org/models/resnet18-5c106cde.pth',
+ 'resnet34': 'https://download.pytorch.org/models/resnet34-333f7ec4.pth',
+ 'resnet50': 'https://download.pytorch.org/models/resnet50-19c8e357.pth',
+ 'resnet101': 'https://download.pytorch.org/models/resnet101-5d3b4d8f.pth',
+ 'resnet152': 'https://download.pytorch.org/models/resnet152-b121ed2d.pth',
+}
+
+
+def conv3x3(in_planes, out_planes, stride=1):
+ """3x3 convolution with padding"""
+ return nn.Conv2d(
+ in_planes,
+ out_planes,
+ kernel_size=3,
+ stride=stride,
+ padding=1,
+ bias=False
+ )
+
+
+class BasicBlock(nn.Module):
+ expansion = 1
+
+ def __init__(self, inplanes, planes, stride=1, downsample=None):
+ super(BasicBlock, self).__init__()
+ self.conv1 = conv3x3(inplanes, planes, stride)
+ self.bn1 = nn.BatchNorm2d(planes)
+ self.relu = nn.ReLU(inplace=True)
+ self.conv2 = conv3x3(planes, planes)
+ self.bn2 = nn.BatchNorm2d(planes)
+ self.downsample = downsample
+ self.stride = stride
+
+ def forward(self, x):
+ residual = x
+
+ out = self.conv1(x)
+ out = self.bn1(out)
+ out = self.relu(out)
+
+ out = self.conv2(out)
+ out = self.bn2(out)
+
+ if self.downsample is not None:
+ residual = self.downsample(x)
+
+ out += residual
+ out = self.relu(out)
+
+ return out
+
+
+class Bottleneck(nn.Module):
+ expansion = 4
+
+ def __init__(self, inplanes, planes, stride=1, downsample=None):
+ super(Bottleneck, self).__init__()
+ self.conv1 = nn.Conv2d(inplanes, planes, kernel_size=1, bias=False)
+ self.bn1 = nn.BatchNorm2d(planes)
+ self.conv2 = nn.Conv2d(
+ planes,
+ planes,
+ kernel_size=3,
+ stride=stride,
+ padding=1,
+ bias=False
+ )
+ self.bn2 = nn.BatchNorm2d(planes)
+ self.conv3 = nn.Conv2d(
+ planes, planes * self.expansion, kernel_size=1, bias=False
+ )
+ self.bn3 = nn.BatchNorm2d(planes * self.expansion)
+ self.relu = nn.ReLU(inplace=True)
+ self.downsample = downsample
+ self.stride = stride
+
+ def forward(self, x):
+ residual = x
+
+ out = self.conv1(x)
+ out = self.bn1(out)
+ out = self.relu(out)
+
+ out = self.conv2(out)
+ out = self.bn2(out)
+ out = self.relu(out)
+
+ out = self.conv3(out)
+ out = self.bn3(out)
+
+ if self.downsample is not None:
+ residual = self.downsample(x)
+
+ out += residual
+ out = self.relu(out)
+
+ return out
+
+
+class ResNetMid(nn.Module):
+ """Residual network + mid-level features.
+
+ Reference:
+ Yu et al. The Devil is in the Middle: Exploiting Mid-level Representations for
+ Cross-Domain Instance Matching. arXiv:1711.08106.
+
+ Public keys:
+ - ``resnet50mid``: ResNet50 + mid-level feature fusion.
+ """
+
+ def __init__(
+ self,
+ num_classes,
+ loss,
+ block,
+ layers,
+ last_stride=2,
+ fc_dims=None,
+ **kwargs
+ ):
+ self.inplanes = 64
+ super(ResNetMid, self).__init__()
+ self.loss = loss
+ self.feature_dim = 512 * block.expansion
+
+ # backbone network
+ self.conv1 = nn.Conv2d(
+ 3, 64, kernel_size=7, stride=2, padding=3, bias=False
+ )
+ self.bn1 = nn.BatchNorm2d(64)
+ self.relu = nn.ReLU(inplace=True)
+ self.maxpool = nn.MaxPool2d(kernel_size=3, stride=2, padding=1)
+ self.layer1 = self._make_layer(block, 64, layers[0])
+ self.layer2 = self._make_layer(block, 128, layers[1], stride=2)
+ self.layer3 = self._make_layer(block, 256, layers[2], stride=2)
+ self.layer4 = self._make_layer(
+ block, 512, layers[3], stride=last_stride
+ )
+
+ self.global_avgpool = nn.AdaptiveAvgPool2d(1)
+ assert fc_dims is not None
+ self.fc_fusion = self._construct_fc_layer(
+ fc_dims, 512 * block.expansion * 2
+ )
+ self.feature_dim += 512 * block.expansion
+ self.classifier = nn.Linear(self.feature_dim, num_classes)
+
+ self._init_params()
+
+ def _make_layer(self, block, planes, blocks, stride=1):
+ downsample = None
+ if stride != 1 or self.inplanes != planes * block.expansion:
+ downsample = nn.Sequential(
+ nn.Conv2d(
+ self.inplanes,
+ planes * block.expansion,
+ kernel_size=1,
+ stride=stride,
+ bias=False
+ ),
+ nn.BatchNorm2d(planes * block.expansion),
+ )
+
+ layers = []
+ layers.append(block(self.inplanes, planes, stride, downsample))
+ self.inplanes = planes * block.expansion
+ for i in range(1, blocks):
+ layers.append(block(self.inplanes, planes))
+
+ return nn.Sequential(*layers)
+
+ def _construct_fc_layer(self, fc_dims, input_dim, dropout_p=None):
+ """Constructs fully connected layer
+
+ Args:
+ fc_dims (list or tuple): dimensions of fc layers, if None, no fc layers are constructed
+ input_dim (int): input dimension
+ dropout_p (float): dropout probability, if None, dropout is unused
+ """
+ if fc_dims is None:
+ self.feature_dim = input_dim
+ return None
+
+ assert isinstance(
+ fc_dims, (list, tuple)
+ ), 'fc_dims must be either list or tuple, but got {}'.format(
+ type(fc_dims)
+ )
+
+ layers = []
+ for dim in fc_dims:
+ layers.append(nn.Linear(input_dim, dim))
+ layers.append(nn.BatchNorm1d(dim))
+ layers.append(nn.ReLU(inplace=True))
+ if dropout_p is not None:
+ layers.append(nn.Dropout(p=dropout_p))
+ input_dim = dim
+
+ self.feature_dim = fc_dims[-1]
+
+ return nn.Sequential(*layers)
+
+ def _init_params(self):
+ for m in self.modules():
+ if isinstance(m, nn.Conv2d):
+ nn.init.kaiming_normal_(
+ m.weight, mode='fan_out', nonlinearity='relu'
+ )
+ if m.bias is not None:
+ nn.init.constant_(m.bias, 0)
+ elif isinstance(m, nn.BatchNorm2d):
+ nn.init.constant_(m.weight, 1)
+ nn.init.constant_(m.bias, 0)
+ elif isinstance(m, nn.BatchNorm1d):
+ nn.init.constant_(m.weight, 1)
+ nn.init.constant_(m.bias, 0)
+ elif isinstance(m, nn.Linear):
+ nn.init.normal_(m.weight, 0, 0.01)
+ if m.bias is not None:
+ nn.init.constant_(m.bias, 0)
+
+ def featuremaps(self, x):
+ x = self.conv1(x)
+ x = self.bn1(x)
+ x = self.relu(x)
+ x = self.maxpool(x)
+ x = self.layer1(x)
+ x = self.layer2(x)
+ x = self.layer3(x)
+ x4a = self.layer4[0](x)
+ x4b = self.layer4[1](x4a)
+ x4c = self.layer4[2](x4b)
+ return x4a, x4b, x4c
+
+ def forward(self, x):
+ x4a, x4b, x4c = self.featuremaps(x)
+
+ v4a = self.global_avgpool(x4a)
+ v4b = self.global_avgpool(x4b)
+ v4c = self.global_avgpool(x4c)
+ v4ab = torch.cat([v4a, v4b], 1)
+ v4ab = v4ab.view(v4ab.size(0), -1)
+ v4ab = self.fc_fusion(v4ab)
+ v4c = v4c.view(v4c.size(0), -1)
+ v = torch.cat([v4ab, v4c], 1)
+
+ if not self.training:
+ return v
+
+ y = self.classifier(v)
+
+ if self.loss == 'softmax':
+ return y
+ elif self.loss == 'triplet':
+ return y, v
+ else:
+ raise KeyError('Unsupported loss: {}'.format(self.loss))
+
+
+def init_pretrained_weights(model, model_url):
+ """Initializes model with pretrained weights.
+
+ Layers that don't match with pretrained layers in name or size are kept unchanged.
+ """
+ pretrain_dict = model_zoo.load_url(model_url)
+ model_dict = model.state_dict()
+ pretrain_dict = {
+ k: v
+ for k, v in pretrain_dict.items()
+ if k in model_dict and model_dict[k].size() == v.size()
+ }
+ model_dict.update(pretrain_dict)
+ model.load_state_dict(model_dict)
+
+
+"""
+Residual network configurations:
+--
+resnet18: block=BasicBlock, layers=[2, 2, 2, 2]
+resnet34: block=BasicBlock, layers=[3, 4, 6, 3]
+resnet50: block=Bottleneck, layers=[3, 4, 6, 3]
+resnet101: block=Bottleneck, layers=[3, 4, 23, 3]
+resnet152: block=Bottleneck, layers=[3, 8, 36, 3]
+"""
+
+
+def resnet50mid(num_classes, loss='softmax', pretrained=True, **kwargs):
+ model = ResNetMid(
+ num_classes=num_classes,
+ loss=loss,
+ block=Bottleneck,
+ layers=[3, 4, 6, 3],
+ last_stride=2,
+ fc_dims=[1024],
+ **kwargs
+ )
+ if pretrained:
+ init_pretrained_weights(model, model_urls['resnet50'])
+ return model
diff --git a/trackers/strong_sort/deep/models/senet.py b/trackers/strong_sort/deep/models/senet.py
new file mode 100644
index 0000000000000000000000000000000000000000..baaf9b0acbe8577bd5e574de47d3f9ef935946db
--- /dev/null
+++ b/trackers/strong_sort/deep/models/senet.py
@@ -0,0 +1,688 @@
+from __future__ import division, absolute_import
+import math
+from collections import OrderedDict
+import torch.nn as nn
+from torch.utils import model_zoo
+
+__all__ = [
+ 'senet154', 'se_resnet50', 'se_resnet101', 'se_resnet152',
+ 'se_resnext50_32x4d', 'se_resnext101_32x4d', 'se_resnet50_fc512'
+]
+"""
+Code imported from https://github.com/Cadene/pretrained-models.pytorch
+"""
+
+pretrained_settings = {
+ 'senet154': {
+ 'imagenet': {
+ 'url':
+ 'http://data.lip6.fr/cadene/pretrainedmodels/senet154-c7b49a05.pth',
+ 'input_space': 'RGB',
+ 'input_size': [3, 224, 224],
+ 'input_range': [0, 1],
+ 'mean': [0.485, 0.456, 0.406],
+ 'std': [0.229, 0.224, 0.225],
+ 'num_classes': 1000
+ }
+ },
+ 'se_resnet50': {
+ 'imagenet': {
+ 'url':
+ 'http://data.lip6.fr/cadene/pretrainedmodels/se_resnet50-ce0d4300.pth',
+ 'input_space': 'RGB',
+ 'input_size': [3, 224, 224],
+ 'input_range': [0, 1],
+ 'mean': [0.485, 0.456, 0.406],
+ 'std': [0.229, 0.224, 0.225],
+ 'num_classes': 1000
+ }
+ },
+ 'se_resnet101': {
+ 'imagenet': {
+ 'url':
+ 'http://data.lip6.fr/cadene/pretrainedmodels/se_resnet101-7e38fcc6.pth',
+ 'input_space': 'RGB',
+ 'input_size': [3, 224, 224],
+ 'input_range': [0, 1],
+ 'mean': [0.485, 0.456, 0.406],
+ 'std': [0.229, 0.224, 0.225],
+ 'num_classes': 1000
+ }
+ },
+ 'se_resnet152': {
+ 'imagenet': {
+ 'url':
+ 'http://data.lip6.fr/cadene/pretrainedmodels/se_resnet152-d17c99b7.pth',
+ 'input_space': 'RGB',
+ 'input_size': [3, 224, 224],
+ 'input_range': [0, 1],
+ 'mean': [0.485, 0.456, 0.406],
+ 'std': [0.229, 0.224, 0.225],
+ 'num_classes': 1000
+ }
+ },
+ 'se_resnext50_32x4d': {
+ 'imagenet': {
+ 'url':
+ 'http://data.lip6.fr/cadene/pretrainedmodels/se_resnext50_32x4d-a260b3a4.pth',
+ 'input_space': 'RGB',
+ 'input_size': [3, 224, 224],
+ 'input_range': [0, 1],
+ 'mean': [0.485, 0.456, 0.406],
+ 'std': [0.229, 0.224, 0.225],
+ 'num_classes': 1000
+ }
+ },
+ 'se_resnext101_32x4d': {
+ 'imagenet': {
+ 'url':
+ 'http://data.lip6.fr/cadene/pretrainedmodels/se_resnext101_32x4d-3b2fe3d8.pth',
+ 'input_space': 'RGB',
+ 'input_size': [3, 224, 224],
+ 'input_range': [0, 1],
+ 'mean': [0.485, 0.456, 0.406],
+ 'std': [0.229, 0.224, 0.225],
+ 'num_classes': 1000
+ }
+ },
+}
+
+
+class SEModule(nn.Module):
+
+ def __init__(self, channels, reduction):
+ super(SEModule, self).__init__()
+ self.avg_pool = nn.AdaptiveAvgPool2d(1)
+ self.fc1 = nn.Conv2d(
+ channels, channels // reduction, kernel_size=1, padding=0
+ )
+ self.relu = nn.ReLU(inplace=True)
+ self.fc2 = nn.Conv2d(
+ channels // reduction, channels, kernel_size=1, padding=0
+ )
+ self.sigmoid = nn.Sigmoid()
+
+ def forward(self, x):
+ module_input = x
+ x = self.avg_pool(x)
+ x = self.fc1(x)
+ x = self.relu(x)
+ x = self.fc2(x)
+ x = self.sigmoid(x)
+ return module_input * x
+
+
+class Bottleneck(nn.Module):
+ """
+ Base class for bottlenecks that implements `forward()` method.
+ """
+
+ def forward(self, x):
+ residual = x
+
+ out = self.conv1(x)
+ out = self.bn1(out)
+ out = self.relu(out)
+
+ out = self.conv2(out)
+ out = self.bn2(out)
+ out = self.relu(out)
+
+ out = self.conv3(out)
+ out = self.bn3(out)
+
+ if self.downsample is not None:
+ residual = self.downsample(x)
+
+ out = self.se_module(out) + residual
+ out = self.relu(out)
+
+ return out
+
+
+class SEBottleneck(Bottleneck):
+ """
+ Bottleneck for SENet154.
+ """
+ expansion = 4
+
+ def __init__(
+ self, inplanes, planes, groups, reduction, stride=1, downsample=None
+ ):
+ super(SEBottleneck, self).__init__()
+ self.conv1 = nn.Conv2d(inplanes, planes * 2, kernel_size=1, bias=False)
+ self.bn1 = nn.BatchNorm2d(planes * 2)
+ self.conv2 = nn.Conv2d(
+ planes * 2,
+ planes * 4,
+ kernel_size=3,
+ stride=stride,
+ padding=1,
+ groups=groups,
+ bias=False
+ )
+ self.bn2 = nn.BatchNorm2d(planes * 4)
+ self.conv3 = nn.Conv2d(
+ planes * 4, planes * 4, kernel_size=1, bias=False
+ )
+ self.bn3 = nn.BatchNorm2d(planes * 4)
+ self.relu = nn.ReLU(inplace=True)
+ self.se_module = SEModule(planes * 4, reduction=reduction)
+ self.downsample = downsample
+ self.stride = stride
+
+
+class SEResNetBottleneck(Bottleneck):
+ """
+ ResNet bottleneck with a Squeeze-and-Excitation module. It follows Caffe
+ implementation and uses `stride=stride` in `conv1` and not in `conv2`
+ (the latter is used in the torchvision implementation of ResNet).
+ """
+ expansion = 4
+
+ def __init__(
+ self, inplanes, planes, groups, reduction, stride=1, downsample=None
+ ):
+ super(SEResNetBottleneck, self).__init__()
+ self.conv1 = nn.Conv2d(
+ inplanes, planes, kernel_size=1, bias=False, stride=stride
+ )
+ self.bn1 = nn.BatchNorm2d(planes)
+ self.conv2 = nn.Conv2d(
+ planes,
+ planes,
+ kernel_size=3,
+ padding=1,
+ groups=groups,
+ bias=False
+ )
+ self.bn2 = nn.BatchNorm2d(planes)
+ self.conv3 = nn.Conv2d(planes, planes * 4, kernel_size=1, bias=False)
+ self.bn3 = nn.BatchNorm2d(planes * 4)
+ self.relu = nn.ReLU(inplace=True)
+ self.se_module = SEModule(planes * 4, reduction=reduction)
+ self.downsample = downsample
+ self.stride = stride
+
+
+class SEResNeXtBottleneck(Bottleneck):
+ """ResNeXt bottleneck type C with a Squeeze-and-Excitation module"""
+ expansion = 4
+
+ def __init__(
+ self,
+ inplanes,
+ planes,
+ groups,
+ reduction,
+ stride=1,
+ downsample=None,
+ base_width=4
+ ):
+ super(SEResNeXtBottleneck, self).__init__()
+ width = int(math.floor(planes * (base_width/64.)) * groups)
+ self.conv1 = nn.Conv2d(
+ inplanes, width, kernel_size=1, bias=False, stride=1
+ )
+ self.bn1 = nn.BatchNorm2d(width)
+ self.conv2 = nn.Conv2d(
+ width,
+ width,
+ kernel_size=3,
+ stride=stride,
+ padding=1,
+ groups=groups,
+ bias=False
+ )
+ self.bn2 = nn.BatchNorm2d(width)
+ self.conv3 = nn.Conv2d(width, planes * 4, kernel_size=1, bias=False)
+ self.bn3 = nn.BatchNorm2d(planes * 4)
+ self.relu = nn.ReLU(inplace=True)
+ self.se_module = SEModule(planes * 4, reduction=reduction)
+ self.downsample = downsample
+ self.stride = stride
+
+
+class SENet(nn.Module):
+ """Squeeze-and-excitation network.
+
+ Reference:
+ Hu et al. Squeeze-and-Excitation Networks. CVPR 2018.
+
+ Public keys:
+ - ``senet154``: SENet154.
+ - ``se_resnet50``: ResNet50 + SE.
+ - ``se_resnet101``: ResNet101 + SE.
+ - ``se_resnet152``: ResNet152 + SE.
+ - ``se_resnext50_32x4d``: ResNeXt50 (groups=32, width=4) + SE.
+ - ``se_resnext101_32x4d``: ResNeXt101 (groups=32, width=4) + SE.
+ - ``se_resnet50_fc512``: (ResNet50 + SE) + FC.
+ """
+
+ def __init__(
+ self,
+ num_classes,
+ loss,
+ block,
+ layers,
+ groups,
+ reduction,
+ dropout_p=0.2,
+ inplanes=128,
+ input_3x3=True,
+ downsample_kernel_size=3,
+ downsample_padding=1,
+ last_stride=2,
+ fc_dims=None,
+ **kwargs
+ ):
+ """
+ Parameters
+ ----------
+ block (nn.Module): Bottleneck class.
+ - For SENet154: SEBottleneck
+ - For SE-ResNet models: SEResNetBottleneck
+ - For SE-ResNeXt models: SEResNeXtBottleneck
+ layers (list of ints): Number of residual blocks for 4 layers of the
+ network (layer1...layer4).
+ groups (int): Number of groups for the 3x3 convolution in each
+ bottleneck block.
+ - For SENet154: 64
+ - For SE-ResNet models: 1
+ - For SE-ResNeXt models: 32
+ reduction (int): Reduction ratio for Squeeze-and-Excitation modules.
+ - For all models: 16
+ dropout_p (float or None): Drop probability for the Dropout layer.
+ If `None` the Dropout layer is not used.
+ - For SENet154: 0.2
+ - For SE-ResNet models: None
+ - For SE-ResNeXt models: None
+ inplanes (int): Number of input channels for layer1.
+ - For SENet154: 128
+ - For SE-ResNet models: 64
+ - For SE-ResNeXt models: 64
+ input_3x3 (bool): If `True`, use three 3x3 convolutions instead of
+ a single 7x7 convolution in layer0.
+ - For SENet154: True
+ - For SE-ResNet models: False
+ - For SE-ResNeXt models: False
+ downsample_kernel_size (int): Kernel size for downsampling convolutions
+ in layer2, layer3 and layer4.
+ - For SENet154: 3
+ - For SE-ResNet models: 1
+ - For SE-ResNeXt models: 1
+ downsample_padding (int): Padding for downsampling convolutions in
+ layer2, layer3 and layer4.
+ - For SENet154: 1
+ - For SE-ResNet models: 0
+ - For SE-ResNeXt models: 0
+ num_classes (int): Number of outputs in `classifier` layer.
+ """
+ super(SENet, self).__init__()
+ self.inplanes = inplanes
+ self.loss = loss
+
+ if input_3x3:
+ layer0_modules = [
+ (
+ 'conv1',
+ nn.Conv2d(3, 64, 3, stride=2, padding=1, bias=False)
+ ),
+ ('bn1', nn.BatchNorm2d(64)),
+ ('relu1', nn.ReLU(inplace=True)),
+ (
+ 'conv2',
+ nn.Conv2d(64, 64, 3, stride=1, padding=1, bias=False)
+ ),
+ ('bn2', nn.BatchNorm2d(64)),
+ ('relu2', nn.ReLU(inplace=True)),
+ (
+ 'conv3',
+ nn.Conv2d(
+ 64, inplanes, 3, stride=1, padding=1, bias=False
+ )
+ ),
+ ('bn3', nn.BatchNorm2d(inplanes)),
+ ('relu3', nn.ReLU(inplace=True)),
+ ]
+ else:
+ layer0_modules = [
+ (
+ 'conv1',
+ nn.Conv2d(
+ 3,
+ inplanes,
+ kernel_size=7,
+ stride=2,
+ padding=3,
+ bias=False
+ )
+ ),
+ ('bn1', nn.BatchNorm2d(inplanes)),
+ ('relu1', nn.ReLU(inplace=True)),
+ ]
+ # To preserve compatibility with Caffe weights `ceil_mode=True`
+ # is used instead of `padding=1`.
+ layer0_modules.append(
+ ('pool', nn.MaxPool2d(3, stride=2, ceil_mode=True))
+ )
+ self.layer0 = nn.Sequential(OrderedDict(layer0_modules))
+ self.layer1 = self._make_layer(
+ block,
+ planes=64,
+ blocks=layers[0],
+ groups=groups,
+ reduction=reduction,
+ downsample_kernel_size=1,
+ downsample_padding=0
+ )
+ self.layer2 = self._make_layer(
+ block,
+ planes=128,
+ blocks=layers[1],
+ stride=2,
+ groups=groups,
+ reduction=reduction,
+ downsample_kernel_size=downsample_kernel_size,
+ downsample_padding=downsample_padding
+ )
+ self.layer3 = self._make_layer(
+ block,
+ planes=256,
+ blocks=layers[2],
+ stride=2,
+ groups=groups,
+ reduction=reduction,
+ downsample_kernel_size=downsample_kernel_size,
+ downsample_padding=downsample_padding
+ )
+ self.layer4 = self._make_layer(
+ block,
+ planes=512,
+ blocks=layers[3],
+ stride=last_stride,
+ groups=groups,
+ reduction=reduction,
+ downsample_kernel_size=downsample_kernel_size,
+ downsample_padding=downsample_padding
+ )
+
+ self.global_avgpool = nn.AdaptiveAvgPool2d(1)
+ self.fc = self._construct_fc_layer(
+ fc_dims, 512 * block.expansion, dropout_p
+ )
+ self.classifier = nn.Linear(self.feature_dim, num_classes)
+
+ def _make_layer(
+ self,
+ block,
+ planes,
+ blocks,
+ groups,
+ reduction,
+ stride=1,
+ downsample_kernel_size=1,
+ downsample_padding=0
+ ):
+ downsample = None
+ if stride != 1 or self.inplanes != planes * block.expansion:
+ downsample = nn.Sequential(
+ nn.Conv2d(
+ self.inplanes,
+ planes * block.expansion,
+ kernel_size=downsample_kernel_size,
+ stride=stride,
+ padding=downsample_padding,
+ bias=False
+ ),
+ nn.BatchNorm2d(planes * block.expansion),
+ )
+
+ layers = []
+ layers.append(
+ block(
+ self.inplanes, planes, groups, reduction, stride, downsample
+ )
+ )
+ self.inplanes = planes * block.expansion
+ for i in range(1, blocks):
+ layers.append(block(self.inplanes, planes, groups, reduction))
+
+ return nn.Sequential(*layers)
+
+ def _construct_fc_layer(self, fc_dims, input_dim, dropout_p=None):
+ """
+ Construct fully connected layer
+
+ - fc_dims (list or tuple): dimensions of fc layers, if None,
+ no fc layers are constructed
+ - input_dim (int): input dimension
+ - dropout_p (float): dropout probability, if None, dropout is unused
+ """
+ if fc_dims is None:
+ self.feature_dim = input_dim
+ return None
+
+ assert isinstance(
+ fc_dims, (list, tuple)
+ ), 'fc_dims must be either list or tuple, but got {}'.format(
+ type(fc_dims)
+ )
+
+ layers = []
+ for dim in fc_dims:
+ layers.append(nn.Linear(input_dim, dim))
+ layers.append(nn.BatchNorm1d(dim))
+ layers.append(nn.ReLU(inplace=True))
+ if dropout_p is not None:
+ layers.append(nn.Dropout(p=dropout_p))
+ input_dim = dim
+
+ self.feature_dim = fc_dims[-1]
+
+ return nn.Sequential(*layers)
+
+ def featuremaps(self, x):
+ x = self.layer0(x)
+ x = self.layer1(x)
+ x = self.layer2(x)
+ x = self.layer3(x)
+ x = self.layer4(x)
+ return x
+
+ def forward(self, x):
+ f = self.featuremaps(x)
+ v = self.global_avgpool(f)
+ v = v.view(v.size(0), -1)
+
+ if self.fc is not None:
+ v = self.fc(v)
+
+ if not self.training:
+ return v
+
+ y = self.classifier(v)
+
+ if self.loss == 'softmax':
+ return y
+ elif self.loss == 'triplet':
+ return y, v
+ else:
+ raise KeyError("Unsupported loss: {}".format(self.loss))
+
+
+def init_pretrained_weights(model, model_url):
+ """Initializes model with pretrained weights.
+
+ Layers that don't match with pretrained layers in name or size are kept unchanged.
+ """
+ pretrain_dict = model_zoo.load_url(model_url)
+ model_dict = model.state_dict()
+ pretrain_dict = {
+ k: v
+ for k, v in pretrain_dict.items()
+ if k in model_dict and model_dict[k].size() == v.size()
+ }
+ model_dict.update(pretrain_dict)
+ model.load_state_dict(model_dict)
+
+
+def senet154(num_classes, loss='softmax', pretrained=True, **kwargs):
+ model = SENet(
+ num_classes=num_classes,
+ loss=loss,
+ block=SEBottleneck,
+ layers=[3, 8, 36, 3],
+ groups=64,
+ reduction=16,
+ dropout_p=0.2,
+ last_stride=2,
+ fc_dims=None,
+ **kwargs
+ )
+ if pretrained:
+ model_url = pretrained_settings['senet154']['imagenet']['url']
+ init_pretrained_weights(model, model_url)
+ return model
+
+
+def se_resnet50(num_classes, loss='softmax', pretrained=True, **kwargs):
+ model = SENet(
+ num_classes=num_classes,
+ loss=loss,
+ block=SEResNetBottleneck,
+ layers=[3, 4, 6, 3],
+ groups=1,
+ reduction=16,
+ dropout_p=None,
+ inplanes=64,
+ input_3x3=False,
+ downsample_kernel_size=1,
+ downsample_padding=0,
+ last_stride=2,
+ fc_dims=None,
+ **kwargs
+ )
+ if pretrained:
+ model_url = pretrained_settings['se_resnet50']['imagenet']['url']
+ init_pretrained_weights(model, model_url)
+ return model
+
+
+def se_resnet50_fc512(num_classes, loss='softmax', pretrained=True, **kwargs):
+ model = SENet(
+ num_classes=num_classes,
+ loss=loss,
+ block=SEResNetBottleneck,
+ layers=[3, 4, 6, 3],
+ groups=1,
+ reduction=16,
+ dropout_p=None,
+ inplanes=64,
+ input_3x3=False,
+ downsample_kernel_size=1,
+ downsample_padding=0,
+ last_stride=1,
+ fc_dims=[512],
+ **kwargs
+ )
+ if pretrained:
+ model_url = pretrained_settings['se_resnet50']['imagenet']['url']
+ init_pretrained_weights(model, model_url)
+ return model
+
+
+def se_resnet101(num_classes, loss='softmax', pretrained=True, **kwargs):
+ model = SENet(
+ num_classes=num_classes,
+ loss=loss,
+ block=SEResNetBottleneck,
+ layers=[3, 4, 23, 3],
+ groups=1,
+ reduction=16,
+ dropout_p=None,
+ inplanes=64,
+ input_3x3=False,
+ downsample_kernel_size=1,
+ downsample_padding=0,
+ last_stride=2,
+ fc_dims=None,
+ **kwargs
+ )
+ if pretrained:
+ model_url = pretrained_settings['se_resnet101']['imagenet']['url']
+ init_pretrained_weights(model, model_url)
+ return model
+
+
+def se_resnet152(num_classes, loss='softmax', pretrained=True, **kwargs):
+ model = SENet(
+ num_classes=num_classes,
+ loss=loss,
+ block=SEResNetBottleneck,
+ layers=[3, 8, 36, 3],
+ groups=1,
+ reduction=16,
+ dropout_p=None,
+ inplanes=64,
+ input_3x3=False,
+ downsample_kernel_size=1,
+ downsample_padding=0,
+ last_stride=2,
+ fc_dims=None,
+ **kwargs
+ )
+ if pretrained:
+ model_url = pretrained_settings['se_resnet152']['imagenet']['url']
+ init_pretrained_weights(model, model_url)
+ return model
+
+
+def se_resnext50_32x4d(num_classes, loss='softmax', pretrained=True, **kwargs):
+ model = SENet(
+ num_classes=num_classes,
+ loss=loss,
+ block=SEResNeXtBottleneck,
+ layers=[3, 4, 6, 3],
+ groups=32,
+ reduction=16,
+ dropout_p=None,
+ inplanes=64,
+ input_3x3=False,
+ downsample_kernel_size=1,
+ downsample_padding=0,
+ last_stride=2,
+ fc_dims=None,
+ **kwargs
+ )
+ if pretrained:
+ model_url = pretrained_settings['se_resnext50_32x4d']['imagenet']['url'
+ ]
+ init_pretrained_weights(model, model_url)
+ return model
+
+
+def se_resnext101_32x4d(
+ num_classes, loss='softmax', pretrained=True, **kwargs
+):
+ model = SENet(
+ num_classes=num_classes,
+ loss=loss,
+ block=SEResNeXtBottleneck,
+ layers=[3, 4, 23, 3],
+ groups=32,
+ reduction=16,
+ dropout_p=None,
+ inplanes=64,
+ input_3x3=False,
+ downsample_kernel_size=1,
+ downsample_padding=0,
+ last_stride=2,
+ fc_dims=None,
+ **kwargs
+ )
+ if pretrained:
+ model_url = pretrained_settings['se_resnext101_32x4d']['imagenet'][
+ 'url']
+ init_pretrained_weights(model, model_url)
+ return model
diff --git a/trackers/strong_sort/deep/models/shufflenet.py b/trackers/strong_sort/deep/models/shufflenet.py
new file mode 100644
index 0000000000000000000000000000000000000000..bc4d34f1c4a631aa981cfb1797b036f23aed4503
--- /dev/null
+++ b/trackers/strong_sort/deep/models/shufflenet.py
@@ -0,0 +1,198 @@
+from __future__ import division, absolute_import
+import torch
+import torch.utils.model_zoo as model_zoo
+from torch import nn
+from torch.nn import functional as F
+
+__all__ = ['shufflenet']
+
+model_urls = {
+ # training epoch = 90, top1 = 61.8
+ 'imagenet':
+ 'https://mega.nz/#!RDpUlQCY!tr_5xBEkelzDjveIYBBcGcovNCOrgfiJO9kiidz9fZM',
+}
+
+
+class ChannelShuffle(nn.Module):
+
+ def __init__(self, num_groups):
+ super(ChannelShuffle, self).__init__()
+ self.g = num_groups
+
+ def forward(self, x):
+ b, c, h, w = x.size()
+ n = c // self.g
+ # reshape
+ x = x.view(b, self.g, n, h, w)
+ # transpose
+ x = x.permute(0, 2, 1, 3, 4).contiguous()
+ # flatten
+ x = x.view(b, c, h, w)
+ return x
+
+
+class Bottleneck(nn.Module):
+
+ def __init__(
+ self,
+ in_channels,
+ out_channels,
+ stride,
+ num_groups,
+ group_conv1x1=True
+ ):
+ super(Bottleneck, self).__init__()
+ assert stride in [1, 2], 'Warning: stride must be either 1 or 2'
+ self.stride = stride
+ mid_channels = out_channels // 4
+ if stride == 2:
+ out_channels -= in_channels
+ # group conv is not applied to first conv1x1 at stage 2
+ num_groups_conv1x1 = num_groups if group_conv1x1 else 1
+ self.conv1 = nn.Conv2d(
+ in_channels,
+ mid_channels,
+ 1,
+ groups=num_groups_conv1x1,
+ bias=False
+ )
+ self.bn1 = nn.BatchNorm2d(mid_channels)
+ self.shuffle1 = ChannelShuffle(num_groups)
+ self.conv2 = nn.Conv2d(
+ mid_channels,
+ mid_channels,
+ 3,
+ stride=stride,
+ padding=1,
+ groups=mid_channels,
+ bias=False
+ )
+ self.bn2 = nn.BatchNorm2d(mid_channels)
+ self.conv3 = nn.Conv2d(
+ mid_channels, out_channels, 1, groups=num_groups, bias=False
+ )
+ self.bn3 = nn.BatchNorm2d(out_channels)
+ if stride == 2:
+ self.shortcut = nn.AvgPool2d(3, stride=2, padding=1)
+
+ def forward(self, x):
+ out = F.relu(self.bn1(self.conv1(x)))
+ out = self.shuffle1(out)
+ out = self.bn2(self.conv2(out))
+ out = self.bn3(self.conv3(out))
+ if self.stride == 2:
+ res = self.shortcut(x)
+ out = F.relu(torch.cat([res, out], 1))
+ else:
+ out = F.relu(x + out)
+ return out
+
+
+# configuration of (num_groups: #out_channels) based on Table 1 in the paper
+cfg = {
+ 1: [144, 288, 576],
+ 2: [200, 400, 800],
+ 3: [240, 480, 960],
+ 4: [272, 544, 1088],
+ 8: [384, 768, 1536],
+}
+
+
+class ShuffleNet(nn.Module):
+ """ShuffleNet.
+
+ Reference:
+ Zhang et al. ShuffleNet: An Extremely Efficient Convolutional Neural
+ Network for Mobile Devices. CVPR 2018.
+
+ Public keys:
+ - ``shufflenet``: ShuffleNet (groups=3).
+ """
+
+ def __init__(self, num_classes, loss='softmax', num_groups=3, **kwargs):
+ super(ShuffleNet, self).__init__()
+ self.loss = loss
+
+ self.conv1 = nn.Sequential(
+ nn.Conv2d(3, 24, 3, stride=2, padding=1, bias=False),
+ nn.BatchNorm2d(24),
+ nn.ReLU(),
+ nn.MaxPool2d(3, stride=2, padding=1),
+ )
+
+ self.stage2 = nn.Sequential(
+ Bottleneck(
+ 24, cfg[num_groups][0], 2, num_groups, group_conv1x1=False
+ ),
+ Bottleneck(cfg[num_groups][0], cfg[num_groups][0], 1, num_groups),
+ Bottleneck(cfg[num_groups][0], cfg[num_groups][0], 1, num_groups),
+ Bottleneck(cfg[num_groups][0], cfg[num_groups][0], 1, num_groups),
+ )
+
+ self.stage3 = nn.Sequential(
+ Bottleneck(cfg[num_groups][0], cfg[num_groups][1], 2, num_groups),
+ Bottleneck(cfg[num_groups][1], cfg[num_groups][1], 1, num_groups),
+ Bottleneck(cfg[num_groups][1], cfg[num_groups][1], 1, num_groups),
+ Bottleneck(cfg[num_groups][1], cfg[num_groups][1], 1, num_groups),
+ Bottleneck(cfg[num_groups][1], cfg[num_groups][1], 1, num_groups),
+ Bottleneck(cfg[num_groups][1], cfg[num_groups][1], 1, num_groups),
+ Bottleneck(cfg[num_groups][1], cfg[num_groups][1], 1, num_groups),
+ Bottleneck(cfg[num_groups][1], cfg[num_groups][1], 1, num_groups),
+ )
+
+ self.stage4 = nn.Sequential(
+ Bottleneck(cfg[num_groups][1], cfg[num_groups][2], 2, num_groups),
+ Bottleneck(cfg[num_groups][2], cfg[num_groups][2], 1, num_groups),
+ Bottleneck(cfg[num_groups][2], cfg[num_groups][2], 1, num_groups),
+ Bottleneck(cfg[num_groups][2], cfg[num_groups][2], 1, num_groups),
+ )
+
+ self.classifier = nn.Linear(cfg[num_groups][2], num_classes)
+ self.feat_dim = cfg[num_groups][2]
+
+ def forward(self, x):
+ x = self.conv1(x)
+ x = self.stage2(x)
+ x = self.stage3(x)
+ x = self.stage4(x)
+ x = F.avg_pool2d(x, x.size()[2:]).view(x.size(0), -1)
+
+ if not self.training:
+ return x
+
+ y = self.classifier(x)
+
+ if self.loss == 'softmax':
+ return y
+ elif self.loss == 'triplet':
+ return y, x
+ else:
+ raise KeyError('Unsupported loss: {}'.format(self.loss))
+
+
+def init_pretrained_weights(model, model_url):
+ """Initializes model with pretrained weights.
+
+ Layers that don't match with pretrained layers in name or size are kept unchanged.
+ """
+ pretrain_dict = model_zoo.load_url(model_url)
+ model_dict = model.state_dict()
+ pretrain_dict = {
+ k: v
+ for k, v in pretrain_dict.items()
+ if k in model_dict and model_dict[k].size() == v.size()
+ }
+ model_dict.update(pretrain_dict)
+ model.load_state_dict(model_dict)
+
+
+def shufflenet(num_classes, loss='softmax', pretrained=True, **kwargs):
+ model = ShuffleNet(num_classes, loss, **kwargs)
+ if pretrained:
+ # init_pretrained_weights(model, model_urls['imagenet'])
+ import warnings
+ warnings.warn(
+ 'The imagenet pretrained weights need to be manually downloaded from {}'
+ .format(model_urls['imagenet'])
+ )
+ return model
diff --git a/trackers/strong_sort/deep/models/shufflenetv2.py b/trackers/strong_sort/deep/models/shufflenetv2.py
new file mode 100644
index 0000000000000000000000000000000000000000..3ff879e8d731b4cb16a77cfa6892035656405f71
--- /dev/null
+++ b/trackers/strong_sort/deep/models/shufflenetv2.py
@@ -0,0 +1,262 @@
+"""
+Code source: https://github.com/pytorch/vision
+"""
+from __future__ import division, absolute_import
+import torch
+import torch.utils.model_zoo as model_zoo
+from torch import nn
+
+__all__ = [
+ 'shufflenet_v2_x0_5', 'shufflenet_v2_x1_0', 'shufflenet_v2_x1_5',
+ 'shufflenet_v2_x2_0'
+]
+
+model_urls = {
+ 'shufflenetv2_x0.5':
+ 'https://download.pytorch.org/models/shufflenetv2_x0.5-f707e7126e.pth',
+ 'shufflenetv2_x1.0':
+ 'https://download.pytorch.org/models/shufflenetv2_x1-5666bf0f80.pth',
+ 'shufflenetv2_x1.5': None,
+ 'shufflenetv2_x2.0': None,
+}
+
+
+def channel_shuffle(x, groups):
+ batchsize, num_channels, height, width = x.data.size()
+ channels_per_group = num_channels // groups
+
+ # reshape
+ x = x.view(batchsize, groups, channels_per_group, height, width)
+
+ x = torch.transpose(x, 1, 2).contiguous()
+
+ # flatten
+ x = x.view(batchsize, -1, height, width)
+
+ return x
+
+
+class InvertedResidual(nn.Module):
+
+ def __init__(self, inp, oup, stride):
+ super(InvertedResidual, self).__init__()
+
+ if not (1 <= stride <= 3):
+ raise ValueError('illegal stride value')
+ self.stride = stride
+
+ branch_features = oup // 2
+ assert (self.stride != 1) or (inp == branch_features << 1)
+
+ if self.stride > 1:
+ self.branch1 = nn.Sequential(
+ self.depthwise_conv(
+ inp, inp, kernel_size=3, stride=self.stride, padding=1
+ ),
+ nn.BatchNorm2d(inp),
+ nn.Conv2d(
+ inp,
+ branch_features,
+ kernel_size=1,
+ stride=1,
+ padding=0,
+ bias=False
+ ),
+ nn.BatchNorm2d(branch_features),
+ nn.ReLU(inplace=True),
+ )
+
+ self.branch2 = nn.Sequential(
+ nn.Conv2d(
+ inp if (self.stride > 1) else branch_features,
+ branch_features,
+ kernel_size=1,
+ stride=1,
+ padding=0,
+ bias=False
+ ),
+ nn.BatchNorm2d(branch_features),
+ nn.ReLU(inplace=True),
+ self.depthwise_conv(
+ branch_features,
+ branch_features,
+ kernel_size=3,
+ stride=self.stride,
+ padding=1
+ ),
+ nn.BatchNorm2d(branch_features),
+ nn.Conv2d(
+ branch_features,
+ branch_features,
+ kernel_size=1,
+ stride=1,
+ padding=0,
+ bias=False
+ ),
+ nn.BatchNorm2d(branch_features),
+ nn.ReLU(inplace=True),
+ )
+
+ @staticmethod
+ def depthwise_conv(i, o, kernel_size, stride=1, padding=0, bias=False):
+ return nn.Conv2d(
+ i, o, kernel_size, stride, padding, bias=bias, groups=i
+ )
+
+ def forward(self, x):
+ if self.stride == 1:
+ x1, x2 = x.chunk(2, dim=1)
+ out = torch.cat((x1, self.branch2(x2)), dim=1)
+ else:
+ out = torch.cat((self.branch1(x), self.branch2(x)), dim=1)
+
+ out = channel_shuffle(out, 2)
+
+ return out
+
+
+class ShuffleNetV2(nn.Module):
+ """ShuffleNetV2.
+
+ Reference:
+ Ma et al. ShuffleNet V2: Practical Guidelines for Efficient CNN Architecture Design. ECCV 2018.
+
+ Public keys:
+ - ``shufflenet_v2_x0_5``: ShuffleNetV2 x0.5.
+ - ``shufflenet_v2_x1_0``: ShuffleNetV2 x1.0.
+ - ``shufflenet_v2_x1_5``: ShuffleNetV2 x1.5.
+ - ``shufflenet_v2_x2_0``: ShuffleNetV2 x2.0.
+ """
+
+ def __init__(
+ self, num_classes, loss, stages_repeats, stages_out_channels, **kwargs
+ ):
+ super(ShuffleNetV2, self).__init__()
+ self.loss = loss
+
+ if len(stages_repeats) != 3:
+ raise ValueError(
+ 'expected stages_repeats as list of 3 positive ints'
+ )
+ if len(stages_out_channels) != 5:
+ raise ValueError(
+ 'expected stages_out_channels as list of 5 positive ints'
+ )
+ self._stage_out_channels = stages_out_channels
+
+ input_channels = 3
+ output_channels = self._stage_out_channels[0]
+ self.conv1 = nn.Sequential(
+ nn.Conv2d(input_channels, output_channels, 3, 2, 1, bias=False),
+ nn.BatchNorm2d(output_channels),
+ nn.ReLU(inplace=True),
+ )
+ input_channels = output_channels
+
+ self.maxpool = nn.MaxPool2d(kernel_size=3, stride=2, padding=1)
+
+ stage_names = ['stage{}'.format(i) for i in [2, 3, 4]]
+ for name, repeats, output_channels in zip(
+ stage_names, stages_repeats, self._stage_out_channels[1:]
+ ):
+ seq = [InvertedResidual(input_channels, output_channels, 2)]
+ for i in range(repeats - 1):
+ seq.append(
+ InvertedResidual(output_channels, output_channels, 1)
+ )
+ setattr(self, name, nn.Sequential(*seq))
+ input_channels = output_channels
+
+ output_channels = self._stage_out_channels[-1]
+ self.conv5 = nn.Sequential(
+ nn.Conv2d(input_channels, output_channels, 1, 1, 0, bias=False),
+ nn.BatchNorm2d(output_channels),
+ nn.ReLU(inplace=True),
+ )
+ self.global_avgpool = nn.AdaptiveAvgPool2d((1, 1))
+
+ self.classifier = nn.Linear(output_channels, num_classes)
+
+ def featuremaps(self, x):
+ x = self.conv1(x)
+ x = self.maxpool(x)
+ x = self.stage2(x)
+ x = self.stage3(x)
+ x = self.stage4(x)
+ x = self.conv5(x)
+ return x
+
+ def forward(self, x):
+ f = self.featuremaps(x)
+ v = self.global_avgpool(f)
+ v = v.view(v.size(0), -1)
+
+ if not self.training:
+ return v
+
+ y = self.classifier(v)
+
+ if self.loss == 'softmax':
+ return y
+ elif self.loss == 'triplet':
+ return y, v
+ else:
+ raise KeyError("Unsupported loss: {}".format(self.loss))
+
+
+def init_pretrained_weights(model, model_url):
+ """Initializes model with pretrained weights.
+
+ Layers that don't match with pretrained layers in name or size are kept unchanged.
+ """
+ if model_url is None:
+ import warnings
+ warnings.warn(
+ 'ImageNet pretrained weights are unavailable for this model'
+ )
+ return
+ pretrain_dict = model_zoo.load_url(model_url)
+ model_dict = model.state_dict()
+ pretrain_dict = {
+ k: v
+ for k, v in pretrain_dict.items()
+ if k in model_dict and model_dict[k].size() == v.size()
+ }
+ model_dict.update(pretrain_dict)
+ model.load_state_dict(model_dict)
+
+
+def shufflenet_v2_x0_5(num_classes, loss='softmax', pretrained=True, **kwargs):
+ model = ShuffleNetV2(
+ num_classes, loss, [4, 8, 4], [24, 48, 96, 192, 1024], **kwargs
+ )
+ if pretrained:
+ init_pretrained_weights(model, model_urls['shufflenetv2_x0.5'])
+ return model
+
+
+def shufflenet_v2_x1_0(num_classes, loss='softmax', pretrained=True, **kwargs):
+ model = ShuffleNetV2(
+ num_classes, loss, [4, 8, 4], [24, 116, 232, 464, 1024], **kwargs
+ )
+ if pretrained:
+ init_pretrained_weights(model, model_urls['shufflenetv2_x1.0'])
+ return model
+
+
+def shufflenet_v2_x1_5(num_classes, loss='softmax', pretrained=True, **kwargs):
+ model = ShuffleNetV2(
+ num_classes, loss, [4, 8, 4], [24, 176, 352, 704, 1024], **kwargs
+ )
+ if pretrained:
+ init_pretrained_weights(model, model_urls['shufflenetv2_x1.5'])
+ return model
+
+
+def shufflenet_v2_x2_0(num_classes, loss='softmax', pretrained=True, **kwargs):
+ model = ShuffleNetV2(
+ num_classes, loss, [4, 8, 4], [24, 244, 488, 976, 2048], **kwargs
+ )
+ if pretrained:
+ init_pretrained_weights(model, model_urls['shufflenetv2_x2.0'])
+ return model
diff --git a/trackers/strong_sort/deep/models/squeezenet.py b/trackers/strong_sort/deep/models/squeezenet.py
new file mode 100644
index 0000000000000000000000000000000000000000..83e8dc9fc46b4e76304bf1b681a14ce5b865b993
--- /dev/null
+++ b/trackers/strong_sort/deep/models/squeezenet.py
@@ -0,0 +1,236 @@
+"""
+Code source: https://github.com/pytorch/vision
+"""
+from __future__ import division, absolute_import
+import torch
+import torch.nn as nn
+import torch.utils.model_zoo as model_zoo
+
+__all__ = ['squeezenet1_0', 'squeezenet1_1', 'squeezenet1_0_fc512']
+
+model_urls = {
+ 'squeezenet1_0':
+ 'https://download.pytorch.org/models/squeezenet1_0-a815701f.pth',
+ 'squeezenet1_1':
+ 'https://download.pytorch.org/models/squeezenet1_1-f364aa15.pth',
+}
+
+
+class Fire(nn.Module):
+
+ def __init__(
+ self, inplanes, squeeze_planes, expand1x1_planes, expand3x3_planes
+ ):
+ super(Fire, self).__init__()
+ self.inplanes = inplanes
+ self.squeeze = nn.Conv2d(inplanes, squeeze_planes, kernel_size=1)
+ self.squeeze_activation = nn.ReLU(inplace=True)
+ self.expand1x1 = nn.Conv2d(
+ squeeze_planes, expand1x1_planes, kernel_size=1
+ )
+ self.expand1x1_activation = nn.ReLU(inplace=True)
+ self.expand3x3 = nn.Conv2d(
+ squeeze_planes, expand3x3_planes, kernel_size=3, padding=1
+ )
+ self.expand3x3_activation = nn.ReLU(inplace=True)
+
+ def forward(self, x):
+ x = self.squeeze_activation(self.squeeze(x))
+ return torch.cat(
+ [
+ self.expand1x1_activation(self.expand1x1(x)),
+ self.expand3x3_activation(self.expand3x3(x))
+ ], 1
+ )
+
+
+class SqueezeNet(nn.Module):
+ """SqueezeNet.
+
+ Reference:
+ Iandola et al. SqueezeNet: AlexNet-level accuracy with 50x fewer parameters
+ and< 0.5 MB model size. arXiv:1602.07360.
+
+ Public keys:
+ - ``squeezenet1_0``: SqueezeNet (version=1.0).
+ - ``squeezenet1_1``: SqueezeNet (version=1.1).
+ - ``squeezenet1_0_fc512``: SqueezeNet (version=1.0) + FC.
+ """
+
+ def __init__(
+ self,
+ num_classes,
+ loss,
+ version=1.0,
+ fc_dims=None,
+ dropout_p=None,
+ **kwargs
+ ):
+ super(SqueezeNet, self).__init__()
+ self.loss = loss
+ self.feature_dim = 512
+
+ if version not in [1.0, 1.1]:
+ raise ValueError(
+ 'Unsupported SqueezeNet version {version}:'
+ '1.0 or 1.1 expected'.format(version=version)
+ )
+
+ if version == 1.0:
+ self.features = nn.Sequential(
+ nn.Conv2d(3, 96, kernel_size=7, stride=2),
+ nn.ReLU(inplace=True),
+ nn.MaxPool2d(kernel_size=3, stride=2, ceil_mode=True),
+ Fire(96, 16, 64, 64),
+ Fire(128, 16, 64, 64),
+ Fire(128, 32, 128, 128),
+ nn.MaxPool2d(kernel_size=3, stride=2, ceil_mode=True),
+ Fire(256, 32, 128, 128),
+ Fire(256, 48, 192, 192),
+ Fire(384, 48, 192, 192),
+ Fire(384, 64, 256, 256),
+ nn.MaxPool2d(kernel_size=3, stride=2, ceil_mode=True),
+ Fire(512, 64, 256, 256),
+ )
+ else:
+ self.features = nn.Sequential(
+ nn.Conv2d(3, 64, kernel_size=3, stride=2),
+ nn.ReLU(inplace=True),
+ nn.MaxPool2d(kernel_size=3, stride=2, ceil_mode=True),
+ Fire(64, 16, 64, 64),
+ Fire(128, 16, 64, 64),
+ nn.MaxPool2d(kernel_size=3, stride=2, ceil_mode=True),
+ Fire(128, 32, 128, 128),
+ Fire(256, 32, 128, 128),
+ nn.MaxPool2d(kernel_size=3, stride=2, ceil_mode=True),
+ Fire(256, 48, 192, 192),
+ Fire(384, 48, 192, 192),
+ Fire(384, 64, 256, 256),
+ Fire(512, 64, 256, 256),
+ )
+
+ self.global_avgpool = nn.AdaptiveAvgPool2d(1)
+ self.fc = self._construct_fc_layer(fc_dims, 512, dropout_p)
+ self.classifier = nn.Linear(self.feature_dim, num_classes)
+
+ self._init_params()
+
+ def _construct_fc_layer(self, fc_dims, input_dim, dropout_p=None):
+ """Constructs fully connected layer
+
+ Args:
+ fc_dims (list or tuple): dimensions of fc layers, if None, no fc layers are constructed
+ input_dim (int): input dimension
+ dropout_p (float): dropout probability, if None, dropout is unused
+ """
+ if fc_dims is None:
+ self.feature_dim = input_dim
+ return None
+
+ assert isinstance(
+ fc_dims, (list, tuple)
+ ), 'fc_dims must be either list or tuple, but got {}'.format(
+ type(fc_dims)
+ )
+
+ layers = []
+ for dim in fc_dims:
+ layers.append(nn.Linear(input_dim, dim))
+ layers.append(nn.BatchNorm1d(dim))
+ layers.append(nn.ReLU(inplace=True))
+ if dropout_p is not None:
+ layers.append(nn.Dropout(p=dropout_p))
+ input_dim = dim
+
+ self.feature_dim = fc_dims[-1]
+
+ return nn.Sequential(*layers)
+
+ def _init_params(self):
+ for m in self.modules():
+ if isinstance(m, nn.Conv2d):
+ nn.init.kaiming_normal_(
+ m.weight, mode='fan_out', nonlinearity='relu'
+ )
+ if m.bias is not None:
+ nn.init.constant_(m.bias, 0)
+ elif isinstance(m, nn.BatchNorm2d):
+ nn.init.constant_(m.weight, 1)
+ nn.init.constant_(m.bias, 0)
+ elif isinstance(m, nn.BatchNorm1d):
+ nn.init.constant_(m.weight, 1)
+ nn.init.constant_(m.bias, 0)
+ elif isinstance(m, nn.Linear):
+ nn.init.normal_(m.weight, 0, 0.01)
+ if m.bias is not None:
+ nn.init.constant_(m.bias, 0)
+
+ def forward(self, x):
+ f = self.features(x)
+ v = self.global_avgpool(f)
+ v = v.view(v.size(0), -1)
+
+ if self.fc is not None:
+ v = self.fc(v)
+
+ if not self.training:
+ return v
+
+ y = self.classifier(v)
+
+ if self.loss == 'softmax':
+ return y
+ elif self.loss == 'triplet':
+ return y, v
+ else:
+ raise KeyError('Unsupported loss: {}'.format(self.loss))
+
+
+def init_pretrained_weights(model, model_url):
+ """Initializes model with pretrained weights.
+
+ Layers that don't match with pretrained layers in name or size are kept unchanged.
+ """
+ pretrain_dict = model_zoo.load_url(model_url, map_location=None)
+ model_dict = model.state_dict()
+ pretrain_dict = {
+ k: v
+ for k, v in pretrain_dict.items()
+ if k in model_dict and model_dict[k].size() == v.size()
+ }
+ model_dict.update(pretrain_dict)
+ model.load_state_dict(model_dict)
+
+
+def squeezenet1_0(num_classes, loss='softmax', pretrained=True, **kwargs):
+ model = SqueezeNet(
+ num_classes, loss, version=1.0, fc_dims=None, dropout_p=None, **kwargs
+ )
+ if pretrained:
+ init_pretrained_weights(model, model_urls['squeezenet1_0'])
+ return model
+
+
+def squeezenet1_0_fc512(
+ num_classes, loss='softmax', pretrained=True, **kwargs
+):
+ model = SqueezeNet(
+ num_classes,
+ loss,
+ version=1.0,
+ fc_dims=[512],
+ dropout_p=None,
+ **kwargs
+ )
+ if pretrained:
+ init_pretrained_weights(model, model_urls['squeezenet1_0'])
+ return model
+
+
+def squeezenet1_1(num_classes, loss='softmax', pretrained=True, **kwargs):
+ model = SqueezeNet(
+ num_classes, loss, version=1.1, fc_dims=None, dropout_p=None, **kwargs
+ )
+ if pretrained:
+ init_pretrained_weights(model, model_urls['squeezenet1_1'])
+ return model
diff --git a/trackers/strong_sort/deep/models/xception.py b/trackers/strong_sort/deep/models/xception.py
new file mode 100644
index 0000000000000000000000000000000000000000..43db4ab53283daf1267f2f4cc5f7d778daf4076a
--- /dev/null
+++ b/trackers/strong_sort/deep/models/xception.py
@@ -0,0 +1,344 @@
+from __future__ import division, absolute_import
+import torch.nn as nn
+import torch.nn.functional as F
+import torch.utils.model_zoo as model_zoo
+
+__all__ = ['xception']
+
+pretrained_settings = {
+ 'xception': {
+ 'imagenet': {
+ 'url':
+ 'http://data.lip6.fr/cadene/pretrainedmodels/xception-43020ad28.pth',
+ 'input_space': 'RGB',
+ 'input_size': [3, 299, 299],
+ 'input_range': [0, 1],
+ 'mean': [0.5, 0.5, 0.5],
+ 'std': [0.5, 0.5, 0.5],
+ 'num_classes': 1000,
+ 'scale':
+ 0.8975 # The resize parameter of the validation transform should be 333, and make sure to center crop at 299x299
+ }
+ }
+}
+
+
+class SeparableConv2d(nn.Module):
+
+ def __init__(
+ self,
+ in_channels,
+ out_channels,
+ kernel_size=1,
+ stride=1,
+ padding=0,
+ dilation=1,
+ bias=False
+ ):
+ super(SeparableConv2d, self).__init__()
+
+ self.conv1 = nn.Conv2d(
+ in_channels,
+ in_channels,
+ kernel_size,
+ stride,
+ padding,
+ dilation,
+ groups=in_channels,
+ bias=bias
+ )
+ self.pointwise = nn.Conv2d(
+ in_channels, out_channels, 1, 1, 0, 1, 1, bias=bias
+ )
+
+ def forward(self, x):
+ x = self.conv1(x)
+ x = self.pointwise(x)
+ return x
+
+
+class Block(nn.Module):
+
+ def __init__(
+ self,
+ in_filters,
+ out_filters,
+ reps,
+ strides=1,
+ start_with_relu=True,
+ grow_first=True
+ ):
+ super(Block, self).__init__()
+
+ if out_filters != in_filters or strides != 1:
+ self.skip = nn.Conv2d(
+ in_filters, out_filters, 1, stride=strides, bias=False
+ )
+ self.skipbn = nn.BatchNorm2d(out_filters)
+ else:
+ self.skip = None
+
+ self.relu = nn.ReLU(inplace=True)
+ rep = []
+
+ filters = in_filters
+ if grow_first:
+ rep.append(self.relu)
+ rep.append(
+ SeparableConv2d(
+ in_filters,
+ out_filters,
+ 3,
+ stride=1,
+ padding=1,
+ bias=False
+ )
+ )
+ rep.append(nn.BatchNorm2d(out_filters))
+ filters = out_filters
+
+ for i in range(reps - 1):
+ rep.append(self.relu)
+ rep.append(
+ SeparableConv2d(
+ filters, filters, 3, stride=1, padding=1, bias=False
+ )
+ )
+ rep.append(nn.BatchNorm2d(filters))
+
+ if not grow_first:
+ rep.append(self.relu)
+ rep.append(
+ SeparableConv2d(
+ in_filters,
+ out_filters,
+ 3,
+ stride=1,
+ padding=1,
+ bias=False
+ )
+ )
+ rep.append(nn.BatchNorm2d(out_filters))
+
+ if not start_with_relu:
+ rep = rep[1:]
+ else:
+ rep[0] = nn.ReLU(inplace=False)
+
+ if strides != 1:
+ rep.append(nn.MaxPool2d(3, strides, 1))
+ self.rep = nn.Sequential(*rep)
+
+ def forward(self, inp):
+ x = self.rep(inp)
+
+ if self.skip is not None:
+ skip = self.skip(inp)
+ skip = self.skipbn(skip)
+ else:
+ skip = inp
+
+ x += skip
+ return x
+
+
+class Xception(nn.Module):
+ """Xception.
+
+ Reference:
+ Chollet. Xception: Deep Learning with Depthwise
+ Separable Convolutions. CVPR 2017.
+
+ Public keys:
+ - ``xception``: Xception.
+ """
+
+ def __init__(
+ self, num_classes, loss, fc_dims=None, dropout_p=None, **kwargs
+ ):
+ super(Xception, self).__init__()
+ self.loss = loss
+
+ self.conv1 = nn.Conv2d(3, 32, 3, 2, 0, bias=False)
+ self.bn1 = nn.BatchNorm2d(32)
+
+ self.conv2 = nn.Conv2d(32, 64, 3, bias=False)
+ self.bn2 = nn.BatchNorm2d(64)
+
+ self.block1 = Block(
+ 64, 128, 2, 2, start_with_relu=False, grow_first=True
+ )
+ self.block2 = Block(
+ 128, 256, 2, 2, start_with_relu=True, grow_first=True
+ )
+ self.block3 = Block(
+ 256, 728, 2, 2, start_with_relu=True, grow_first=True
+ )
+
+ self.block4 = Block(
+ 728, 728, 3, 1, start_with_relu=True, grow_first=True
+ )
+ self.block5 = Block(
+ 728, 728, 3, 1, start_with_relu=True, grow_first=True
+ )
+ self.block6 = Block(
+ 728, 728, 3, 1, start_with_relu=True, grow_first=True
+ )
+ self.block7 = Block(
+ 728, 728, 3, 1, start_with_relu=True, grow_first=True
+ )
+
+ self.block8 = Block(
+ 728, 728, 3, 1, start_with_relu=True, grow_first=True
+ )
+ self.block9 = Block(
+ 728, 728, 3, 1, start_with_relu=True, grow_first=True
+ )
+ self.block10 = Block(
+ 728, 728, 3, 1, start_with_relu=True, grow_first=True
+ )
+ self.block11 = Block(
+ 728, 728, 3, 1, start_with_relu=True, grow_first=True
+ )
+
+ self.block12 = Block(
+ 728, 1024, 2, 2, start_with_relu=True, grow_first=False
+ )
+
+ self.conv3 = SeparableConv2d(1024, 1536, 3, 1, 1)
+ self.bn3 = nn.BatchNorm2d(1536)
+
+ self.conv4 = SeparableConv2d(1536, 2048, 3, 1, 1)
+ self.bn4 = nn.BatchNorm2d(2048)
+
+ self.global_avgpool = nn.AdaptiveAvgPool2d(1)
+ self.feature_dim = 2048
+ self.fc = self._construct_fc_layer(fc_dims, 2048, dropout_p)
+ self.classifier = nn.Linear(self.feature_dim, num_classes)
+
+ self._init_params()
+
+ def _construct_fc_layer(self, fc_dims, input_dim, dropout_p=None):
+ """Constructs fully connected layer.
+
+ Args:
+ fc_dims (list or tuple): dimensions of fc layers, if None, no fc layers are constructed
+ input_dim (int): input dimension
+ dropout_p (float): dropout probability, if None, dropout is unused
+ """
+ if fc_dims is None:
+ self.feature_dim = input_dim
+ return None
+
+ assert isinstance(
+ fc_dims, (list, tuple)
+ ), 'fc_dims must be either list or tuple, but got {}'.format(
+ type(fc_dims)
+ )
+
+ layers = []
+ for dim in fc_dims:
+ layers.append(nn.Linear(input_dim, dim))
+ layers.append(nn.BatchNorm1d(dim))
+ layers.append(nn.ReLU(inplace=True))
+ if dropout_p is not None:
+ layers.append(nn.Dropout(p=dropout_p))
+ input_dim = dim
+
+ self.feature_dim = fc_dims[-1]
+
+ return nn.Sequential(*layers)
+
+ def _init_params(self):
+ for m in self.modules():
+ if isinstance(m, nn.Conv2d):
+ nn.init.kaiming_normal_(
+ m.weight, mode='fan_out', nonlinearity='relu'
+ )
+ if m.bias is not None:
+ nn.init.constant_(m.bias, 0)
+ elif isinstance(m, nn.BatchNorm2d):
+ nn.init.constant_(m.weight, 1)
+ nn.init.constant_(m.bias, 0)
+ elif isinstance(m, nn.BatchNorm1d):
+ nn.init.constant_(m.weight, 1)
+ nn.init.constant_(m.bias, 0)
+ elif isinstance(m, nn.Linear):
+ nn.init.normal_(m.weight, 0, 0.01)
+ if m.bias is not None:
+ nn.init.constant_(m.bias, 0)
+
+ def featuremaps(self, input):
+ x = self.conv1(input)
+ x = self.bn1(x)
+ x = F.relu(x, inplace=True)
+
+ x = self.conv2(x)
+ x = self.bn2(x)
+ x = F.relu(x, inplace=True)
+
+ x = self.block1(x)
+ x = self.block2(x)
+ x = self.block3(x)
+ x = self.block4(x)
+ x = self.block5(x)
+ x = self.block6(x)
+ x = self.block7(x)
+ x = self.block8(x)
+ x = self.block9(x)
+ x = self.block10(x)
+ x = self.block11(x)
+ x = self.block12(x)
+
+ x = self.conv3(x)
+ x = self.bn3(x)
+ x = F.relu(x, inplace=True)
+
+ x = self.conv4(x)
+ x = self.bn4(x)
+ x = F.relu(x, inplace=True)
+ return x
+
+ def forward(self, x):
+ f = self.featuremaps(x)
+ v = self.global_avgpool(f)
+ v = v.view(v.size(0), -1)
+
+ if self.fc is not None:
+ v = self.fc(v)
+
+ if not self.training:
+ return v
+
+ y = self.classifier(v)
+
+ if self.loss == 'softmax':
+ return y
+ elif self.loss == 'triplet':
+ return y, v
+ else:
+ raise KeyError('Unsupported loss: {}'.format(self.loss))
+
+
+def init_pretrained_weights(model, model_url):
+ """Initialize models with pretrained weights.
+
+ Layers that don't match with pretrained layers in name or size are kept unchanged.
+ """
+ pretrain_dict = model_zoo.load_url(model_url)
+ model_dict = model.state_dict()
+ pretrain_dict = {
+ k: v
+ for k, v in pretrain_dict.items()
+ if k in model_dict and model_dict[k].size() == v.size()
+ }
+ model_dict.update(pretrain_dict)
+ model.load_state_dict(model_dict)
+
+
+def xception(num_classes, loss='softmax', pretrained=True, **kwargs):
+ model = Xception(num_classes, loss, fc_dims=None, dropout_p=None, **kwargs)
+ if pretrained:
+ model_url = pretrained_settings['xception']['imagenet']['url']
+ init_pretrained_weights(model, model_url)
+ return model
diff --git a/trackers/strong_sort/deep/reid/.flake8 b/trackers/strong_sort/deep/reid/.flake8
new file mode 100644
index 0000000000000000000000000000000000000000..4fc103cb101294ae7c3ad8ed536feb660ae6c0d6
--- /dev/null
+++ b/trackers/strong_sort/deep/reid/.flake8
@@ -0,0 +1,18 @@
+[flake8]
+ignore =
+ # At least two spaces before inline comment
+ E261,
+ # Line lengths are recommended to be no greater than 79 characters
+ E501,
+ # Missing whitespace around arithmetic operator
+ E226,
+ # Blank line contains whitespace
+ W293,
+ # Do not use bare 'except'
+ E722,
+ # Line break after binary operator
+ W504,
+ # isort found an import in the wrong position
+ I001
+max-line-length = 79
+exclude = __init__.py, build, torchreid/metrics/rank_cylib/
\ No newline at end of file
diff --git a/trackers/strong_sort/deep/reid/.gitignore b/trackers/strong_sort/deep/reid/.gitignore
new file mode 100644
index 0000000000000000000000000000000000000000..b966cd94452c8c367a7506cf165fe2cdced0c3be
--- /dev/null
+++ b/trackers/strong_sort/deep/reid/.gitignore
@@ -0,0 +1,141 @@
+.idea/
+# Byte-compiled / optimized / DLL files
+__pycache__/
+*.py[cod]
+*$py.class
+
+# C extensions
+*.so
+
+# Distribution / packaging
+.Python
+build/
+develop-eggs/
+dist/
+downloads/
+eggs/
+.eggs/
+lib/
+lib64/
+parts/
+sdist/
+var/
+wheels/
+pip-wheel-metadata/
+share/python-wheels/
+*.egg-info/
+.installed.cfg
+*.egg
+MANIFEST
+
+# PyInstaller
+# Usually these files are written by a python script from a template
+# before PyInstaller builds the exe, so as to inject date/other infos into it.
+*.manifest
+*.spec
+
+# Installer logs
+pip-log.txt
+pip-delete-this-directory.txt
+
+# Unit test / coverage reports
+htmlcov/
+.tox/
+.nox/
+.coverage
+.coverage.*
+.cache
+nosetests.xml
+coverage.xml
+*.cover
+.hypothesis/
+.pytest_cache/
+
+# Translations
+*.mo
+*.pot
+
+# Django stuff:
+*.log
+local_settings.py
+db.sqlite3
+
+# Flask stuff:
+instance/
+.webassets-cache
+
+# Scrapy stuff:
+.scrapy
+
+# Sphinx documentation
+docs/_build/
+
+# PyBuilder
+target/
+
+# Jupyter Notebook
+.ipynb_checkpoints
+
+# IPython
+profile_default/
+ipython_config.py
+
+# pyenv
+.python-version
+
+# pipenv
+# According to pypa/pipenv#598, it is recommended to include Pipfile.lock in version control.
+# However, in case of collaboration, if having platform-specific dependencies or dependencies
+# having no cross-platform support, pipenv may install dependencies that don't work, or not
+# install all needed dependencies.
+#Pipfile.lock
+
+# celery beat schedule file
+celerybeat-schedule
+
+# SageMath parsed files
+*.sage.py
+
+# Environments
+.env
+.venv
+env/
+venv/
+ENV/
+env.bak/
+venv.bak/
+
+# Spyder project settings
+.spyderproject
+.spyproject
+
+# Rope project settings
+.ropeproject
+
+# mkdocs documentation
+/site
+
+# mypy
+.mypy_cache/
+.dmypy.json
+dmypy.json
+
+# Pyre type checker
+.pyre/
+
+# Cython eval code
+*.c
+*.html
+
+# OS X
+.DS_Store
+.Spotlight-V100
+.Trashes
+._*
+
+# ReID
+reid-data/
+log/
+saved-models/
+model-zoo/
+debug*
diff --git a/trackers/strong_sort/deep/reid/.isort.cfg b/trackers/strong_sort/deep/reid/.isort.cfg
new file mode 100644
index 0000000000000000000000000000000000000000..8039326b5c5825760806cfb5e1667fc0815fc3f2
--- /dev/null
+++ b/trackers/strong_sort/deep/reid/.isort.cfg
@@ -0,0 +1,10 @@
+[isort]
+line_length=79
+multi_line_output=3
+length_sort=true
+known_standard_library=numpy,setuptools
+known_myself=torchreid
+known_third_party=matplotlib,cv2,torch,torchvision,PIL,yacs
+no_lines_before=STDLIB,THIRDPARTY
+sections=FUTURE,STDLIB,THIRDPARTY,myself,FIRSTPARTY,LOCALFOLDER
+default_section=FIRSTPARTY
\ No newline at end of file
diff --git a/trackers/strong_sort/deep/reid/.style.yapf b/trackers/strong_sort/deep/reid/.style.yapf
new file mode 100644
index 0000000000000000000000000000000000000000..29d8e52cc8d04fb2f6a71cdb400fb9e55bdeea45
--- /dev/null
+++ b/trackers/strong_sort/deep/reid/.style.yapf
@@ -0,0 +1,7 @@
+[style]
+BASED_ON_STYLE = pep8
+BLANK_LINE_BEFORE_NESTED_CLASS_OR_DEF = true
+SPLIT_BEFORE_EXPRESSION_AFTER_OPENING_PAREN = true
+DEDENT_CLOSING_BRACKETS = true
+SPACES_BEFORE_COMMENT = 1
+ARITHMETIC_PRECEDENCE_INDICATION = true
\ No newline at end of file
diff --git a/trackers/strong_sort/deep/reid/Dockerfile b/trackers/strong_sort/deep/reid/Dockerfile
new file mode 100644
index 0000000000000000000000000000000000000000..b5372979b2e9021d7bf553ed4376e267a3c50351
--- /dev/null
+++ b/trackers/strong_sort/deep/reid/Dockerfile
@@ -0,0 +1,22 @@
+FROM nvidia/cuda:11.1.1-cudnn8-devel-ubuntu18.04
+
+ENV DEBIAN_FRONTEND noninteractive
+RUN apt-get update && apt-get install -y \
+ python3-opencv ca-certificates python3-dev git wget sudo ninja-build
+RUN ln -sv /usr/bin/python3 /usr/bin/python
+
+COPY . /home/appuser
+WORKDIR /home/appuser
+
+# https://github.com/facebookresearch/detectron2/issues/3933
+ENV PATH="/home/appuser/.local/bin:${PATH}"
+RUN wget https://bootstrap.pypa.io/pip/3.6/get-pip.py && \
+ python3 get-pip.py && \
+ rm get-pip.py
+
+# install dependencies
+# See https://pytorch.org/ for other options if you use a different version of CUDA
+RUN pip install -r requirements.txt
+RUN pip install torch==1.10 torchvision==0.11.1 -f https://download.pytorch.org/whl/cu111/torch_stable.html
+RUN pip install setuptools==59.5.0
+RUN python setup.py develop
diff --git a/trackers/strong_sort/deep/reid/LICENSE b/trackers/strong_sort/deep/reid/LICENSE
new file mode 100644
index 0000000000000000000000000000000000000000..d2bcb88271ffbb1b7255fe202c562d590e91b533
--- /dev/null
+++ b/trackers/strong_sort/deep/reid/LICENSE
@@ -0,0 +1,21 @@
+MIT License
+
+Copyright (c) 2018 Kaiyang Zhou
+
+Permission is hereby granted, free of charge, to any person obtaining a copy
+of this software and associated documentation files (the "Software"), to deal
+in the Software without restriction, including without limitation the rights
+to use, copy, modify, merge, publish, distribute, sublicense, and/or sell
+copies of the Software, and to permit persons to whom the Software is
+furnished to do so, subject to the following conditions:
+
+The above copyright notice and this permission notice shall be included in all
+copies or substantial portions of the Software.
+
+THE SOFTWARE IS PROVIDED "AS IS", WITHOUT WARRANTY OF ANY KIND, EXPRESS OR
+IMPLIED, INCLUDING BUT NOT LIMITED TO THE WARRANTIES OF MERCHANTABILITY,
+FITNESS FOR A PARTICULAR PURPOSE AND NONINFRINGEMENT. IN NO EVENT SHALL THE
+AUTHORS OR COPYRIGHT HOLDERS BE LIABLE FOR ANY CLAIM, DAMAGES OR OTHER
+LIABILITY, WHETHER IN AN ACTION OF CONTRACT, TORT OR OTHERWISE, ARISING FROM,
+OUT OF OR IN CONNECTION WITH THE SOFTWARE OR THE USE OR OTHER DEALINGS IN THE
+SOFTWARE.
diff --git a/trackers/strong_sort/deep/reid/Makefile b/trackers/strong_sort/deep/reid/Makefile
new file mode 100644
index 0000000000000000000000000000000000000000..3d315a8cf5c3b9f50fcd2654961c6b63bb6ee7dc
--- /dev/null
+++ b/trackers/strong_sort/deep/reid/Makefile
@@ -0,0 +1,8 @@
+clean:
+ docker rm -f $$(docker ps -qa)
+
+build-image:
+ docker build -t=deeppreid:v0 .
+
+run:
+ nvidia-docker run -v ${PWD}:/home/appuser --name=deeppreid --net=host --ipc=host -it deeppreid:v0
diff --git a/trackers/strong_sort/deep/reid/README.rst b/trackers/strong_sort/deep/reid/README.rst
new file mode 100644
index 0000000000000000000000000000000000000000..259fda3f47a6f0443bf1e16cc50988ac63e57ecc
--- /dev/null
+++ b/trackers/strong_sort/deep/reid/README.rst
@@ -0,0 +1,322 @@
+Torchreid
+===========
+Torchreid is a library for deep-learning person re-identification, written in `PyTorch `_ and developed for our ICCV'19 project, `Omni-Scale Feature Learning for Person Re-Identification `_.
+
+It features:
+
+- multi-GPU training
+- support both image- and video-reid
+- end-to-end training and evaluation
+- incredibly easy preparation of reid datasets
+- multi-dataset training
+- cross-dataset evaluation
+- standard protocol used by most research papers
+- highly extensible (easy to add models, datasets, training methods, etc.)
+- implementations of state-of-the-art deep reid models
+- access to pretrained reid models
+- advanced training techniques
+- visualization tools (tensorboard, ranks, etc.)
+
+
+Code: https://github.com/KaiyangZhou/deep-person-reid.
+
+Documentation: https://kaiyangzhou.github.io/deep-person-reid/.
+
+How-to instructions: https://kaiyangzhou.github.io/deep-person-reid/user_guide.
+
+Model zoo: https://kaiyangzhou.github.io/deep-person-reid/MODEL_ZOO.
+
+Tech report: https://arxiv.org/abs/1910.10093.
+
+You can find some research projects that are built on top of Torchreid `here `_.
+
+
+What's new
+------------
+- [Aug 2022] We have added model export capabilities to the following frameworks: ONNX, OpenVINO and TFLite. The export script can be found `here `_
+- [Aug 2021] We have released the ImageNet-pretrained models of ``osnet_ain_x0_75``, ``osnet_ain_x0_5`` and ``osnet_ain_x0_25``. The pretraining setup follows `pycls `_.
+- [Apr 2021] We have updated the appendix in the `TPAMI version of OSNet `_ to include results in the multi-source domain generalization setting. The trained models can be found in the `Model Zoo `_.
+- [Apr 2021] We have added a script to automate the process of calculating average results over multiple splits. For more details please see ``tools/parse_test_res.py``.
+- [Apr 2021] ``v1.4.0``: We added the person search dataset, `CUHK-SYSU `_. Please see the `documentation `_ regarding how to download the dataset (it contains cropped person images).
+- [Apr 2021] All models in the model zoo have been moved to google drive. Please raise an issue if any model's performance is inconsistent with the numbers shown in the model zoo page (could be caused by wrong links).
+- [Mar 2021] `OSNet `_ will appear in the TPAMI journal! Compared with the conference version, which focuses on discriminative feature learning using the omni-scale building block, this journal extension further considers generalizable feature learning by integrating `instance normalization layers `_ with the OSNet architecture. We hope this journal paper can motivate more future work to taclke the generalization issue in cross-dataset re-ID.
+- [Mar 2021] Generalization across domains (datasets) in person re-ID is crucial in real-world applications, which is closely related to the topic of *domain generalization*. Interested in learning how the field of domain generalization has developed over the last decade? Check our recent survey in this topic at https://arxiv.org/abs/2103.02503, with coverage on the history, datasets, related problems, methodologies, potential directions, and so on (*methods designed for generalizable re-ID are also covered*!).
+- [Feb 2021] ``v1.3.6`` Added `University-1652 `_, a new dataset for multi-view multi-source geo-localization (credit to `Zhedong Zheng `_).
+- [Feb 2021] ``v1.3.5``: Now the `cython code `_ works on Windows (credit to `lablabla `_).
+- [Jan 2021] Our recent work, `MixStyle `_ (mixing instance-level feature statistics of samples of different domains for improving domain generalization), has been accepted to ICLR'21. The code has been released at https://github.com/KaiyangZhou/mixstyle-release where the person re-ID part is based on Torchreid.
+- [Jan 2021] A new evaluation metric called `mean Inverse Negative Penalty (mINP)` for person re-ID has been introduced in `Deep Learning for Person Re-identification: A Survey and Outlook (TPAMI 2021) `_. Their code can be accessed at ``_.
+- [Aug 2020] ``v1.3.3``: Fixed bug in ``visrank`` (caused by not unpacking ``dsetid``).
+- [Aug 2020] ``v1.3.2``: Added ``_junk_pids`` to ``grid`` and ``prid``. This avoids using mislabeled gallery images for training when setting ``combineall=True``.
+- [Aug 2020] ``v1.3.0``: (1) Added ``dsetid`` to the existing 3-tuple data source, resulting in ``(impath, pid, camid, dsetid)``. This variable denotes the dataset ID and is useful when combining multiple datasets for training (as a dataset indicator). E.g., when combining ``market1501`` and ``cuhk03``, the former will be assigned ``dsetid=0`` while the latter will be assigned ``dsetid=1``. (2) Added ``RandomDatasetSampler``. Analogous to ``RandomDomainSampler``, ``RandomDatasetSampler`` samples a certain number of images (``batch_size // num_datasets``) from each of specified datasets (the amount is determined by ``num_datasets``).
+- [Aug 2020] ``v1.2.6``: Added ``RandomDomainSampler`` (it samples ``num_cams`` cameras each with ``batch_size // num_cams`` images to form a mini-batch).
+- [Jun 2020] ``v1.2.5``: (1) Dataloader's output from ``__getitem__`` has been changed from ``list`` to ``dict``. Previously, an element, e.g. image tensor, was fetched with ``imgs=data[0]``. Now it should be obtained by ``imgs=data['img']``. See this `commit `_ for detailed changes. (2) Added ``k_tfm`` as an option to image data loader, which allows data augmentation to be applied ``k_tfm`` times *independently* to an image. If ``k_tfm > 1``, ``imgs=data['img']`` returns a list with ``k_tfm`` image tensors.
+- [May 2020] Added the person attribute recognition code used in `Omni-Scale Feature Learning for Person Re-Identification (ICCV'19) `_. See ``projects/attribute_recognition/``.
+- [May 2020] ``v1.2.1``: Added a simple API for feature extraction (``torchreid/utils/feature_extractor.py``). See the `documentation `_ for the instruction.
+- [Apr 2020] Code for reproducing the experiments of `deep mutual learning `_ in the `OSNet paper `__ (Supp. B) has been released at ``projects/DML``.
+- [Apr 2020] Upgraded to ``v1.2.0``. The engine class has been made more model-agnostic to improve extensibility. See `Engine `_ and `ImageSoftmaxEngine `_ for more details. Credit to `Dassl.pytorch `_.
+- [Dec 2019] Our `OSNet paper `_ has been updated, with additional experiments (in section B of the supplementary) showing some useful techniques for improving OSNet's performance in practice.
+- [Nov 2019] ``ImageDataManager`` can load training data from target datasets by setting ``load_train_targets=True``, and the train-loader can be accessed with ``train_loader_t = datamanager.train_loader_t``. This feature is useful for domain adaptation research.
+
+
+Installation
+---------------
+
+Make sure `conda `_ is installed.
+
+
+.. code-block:: bash
+
+ # cd to your preferred directory and clone this repo
+ git clone https://github.com/KaiyangZhou/deep-person-reid.git
+
+ # create environment
+ cd deep-person-reid/
+ conda create --name torchreid python=3.7
+ conda activate torchreid
+
+ # install dependencies
+ # make sure `which python` and `which pip` point to the correct path
+ pip install -r requirements.txt
+
+ # install torch and torchvision (select the proper cuda version to suit your machine)
+ conda install pytorch torchvision cudatoolkit=9.0 -c pytorch
+
+ # install torchreid (don't need to re-build it if you modify the source code)
+ python setup.py develop
+
+Another way to install is to run everything inside docker container:
+
+- build: ``make build-image``
+- run: ``make run``
+
+Get started: 30 seconds to Torchreid
+-------------------------------------
+1. Import ``torchreid``
+
+.. code-block:: python
+
+ import torchreid
+
+2. Load data manager
+
+.. code-block:: python
+
+ datamanager = torchreid.data.ImageDataManager(
+ root="reid-data",
+ sources="market1501",
+ targets="market1501",
+ height=256,
+ width=128,
+ batch_size_train=32,
+ batch_size_test=100,
+ transforms=["random_flip", "random_crop"]
+ )
+
+3 Build model, optimizer and lr_scheduler
+
+.. code-block:: python
+
+ model = torchreid.models.build_model(
+ name="resnet50",
+ num_classes=datamanager.num_train_pids,
+ loss="softmax",
+ pretrained=True
+ )
+
+ model = model.cuda()
+
+ optimizer = torchreid.optim.build_optimizer(
+ model,
+ optim="adam",
+ lr=0.0003
+ )
+
+ scheduler = torchreid.optim.build_lr_scheduler(
+ optimizer,
+ lr_scheduler="single_step",
+ stepsize=20
+ )
+
+4. Build engine
+
+.. code-block:: python
+
+ engine = torchreid.engine.ImageSoftmaxEngine(
+ datamanager,
+ model,
+ optimizer=optimizer,
+ scheduler=scheduler,
+ label_smooth=True
+ )
+
+5. Run training and test
+
+.. code-block:: python
+
+ engine.run(
+ save_dir="log/resnet50",
+ max_epoch=60,
+ eval_freq=10,
+ print_freq=10,
+ test_only=False
+ )
+
+
+A unified interface
+-----------------------
+In "deep-person-reid/scripts/", we provide a unified interface to train and test a model. See "scripts/main.py" and "scripts/default_config.py" for more details. The folder "configs/" contains some predefined configs which you can use as a starting point.
+
+Below we provide an example to train and test `OSNet (Zhou et al. ICCV'19) `_. Assume :code:`PATH_TO_DATA` is the directory containing reid datasets. The environmental variable :code:`CUDA_VISIBLE_DEVICES` is omitted, which you need to specify if you have a pool of gpus and want to use a specific set of them.
+
+Conventional setting
+^^^^^^^^^^^^^^^^^^^^^
+
+To train OSNet on Market1501, do
+
+.. code-block:: bash
+
+ python scripts/main.py \
+ --config-file configs/im_osnet_x1_0_softmax_256x128_amsgrad_cosine.yaml \
+ --transforms random_flip random_erase \
+ --root $PATH_TO_DATA
+
+
+The config file sets Market1501 as the default dataset. If you wanna use DukeMTMC-reID, do
+
+.. code-block:: bash
+
+ python scripts/main.py \
+ --config-file configs/im_osnet_x1_0_softmax_256x128_amsgrad_cosine.yaml \
+ -s dukemtmcreid \
+ -t dukemtmcreid \
+ --transforms random_flip random_erase \
+ --root $PATH_TO_DATA \
+ data.save_dir log/osnet_x1_0_dukemtmcreid_softmax_cosinelr
+
+The code will automatically (download and) load the ImageNet pretrained weights. After the training is done, the model will be saved as "log/osnet_x1_0_market1501_softmax_cosinelr/model.pth.tar-250". Under the same folder, you can find the `tensorboard `_ file. To visualize the learning curves using tensorboard, you can run :code:`tensorboard --logdir=log/osnet_x1_0_market1501_softmax_cosinelr` in the terminal and visit :code:`http://localhost:6006/` in your web browser.
+
+Evaluation is automatically performed at the end of training. To run the test again using the trained model, do
+
+.. code-block:: bash
+
+ python scripts/main.py \
+ --config-file configs/im_osnet_x1_0_softmax_256x128_amsgrad_cosine.yaml \
+ --root $PATH_TO_DATA \
+ model.load_weights log/osnet_x1_0_market1501_softmax_cosinelr/model.pth.tar-250 \
+ test.evaluate True
+
+
+Cross-domain setting
+^^^^^^^^^^^^^^^^^^^^^
+
+Suppose you wanna train OSNet on DukeMTMC-reID and test its performance on Market1501, you can do
+
+.. code-block:: bash
+
+ python scripts/main.py \
+ --config-file configs/im_osnet_x1_0_softmax_256x128_amsgrad.yaml \
+ -s dukemtmcreid \
+ -t market1501 \
+ --transforms random_flip color_jitter \
+ --root $PATH_TO_DATA
+
+Here we only test the cross-domain performance. However, if you also want to test the performance on the source dataset, i.e. DukeMTMC-reID, you can set :code:`-t dukemtmcreid market1501`, which will evaluate the model on the two datasets separately.
+
+Different from the same-domain setting, here we replace :code:`random_erase` with :code:`color_jitter`. This can improve the generalization performance on the unseen target dataset.
+
+Pretrained models are available in the `Model Zoo `_.
+
+
+Datasets
+--------
+
+Image-reid datasets
+^^^^^^^^^^^^^^^^^^^^^
+- `Market1501 `_
+- `CUHK03 `_
+- `DukeMTMC-reID `_
+- `MSMT17 `_
+- `VIPeR `_
+- `GRID `_
+- `CUHK01 `_
+- `SenseReID `_
+- `QMUL-iLIDS `_
+- `PRID `_
+
+Geo-localization datasets
+^^^^^^^^^^^^^^^^^^^^^^^^^^^
+- `University-1652 `_
+
+Video-reid datasets
+^^^^^^^^^^^^^^^^^^^^^^^
+- `MARS `_
+- `iLIDS-VID `_
+- `PRID2011 `_
+- `DukeMTMC-VideoReID `_
+
+
+Models
+-------
+
+ImageNet classification models
+^^^^^^^^^^^^^^^^^^^^^^^^^^^^^^^^
+- `ResNet `_
+- `ResNeXt `_
+- `SENet `_
+- `DenseNet `_
+- `Inception-ResNet-V2 `_
+- `Inception-V4 `_
+- `Xception `_
+- `IBN-Net `_
+
+Lightweight models
+^^^^^^^^^^^^^^^^^^^
+- `NASNet `_
+- `MobileNetV2 `_
+- `ShuffleNet `_
+- `ShuffleNetV2 `_
+- `SqueezeNet `_
+
+ReID-specific models
+^^^^^^^^^^^^^^^^^^^^^^
+- `MuDeep `_
+- `ResNet-mid `_
+- `HACNN `_
+- `PCB `_
+- `MLFN `_
+- `OSNet `_
+- `OSNet-AIN `_
+
+
+Useful links
+-------------
+- `OSNet-IBN1-Lite (test-only code with lite docker container) `_
+- `Deep Learning for Person Re-identification: A Survey and Outlook `_
+
+
+Citation
+---------
+If you use this code or the models in your research, please give credit to the following papers:
+
+.. code-block:: bash
+
+ @article{torchreid,
+ title={Torchreid: A Library for Deep Learning Person Re-Identification in Pytorch},
+ author={Zhou, Kaiyang and Xiang, Tao},
+ journal={arXiv preprint arXiv:1910.10093},
+ year={2019}
+ }
+
+ @inproceedings{zhou2019osnet,
+ title={Omni-Scale Feature Learning for Person Re-Identification},
+ author={Zhou, Kaiyang and Yang, Yongxin and Cavallaro, Andrea and Xiang, Tao},
+ booktitle={ICCV},
+ year={2019}
+ }
+
+ @article{zhou2021osnet,
+ title={Learning Generalisable Omni-Scale Representations for Person Re-Identification},
+ author={Zhou, Kaiyang and Yang, Yongxin and Cavallaro, Andrea and Xiang, Tao},
+ journal={TPAMI},
+ year={2021}
+ }
diff --git a/trackers/strong_sort/deep/reid/configs/im_osnet_ain_x1_0_softmax_256x128_amsgrad_cosine.yaml b/trackers/strong_sort/deep/reid/configs/im_osnet_ain_x1_0_softmax_256x128_amsgrad_cosine.yaml
new file mode 100644
index 0000000000000000000000000000000000000000..c8b63ec3ea11798edf50d3a052b87f0b5494a645
--- /dev/null
+++ b/trackers/strong_sort/deep/reid/configs/im_osnet_ain_x1_0_softmax_256x128_amsgrad_cosine.yaml
@@ -0,0 +1,35 @@
+model:
+ name: 'osnet_ain_x1_0'
+ pretrained: True
+
+data:
+ type: 'image'
+ sources: ['market1501']
+ targets: ['market1501', 'dukemtmcreid']
+ height: 256
+ width: 128
+ combineall: False
+ transforms: ['random_flip', 'color_jitter']
+ save_dir: 'log/osnet_ain_x1_0_market1501_softmax_cosinelr'
+
+loss:
+ name: 'softmax'
+ softmax:
+ label_smooth: True
+
+train:
+ optim: 'amsgrad'
+ lr: 0.0015
+ max_epoch: 100
+ batch_size: 64
+ fixbase_epoch: 10
+ open_layers: ['classifier']
+ lr_scheduler: 'cosine'
+
+test:
+ batch_size: 300
+ dist_metric: 'cosine'
+ normalize_feature: False
+ evaluate: False
+ eval_freq: -1
+ rerank: False
\ No newline at end of file
diff --git a/trackers/strong_sort/deep/reid/configs/im_osnet_ibn_x1_0_softmax_256x128_amsgrad.yaml b/trackers/strong_sort/deep/reid/configs/im_osnet_ibn_x1_0_softmax_256x128_amsgrad.yaml
new file mode 100644
index 0000000000000000000000000000000000000000..b4f89033d1337f8ab173d920bd5f3357981e350f
--- /dev/null
+++ b/trackers/strong_sort/deep/reid/configs/im_osnet_ibn_x1_0_softmax_256x128_amsgrad.yaml
@@ -0,0 +1,36 @@
+model:
+ name: 'osnet_ibn_x1_0'
+ pretrained: True
+
+data:
+ type: 'image'
+ sources: ['market1501']
+ targets: ['dukemtmcreid']
+ height: 256
+ width: 128
+ combineall: False
+ transforms: ['random_flip', 'color_jitter']
+ save_dir: 'log/osnet_ibn_x1_0_market2duke_softmax'
+
+loss:
+ name: 'softmax'
+ softmax:
+ label_smooth: True
+
+train:
+ optim: 'amsgrad'
+ lr: 0.0015
+ max_epoch: 150
+ batch_size: 64
+ fixbase_epoch: 10
+ open_layers: ['classifier']
+ lr_scheduler: 'single_step'
+ stepsize: [60]
+
+test:
+ batch_size: 300
+ dist_metric: 'euclidean'
+ normalize_feature: False
+ evaluate: False
+ eval_freq: -1
+ rerank: False
\ No newline at end of file
diff --git a/trackers/strong_sort/deep/reid/configs/im_osnet_x0_25_softmax_256x128_amsgrad.yaml b/trackers/strong_sort/deep/reid/configs/im_osnet_x0_25_softmax_256x128_amsgrad.yaml
new file mode 100644
index 0000000000000000000000000000000000000000..d256a6b27399a6aca15ce264ae8f6853afca37c5
--- /dev/null
+++ b/trackers/strong_sort/deep/reid/configs/im_osnet_x0_25_softmax_256x128_amsgrad.yaml
@@ -0,0 +1,36 @@
+model:
+ name: 'osnet_x0_25'
+ pretrained: True
+
+data:
+ type: 'image'
+ sources: ['market1501']
+ targets: ['market1501']
+ height: 256
+ width: 128
+ combineall: False
+ transforms: ['random_flip']
+ save_dir: 'log/osnet_x0_25_market1501_softmax'
+
+loss:
+ name: 'softmax'
+ softmax:
+ label_smooth: True
+
+train:
+ optim: 'amsgrad'
+ lr: 0.003
+ max_epoch: 180
+ batch_size: 128
+ fixbase_epoch: 10
+ open_layers: ['classifier']
+ lr_scheduler: 'single_step'
+ stepsize: [80]
+
+test:
+ batch_size: 300
+ dist_metric: 'euclidean'
+ normalize_feature: False
+ evaluate: False
+ eval_freq: -1
+ rerank: False
\ No newline at end of file
diff --git a/trackers/strong_sort/deep/reid/configs/im_osnet_x0_5_softmax_256x128_amsgrad.yaml b/trackers/strong_sort/deep/reid/configs/im_osnet_x0_5_softmax_256x128_amsgrad.yaml
new file mode 100644
index 0000000000000000000000000000000000000000..467305f8ba7f25d4794732ccc4ebd53de9f9927d
--- /dev/null
+++ b/trackers/strong_sort/deep/reid/configs/im_osnet_x0_5_softmax_256x128_amsgrad.yaml
@@ -0,0 +1,36 @@
+model:
+ name: 'osnet_x0_5'
+ pretrained: True
+
+data:
+ type: 'image'
+ sources: ['market1501']
+ targets: ['market1501']
+ height: 256
+ width: 128
+ combineall: False
+ transforms: ['random_flip']
+ save_dir: 'log/osnet_x0_5_market1501_softmax'
+
+loss:
+ name: 'softmax'
+ softmax:
+ label_smooth: True
+
+train:
+ optim: 'amsgrad'
+ lr: 0.003
+ max_epoch: 180
+ batch_size: 128
+ fixbase_epoch: 10
+ open_layers: ['classifier']
+ lr_scheduler: 'single_step'
+ stepsize: [80]
+
+test:
+ batch_size: 300
+ dist_metric: 'euclidean'
+ normalize_feature: False
+ evaluate: False
+ eval_freq: -1
+ rerank: False
\ No newline at end of file
diff --git a/trackers/strong_sort/deep/reid/configs/im_osnet_x0_75_softmax_256x128_amsgrad.yaml b/trackers/strong_sort/deep/reid/configs/im_osnet_x0_75_softmax_256x128_amsgrad.yaml
new file mode 100644
index 0000000000000000000000000000000000000000..04203fd0fbe7f5c4185d7d20ec251dcf1ba6398b
--- /dev/null
+++ b/trackers/strong_sort/deep/reid/configs/im_osnet_x0_75_softmax_256x128_amsgrad.yaml
@@ -0,0 +1,36 @@
+model:
+ name: 'osnet_x0_75'
+ pretrained: True
+
+data:
+ type: 'image'
+ sources: ['market1501']
+ targets: ['market1501']
+ height: 256
+ width: 128
+ combineall: False
+ transforms: ['random_flip']
+ save_dir: 'log/osnet_x0_75_market1501_softmax'
+
+loss:
+ name: 'softmax'
+ softmax:
+ label_smooth: True
+
+train:
+ optim: 'amsgrad'
+ lr: 0.0015
+ max_epoch: 150
+ batch_size: 64
+ fixbase_epoch: 10
+ open_layers: ['classifier']
+ lr_scheduler: 'single_step'
+ stepsize: [60]
+
+test:
+ batch_size: 300
+ dist_metric: 'euclidean'
+ normalize_feature: False
+ evaluate: False
+ eval_freq: -1
+ rerank: False
\ No newline at end of file
diff --git a/trackers/strong_sort/deep/reid/configs/im_osnet_x1_0_softmax_256x128_amsgrad.yaml b/trackers/strong_sort/deep/reid/configs/im_osnet_x1_0_softmax_256x128_amsgrad.yaml
new file mode 100644
index 0000000000000000000000000000000000000000..f1c970fed20c7aae81b7c5424caa94bbc7bf939e
--- /dev/null
+++ b/trackers/strong_sort/deep/reid/configs/im_osnet_x1_0_softmax_256x128_amsgrad.yaml
@@ -0,0 +1,36 @@
+model:
+ name: 'osnet_x1_0'
+ pretrained: True
+
+data:
+ type: 'image'
+ sources: ['market1501']
+ targets: ['market1501']
+ height: 256
+ width: 128
+ combineall: False
+ transforms: ['random_flip']
+ save_dir: 'log/osnet_x1_0_market1501_softmax'
+
+loss:
+ name: 'softmax'
+ softmax:
+ label_smooth: True
+
+train:
+ optim: 'amsgrad'
+ lr: 0.0015
+ max_epoch: 150
+ batch_size: 64
+ fixbase_epoch: 10
+ open_layers: ['classifier']
+ lr_scheduler: 'single_step'
+ stepsize: [60]
+
+test:
+ batch_size: 300
+ dist_metric: 'euclidean'
+ normalize_feature: False
+ evaluate: False
+ eval_freq: -1
+ rerank: False
\ No newline at end of file
diff --git a/trackers/strong_sort/deep/reid/configs/im_osnet_x1_0_softmax_256x128_amsgrad_cosine.yaml b/trackers/strong_sort/deep/reid/configs/im_osnet_x1_0_softmax_256x128_amsgrad_cosine.yaml
new file mode 100644
index 0000000000000000000000000000000000000000..29750bc2e3cce5747ab703905f74fcbed74b5a57
--- /dev/null
+++ b/trackers/strong_sort/deep/reid/configs/im_osnet_x1_0_softmax_256x128_amsgrad_cosine.yaml
@@ -0,0 +1,35 @@
+model:
+ name: 'osnet_x1_0'
+ pretrained: True
+
+data:
+ type: 'image'
+ sources: ['market1501']
+ targets: ['market1501']
+ height: 256
+ width: 128
+ combineall: False
+ transforms: ['random_flip']
+ save_dir: 'log/osnet_x1_0_market1501_softmax_cosinelr'
+
+loss:
+ name: 'softmax'
+ softmax:
+ label_smooth: True
+
+train:
+ optim: 'amsgrad'
+ lr: 0.0015
+ max_epoch: 250
+ batch_size: 64
+ fixbase_epoch: 10
+ open_layers: ['classifier']
+ lr_scheduler: 'cosine'
+
+test:
+ batch_size: 300
+ dist_metric: 'euclidean'
+ normalize_feature: False
+ evaluate: False
+ eval_freq: -1
+ rerank: False
\ No newline at end of file
diff --git a/trackers/strong_sort/deep/reid/configs/im_r50_softmax_256x128_amsgrad.yaml b/trackers/strong_sort/deep/reid/configs/im_r50_softmax_256x128_amsgrad.yaml
new file mode 100644
index 0000000000000000000000000000000000000000..61735898ac1f2e72220f739445a186f787dfe568
--- /dev/null
+++ b/trackers/strong_sort/deep/reid/configs/im_r50_softmax_256x128_amsgrad.yaml
@@ -0,0 +1,36 @@
+model:
+ name: 'resnet50_fc512'
+ pretrained: True
+
+data:
+ type: 'image'
+ sources: ['market1501']
+ targets: ['market1501']
+ height: 256
+ width: 128
+ combineall: False
+ transforms: ['random_flip']
+ save_dir: 'log/resnet50_market1501_softmax'
+
+loss:
+ name: 'softmax'
+ softmax:
+ label_smooth: True
+
+train:
+ optim: 'amsgrad'
+ lr: 0.0003
+ max_epoch: 60
+ batch_size: 32
+ fixbase_epoch: 5
+ open_layers: ['classifier']
+ lr_scheduler: 'single_step'
+ stepsize: [20]
+
+test:
+ batch_size: 100
+ dist_metric: 'euclidean'
+ normalize_feature: False
+ evaluate: False
+ eval_freq: -1
+ rerank: False
\ No newline at end of file
diff --git a/trackers/strong_sort/deep/reid/configs/im_r50fc512_softmax_256x128_amsgrad.yaml b/trackers/strong_sort/deep/reid/configs/im_r50fc512_softmax_256x128_amsgrad.yaml
new file mode 100644
index 0000000000000000000000000000000000000000..5ec2b705db03439220fb257d7e54195844b8cdef
--- /dev/null
+++ b/trackers/strong_sort/deep/reid/configs/im_r50fc512_softmax_256x128_amsgrad.yaml
@@ -0,0 +1,36 @@
+model:
+ name: 'resnet50_fc512'
+ pretrained: True
+
+data:
+ type: 'image'
+ sources: ['market1501']
+ targets: ['market1501']
+ height: 256
+ width: 128
+ combineall: False
+ transforms: ['random_flip']
+ save_dir: 'log/resnet50_fc512_market1501_softmax'
+
+loss:
+ name: 'softmax'
+ softmax:
+ label_smooth: True
+
+train:
+ optim: 'amsgrad'
+ lr: 0.0003
+ max_epoch: 60
+ batch_size: 32
+ fixbase_epoch: 5
+ open_layers: ['fc', 'classifier']
+ lr_scheduler: 'single_step'
+ stepsize: [20]
+
+test:
+ batch_size: 100
+ dist_metric: 'euclidean'
+ normalize_feature: False
+ evaluate: False
+ eval_freq: -1
+ rerank: False
\ No newline at end of file
diff --git a/trackers/strong_sort/deep/reid/docs/AWESOME_REID.md b/trackers/strong_sort/deep/reid/docs/AWESOME_REID.md
new file mode 100644
index 0000000000000000000000000000000000000000..31215356af8f15f40263542167b1ced97f4f402e
--- /dev/null
+++ b/trackers/strong_sort/deep/reid/docs/AWESOME_REID.md
@@ -0,0 +1,69 @@
+# Awesome-ReID
+Here is a collection of ReID-related research with links to papers and code. You are welcome to submit [PR](https://help.github.com/articles/creating-a-pull-request/)s if you find something missing.
+
+
+- [TPAMI21] Learning Generalisable Omni-Scale Representations for Person Re-Identification [[paper](https://arxiv.org/abs/1910.06827)][[code](https://github.com/KaiyangZhou/deep-person-reid)]
+
+- [TPAMI21] Deep Learning for Person Re-identification: A Survey and Outlook [[paper](https://arxiv.org/abs/2001.04193)] [[code](https://github.com/mangye16/ReID-Survey)]
+
+- [ICCV19] RGB-Infrared Cross-Modality Person Re-Identification via Joint Pixel and Feature Alignment. [[paper](http://openaccess.thecvf.com/content_ICCV_2019/papers/Wang_RGB-Infrared_Cross-Modality_Person_Re-Identification_via_Joint_Pixel_and_Feature_Alignment_ICCV_2019_paper.pdf)] [[code](https://github.com/wangguanan/AlignGAN)]
+
+- [ICCV19] Unsupervised Graph Association for Person Re-identification. [[paper](https://github.com/yichuan9527/Unsupervised-Graph-Association-for-Person-Re-identification)] [[code](https://github.com/yichuan9527/Unsupervised-Graph-Association-for-Person-Re-identification)]
+
+- [ICCV19] Self-similarity Grouping: A Simple Unsupervised Cross Domain Adaptation Approach for Person Re-identification. [[paper](http://openaccess.thecvf.com/content_ICCV_2019/papers/Fu_Self-Similarity_Grouping_A_Simple_Unsupervised_Cross_Domain_Adaptation_Approach_for_ICCV_2019_paper.pdf)] [[code](https://github.com/OasisYang/SSG)]
+
+- [ICCV19] Spectral Feature Transformation for Person Re-Identification. [[paper](http://openaccess.thecvf.com/content_ICCV_2019/papers/Luo_Spectral_Feature_Transformation_for_Person_Re-Identification_ICCV_2019_paper.pdf)] [[code](https://github.com/LuckyDC/SFT_REID)]
+
+- [ICCV19] Beyond Human Parts: Dual Part-Aligned Representations for Person Re-Identification. [[paper](http://openaccess.thecvf.com/content_ICCV_2019/papers/Guo_Beyond_Human_Parts_Dual_Part-Aligned_Representations_for_Person_Re-Identification_ICCV_2019_paper.pdf)] [[code](https://github.com/ggjy/P2Net.pytorch)]
+
+- [ICCV19] Co-segmentation Inspired Attention Networks for Video-based Person Re-identification. [[paper](http://openaccess.thecvf.com/content_ICCV_2019/papers/Subramaniam_Co-Segmentation_Inspired_Attention_Networks_for_Video-Based_Person_Re-Identification_ICCV_2019_paper.pdf)][[code](https://github.com/InnovArul/vidreid_cosegmentation)]
+
+- [ICCV19] Mixed High-Order Attention Network for Person Re-Identification. [[paper](https://arxiv.org/abs/1908.05819)][[code](https://github.com/chenbinghui1/MHN)]
+
+- [ICCV19] ABD-Net: Attentive but Diverse Person Re-Identification. [[paper](https://arxiv.org/abs/1908.01114)] [[code](https://github.com/TAMU-VITA/ABD-Net)]
+
+- [ICCV19] Omni-Scale Feature Learning for Person Re-Identification. [[paper](https://arxiv.org/abs/1905.00953)] [[code](https://github.com/KaiyangZhou/deep-person-reid)]
+
+- [CVPR19] Joint Discriminative and Generative Learning for Person Re-identification. [[paper](https://arxiv.org/abs/1904.07223)][[code](https://github.com/NVlabs/DG-Net)]
+- [CVPR19] Invariance Matters: Exemplar Memory for Domain Adaptive Person Re-identification. [[paper](https://arxiv.org/abs/1904.01990)][[code](https://github.com/zhunzhong07/ECN)]
+- [CVPR19] Dissecting Person Re-identification from the Viewpoint of Viewpoint. [[paper](https://arxiv.org/abs/1812.02162)][[code](https://github.com/sxzrt/Dissecting-Person-Re-ID-from-the-Viewpoint-of-Viewpoint)]
+- [CVPR19] Unsupervised Person Re-identification by Soft Multilabel Learning. [[paper](https://arxiv.org/abs/1903.06325)][[code](https://github.com/KovenYu/MAR)]
+- [CVPR19] Patch-based Discriminative Feature Learning for Unsupervised Person Re-identification. [[paper](https://kovenyu.com/publication/2019-cvpr-pedal/)][[code](https://github.com/QizeYang/PAUL)]
+
+- [AAAI19] Spatial and Temporal Mutual Promotion for Video-based Person Re-identification. [[paper](https://arxiv.org/abs/1812.10305)][[code](https://github.com/yolomax/person-reid-lib)]
+
+- [AAAI19] Spatial-Temporal Person Re-identification. [[paper](https://arxiv.org/abs/1812.03282)][[code](https://github.com/Wanggcong/Spatial-Temporal-Re-identification)]
+
+- [AAAI19] Horizontal Pyramid Matching for Person Re-identification. [[paper](https://arxiv.org/abs/1804.05275)][[code](https://github.com/OasisYang/HPM)]
+
+- [AAAI19] Backbone Can Not be Trained at Once: Rolling Back to Pre-trained Network for Person Re-identification. [[paper](https://arxiv.org/abs/1901.06140)][[code](https://github.com/youngminPIL/rollback)]
+
+- [AAAI19] A Bottom-Up Clustering Approach to Unsupervised Person Re-identification. [[paper](https://vana77.github.io/vana77.github.io/images/AAAI19.pdf)][[code](https://github.com/vana77/Bottom-up-Clustering-Person-Re-identification)]
+
+- [NIPS18] FD-GAN: Pose-guided Feature Distilling GAN for Robust Person Re-identification. [[paper](https://arxiv.org/abs/1810.02936)][[code](https://github.com/yxgeee/FD-GAN)]
+
+- [ECCV18] Generalizing A Person Retrieval Model Hetero- and Homogeneously. [[paper](http://openaccess.thecvf.com/content_ECCV_2018/papers/Zhun_Zhong_Generalizing_A_Person_ECCV_2018_paper.pdf)][[code](https://github.com/zhunzhong07/HHL)]
+
+- [ECCV18] Pose-Normalized Image Generation for Person Re-identification. [[paper](https://arxiv.org/abs/1712.02225)][[code](https://github.com/naiq/PN_GAN)]
+
+- [CVPR18] Camera Style Adaptation for Person Re-Identification. [[paper](https://arxiv.org/abs/1711.10295)][[code](https://github.com/zhunzhong07/CamStyle)]
+
+- [CVPR18] Deep Group-Shuffling Random Walk for Person Re-Identification. [[paper](https://arxiv.org/abs/1807.11178)][[code](https://github.com/YantaoShen/kpm_rw_person_reid)]
+
+- [CVPR18] End-to-End Deep Kronecker-Product Matching for Person Re-identification. [[paper](https://arxiv.org/abs/1807.11182)][[code](https://github.com/YantaoShen/kpm_rw_person_reid)]
+
+- [CVPR18] Features for Multi-Target Multi-Camera Tracking and Re-Identification. [[paper](https://arxiv.org/abs/1803.10859)][[code](https://github.com/ergysr/DeepCC)]
+
+- [CVPR18] Group Consistent Similarity Learning via Deep CRF for Person Re-Identification. [[paper](http://openaccess.thecvf.com/content_cvpr_2018/papers/Chen_Group_Consistent_Similarity_CVPR_2018_paper.pdf)][[code](https://github.com/dapengchen123/crf_affinity)]
+
+- [CVPR18] Harmonious Attention Network for Person Re-Identification. [[paper](https://arxiv.org/abs/1802.08122)][[code](https://github.com/KaiyangZhou/deep-person-reid)]
+
+- [CVPR18] Human Semantic Parsing for Person Re-Identification. [[paper](https://arxiv.org/abs/1804.00216)][[code](https://github.com/emrahbasaran/SPReID)]
+
+- [CVPR18] Multi-Level Factorisation Net for Person Re-Identification. [[paper](https://arxiv.org/abs/1803.09132)][[code](https://github.com/KaiyangZhou/deep-person-reid)]
+
+- [CVPR18] Resource Aware Person Re-identification across Multiple Resolutions. [[paper](https://arxiv.org/abs/1805.08805)][[code](https://github.com/mileyan/DARENet)]
+
+- [CVPR18] Exploit the Unknown Gradually: One-Shot Video-Based Person Re-Identification by Stepwise Learning. [[paper](https://yu-wu.net/pdf/CVPR2018_Exploit-Unknown-Gradually.pdf)][[code](https://github.com/Yu-Wu/Exploit-Unknown-Gradually)]
+
+- [ArXiv18] Revisiting Temporal Modeling for Video-based Person ReID. [[paper](https://arxiv.org/abs/1805.02104)][[code](https://github.com/jiyanggao/Video-Person-ReID)]
diff --git a/trackers/strong_sort/deep/reid/docs/MODEL_ZOO.md b/trackers/strong_sort/deep/reid/docs/MODEL_ZOO.md
new file mode 100644
index 0000000000000000000000000000000000000000..8a9306fe0dccf6259add21bf1de1bdfbd5deeb7f
--- /dev/null
+++ b/trackers/strong_sort/deep/reid/docs/MODEL_ZOO.md
@@ -0,0 +1,93 @@
+# Model Zoo
+
+- Results are presented in the format of **.
+- When computing model size and FLOPs, only layers that are used at test time are considered (see `torchreid.utils.compute_model_complexity`).
+- Asterisk (\*) means the model is trained from scratch.
+- `combineall=True` means all images in the dataset are used for model training.
+- Why not use heavy data augmentation like [random erasing](https://arxiv.org/abs/1708.04896) for model training? It's because heavy data augmentation might harm the cross-dataset generalization performance (see [this paper](https://arxiv.org/abs/1708.04896)).
+
+
+## ImageNet pretrained models
+
+
+| Model | Download |
+| :--- | :---: |
+| shufflenet | [model](https://drive.google.com/file/d/1RFnYcHK1TM-yt3yLsNecaKCoFO4Yb6a-/view?usp=sharing) |
+| mobilenetv2_x1_0 | [model](https://drive.google.com/file/d/1K7_CZE_L_Tf-BRY6_vVm0G-0ZKjVWh3R/view?usp=sharing) |
+| mobilenetv2_x1_4 | [model](https://drive.google.com/file/d/10c0ToIGIVI0QZTx284nJe8QfSJl5bIta/view?usp=sharing) |
+| mlfn | [model](https://drive.google.com/file/d/1PP8Eygct5OF4YItYRfA3qypYY9xiqHuV/view?usp=sharing) |
+| osnet_x1_0 | [model](https://drive.google.com/file/d/1LaG1EJpHrxdAxKnSCJ_i0u-nbxSAeiFY/view?usp=sharing) |
+| osnet_x0_75 | [model](https://drive.google.com/file/d/1uwA9fElHOk3ZogwbeY5GkLI6QPTX70Hq/view?usp=sharing) |
+| osnet_x0_5 | [model](https://drive.google.com/file/d/16DGLbZukvVYgINws8u8deSaOqjybZ83i/view?usp=sharing) |
+| osnet_x0_25 | [model](https://drive.google.com/file/d/1rb8UN5ZzPKRc_xvtHlyDh-cSz88YX9hs/view?usp=sharing) |
+| osnet_ibn_x1_0 | [model](https://drive.google.com/file/d/1sr90V6irlYYDd4_4ISU2iruoRG8J__6l/view?usp=sharing) |
+| osnet_ain_x1_0 | [model](https://drive.google.com/file/d/1-CaioD9NaqbHK_kzSMW8VE4_3KcsRjEo/view?usp=sharing) |
+| osnet_ain_x0_75 | [model](https://drive.google.com/file/d/1apy0hpsMypqstfencdH-jKIUEFOW4xoM/view?usp=sharing) |
+| osnet_ain_x0_5 | [model](https://drive.google.com/file/d/1KusKvEYyKGDTUBVRxRiz55G31wkihB6l/view?usp=sharing) |
+| osnet_ain_x0_25 | [model](https://drive.google.com/file/d/1SxQt2AvmEcgWNhaRb2xC4rP6ZwVDP0Wt/view?usp=sharing) |
+
+
+## Same-domain ReID
+
+
+| Model | # Param (10^6) | GFLOPs | Loss | Input | Transforms | Distance | market1501 | dukemtmcreid | msmt17 |
+| :--- | :---: | :---: | :---: | :---: | :---: | :---: | :---: | :---: | :---: |
+| resnet50 | 23.5 | 2.7 | softmax | (256, 128) | `random_flip`, `random_crop` | `euclidean` | [87.9 (70.4)](https://drive.google.com/file/d/1dUUZ4rHDWohmsQXCRe2C_HbYkzz94iBV/view?usp=sharing) | [78.3 (58.9)](https://drive.google.com/file/d/17ymnLglnc64NRvGOitY3BqMRS9UWd1wg/view?usp=sharing) | [63.2 (33.9)](https://drive.google.com/file/d/1ep7RypVDOthCRIAqDnn4_N-UhkkFHJsj/view?usp=sharing) |
+| resnet50_fc512 | 24.6 | 4.1 | softmax | (256, 128) | `random_flip`, `random_crop` | `euclidean` | [90.8 (75.3)](https://drive.google.com/file/d/1kv8l5laX_YCdIGVCetjlNdzKIA3NvsSt/view?usp=sharing) | [81.0 (64.0)](https://drive.google.com/file/d/13QN8Mp3XH81GK4BPGXobKHKyTGH50Rtx/view?usp=sharing) | [69.6 (38.4)](https://drive.google.com/file/d/1fDJLcz4O5wxNSUvImIIjoaIF9u1Rwaud/view?usp=sharing) |
+| mlfn | 32.5 | 2.8 | softmax | (256, 128) | `random_flip`, `random_crop` | `euclidean` | [90.1 (74.3)](https://drive.google.com/file/d/1wXcvhA_b1kpDfrt9s2Pma-MHxtj9pmvS/view?usp=sharing) | [81.1 (63.2)](https://drive.google.com/file/d/1rExgrTNb0VCIcOnXfMsbwSUW1h2L1Bum/view?usp=sharing) | [66.4 (37.2)](https://drive.google.com/file/d/18JzsZlJb3Wm7irCbZbZ07TN4IFKvR6p-/view?usp=sharing) |
+| hacnn* | 4.5 | 0.5 | softmax | (160, 64) | `random_flip`, `random_crop` | `euclidean` | [90.9 (75.6)](https://drive.google.com/file/d/1LRKIQduThwGxMDQMiVkTScBwR7WidmYF/view?usp=sharing) | [80.1 (63.2)](https://drive.google.com/file/d/1zNm6tP4ozFUCUQ7Sv1Z98EAJWXJEhtYH/view?usp=sharing) | [64.7 (37.2)](https://drive.google.com/file/d/1MsKRtPM5WJ3_Tk2xC0aGOO7pM3VaFDNZ/view?usp=sharing) |
+| mobilenetv2_x1_0 | 2.2 | 0.2 | softmax | (256, 128) | `random_flip`, `random_crop` | `euclidean` | [85.6 (67.3)](https://drive.google.com/file/d/18DgHC2ZJkjekVoqBWszD8_Xiikz-fewp/view?usp=sharing) | [74.2 (54.7)](https://drive.google.com/file/d/1q1WU2FETRJ3BXcpVtfJUuqq4z3psetds/view?usp=sharing) | [57.4 (29.3)](https://drive.google.com/file/d/1j50Hv14NOUAg7ZeB3frzfX-WYLi7SrhZ/view?usp=sharing) |
+| mobilenetv2_x1_4 | 4.3 | 0.4 | softmax | (256, 128) | `random_flip`, `random_crop` | `euclidean` | [87.0 (68.5)](https://drive.google.com/file/d/1t6JCqphJG-fwwPVkRLmGGyEBhGOf2GO5/view?usp=sharing) | [76.2 (55.8)](https://drive.google.com/file/d/12uD5FeVqLg9-AFDju2L7SQxjmPb4zpBN/view?usp=sharing) | [60.1 (31.5)](https://drive.google.com/file/d/1ZY5P2Zgm-3RbDpbXM0kIBMPvspeNIbXz/view?usp=sharing) |
+| osnet_x1_0 | 2.2 | 0.98 | softmax | (256, 128) | `random_flip` | `euclidean` | [94.2 (82.6)](https://drive.google.com/file/d/1vduhq5DpN2q1g4fYEZfPI17MJeh9qyrA/view?usp=sharing) | [87.0 (70.2)](https://drive.google.com/file/d/1QZO_4sNf4hdOKKKzKc-TZU9WW1v6zQbq/view?usp=sharing) | [74.9 (43.8)](https://drive.google.com/file/d/112EMUfBPYeYg70w-syK6V6Mx8-Qb9Q1M/view?usp=sharing) |
+| osnet_x0_75 | 1.3 | 0.57 | softmax | (256, 128) | `random_flip` | `euclidean` | [93.7 (81.2)](https://drive.google.com/file/d/1ozRaDSQw_EQ8_93OUmjDbvLXw9TnfPer/view?usp=sharing) | [85.8 (69.8)](https://drive.google.com/file/d/1IE3KRaTPp4OUa6PGTFL_d5_KQSJbP0Or/view?usp=sharing) | [72.8 (41.4)](https://drive.google.com/file/d/1QEGO6WnJ-BmUzVPd3q9NoaO_GsPNlmWc/view?usp=sharing) |
+| osnet_x0_5 | 0.6 | 0.27 | softmax | (256, 128) | `random_flip` | `euclidean` | [92.5 (79.8)](https://drive.google.com/file/d/1PLB9rgqrUM7blWrg4QlprCuPT7ILYGKT/view?usp=sharing) | [85.1 (67.4)](https://drive.google.com/file/d/1KoUVqmiST175hnkALg9XuTi1oYpqcyTu/view?usp=sharing) | [69.7 (37.5)](https://drive.google.com/file/d/1UT3AxIaDvS2PdxzZmbkLmjtiqq7AIKCv/view?usp=sharing) |
+| osnet_x0_25 | 0.2 | 0.08 | softmax | (256, 128) | `random_flip` | `euclidean` | [91.2 (75.0)](https://drive.google.com/file/d/1z1UghYvOTtjx7kEoRfmqSMu-z62J6MAj/view?usp=sharing) | [82.0 (61.4)](https://drive.google.com/file/d/1eumrtiXT4NOspjyEV4j8cHmlOaaCGk5l/view?usp=sharing) | [61.4 (29.5)](https://drive.google.com/file/d/1sSwXSUlj4_tHZequ_iZ8w_Jh0VaRQMqF/view?usp=sharing) |
+
+
+## Cross-domain ReID
+
+#### Market1501 -> DukeMTMC-reID
+
+
+| Model | # Param (10^6) | GFLOPs | Loss | Input | Transforms | Distance | Rank-1 | Rank-5 | Rank-10 | mAP | Download |
+| :--- | :---: | :---: | :---: | :---: | :---: | :---: | :---: | :---: | :---: | :---: | :---: |
+| osnet_ibn_x1_0 | 2.2 | 0.98 | softmax | (256, 128) | `random_flip`, `color_jitter` | `euclidean` | 48.5 | 62.3 | 67.4 | 26.7 | [model](https://drive.google.com/file/d/1uWW7_z_IcUmRNPqQOrEBdsvic94fWH37/view?usp=sharing) |
+| osnet_ain_x1_0 | 2.2 | 0.98 | softmax | (256, 128) | `random_flip`, `color_jitter` | `cosine` | 52.4 | 66.1 | 71.2 | 30.5 | [model](https://drive.google.com/file/d/14bNFGm0FhwHEkEpYKqKiDWjLNhXywFAd/view?usp=sharing) |
+
+
+#### DukeMTMC-reID -> Market1501
+
+
+| Model | # Param (10^6) | GFLOPs | Loss | Input | Transforms | Distance | Rank-1 | Rank-5 | Rank-10 | mAP | Download |
+| :--- | :---: | :---: | :---: | :---: | :---: | :---: | :---: | :---: | :---: | :---: | :---: |
+| osnet_ibn_x1_0 | 2.2 | 0.98 | softmax | (256, 128) | `random_flip`, `color_jitter` | `euclidean` | 57.7 | 73.7 | 80.0 | 26.1 | [model](https://drive.google.com/file/d/1CNxL1IP0BjcE1TSttiVOID1VNipAjiF3/view?usp=sharing) |
+| osnet_ain_x1_0 | 2.2 | 0.98 | softmax | (256, 128) | `random_flip`, `color_jitter` | `cosine` | 61.0 | 77.0 | 82.5 | 30.6 | [model](https://drive.google.com/file/d/1hypJvq8G04SOby6jvF337GEkg5K_bmCw/view?usp=sharing) |
+
+
+#### MSMT17 (`combineall=True`) -> Market1501 & DukeMTMC-reID
+
+
+| Model | # Param (10^6) | GFLOPs | Loss | Input | Transforms | Distance | msmt17 -> market1501 | msmt17 -> dukemtmcreid | Download |
+| :--- | :---: | :---: | :---: | :---: | :---: | :---: | :---: | :---: | :---: |
+| resnet50 | 23.5 | 2.7 | softmax | (256, 128) | `random_flip`, `color_jitter` | `euclidean` | 46.3 (22.8) | 52.3 (32.1) | [model](https://drive.google.com/file/d/1yiBteqgIZoOeywE8AhGmEQl7FTVwrQmf/view?usp=sharing) |
+| osnet_x1_0 | 2.2 | 0.98 | softmax | (256, 128) | `random_flip`, `color_jitter` | `euclidean` | 66.6 (37.5) | 66.0 (45.3) | [model](https://drive.google.com/file/d/1IosIFlLiulGIjwW3H8uMRmx3MzPwf86x/view?usp=sharing) |
+| osnet_x0_75 | 1.3 | 0.57 | softmax | (256, 128) | `random_flip`, `color_jitter` | `euclidean` | 63.6 (35.5) | 65.3 (44.5) | [model](https://drive.google.com/file/d/1fhjSS_7SUGCioIf2SWXaRGPqIY9j7-uw/view?usp=sharing) |
+| osnet_x0_5 | 0.6 | 0.27 | softmax | (256, 128) | `random_flip`, `color_jitter` | `euclidean` | 64.3 (34.9) | 65.2 (43.3) | [model](https://drive.google.com/file/d/1DHgmb6XV4fwG3n-CnCM0zdL9nMsZ9_RF/view?usp=sharing) |
+| osnet_x0_25 | 0.2 | 0.08 | softmax | (256, 128) | `random_flip`, `color_jitter` | `euclidean` | 59.9 (31.0) | 61.5 (39.6) | [model](https://drive.google.com/file/d/1Kkx2zW89jq_NETu4u42CFZTMVD5Hwm6e/view?usp=sharing) |
+| osnet_ibn_x1_0 | 2.2 | 0.98 | softmax | (256, 128) | `random_flip`, `color_jitter` | `euclidean` | 66.5 (37.2) | 67.4 (45.6) | [model](https://drive.google.com/file/d/1q3Sj2ii34NlfxA4LvmHdWO_75NDRmECJ/view?usp=sharing) |
+| osnet_ain_x1_0 | 2.2 | 0.98 | softmax | (256, 128) | `random_flip`, `color_jitter` | `cosine` | 70.1 (43.3) | 71.1 (52.7) | [model](https://drive.google.com/file/d/1SigwBE6mPdqiJMqhuIY4aqC7--5CsMal/view?usp=sharing) |
+
+
+#### Multi-source domain generalization
+
+The models below are trained using multiple source datasets, as described in [Zhou et al. TPAMI'21](https://arxiv.org/abs/1910.06827).
+
+Regarding the abbreviations, MS is MSMT17; M is Market1501; D is DukeMTMC-reID; and C is CUHK03.
+
+All models were trained with [im_osnet_ain_x1_0_softmax_256x128_amsgrad_cosine.yaml](https://github.com/KaiyangZhou/deep-person-reid/blob/master/configs/im_osnet_ain_x1_0_softmax_256x128_amsgrad_cosine.yaml) and `max_epoch=50`.
+
+| Model | # Param (10^6) | GFLOPs | Loss | Input | Transforms | Distance | MS+D+C->M | MS+M+C->D | MS+D+M->C |D+M+C->MS |
+| :--- | :---: | :---: | :---: | :---: | :---: | :---: | :---: | :---: | :---: | :---: |
+| osnet_x1_0 | 2.2 | 0.98 | softmax | (256, 128) | `random_flip`, `color_jitter` | `cosine` | [72.5 (44.2)](https://drive.google.com/file/d/1tuYY1vQXReEd8N8_npUkc7npPDDmjNCV/view?usp=sharing) | [65.2 (47.0)](https://drive.google.com/file/d/1UxUI4NsE108UCvcy3O1Ufe73nIVPKCiu/view?usp=sharing) | [23.9 (23.3)](https://drive.google.com/file/d/1kAA6qHJvbaJtyh1b39ZyEqWROwUgWIhl/view?usp=sharing) | [33.2 (12.6)](https://drive.google.com/file/d/1wAHuYVTzj8suOwqCNcEmu6YdbVnHDvA2/view?usp=sharing) |
+| osnet_ibn_x1_0 | 2.2 | 0.98 | softmax | (256, 128) | `random_flip`, `color_jitter` | `cosine` | [73.0 (44.9)](https://drive.google.com/file/d/14sH6yZwuNHPTElVoEZ26zozOOZIej5Mf/view?usp=sharing) | [64.6 (45.7)](https://drive.google.com/file/d/1Sk-2SSwKAF8n1Z4p_Lm_pl0E6v2WlIBn/view?usp=sharing) | [25.7 (25.4)](https://drive.google.com/file/d/1actHP7byqWcK4eBE1ojnspSMdo7k2W4G/view?usp=sharing) | [39.8 (16.2)](https://drive.google.com/file/d/1BGOSdLdZgqHe2qFafatb-5sPY40JlYfp/view?usp=sharing) |
+| osnet_ain_x1_0 | 2.2 | 0.98 | softmax | (256, 128) | `random_flip`, `color_jitter` | `cosine` | [73.3 (45.8)](https://drive.google.com/file/d/1nIrszJVYSHf3Ej8-j6DTFdWz8EnO42PB/view?usp=sharing) | [65.6 (47.2)](https://drive.google.com/file/d/1YjJ1ZprCmaKG6MH2P9nScB9FL_Utf9t1/view?usp=sharing) | [27.4 (27.1)](https://drive.google.com/file/d/1IxIg5P0cei3KPOJQ9ZRWDE_Mdrz01ha2/view?usp=sharing) | [40.2 (16.2)](https://drive.google.com/file/d/1KcoUKzLmsUoGHI7B6as_Z2fXL50gzexS/view?usp=sharing) |
diff --git a/trackers/strong_sort/deep/reid/docs/Makefile b/trackers/strong_sort/deep/reid/docs/Makefile
new file mode 100644
index 0000000000000000000000000000000000000000..298ea9e213e8c4c11f0431077510d4e325733c65
--- /dev/null
+++ b/trackers/strong_sort/deep/reid/docs/Makefile
@@ -0,0 +1,19 @@
+# Minimal makefile for Sphinx documentation
+#
+
+# You can set these variables from the command line.
+SPHINXOPTS =
+SPHINXBUILD = sphinx-build
+SOURCEDIR = .
+BUILDDIR = _build
+
+# Put it first so that "make" without argument is like "make help".
+help:
+ @$(SPHINXBUILD) -M help "$(SOURCEDIR)" "$(BUILDDIR)" $(SPHINXOPTS) $(O)
+
+.PHONY: help Makefile
+
+# Catch-all target: route all unknown targets to Sphinx using the new
+# "make mode" option. $(O) is meant as a shortcut for $(SPHINXOPTS).
+%: Makefile
+ @$(SPHINXBUILD) -M $@ "$(SOURCEDIR)" "$(BUILDDIR)" $(SPHINXOPTS) $(O)
\ No newline at end of file
diff --git a/trackers/strong_sort/deep/reid/docs/conf.py b/trackers/strong_sort/deep/reid/docs/conf.py
new file mode 100644
index 0000000000000000000000000000000000000000..4d27eedbac1d47dd6d1226b37e846a07364a0f1c
--- /dev/null
+++ b/trackers/strong_sort/deep/reid/docs/conf.py
@@ -0,0 +1,181 @@
+# -*- coding: utf-8 -*-
+#
+# Configuration file for the Sphinx documentation builder.
+#
+# This file does only contain a selection of the most common options. For a
+# full list see the documentation:
+# http://www.sphinx-doc.org/en/master/config
+
+# -- Path setup --------------------------------------------------------------
+
+# If extensions (or modules to document with autodoc) are in another directory,
+# add these directories to sys.path here. If the directory is relative to the
+# documentation root, use os.path.abspath to make it absolute, like shown here.
+#
+import os
+import sys
+
+sys.path.insert(0, os.path.abspath('..'))
+
+# -- Project information -----------------------------------------------------
+
+project = u'torchreid'
+copyright = u'2019, Kaiyang Zhou'
+author = u'Kaiyang Zhou'
+
+version_file = '../torchreid/__init__.py'
+with open(version_file, 'r') as f:
+ exec(compile(f.read(), version_file, 'exec'))
+__version__ = locals()['__version__']
+
+# The short X.Y version
+version = __version__
+# The full version, including alpha/beta/rc tags
+release = __version__
+
+# -- General configuration ---------------------------------------------------
+
+# If your documentation needs a minimal Sphinx version, state it here.
+#
+# needs_sphinx = '1.0'
+
+# Add any Sphinx extension module names here, as strings. They can be
+# extensions coming with Sphinx (named 'sphinx.ext.*') or your custom
+# ones.
+extensions = [
+ 'sphinx.ext.autodoc',
+ 'sphinxcontrib.napoleon',
+ 'sphinx.ext.viewcode',
+ 'sphinx.ext.githubpages',
+ 'sphinx_markdown_tables',
+]
+
+# Add any paths that contain templates here, relative to this directory.
+templates_path = ['_templates']
+
+# The suffix(es) of source filenames.
+# You can specify multiple suffix as a list of string:
+#
+source_suffix = ['.rst', '.md']
+# source_suffix = '.rst'
+source_parsers = {'.md': 'recommonmark.parser.CommonMarkParser'}
+
+# The master toctree document.
+master_doc = 'index'
+
+# The language for content autogenerated by Sphinx. Refer to documentation
+# for a list of supported languages.
+#
+# This is also used if you do content translation via gettext catalogs.
+# Usually you set "language" from the command line for these cases.
+language = None
+
+# List of patterns, relative to source directory, that match files and
+# directories to ignore when looking for source files.
+# This pattern also affects html_static_path and html_extra_path.
+exclude_patterns = [u'_build', 'Thumbs.db', '.DS_Store']
+
+# The name of the Pygments (syntax highlighting) style to use.
+pygments_style = None
+
+# -- Options for HTML output -------------------------------------------------
+
+# The theme to use for HTML and HTML Help pages. See the documentation for
+# a list of builtin themes.
+#
+html_theme = 'sphinx_rtd_theme'
+
+# Theme options are theme-specific and customize the look and feel of a theme
+# further. For a list of options available for each theme, see the
+# documentation.
+#
+# html_theme_options = {}
+
+# Add any paths that contain custom static files (such as style sheets) here,
+# relative to this directory. They are copied after the builtin static files,
+# so a file named "default.css" will overwrite the builtin "default.css".
+html_static_path = ['_static']
+
+# Custom sidebar templates, must be a dictionary that maps document names
+# to template names.
+#
+# The default sidebars (for documents that don't match any pattern) are
+# defined by theme itself. Builtin themes are using these templates by
+# default: ``['localtoc.html', 'relations.html', 'sourcelink.html',
+# 'searchbox.html']``.
+#
+# html_sidebars = {}
+
+# -- Options for HTMLHelp output ---------------------------------------------
+
+# Output file base name for HTML help builder.
+htmlhelp_basename = 'torchreiddoc'
+
+# -- Options for LaTeX output ------------------------------------------------
+
+latex_elements = {
+ # The paper size ('letterpaper' or 'a4paper').
+ #
+ # 'papersize': 'letterpaper',
+
+ # The font size ('10pt', '11pt' or '12pt').
+ #
+ # 'pointsize': '10pt',
+
+ # Additional stuff for the LaTeX preamble.
+ #
+ # 'preamble': '',
+
+ # Latex figure (float) alignment
+ #
+ # 'figure_align': 'htbp',
+}
+
+# Grouping the document tree into LaTeX files. List of tuples
+# (source start file, target name, title,
+# author, documentclass [howto, manual, or own class]).
+latex_documents = [
+ (
+ master_doc, 'torchreid.tex', u'torchreid Documentation',
+ u'Kaiyang Zhou', 'manual'
+ ),
+]
+
+# -- Options for manual page output ------------------------------------------
+
+# One entry per manual page. List of tuples
+# (source start file, name, description, authors, manual section).
+man_pages = [
+ (master_doc, 'torchreid', u'torchreid Documentation', [author], 1)
+]
+
+# -- Options for Texinfo output ----------------------------------------------
+
+# Grouping the document tree into Texinfo files. List of tuples
+# (source start file, target name, title, author,
+# dir menu entry, description, category)
+texinfo_documents = [
+ (
+ master_doc, 'torchreid', u'torchreid Documentation', author,
+ 'torchreid', 'One line description of project.', 'Miscellaneous'
+ ),
+]
+
+# -- Options for Epub output -------------------------------------------------
+
+# Bibliographic Dublin Core info.
+epub_title = project
+
+# The unique identifier of the text. This can be a ISBN number
+# or the project homepage.
+#
+# epub_identifier = ''
+
+# A unique identification for the text.
+#
+# epub_uid = ''
+
+# A list of files that should not be packed into the epub file.
+epub_exclude_files = ['search.html']
+
+# -- Extension configuration -------------------------------------------------
diff --git a/trackers/strong_sort/deep/reid/docs/datasets.rst b/trackers/strong_sort/deep/reid/docs/datasets.rst
new file mode 100644
index 0000000000000000000000000000000000000000..31f861133a4040e4228348e18e03cb0cd969a53a
--- /dev/null
+++ b/trackers/strong_sort/deep/reid/docs/datasets.rst
@@ -0,0 +1,264 @@
+.. _datasets:
+
+Datasets
+=========
+
+Here we provide a comprehensive guide on how to prepare the datasets.
+
+Suppose you want to store the reid data in a directory called "path/to/reid-data/", you need to specify the ``root`` as *root='path/to/reid-data/'* when initializing ``DataManager``. Below we use ``$REID`` to denote "path/to/reid-data".
+
+Please refer to :ref:`torchreid_data` for details regarding the arguments.
+
+
+.. note::
+ Dataset with a :math:`\dagger` symbol means that the process is automated, so you can directly call the dataset in ``DataManager`` (which automatically downloads the dataset and organizes the data structure). However, we also provide a way below to help the manual setup in case the automation fails.
+
+
+.. note::
+ The keys to use specific datasets are enclosed in the parantheses beside the datasets' names.
+
+
+.. note::
+ You are suggested to use the provided names for dataset folders such as "market1501" for Market1501 and "dukemtmcreid" for DukeMTMC-reID when doing the manual setup, otherwise you need to modify the source code accordingly (i.e. the ``dataset_dir`` attribute).
+
+.. note::
+ Some download links provided by the original authors might not work. You can email `Kaiyang Zhou `_ to reqeust new links. Please do provide your full name, institution, and purpose of using the data in the email (best use your work email address).
+
+.. contents::
+ :local:
+
+
+Image Datasets
+--------------
+
+Market1501 :math:`^\dagger` (``market1501``)
+^^^^^^^^^^^^^^^^^^^^^^^^^^^^^^^^^^^^^^^^^^^^^^^^^^^^^^^
+- Create a directory named "market1501" under ``$REID``.
+- Download the dataset to "market1501" from http://www.liangzheng.org/Project/project_reid.html and extract the files.
+- The data structure should look like
+
+.. code-block:: none
+
+ market1501/
+ Market-1501-v15.09.15/
+ query/
+ bounding_box_train/
+ bounding_box_test/
+
+- To use the extra 500K distractors (i.e. Market1501 + 500K), go to the **Market-1501+500k Dataset** section at http://www.liangzheng.org/Project/project_reid.html, download the zip file "distractors_500k.zip" and extract it under "market1501/Market-1501-v15.09.15". The argument to use these 500K distrctors is ``market1501_500k`` in ``ImageDataManager``.
+
+
+CUHK03 (``cuhk03``)
+^^^^^^^^^^^^^^^^^^^^^
+- Create a folder named "cuhk03" under ``$REID``.
+- Download the dataset to "cuhk03/" from http://www.ee.cuhk.edu.hk/~xgwang/CUHK_identification.html and extract "cuhk03_release.zip", resulting in "cuhk03/cuhk03_release/".
+- Download the new split (767/700) from `person-re-ranking `_. What you need are "cuhk03_new_protocol_config_detected.mat" and "cuhk03_new_protocol_config_labeled.mat". Put these two mat files under "cuhk03/".
+- The data structure should look like
+
+.. code-block:: none
+
+ cuhk03/
+ cuhk03_release/
+ cuhk03_new_protocol_config_detected.mat
+ cuhk03_new_protocol_config_labeled.mat
+
+
+- In the default mode, we load data using the new split (767/700). If you wanna use the original (20) splits (1367/100), please set ``cuhk03_classic_split`` to True in ``ImageDataManager``. As the CMC is computed differently from Market1501 for the 1367/100 split (see `here `_), you need to enable ``use_metric_cuhk03`` in ``ImageDataManager`` to activate the *single-gallery-shot* metric for fair comparison with some methods that adopt the old splits (*do not need to report mAP*). In addition, we support both *labeled* and *detected* modes. The default mode loads *detected* images. Enable ``cuhk03_labeled`` in ``ImageDataManager`` if you wanna train and test on *labeled* images.
+
+.. note::
+ The code will extract images in "cuhk-03.mat" and save them under "cuhk03/images_detected" and "cuhk03/images_labeled". Also, four json files will be automatically generated, i.e. "splits_classic_detected.json", "splits_classic_labeled.json", "splits_new_detected.json" and "splits_new_labeled.json". If the parent path of ``$REID`` is changed, these json files should be manually deleted. The code can automatically generate new json files to match the new path.
+
+
+DukeMTMC-reID :math:`^\dagger` (``dukemtmcreid``)
+^^^^^^^^^^^^^^^^^^^^^^^^^^^^^^^^^^^^^^^^^^^^^^^^^
+- Create a directory called "dukemtmc-reid" under ``$REID``.
+- Download "DukeMTMC-reID" from http://vision.cs.duke.edu/DukeMTMC/ and extract it under "dukemtmc-reid".
+- The data structure should look like
+
+.. code-block:: none
+
+ dukemtmc-reid/
+ DukeMTMC-reID/
+ query/
+ bounding_box_train/
+ bounding_box_test/
+ ...
+
+MSMT17 (``msmt17``)
+^^^^^^^^^^^^^^^^^^^^^
+- Create a directory called "msmt17" under ``$REID``.
+- Download the dataset from http://www.pkuvmc.com/publications/msmt17.html to "msmt17" and extract the files.
+- The data structure should look like
+
+.. code-block:: none
+
+ msmt17/
+ MSMT17_V1/ # or MSMT17_V2
+ train/
+ test/
+ list_train.txt
+ list_query.txt
+ list_gallery.txt
+ list_val.txt
+
+VIPeR :math:`^\dagger` (``viper``)
+^^^^^^^^^^^^^^^^^^^^^^^^^^^^^^^^^^^^^^^
+- The download link is http://users.soe.ucsc.edu/~manduchi/VIPeR.v1.0.zip.
+- Organize the dataset in a folder named "viper" as follows
+
+.. code-block:: none
+
+ viper/
+ VIPeR/
+ cam_a/
+ cam_b/
+
+GRID :math:`^\dagger` (``grid``)
+^^^^^^^^^^^^^^^^^^^^^^^^^^^^^^^^^^^^^
+- The download link is http://personal.ie.cuhk.edu.hk/~ccloy/files/datasets/underground_reid.zip.
+- Organize the dataset in a folder named "grid" as follows
+
+.. code-block:: none
+
+ grid/
+ underground_reid/
+ probe/
+ gallery/
+ ...
+
+CUHK01 (``cuhk01``)
+^^^^^^^^^^^^^^^^^^^^^^^^
+- Create a folder named "cuhk01" under ``$REID``.
+- Download "CUHK01.zip" from http://www.ee.cuhk.edu.hk/~xgwang/CUHK_identification.html and place it under "cuhk01/".
+- The code can automatically extract the files, or you can do it yourself.
+- The data structure should look like
+
+.. code-block:: none
+
+ cuhk01/
+ campus/
+
+SenseReID (``sensereid``)
+^^^^^^^^^^^^^^^^^^^^^^^^^^^
+- Create "sensereid" under ``$REID``.
+- Download the dataset from this `link `_ and extract it to "sensereid".
+- Organize the data to be like
+
+.. code-block:: none
+
+ sensereid/
+ SenseReID/
+ test_probe/
+ test_gallery/
+
+QMUL-iLIDS :math:`^\dagger` (``ilids``)
+^^^^^^^^^^^^^^^^^^^^^^^^^^^^^^^^^^^^^^^
+- Create a folder named "ilids" under ``$REID``.
+- Download the dataset from http://www.eecs.qmul.ac.uk/~jason/data/i-LIDS_Pedestrian.tgz and organize it to look like
+
+.. code-block:: none
+
+ ilids/
+ i-LIDS_Pedestrian/
+ Persons/
+
+PRID (``prid``)
+^^^^^^^^^^^^^^^^^^^
+- Create a directory named "prid2011" under ``$REID``.
+- Download the dataset from https://www.tugraz.at/institute/icg/research/team-bischof/lrs/downloads/PRID11/ and extract it under "prid2011".
+- The data structure should end up with
+
+.. code-block:: none
+
+ prid2011/
+ prid_2011/
+ single_shot/
+ multi_shot/
+
+CUHK02 (``cuhk02``)
+^^^^^^^^^^^^^^^^^^^^^
+- Create a folder named "cuhk02" under ``$REID``.
+- Download the data from http://www.ee.cuhk.edu.hk/~xgwang/CUHK_identification.html and put it under "cuhk02/".
+- Extract the file so the data structure looks like
+
+.. code-block:: none
+
+ cuhk02/
+ Dataset/
+ P1/
+ P2/
+ P3/
+ P4/
+ P5/
+
+CUHKSYSU (``cuhksysu``)
+^^^^^^^^^^^^^^^^^^^^^^^^^^
+- Create a folder named "cuhksysu" under ``$REID``.
+- Download the data to "cuhksysu/" from this `google drive link `_.
+- Extract the zip file under "cuhksysu/".
+- The data structure should look like
+
+.. code-block:: none
+
+ cuhksysu/
+ cropped_images
+
+
+Video Datasets
+--------------
+
+MARS (``mars``)
+^^^^^^^^^^^^^^^^^
+- Create "mars/" under ``$REID``.
+- Download the dataset from http://www.liangzheng.com.cn/Project/project_mars.html and place it in "mars/".
+- Extract "bbox_train.zip" and "bbox_test.zip".
+- Download the split metadata from https://github.com/liangzheng06/MARS-evaluation/tree/master/info and put "info/" in "mars/".
+- The data structure should end up with
+
+.. code-block:: none
+
+ mars/
+ bbox_test/
+ bbox_train/
+ info/
+
+iLIDS-VID :math:`^\dagger` (``ilidsvid``)
+^^^^^^^^^^^^^^^^^^^^^^^^^^^^^^^^^^^^^^^^^
+- Create "ilids-vid" under ``$REID``.
+- Download the dataset from https://xiatian-zhu.github.io/downloads_qmul_iLIDS-VID_ReID_dataset.html to "ilids-vid".
+- Organize the data structure to match
+
+.. code-block:: none
+
+ ilids-vid/
+ i-LIDS-VID/
+ train-test people splits/
+
+PRID2011 (``prid2011``)
+^^^^^^^^^^^^^^^^^^^^^^^^^
+- Create a directory named "prid2011" under ``$REID``.
+- Download the dataset from https://www.tugraz.at/institute/icg/research/team-bischof/lrs/downloads/PRID11/ and extract it under "prid2011".
+- Download the split created by *iLIDS-VID* from `this google drive `_ and put it under "prid2011/". Following the standard protocol, only 178 persons whose sequences are more than a threshold are used.
+- The data structure should end up with
+
+.. code-block:: none
+
+ prid2011/
+ splits_prid2011.json
+ prid_2011/
+ single_shot/
+ multi_shot/
+
+DukeMTMC-VideoReID :math:`^\dagger` (``dukemtmcvidreid``)
+^^^^^^^^^^^^^^^^^^^^^^^^^^^^^^^^^^^^^^^^^^^^^^^^^^^^^^^^^^
+- Create "dukemtmc-vidreid" under ``$REID``.
+- Download "DukeMTMC-VideoReID" from http://vision.cs.duke.edu/DukeMTMC/ and unzip the file to "dukemtmc-vidreid/".
+- The data structure should look like
+
+.. code-block:: none
+
+ dukemtmc-vidreid/
+ DukeMTMC-VideoReID/
+ train/
+ query/
+ gallery/
diff --git a/trackers/strong_sort/deep/reid/docs/evaluation.rst b/trackers/strong_sort/deep/reid/docs/evaluation.rst
new file mode 100644
index 0000000000000000000000000000000000000000..979ec7532c738e03495e9b96bf7de0c40020547e
--- /dev/null
+++ b/trackers/strong_sort/deep/reid/docs/evaluation.rst
@@ -0,0 +1,21 @@
+Evaluation
+==========
+
+Image ReID
+-----------
+- **Market1501**, **DukeMTMC-reID**, **CUHK03 (767/700 split)** and **MSMT17** have fixed split so keeping ``split_id=0`` is fine.
+- **CUHK03 (classic split)** has 20 fixed splits, so do ``split_id=0~19``.
+- **VIPeR** contains 632 identities each with 2 images under two camera views. Evaluation should be done for 10 random splits. Each split randomly divides 632 identities to 316 train ids (632 images) and the other 316 test ids (632 images). Note that, in each random split, there are two sub-splits, one using camera-A as query and camera-B as gallery while the other one using camera-B as query and camera-A as gallery. Thus, there are totally 20 splits generated with ``split_id`` starting from 0 to 19. Models can be trained on ``split_id=[0, 2, 4, 6, 8, 10, 12, 14, 16, 18]`` (because ``split_id=0`` and ``split_id=1`` share the same train set, and so on and so forth.). At test time, models trained on ``split_id=0`` can be directly evaluated on ``split_id=1``, models trained on ``split_id=2`` can be directly evaluated on ``split_id=3``, and so on and so forth.
+- **CUHK01** is similar to VIPeR in the split generation.
+- **GRID** , **iLIDS** and **PRID** have 10 random splits, so evaluation should be done by varying ``split_id`` from 0 to 9.
+- **SenseReID** has no training images and is used for evaluation only.
+
+
+.. note::
+ The ``split_id`` argument is defined in ``ImageDataManager`` and ``VideoDataManager``. Please refer to :ref:`torchreid_data`.
+
+
+Video ReID
+-----------
+- **MARS** and **DukeMTMC-VideoReID** have fixed single split so using ``split_id=0`` is ok.
+- **iLIDS-VID** and **PRID2011** have 10 predefined splits so evaluation should be done by varying ``split_id`` from 0 to 9.
\ No newline at end of file
diff --git a/trackers/strong_sort/deep/reid/docs/figures/actmap.jpg b/trackers/strong_sort/deep/reid/docs/figures/actmap.jpg
new file mode 100644
index 0000000000000000000000000000000000000000..1a0522b7e026f5c96ec5c2af21ae275a74dc5ac8
Binary files /dev/null and b/trackers/strong_sort/deep/reid/docs/figures/actmap.jpg differ
diff --git a/trackers/strong_sort/deep/reid/docs/figures/ranking_results.jpg b/trackers/strong_sort/deep/reid/docs/figures/ranking_results.jpg
new file mode 100644
index 0000000000000000000000000000000000000000..a759dfc17cac44734fe6a3ae03db58ea96485aef
Binary files /dev/null and b/trackers/strong_sort/deep/reid/docs/figures/ranking_results.jpg differ
diff --git a/trackers/strong_sort/deep/reid/docs/index.rst b/trackers/strong_sort/deep/reid/docs/index.rst
new file mode 100644
index 0000000000000000000000000000000000000000..c437deaff36e09a775ec783e0a7f68c35379aaf8
--- /dev/null
+++ b/trackers/strong_sort/deep/reid/docs/index.rst
@@ -0,0 +1,35 @@
+.. include:: ../README.rst
+
+
+.. toctree::
+ :hidden:
+
+ user_guide
+ datasets
+ evaluation
+
+.. toctree::
+ :caption: Package Reference
+ :hidden:
+
+ pkg/data
+ pkg/engine
+ pkg/losses
+ pkg/metrics
+ pkg/models
+ pkg/optim
+ pkg/utils
+
+.. toctree::
+ :caption: Resources
+ :hidden:
+
+ AWESOME_REID.md
+ MODEL_ZOO.md
+
+
+Indices and tables
+==================
+
+* :ref:`genindex`
+* :ref:`modindex`
\ No newline at end of file
diff --git a/trackers/strong_sort/deep/reid/docs/pkg/data.rst b/trackers/strong_sort/deep/reid/docs/pkg/data.rst
new file mode 100644
index 0000000000000000000000000000000000000000..3dc47d6ae9790042d0902b6cf1612112539886ce
--- /dev/null
+++ b/trackers/strong_sort/deep/reid/docs/pkg/data.rst
@@ -0,0 +1,86 @@
+.. _torchreid_data:
+
+torchreid.data
+==============
+
+
+Data Manager
+---------------------------
+
+.. automodule:: torchreid.data.datamanager
+ :members:
+
+
+Sampler
+-----------------------
+
+.. automodule:: torchreid.data.sampler
+ :members:
+
+
+Transforms
+---------------------------
+
+.. automodule:: torchreid.data.transforms
+ :members:
+
+
+Dataset
+---------------------------
+
+.. automodule:: torchreid.data.datasets.dataset
+ :members:
+
+
+.. automodule:: torchreid.data.datasets.__init__
+ :members:
+
+
+Image Datasets
+------------------------------
+
+.. automodule:: torchreid.data.datasets.image.market1501
+ :members:
+
+.. automodule:: torchreid.data.datasets.image.cuhk03
+ :members:
+
+.. automodule:: torchreid.data.datasets.image.dukemtmcreid
+ :members:
+
+.. automodule:: torchreid.data.datasets.image.msmt17
+ :members:
+
+.. automodule:: torchreid.data.datasets.image.viper
+ :members:
+
+.. automodule:: torchreid.data.datasets.image.grid
+ :members:
+
+.. automodule:: torchreid.data.datasets.image.cuhk01
+ :members:
+
+.. automodule:: torchreid.data.datasets.image.ilids
+ :members:
+
+.. automodule:: torchreid.data.datasets.image.sensereid
+ :members:
+
+.. automodule:: torchreid.data.datasets.image.prid
+ :members:
+
+
+Video Datasets
+------------------------------
+
+.. automodule:: torchreid.data.datasets.video.mars
+ :members:
+
+.. automodule:: torchreid.data.datasets.video.ilidsvid
+ :members:
+
+.. automodule:: torchreid.data.datasets.video.prid2011
+ :members:
+
+.. automodule:: torchreid.data.datasets.video.dukemtmcvidreid
+ :members:
\ No newline at end of file
diff --git a/trackers/strong_sort/deep/reid/docs/pkg/engine.rst b/trackers/strong_sort/deep/reid/docs/pkg/engine.rst
new file mode 100644
index 0000000000000000000000000000000000000000..ae2bc6859c090af48304dbb3d383efebe73ea9b2
--- /dev/null
+++ b/trackers/strong_sort/deep/reid/docs/pkg/engine.rst
@@ -0,0 +1,31 @@
+.. _torchreid_engine:
+
+torchreid.engine
+==================
+
+
+Base Engine
+------------
+
+.. autoclass:: torchreid.engine.engine.Engine
+ :members:
+
+
+Image Engines
+-------------
+
+.. autoclass:: torchreid.engine.image.softmax.ImageSoftmaxEngine
+ :members:
+
+
+.. autoclass:: torchreid.engine.image.triplet.ImageTripletEngine
+ :members:
+
+
+Video Engines
+-------------
+
+.. autoclass:: torchreid.engine.video.softmax.VideoSoftmaxEngine
+
+
+.. autoclass:: torchreid.engine.video.triplet.VideoTripletEngine
\ No newline at end of file
diff --git a/trackers/strong_sort/deep/reid/docs/pkg/losses.rst b/trackers/strong_sort/deep/reid/docs/pkg/losses.rst
new file mode 100644
index 0000000000000000000000000000000000000000..33fd9bc46fdbb141aee78cce63618dd99fc51f9d
--- /dev/null
+++ b/trackers/strong_sort/deep/reid/docs/pkg/losses.rst
@@ -0,0 +1,18 @@
+.. _torchreid_losses:
+
+torchreid.losses
+=================
+
+
+Softmax
+--------
+
+.. automodule:: torchreid.losses.cross_entropy_loss
+ :members:
+
+
+Triplet
+-------
+
+.. automodule:: torchreid.losses.hard_mine_triplet_loss
+ :members:
\ No newline at end of file
diff --git a/trackers/strong_sort/deep/reid/docs/pkg/metrics.rst b/trackers/strong_sort/deep/reid/docs/pkg/metrics.rst
new file mode 100644
index 0000000000000000000000000000000000000000..5a52a90128a944061a609f982e21b1a9e53204e5
--- /dev/null
+++ b/trackers/strong_sort/deep/reid/docs/pkg/metrics.rst
@@ -0,0 +1,25 @@
+.. _torchreid_metrics:
+
+torchreid.metrics
+=================
+
+
+Distance
+---------
+
+.. automodule:: torchreid.metrics.distance
+ :members:
+
+
+Accuracy
+--------
+
+.. automodule:: torchreid.metrics.accuracy
+ :members:
+
+
+Rank
+-----
+
+.. automodule:: torchreid.metrics.rank
+ :members: evaluate_rank
\ No newline at end of file
diff --git a/trackers/strong_sort/deep/reid/docs/pkg/models.rst b/trackers/strong_sort/deep/reid/docs/pkg/models.rst
new file mode 100644
index 0000000000000000000000000000000000000000..685bc73e81521627b91c9f3098e26c603c3e7b41
--- /dev/null
+++ b/trackers/strong_sort/deep/reid/docs/pkg/models.rst
@@ -0,0 +1,43 @@
+.. _torchreid_models:
+
+torchreid.models
+=================
+
+Interface
+---------
+
+.. automodule:: torchreid.models.__init__
+ :members:
+
+
+ImageNet Classification Models
+-------------------------------
+
+.. autoclass:: torchreid.models.resnet.ResNet
+.. autoclass:: torchreid.models.senet.SENet
+.. autoclass:: torchreid.models.densenet.DenseNet
+.. autoclass:: torchreid.models.inceptionresnetv2.InceptionResNetV2
+.. autoclass:: torchreid.models.inceptionv4.InceptionV4
+.. autoclass:: torchreid.models.xception.Xception
+
+
+Lightweight Models
+------------------
+
+.. autoclass:: torchreid.models.nasnet.NASNetAMobile
+.. autoclass:: torchreid.models.mobilenetv2.MobileNetV2
+.. autoclass:: torchreid.models.shufflenet.ShuffleNet
+.. autoclass:: torchreid.models.squeezenet.SqueezeNet
+.. autoclass:: torchreid.models.shufflenetv2.ShuffleNetV2
+
+
+ReID-specific Models
+--------------------
+
+.. autoclass:: torchreid.models.mudeep.MuDeep
+.. autoclass:: torchreid.models.resnetmid.ResNetMid
+.. autoclass:: torchreid.models.hacnn.HACNN
+.. autoclass:: torchreid.models.pcb.PCB
+.. autoclass:: torchreid.models.mlfn.MLFN
+.. autoclass:: torchreid.models.osnet.OSNet
+.. autoclass:: torchreid.models.osnet_ain.OSNet
\ No newline at end of file
diff --git a/trackers/strong_sort/deep/reid/docs/pkg/optim.rst b/trackers/strong_sort/deep/reid/docs/pkg/optim.rst
new file mode 100644
index 0000000000000000000000000000000000000000..560162340938ab8ad4221e547154c3d923a59f8c
--- /dev/null
+++ b/trackers/strong_sort/deep/reid/docs/pkg/optim.rst
@@ -0,0 +1,18 @@
+.. _torchreid_optim:
+
+torchreid.optim
+=================
+
+
+Optimizer
+----------
+
+.. automodule:: torchreid.optim.optimizer
+ :members: build_optimizer
+
+
+LR Scheduler
+-------------
+
+.. automodule:: torchreid.optim.lr_scheduler
+ :members: build_lr_scheduler
\ No newline at end of file
diff --git a/trackers/strong_sort/deep/reid/docs/pkg/utils.rst b/trackers/strong_sort/deep/reid/docs/pkg/utils.rst
new file mode 100644
index 0000000000000000000000000000000000000000..1545bc29c88f98cd0a7750e42776a3fd682bd93d
--- /dev/null
+++ b/trackers/strong_sort/deep/reid/docs/pkg/utils.rst
@@ -0,0 +1,41 @@
+.. _torchreid_utils:
+
+torchreid.utils
+=================
+
+Average Meter
+--------------
+
+.. automodule:: torchreid.utils.avgmeter
+ :members:
+
+
+Loggers
+-------
+
+.. automodule:: torchreid.utils.loggers
+ :members:
+
+
+Generic Tools
+---------------
+.. automodule:: torchreid.utils.tools
+ :members:
+
+
+ReID Tools
+----------
+
+.. automodule:: torchreid.utils.reidtools
+ :members:
+
+
+Torch Tools
+------------
+
+.. automodule:: torchreid.utils.torchtools
+ :members:
+
+
+.. automodule:: torchreid.utils.model_complexity
+ :members:
diff --git a/trackers/strong_sort/deep/reid/docs/requirements.txt b/trackers/strong_sort/deep/reid/docs/requirements.txt
new file mode 100644
index 0000000000000000000000000000000000000000..b6f5d7db27b223bfed9bb617fe9fb6364f28fb40
--- /dev/null
+++ b/trackers/strong_sort/deep/reid/docs/requirements.txt
@@ -0,0 +1,6 @@
+sphinx==2.2.0
+sphinx-markdown-tables
+sphinx-rtd-theme
+sphinxcontrib-napoleon
+sphinxcontrib-websupport
+recommonmark
\ No newline at end of file
diff --git a/trackers/strong_sort/deep/reid/docs/user_guide.rst b/trackers/strong_sort/deep/reid/docs/user_guide.rst
new file mode 100644
index 0000000000000000000000000000000000000000..5415109fab92a170e87e5af1917f35d3ece76026
--- /dev/null
+++ b/trackers/strong_sort/deep/reid/docs/user_guide.rst
@@ -0,0 +1,351 @@
+How-to
+============
+
+.. contents::
+ :local:
+
+
+Prepare datasets
+-----------------
+See :ref:`datasets`.
+
+
+Find model keys
+-----------------
+Keys are listed under the *Public keys* section within each model class in :ref:`torchreid_models`.
+
+
+Show available models
+----------------------
+
+.. code-block:: python
+
+ import torchreid
+ torchreid.models.show_avai_models()
+
+
+Change the training sampler
+-----------------------------
+The default ``train_sampler`` is "RandomSampler". You can give the specific sampler name as input to ``train_sampler``, e.g. ``train_sampler='RandomIdentitySampler'`` for triplet loss.
+
+
+Choose an optimizer/lr_scheduler
+----------------------------------
+Please refer to the source code of ``build_optimizer``/``build_lr_scheduler`` in :ref:`torchreid_optim` for details.
+
+
+Resume training
+----------------
+Suppose the checkpoint is saved in "log/resnet50/model.pth.tar-30", you can do
+
+.. code-block:: python
+
+ start_epoch = torchreid.utils.resume_from_checkpoint(
+ 'log/resnet50/model.pth.tar-30',
+ model,
+ optimizer
+ )
+
+ engine.run(
+ save_dir='log/resnet50',
+ max_epoch=60,
+ start_epoch=start_epoch
+ )
+
+
+Compute model complexity
+--------------------------
+We provide a tool in ``torchreid.utils.model_complexity.py`` to automatically compute the model complexity, i.e. number of parameters and FLOPs.
+
+.. code-block:: python
+
+ from torchreid import models, utils
+
+ model = models.build_model(name='resnet50', num_classes=1000)
+ num_params, flops = utils.compute_model_complexity(model, (1, 3, 256, 128))
+
+ # show detailed complexity for each module
+ utils.compute_model_complexity(model, (1, 3, 256, 128), verbose=True)
+
+ # count flops for all layers including ReLU and BatchNorm
+ utils.compute_model_complexity(model, (1, 3, 256, 128), verbose=True, only_conv_linear=False)
+
+Note that (1) this function only provides an estimate of the theoretical time complexity rather than the actual running time which depends on implementations and hardware; (2) the FLOPs is only counted for layers that are used at test time. This means that redundant layers such as person ID classification layer will be ignored. The inference graph depends on how you define the computations in ``forward()``.
+
+
+Combine multiple datasets
+---------------------------
+Easy. Just give whatever datasets (keys) you want to the ``sources`` argument when instantiating a data manager. For example,
+
+.. code-block:: python
+
+ datamanager = torchreid.data.ImageDataManager(
+ root='reid-data',
+ sources=['market1501', 'dukemtmcreid', 'cuhk03', 'msmt17'],
+ height=256,
+ width=128,
+ batch_size=32
+ )
+
+In this example, the target datasets are Market1501, DukeMTMC-reID, CUHK03 and MSMT17 as the ``targets`` argument is not specified. Please refer to ``Engine.test()`` in :ref:`torchreid_engine` for details regarding how evaluation is performed.
+
+
+Do cross-dataset evaluation
+-----------------------------
+Easy. Just give whatever datasets (keys) you want to the argument ``targets``, like
+
+.. code-block:: python
+
+ datamanager = torchreid.data.ImageDataManager(
+ root='reid-data',
+ sources='market1501',
+ targets='dukemtmcreid', # or targets='cuhk03' or targets=['dukemtmcreid', 'cuhk03']
+ height=256,
+ width=128,
+ batch_size=32
+ )
+
+
+Combine train, query and gallery
+---------------------------------
+This can be easily done by setting ``combineall=True`` when instantiating a data manager. Below is an example of using Market1501,
+
+.. code-block:: python
+
+ datamanager = torchreid.data.ImageDataManager(
+ root='reid-data',
+ sources='market1501',
+ height=256,
+ width=128,
+ batch_size=32,
+ market1501_500k=False,
+ combineall=True # it's me, here
+ )
+
+More specifically, with ``combineall=False``, you will get
+
+.. code-block:: none
+
+ => Loaded Market1501
+ ----------------------------------------
+ subset | # ids | # images | # cameras
+ ----------------------------------------
+ train | 751 | 12936 | 6
+ query | 750 | 3368 | 6
+ gallery | 751 | 15913 | 6
+ ---------------------------------------
+
+with ``combineall=True``, you will get
+
+.. code-block:: none
+
+ => Loaded Market1501
+ ----------------------------------------
+ subset | # ids | # images | # cameras
+ ----------------------------------------
+ train | 1501 | 29419 | 6
+ query | 750 | 3368 | 6
+ gallery | 751 | 15913 | 6
+ ---------------------------------------
+
+
+Optimize layers with different learning rates
+-----------------------------------------------
+A common practice for fine-tuning pretrained models is to use a smaller learning rate for base layers and a large learning rate for randomly initialized layers (referred to as ``new_layers``). ``torchreid.optim.optimizer`` has implemented such feature. What you need to do is to set ``staged_lr=True`` and give the names of ``new_layers`` such as "classifier".
+
+Below is an example of setting different learning rates for base layers and new layers in ResNet50,
+
+.. code-block:: python
+
+ # New layer "classifier" has a learning rate of 0.01
+ # The base layers have a learning rate of 0.001
+ optimizer = torchreid.optim.build_optimizer(
+ model,
+ optim='sgd',
+ lr=0.01,
+ staged_lr=True,
+ new_layers='classifier',
+ base_lr_mult=0.1
+ )
+
+Please refer to :ref:`torchreid_optim` for more details.
+
+
+Do two-stepped transfer learning
+-------------------------------------
+To prevent the pretrained layers from being damaged by harmful gradients back-propagated from randomly initialized layers, one can adopt the *two-stepped transfer learning strategy* presented in `Deep Transfer Learning for Person Re-identification `_. The basic idea is to pretrain the randomly initialized layers for few epochs while keeping the base layers frozen before training all layers end-to-end.
+
+This has been implemented in ``Engine.train()`` (see :ref:`torchreid_engine`). The arguments related to this feature are ``fixbase_epoch`` and ``open_layers``. Intuitively, ``fixbase_epoch`` denotes the number of epochs to keep the base layers frozen; ``open_layers`` means which layer is open for training.
+
+For example, say you want to pretrain the classification layer named "classifier" in ResNet50 for 5 epochs before training all layers, you can do
+
+.. code-block:: python
+
+ engine.run(
+ save_dir='log/resnet50',
+ max_epoch=60,
+ eval_freq=10,
+ print_freq=10,
+ test_only=False,
+ fixbase_epoch=5,
+ open_layers='classifier'
+ )
+ # or open_layers=['fc', 'classifier'] if there is another fc layer that
+ # is randomly initialized, like resnet50_fc512
+
+Note that ``fixbase_epoch`` is counted into ``max_epoch``. In the above example, the base network will be fixed for 5 epochs and then open for training for 55 epochs. Thus, if you want to freeze some layers throughout the training, what you can do is to set ``fixbase_epoch`` equal to ``max_epoch`` and put the layer names in ``open_layers`` which you want to train.
+
+
+Test a trained model
+----------------------
+You can load a trained model using :code:`torchreid.utils.load_pretrained_weights(model, weight_path)` and set ``test_only=True`` in ``engine.run()``.
+
+
+Fine-tune a model pre-trained on reid datasets
+-----------------------------------------------
+Use :code:`torchreid.utils.load_pretrained_weights(model, weight_path)` to load the pre-trained weights and then fine-tune on the dataset you want.
+
+
+Visualize learning curves with tensorboard
+--------------------------------------------
+The ``SummaryWriter()`` for tensorboard will be automatically initialized in ``engine.run()`` when you are training your model. Therefore, you do not need to do extra jobs. After the training is done, the ``*tf.events*`` file will be saved in ``save_dir``. Then, you just call ``tensorboard --logdir=your_save_dir`` in your terminal and visit ``http://localhost:6006/`` in a web browser. See `pytorch tensorboard `_ for further information.
+
+
+Visualize ranking results
+---------------------------
+This can be achieved by setting ``visrank`` to true in ``engine.run()``. ``visrank_topk`` determines the top-k images to be visualized (Default is ``visrank_topk=10``). Note that ``visrank`` can only be used in test mode, i.e. ``test_only=True`` in ``engine.run()``. The output will be saved under ``save_dir/visrank_DATASETNAME`` where each plot contains the top-k similar gallery images given a query. An example is shown below where red and green denote incorrect and correct matches respectively.
+
+.. image:: figures/ranking_results.jpg
+ :width: 800px
+ :align: center
+
+
+Visualize activation maps
+--------------------------
+To understand where the CNN focuses on to extract features for ReID, you can visualize the activation maps as in `OSNet `_. This is implemented in ``tools/visualize_actmap.py`` (check the code for more details). An example running command is
+
+.. code-block:: shell
+
+ python tools/visualize_actmap.py \
+ --root $DATA/reid \
+ -d market1501 \
+ -m osnet_x1_0 \
+ --weights PATH_TO_PRETRAINED_WEIGHTS \
+ --save-dir log/visactmap_osnet_x1_0_market1501
+
+The output will look like (from left to right: image, activation map, overlapped image)
+
+.. image:: figures/actmap.jpg
+ :width: 300px
+ :align: center
+
+
+.. note::
+ In order to visualize activation maps, the CNN needs to output the last convolutional feature maps at eval mode. See ``torchreid/models/osnet.py`` for example.
+
+
+Use your own dataset
+----------------------
+1. Write your own dataset class. Below is a template for image dataset. However, it can also be applied to a video dataset class, for which you simply change ``ImageDataset`` to ``VideoDataset``.
+
+.. code-block:: python
+
+ from __future__ import absolute_import
+ from __future__ import print_function
+ from __future__ import division
+
+ import sys
+ import os
+ import os.path as osp
+
+ from torchreid.data import ImageDataset
+
+
+ class NewDataset(ImageDataset):
+ dataset_dir = 'new_dataset'
+
+ def __init__(self, root='', **kwargs):
+ self.root = osp.abspath(osp.expanduser(root))
+ self.dataset_dir = osp.join(self.root, self.dataset_dir)
+
+ # All you need to do here is to generate three lists,
+ # which are train, query and gallery.
+ # Each list contains tuples of (img_path, pid, camid),
+ # where
+ # - img_path (str): absolute path to an image.
+ # - pid (int): person ID, e.g. 0, 1.
+ # - camid (int): camera ID, e.g. 0, 1.
+ # Note that
+ # - pid and camid should be 0-based.
+ # - query and gallery should share the same pid scope (e.g.
+ # pid=0 in query refers to the same person as pid=0 in gallery).
+ # - train, query and gallery share the same camid scope (e.g.
+ # camid=0 in train refers to the same camera as camid=0
+ # in query/gallery).
+ train = ...
+ query = ...
+ gallery = ...
+
+ super(NewDataset, self).__init__(train, query, gallery, **kwargs)
+
+
+2. Register your dataset.
+
+.. code-block:: python
+
+ import torchreid
+ torchreid.data.register_image_dataset('new_dataset', NewDataset)
+
+
+3. Initialize a data manager with your dataset.
+
+.. code-block:: python
+
+ # use your own dataset only
+ datamanager = torchreid.data.ImageDataManager(
+ root='reid-data',
+ sources='new_dataset'
+ )
+ # combine with other datasets
+ datamanager = torchreid.data.ImageDataManager(
+ root='reid-data',
+ sources=['new_dataset', 'dukemtmcreid']
+ )
+ # cross-dataset evaluation
+ datamanager = torchreid.data.ImageDataManager(
+ root='reid-data',
+ sources=['new_dataset', 'dukemtmcreid'],
+ targets='market1501' # or targets=['market1501', 'cuhk03']
+ )
+
+
+
+Design your own Engine
+------------------------
+A new Engine should be designed if you have your own loss function. The base Engine class ``torchreid.engine.Engine`` has implemented some generic methods which you can inherit to avoid re-writing. Please refer to the source code for more details. You are suggested to see how ``ImageSoftmaxEngine`` and ``ImageTripletEngine`` are constructed (also ``VideoSoftmaxEngine`` and ``VideoTripletEngine``). All you need to implement might be just a ``forward_backward()`` function.
+
+
+Use Torchreid as a feature extractor in your projects
+-------------------------------------------------------
+We have provided a simple API for feature extraction, which accepts input of various types such as a list of image paths or numpy arrays. More details can be found in the code at ``torchreid/utils/feature_extractor.py``. Here we show a simple example of how to extract features given a list of image paths.
+
+.. code-block:: python
+
+ from torchreid.utils import FeatureExtractor
+
+ extractor = FeatureExtractor(
+ model_name='osnet_x1_0',
+ model_path='a/b/c/model.pth.tar',
+ device='cuda'
+ )
+
+ image_list = [
+ 'a/b/c/image001.jpg',
+ 'a/b/c/image002.jpg',
+ 'a/b/c/image003.jpg',
+ 'a/b/c/image004.jpg',
+ 'a/b/c/image005.jpg'
+ ]
+
+ features = extractor(image_list)
+ print(features.shape) # output (5, 512)
diff --git a/trackers/strong_sort/deep/reid/linter.sh b/trackers/strong_sort/deep/reid/linter.sh
new file mode 100644
index 0000000000000000000000000000000000000000..9db34f9f86e44fad1263758a6ccaf09eb816c11a
--- /dev/null
+++ b/trackers/strong_sort/deep/reid/linter.sh
@@ -0,0 +1,11 @@
+echo "Running isort"
+isort -y -sp .
+echo "Done"
+
+echo "Running yapf"
+yapf -i -r -vv -e build .
+echo "Done"
+
+echo "Running flake8"
+flake8 .
+echo "Done"
\ No newline at end of file
diff --git a/trackers/strong_sort/deep/reid/projects/DML/README.md b/trackers/strong_sort/deep/reid/projects/DML/README.md
new file mode 100644
index 0000000000000000000000000000000000000000..e81be7fe73b201236534d2785c6b2de7a231e017
--- /dev/null
+++ b/trackers/strong_sort/deep/reid/projects/DML/README.md
@@ -0,0 +1,16 @@
+# Deep mutual learning
+
+This repo implements [Deep Mutual Learning (CVPR'18)](https://zpascal.net/cvpr2018/Zhang_Deep_Mutual_Learning_CVPR_2018_paper.pdf) (DML) for person re-id.
+
+We used this code in our [OSNet](https://arxiv.org/pdf/1905.00953.pdf) paper (see Supp. B). The training command to reproduce the result of "triplet + DML" (Table 12f in the paper) is
+```bash
+python main.py \
+--config-file im_osnet_x1_0_dml_256x128_amsgrad_cosine.yaml \
+--root $DATA
+```
+
+`$DATA` corresponds to the path to your dataset folder.
+
+Change `model.deploy` to `both` if you wanna enable model ensembling.
+
+If you have any questions, please raise an issue in the Issues area.
\ No newline at end of file
diff --git a/trackers/strong_sort/deep/reid/projects/DML/default_config.py b/trackers/strong_sort/deep/reid/projects/DML/default_config.py
new file mode 100644
index 0000000000000000000000000000000000000000..9b15e35f5e33028eac0cca3c92dee3c7a97130b7
--- /dev/null
+++ b/trackers/strong_sort/deep/reid/projects/DML/default_config.py
@@ -0,0 +1,207 @@
+from yacs.config import CfgNode as CN
+
+
+def get_default_config():
+ cfg = CN()
+
+ # model
+ cfg.model = CN()
+ cfg.model.name = 'resnet50'
+ cfg.model.pretrained = True # automatically load pretrained model weights if available
+ cfg.model.load_weights1 = '' # path to model-1 weights
+ cfg.model.load_weights2 = '' # path to model-2 weights
+ cfg.model.resume1 = '' # path to checkpoint for resume training
+ cfg.model.resume2 = '' # path to checkpoint for resume training
+ cfg.model.deploy = 'model1' # model1, model2 or both
+
+ # data
+ cfg.data = CN()
+ cfg.data.type = 'image'
+ cfg.data.root = 'reid-data'
+ cfg.data.sources = ['market1501']
+ cfg.data.targets = ['market1501']
+ cfg.data.workers = 4 # number of data loading workers
+ cfg.data.split_id = 0 # split index
+ cfg.data.height = 256 # image height
+ cfg.data.width = 128 # image width
+ cfg.data.combineall = False # combine train, query and gallery for training
+ cfg.data.transforms = ['random_flip'] # data augmentation
+ cfg.data.norm_mean = [0.485, 0.456, 0.406] # default is imagenet mean
+ cfg.data.norm_std = [0.229, 0.224, 0.225] # default is imagenet std
+ cfg.data.save_dir = 'log' # path to save log
+ cfg.data.load_train_targets = False
+
+ # specific datasets
+ cfg.market1501 = CN()
+ cfg.market1501.use_500k_distractors = False # add 500k distractors to the gallery set for market1501
+ cfg.cuhk03 = CN()
+ cfg.cuhk03.labeled_images = False # use labeled images, if False, use detected images
+ cfg.cuhk03.classic_split = False # use classic split by Li et al. CVPR14
+ cfg.cuhk03.use_metric_cuhk03 = False # use cuhk03's metric for evaluation
+
+ # sampler
+ cfg.sampler = CN()
+ cfg.sampler.train_sampler = 'RandomSampler'
+ cfg.sampler.num_instances = 4 # number of instances per identity for RandomIdentitySampler
+
+ # video reid setting
+ cfg.video = CN()
+ cfg.video.seq_len = 15 # number of images to sample in a tracklet
+ cfg.video.sample_method = 'evenly' # how to sample images from a tracklet
+ cfg.video.pooling_method = 'avg' # how to pool features over a tracklet
+
+ # train
+ cfg.train = CN()
+ cfg.train.optim = 'adam'
+ cfg.train.lr = 0.0003
+ cfg.train.weight_decay = 5e-4
+ cfg.train.max_epoch = 60
+ cfg.train.start_epoch = 0
+ cfg.train.batch_size = 32
+ cfg.train.fixbase_epoch = 0 # number of epochs to fix base layers
+ cfg.train.open_layers = [
+ 'classifier'
+ ] # layers for training while keeping others frozen
+ cfg.train.staged_lr = False # set different lr to different layers
+ cfg.train.new_layers = ['classifier'] # newly added layers with default lr
+ cfg.train.base_lr_mult = 0.1 # learning rate multiplier for base layers
+ cfg.train.lr_scheduler = 'single_step'
+ cfg.train.stepsize = [20] # stepsize to decay learning rate
+ cfg.train.gamma = 0.1 # learning rate decay multiplier
+ cfg.train.print_freq = 20 # print frequency
+ cfg.train.seed = 1 # random seed
+
+ # optimizer
+ cfg.sgd = CN()
+ cfg.sgd.momentum = 0.9 # momentum factor for sgd and rmsprop
+ cfg.sgd.dampening = 0. # dampening for momentum
+ cfg.sgd.nesterov = False # Nesterov momentum
+ cfg.rmsprop = CN()
+ cfg.rmsprop.alpha = 0.99 # smoothing constant
+ cfg.adam = CN()
+ cfg.adam.beta1 = 0.9 # exponential decay rate for first moment
+ cfg.adam.beta2 = 0.999 # exponential decay rate for second moment
+
+ # loss
+ cfg.loss = CN()
+ cfg.loss.name = 'triplet'
+ cfg.loss.softmax = CN()
+ cfg.loss.softmax.label_smooth = True # use label smoothing regularizer
+ cfg.loss.triplet = CN()
+ cfg.loss.triplet.margin = 0.3 # distance margin
+ cfg.loss.triplet.weight_t = 1. # weight to balance hard triplet loss
+ cfg.loss.triplet.weight_x = 0. # weight to balance cross entropy loss
+ cfg.loss.dml = CN()
+ cfg.loss.dml.weight_ml = 1. # weight for mutual learning loss
+
+ # test
+ cfg.test = CN()
+ cfg.test.batch_size = 100
+ cfg.test.dist_metric = 'euclidean' # distance metric, ['euclidean', 'cosine']
+ cfg.test.normalize_feature = False # normalize feature vectors before computing distance
+ cfg.test.ranks = [1, 5, 10, 20] # cmc ranks
+ cfg.test.evaluate = False # test only
+ cfg.test.eval_freq = -1 # evaluation frequency (-1 means to only test after training)
+ cfg.test.start_eval = 0 # start to evaluate after a specific epoch
+ cfg.test.rerank = False # use person re-ranking
+ cfg.test.visrank = False # visualize ranked results (only available when cfg.test.evaluate=True)
+ cfg.test.visrank_topk = 10 # top-k ranks to visualize
+
+ return cfg
+
+
+def imagedata_kwargs(cfg):
+ return {
+ 'root': cfg.data.root,
+ 'sources': cfg.data.sources,
+ 'targets': cfg.data.targets,
+ 'height': cfg.data.height,
+ 'width': cfg.data.width,
+ 'transforms': cfg.data.transforms,
+ 'norm_mean': cfg.data.norm_mean,
+ 'norm_std': cfg.data.norm_std,
+ 'use_gpu': cfg.use_gpu,
+ 'split_id': cfg.data.split_id,
+ 'combineall': cfg.data.combineall,
+ 'load_train_targets': cfg.data.load_train_targets,
+ 'batch_size_train': cfg.train.batch_size,
+ 'batch_size_test': cfg.test.batch_size,
+ 'workers': cfg.data.workers,
+ 'num_instances': cfg.sampler.num_instances,
+ 'train_sampler': cfg.sampler.train_sampler,
+ # image
+ 'cuhk03_labeled': cfg.cuhk03.labeled_images,
+ 'cuhk03_classic_split': cfg.cuhk03.classic_split,
+ 'market1501_500k': cfg.market1501.use_500k_distractors,
+ }
+
+
+def videodata_kwargs(cfg):
+ return {
+ 'root': cfg.data.root,
+ 'sources': cfg.data.sources,
+ 'targets': cfg.data.targets,
+ 'height': cfg.data.height,
+ 'width': cfg.data.width,
+ 'transforms': cfg.data.transforms,
+ 'norm_mean': cfg.data.norm_mean,
+ 'norm_std': cfg.data.norm_std,
+ 'use_gpu': cfg.use_gpu,
+ 'split_id': cfg.data.split_id,
+ 'combineall': cfg.data.combineall,
+ 'batch_size_train': cfg.train.batch_size,
+ 'batch_size_test': cfg.test.batch_size,
+ 'workers': cfg.data.workers,
+ 'num_instances': cfg.sampler.num_instances,
+ 'train_sampler': cfg.sampler.train_sampler,
+ # video
+ 'seq_len': cfg.video.seq_len,
+ 'sample_method': cfg.video.sample_method
+ }
+
+
+def optimizer_kwargs(cfg):
+ return {
+ 'optim': cfg.train.optim,
+ 'lr': cfg.train.lr,
+ 'weight_decay': cfg.train.weight_decay,
+ 'momentum': cfg.sgd.momentum,
+ 'sgd_dampening': cfg.sgd.dampening,
+ 'sgd_nesterov': cfg.sgd.nesterov,
+ 'rmsprop_alpha': cfg.rmsprop.alpha,
+ 'adam_beta1': cfg.adam.beta1,
+ 'adam_beta2': cfg.adam.beta2,
+ 'staged_lr': cfg.train.staged_lr,
+ 'new_layers': cfg.train.new_layers,
+ 'base_lr_mult': cfg.train.base_lr_mult
+ }
+
+
+def lr_scheduler_kwargs(cfg):
+ return {
+ 'lr_scheduler': cfg.train.lr_scheduler,
+ 'stepsize': cfg.train.stepsize,
+ 'gamma': cfg.train.gamma,
+ 'max_epoch': cfg.train.max_epoch
+ }
+
+
+def engine_run_kwargs(cfg):
+ return {
+ 'save_dir': cfg.data.save_dir,
+ 'max_epoch': cfg.train.max_epoch,
+ 'start_epoch': cfg.train.start_epoch,
+ 'fixbase_epoch': cfg.train.fixbase_epoch,
+ 'open_layers': cfg.train.open_layers,
+ 'start_eval': cfg.test.start_eval,
+ 'eval_freq': cfg.test.eval_freq,
+ 'test_only': cfg.test.evaluate,
+ 'print_freq': cfg.train.print_freq,
+ 'dist_metric': cfg.test.dist_metric,
+ 'normalize_feature': cfg.test.normalize_feature,
+ 'visrank': cfg.test.visrank,
+ 'visrank_topk': cfg.test.visrank_topk,
+ 'use_metric_cuhk03': cfg.cuhk03.use_metric_cuhk03,
+ 'ranks': cfg.test.ranks,
+ 'rerank': cfg.test.rerank
+ }
diff --git a/trackers/strong_sort/deep/reid/projects/DML/dml.py b/trackers/strong_sort/deep/reid/projects/DML/dml.py
new file mode 100644
index 0000000000000000000000000000000000000000..546e573d6ba5d3d41bebbb51062bf9ad451d7344
--- /dev/null
+++ b/trackers/strong_sort/deep/reid/projects/DML/dml.py
@@ -0,0 +1,149 @@
+from __future__ import division, print_function, absolute_import
+import torch
+from torch.nn import functional as F
+
+from torchreid.utils import open_all_layers, open_specified_layers
+from torchreid.engine import Engine
+from torchreid.losses import TripletLoss, CrossEntropyLoss
+
+
+class ImageDMLEngine(Engine):
+
+ def __init__(
+ self,
+ datamanager,
+ model1,
+ optimizer1,
+ scheduler1,
+ model2,
+ optimizer2,
+ scheduler2,
+ margin=0.3,
+ weight_t=0.5,
+ weight_x=1.,
+ weight_ml=1.,
+ use_gpu=True,
+ label_smooth=True,
+ deploy='model1'
+ ):
+ super(ImageDMLEngine, self).__init__(datamanager, use_gpu)
+
+ self.model1 = model1
+ self.optimizer1 = optimizer1
+ self.scheduler1 = scheduler1
+ self.register_model('model1', model1, optimizer1, scheduler1)
+
+ self.model2 = model2
+ self.optimizer2 = optimizer2
+ self.scheduler2 = scheduler2
+ self.register_model('model2', model2, optimizer2, scheduler2)
+
+ self.weight_t = weight_t
+ self.weight_x = weight_x
+ self.weight_ml = weight_ml
+
+ assert deploy in ['model1', 'model2', 'both']
+ self.deploy = deploy
+
+ self.criterion_t = TripletLoss(margin=margin)
+ self.criterion_x = CrossEntropyLoss(
+ num_classes=self.datamanager.num_train_pids,
+ use_gpu=self.use_gpu,
+ label_smooth=label_smooth
+ )
+
+ def forward_backward(self, data):
+ imgs, pids = self.parse_data_for_train(data)
+
+ if self.use_gpu:
+ imgs = imgs.cuda()
+ pids = pids.cuda()
+
+ outputs1, features1 = self.model1(imgs)
+ loss1_x = self.compute_loss(self.criterion_x, outputs1, pids)
+ loss1_t = self.compute_loss(self.criterion_t, features1, pids)
+
+ outputs2, features2 = self.model2(imgs)
+ loss2_x = self.compute_loss(self.criterion_x, outputs2, pids)
+ loss2_t = self.compute_loss(self.criterion_t, features2, pids)
+
+ loss1_ml = self.compute_kl_div(
+ outputs2.detach(), outputs1, is_logit=True
+ )
+ loss2_ml = self.compute_kl_div(
+ outputs1.detach(), outputs2, is_logit=True
+ )
+
+ loss1 = 0
+ loss1 += loss1_x * self.weight_x
+ loss1 += loss1_t * self.weight_t
+ loss1 += loss1_ml * self.weight_ml
+
+ loss2 = 0
+ loss2 += loss2_x * self.weight_x
+ loss2 += loss2_t * self.weight_t
+ loss2 += loss2_ml * self.weight_ml
+
+ self.optimizer1.zero_grad()
+ loss1.backward()
+ self.optimizer1.step()
+
+ self.optimizer2.zero_grad()
+ loss2.backward()
+ self.optimizer2.step()
+
+ loss_dict = {
+ 'loss1_x': loss1_x.item(),
+ 'loss1_t': loss1_t.item(),
+ 'loss1_ml': loss1_ml.item(),
+ 'loss2_x': loss1_x.item(),
+ 'loss2_t': loss1_t.item(),
+ 'loss2_ml': loss1_ml.item()
+ }
+
+ return loss_dict
+
+ @staticmethod
+ def compute_kl_div(p, q, is_logit=True):
+ if is_logit:
+ p = F.softmax(p, dim=1)
+ q = F.softmax(q, dim=1)
+ return -(p * torch.log(q + 1e-8)).sum(1).mean()
+
+ def two_stepped_transfer_learning(
+ self, epoch, fixbase_epoch, open_layers, model=None
+ ):
+ """Two stepped transfer learning.
+
+ The idea is to freeze base layers for a certain number of epochs
+ and then open all layers for training.
+
+ Reference: https://arxiv.org/abs/1611.05244
+ """
+ model1 = self.model1
+ model2 = self.model2
+
+ if (epoch + 1) <= fixbase_epoch and open_layers is not None:
+ print(
+ '* Only train {} (epoch: {}/{})'.format(
+ open_layers, epoch + 1, fixbase_epoch
+ )
+ )
+ open_specified_layers(model1, open_layers)
+ open_specified_layers(model2, open_layers)
+ else:
+ open_all_layers(model1)
+ open_all_layers(model2)
+
+ def extract_features(self, input):
+ if self.deploy == 'model1':
+ return self.model1(input)
+
+ elif self.deploy == 'model2':
+ return self.model2(input)
+
+ else:
+ features = []
+ features.append(self.model1(input))
+ features.append(self.model2(input))
+ return torch.cat(features, 1)
diff --git a/trackers/strong_sort/deep/reid/projects/DML/im_osnet_x1_0_dml_256x128_amsgrad_cosine.yaml b/trackers/strong_sort/deep/reid/projects/DML/im_osnet_x1_0_dml_256x128_amsgrad_cosine.yaml
new file mode 100644
index 0000000000000000000000000000000000000000..3a240b142eae2d124d98166077eb7e572a30be25
--- /dev/null
+++ b/trackers/strong_sort/deep/reid/projects/DML/im_osnet_x1_0_dml_256x128_amsgrad_cosine.yaml
@@ -0,0 +1,42 @@
+model:
+ name: 'osnet_x1_0'
+ pretrained: True
+ deploy: 'model1'
+
+data:
+ type: 'image'
+ sources: ['market1501']
+ targets: ['market1501']
+ height: 256
+ width: 128
+ combineall: False
+ transforms: ['random_flip', 'random_erase']
+ save_dir: 'log/osnet_x1_0_market1501_dml_cosinelr'
+
+loss:
+ name: 'triplet'
+ softmax:
+ label_smooth: True
+ triplet:
+ margin: 0.3
+ weight_t: 0.5
+ weight_x: 1.
+ dml:
+ weight_ml: 1.
+
+train:
+ optim: 'amsgrad'
+ lr: 0.0015
+ max_epoch: 250
+ batch_size: 64
+ fixbase_epoch: 10
+ open_layers: ['classifier']
+ lr_scheduler: 'cosine'
+
+test:
+ batch_size: 300
+ dist_metric: 'cosine'
+ normalize_feature: False
+ evaluate: False
+ eval_freq: -1
+ rerank: False
\ No newline at end of file
diff --git a/trackers/strong_sort/deep/reid/projects/DML/main.py b/trackers/strong_sort/deep/reid/projects/DML/main.py
new file mode 100644
index 0000000000000000000000000000000000000000..9af33d93ba16b80e56b65caeb2ad45f8ad0ac5da
--- /dev/null
+++ b/trackers/strong_sort/deep/reid/projects/DML/main.py
@@ -0,0 +1,166 @@
+import sys
+import copy
+import time
+import os.path as osp
+import argparse
+import torch
+import torch.nn as nn
+
+import torchreid
+from torchreid.utils import (
+ Logger, check_isfile, set_random_seed, collect_env_info,
+ resume_from_checkpoint, load_pretrained_weights, compute_model_complexity
+)
+
+from dml import ImageDMLEngine
+from default_config import (
+ imagedata_kwargs, optimizer_kwargs, engine_run_kwargs, get_default_config,
+ lr_scheduler_kwargs
+)
+
+
+def reset_config(cfg, args):
+ if args.root:
+ cfg.data.root = args.root
+ if args.sources:
+ cfg.data.sources = args.sources
+ if args.targets:
+ cfg.data.targets = args.targets
+ if args.transforms:
+ cfg.data.transforms = args.transforms
+
+
+def main():
+ parser = argparse.ArgumentParser(
+ formatter_class=argparse.ArgumentDefaultsHelpFormatter
+ )
+ parser.add_argument(
+ '--config-file', type=str, default='', help='path to config file'
+ )
+ parser.add_argument(
+ '-s',
+ '--sources',
+ type=str,
+ nargs='+',
+ help='source datasets (delimited by space)'
+ )
+ parser.add_argument(
+ '-t',
+ '--targets',
+ type=str,
+ nargs='+',
+ help='target datasets (delimited by space)'
+ )
+ parser.add_argument(
+ '--transforms', type=str, nargs='+', help='data augmentation'
+ )
+ parser.add_argument(
+ '--root', type=str, default='', help='path to data root'
+ )
+ parser.add_argument(
+ 'opts',
+ default=None,
+ nargs=argparse.REMAINDER,
+ help='Modify config options using the command-line'
+ )
+ args = parser.parse_args()
+
+ cfg = get_default_config()
+ cfg.use_gpu = torch.cuda.is_available()
+ if args.config_file:
+ cfg.merge_from_file(args.config_file)
+ reset_config(cfg, args)
+ cfg.merge_from_list(args.opts)
+ set_random_seed(cfg.train.seed)
+
+ log_name = 'test.log' if cfg.test.evaluate else 'train.log'
+ log_name += time.strftime('-%Y-%m-%d-%H-%M-%S')
+ sys.stdout = Logger(osp.join(cfg.data.save_dir, log_name))
+
+ print('Show configuration\n{}\n'.format(cfg))
+ print('Collecting env info ...')
+ print('** System info **\n{}\n'.format(collect_env_info()))
+
+ if cfg.use_gpu:
+ torch.backends.cudnn.benchmark = True
+
+ datamanager = torchreid.data.ImageDataManager(**imagedata_kwargs(cfg))
+
+ print('Building model-1: {}'.format(cfg.model.name))
+ model1 = torchreid.models.build_model(
+ name=cfg.model.name,
+ num_classes=datamanager.num_train_pids,
+ loss=cfg.loss.name,
+ pretrained=cfg.model.pretrained,
+ use_gpu=cfg.use_gpu
+ )
+ num_params, flops = compute_model_complexity(
+ model1, (1, 3, cfg.data.height, cfg.data.width)
+ )
+ print('Model complexity: params={:,} flops={:,}'.format(num_params, flops))
+
+ print('Copying model-1 to model-2')
+ model2 = copy.deepcopy(model1)
+
+ if cfg.model.load_weights1 and check_isfile(cfg.model.load_weights1):
+ load_pretrained_weights(model1, cfg.model.load_weights1)
+
+ if cfg.model.load_weights2 and check_isfile(cfg.model.load_weights2):
+ load_pretrained_weights(model2, cfg.model.load_weights2)
+
+ if cfg.use_gpu:
+ model1 = nn.DataParallel(model1).cuda()
+ model2 = nn.DataParallel(model2).cuda()
+
+ optimizer1 = torchreid.optim.build_optimizer(
+ model1, **optimizer_kwargs(cfg)
+ )
+ scheduler1 = torchreid.optim.build_lr_scheduler(
+ optimizer1, **lr_scheduler_kwargs(cfg)
+ )
+
+ optimizer2 = torchreid.optim.build_optimizer(
+ model2, **optimizer_kwargs(cfg)
+ )
+ scheduler2 = torchreid.optim.build_lr_scheduler(
+ optimizer2, **lr_scheduler_kwargs(cfg)
+ )
+
+ if cfg.model.resume1 and check_isfile(cfg.model.resume1):
+ cfg.train.start_epoch = resume_from_checkpoint(
+ cfg.model.resume1,
+ model1,
+ optimizer=optimizer1,
+ scheduler=scheduler1
+ )
+
+ if cfg.model.resume2 and check_isfile(cfg.model.resume2):
+ resume_from_checkpoint(
+ cfg.model.resume2,
+ model2,
+ optimizer=optimizer2,
+ scheduler=scheduler2
+ )
+
+ print('Building DML-engine for image-reid')
+ engine = ImageDMLEngine(
+ datamanager,
+ model1,
+ optimizer1,
+ scheduler1,
+ model2,
+ optimizer2,
+ scheduler2,
+ margin=cfg.loss.triplet.margin,
+ weight_t=cfg.loss.triplet.weight_t,
+ weight_x=cfg.loss.triplet.weight_x,
+ weight_ml=cfg.loss.dml.weight_ml,
+ use_gpu=cfg.use_gpu,
+ label_smooth=cfg.loss.softmax.label_smooth,
+ deploy=cfg.model.deploy
+ )
+ engine.run(**engine_run_kwargs(cfg))
+
+
+if __name__ == '__main__':
+ main()
diff --git a/trackers/strong_sort/deep/reid/projects/OSNet_AIN/README.md b/trackers/strong_sort/deep/reid/projects/OSNet_AIN/README.md
new file mode 100644
index 0000000000000000000000000000000000000000..b3131830ef92ebfda98f13e9e3c0d0eac4b8c4a6
--- /dev/null
+++ b/trackers/strong_sort/deep/reid/projects/OSNet_AIN/README.md
@@ -0,0 +1,38 @@
+# Differentiable NAS for OSNet-AIN
+
+## Introduction
+This repository contains the neural architecture search (NAS) code (based on [Torchreid](https://arxiv.org/abs/1910.10093)) for [OSNet-AIN](https://arxiv.org/abs/1910.06827), an extension of [OSNet](https://arxiv.org/abs/1905.00953) that achieves strong performance on cross-domain person re-identification (re-ID) benchmarks (*without using any target data*). OSNet-AIN builds on the idea of using [instance normalisation](https://arxiv.org/abs/1607.08022) (IN) layers to eliminate instance-specific contrast in images for domain-generalisable representation learning. This is inspired by the [neural style transfer](https://arxiv.org/abs/1703.06868) works that use IN to remove image styles. Though IN naturally suits the cross-domain person re-ID task, it still remains unclear that where to insert IN to a re-ID CNN can maximise the performance gain. To avoid exhaustively evaluating all possible designs, OSNet-AIN learns to search for the optimal OSNet+IN design from data using a differentiable NAS algorithm. For technical details, please refer to our paper at https://arxiv.org/abs/1910.06827.
+
+
+
+
+
+## Training
+Assume the reid data is stored at `$DATA`. Run
+```
+python main.py --config-file nas.yaml --root $DATA
+```
+
+The structure of the found architecture will be shown at the end of training.
+
+The default config was designed for 8 Tesla V100 32GB GPUs. You can modify the batch size based on your device memory.
+
+**Note** that the test result obtained at the end of architecture search is not meaningful (due to the stochastic sampling layers). Therefore, do not rely on the result to judge the model performance. Instead, you should construct the found architecture in `osnet_child.py` and re-train and evaluate the model on the reid datasets.
+
+## Citation
+If you find this code useful to your research, please consider citing the following papers.
+```
+@article{zhou2021osnet,
+ title={Learning Generalisable Omni-Scale Representations for Person Re-Identification},
+ author={Zhou, Kaiyang and Yang, Yongxin and Cavallaro, Andrea and Xiang, Tao},
+ journal={TPAMI},
+ year={2021}
+}
+
+@inproceedings{zhou2019osnet,
+ title={Omni-Scale Feature Learning for Person Re-Identification},
+ author={Zhou, Kaiyang and Yang, Yongxin and Cavallaro, Andrea and Xiang, Tao},
+ booktitle={ICCV},
+ year={2019}
+}
+```
\ No newline at end of file
diff --git a/trackers/strong_sort/deep/reid/projects/OSNet_AIN/default_config.py b/trackers/strong_sort/deep/reid/projects/OSNet_AIN/default_config.py
new file mode 100644
index 0000000000000000000000000000000000000000..733a9f026202eca60722963ef735341cd9368650
--- /dev/null
+++ b/trackers/strong_sort/deep/reid/projects/OSNet_AIN/default_config.py
@@ -0,0 +1,210 @@
+from yacs.config import CfgNode as CN
+
+
+def get_default_config():
+ cfg = CN()
+
+ # model
+ cfg.model = CN()
+ cfg.model.name = 'resnet50'
+ cfg.model.pretrained = True # automatically load pretrained model weights if available
+ cfg.model.load_weights = '' # path to model weights
+ cfg.model.resume = '' # path to checkpoint for resume training
+
+ # NAS
+ cfg.nas = CN()
+ cfg.nas.mc_iter = 1 # Monte Carlo sampling
+ cfg.nas.init_lmda = 10. # initial lambda value
+ cfg.nas.min_lmda = 1. # minimum lambda value
+ cfg.nas.lmda_decay_step = 20 # decay step for lambda
+ cfg.nas.lmda_decay_rate = 0.5 # decay rate for lambda
+ cfg.nas.fixed_lmda = False # keep lambda unchanged
+
+ # data
+ cfg.data = CN()
+ cfg.data.type = 'image'
+ cfg.data.root = 'reid-data'
+ cfg.data.sources = ['market1501']
+ cfg.data.targets = ['market1501']
+ cfg.data.workers = 4 # number of data loading workers
+ cfg.data.split_id = 0 # split index
+ cfg.data.height = 256 # image height
+ cfg.data.width = 128 # image width
+ cfg.data.combineall = False # combine train, query and gallery for training
+ cfg.data.transforms = ['random_flip'] # data augmentation
+ cfg.data.norm_mean = [0.485, 0.456, 0.406] # default is imagenet mean
+ cfg.data.norm_std = [0.229, 0.224, 0.225] # default is imagenet std
+ cfg.data.save_dir = 'log' # path to save log
+
+ # specific datasets
+ cfg.market1501 = CN()
+ cfg.market1501.use_500k_distractors = False # add 500k distractors to the gallery set for market1501
+ cfg.cuhk03 = CN()
+ cfg.cuhk03.labeled_images = False # use labeled images, if False, use detected images
+ cfg.cuhk03.classic_split = False # use classic split by Li et al. CVPR14
+ cfg.cuhk03.use_metric_cuhk03 = False # use cuhk03's metric for evaluation
+
+ # sampler
+ cfg.sampler = CN()
+ cfg.sampler.train_sampler = 'RandomSampler'
+ cfg.sampler.num_instances = 4 # number of instances per identity for RandomIdentitySampler
+
+ # video reid setting
+ cfg.video = CN()
+ cfg.video.seq_len = 15 # number of images to sample in a tracklet
+ cfg.video.sample_method = 'evenly' # how to sample images from a tracklet
+ cfg.video.pooling_method = 'avg' # how to pool features over a tracklet
+
+ # train
+ cfg.train = CN()
+ cfg.train.optim = 'adam'
+ cfg.train.lr = 0.0003
+ cfg.train.weight_decay = 5e-4
+ cfg.train.max_epoch = 60
+ cfg.train.start_epoch = 0
+ cfg.train.batch_size = 32
+ cfg.train.fixbase_epoch = 0 # number of epochs to fix base layers
+ cfg.train.open_layers = [
+ 'classifier'
+ ] # layers for training while keeping others frozen
+ cfg.train.staged_lr = False # set different lr to different layers
+ cfg.train.new_layers = ['classifier'] # newly added layers with default lr
+ cfg.train.base_lr_mult = 0.1 # learning rate multiplier for base layers
+ cfg.train.lr_scheduler = 'single_step'
+ cfg.train.stepsize = [20] # stepsize to decay learning rate
+ cfg.train.gamma = 0.1 # learning rate decay multiplier
+ cfg.train.print_freq = 20 # print frequency
+ cfg.train.seed = 1 # random seed
+
+ # optimizer
+ cfg.sgd = CN()
+ cfg.sgd.momentum = 0.9 # momentum factor for sgd and rmsprop
+ cfg.sgd.dampening = 0. # dampening for momentum
+ cfg.sgd.nesterov = False # Nesterov momentum
+ cfg.rmsprop = CN()
+ cfg.rmsprop.alpha = 0.99 # smoothing constant
+ cfg.adam = CN()
+ cfg.adam.beta1 = 0.9 # exponential decay rate for first moment
+ cfg.adam.beta2 = 0.999 # exponential decay rate for second moment
+
+ # loss
+ cfg.loss = CN()
+ cfg.loss.name = 'softmax'
+ cfg.loss.softmax = CN()
+ cfg.loss.softmax.label_smooth = True # use label smoothing regularizer
+ cfg.loss.triplet = CN()
+ cfg.loss.triplet.margin = 0.3 # distance margin
+ cfg.loss.triplet.weight_t = 1. # weight to balance hard triplet loss
+ cfg.loss.triplet.weight_x = 0. # weight to balance cross entropy loss
+
+ # test
+ cfg.test = CN()
+ cfg.test.batch_size = 100
+ cfg.test.dist_metric = 'euclidean' # distance metric, ['euclidean', 'cosine']
+ cfg.test.normalize_feature = False # normalize feature vectors before computing distance
+ cfg.test.ranks = [1, 5, 10, 20] # cmc ranks
+ cfg.test.evaluate = False # test only
+ cfg.test.eval_freq = -1 # evaluation frequency (-1 means to only test after training)
+ cfg.test.start_eval = 0 # start to evaluate after a specific epoch
+ cfg.test.rerank = False # use person re-ranking
+ cfg.test.visrank = False # visualize ranked results (only available when cfg.test.evaluate=True)
+ cfg.test.visrank_topk = 10 # top-k ranks to visualize
+ cfg.test.visactmap = False # visualize CNN activation maps
+
+ return cfg
+
+
+def imagedata_kwargs(cfg):
+ return {
+ 'root': cfg.data.root,
+ 'sources': cfg.data.sources,
+ 'targets': cfg.data.targets,
+ 'height': cfg.data.height,
+ 'width': cfg.data.width,
+ 'transforms': cfg.data.transforms,
+ 'norm_mean': cfg.data.norm_mean,
+ 'norm_std': cfg.data.norm_std,
+ 'use_gpu': cfg.use_gpu,
+ 'split_id': cfg.data.split_id,
+ 'combineall': cfg.data.combineall,
+ 'batch_size_train': cfg.train.batch_size,
+ 'batch_size_test': cfg.test.batch_size,
+ 'workers': cfg.data.workers,
+ 'num_instances': cfg.sampler.num_instances,
+ 'train_sampler': cfg.sampler.train_sampler,
+ # image
+ 'cuhk03_labeled': cfg.cuhk03.labeled_images,
+ 'cuhk03_classic_split': cfg.cuhk03.classic_split,
+ 'market1501_500k': cfg.market1501.use_500k_distractors,
+ }
+
+
+def videodata_kwargs(cfg):
+ return {
+ 'root': cfg.data.root,
+ 'sources': cfg.data.sources,
+ 'targets': cfg.data.targets,
+ 'height': cfg.data.height,
+ 'width': cfg.data.width,
+ 'transforms': cfg.data.transforms,
+ 'norm_mean': cfg.data.norm_mean,
+ 'norm_std': cfg.data.norm_std,
+ 'use_gpu': cfg.use_gpu,
+ 'split_id': cfg.data.split_id,
+ 'combineall': cfg.data.combineall,
+ 'batch_size_train': cfg.train.batch_size,
+ 'batch_size_test': cfg.test.batch_size,
+ 'workers': cfg.data.workers,
+ 'num_instances': cfg.sampler.num_instances,
+ 'train_sampler': cfg.sampler.train_sampler,
+ # video
+ 'seq_len': cfg.video.seq_len,
+ 'sample_method': cfg.video.sample_method
+ }
+
+
+def optimizer_kwargs(cfg):
+ return {
+ 'optim': cfg.train.optim,
+ 'lr': cfg.train.lr,
+ 'weight_decay': cfg.train.weight_decay,
+ 'momentum': cfg.sgd.momentum,
+ 'sgd_dampening': cfg.sgd.dampening,
+ 'sgd_nesterov': cfg.sgd.nesterov,
+ 'rmsprop_alpha': cfg.rmsprop.alpha,
+ 'adam_beta1': cfg.adam.beta1,
+ 'adam_beta2': cfg.adam.beta2,
+ 'staged_lr': cfg.train.staged_lr,
+ 'new_layers': cfg.train.new_layers,
+ 'base_lr_mult': cfg.train.base_lr_mult
+ }
+
+
+def lr_scheduler_kwargs(cfg):
+ return {
+ 'lr_scheduler': cfg.train.lr_scheduler,
+ 'stepsize': cfg.train.stepsize,
+ 'gamma': cfg.train.gamma,
+ 'max_epoch': cfg.train.max_epoch
+ }
+
+
+def engine_run_kwargs(cfg):
+ return {
+ 'save_dir': cfg.data.save_dir,
+ 'max_epoch': cfg.train.max_epoch,
+ 'start_epoch': cfg.train.start_epoch,
+ 'fixbase_epoch': cfg.train.fixbase_epoch,
+ 'open_layers': cfg.train.open_layers,
+ 'start_eval': cfg.test.start_eval,
+ 'eval_freq': cfg.test.eval_freq,
+ 'test_only': cfg.test.evaluate,
+ 'print_freq': cfg.train.print_freq,
+ 'dist_metric': cfg.test.dist_metric,
+ 'normalize_feature': cfg.test.normalize_feature,
+ 'visrank': cfg.test.visrank,
+ 'visrank_topk': cfg.test.visrank_topk,
+ 'use_metric_cuhk03': cfg.cuhk03.use_metric_cuhk03,
+ 'ranks': cfg.test.ranks,
+ 'rerank': cfg.test.rerank
+ }
diff --git a/trackers/strong_sort/deep/reid/projects/OSNet_AIN/main.py b/trackers/strong_sort/deep/reid/projects/OSNet_AIN/main.py
new file mode 100644
index 0000000000000000000000000000000000000000..f59177073dfafe1b4b712691c780af32b30fcd2c
--- /dev/null
+++ b/trackers/strong_sort/deep/reid/projects/OSNet_AIN/main.py
@@ -0,0 +1,145 @@
+import os
+import sys
+import time
+import os.path as osp
+import argparse
+import torch
+import torch.nn as nn
+
+import torchreid
+from torchreid.utils import (
+ Logger, check_isfile, set_random_seed, collect_env_info,
+ resume_from_checkpoint, compute_model_complexity
+)
+
+import osnet_search as osnet_models
+from softmax_nas import ImageSoftmaxNASEngine
+from default_config import (
+ imagedata_kwargs, optimizer_kwargs, engine_run_kwargs, get_default_config,
+ lr_scheduler_kwargs
+)
+
+
+def reset_config(cfg, args):
+ if args.root:
+ cfg.data.root = args.root
+ if args.sources:
+ cfg.data.sources = args.sources
+ if args.targets:
+ cfg.data.targets = args.targets
+ if args.transforms:
+ cfg.data.transforms = args.transforms
+
+
+def main():
+ parser = argparse.ArgumentParser(
+ formatter_class=argparse.ArgumentDefaultsHelpFormatter
+ )
+ parser.add_argument(
+ '--config-file', type=str, default='', help='path to config file'
+ )
+ parser.add_argument(
+ '-s',
+ '--sources',
+ type=str,
+ nargs='+',
+ help='source datasets (delimited by space)'
+ )
+ parser.add_argument(
+ '-t',
+ '--targets',
+ type=str,
+ nargs='+',
+ help='target datasets (delimited by space)'
+ )
+ parser.add_argument(
+ '--transforms', type=str, nargs='+', help='data augmentation'
+ )
+ parser.add_argument(
+ '--root', type=str, default='', help='path to data root'
+ )
+ parser.add_argument(
+ '--gpu-devices',
+ type=str,
+ default='',
+ )
+ parser.add_argument(
+ 'opts',
+ default=None,
+ nargs=argparse.REMAINDER,
+ help='Modify config options using the command-line'
+ )
+ args = parser.parse_args()
+
+ cfg = get_default_config()
+ cfg.use_gpu = torch.cuda.is_available()
+ if args.config_file:
+ cfg.merge_from_file(args.config_file)
+ reset_config(cfg, args)
+ cfg.merge_from_list(args.opts)
+ set_random_seed(cfg.train.seed)
+
+ if cfg.use_gpu and args.gpu_devices:
+ # if gpu_devices is not specified, all available gpus will be used
+ os.environ['CUDA_VISIBLE_DEVICES'] = args.gpu_devices
+ log_name = 'test.log' if cfg.test.evaluate else 'train.log'
+ log_name += time.strftime('-%Y-%m-%d-%H-%M-%S')
+ sys.stdout = Logger(osp.join(cfg.data.save_dir, log_name))
+
+ print('Show configuration\n{}\n'.format(cfg))
+ print('Collecting env info ...')
+ print('** System info **\n{}\n'.format(collect_env_info()))
+
+ if cfg.use_gpu:
+ torch.backends.cudnn.benchmark = True
+
+ datamanager = torchreid.data.ImageDataManager(**imagedata_kwargs(cfg))
+
+ print('Building model: {}'.format(cfg.model.name))
+ model = osnet_models.build_model(
+ cfg.model.name, num_classes=datamanager.num_train_pids
+ )
+ num_params, flops = compute_model_complexity(
+ model, (1, 3, cfg.data.height, cfg.data.width)
+ )
+ print('Model complexity: params={:,} flops={:,}'.format(num_params, flops))
+
+ if cfg.use_gpu:
+ model = nn.DataParallel(model).cuda()
+
+ optimizer = torchreid.optim.build_optimizer(model, **optimizer_kwargs(cfg))
+ scheduler = torchreid.optim.build_lr_scheduler(
+ optimizer, **lr_scheduler_kwargs(cfg)
+ )
+
+ if cfg.model.resume and check_isfile(cfg.model.resume):
+ cfg.train.start_epoch = resume_from_checkpoint(
+ cfg.model.resume, model, optimizer=optimizer
+ )
+
+ print('Building NAS engine')
+ engine = ImageSoftmaxNASEngine(
+ datamanager,
+ model,
+ optimizer,
+ scheduler=scheduler,
+ use_gpu=cfg.use_gpu,
+ label_smooth=cfg.loss.softmax.label_smooth,
+ mc_iter=cfg.nas.mc_iter,
+ init_lmda=cfg.nas.init_lmda,
+ min_lmda=cfg.nas.min_lmda,
+ lmda_decay_step=cfg.nas.lmda_decay_step,
+ lmda_decay_rate=cfg.nas.lmda_decay_rate,
+ fixed_lmda=cfg.nas.fixed_lmda
+ )
+ engine.run(**engine_run_kwargs(cfg))
+
+ print('*** Display the found architecture ***')
+ if cfg.use_gpu:
+ model.module.build_child_graph()
+ else:
+ model.build_child_graph()
+
+
+if __name__ == '__main__':
+ main()
diff --git a/trackers/strong_sort/deep/reid/projects/OSNet_AIN/nas.yaml b/trackers/strong_sort/deep/reid/projects/OSNet_AIN/nas.yaml
new file mode 100644
index 0000000000000000000000000000000000000000..507b2ecfe44e8a7ab54d74c74aef808fea1a4951
--- /dev/null
+++ b/trackers/strong_sort/deep/reid/projects/OSNet_AIN/nas.yaml
@@ -0,0 +1,44 @@
+model:
+ name: 'osnet_nas'
+ pretrained: False
+
+nas:
+ mc_iter: 1
+ init_lmda: 10.
+ min_lmda: 1.
+ lmda_decay_step: 20
+ lmda_decay_rate: 0.5
+ fixed_lmda: False
+
+data:
+ type: 'image'
+ sources: ['msmt17']
+ targets: ['market1501']
+ height: 256
+ width: 128
+ combineall: True
+ transforms: ['random_flip', 'color_jitter']
+ save_dir: 'log/osnet_nas'
+
+loss:
+ name: 'softmax'
+ softmax:
+ label_smooth: True
+
+train:
+ optim: 'sgd'
+ lr: 0.1
+ max_epoch: 120
+ batch_size: 512
+ fixbase_epoch: 0
+ open_layers: ['classifier']
+ lr_scheduler: 'cosine'
+
+test:
+ batch_size: 300
+ dist_metric: 'cosine'
+ normalize_feature: False
+ evaluate: False
+ eval_freq: -1
+ rerank: False
+ visactmap: False
\ No newline at end of file
diff --git a/trackers/strong_sort/deep/reid/projects/OSNet_AIN/osnet_child.py b/trackers/strong_sort/deep/reid/projects/OSNet_AIN/osnet_child.py
new file mode 100644
index 0000000000000000000000000000000000000000..b47d747d03d90748fff902e67e49e05a65b82d0c
--- /dev/null
+++ b/trackers/strong_sort/deep/reid/projects/OSNet_AIN/osnet_child.py
@@ -0,0 +1,535 @@
+from __future__ import division, absolute_import
+from torch import nn
+from torch.nn import functional as F
+
+
+##########
+# Basic layers
+##########
+class ConvLayer(nn.Module):
+ """Convolution layer (conv + bn + relu)."""
+
+ def __init__(
+ self,
+ in_channels,
+ out_channels,
+ kernel_size,
+ stride=1,
+ padding=0,
+ groups=1,
+ IN=False
+ ):
+ super(ConvLayer, self).__init__()
+ self.conv = nn.Conv2d(
+ in_channels,
+ out_channels,
+ kernel_size,
+ stride=stride,
+ padding=padding,
+ bias=False,
+ groups=groups
+ )
+ if IN:
+ self.bn = nn.InstanceNorm2d(out_channels, affine=True)
+ else:
+ self.bn = nn.BatchNorm2d(out_channels)
+ self.relu = nn.ReLU(inplace=True)
+
+ def forward(self, x):
+ x = self.conv(x)
+ x = self.bn(x)
+ return self.relu(x)
+
+
+class Conv1x1(nn.Module):
+ """1x1 convolution + bn + relu."""
+
+ def __init__(self, in_channels, out_channels, stride=1, groups=1):
+ super(Conv1x1, self).__init__()
+ self.conv = nn.Conv2d(
+ in_channels,
+ out_channels,
+ 1,
+ stride=stride,
+ padding=0,
+ bias=False,
+ groups=groups
+ )
+ self.bn = nn.BatchNorm2d(out_channels)
+ self.relu = nn.ReLU(inplace=True)
+
+ def forward(self, x):
+ x = self.conv(x)
+ x = self.bn(x)
+ return self.relu(x)
+
+
+class Conv1x1Linear(nn.Module):
+ """1x1 convolution + bn (w/o non-linearity)."""
+
+ def __init__(self, in_channels, out_channels, stride=1, bn=True):
+ super(Conv1x1Linear, self).__init__()
+ self.conv = nn.Conv2d(
+ in_channels, out_channels, 1, stride=stride, padding=0, bias=False
+ )
+ self.bn = None
+ if bn:
+ self.bn = nn.BatchNorm2d(out_channels)
+
+ def forward(self, x):
+ x = self.conv(x)
+ if self.bn is not None:
+ x = self.bn(x)
+ return x
+
+
+class Conv3x3(nn.Module):
+ """3x3 convolution + bn + relu."""
+
+ def __init__(self, in_channels, out_channels, stride=1, groups=1):
+ super(Conv3x3, self).__init__()
+ self.conv = nn.Conv2d(
+ in_channels,
+ out_channels,
+ 3,
+ stride=stride,
+ padding=1,
+ bias=False,
+ groups=groups
+ )
+ self.bn = nn.BatchNorm2d(out_channels)
+ self.relu = nn.ReLU(inplace=True)
+
+ def forward(self, x):
+ x = self.conv(x)
+ x = self.bn(x)
+ return self.relu(x)
+
+
+class LightConv3x3(nn.Module):
+ """Lightweight 3x3 convolution.
+
+ 1x1 (linear) + dw 3x3 (nonlinear).
+ """
+
+ def __init__(self, in_channels, out_channels):
+ super(LightConv3x3, self).__init__()
+ self.conv1 = nn.Conv2d(
+ in_channels, out_channels, 1, stride=1, padding=0, bias=False
+ )
+ self.conv2 = nn.Conv2d(
+ out_channels,
+ out_channels,
+ 3,
+ stride=1,
+ padding=1,
+ bias=False,
+ groups=out_channels
+ )
+ self.bn = nn.BatchNorm2d(out_channels)
+ self.relu = nn.ReLU(inplace=True)
+
+ def forward(self, x):
+ x = self.conv1(x)
+ x = self.conv2(x)
+ x = self.bn(x)
+ return self.relu(x)
+
+
+class LightConvStream(nn.Module):
+ """Lightweight convolution stream."""
+
+ def __init__(self, in_channels, out_channels, depth):
+ super(LightConvStream, self).__init__()
+ assert depth >= 1, 'depth must be equal to or larger than 1, but got {}'.format(
+ depth
+ )
+ layers = []
+ layers += [LightConv3x3(in_channels, out_channels)]
+ for i in range(depth - 1):
+ layers += [LightConv3x3(out_channels, out_channels)]
+ self.layers = nn.Sequential(*layers)
+
+ def forward(self, x):
+ return self.layers(x)
+
+
+##########
+# Building blocks for omni-scale feature learning
+##########
+class ChannelGate(nn.Module):
+ """A mini-network that generates channel-wise gates conditioned on input tensor."""
+
+ def __init__(
+ self,
+ in_channels,
+ num_gates=None,
+ return_gates=False,
+ gate_activation='sigmoid',
+ reduction=16,
+ layer_norm=False
+ ):
+ super(ChannelGate, self).__init__()
+ if num_gates is None:
+ num_gates = in_channels
+ self.return_gates = return_gates
+ self.global_avgpool = nn.AdaptiveAvgPool2d(1)
+ self.fc1 = nn.Conv2d(
+ in_channels,
+ in_channels // reduction,
+ kernel_size=1,
+ bias=True,
+ padding=0
+ )
+ self.norm1 = None
+ if layer_norm:
+ self.norm1 = nn.LayerNorm((in_channels // reduction, 1, 1))
+ self.relu = nn.ReLU(inplace=True)
+ self.fc2 = nn.Conv2d(
+ in_channels // reduction,
+ num_gates,
+ kernel_size=1,
+ bias=True,
+ padding=0
+ )
+ if gate_activation == 'sigmoid':
+ self.gate_activation = nn.Sigmoid()
+ elif gate_activation == 'relu':
+ self.gate_activation = nn.ReLU(inplace=True)
+ elif gate_activation == 'linear':
+ self.gate_activation = None
+ else:
+ raise RuntimeError(
+ "Unknown gate activation: {}".format(gate_activation)
+ )
+
+ def forward(self, x):
+ input = x
+ x = self.global_avgpool(x)
+ x = self.fc1(x)
+ if self.norm1 is not None:
+ x = self.norm1(x)
+ x = self.relu(x)
+ x = self.fc2(x)
+ if self.gate_activation is not None:
+ x = self.gate_activation(x)
+ if self.return_gates:
+ return x
+ return input * x
+
+
+class OSBlock(nn.Module):
+ """Omni-scale feature learning block."""
+
+ def __init__(self, in_channels, out_channels, reduction=4, T=4, **kwargs):
+ super(OSBlock, self).__init__()
+ assert T >= 1
+ assert out_channels >= reduction and out_channels % reduction == 0
+ mid_channels = out_channels // reduction
+
+ self.conv1 = Conv1x1(in_channels, mid_channels)
+ self.conv2 = nn.ModuleList()
+ for t in range(1, T + 1):
+ self.conv2 += [LightConvStream(mid_channels, mid_channels, t)]
+ self.gate = ChannelGate(mid_channels)
+ self.conv3 = Conv1x1Linear(mid_channels, out_channels)
+ self.downsample = None
+ if in_channels != out_channels:
+ self.downsample = Conv1x1Linear(in_channels, out_channels)
+
+ def forward(self, x):
+ identity = x
+ x1 = self.conv1(x)
+ x2 = 0
+ for conv2_t in self.conv2:
+ x2_t = conv2_t(x1)
+ x2 = x2 + self.gate(x2_t)
+ x3 = self.conv3(x2)
+ if self.downsample is not None:
+ identity = self.downsample(identity)
+ out = x3 + identity
+ return F.relu(out)
+
+
+class OSBlockINv1(nn.Module):
+ """Omni-scale feature learning block with instance normalization."""
+
+ def __init__(self, in_channels, out_channels, reduction=4, T=4, **kwargs):
+ super(OSBlockINv1, self).__init__()
+ assert T >= 1
+ assert out_channels >= reduction and out_channels % reduction == 0
+ mid_channels = out_channels // reduction
+
+ self.conv1 = Conv1x1(in_channels, mid_channels)
+ self.conv2 = nn.ModuleList()
+ for t in range(1, T + 1):
+ self.conv2 += [LightConvStream(mid_channels, mid_channels, t)]
+ self.gate = ChannelGate(mid_channels)
+ self.conv3 = Conv1x1Linear(mid_channels, out_channels, bn=False)
+ self.downsample = None
+ if in_channels != out_channels:
+ self.downsample = Conv1x1Linear(in_channels, out_channels)
+ self.IN = nn.InstanceNorm2d(out_channels, affine=True)
+
+ def forward(self, x):
+ identity = x
+ x1 = self.conv1(x)
+ x2 = 0
+ for conv2_t in self.conv2:
+ x2_t = conv2_t(x1)
+ x2 = x2 + self.gate(x2_t)
+ x3 = self.conv3(x2)
+ x3 = self.IN(x3) # IN inside residual
+ if self.downsample is not None:
+ identity = self.downsample(identity)
+ out = x3 + identity
+ return F.relu(out)
+
+
+class OSBlockINv2(nn.Module):
+ """Omni-scale feature learning block with instance normalization."""
+
+ def __init__(self, in_channels, out_channels, reduction=4, T=4, **kwargs):
+ super(OSBlockINv2, self).__init__()
+ assert T >= 1
+ assert out_channels >= reduction and out_channels % reduction == 0
+ mid_channels = out_channels // reduction
+
+ self.conv1 = Conv1x1(in_channels, mid_channels)
+ self.conv2 = nn.ModuleList()
+ for t in range(1, T + 1):
+ self.conv2 += [LightConvStream(mid_channels, mid_channels, t)]
+ self.gate = ChannelGate(mid_channels)
+ self.conv3 = Conv1x1Linear(mid_channels, out_channels)
+ self.downsample = None
+ if in_channels != out_channels:
+ self.downsample = Conv1x1Linear(in_channels, out_channels)
+ self.IN = nn.InstanceNorm2d(out_channels, affine=True)
+
+ def forward(self, x):
+ identity = x
+ x1 = self.conv1(x)
+ x2 = 0
+ for conv2_t in self.conv2:
+ x2_t = conv2_t(x1)
+ x2 = x2 + self.gate(x2_t)
+ x3 = self.conv3(x2)
+ if self.downsample is not None:
+ identity = self.downsample(identity)
+ out = x3 + identity
+ out = self.IN(out) # IN outside residual
+ return F.relu(out)
+
+
+class OSBlockINv3(nn.Module):
+ """Omni-scale feature learning block with instance normalization."""
+
+ def __init__(self, in_channels, out_channels, reduction=4, T=4, **kwargs):
+ super(OSBlockINv3, self).__init__()
+ assert T >= 1
+ assert out_channels >= reduction and out_channels % reduction == 0
+ mid_channels = out_channels // reduction
+
+ self.conv1 = Conv1x1(in_channels, mid_channels)
+ self.conv2 = nn.ModuleList()
+ for t in range(1, T + 1):
+ self.conv2 += [LightConvStream(mid_channels, mid_channels, t)]
+ self.gate = ChannelGate(mid_channels)
+ self.conv3 = Conv1x1Linear(mid_channels, out_channels, bn=False)
+ self.downsample = None
+ if in_channels != out_channels:
+ self.downsample = Conv1x1Linear(in_channels, out_channels)
+ self.IN_in = nn.InstanceNorm2d(out_channels, affine=True)
+ self.IN_out = nn.InstanceNorm2d(out_channels, affine=True)
+
+ def forward(self, x):
+ identity = x
+ x1 = self.conv1(x)
+ x2 = 0
+ for conv2_t in self.conv2:
+ x2_t = conv2_t(x1)
+ x2 = x2 + self.gate(x2_t)
+ x3 = self.conv3(x2)
+ x3 = self.IN_in(x3) # IN inside residual
+ if self.downsample is not None:
+ identity = self.downsample(identity)
+ out = x3 + identity
+ out = self.IN_out(out) # IN outside residual
+ return F.relu(out)
+
+
+##########
+# Network architecture
+##########
+class OSNet(nn.Module):
+ """Omni-Scale Network.
+
+ Reference:
+ - Zhou et al. Omni-Scale Feature Learning for Person Re-Identification. ICCV, 2019.
+ - Zhou et al. Learning Generalisable Omni-Scale Representations
+ for Person Re-Identification. TPAMI, 2021.
+ """
+
+ def __init__(
+ self,
+ num_classes,
+ blocks,
+ layers,
+ channels,
+ feature_dim=512,
+ loss='softmax',
+ conv1_IN=True,
+ **kwargs
+ ):
+ super(OSNet, self).__init__()
+ num_blocks = len(blocks)
+ assert num_blocks == len(layers)
+ assert num_blocks == len(channels) - 1
+ self.loss = loss
+ self.feature_dim = feature_dim
+
+ # convolutional backbone
+ self.conv1 = ConvLayer(
+ 3, channels[0], 7, stride=2, padding=3, IN=conv1_IN
+ )
+ self.maxpool = nn.MaxPool2d(3, stride=2, padding=1)
+ self.conv2 = self._make_layer(
+ blocks[0], layers[0], channels[0], channels[1]
+ )
+ self.pool2 = nn.Sequential(
+ Conv1x1(channels[1], channels[1]), nn.AvgPool2d(2, stride=2)
+ )
+ self.conv3 = self._make_layer(
+ blocks[1], layers[1], channels[1], channels[2]
+ )
+ self.pool3 = nn.Sequential(
+ Conv1x1(channels[2], channels[2]), nn.AvgPool2d(2, stride=2)
+ )
+ self.conv4 = self._make_layer(
+ blocks[2], layers[2], channels[2], channels[3]
+ )
+ self.conv5 = Conv1x1(channels[3], channels[3])
+ self.global_avgpool = nn.AdaptiveAvgPool2d(1)
+ # fully connected layer
+ self.fc = self._construct_fc_layer(
+ self.feature_dim, channels[3], dropout_p=None
+ )
+ # identity classification layer
+ self.classifier = nn.Linear(self.feature_dim, num_classes)
+
+ self._init_params()
+
+ def _make_layer(self, blocks, layer, in_channels, out_channels):
+ layers = []
+ layers += [blocks[0](in_channels, out_channels)]
+ for i in range(1, len(blocks)):
+ layers += [blocks[i](out_channels, out_channels)]
+ return nn.Sequential(*layers)
+
+ def _construct_fc_layer(self, fc_dims, input_dim, dropout_p=None):
+ if fc_dims is None or fc_dims < 0:
+ self.feature_dim = input_dim
+ return None
+
+ if isinstance(fc_dims, int):
+ fc_dims = [fc_dims]
+
+ layers = []
+ for dim in fc_dims:
+ layers.append(nn.Linear(input_dim, dim))
+ layers.append(nn.BatchNorm1d(dim))
+ layers.append(nn.ReLU(inplace=True))
+ if dropout_p is not None:
+ layers.append(nn.Dropout(p=dropout_p))
+ input_dim = dim
+
+ self.feature_dim = fc_dims[-1]
+
+ return nn.Sequential(*layers)
+
+ def _init_params(self):
+ for m in self.modules():
+ if isinstance(m, nn.Conv2d):
+ nn.init.kaiming_normal_(
+ m.weight, mode='fan_out', nonlinearity='relu'
+ )
+ if m.bias is not None:
+ nn.init.constant_(m.bias, 0)
+
+ elif isinstance(m, nn.BatchNorm2d):
+ nn.init.constant_(m.weight, 1)
+ nn.init.constant_(m.bias, 0)
+
+ elif isinstance(m, nn.BatchNorm1d):
+ nn.init.constant_(m.weight, 1)
+ nn.init.constant_(m.bias, 0)
+
+ elif isinstance(m, nn.InstanceNorm2d):
+ nn.init.constant_(m.weight, 1)
+ nn.init.constant_(m.bias, 0)
+
+ elif isinstance(m, nn.Linear):
+ nn.init.normal_(m.weight, 0, 0.01)
+ if m.bias is not None:
+ nn.init.constant_(m.bias, 0)
+
+ def featuremaps(self, x):
+ x = self.conv1(x)
+ x = self.maxpool(x)
+ x = self.conv2(x)
+ x = self.pool2(x)
+ x = self.conv3(x)
+ x = self.pool3(x)
+ x = self.conv4(x)
+ return self.conv5(x)
+
+ def forward(self, x, return_featuremaps=False, **kwargs):
+ x = self.featuremaps(x)
+ if return_featuremaps:
+ return x
+ v = self.global_avgpool(x)
+ v = v.view(v.size(0), -1)
+ if self.fc is not None:
+ v = self.fc(v)
+ if not self.training:
+ return v
+ y = self.classifier(v)
+ if self.loss == 'softmax':
+ return y
+ elif self.loss == 'triplet':
+ return y, v
+ else:
+ raise KeyError("Unsupported loss: {}".format(self.loss))
+
+
+##########
+# Instantiation
+##########
+def osnet_ain_x1_0(
+ num_classes=1000, pretrained=True, loss='softmax', **kwargs
+):
+ model = OSNet(
+ num_classes,
+ blocks=[
+ [OSBlockINv1, OSBlockINv1], [OSBlock, OSBlockINv1],
+ [OSBlockINv1, OSBlock]
+ ],
+ layers=[2, 2, 2],
+ channels=[64, 256, 384, 512],
+ loss=loss,
+ conv1_IN=True,
+ **kwargs
+ )
+ return model
+
+
+__models = {'osnet_ain_x1_0': osnet_ain_x1_0}
+
+
+def build_model(name, num_classes=100):
+ avai_models = list(__models.keys())
+ if name not in avai_models:
+ raise KeyError(
+ 'Unknown model: {}. Must be one of {}'.format(name, avai_models)
+ )
+ return __models[name](num_classes=num_classes)
diff --git a/trackers/strong_sort/deep/reid/projects/OSNet_AIN/osnet_search.py b/trackers/strong_sort/deep/reid/projects/OSNet_AIN/osnet_search.py
new file mode 100644
index 0000000000000000000000000000000000000000..182014489ab013bdf44a1cd213ce369deee76478
--- /dev/null
+++ b/trackers/strong_sort/deep/reid/projects/OSNet_AIN/osnet_search.py
@@ -0,0 +1,584 @@
+from __future__ import division, absolute_import
+import torch
+from torch import nn
+from torch.nn import functional as F
+
+EPS = 1e-12
+NORM_AFFINE = False # enable affine transformations for normalization layer
+
+
+##########
+# Basic layers
+##########
+class IBN(nn.Module):
+ """Instance + Batch Normalization."""
+
+ def __init__(self, num_channels):
+ super(IBN, self).__init__()
+ half1 = int(num_channels / 2)
+ self.half = half1
+ half2 = num_channels - half1
+ self.IN = nn.InstanceNorm2d(half1, affine=NORM_AFFINE)
+ self.BN = nn.BatchNorm2d(half2, affine=NORM_AFFINE)
+
+ def forward(self, x):
+ split = torch.split(x, self.half, 1)
+ out1 = self.IN(split[0].contiguous())
+ out2 = self.BN(split[1].contiguous())
+ return torch.cat((out1, out2), 1)
+
+
+class ConvLayer(nn.Module):
+ """Convolution layer (conv + bn + relu)."""
+
+ def __init__(
+ self,
+ in_channels,
+ out_channels,
+ kernel_size,
+ stride=1,
+ padding=0,
+ groups=1,
+ IN=False
+ ):
+ super(ConvLayer, self).__init__()
+ self.conv = nn.Conv2d(
+ in_channels,
+ out_channels,
+ kernel_size,
+ stride=stride,
+ padding=padding,
+ bias=False,
+ groups=groups
+ )
+ if IN:
+ self.bn = nn.InstanceNorm2d(out_channels, affine=NORM_AFFINE)
+ else:
+ self.bn = nn.BatchNorm2d(out_channels, affine=NORM_AFFINE)
+ self.relu = nn.ReLU(inplace=True)
+
+ def forward(self, x):
+ x = self.conv(x)
+ x = self.bn(x)
+ return self.relu(x)
+
+
+class Conv1x1(nn.Module):
+ """1x1 convolution + bn + relu."""
+
+ def __init__(
+ self, in_channels, out_channels, stride=1, groups=1, ibn=False
+ ):
+ super(Conv1x1, self).__init__()
+ self.conv = nn.Conv2d(
+ in_channels,
+ out_channels,
+ 1,
+ stride=stride,
+ padding=0,
+ bias=False,
+ groups=groups
+ )
+ if ibn:
+ self.bn = IBN(out_channels)
+ else:
+ self.bn = nn.BatchNorm2d(out_channels, affine=NORM_AFFINE)
+ self.relu = nn.ReLU(inplace=True)
+
+ def forward(self, x):
+ x = self.conv(x)
+ x = self.bn(x)
+ return self.relu(x)
+
+
+class Conv1x1Linear(nn.Module):
+ """1x1 convolution + bn (w/o non-linearity)."""
+
+ def __init__(self, in_channels, out_channels, stride=1, bn=True):
+ super(Conv1x1Linear, self).__init__()
+ self.conv = nn.Conv2d(
+ in_channels, out_channels, 1, stride=stride, padding=0, bias=False
+ )
+ self.bn = None
+ if bn:
+ self.bn = nn.BatchNorm2d(out_channels, affine=NORM_AFFINE)
+
+ def forward(self, x):
+ x = self.conv(x)
+ if self.bn is not None:
+ x = self.bn(x)
+ return x
+
+
+class Conv3x3(nn.Module):
+ """3x3 convolution + bn + relu."""
+
+ def __init__(self, in_channels, out_channels, stride=1, groups=1):
+ super(Conv3x3, self).__init__()
+ self.conv = nn.Conv2d(
+ in_channels,
+ out_channels,
+ 3,
+ stride=stride,
+ padding=1,
+ bias=False,
+ groups=groups
+ )
+ self.bn = nn.BatchNorm2d(out_channels, affine=NORM_AFFINE)
+ self.relu = nn.ReLU(inplace=True)
+
+ def forward(self, x):
+ x = self.conv(x)
+ x = self.bn(x)
+ return self.relu(x)
+
+
+class LightConv3x3(nn.Module):
+ """Lightweight 3x3 convolution.
+
+ 1x1 (linear) + dw 3x3 (nonlinear).
+ """
+
+ def __init__(self, in_channels, out_channels):
+ super(LightConv3x3, self).__init__()
+ self.conv1 = nn.Conv2d(
+ in_channels, out_channels, 1, stride=1, padding=0, bias=False
+ )
+ self.conv2 = nn.Conv2d(
+ out_channels,
+ out_channels,
+ 3,
+ stride=1,
+ padding=1,
+ bias=False,
+ groups=out_channels
+ )
+ self.bn = nn.BatchNorm2d(out_channels, affine=NORM_AFFINE)
+ self.relu = nn.ReLU(inplace=True)
+
+ def forward(self, x):
+ x = self.conv1(x)
+ x = self.conv2(x)
+ x = self.bn(x)
+ return self.relu(x)
+
+
+class LightConvStream(nn.Module):
+ """Lightweight convolution stream."""
+
+ def __init__(self, in_channels, out_channels, depth):
+ super(LightConvStream, self).__init__()
+ assert depth >= 1, 'depth must be equal to or larger than 1, but got {}'.format(
+ depth
+ )
+ layers = []
+ layers += [LightConv3x3(in_channels, out_channels)]
+ for i in range(depth - 1):
+ layers += [LightConv3x3(out_channels, out_channels)]
+ self.layers = nn.Sequential(*layers)
+
+ def forward(self, x):
+ return self.layers(x)
+
+
+##########
+# Building blocks for omni-scale feature learning
+##########
+class ChannelGate(nn.Module):
+ """A mini-network that generates channel-wise gates conditioned on input tensor."""
+
+ def __init__(
+ self,
+ in_channels,
+ num_gates=None,
+ return_gates=False,
+ gate_activation='sigmoid',
+ reduction=16,
+ layer_norm=False
+ ):
+ super(ChannelGate, self).__init__()
+ if num_gates is None:
+ num_gates = in_channels
+ self.return_gates = return_gates
+ self.global_avgpool = nn.AdaptiveAvgPool2d(1)
+ self.fc1 = nn.Conv2d(
+ in_channels,
+ in_channels // reduction,
+ kernel_size=1,
+ bias=True,
+ padding=0
+ )
+ self.norm1 = None
+ if layer_norm:
+ self.norm1 = nn.LayerNorm((in_channels // reduction, 1, 1))
+ self.relu = nn.ReLU(inplace=True)
+ self.fc2 = nn.Conv2d(
+ in_channels // reduction,
+ num_gates,
+ kernel_size=1,
+ bias=True,
+ padding=0
+ )
+ if gate_activation == 'sigmoid':
+ self.gate_activation = nn.Sigmoid()
+ elif gate_activation == 'relu':
+ self.gate_activation = nn.ReLU(inplace=True)
+ elif gate_activation == 'linear':
+ self.gate_activation = None
+ else:
+ raise RuntimeError(
+ "Unknown gate activation: {}".format(gate_activation)
+ )
+
+ def forward(self, x):
+ input = x
+ x = self.global_avgpool(x)
+ x = self.fc1(x)
+ if self.norm1 is not None:
+ x = self.norm1(x)
+ x = self.relu(x)
+ x = self.fc2(x)
+ if self.gate_activation is not None:
+ x = self.gate_activation(x)
+ if self.return_gates:
+ return x
+ return input * x
+
+
+class OSBlock(nn.Module):
+ """Omni-scale feature learning block."""
+
+ def __init__(self, in_channels, out_channels, reduction=4, T=4, **kwargs):
+ super(OSBlock, self).__init__()
+ assert T >= 1
+ assert out_channels >= reduction and out_channels % reduction == 0
+ mid_channels = out_channels // reduction
+
+ self.conv1 = Conv1x1(in_channels, mid_channels)
+ self.conv2 = nn.ModuleList()
+ for t in range(1, T + 1):
+ self.conv2 += [LightConvStream(mid_channels, mid_channels, t)]
+ self.gate = ChannelGate(mid_channels)
+ self.conv3 = Conv1x1Linear(mid_channels, out_channels)
+ self.downsample = None
+ if in_channels != out_channels:
+ self.downsample = Conv1x1Linear(in_channels, out_channels)
+
+ def forward(self, x):
+ identity = x
+ x1 = self.conv1(x)
+ x2 = 0
+ for conv2_t in self.conv2:
+ x2_t = conv2_t(x1)
+ x2 = x2 + self.gate(x2_t)
+ x3 = self.conv3(x2)
+ if self.downsample is not None:
+ identity = self.downsample(identity)
+ out = x3 + identity
+ return F.relu(out)
+
+
+class OSBlockINv1(nn.Module):
+ """Omni-scale feature learning block with instance normalization."""
+
+ def __init__(self, in_channels, out_channels, reduction=4, T=4, **kwargs):
+ super(OSBlockINv1, self).__init__()
+ assert T >= 1
+ assert out_channels >= reduction and out_channels % reduction == 0
+ mid_channels = out_channels // reduction
+
+ self.conv1 = Conv1x1(in_channels, mid_channels)
+ self.conv2 = nn.ModuleList()
+ for t in range(1, T + 1):
+ self.conv2 += [LightConvStream(mid_channels, mid_channels, t)]
+ self.gate = ChannelGate(mid_channels)
+ self.conv3 = Conv1x1Linear(mid_channels, out_channels, bn=False)
+ self.downsample = None
+ if in_channels != out_channels:
+ self.downsample = Conv1x1Linear(in_channels, out_channels)
+ self.IN = nn.InstanceNorm2d(out_channels, affine=NORM_AFFINE)
+
+ def forward(self, x):
+ identity = x
+ x1 = self.conv1(x)
+ x2 = 0
+ for conv2_t in self.conv2:
+ x2_t = conv2_t(x1)
+ x2 = x2 + self.gate(x2_t)
+ x3 = self.conv3(x2)
+ x3 = self.IN(x3) # IN inside residual
+ if self.downsample is not None:
+ identity = self.downsample(identity)
+ out = x3 + identity
+ return F.relu(out)
+
+
+class OSBlockINv2(nn.Module):
+ """Omni-scale feature learning block with instance normalization."""
+
+ def __init__(self, in_channels, out_channels, reduction=4, T=4, **kwargs):
+ super(OSBlockINv2, self).__init__()
+ assert T >= 1
+ assert out_channels >= reduction and out_channels % reduction == 0
+ mid_channels = out_channels // reduction
+
+ self.conv1 = Conv1x1(in_channels, mid_channels)
+ self.conv2 = nn.ModuleList()
+ for t in range(1, T + 1):
+ self.conv2 += [LightConvStream(mid_channels, mid_channels, t)]
+ self.gate = ChannelGate(mid_channels)
+ self.conv3 = Conv1x1Linear(mid_channels, out_channels)
+ self.downsample = None
+ if in_channels != out_channels:
+ self.downsample = Conv1x1Linear(in_channels, out_channels)
+ self.IN = nn.InstanceNorm2d(out_channels, affine=NORM_AFFINE)
+
+ def forward(self, x):
+ identity = x
+ x1 = self.conv1(x)
+ x2 = 0
+ for conv2_t in self.conv2:
+ x2_t = conv2_t(x1)
+ x2 = x2 + self.gate(x2_t)
+ x3 = self.conv3(x2)
+ if self.downsample is not None:
+ identity = self.downsample(identity)
+ out = x3 + identity
+ out = self.IN(out) # IN outside residual
+ return F.relu(out)
+
+
+class OSBlockINv3(nn.Module):
+ """Omni-scale feature learning block with instance normalization."""
+
+ def __init__(self, in_channels, out_channels, reduction=4, T=4, **kwargs):
+ super(OSBlockINv3, self).__init__()
+ assert T >= 1
+ assert out_channels >= reduction and out_channels % reduction == 0
+ mid_channels = out_channels // reduction
+
+ self.conv1 = Conv1x1(in_channels, mid_channels)
+ self.conv2 = nn.ModuleList()
+ for t in range(1, T + 1):
+ self.conv2 += [LightConvStream(mid_channels, mid_channels, t)]
+ self.gate = ChannelGate(mid_channels)
+ self.conv3 = Conv1x1Linear(mid_channels, out_channels, bn=False)
+ self.downsample = None
+ if in_channels != out_channels:
+ self.downsample = Conv1x1Linear(in_channels, out_channels)
+ self.IN_in = nn.InstanceNorm2d(out_channels, affine=NORM_AFFINE)
+ self.IN_out = nn.InstanceNorm2d(out_channels, affine=NORM_AFFINE)
+
+ def forward(self, x):
+ identity = x
+ x1 = self.conv1(x)
+ x2 = 0
+ for conv2_t in self.conv2:
+ x2_t = conv2_t(x1)
+ x2 = x2 + self.gate(x2_t)
+ x3 = self.conv3(x2)
+ x3 = self.IN_in(x3) # inside residual
+ if self.downsample is not None:
+ identity = self.downsample(identity)
+ out = x3 + identity
+ out = self.IN_out(out) # IN outside residual
+ return F.relu(out)
+
+
+class NASBlock(nn.Module):
+ """Neural architecture search layer."""
+
+ def __init__(self, in_channels, out_channels, search_space=None):
+ super(NASBlock, self).__init__()
+ self._is_child_graph = False
+ self.search_space = search_space
+ if self.search_space is None:
+ raise ValueError('search_space is None')
+
+ self.os_block = nn.ModuleList()
+ for block in self.search_space:
+ self.os_block += [block(in_channels, out_channels)]
+ self.weights = nn.Parameter(torch.ones(len(self.search_space)))
+
+ def build_child_graph(self):
+ if self._is_child_graph:
+ raise RuntimeError('build_child_graph() can only be called once')
+
+ idx = self.weights.data.max(dim=0)[1].item()
+ self.os_block = self.os_block[idx]
+ self.weights = None
+ self._is_child_graph = True
+ return self.search_space[idx]
+
+ def forward(self, x, lmda=1.):
+ if self._is_child_graph:
+ return self.os_block(x)
+
+ uniform = torch.rand_like(self.weights)
+ gumbel = -torch.log(-torch.log(uniform + EPS))
+ nonneg_weights = F.relu(self.weights)
+ logits = torch.log(nonneg_weights + EPS) + gumbel
+ exp = torch.exp(logits / lmda)
+ weights_softmax = exp / (exp.sum() + EPS)
+
+ output = 0
+ for i, weight in enumerate(weights_softmax):
+ output = output + weight * self.os_block[i](x)
+ return output
+
+
+##########
+# Network architecture
+##########
+class OSNet(nn.Module):
+ """Omni-Scale Network.
+
+ Reference:
+ - Zhou et al. Omni-Scale Feature Learning for Person Re-Identification. ICCV, 2019.
+ - Zhou et al. Learning Generalisable Omni-Scale Representations
+ for Person Re-Identification. TPAMI, 2021.
+ """
+
+ def __init__(
+ self,
+ num_classes,
+ blocks,
+ layers,
+ channels,
+ feature_dim=512,
+ loss='softmax',
+ search_space=None,
+ **kwargs
+ ):
+ super(OSNet, self).__init__()
+ num_blocks = len(blocks)
+ assert num_blocks == len(layers)
+ assert num_blocks == len(channels) - 1
+ # no matter what loss is specified, the model only returns the ID predictions
+ self.loss = loss
+ self.feature_dim = feature_dim
+
+ # convolutional backbone
+ self.conv1 = ConvLayer(3, channels[0], 7, stride=2, padding=3, IN=True)
+ self.maxpool = nn.MaxPool2d(3, stride=2, padding=1)
+ self.conv2 = self._make_layer(
+ blocks[0], layers[0], channels[0], channels[1], search_space
+ )
+ self.pool2 = nn.Sequential(
+ Conv1x1(channels[1], channels[1]), nn.AvgPool2d(2, stride=2)
+ )
+ self.conv3 = self._make_layer(
+ blocks[1], layers[1], channels[1], channels[2], search_space
+ )
+ self.pool3 = nn.Sequential(
+ Conv1x1(channels[2], channels[2]), nn.AvgPool2d(2, stride=2)
+ )
+ self.conv4 = self._make_layer(
+ blocks[2], layers[2], channels[2], channels[3], search_space
+ )
+ self.conv5 = Conv1x1(channels[3], channels[3])
+ self.global_avgpool = nn.AdaptiveAvgPool2d(1)
+ # fully connected layer
+ self.fc = self._construct_fc_layer(
+ self.feature_dim, channels[3], dropout_p=None
+ )
+ # identity classification layer
+ self.classifier = nn.Linear(self.feature_dim, num_classes)
+
+ def _make_layer(
+ self, block, layer, in_channels, out_channels, search_space
+ ):
+ layers = nn.ModuleList()
+ layers += [block(in_channels, out_channels, search_space=search_space)]
+ for i in range(1, layer):
+ layers += [
+ block(out_channels, out_channels, search_space=search_space)
+ ]
+ return layers
+
+ def _construct_fc_layer(self, fc_dims, input_dim, dropout_p=None):
+ if fc_dims is None or fc_dims < 0:
+ self.feature_dim = input_dim
+ return None
+
+ if isinstance(fc_dims, int):
+ fc_dims = [fc_dims]
+
+ layers = []
+ for dim in fc_dims:
+ layers.append(nn.Linear(input_dim, dim))
+ layers.append(nn.BatchNorm1d(dim, affine=NORM_AFFINE))
+ layers.append(nn.ReLU(inplace=True))
+ if dropout_p is not None:
+ layers.append(nn.Dropout(p=dropout_p))
+ input_dim = dim
+
+ self.feature_dim = fc_dims[-1]
+
+ return nn.Sequential(*layers)
+
+ def build_child_graph(self):
+ print('Building child graph')
+ for i, conv in enumerate(self.conv2):
+ block = conv.build_child_graph()
+ print('- conv2-{} Block={}'.format(i + 1, block.__name__))
+ for i, conv in enumerate(self.conv3):
+ block = conv.build_child_graph()
+ print('- conv3-{} Block={}'.format(i + 1, block.__name__))
+ for i, conv in enumerate(self.conv4):
+ block = conv.build_child_graph()
+ print('- conv4-{} Block={}'.format(i + 1, block.__name__))
+
+ def featuremaps(self, x, lmda):
+ x = self.conv1(x)
+ x = self.maxpool(x)
+ for conv in self.conv2:
+ x = conv(x, lmda)
+ x = self.pool2(x)
+ for conv in self.conv3:
+ x = conv(x, lmda)
+ x = self.pool3(x)
+ for conv in self.conv4:
+ x = conv(x, lmda)
+ return self.conv5(x)
+
+ def forward(self, x, lmda=1., return_featuremaps=False):
+ # lmda (float): temperature parameter for concrete distribution
+ x = self.featuremaps(x, lmda)
+ if return_featuremaps:
+ return x
+ v = self.global_avgpool(x)
+ v = v.view(v.size(0), -1)
+ if self.fc is not None:
+ v = self.fc(v)
+ if not self.training:
+ return v
+ return self.classifier(v)
+
+
+##########
+# Instantiation
+##########
+def osnet_nas(num_classes=1000, loss='softmax', **kwargs):
+ # standard size (width x1.0)
+ return OSNet(
+ num_classes,
+ blocks=[NASBlock, NASBlock, NASBlock],
+ layers=[2, 2, 2],
+ channels=[64, 256, 384, 512],
+ loss=loss,
+ search_space=[OSBlock, OSBlockINv1, OSBlockINv2, OSBlockINv3],
+ **kwargs
+ )
+
+
+__NAS_models = {'osnet_nas': osnet_nas}
+
+
+def build_model(name, num_classes=100):
+ avai_models = list(__NAS_models.keys())
+ if name not in avai_models:
+ raise KeyError(
+ 'Unknown model: {}. Must be one of {}'.format(name, avai_models)
+ )
+ return __NAS_models[name](num_classes=num_classes)
diff --git a/trackers/strong_sort/deep/reid/projects/OSNet_AIN/softmax_nas.py b/trackers/strong_sort/deep/reid/projects/OSNet_AIN/softmax_nas.py
new file mode 100644
index 0000000000000000000000000000000000000000..e2a03b6be4e82fabac4c89714c700c75d8cb1b23
--- /dev/null
+++ b/trackers/strong_sort/deep/reid/projects/OSNet_AIN/softmax_nas.py
@@ -0,0 +1,73 @@
+from __future__ import division, print_function, absolute_import
+
+from torchreid import metrics
+from torchreid.engine import Engine
+from torchreid.losses import CrossEntropyLoss
+
+
+class ImageSoftmaxNASEngine(Engine):
+
+ def __init__(
+ self,
+ datamanager,
+ model,
+ optimizer,
+ scheduler=None,
+ use_gpu=False,
+ label_smooth=True,
+ mc_iter=1,
+ init_lmda=1.,
+ min_lmda=1.,
+ lmda_decay_step=20,
+ lmda_decay_rate=0.5,
+ fixed_lmda=False
+ ):
+ super(ImageSoftmaxNASEngine, self).__init__(datamanager, use_gpu)
+ self.mc_iter = mc_iter
+ self.init_lmda = init_lmda
+ self.min_lmda = min_lmda
+ self.lmda_decay_step = lmda_decay_step
+ self.lmda_decay_rate = lmda_decay_rate
+ self.fixed_lmda = fixed_lmda
+
+ self.model = model
+ self.optimizer = optimizer
+ self.scheduler = scheduler
+ self.register_model('model', model, optimizer, scheduler)
+
+ self.criterion = CrossEntropyLoss(
+ num_classes=self.datamanager.num_train_pids,
+ use_gpu=self.use_gpu,
+ label_smooth=label_smooth
+ )
+
+ def forward_backward(self, data):
+ imgs, pids = self.parse_data_for_train(data)
+
+ if self.use_gpu:
+ imgs = imgs.cuda()
+ pids = pids.cuda()
+
+ # softmax temporature
+ if self.fixed_lmda or self.lmda_decay_step == -1:
+ lmda = self.init_lmda
+ else:
+ lmda = self.init_lmda * self.lmda_decay_rate**(
+ self.epoch // self.lmda_decay_step
+ )
+ if lmda < self.min_lmda:
+ lmda = self.min_lmda
+
+ for k in range(self.mc_iter):
+ outputs = self.model(imgs, lmda=lmda)
+ loss = self.compute_loss(self.criterion, outputs, pids)
+ self.optimizer.zero_grad()
+ loss.backward()
+ self.optimizer.step()
+
+ loss_dict = {
+ 'loss': loss.item(),
+ 'acc': metrics.accuracy(outputs, pids)[0].item()
+ }
+
+ return loss_dict
diff --git a/trackers/strong_sort/deep/reid/projects/README.md b/trackers/strong_sort/deep/reid/projects/README.md
new file mode 100644
index 0000000000000000000000000000000000000000..fa63919ffdd31eaa0b7b6bf8032c9c589b4f233d
--- /dev/null
+++ b/trackers/strong_sort/deep/reid/projects/README.md
@@ -0,0 +1,5 @@
+Here are some research projects built on [Torchreid](https://arxiv.org/abs/1910.10093).
+
++ `OSNet_AIN`: [Learning Generalisable Omni-Scale Representations for Person Re-Identification](https://arxiv.org/abs/1910.06827)
++ `DML`: [Deep Mutual Learning (CVPR'18)](https://arxiv.org/abs/1706.00384)
++ `attribute_recognition`: [Omni-Scale Feature Learning for Person Re-Identification (ICCV'19)](https://arxiv.org/abs/1905.00953)
\ No newline at end of file
diff --git a/trackers/strong_sort/deep/reid/projects/attribute_recognition/README.md b/trackers/strong_sort/deep/reid/projects/attribute_recognition/README.md
new file mode 100644
index 0000000000000000000000000000000000000000..a20b6e7f1c279c065f8f2f81e078410796829951
--- /dev/null
+++ b/trackers/strong_sort/deep/reid/projects/attribute_recognition/README.md
@@ -0,0 +1,18 @@
+# Person Attribute Recognition
+This code was developed for the experiment of person attribute recognition in [Omni-Scale Feature Learning for Person Re-Identification (ICCV'19)](https://arxiv.org/abs/1905.00953).
+
+## Download data
+Download the PA-100K dataset from [https://github.com/xh-liu/HydraPlus-Net](https://github.com/xh-liu/HydraPlus-Net), and extract the file under the folder where you store your data (say $DATASET). The folder structure should look like
+```bash
+$DATASET/
+ pa100k/
+ data/ # images
+ annotation/
+ annotation.mat
+```
+
+## Train
+The training command is provided in `train.sh`. Run `bash train.sh $DATASET` to start training.
+
+## Test
+To test a pretrained model, add the following two arguments to `train.sh`: `--load-weights $PATH_TO_WEIGHTS --evaluate`.
\ No newline at end of file
diff --git a/trackers/strong_sort/deep/reid/projects/attribute_recognition/datasets/__init__.py b/trackers/strong_sort/deep/reid/projects/attribute_recognition/datasets/__init__.py
new file mode 100644
index 0000000000000000000000000000000000000000..664598c71865da78ce937605de3bbf29ef304152
--- /dev/null
+++ b/trackers/strong_sort/deep/reid/projects/attribute_recognition/datasets/__init__.py
@@ -0,0 +1,15 @@
+from __future__ import division, print_function, absolute_import
+
+from .pa100k import PA100K
+
+__datasets = {'pa100k': PA100K}
+
+
+def init_dataset(name, **kwargs):
+ avai_datasets = list(__datasets.keys())
+ if name not in avai_datasets:
+ raise ValueError(
+ 'Invalid dataset name. Received "{}", '
+ 'but expected to be one of {}'.format(name, avai_datasets)
+ )
+ return __datasets[name](**kwargs)
diff --git a/trackers/strong_sort/deep/reid/projects/attribute_recognition/datasets/dataset.py b/trackers/strong_sort/deep/reid/projects/attribute_recognition/datasets/dataset.py
new file mode 100644
index 0000000000000000000000000000000000000000..7ce4ebff15a52140b7e713322acfbb42eb013fdc
--- /dev/null
+++ b/trackers/strong_sort/deep/reid/projects/attribute_recognition/datasets/dataset.py
@@ -0,0 +1,87 @@
+from __future__ import division, print_function, absolute_import
+import os.path as osp
+
+from torchreid.utils import read_image
+
+
+class Dataset(object):
+
+ def __init__(
+ self,
+ train,
+ val,
+ test,
+ attr_dict,
+ transform=None,
+ mode='train',
+ verbose=True,
+ **kwargs
+ ):
+ self.train = train
+ self.val = val
+ self.test = test
+ self._attr_dict = attr_dict
+ self._num_attrs = len(self.attr_dict)
+ self.transform = transform
+
+ if mode == 'train':
+ self.data = self.train
+ elif mode == 'val':
+ self.data = self.val
+ else:
+ self.data = self.test
+
+ if verbose:
+ self.show_summary()
+
+ @property
+ def num_attrs(self):
+ return self._num_attrs
+
+ @property
+ def attr_dict(self):
+ return self._attr_dict
+
+ def __len__(self):
+ return len(self.data)
+
+ def __getitem__(self, index):
+ img_path, attrs = self.data[index]
+ img = read_image(img_path)
+ if self.transform is not None:
+ img = self.transform(img)
+ return img, attrs, img_path
+
+ def check_before_run(self, required_files):
+ """Checks if required files exist before going deeper.
+ Args:
+ required_files (str or list): string file name(s).
+ """
+ if isinstance(required_files, str):
+ required_files = [required_files]
+
+ for fpath in required_files:
+ if not osp.exists(fpath):
+ raise RuntimeError('"{}" is not found'.format(fpath))
+
+ def show_summary(self):
+ num_train = len(self.train)
+ num_val = len(self.val)
+ num_test = len(self.test)
+ num_total = num_train + num_val + num_test
+
+ print('=> Loaded {}'.format(self.__class__.__name__))
+ print(" ------------------------------")
+ print(" subset | # images")
+ print(" ------------------------------")
+ print(" train | {:8d}".format(num_train))
+ print(" val | {:8d}".format(num_val))
+ print(" test | {:8d}".format(num_test))
+ print(" ------------------------------")
+ print(" total | {:8d}".format(num_total))
+ print(" ------------------------------")
+ print(" # attributes: {}".format(len(self.attr_dict)))
+ print(" attributes:")
+ for label, attr in self.attr_dict.items():
+ print(' {:3d}: {}'.format(label, attr))
+ print(" ------------------------------")
diff --git a/trackers/strong_sort/deep/reid/projects/attribute_recognition/datasets/pa100k.py b/trackers/strong_sort/deep/reid/projects/attribute_recognition/datasets/pa100k.py
new file mode 100644
index 0000000000000000000000000000000000000000..61dd26cf54eef209e3ee3a8081802a0da8a77f66
--- /dev/null
+++ b/trackers/strong_sort/deep/reid/projects/attribute_recognition/datasets/pa100k.py
@@ -0,0 +1,59 @@
+from __future__ import division, print_function, absolute_import
+import numpy as np
+import os.path as osp
+from scipy.io import loadmat
+
+from .dataset import Dataset
+
+
+class PA100K(Dataset):
+ """Pedestrian attribute dataset.
+
+ 80k training images + 20k test images.
+
+ The folder structure should be:
+ pa100k/
+ data/ # images
+ annotation/
+ annotation.mat
+ """
+ dataset_dir = 'pa100k'
+
+ def __init__(self, root='', **kwargs):
+ self.root = osp.abspath(osp.expanduser(root))
+ self.dataset_dir = osp.join(self.root, self.dataset_dir)
+ self.data_dir = osp.join(self.dataset_dir, 'data')
+ self.anno_mat_path = osp.join(
+ self.dataset_dir, 'annotation', 'annotation.mat'
+ )
+
+ required_files = [self.data_dir, self.anno_mat_path]
+ self.check_before_run(required_files)
+
+ train, val, test, attr_dict = self.extract_data()
+ super(PA100K, self).__init__(train, val, test, attr_dict, **kwargs)
+
+ def extract_data(self):
+ # anno_mat is a dictionary with keys: ['test_images_name', 'val_images_name',
+ # 'train_images_name', 'val_label', 'attributes', 'test_label', 'train_label']
+ anno_mat = loadmat(self.anno_mat_path)
+
+ def _extract(key_name, key_label):
+ names = anno_mat[key_name]
+ labels = anno_mat[key_label]
+ num_imgs = names.shape[0]
+ data = []
+ for i in range(num_imgs):
+ name = names[i, 0][0]
+ attrs = labels[i, :].astype(np.float32)
+ img_path = osp.join(self.data_dir, name)
+ data.append((img_path, attrs))
+ return data
+
+ train = _extract('train_images_name', 'train_label')
+ val = _extract('val_images_name', 'val_label')
+ test = _extract('test_images_name', 'test_label')
+ attrs = anno_mat['attributes']
+ attr_dict = {i: str(attr[0][0]) for i, attr in enumerate(attrs)}
+
+ return train, val, test, attr_dict
diff --git a/trackers/strong_sort/deep/reid/projects/attribute_recognition/default_parser.py b/trackers/strong_sort/deep/reid/projects/attribute_recognition/default_parser.py
new file mode 100644
index 0000000000000000000000000000000000000000..d19a8be9716455b15d5fdffe6b99f9a3c3d8c209
--- /dev/null
+++ b/trackers/strong_sort/deep/reid/projects/attribute_recognition/default_parser.py
@@ -0,0 +1,243 @@
+from __future__ import print_function, absolute_import
+import argparse
+
+
+def init_parser():
+ parser = argparse.ArgumentParser(
+ formatter_class=argparse.ArgumentDefaultsHelpFormatter
+ )
+
+ # ************************************************************
+ # Datasets
+ # ************************************************************
+ parser.add_argument(
+ '--root',
+ type=str,
+ default='',
+ required=True,
+ help='root path to data directory'
+ )
+ parser.add_argument(
+ '-d',
+ '--dataset',
+ type=str,
+ required=True,
+ help='which dataset to choose'
+ )
+ parser.add_argument(
+ '-j',
+ '--workers',
+ type=int,
+ default=4,
+ help='number of data loading workers (tips: 4 or 8 times number of gpus)'
+ )
+ parser.add_argument(
+ '--height', type=int, default=256, help='height of an image'
+ )
+ parser.add_argument(
+ '--width', type=int, default=128, help='width of an image'
+ )
+
+ # ************************************************************
+ # Optimization options
+ # ************************************************************
+ parser.add_argument(
+ '--optim',
+ type=str,
+ default='adam',
+ help='optimization algorithm (see optimizers.py)'
+ )
+ parser.add_argument(
+ '--lr', type=float, default=0.0003, help='initial learning rate'
+ )
+ parser.add_argument(
+ '--weight-decay', type=float, default=5e-04, help='weight decay'
+ )
+ # sgd
+ parser.add_argument(
+ '--momentum',
+ type=float,
+ default=0.9,
+ help='momentum factor for sgd and rmsprop'
+ )
+ parser.add_argument(
+ '--sgd-dampening',
+ type=float,
+ default=0,
+ help='sgd\'s dampening for momentum'
+ )
+ parser.add_argument(
+ '--sgd-nesterov',
+ action='store_true',
+ help='whether to enable sgd\'s Nesterov momentum'
+ )
+ # rmsprop
+ parser.add_argument(
+ '--rmsprop-alpha',
+ type=float,
+ default=0.99,
+ help='rmsprop\'s smoothing constant'
+ )
+ # adam/amsgrad
+ parser.add_argument(
+ '--adam-beta1',
+ type=float,
+ default=0.9,
+ help='exponential decay rate for adam\'s first moment'
+ )
+ parser.add_argument(
+ '--adam-beta2',
+ type=float,
+ default=0.999,
+ help='exponential decay rate for adam\'s second moment'
+ )
+
+ # ************************************************************
+ # Training hyperparameters
+ # ************************************************************
+ parser.add_argument(
+ '--max-epoch', type=int, default=60, help='maximum epochs to run'
+ )
+ parser.add_argument(
+ '--start-epoch',
+ type=int,
+ default=0,
+ help='manual epoch number (useful when restart)'
+ )
+ parser.add_argument(
+ '--batch-size', type=int, default=32, help='batch size'
+ )
+
+ parser.add_argument(
+ '--fixbase-epoch',
+ type=int,
+ default=0,
+ help='number of epochs to fix base layers'
+ )
+ parser.add_argument(
+ '--open-layers',
+ type=str,
+ nargs='+',
+ default=['classifier'],
+ help='open specified layers for training while keeping others frozen'
+ )
+
+ parser.add_argument(
+ '--staged-lr',
+ action='store_true',
+ help='set different lr to different layers'
+ )
+ parser.add_argument(
+ '--new-layers',
+ type=str,
+ nargs='+',
+ default=['classifier'],
+ help='newly added layers with default lr'
+ )
+ parser.add_argument(
+ '--base-lr-mult',
+ type=float,
+ default=0.1,
+ help='learning rate multiplier for base layers'
+ )
+
+ # ************************************************************
+ # Learning rate scheduler options
+ # ************************************************************
+ parser.add_argument(
+ '--lr-scheduler',
+ type=str,
+ default='multi_step',
+ help='learning rate scheduler (see lr_schedulers.py)'
+ )
+ parser.add_argument(
+ '--stepsize',
+ type=int,
+ default=[20, 40],
+ nargs='+',
+ help='stepsize to decay learning rate'
+ )
+ parser.add_argument(
+ '--gamma', type=float, default=0.1, help='learning rate decay'
+ )
+
+ # ************************************************************
+ # Architecture
+ # ************************************************************
+ parser.add_argument(
+ '-a', '--arch', type=str, default='', help='model architecture'
+ )
+ parser.add_argument(
+ '--no-pretrained',
+ action='store_true',
+ help='do not load pretrained weights'
+ )
+
+ # ************************************************************
+ # Loss
+ # ************************************************************
+ parser.add_argument(
+ '--weighted-bce', action='store_true', help='use weighted BCELoss'
+ )
+
+ # ************************************************************
+ # Test settings
+ # ************************************************************
+ parser.add_argument(
+ '--load-weights', type=str, default='', help='load pretrained weights'
+ )
+ parser.add_argument(
+ '--evaluate', action='store_true', help='evaluate only'
+ )
+ parser.add_argument(
+ '--save-prediction', action='store_true', help='save prediction'
+ )
+
+ # ************************************************************
+ # Miscs
+ # ************************************************************
+ parser.add_argument(
+ '--print-freq', type=int, default=20, help='print frequency'
+ )
+ parser.add_argument('--seed', type=int, default=1, help='manual seed')
+ parser.add_argument(
+ '--resume',
+ type=str,
+ default='',
+ metavar='PATH',
+ help='resume from a checkpoint'
+ )
+ parser.add_argument(
+ '--save-dir',
+ type=str,
+ default='log',
+ help='path to save log and model weights'
+ )
+ parser.add_argument('--use-cpu', action='store_true', help='use cpu')
+
+ return parser
+
+
+def optimizer_kwargs(parsed_args):
+ return {
+ 'optim': parsed_args.optim,
+ 'lr': parsed_args.lr,
+ 'weight_decay': parsed_args.weight_decay,
+ 'momentum': parsed_args.momentum,
+ 'sgd_dampening': parsed_args.sgd_dampening,
+ 'sgd_nesterov': parsed_args.sgd_nesterov,
+ 'rmsprop_alpha': parsed_args.rmsprop_alpha,
+ 'adam_beta1': parsed_args.adam_beta1,
+ 'adam_beta2': parsed_args.adam_beta2,
+ 'staged_lr': parsed_args.staged_lr,
+ 'new_layers': parsed_args.new_layers,
+ 'base_lr_mult': parsed_args.base_lr_mult
+ }
+
+
+def lr_scheduler_kwargs(parsed_args):
+ return {
+ 'lr_scheduler': parsed_args.lr_scheduler,
+ 'stepsize': parsed_args.stepsize,
+ 'gamma': parsed_args.gamma
+ }
diff --git a/trackers/strong_sort/deep/reid/projects/attribute_recognition/main.py b/trackers/strong_sort/deep/reid/projects/attribute_recognition/main.py
new file mode 100644
index 0000000000000000000000000000000000000000..1fb423802f4c1ee5632a2e5191a5da769ccce079
--- /dev/null
+++ b/trackers/strong_sort/deep/reid/projects/attribute_recognition/main.py
@@ -0,0 +1,399 @@
+from __future__ import division, print_function
+import sys
+import copy
+import time
+import numpy as np
+import os.path as osp
+import datetime
+import warnings
+import torch
+import torch.nn as nn
+
+import torchreid
+from torchreid.utils import (
+ Logger, AverageMeter, check_isfile, open_all_layers, save_checkpoint,
+ set_random_seed, collect_env_info, open_specified_layers,
+ load_pretrained_weights, compute_model_complexity
+)
+from torchreid.data.transforms import (
+ Resize, Compose, ToTensor, Normalize, Random2DTranslation,
+ RandomHorizontalFlip
+)
+
+import models
+import datasets
+from default_parser import init_parser, optimizer_kwargs, lr_scheduler_kwargs
+
+parser = init_parser()
+args = parser.parse_args()
+
+
+def init_dataset(use_gpu):
+ normalize = Normalize(
+ mean=[0.485, 0.456, 0.406], std=[0.229, 0.224, 0.225]
+ )
+
+ transform_tr = Compose(
+ [
+ Random2DTranslation(args.height, args.width, p=0.5),
+ RandomHorizontalFlip(),
+ ToTensor(), normalize
+ ]
+ )
+
+ transform_te = Compose(
+ [Resize([args.height, args.width]),
+ ToTensor(), normalize]
+ )
+
+ trainset = datasets.init_dataset(
+ args.dataset,
+ root=args.root,
+ transform=transform_tr,
+ mode='train',
+ verbose=True
+ )
+
+ valset = datasets.init_dataset(
+ args.dataset,
+ root=args.root,
+ transform=transform_te,
+ mode='val',
+ verbose=False
+ )
+
+ testset = datasets.init_dataset(
+ args.dataset,
+ root=args.root,
+ transform=transform_te,
+ mode='test',
+ verbose=False
+ )
+
+ num_attrs = trainset.num_attrs
+ attr_dict = trainset.attr_dict
+
+ trainloader = torch.utils.data.DataLoader(
+ trainset,
+ batch_size=args.batch_size,
+ shuffle=True,
+ num_workers=args.workers,
+ pin_memory=use_gpu,
+ drop_last=True
+ )
+
+ valloader = torch.utils.data.DataLoader(
+ valset,
+ batch_size=args.batch_size,
+ shuffle=False,
+ num_workers=args.workers,
+ pin_memory=use_gpu,
+ drop_last=False
+ )
+
+ testloader = torch.utils.data.DataLoader(
+ testset,
+ batch_size=args.batch_size,
+ shuffle=False,
+ num_workers=args.workers,
+ pin_memory=use_gpu,
+ drop_last=False
+ )
+
+ return trainloader, valloader, testloader, num_attrs, attr_dict
+
+
+def main():
+ global args
+
+ set_random_seed(args.seed)
+ use_gpu = torch.cuda.is_available() and not args.use_cpu
+ log_name = 'test.log' if args.evaluate else 'train.log'
+ sys.stdout = Logger(osp.join(args.save_dir, log_name))
+
+ print('** Arguments **')
+ arg_keys = list(args.__dict__.keys())
+ arg_keys.sort()
+ for key in arg_keys:
+ print('{}: {}'.format(key, args.__dict__[key]))
+ print('\n')
+ print('Collecting env info ...')
+ print('** System info **\n{}\n'.format(collect_env_info()))
+
+ if use_gpu:
+ torch.backends.cudnn.benchmark = True
+ else:
+ warnings.warn(
+ 'Currently using CPU, however, GPU is highly recommended'
+ )
+
+ dataset_vars = init_dataset(use_gpu)
+ trainloader, valloader, testloader, num_attrs, attr_dict = dataset_vars
+
+ if args.weighted_bce:
+ print('Use weighted binary cross entropy')
+ print('Computing the weights ...')
+ bce_weights = torch.zeros(num_attrs, dtype=torch.float)
+ for _, attrs, _ in trainloader:
+ bce_weights += attrs.sum(0) # sum along the batch dim
+ bce_weights /= len(trainloader) * args.batch_size
+ print('Sample ratio for each attribute: {}'.format(bce_weights))
+ bce_weights = torch.exp(-1 * bce_weights)
+ print('BCE weights: {}'.format(bce_weights))
+ bce_weights = bce_weights.expand(args.batch_size, num_attrs)
+ criterion = nn.BCEWithLogitsLoss(weight=bce_weights)
+
+ else:
+ print('Use plain binary cross entropy')
+ criterion = nn.BCEWithLogitsLoss()
+
+ print('Building model: {}'.format(args.arch))
+ model = models.build_model(
+ args.arch,
+ num_attrs,
+ pretrained=not args.no_pretrained,
+ use_gpu=use_gpu
+ )
+ num_params, flops = compute_model_complexity(
+ model, (1, 3, args.height, args.width)
+ )
+ print('Model complexity: params={:,} flops={:,}'.format(num_params, flops))
+
+ if args.load_weights and check_isfile(args.load_weights):
+ load_pretrained_weights(model, args.load_weights)
+
+ if use_gpu:
+ model = nn.DataParallel(model).cuda()
+ criterion = criterion.cuda()
+
+ if args.evaluate:
+ test(model, testloader, attr_dict, use_gpu)
+ return
+
+ optimizer = torchreid.optim.build_optimizer(
+ model, **optimizer_kwargs(args)
+ )
+ scheduler = torchreid.optim.build_lr_scheduler(
+ optimizer, **lr_scheduler_kwargs(args)
+ )
+
+ start_epoch = args.start_epoch
+ best_result = -np.inf
+ if args.resume and check_isfile(args.resume):
+ checkpoint = torch.load(args.resume)
+ model.load_state_dict(checkpoint['state_dict'])
+ optimizer.load_state_dict(checkpoint['optimizer'])
+ start_epoch = checkpoint['epoch']
+ best_result = checkpoint['label_mA']
+ print('Loaded checkpoint from "{}"'.format(args.resume))
+ print('- start epoch: {}'.format(start_epoch))
+ print('- label_mA: {}'.format(best_result))
+
+ time_start = time.time()
+
+ for epoch in range(start_epoch, args.max_epoch):
+ train(
+ epoch, model, criterion, optimizer, scheduler, trainloader, use_gpu
+ )
+ test_outputs = test(model, testloader, attr_dict, use_gpu)
+ label_mA = test_outputs[0]
+ is_best = label_mA > best_result
+ if is_best:
+ best_result = label_mA
+
+ save_checkpoint(
+ {
+ 'state_dict': model.state_dict(),
+ 'epoch': epoch + 1,
+ 'label_mA': label_mA,
+ 'optimizer': optimizer.state_dict(),
+ },
+ args.save_dir,
+ is_best=is_best
+ )
+
+ elapsed = round(time.time() - time_start)
+ elapsed = str(datetime.timedelta(seconds=elapsed))
+ print('Elapsed {}'.format(elapsed))
+
+
+def train(epoch, model, criterion, optimizer, scheduler, trainloader, use_gpu):
+ losses = AverageMeter()
+ batch_time = AverageMeter()
+ data_time = AverageMeter()
+ model.train()
+
+ if (epoch + 1) <= args.fixbase_epoch and args.open_layers is not None:
+ print(
+ '* Only train {} (epoch: {}/{})'.format(
+ args.open_layers, epoch + 1, args.fixbase_epoch
+ )
+ )
+ open_specified_layers(model, args.open_layers)
+ else:
+ open_all_layers(model)
+
+ end = time.time()
+ for batch_idx, data in enumerate(trainloader):
+ data_time.update(time.time() - end)
+
+ imgs, attrs = data[0], data[1]
+ if use_gpu:
+ imgs = imgs.cuda()
+ attrs = attrs.cuda()
+
+ optimizer.zero_grad()
+ outputs = model(imgs)
+ loss = criterion(outputs, attrs)
+ loss.backward()
+ optimizer.step()
+
+ batch_time.update(time.time() - end)
+
+ losses.update(loss.item(), imgs.size(0))
+
+ if (batch_idx+1) % args.print_freq == 0:
+ # estimate remaining time
+ num_batches = len(trainloader)
+ eta_seconds = batch_time.avg * (
+ num_batches - (batch_idx+1) + (args.max_epoch -
+ (epoch+1)) * num_batches
+ )
+ eta_str = str(datetime.timedelta(seconds=int(eta_seconds)))
+ print(
+ 'Epoch: [{0}/{1}][{2}/{3}]\t'
+ 'Time {batch_time.val:.3f} ({batch_time.avg:.3f})\t'
+ 'Data {data_time.val:.3f} ({data_time.avg:.3f})\t'
+ 'Loss {loss.val:.4f} ({loss.avg:.4f})\t'
+ 'Lr {lr:.6f}\t'
+ 'Eta {eta}'.format(
+ epoch + 1,
+ args.max_epoch,
+ batch_idx + 1,
+ len(trainloader),
+ batch_time=batch_time,
+ data_time=data_time,
+ loss=losses,
+ lr=optimizer.param_groups[0]['lr'],
+ eta=eta_str
+ )
+ )
+
+ end = time.time()
+
+ scheduler.step()
+
+
+@torch.no_grad()
+def test(model, testloader, attr_dict, use_gpu):
+ batch_time = AverageMeter()
+ model.eval()
+
+ num_persons = 0
+ prob_thre = 0.5
+ ins_acc = 0
+ ins_prec = 0
+ ins_rec = 0
+ mA_history = {
+ 'correct_pos': 0,
+ 'real_pos': 0,
+ 'correct_neg': 0,
+ 'real_neg': 0
+ }
+
+ print('Testing ...')
+
+ for batch_idx, data in enumerate(testloader):
+ imgs, attrs, img_paths = data
+ if use_gpu:
+ imgs = imgs.cuda()
+
+ end = time.time()
+ orig_outputs = model(imgs)
+ batch_time.update(time.time() - end)
+
+ orig_outputs = orig_outputs.data.cpu().numpy()
+ attrs = attrs.data.numpy()
+
+ # transform raw outputs to attributes (binary codes)
+ outputs = copy.deepcopy(orig_outputs)
+ outputs[outputs < prob_thre] = 0
+ outputs[outputs >= prob_thre] = 1
+
+ # compute label-based metric
+ overlaps = outputs * attrs
+ mA_history['correct_pos'] += overlaps.sum(0)
+ mA_history['real_pos'] += attrs.sum(0)
+ inv_overlaps = (1-outputs) * (1-attrs)
+ mA_history['correct_neg'] += inv_overlaps.sum(0)
+ mA_history['real_neg'] += (1 - attrs).sum(0)
+
+ outputs = outputs.astype(bool)
+ attrs = attrs.astype(bool)
+
+ # compute instabce-based accuracy
+ intersect = (outputs & attrs).astype(float)
+ union = (outputs | attrs).astype(float)
+ ins_acc += (intersect.sum(1) / union.sum(1)).sum()
+ ins_prec += (intersect.sum(1) / outputs.astype(float).sum(1)).sum()
+ ins_rec += (intersect.sum(1) / attrs.astype(float).sum(1)).sum()
+
+ num_persons += imgs.size(0)
+
+ if (batch_idx+1) % args.print_freq == 0:
+ print(
+ 'Processed batch {}/{}'.format(batch_idx + 1, len(testloader))
+ )
+
+ if args.save_prediction:
+ txtfile = open(osp.join(args.save_dir, 'prediction.txt'), 'a')
+ for idx in range(imgs.size(0)):
+ img_path = img_paths[idx]
+ probs = orig_outputs[idx, :]
+ labels = attrs[idx, :]
+ txtfile.write('{}\n'.format(img_path))
+ txtfile.write('*** Correct prediction ***\n')
+ for attr_idx, (label, prob) in enumerate(zip(labels, probs)):
+ if label:
+ attr_name = attr_dict[attr_idx]
+ info = '{}: {:.1%} '.format(attr_name, prob)
+ txtfile.write(info)
+ txtfile.write('\n*** Incorrect prediction ***\n')
+ for attr_idx, (label, prob) in enumerate(zip(labels, probs)):
+ if not label and prob > 0.5:
+ attr_name = attr_dict[attr_idx]
+ info = '{}: {:.1%} '.format(attr_name, prob)
+ txtfile.write(info)
+ txtfile.write('\n\n')
+ txtfile.close()
+
+ print(
+ '=> BatchTime(s)/BatchSize(img): {:.4f}/{}'.format(
+ batch_time.avg, args.batch_size
+ )
+ )
+
+ ins_acc /= num_persons
+ ins_prec /= num_persons
+ ins_rec /= num_persons
+ ins_f1 = (2*ins_prec*ins_rec) / (ins_prec+ins_rec)
+
+ term1 = mA_history['correct_pos'] / mA_history['real_pos']
+ term2 = mA_history['correct_neg'] / mA_history['real_neg']
+ label_mA_verbose = (term1+term2) * 0.5
+ label_mA = label_mA_verbose.mean()
+
+ print('* Results *')
+ print(' # test persons: {}'.format(num_persons))
+ print(' (instance-based) accuracy: {:.1%}'.format(ins_acc))
+ print(' (instance-based) precition: {:.1%}'.format(ins_prec))
+ print(' (instance-based) recall: {:.1%}'.format(ins_rec))
+ print(' (instance-based) f1-score: {:.1%}'.format(ins_f1))
+ print(' (label-based) mean accuracy: {:.1%}'.format(label_mA))
+ print(' mA for each attribute: {}'.format(label_mA_verbose))
+
+ return label_mA, ins_acc, ins_prec, ins_rec, ins_f1
+
+
+if __name__ == '__main__':
+ main()
diff --git a/trackers/strong_sort/deep/reid/projects/attribute_recognition/models/__init__.py b/trackers/strong_sort/deep/reid/projects/attribute_recognition/models/__init__.py
new file mode 100644
index 0000000000000000000000000000000000000000..ff0f0eda86c5cbc8b045a3d8469dbc7ba5a1ffcf
--- /dev/null
+++ b/trackers/strong_sort/deep/reid/projects/attribute_recognition/models/__init__.py
@@ -0,0 +1,17 @@
+from __future__ import absolute_import
+
+from .osnet import *
+
+__model_factory = {
+ 'osnet_avgpool': osnet_avgpool,
+ 'osnet_maxpool': osnet_maxpool
+}
+
+
+def build_model(name, num_classes, pretrained=True, use_gpu=True):
+ avai_models = list(__model_factory.keys())
+ if name not in avai_models:
+ raise KeyError
+ return __model_factory[name](
+ num_classes=num_classes, pretrained=pretrained, use_gpu=use_gpu
+ )
diff --git a/trackers/strong_sort/deep/reid/projects/attribute_recognition/models/osnet.py b/trackers/strong_sort/deep/reid/projects/attribute_recognition/models/osnet.py
new file mode 100644
index 0000000000000000000000000000000000000000..12569da08eb3c7e5c99abd3100e94b01e7924189
--- /dev/null
+++ b/trackers/strong_sort/deep/reid/projects/attribute_recognition/models/osnet.py
@@ -0,0 +1,414 @@
+from __future__ import division, absolute_import
+import torch
+from torch import nn
+from torch.nn import functional as F
+
+__all__ = ['osnet_avgpool', 'osnet_maxpool']
+
+
+##########
+# Basic layers
+##########
+class ConvLayer(nn.Module):
+ """Convolution layer."""
+
+ def __init__(
+ self,
+ in_channels,
+ out_channels,
+ kernel_size,
+ stride=1,
+ padding=0,
+ groups=1
+ ):
+ super(ConvLayer, self).__init__()
+ self.conv = nn.Conv2d(
+ in_channels,
+ out_channels,
+ kernel_size,
+ stride=stride,
+ padding=padding,
+ bias=False,
+ groups=groups
+ )
+ self.bn = nn.BatchNorm2d(out_channels)
+ self.relu = nn.ReLU(inplace=True)
+
+ def forward(self, x):
+ x = self.conv(x)
+ x = self.bn(x)
+ x = self.relu(x)
+ return x
+
+
+class Conv1x1(nn.Module):
+ """1x1 convolution."""
+
+ def __init__(self, in_channels, out_channels, stride=1, groups=1):
+ super(Conv1x1, self).__init__()
+ self.conv = nn.Conv2d(
+ in_channels,
+ out_channels,
+ 1,
+ stride=stride,
+ padding=0,
+ bias=False,
+ groups=groups
+ )
+ self.bn = nn.BatchNorm2d(out_channels)
+ self.relu = nn.ReLU(inplace=True)
+
+ def forward(self, x):
+ x = self.conv(x)
+ x = self.bn(x)
+ x = self.relu(x)
+ return x
+
+
+class Conv1x1Linear(nn.Module):
+ """1x1 convolution without non-linearity."""
+
+ def __init__(self, in_channels, out_channels, stride=1):
+ super(Conv1x1Linear, self).__init__()
+ self.conv = nn.Conv2d(
+ in_channels, out_channels, 1, stride=stride, padding=0, bias=False
+ )
+ self.bn = nn.BatchNorm2d(out_channels)
+
+ def forward(self, x):
+ x = self.conv(x)
+ x = self.bn(x)
+ return x
+
+
+class Conv3x3(nn.Module):
+ """3x3 convolution."""
+
+ def __init__(self, in_channels, out_channels, stride=1, groups=1):
+ super(Conv3x3, self).__init__()
+ self.conv = nn.Conv2d(
+ in_channels,
+ out_channels,
+ 3,
+ stride=stride,
+ padding=1,
+ bias=False,
+ groups=groups
+ )
+ self.bn = nn.BatchNorm2d(out_channels)
+ self.relu = nn.ReLU(inplace=True)
+
+ def forward(self, x):
+ x = self.conv(x)
+ x = self.bn(x)
+ x = self.relu(x)
+ return x
+
+
+class LightConv3x3(nn.Module):
+ """Lightweight 3x3 convolution.
+
+ 1x1 (linear) + dw 3x3 (nonlinear).
+ """
+
+ def __init__(self, in_channels, out_channels):
+ super(LightConv3x3, self).__init__()
+ self.conv1 = nn.Conv2d(
+ in_channels, out_channels, 1, stride=1, padding=0, bias=False
+ )
+ self.conv2 = nn.Conv2d(
+ out_channels,
+ out_channels,
+ 3,
+ stride=1,
+ padding=1,
+ bias=False,
+ groups=out_channels
+ )
+ self.bn = nn.BatchNorm2d(out_channels)
+ self.relu = nn.ReLU(inplace=True)
+
+ def forward(self, x):
+ x = self.conv1(x)
+ x = self.conv2(x)
+ x = self.bn(x)
+ x = self.relu(x)
+ return x
+
+
+##########
+# Building blocks for omni-scale feature learning
+##########
+class ChannelGate(nn.Module):
+ """A mini-network that generates channel-wise gates conditioned on input."""
+
+ def __init__(
+ self,
+ in_channels,
+ num_gates=None,
+ return_gates=False,
+ gate_activation='sigmoid',
+ reduction=16,
+ layer_norm=False
+ ):
+ super(ChannelGate, self).__init__()
+ if num_gates is None:
+ num_gates = in_channels
+ self.return_gates = return_gates
+ self.global_avgpool = nn.AdaptiveAvgPool2d(1)
+ self.fc1 = nn.Conv2d(
+ in_channels,
+ in_channels // reduction,
+ kernel_size=1,
+ bias=True,
+ padding=0
+ )
+ self.norm1 = None
+ if layer_norm:
+ self.norm1 = nn.LayerNorm((in_channels // reduction, 1, 1))
+ self.relu = nn.ReLU(inplace=True)
+ self.fc2 = nn.Conv2d(
+ in_channels // reduction,
+ num_gates,
+ kernel_size=1,
+ bias=True,
+ padding=0
+ )
+ if gate_activation == 'sigmoid':
+ self.gate_activation = nn.Sigmoid()
+ elif gate_activation == 'relu':
+ self.gate_activation = nn.ReLU(inplace=True)
+ elif gate_activation == 'linear':
+ self.gate_activation = None
+ else:
+ raise RuntimeError(
+ "Unknown gate activation: {}".format(gate_activation)
+ )
+
+ def forward(self, x):
+ input = x
+ x = self.global_avgpool(x)
+ x = self.fc1(x)
+ if self.norm1 is not None:
+ x = self.norm1(x)
+ x = self.relu(x)
+ x = self.fc2(x)
+ if self.gate_activation is not None:
+ x = self.gate_activation(x)
+ if self.return_gates:
+ return x
+ return input * x
+
+
+class OSBlock(nn.Module):
+ """Omni-scale feature learning block."""
+
+ def __init__(self, in_channels, out_channels, **kwargs):
+ super(OSBlock, self).__init__()
+ mid_channels = out_channels // 4
+ self.conv1 = Conv1x1(in_channels, mid_channels)
+ self.conv2a = LightConv3x3(mid_channels, mid_channels)
+ self.conv2b = nn.Sequential(
+ LightConv3x3(mid_channels, mid_channels),
+ LightConv3x3(mid_channels, mid_channels),
+ )
+ self.conv2c = nn.Sequential(
+ LightConv3x3(mid_channels, mid_channels),
+ LightConv3x3(mid_channels, mid_channels),
+ LightConv3x3(mid_channels, mid_channels),
+ )
+ self.conv2d = nn.Sequential(
+ LightConv3x3(mid_channels, mid_channels),
+ LightConv3x3(mid_channels, mid_channels),
+ LightConv3x3(mid_channels, mid_channels),
+ LightConv3x3(mid_channels, mid_channels),
+ )
+ self.gate = ChannelGate(mid_channels)
+ self.conv3 = Conv1x1Linear(mid_channels, out_channels)
+ self.downsample = None
+ if in_channels != out_channels:
+ self.downsample = Conv1x1Linear(in_channels, out_channels)
+
+ def forward(self, x):
+ residual = x
+ x1 = self.conv1(x)
+ x2a = self.conv2a(x1)
+ x2b = self.conv2b(x1)
+ x2c = self.conv2c(x1)
+ x2d = self.conv2d(x1)
+ x2 = self.gate(x2a) + self.gate(x2b) + self.gate(x2c) + self.gate(x2d)
+ x3 = self.conv3(x2)
+ if self.downsample is not None:
+ residual = self.downsample(residual)
+ out = x3 + residual
+ return F.relu(out)
+
+
+##########
+# Network architecture
+##########
+class BaseNet(nn.Module):
+
+ def _make_layer(
+ self, block, layer, in_channels, out_channels, reduce_spatial_size
+ ):
+ layers = []
+
+ layers.append(block(in_channels, out_channels))
+ for i in range(1, layer):
+ layers.append(block(out_channels, out_channels))
+
+ if reduce_spatial_size:
+ layers.append(
+ nn.Sequential(
+ Conv1x1(out_channels, out_channels),
+ nn.AvgPool2d(2, stride=2)
+ )
+ )
+
+ return nn.Sequential(*layers)
+
+ def _construct_fc_layer(self, fc_dims, input_dim, dropout_p=None):
+ if fc_dims is None or fc_dims < 0:
+ self.feature_dim = input_dim
+ return None
+
+ if isinstance(fc_dims, int):
+ fc_dims = [fc_dims]
+
+ layers = []
+ for dim in fc_dims:
+ layers.append(nn.Linear(input_dim, dim))
+ layers.append(nn.BatchNorm1d(dim))
+ layers.append(nn.ReLU(inplace=True))
+ if dropout_p is not None:
+ layers.append(nn.Dropout(p=dropout_p))
+ input_dim = dim
+
+ self.feature_dim = fc_dims[-1]
+
+ return nn.Sequential(*layers)
+
+ def init_params(self):
+ for m in self.modules():
+ if isinstance(m, nn.Conv2d):
+ nn.init.kaiming_normal_(
+ m.weight, mode='fan_out', nonlinearity='relu'
+ )
+ if m.bias is not None:
+ nn.init.constant_(m.bias, 0)
+
+ elif isinstance(m, nn.BatchNorm2d):
+ nn.init.constant_(m.weight, 1)
+ nn.init.constant_(m.bias, 0)
+
+ elif isinstance(m, nn.BatchNorm1d):
+ nn.init.constant_(m.weight, 1)
+ nn.init.constant_(m.bias, 0)
+
+ elif isinstance(m, nn.Linear):
+ nn.init.normal_(m.weight, 0, 0.01)
+ if m.bias is not None:
+ nn.init.constant_(m.bias, 0)
+
+
+class OSNet(BaseNet):
+
+ def __init__(
+ self,
+ num_classes,
+ blocks,
+ layers,
+ channels,
+ feature_dim=512,
+ loss='softmax',
+ pool='avg',
+ **kwargs
+ ):
+ super(OSNet, self).__init__()
+ num_blocks = len(blocks)
+ assert num_blocks == len(layers)
+ assert num_blocks == len(channels) - 1
+ self.loss = loss
+
+ # convolutional backbone
+ self.conv1 = ConvLayer(3, channels[0], 7, stride=2, padding=3)
+ self.maxpool = nn.MaxPool2d(3, stride=2, padding=1)
+ self.conv2 = self._make_layer(
+ blocks[0],
+ layers[0],
+ channels[0],
+ channels[1],
+ reduce_spatial_size=True
+ )
+ self.conv3 = self._make_layer(
+ blocks[1],
+ layers[1],
+ channels[1],
+ channels[2],
+ reduce_spatial_size=True
+ )
+ self.conv4 = self._make_layer(
+ blocks[2],
+ layers[2],
+ channels[2],
+ channels[3],
+ reduce_spatial_size=False
+ )
+ self.conv5 = Conv1x1(channels[3], channels[3])
+ if pool == 'avg':
+ self.global_pool = nn.AdaptiveAvgPool2d(1)
+ else:
+ self.global_pool = nn.AdaptiveMaxPool2d(1)
+ # fully connected layer
+ self.fc = self._construct_fc_layer(
+ feature_dim, channels[3], dropout_p=None
+ )
+ # classification layer
+ self.classifier = nn.Linear(self.feature_dim, num_classes)
+
+ self.init_params()
+
+ def featuremaps(self, x):
+ x = self.conv1(x)
+ x = self.maxpool(x)
+ x = self.conv2(x)
+ x = self.conv3(x)
+ x = self.conv4(x)
+ x = self.conv5(x)
+ return x
+
+ def forward(self, x):
+ x = self.featuremaps(x)
+ v = self.global_pool(x)
+ v = v.view(v.size(0), -1)
+ if self.fc is not None:
+ v = self.fc(v)
+ y = self.classifier(v)
+ if not self.training:
+ y = torch.sigmoid(y)
+ return y
+
+
+def osnet_avgpool(num_classes=1000, loss='softmax', **kwargs):
+ return OSNet(
+ num_classes,
+ blocks=[OSBlock, OSBlock, OSBlock],
+ layers=[2, 2, 2],
+ channels=[64, 256, 384, 512],
+ loss=loss,
+ pool='avg',
+ **kwargs
+ )
+
+
+def osnet_maxpool(num_classes=1000, loss='softmax', **kwargs):
+ return OSNet(
+ num_classes,
+ blocks=[OSBlock, OSBlock, OSBlock],
+ layers=[2, 2, 2],
+ channels=[64, 256, 384, 512],
+ loss=loss,
+ pool='max',
+ **kwargs
+ )
diff --git a/trackers/strong_sort/deep/reid/projects/attribute_recognition/train.sh b/trackers/strong_sort/deep/reid/projects/attribute_recognition/train.sh
new file mode 100644
index 0000000000000000000000000000000000000000..9080a66786671b6bf869a5ed3ed574432acf7fb7
--- /dev/null
+++ b/trackers/strong_sort/deep/reid/projects/attribute_recognition/train.sh
@@ -0,0 +1,16 @@
+#!/bin/bash
+
+# DATASET points to the directory containing pa100k/
+DATASET=$1
+
+python main.py \
+--root ${DATASET} \
+-d pa100k \
+-a osnet_maxpool \
+--max-epoch 50 \
+--stepsize 30 40 \
+--batch-size 32 \
+--lr 0.065 \
+--optim sgd \
+--weighted-bce \
+--save-dir log/pa100k-osnet_maxpool
\ No newline at end of file
diff --git a/trackers/strong_sort/deep/reid/requirements.txt b/trackers/strong_sort/deep/reid/requirements.txt
new file mode 100644
index 0000000000000000000000000000000000000000..f3c94ffd838ee47525a65660882b2e504d48c5a1
--- /dev/null
+++ b/trackers/strong_sort/deep/reid/requirements.txt
@@ -0,0 +1,24 @@
+numpy
+Cython
+h5py
+Pillow
+six
+scipy
+opencv-python
+matplotlib
+tb-nightly
+future
+yacs
+gdown
+flake8
+yapf
+isort==4.3.21
+imageio
+chardet
+# Export --------------------------------------
+# onnx
+# onnx-simplified
+# openvino-dev
+# openvino2tensorflow
+# tensorflow
+# tensorflow_datasets
diff --git a/trackers/strong_sort/deep/reid/scripts/default_config.py b/trackers/strong_sort/deep/reid/scripts/default_config.py
new file mode 100644
index 0000000000000000000000000000000000000000..da448e3637521786d56482c4fa30f47f7c7f26cf
--- /dev/null
+++ b/trackers/strong_sort/deep/reid/scripts/default_config.py
@@ -0,0 +1,212 @@
+from yacs.config import CfgNode as CN
+
+
+def get_default_config():
+ cfg = CN()
+
+ # model
+ cfg.model = CN()
+ cfg.model.name = 'resnet50'
+ cfg.model.pretrained = True # automatically load pretrained model weights if available
+ cfg.model.load_weights = '' # path to model weights
+ cfg.model.resume = '' # path to checkpoint for resume training
+
+ # data
+ cfg.data = CN()
+ cfg.data.type = 'image'
+ cfg.data.root = 'reid-data'
+ cfg.data.sources = ['market1501']
+ cfg.data.targets = ['market1501']
+ cfg.data.workers = 4 # number of data loading workers
+ cfg.data.split_id = 0 # split index
+ cfg.data.height = 256 # image height
+ cfg.data.width = 128 # image width
+ cfg.data.combineall = False # combine train, query and gallery for training
+ cfg.data.transforms = ['random_flip'] # data augmentation
+ cfg.data.k_tfm = 1 # number of times to apply augmentation to an image independently
+ cfg.data.norm_mean = [0.485, 0.456, 0.406] # default is imagenet mean
+ cfg.data.norm_std = [0.229, 0.224, 0.225] # default is imagenet std
+ cfg.data.save_dir = 'log' # path to save log
+ cfg.data.load_train_targets = False # load training set from target dataset
+
+ # specific datasets
+ cfg.market1501 = CN()
+ cfg.market1501.use_500k_distractors = False # add 500k distractors to the gallery set for market1501
+ cfg.cuhk03 = CN()
+ cfg.cuhk03.labeled_images = False # use labeled images, if False, use detected images
+ cfg.cuhk03.classic_split = False # use classic split by Li et al. CVPR14
+ cfg.cuhk03.use_metric_cuhk03 = False # use cuhk03's metric for evaluation
+
+ # sampler
+ cfg.sampler = CN()
+ cfg.sampler.train_sampler = 'RandomSampler' # sampler for source train loader
+ cfg.sampler.train_sampler_t = 'RandomSampler' # sampler for target train loader
+ cfg.sampler.num_instances = 4 # number of instances per identity for RandomIdentitySampler
+ cfg.sampler.num_cams = 1 # number of cameras to sample in a batch (for RandomDomainSampler)
+ cfg.sampler.num_datasets = 1 # number of datasets to sample in a batch (for RandomDatasetSampler)
+
+ # video reid setting
+ cfg.video = CN()
+ cfg.video.seq_len = 15 # number of images to sample in a tracklet
+ cfg.video.sample_method = 'evenly' # how to sample images from a tracklet
+ cfg.video.pooling_method = 'avg' # how to pool features over a tracklet
+
+ # train
+ cfg.train = CN()
+ cfg.train.optim = 'adam'
+ cfg.train.lr = 0.0003
+ cfg.train.weight_decay = 5e-4
+ cfg.train.max_epoch = 60
+ cfg.train.start_epoch = 0
+ cfg.train.batch_size = 32
+ cfg.train.fixbase_epoch = 0 # number of epochs to fix base layers
+ cfg.train.open_layers = [
+ 'classifier'
+ ] # layers for training while keeping others frozen
+ cfg.train.staged_lr = False # set different lr to different layers
+ cfg.train.new_layers = ['classifier'] # newly added layers with default lr
+ cfg.train.base_lr_mult = 0.1 # learning rate multiplier for base layers
+ cfg.train.lr_scheduler = 'single_step'
+ cfg.train.stepsize = [20] # stepsize to decay learning rate
+ cfg.train.gamma = 0.1 # learning rate decay multiplier
+ cfg.train.print_freq = 20 # print frequency
+ cfg.train.seed = 1 # random seed
+
+ # optimizer
+ cfg.sgd = CN()
+ cfg.sgd.momentum = 0.9 # momentum factor for sgd and rmsprop
+ cfg.sgd.dampening = 0. # dampening for momentum
+ cfg.sgd.nesterov = False # Nesterov momentum
+ cfg.rmsprop = CN()
+ cfg.rmsprop.alpha = 0.99 # smoothing constant
+ cfg.adam = CN()
+ cfg.adam.beta1 = 0.9 # exponential decay rate for first moment
+ cfg.adam.beta2 = 0.999 # exponential decay rate for second moment
+
+ # loss
+ cfg.loss = CN()
+ cfg.loss.name = 'softmax'
+ cfg.loss.softmax = CN()
+ cfg.loss.softmax.label_smooth = True # use label smoothing regularizer
+ cfg.loss.triplet = CN()
+ cfg.loss.triplet.margin = 0.3 # distance margin
+ cfg.loss.triplet.weight_t = 1. # weight to balance hard triplet loss
+ cfg.loss.triplet.weight_x = 0. # weight to balance cross entropy loss
+
+ # test
+ cfg.test = CN()
+ cfg.test.batch_size = 100
+ cfg.test.dist_metric = 'euclidean' # distance metric, ['euclidean', 'cosine']
+ cfg.test.normalize_feature = False # normalize feature vectors before computing distance
+ cfg.test.ranks = [1, 5, 10, 20] # cmc ranks
+ cfg.test.evaluate = False # test only
+ cfg.test.eval_freq = -1 # evaluation frequency (-1 means to only test after training)
+ cfg.test.start_eval = 0 # start to evaluate after a specific epoch
+ cfg.test.rerank = False # use person re-ranking
+ cfg.test.visrank = False # visualize ranked results (only available when cfg.test.evaluate=True)
+ cfg.test.visrank_topk = 10 # top-k ranks to visualize
+
+ return cfg
+
+
+def imagedata_kwargs(cfg):
+ return {
+ 'root': cfg.data.root,
+ 'sources': cfg.data.sources,
+ 'targets': cfg.data.targets,
+ 'height': cfg.data.height,
+ 'width': cfg.data.width,
+ 'transforms': cfg.data.transforms,
+ 'k_tfm': cfg.data.k_tfm,
+ 'norm_mean': cfg.data.norm_mean,
+ 'norm_std': cfg.data.norm_std,
+ 'use_gpu': cfg.use_gpu,
+ 'split_id': cfg.data.split_id,
+ 'combineall': cfg.data.combineall,
+ 'load_train_targets': cfg.data.load_train_targets,
+ 'batch_size_train': cfg.train.batch_size,
+ 'batch_size_test': cfg.test.batch_size,
+ 'workers': cfg.data.workers,
+ 'num_instances': cfg.sampler.num_instances,
+ 'num_cams': cfg.sampler.num_cams,
+ 'num_datasets': cfg.sampler.num_datasets,
+ 'train_sampler': cfg.sampler.train_sampler,
+ 'train_sampler_t': cfg.sampler.train_sampler_t,
+ # image dataset specific
+ 'cuhk03_labeled': cfg.cuhk03.labeled_images,
+ 'cuhk03_classic_split': cfg.cuhk03.classic_split,
+ 'market1501_500k': cfg.market1501.use_500k_distractors,
+ }
+
+
+def videodata_kwargs(cfg):
+ return {
+ 'root': cfg.data.root,
+ 'sources': cfg.data.sources,
+ 'targets': cfg.data.targets,
+ 'height': cfg.data.height,
+ 'width': cfg.data.width,
+ 'transforms': cfg.data.transforms,
+ 'norm_mean': cfg.data.norm_mean,
+ 'norm_std': cfg.data.norm_std,
+ 'use_gpu': cfg.use_gpu,
+ 'split_id': cfg.data.split_id,
+ 'combineall': cfg.data.combineall,
+ 'batch_size_train': cfg.train.batch_size,
+ 'batch_size_test': cfg.test.batch_size,
+ 'workers': cfg.data.workers,
+ 'num_instances': cfg.sampler.num_instances,
+ 'num_cams': cfg.sampler.num_cams,
+ 'num_datasets': cfg.sampler.num_datasets,
+ 'train_sampler': cfg.sampler.train_sampler,
+ # video dataset specific
+ 'seq_len': cfg.video.seq_len,
+ 'sample_method': cfg.video.sample_method
+ }
+
+
+def optimizer_kwargs(cfg):
+ return {
+ 'optim': cfg.train.optim,
+ 'lr': cfg.train.lr,
+ 'weight_decay': cfg.train.weight_decay,
+ 'momentum': cfg.sgd.momentum,
+ 'sgd_dampening': cfg.sgd.dampening,
+ 'sgd_nesterov': cfg.sgd.nesterov,
+ 'rmsprop_alpha': cfg.rmsprop.alpha,
+ 'adam_beta1': cfg.adam.beta1,
+ 'adam_beta2': cfg.adam.beta2,
+ 'staged_lr': cfg.train.staged_lr,
+ 'new_layers': cfg.train.new_layers,
+ 'base_lr_mult': cfg.train.base_lr_mult
+ }
+
+
+def lr_scheduler_kwargs(cfg):
+ return {
+ 'lr_scheduler': cfg.train.lr_scheduler,
+ 'stepsize': cfg.train.stepsize,
+ 'gamma': cfg.train.gamma,
+ 'max_epoch': cfg.train.max_epoch
+ }
+
+
+def engine_run_kwargs(cfg):
+ return {
+ 'save_dir': cfg.data.save_dir,
+ 'max_epoch': cfg.train.max_epoch,
+ 'start_epoch': cfg.train.start_epoch,
+ 'fixbase_epoch': cfg.train.fixbase_epoch,
+ 'open_layers': cfg.train.open_layers,
+ 'start_eval': cfg.test.start_eval,
+ 'eval_freq': cfg.test.eval_freq,
+ 'test_only': cfg.test.evaluate,
+ 'print_freq': cfg.train.print_freq,
+ 'dist_metric': cfg.test.dist_metric,
+ 'normalize_feature': cfg.test.normalize_feature,
+ 'visrank': cfg.test.visrank,
+ 'visrank_topk': cfg.test.visrank_topk,
+ 'use_metric_cuhk03': cfg.cuhk03.use_metric_cuhk03,
+ 'ranks': cfg.test.ranks,
+ 'rerank': cfg.test.rerank
+ }
diff --git a/trackers/strong_sort/deep/reid/scripts/main.py b/trackers/strong_sort/deep/reid/scripts/main.py
new file mode 100644
index 0000000000000000000000000000000000000000..61aa49ddd3cb8ba9c9bf1e4c281b765b8d971c10
--- /dev/null
+++ b/trackers/strong_sort/deep/reid/scripts/main.py
@@ -0,0 +1,191 @@
+import sys
+import time
+import os.path as osp
+import argparse
+import torch
+import torch.nn as nn
+
+import torchreid
+from torchreid.utils import (
+ Logger, check_isfile, set_random_seed, collect_env_info,
+ resume_from_checkpoint, load_pretrained_weights, compute_model_complexity
+)
+
+from default_config import (
+ imagedata_kwargs, optimizer_kwargs, videodata_kwargs, engine_run_kwargs,
+ get_default_config, lr_scheduler_kwargs
+)
+
+
+def build_datamanager(cfg):
+ if cfg.data.type == 'image':
+ return torchreid.data.ImageDataManager(**imagedata_kwargs(cfg))
+ else:
+ return torchreid.data.VideoDataManager(**videodata_kwargs(cfg))
+
+
+def build_engine(cfg, datamanager, model, optimizer, scheduler):
+ if cfg.data.type == 'image':
+ if cfg.loss.name == 'softmax':
+ engine = torchreid.engine.ImageSoftmaxEngine(
+ datamanager,
+ model,
+ optimizer=optimizer,
+ scheduler=scheduler,
+ use_gpu=cfg.use_gpu,
+ label_smooth=cfg.loss.softmax.label_smooth
+ )
+
+ else:
+ engine = torchreid.engine.ImageTripletEngine(
+ datamanager,
+ model,
+ optimizer=optimizer,
+ margin=cfg.loss.triplet.margin,
+ weight_t=cfg.loss.triplet.weight_t,
+ weight_x=cfg.loss.triplet.weight_x,
+ scheduler=scheduler,
+ use_gpu=cfg.use_gpu,
+ label_smooth=cfg.loss.softmax.label_smooth
+ )
+
+ else:
+ if cfg.loss.name == 'softmax':
+ engine = torchreid.engine.VideoSoftmaxEngine(
+ datamanager,
+ model,
+ optimizer=optimizer,
+ scheduler=scheduler,
+ use_gpu=cfg.use_gpu,
+ label_smooth=cfg.loss.softmax.label_smooth,
+ pooling_method=cfg.video.pooling_method
+ )
+
+ else:
+ engine = torchreid.engine.VideoTripletEngine(
+ datamanager,
+ model,
+ optimizer=optimizer,
+ margin=cfg.loss.triplet.margin,
+ weight_t=cfg.loss.triplet.weight_t,
+ weight_x=cfg.loss.triplet.weight_x,
+ scheduler=scheduler,
+ use_gpu=cfg.use_gpu,
+ label_smooth=cfg.loss.softmax.label_smooth
+ )
+
+ return engine
+
+
+def reset_config(cfg, args):
+ if args.root:
+ cfg.data.root = args.root
+ if args.sources:
+ cfg.data.sources = args.sources
+ if args.targets:
+ cfg.data.targets = args.targets
+ if args.transforms:
+ cfg.data.transforms = args.transforms
+
+
+def check_cfg(cfg):
+ if cfg.loss.name == 'triplet' and cfg.loss.triplet.weight_x == 0:
+ assert cfg.train.fixbase_epoch == 0, \
+ 'The output of classifier is not included in the computational graph'
+
+
+def main():
+ parser = argparse.ArgumentParser(
+ formatter_class=argparse.ArgumentDefaultsHelpFormatter
+ )
+ parser.add_argument(
+ '--config-file', type=str, default='', help='path to config file'
+ )
+ parser.add_argument(
+ '-s',
+ '--sources',
+ type=str,
+ nargs='+',
+ help='source datasets (delimited by space)'
+ )
+ parser.add_argument(
+ '-t',
+ '--targets',
+ type=str,
+ nargs='+',
+ help='target datasets (delimited by space)'
+ )
+ parser.add_argument(
+ '--transforms', type=str, nargs='+', help='data augmentation'
+ )
+ parser.add_argument(
+ '--root', type=str, default='', help='path to data root'
+ )
+ parser.add_argument(
+ 'opts',
+ default=None,
+ nargs=argparse.REMAINDER,
+ help='Modify config options using the command-line'
+ )
+ args = parser.parse_args()
+
+ cfg = get_default_config()
+ cfg.use_gpu = torch.cuda.is_available()
+ if args.config_file:
+ cfg.merge_from_file(args.config_file)
+ reset_config(cfg, args)
+ cfg.merge_from_list(args.opts)
+ set_random_seed(cfg.train.seed)
+ check_cfg(cfg)
+
+ log_name = 'test.log' if cfg.test.evaluate else 'train.log'
+ log_name += time.strftime('-%Y-%m-%d-%H-%M-%S')
+ sys.stdout = Logger(osp.join(cfg.data.save_dir, log_name))
+
+ print('Show configuration\n{}\n'.format(cfg))
+ print('Collecting env info ...')
+ print('** System info **\n{}\n'.format(collect_env_info()))
+
+ if cfg.use_gpu:
+ torch.backends.cudnn.benchmark = True
+
+ datamanager = build_datamanager(cfg)
+
+ print('Building model: {}'.format(cfg.model.name))
+ model = torchreid.models.build_model(
+ name=cfg.model.name,
+ num_classes=datamanager.num_train_pids,
+ loss=cfg.loss.name,
+ pretrained=cfg.model.pretrained,
+ use_gpu=cfg.use_gpu
+ )
+ num_params, flops = compute_model_complexity(
+ model, (1, 3, cfg.data.height, cfg.data.width)
+ )
+ print('Model complexity: params={:,} flops={:,}'.format(num_params, flops))
+
+ if cfg.model.load_weights and check_isfile(cfg.model.load_weights):
+ load_pretrained_weights(model, cfg.model.load_weights)
+
+ if cfg.use_gpu:
+ model = nn.DataParallel(model).cuda()
+
+ optimizer = torchreid.optim.build_optimizer(model, **optimizer_kwargs(cfg))
+ scheduler = torchreid.optim.build_lr_scheduler(
+ optimizer, **lr_scheduler_kwargs(cfg)
+ )
+
+ if cfg.model.resume and check_isfile(cfg.model.resume):
+ cfg.train.start_epoch = resume_from_checkpoint(
+ cfg.model.resume, model, optimizer=optimizer, scheduler=scheduler
+ )
+
+ print(
+ 'Building {}-engine for {}-reid'.format(cfg.loss.name, cfg.data.type)
+ )
+ engine = build_engine(cfg, datamanager, model, optimizer, scheduler)
+ engine.run(**engine_run_kwargs(cfg))
+
+
+if __name__ == '__main__':
+ main()
diff --git a/trackers/strong_sort/deep/reid/setup.py b/trackers/strong_sort/deep/reid/setup.py
new file mode 100644
index 0000000000000000000000000000000000000000..a8ee83e8fa28b7bfbde1ed817d1bb2c4f57c33f3
--- /dev/null
+++ b/trackers/strong_sort/deep/reid/setup.py
@@ -0,0 +1,57 @@
+import numpy as np
+import os.path as osp
+from setuptools import setup, find_packages
+from distutils.extension import Extension
+from Cython.Build import cythonize
+
+
+def readme():
+ with open('README.rst') as f:
+ content = f.read()
+ return content
+
+
+def find_version():
+ version_file = 'torchreid/__init__.py'
+ with open(version_file, 'r') as f:
+ exec(compile(f.read(), version_file, 'exec'))
+ return locals()['__version__']
+
+
+def numpy_include():
+ try:
+ numpy_include = np.get_include()
+ except AttributeError:
+ numpy_include = np.get_numpy_include()
+ return numpy_include
+
+
+ext_modules = [
+ Extension(
+ 'torchreid.metrics.rank_cylib.rank_cy',
+ ['torchreid/metrics/rank_cylib/rank_cy.pyx'],
+ include_dirs=[numpy_include()],
+ )
+]
+
+
+def get_requirements(filename='requirements.txt'):
+ here = osp.dirname(osp.realpath(__file__))
+ with open(osp.join(here, filename), 'r') as f:
+ requires = [line.replace('\n', '') for line in f.readlines()]
+ return requires
+
+
+setup(
+ name='torchreid',
+ version=find_version(),
+ description='A library for deep learning person re-ID in PyTorch',
+ author='Kaiyang Zhou',
+ license='MIT',
+ long_description=readme(),
+ url='https://github.com/KaiyangZhou/deep-person-reid',
+ packages=find_packages(),
+ install_requires=get_requirements(),
+ keywords=['Person Re-Identification', 'Deep Learning', 'Computer Vision'],
+ ext_modules=cythonize(ext_modules)
+)
diff --git a/trackers/strong_sort/deep/reid/tools/compute_mean_std.py b/trackers/strong_sort/deep/reid/tools/compute_mean_std.py
new file mode 100644
index 0000000000000000000000000000000000000000..e0a5dbe8dfa746c164b951908fb0b105e4cadbfc
--- /dev/null
+++ b/trackers/strong_sort/deep/reid/tools/compute_mean_std.py
@@ -0,0 +1,59 @@
+"""
+Compute channel-wise mean and standard deviation of a dataset.
+
+Usage:
+$ python compute_mean_std.py DATASET_ROOT DATASET_KEY
+
+- The first argument points to the root path where you put the datasets.
+- The second argument means the specific dataset key.
+
+For instance, your datasets are put under $DATA and you wanna
+compute the statistics of Market1501, do
+$ python compute_mean_std.py $DATA market1501
+"""
+import argparse
+
+import torchreid
+
+
+def main():
+ parser = argparse.ArgumentParser()
+ parser.add_argument('root', type=str)
+ parser.add_argument('sources', type=str)
+ args = parser.parse_args()
+
+ datamanager = torchreid.data.ImageDataManager(
+ root=args.root,
+ sources=args.sources,
+ targets=None,
+ height=256,
+ width=128,
+ batch_size_train=100,
+ batch_size_test=100,
+ transforms=None,
+ norm_mean=[0., 0., 0.],
+ norm_std=[1., 1., 1.],
+ train_sampler='SequentialSampler'
+ )
+ train_loader = datamanager.train_loader
+
+ print('Computing mean and std ...')
+ mean = 0.
+ std = 0.
+ n_samples = 0.
+ for data in train_loader:
+ data = data['img']
+ batch_size = data.size(0)
+ data = data.view(batch_size, data.size(1), -1)
+ mean += data.mean(2).sum(0)
+ std += data.std(2).sum(0)
+ n_samples += batch_size
+
+ mean /= n_samples
+ std /= n_samples
+ print('Mean: {}'.format(mean))
+ print('Std: {}'.format(std))
+
+
+if __name__ == '__main__':
+ main()
diff --git a/trackers/strong_sort/deep/reid/tools/export.py b/trackers/strong_sort/deep/reid/tools/export.py
new file mode 100644
index 0000000000000000000000000000000000000000..28dfde283408c9a35f576007849bda369b1e1c8d
--- /dev/null
+++ b/trackers/strong_sort/deep/reid/tools/export.py
@@ -0,0 +1,204 @@
+import argparse
+import os
+import sys
+import numpy as np
+from pathlib import Path
+import torch
+import pandas as pd
+import subprocess
+
+from torchreid.utils.feature_extractor import FeatureExtractor
+from torchreid.models import build_model
+
+__model_types = [
+ 'resnet50', 'mlfn', 'hacnn', 'mobilenetv2_x1_0', 'mobilenetv2_x1_4',
+ 'osnet_x1_0', 'osnet_x0_75', 'osnet_x0_5', 'osnet_x0_25',
+ 'osnet_ibn_x1_0', 'osnet_ain_x1_0']
+
+def file_size(path):
+ # Return file/dir size (MB)
+ path = Path(path)
+ if path.is_file():
+ return path.stat().st_size / 1E6
+ elif path.is_dir():
+ return sum(f.stat().st_size for f in path.glob('**/*') if f.is_file()) / 1E6
+ else:
+ return 0.0
+
+
+def get_model_name(model):
+ model = str(model).rsplit('/', 1)[-1].split('.')[0]
+ for x in __model_types:
+ if x in model:
+ return x
+ return None
+
+def export_formats():
+ # YOLOv5 export formats
+ x = [
+ ['PyTorch', '-', '.pt', True, True],
+ ['ONNX', 'onnx', '.onnx', True, True],
+ ['OpenVINO', 'openvino', '_openvino_model', True, False],
+ ['TensorFlow Lite', 'tflite', '.tflite', True, False],
+ ]
+ return pd.DataFrame(x, columns=['Format', 'Argument', 'Suffix', 'CPU', 'GPU'])
+
+
+def export_onnx(model, im, file, opset, train=False, dynamic=True, simplify=False):
+ # ONNX export
+ try:
+ import onnx
+
+ f = file.with_suffix('.onnx')
+ print(f'\nStarting export with onnx {onnx.__version__}...')
+
+ torch.onnx.export(
+ model.cpu() if dynamic else model, # --dynamic only compatible with cpu
+ im.cpu() if dynamic else im,
+ f,
+ verbose=False,
+ opset_version=opset,
+ training=torch.onnx.TrainingMode.TRAINING if train else torch.onnx.TrainingMode.EVAL,
+ do_constant_folding=not train,
+ input_names=['images'],
+ output_names=['output'],
+ dynamic_axes={
+ 'images': {
+ 0: 'batch',
+ }, # shape(x,3,256,128)
+ 'output': {
+ 0: 'batch',
+ } # shape(x,2048)
+ } if dynamic else None
+ )
+ # Checks
+ model_onnx = onnx.load(f) # load onnx model
+ onnx.checker.check_model(model_onnx) # check onnx model
+ onnx.save(model_onnx, f)
+
+ # Simplify
+ if simplify:
+ try:
+ cuda = torch.cuda.is_available()
+ import onnxsim
+
+ print(f'simplifying with onnx-simplifier {onnxsim.__version__}...')
+ model_onnx, check = onnxsim.simplify(
+ model_onnx,
+ dynamic_input_shape=dynamic,
+ input_shapes={'t0': list(im.shape)} if dynamic else None)
+ assert check, 'assert check failed'
+ onnx.save(model_onnx, f)
+ except Exception as e:
+ print(f'simplifier failure: {e}')
+ print(f'export success, saved as {f} ({file_size(f):.1f} MB)')
+ print(f"run --dynamic ONNX model inference with: 'python detect.py --weights {f}'")
+ except Exception as e:
+ print(f'export failure: {e}')
+ return f
+
+
+def export_openvino(file, dynamic, half):
+ f = str(file).replace('.onnx', f'_openvino_model{os.sep}')
+ # YOLOv5 OpenVINO export
+ try:
+ import openvino.inference_engine as ie
+
+ print(f'\nStarting export with openvino {ie.__version__}...')
+ f = str(file).replace('.onnx', f'_openvino_model{os.sep}')
+ dyn_shape = [-1,3,256,128] if dynamic else None
+ cmd = f"mo \
+ --input_model {file} \
+ --output_dir {f} \
+ --data_type {'FP16' if half else 'FP32'}"
+
+ if dyn_shape is not None:
+ cmd + f"--input_shape {dyn_shape}"
+
+ subprocess.check_output(cmd.split()) # export
+
+ print(f'Export success, saved as {f} ({file_size(f):.1f} MB)')
+ return f
+ except Exception as e:
+ print(f'\nExport failure: {e}')
+ return f
+
+
+def export_tflite(file, half):
+ # YOLOv5 OpenVINO export
+ try:
+ import openvino.inference_engine as ie
+ print(f'\nStarting export with openvino {ie.__version__}...')
+ output = Path(str(file).replace(f'_openvino_model{os.sep}', f'_tflite_model{os.sep}'))
+ modelxml = list(Path(file).glob('*.xml'))[0]
+ cmd = f"openvino2tensorflow \
+ --model_path {modelxml} \
+ --model_output_path {output} \
+ --output_pb \
+ --output_saved_model \
+ --output_no_quant_float32_tflite \
+ --output_dynamic_range_quant_tflite"
+ subprocess.check_output(cmd.split()) # export
+
+ print(f'Export success, results saved in {output} ({file_size(f):.1f} MB)')
+ return f
+ except Exception as e:
+ print(f'\nExport failure: {e}')
+
+
+if __name__ == "__main__":
+
+ parser = argparse.ArgumentParser(description="CPHD train")
+ parser.add_argument(
+ "-d",
+ "--dynamic",
+ action="store_true",
+ help="dynamic model input",
+ )
+ parser.add_argument(
+ "-p",
+ "--weights",
+ type=Path,
+ default="./mobilenetv2_x1_0_msmt17.pt",
+ help="Path to weights",
+ )
+ parser.add_argument(
+ "-hp",
+ "--half_precision",
+ action="store_true",
+ help="transform model to half precision",
+ )
+ parser.add_argument(
+ '--imgsz', '--img', '--img-size',
+ nargs='+',
+ type=int,
+ default=[256, 128],
+ help='image (h, w)'
+ )
+ parser.add_argument('--include',
+ nargs='+',
+ default=['onnx', 'openvino', 'tflite'],
+ help='onnx, openvino, tflite')
+ args = parser.parse_args()
+
+ # Build model
+ extractor = FeatureExtractor(
+ # get rid of dataset information DeepSort model name
+ model_name=get_model_name(args.weights),
+ model_path=args.weights,
+ device=str('cpu')
+ )
+
+ include = [x.lower() for x in args.include] # to lowercase
+ fmts = tuple(export_formats()['Argument'][1:]) # --include arguments
+ flags = [x in include for x in fmts]
+ assert sum(flags) == len(include), f'ERROR: Invalid --include {include}, valid --include arguments are {fmts}'
+ onnx, openvino, tflite = flags # export booleans
+
+ im = torch.zeros(1, 3, args.imgsz[0], args.imgsz[1]).to('cpu') # image size(1,3,640,480) BCHW iDetection
+ if onnx:
+ f = export_onnx(extractor.model.eval(), im, args.weights, 12, train=False, dynamic=args.dynamic, simplify=True) # opset 12
+ if openvino:
+ f = export_openvino(f, dynamic=args.dynamic, half=False)
+ if tflite:
+ export_tflite(f, False)
diff --git a/trackers/strong_sort/deep/reid/tools/parse_test_res.py b/trackers/strong_sort/deep/reid/tools/parse_test_res.py
new file mode 100644
index 0000000000000000000000000000000000000000..fd5b0189d4ef130b0e772d483cf4c1e5643dff06
--- /dev/null
+++ b/trackers/strong_sort/deep/reid/tools/parse_test_res.py
@@ -0,0 +1,103 @@
+"""
+This script aims to automate the process of calculating average results
+stored in the test.log files over multiple splits.
+
+How to use:
+For example, you have done evaluation over 20 splits on VIPeR, leading to
+the following file structure
+
+log/
+ eval_viper/
+ split_0/
+ test.log-xxxx
+ split_1/
+ test.log-xxxx
+ split_2/
+ test.log-xxxx
+ ...
+
+You can run the following command in your terminal to get the average performance:
+$ python tools/parse_test_res.py log/eval_viper
+"""
+import os
+import re
+import glob
+import numpy as np
+import argparse
+from collections import defaultdict
+
+from torchreid.utils import check_isfile, listdir_nohidden
+
+
+def parse_file(filepath, regex_mAP, regex_r1, regex_r5, regex_r10, regex_r20):
+ results = {}
+
+ with open(filepath, 'r') as f:
+ lines = f.readlines()
+
+ for line in lines:
+ line = line.strip()
+
+ match_mAP = regex_mAP.search(line)
+ if match_mAP:
+ mAP = float(match_mAP.group(1))
+ results['mAP'] = mAP
+
+ match_r1 = regex_r1.search(line)
+ if match_r1:
+ r1 = float(match_r1.group(1))
+ results['r1'] = r1
+
+ match_r5 = regex_r5.search(line)
+ if match_r5:
+ r5 = float(match_r5.group(1))
+ results['r5'] = r5
+
+ match_r10 = regex_r10.search(line)
+ if match_r10:
+ r10 = float(match_r10.group(1))
+ results['r10'] = r10
+
+ match_r20 = regex_r20.search(line)
+ if match_r20:
+ r20 = float(match_r20.group(1))
+ results['r20'] = r20
+
+ return results
+
+
+def main(args):
+ regex_mAP = re.compile(r'mAP: ([\.\deE+-]+)%')
+ regex_r1 = re.compile(r'Rank-1 : ([\.\deE+-]+)%')
+ regex_r5 = re.compile(r'Rank-5 : ([\.\deE+-]+)%')
+ regex_r10 = re.compile(r'Rank-10 : ([\.\deE+-]+)%')
+ regex_r20 = re.compile(r'Rank-20 : ([\.\deE+-]+)%')
+
+ final_res = defaultdict(list)
+
+ directories = listdir_nohidden(args.directory, sort=True)
+ num_dirs = len(directories)
+ for directory in directories:
+ fullpath = os.path.join(args.directory, directory)
+ filepath = glob.glob(os.path.join(fullpath, 'test.log*'))[0]
+ check_isfile(filepath)
+ print(f'Parsing {filepath}')
+ res = parse_file(
+ filepath, regex_mAP, regex_r1, regex_r5, regex_r10, regex_r20
+ )
+ for key, value in res.items():
+ final_res[key].append(value)
+
+ print('Finished parsing')
+ print(f'The average results over {num_dirs} splits are shown below')
+
+ for key, values in final_res.items():
+ mean_val = np.mean(values)
+ print(f'{key}: {mean_val:.1f}')
+
+
+if __name__ == '__main__':
+ parser = argparse.ArgumentParser()
+ parser.add_argument('directory', type=str, help='Path to directory')
+ args = parser.parse_args()
+ main(args)
diff --git a/trackers/strong_sort/deep/reid/tools/visualize_actmap.py b/trackers/strong_sort/deep/reid/tools/visualize_actmap.py
new file mode 100644
index 0000000000000000000000000000000000000000..ae699913caf2431f96da6584b7ddc57ac6243214
--- /dev/null
+++ b/trackers/strong_sort/deep/reid/tools/visualize_actmap.py
@@ -0,0 +1,173 @@
+"""Visualizes CNN activation maps to see where the CNN focuses on to extract features.
+
+Reference:
+ - Zagoruyko and Komodakis. Paying more attention to attention: Improving the
+ performance of convolutional neural networks via attention transfer. ICLR, 2017
+ - Zhou et al. Omni-Scale Feature Learning for Person Re-Identification. ICCV, 2019.
+"""
+import numpy as np
+import os.path as osp
+import argparse
+import cv2
+import torch
+from torch.nn import functional as F
+
+import torchreid
+from torchreid.utils import (
+ check_isfile, mkdir_if_missing, load_pretrained_weights
+)
+
+IMAGENET_MEAN = [0.485, 0.456, 0.406]
+IMAGENET_STD = [0.229, 0.224, 0.225]
+GRID_SPACING = 10
+
+
+@torch.no_grad()
+def visactmap(
+ model,
+ test_loader,
+ save_dir,
+ width,
+ height,
+ use_gpu,
+ img_mean=None,
+ img_std=None
+):
+ if img_mean is None or img_std is None:
+ # use imagenet mean and std
+ img_mean = IMAGENET_MEAN
+ img_std = IMAGENET_STD
+
+ model.eval()
+
+ for target in list(test_loader.keys()):
+ data_loader = test_loader[target]['query'] # only process query images
+ # original images and activation maps are saved individually
+ actmap_dir = osp.join(save_dir, 'actmap_' + target)
+ mkdir_if_missing(actmap_dir)
+ print('Visualizing activation maps for {} ...'.format(target))
+
+ for batch_idx, data in enumerate(data_loader):
+ imgs, paths = data['img'], data['impath']
+ if use_gpu:
+ imgs = imgs.cuda()
+
+ # forward to get convolutional feature maps
+ try:
+ outputs = model(imgs, return_featuremaps=True)
+ except TypeError:
+ raise TypeError(
+ 'forward() got unexpected keyword argument "return_featuremaps". '
+ 'Please add return_featuremaps as an input argument to forward(). When '
+ 'return_featuremaps=True, return feature maps only.'
+ )
+
+ if outputs.dim() != 4:
+ raise ValueError(
+ 'The model output is supposed to have '
+ 'shape of (b, c, h, w), i.e. 4 dimensions, but got {} dimensions. '
+ 'Please make sure you set the model output at eval mode '
+ 'to be the last convolutional feature maps'.format(
+ outputs.dim()
+ )
+ )
+
+ # compute activation maps
+ outputs = (outputs**2).sum(1)
+ b, h, w = outputs.size()
+ outputs = outputs.view(b, h * w)
+ outputs = F.normalize(outputs, p=2, dim=1)
+ outputs = outputs.view(b, h, w)
+
+ if use_gpu:
+ imgs, outputs = imgs.cpu(), outputs.cpu()
+
+ for j in range(outputs.size(0)):
+ # get image name
+ path = paths[j]
+ imname = osp.basename(osp.splitext(path)[0])
+
+ # RGB image
+ img = imgs[j, ...]
+ for t, m, s in zip(img, img_mean, img_std):
+ t.mul_(s).add_(m).clamp_(0, 1)
+ img_np = np.uint8(np.floor(img.numpy() * 255))
+ img_np = img_np.transpose((1, 2, 0)) # (c, h, w) -> (h, w, c)
+
+ # activation map
+ am = outputs[j, ...].numpy()
+ am = cv2.resize(am, (width, height))
+ am = 255 * (am - np.min(am)) / (
+ np.max(am) - np.min(am) + 1e-12
+ )
+ am = np.uint8(np.floor(am))
+ am = cv2.applyColorMap(am, cv2.COLORMAP_JET)
+
+ # overlapped
+ overlapped = img_np*0.3 + am*0.7
+ overlapped[overlapped > 255] = 255
+ overlapped = overlapped.astype(np.uint8)
+
+ # save images in a single figure (add white spacing between images)
+ # from left to right: original image, activation map, overlapped image
+ grid_img = 255 * np.ones(
+ (height, 3*width + 2*GRID_SPACING, 3), dtype=np.uint8
+ )
+ grid_img[:, :width, :] = img_np[:, :, ::-1]
+ grid_img[:,
+ width + GRID_SPACING:2*width + GRID_SPACING, :] = am
+ grid_img[:, 2*width + 2*GRID_SPACING:, :] = overlapped
+ cv2.imwrite(osp.join(actmap_dir, imname + '.jpg'), grid_img)
+
+ if (batch_idx+1) % 10 == 0:
+ print(
+ '- done batch {}/{}'.format(
+ batch_idx + 1, len(data_loader)
+ )
+ )
+
+
+def main():
+ parser = argparse.ArgumentParser()
+ parser.add_argument('--root', type=str)
+ parser.add_argument('-d', '--dataset', type=str, default='market1501')
+ parser.add_argument('-m', '--model', type=str, default='osnet_x1_0')
+ parser.add_argument('--weights', type=str)
+ parser.add_argument('--save-dir', type=str, default='log')
+ parser.add_argument('--height', type=int, default=256)
+ parser.add_argument('--width', type=int, default=128)
+ args = parser.parse_args()
+
+ use_gpu = torch.cuda.is_available()
+
+ datamanager = torchreid.data.ImageDataManager(
+ root=args.root,
+ sources=args.dataset,
+ height=args.height,
+ width=args.width,
+ batch_size_train=100,
+ batch_size_test=100,
+ transforms=None,
+ train_sampler='SequentialSampler'
+ )
+ test_loader = datamanager.test_loader
+
+ model = torchreid.models.build_model(
+ name=args.model,
+ num_classes=datamanager.num_train_pids,
+ use_gpu=use_gpu
+ )
+
+ if use_gpu:
+ model = model.cuda()
+
+ if args.weights and check_isfile(args.weights):
+ load_pretrained_weights(model, args.weights)
+
+ visactmap(
+ model, test_loader, args.save_dir, args.width, args.height, use_gpu
+ )
+
+
+if __name__ == '__main__':
+ main()
diff --git a/trackers/strong_sort/deep/reid/torchreid/__init__.py b/trackers/strong_sort/deep/reid/torchreid/__init__.py
new file mode 100644
index 0000000000000000000000000000000000000000..35eeca675432d30bc360cede699ed9c655dccb80
--- /dev/null
+++ b/trackers/strong_sort/deep/reid/torchreid/__init__.py
@@ -0,0 +1,9 @@
+from __future__ import print_function, absolute_import
+
+from torchreid import data, optim, utils, engine, losses, models, metrics
+
+__version__ = '1.4.0'
+__author__ = 'Kaiyang Zhou'
+__homepage__ = 'https://kaiyangzhou.github.io/'
+__description__ = 'Deep learning person re-identification in PyTorch'
+__url__ = 'https://github.com/KaiyangZhou/deep-person-reid'
diff --git a/trackers/strong_sort/deep/reid/torchreid/data/__init__.py b/trackers/strong_sort/deep/reid/torchreid/data/__init__.py
new file mode 100644
index 0000000000000000000000000000000000000000..5318a16326d671a1b5a8ba949750124fd1d6b05c
--- /dev/null
+++ b/trackers/strong_sort/deep/reid/torchreid/data/__init__.py
@@ -0,0 +1,7 @@
+from __future__ import print_function, absolute_import
+
+from .datasets import (
+ Dataset, ImageDataset, VideoDataset, register_image_dataset,
+ register_video_dataset
+)
+from .datamanager import ImageDataManager, VideoDataManager
diff --git a/trackers/strong_sort/deep/reid/torchreid/data/datamanager.py b/trackers/strong_sort/deep/reid/torchreid/data/datamanager.py
new file mode 100644
index 0000000000000000000000000000000000000000..7ae28cbafacb935619272f56576c4c1e2c30dfec
--- /dev/null
+++ b/trackers/strong_sort/deep/reid/torchreid/data/datamanager.py
@@ -0,0 +1,554 @@
+from __future__ import division, print_function, absolute_import
+import torch
+
+from torchreid.data.sampler import build_train_sampler
+from torchreid.data.datasets import init_image_dataset, init_video_dataset
+from torchreid.data.transforms import build_transforms
+
+
+class DataManager(object):
+ r"""Base data manager.
+
+ Args:
+ sources (str or list): source dataset(s).
+ targets (str or list, optional): target dataset(s). If not given,
+ it equals to ``sources``.
+ height (int, optional): target image height. Default is 256.
+ width (int, optional): target image width. Default is 128.
+ transforms (str or list of str, optional): transformations applied to model training.
+ Default is 'random_flip'.
+ norm_mean (list or None, optional): data mean. Default is None (use imagenet mean).
+ norm_std (list or None, optional): data std. Default is None (use imagenet std).
+ use_gpu (bool, optional): use gpu. Default is True.
+ """
+
+ def __init__(
+ self,
+ sources=None,
+ targets=None,
+ height=256,
+ width=128,
+ transforms='random_flip',
+ norm_mean=None,
+ norm_std=None,
+ use_gpu=False
+ ):
+ self.sources = sources
+ self.targets = targets
+ self.height = height
+ self.width = width
+
+ if self.sources is None:
+ raise ValueError('sources must not be None')
+
+ if isinstance(self.sources, str):
+ self.sources = [self.sources]
+
+ if self.targets is None:
+ self.targets = self.sources
+
+ if isinstance(self.targets, str):
+ self.targets = [self.targets]
+
+ self.transform_tr, self.transform_te = build_transforms(
+ self.height,
+ self.width,
+ transforms=transforms,
+ norm_mean=norm_mean,
+ norm_std=norm_std
+ )
+
+ self.use_gpu = (torch.cuda.is_available() and use_gpu)
+
+ @property
+ def num_train_pids(self):
+ """Returns the number of training person identities."""
+ return self._num_train_pids
+
+ @property
+ def num_train_cams(self):
+ """Returns the number of training cameras."""
+ return self._num_train_cams
+
+ def fetch_test_loaders(self, name):
+ """Returns query and gallery of a test dataset, each containing
+ tuples of (img_path(s), pid, camid).
+
+ Args:
+ name (str): dataset name.
+ """
+ query_loader = self.test_dataset[name]['query']
+ gallery_loader = self.test_dataset[name]['gallery']
+ return query_loader, gallery_loader
+
+ def preprocess_pil_img(self, img):
+ """Transforms a PIL image to torch tensor for testing."""
+ return self.transform_te(img)
+
+
+class ImageDataManager(DataManager):
+ r"""Image data manager.
+
+ Args:
+ root (str): root path to datasets.
+ sources (str or list): source dataset(s).
+ targets (str or list, optional): target dataset(s). If not given,
+ it equals to ``sources``.
+ height (int, optional): target image height. Default is 256.
+ width (int, optional): target image width. Default is 128.
+ transforms (str or list of str, optional): transformations applied to model training.
+ Default is 'random_flip'.
+ k_tfm (int): number of times to apply augmentation to an image
+ independently. If k_tfm > 1, the transform function will be
+ applied k_tfm times to an image. This variable will only be
+ useful for training and is currently valid for image datasets only.
+ norm_mean (list or None, optional): data mean. Default is None (use imagenet mean).
+ norm_std (list or None, optional): data std. Default is None (use imagenet std).
+ use_gpu (bool, optional): use gpu. Default is True.
+ split_id (int, optional): split id (*0-based*). Default is 0.
+ combineall (bool, optional): combine train, query and gallery in a dataset for
+ training. Default is False.
+ load_train_targets (bool, optional): construct train-loader for target datasets.
+ Default is False. This is useful for domain adaptation research.
+ batch_size_train (int, optional): number of images in a training batch. Default is 32.
+ batch_size_test (int, optional): number of images in a test batch. Default is 32.
+ workers (int, optional): number of workers. Default is 4.
+ num_instances (int, optional): number of instances per identity in a batch.
+ Default is 4.
+ num_cams (int, optional): number of cameras to sample in a batch (when using
+ ``RandomDomainSampler``). Default is 1.
+ num_datasets (int, optional): number of datasets to sample in a batch (when
+ using ``RandomDatasetSampler``). Default is 1.
+ train_sampler (str, optional): sampler. Default is RandomSampler.
+ train_sampler_t (str, optional): sampler for target train loader. Default is RandomSampler.
+ cuhk03_labeled (bool, optional): use cuhk03 labeled images.
+ Default is False (defaul is to use detected images).
+ cuhk03_classic_split (bool, optional): use the classic split in cuhk03.
+ Default is False.
+ market1501_500k (bool, optional): add 500K distractors to the gallery
+ set in market1501. Default is False.
+
+ Examples::
+
+ datamanager = torchreid.data.ImageDataManager(
+ root='path/to/reid-data',
+ sources='market1501',
+ height=256,
+ width=128,
+ batch_size_train=32,
+ batch_size_test=100
+ )
+
+ # return train loader of source data
+ train_loader = datamanager.train_loader
+
+ # return test loader of target data
+ test_loader = datamanager.test_loader
+
+ # return train loader of target data
+ train_loader_t = datamanager.train_loader_t
+ """
+ data_type = 'image'
+
+ def __init__(
+ self,
+ root='',
+ sources=None,
+ targets=None,
+ height=256,
+ width=128,
+ transforms='random_flip',
+ k_tfm=1,
+ norm_mean=None,
+ norm_std=None,
+ use_gpu=True,
+ split_id=0,
+ combineall=False,
+ load_train_targets=False,
+ batch_size_train=32,
+ batch_size_test=32,
+ workers=4,
+ num_instances=4,
+ num_cams=1,
+ num_datasets=1,
+ train_sampler='RandomSampler',
+ train_sampler_t='RandomSampler',
+ cuhk03_labeled=False,
+ cuhk03_classic_split=False,
+ market1501_500k=False
+ ):
+
+ super(ImageDataManager, self).__init__(
+ sources=sources,
+ targets=targets,
+ height=height,
+ width=width,
+ transforms=transforms,
+ norm_mean=norm_mean,
+ norm_std=norm_std,
+ use_gpu=use_gpu
+ )
+
+ print('=> Loading train (source) dataset')
+ trainset = []
+ for name in self.sources:
+ trainset_ = init_image_dataset(
+ name,
+ transform=self.transform_tr,
+ k_tfm=k_tfm,
+ mode='train',
+ combineall=combineall,
+ root=root,
+ split_id=split_id,
+ cuhk03_labeled=cuhk03_labeled,
+ cuhk03_classic_split=cuhk03_classic_split,
+ market1501_500k=market1501_500k
+ )
+ trainset.append(trainset_)
+ trainset = sum(trainset)
+
+ self._num_train_pids = trainset.num_train_pids
+ self._num_train_cams = trainset.num_train_cams
+
+ self.train_loader = torch.utils.data.DataLoader(
+ trainset,
+ sampler=build_train_sampler(
+ trainset.train,
+ train_sampler,
+ batch_size=batch_size_train,
+ num_instances=num_instances,
+ num_cams=num_cams,
+ num_datasets=num_datasets
+ ),
+ batch_size=batch_size_train,
+ shuffle=False,
+ num_workers=workers,
+ pin_memory=self.use_gpu,
+ drop_last=True
+ )
+
+ self.train_loader_t = None
+ if load_train_targets:
+ # check if sources and targets are identical
+ assert len(set(self.sources) & set(self.targets)) == 0, \
+ 'sources={} and targets={} must not have overlap'.format(self.sources, self.targets)
+
+ print('=> Loading train (target) dataset')
+ trainset_t = []
+ for name in self.targets:
+ trainset_t_ = init_image_dataset(
+ name,
+ transform=self.transform_tr,
+ k_tfm=k_tfm,
+ mode='train',
+ combineall=False, # only use the training data
+ root=root,
+ split_id=split_id,
+ cuhk03_labeled=cuhk03_labeled,
+ cuhk03_classic_split=cuhk03_classic_split,
+ market1501_500k=market1501_500k
+ )
+ trainset_t.append(trainset_t_)
+ trainset_t = sum(trainset_t)
+
+ self.train_loader_t = torch.utils.data.DataLoader(
+ trainset_t,
+ sampler=build_train_sampler(
+ trainset_t.train,
+ train_sampler_t,
+ batch_size=batch_size_train,
+ num_instances=num_instances,
+ num_cams=num_cams,
+ num_datasets=num_datasets
+ ),
+ batch_size=batch_size_train,
+ shuffle=False,
+ num_workers=workers,
+ pin_memory=self.use_gpu,
+ drop_last=True
+ )
+
+ print('=> Loading test (target) dataset')
+ self.test_loader = {
+ name: {
+ 'query': None,
+ 'gallery': None
+ }
+ for name in self.targets
+ }
+ self.test_dataset = {
+ name: {
+ 'query': None,
+ 'gallery': None
+ }
+ for name in self.targets
+ }
+
+ for name in self.targets:
+ # build query loader
+ queryset = init_image_dataset(
+ name,
+ transform=self.transform_te,
+ mode='query',
+ combineall=combineall,
+ root=root,
+ split_id=split_id,
+ cuhk03_labeled=cuhk03_labeled,
+ cuhk03_classic_split=cuhk03_classic_split,
+ market1501_500k=market1501_500k
+ )
+ self.test_loader[name]['query'] = torch.utils.data.DataLoader(
+ queryset,
+ batch_size=batch_size_test,
+ shuffle=False,
+ num_workers=workers,
+ pin_memory=self.use_gpu,
+ drop_last=False
+ )
+
+ # build gallery loader
+ galleryset = init_image_dataset(
+ name,
+ transform=self.transform_te,
+ mode='gallery',
+ combineall=combineall,
+ verbose=False,
+ root=root,
+ split_id=split_id,
+ cuhk03_labeled=cuhk03_labeled,
+ cuhk03_classic_split=cuhk03_classic_split,
+ market1501_500k=market1501_500k
+ )
+ self.test_loader[name]['gallery'] = torch.utils.data.DataLoader(
+ galleryset,
+ batch_size=batch_size_test,
+ shuffle=False,
+ num_workers=workers,
+ pin_memory=self.use_gpu,
+ drop_last=False
+ )
+
+ self.test_dataset[name]['query'] = queryset.query
+ self.test_dataset[name]['gallery'] = galleryset.gallery
+
+ print('\n')
+ print(' **************** Summary ****************')
+ print(' source : {}'.format(self.sources))
+ print(' # source datasets : {}'.format(len(self.sources)))
+ print(' # source ids : {}'.format(self.num_train_pids))
+ print(' # source images : {}'.format(len(trainset)))
+ print(' # source cameras : {}'.format(self.num_train_cams))
+ if load_train_targets:
+ print(
+ ' # target images : {} (unlabeled)'.format(len(trainset_t))
+ )
+ print(' target : {}'.format(self.targets))
+ print(' *****************************************')
+ print('\n')
+
+
+class VideoDataManager(DataManager):
+ r"""Video data manager.
+
+ Args:
+ root (str): root path to datasets.
+ sources (str or list): source dataset(s).
+ targets (str or list, optional): target dataset(s). If not given,
+ it equals to ``sources``.
+ height (int, optional): target image height. Default is 256.
+ width (int, optional): target image width. Default is 128.
+ transforms (str or list of str, optional): transformations applied to model training.
+ Default is 'random_flip'.
+ norm_mean (list or None, optional): data mean. Default is None (use imagenet mean).
+ norm_std (list or None, optional): data std. Default is None (use imagenet std).
+ use_gpu (bool, optional): use gpu. Default is True.
+ split_id (int, optional): split id (*0-based*). Default is 0.
+ combineall (bool, optional): combine train, query and gallery in a dataset for
+ training. Default is False.
+ batch_size_train (int, optional): number of tracklets in a training batch. Default is 3.
+ batch_size_test (int, optional): number of tracklets in a test batch. Default is 3.
+ workers (int, optional): number of workers. Default is 4.
+ num_instances (int, optional): number of instances per identity in a batch.
+ Default is 4.
+ num_cams (int, optional): number of cameras to sample in a batch (when using
+ ``RandomDomainSampler``). Default is 1.
+ num_datasets (int, optional): number of datasets to sample in a batch (when
+ using ``RandomDatasetSampler``). Default is 1.
+ train_sampler (str, optional): sampler. Default is RandomSampler.
+ seq_len (int, optional): how many images to sample in a tracklet. Default is 15.
+ sample_method (str, optional): how to sample images in a tracklet. Default is "evenly".
+ Choices are ["evenly", "random", "all"]. "evenly" and "random" will sample ``seq_len``
+ images in a tracklet while "all" samples all images in a tracklet, where the batch size
+ needs to be set to 1.
+
+ Examples::
+
+ datamanager = torchreid.data.VideoDataManager(
+ root='path/to/reid-data',
+ sources='mars',
+ height=256,
+ width=128,
+ batch_size_train=3,
+ batch_size_test=3,
+ seq_len=15,
+ sample_method='evenly'
+ )
+
+ # return train loader of source data
+ train_loader = datamanager.train_loader
+
+ # return test loader of target data
+ test_loader = datamanager.test_loader
+
+ .. note::
+ The current implementation only supports image-like training. Therefore, each image in a
+ sampled tracklet will undergo independent transformation functions. To achieve tracklet-aware
+ training, you need to modify the transformation functions for video reid such that each function
+ applies the same operation to all images in a tracklet to keep consistency.
+ """
+ data_type = 'video'
+
+ def __init__(
+ self,
+ root='',
+ sources=None,
+ targets=None,
+ height=256,
+ width=128,
+ transforms='random_flip',
+ norm_mean=None,
+ norm_std=None,
+ use_gpu=True,
+ split_id=0,
+ combineall=False,
+ batch_size_train=3,
+ batch_size_test=3,
+ workers=4,
+ num_instances=4,
+ num_cams=1,
+ num_datasets=1,
+ train_sampler='RandomSampler',
+ seq_len=15,
+ sample_method='evenly'
+ ):
+
+ super(VideoDataManager, self).__init__(
+ sources=sources,
+ targets=targets,
+ height=height,
+ width=width,
+ transforms=transforms,
+ norm_mean=norm_mean,
+ norm_std=norm_std,
+ use_gpu=use_gpu
+ )
+
+ print('=> Loading train (source) dataset')
+ trainset = []
+ for name in self.sources:
+ trainset_ = init_video_dataset(
+ name,
+ transform=self.transform_tr,
+ mode='train',
+ combineall=combineall,
+ root=root,
+ split_id=split_id,
+ seq_len=seq_len,
+ sample_method=sample_method
+ )
+ trainset.append(trainset_)
+ trainset = sum(trainset)
+
+ self._num_train_pids = trainset.num_train_pids
+ self._num_train_cams = trainset.num_train_cams
+
+ train_sampler = build_train_sampler(
+ trainset.train,
+ train_sampler,
+ batch_size=batch_size_train,
+ num_instances=num_instances,
+ num_cams=num_cams,
+ num_datasets=num_datasets
+ )
+
+ self.train_loader = torch.utils.data.DataLoader(
+ trainset,
+ sampler=train_sampler,
+ batch_size=batch_size_train,
+ shuffle=False,
+ num_workers=workers,
+ pin_memory=self.use_gpu,
+ drop_last=True
+ )
+
+ print('=> Loading test (target) dataset')
+ self.test_loader = {
+ name: {
+ 'query': None,
+ 'gallery': None
+ }
+ for name in self.targets
+ }
+ self.test_dataset = {
+ name: {
+ 'query': None,
+ 'gallery': None
+ }
+ for name in self.targets
+ }
+
+ for name in self.targets:
+ # build query loader
+ queryset = init_video_dataset(
+ name,
+ transform=self.transform_te,
+ mode='query',
+ combineall=combineall,
+ root=root,
+ split_id=split_id,
+ seq_len=seq_len,
+ sample_method=sample_method
+ )
+ self.test_loader[name]['query'] = torch.utils.data.DataLoader(
+ queryset,
+ batch_size=batch_size_test,
+ shuffle=False,
+ num_workers=workers,
+ pin_memory=self.use_gpu,
+ drop_last=False
+ )
+
+ # build gallery loader
+ galleryset = init_video_dataset(
+ name,
+ transform=self.transform_te,
+ mode='gallery',
+ combineall=combineall,
+ verbose=False,
+ root=root,
+ split_id=split_id,
+ seq_len=seq_len,
+ sample_method=sample_method
+ )
+ self.test_loader[name]['gallery'] = torch.utils.data.DataLoader(
+ galleryset,
+ batch_size=batch_size_test,
+ shuffle=False,
+ num_workers=workers,
+ pin_memory=self.use_gpu,
+ drop_last=False
+ )
+
+ self.test_dataset[name]['query'] = queryset.query
+ self.test_dataset[name]['gallery'] = galleryset.gallery
+
+ print('\n')
+ print(' **************** Summary ****************')
+ print(' source : {}'.format(self.sources))
+ print(' # source datasets : {}'.format(len(self.sources)))
+ print(' # source ids : {}'.format(self.num_train_pids))
+ print(' # source tracklets : {}'.format(len(trainset)))
+ print(' # source cameras : {}'.format(self.num_train_cams))
+ print(' target : {}'.format(self.targets))
+ print(' *****************************************')
+ print('\n')
diff --git a/trackers/strong_sort/deep/reid/torchreid/data/datasets/__init__.py b/trackers/strong_sort/deep/reid/torchreid/data/datasets/__init__.py
new file mode 100644
index 0000000000000000000000000000000000000000..afb02a2bf2ce740ade52cb974a862e8987222088
--- /dev/null
+++ b/trackers/strong_sort/deep/reid/torchreid/data/datasets/__init__.py
@@ -0,0 +1,119 @@
+from __future__ import print_function, absolute_import
+
+from .image import (
+ GRID, PRID, CUHK01, CUHK02, CUHK03, MSMT17, CUHKSYSU, VIPeR, SenseReID,
+ Market1501, DukeMTMCreID, University1652, iLIDS
+)
+from .video import PRID2011, Mars, DukeMTMCVidReID, iLIDSVID
+from .dataset import Dataset, ImageDataset, VideoDataset
+
+__image_datasets = {
+ 'market1501': Market1501,
+ 'cuhk03': CUHK03,
+ 'dukemtmcreid': DukeMTMCreID,
+ 'msmt17': MSMT17,
+ 'viper': VIPeR,
+ 'grid': GRID,
+ 'cuhk01': CUHK01,
+ 'ilids': iLIDS,
+ 'sensereid': SenseReID,
+ 'prid': PRID,
+ 'cuhk02': CUHK02,
+ 'university1652': University1652,
+ 'cuhksysu': CUHKSYSU
+}
+
+__video_datasets = {
+ 'mars': Mars,
+ 'ilidsvid': iLIDSVID,
+ 'prid2011': PRID2011,
+ 'dukemtmcvidreid': DukeMTMCVidReID
+}
+
+
+def init_image_dataset(name, **kwargs):
+ """Initializes an image dataset."""
+ avai_datasets = list(__image_datasets.keys())
+ if name not in avai_datasets:
+ raise ValueError(
+ 'Invalid dataset name. Received "{}", '
+ 'but expected to be one of {}'.format(name, avai_datasets)
+ )
+ return __image_datasets[name](**kwargs)
+
+
+def init_video_dataset(name, **kwargs):
+ """Initializes a video dataset."""
+ avai_datasets = list(__video_datasets.keys())
+ if name not in avai_datasets:
+ raise ValueError(
+ 'Invalid dataset name. Received "{}", '
+ 'but expected to be one of {}'.format(name, avai_datasets)
+ )
+ return __video_datasets[name](**kwargs)
+
+
+def register_image_dataset(name, dataset):
+ """Registers a new image dataset.
+
+ Args:
+ name (str): key corresponding to the new dataset.
+ dataset (Dataset): the new dataset class.
+
+ Examples::
+
+ import torchreid
+ import NewDataset
+ torchreid.data.register_image_dataset('new_dataset', NewDataset)
+ # single dataset case
+ datamanager = torchreid.data.ImageDataManager(
+ root='reid-data',
+ sources='new_dataset'
+ )
+ # multiple dataset case
+ datamanager = torchreid.data.ImageDataManager(
+ root='reid-data',
+ sources=['new_dataset', 'dukemtmcreid']
+ )
+ """
+ global __image_datasets
+ curr_datasets = list(__image_datasets.keys())
+ if name in curr_datasets:
+ raise ValueError(
+ 'The given name already exists, please choose '
+ 'another name excluding {}'.format(curr_datasets)
+ )
+ __image_datasets[name] = dataset
+
+
+def register_video_dataset(name, dataset):
+ """Registers a new video dataset.
+
+ Args:
+ name (str): key corresponding to the new dataset.
+ dataset (Dataset): the new dataset class.
+
+ Examples::
+
+ import torchreid
+ import NewDataset
+ torchreid.data.register_video_dataset('new_dataset', NewDataset)
+ # single dataset case
+ datamanager = torchreid.data.VideoDataManager(
+ root='reid-data',
+ sources='new_dataset'
+ )
+ # multiple dataset case
+ datamanager = torchreid.data.VideoDataManager(
+ root='reid-data',
+ sources=['new_dataset', 'ilidsvid']
+ )
+ """
+ global __video_datasets
+ curr_datasets = list(__video_datasets.keys())
+ if name in curr_datasets:
+ raise ValueError(
+ 'The given name already exists, please choose '
+ 'another name excluding {}'.format(curr_datasets)
+ )
+ __video_datasets[name] = dataset
diff --git a/trackers/strong_sort/deep/reid/torchreid/data/datasets/dataset.py b/trackers/strong_sort/deep/reid/torchreid/data/datasets/dataset.py
new file mode 100644
index 0000000000000000000000000000000000000000..66b1e7a6ea224e6d4fca75b9df7c0342de878553
--- /dev/null
+++ b/trackers/strong_sort/deep/reid/torchreid/data/datasets/dataset.py
@@ -0,0 +1,482 @@
+from __future__ import division, print_function, absolute_import
+import copy
+import numpy as np
+import os.path as osp
+import tarfile
+import zipfile
+import torch
+
+from torchreid.utils import read_image, download_url, mkdir_if_missing
+
+
+class Dataset(object):
+ """An abstract class representing a Dataset.
+
+ This is the base class for ``ImageDataset`` and ``VideoDataset``.
+
+ Args:
+ train (list): contains tuples of (img_path(s), pid, camid).
+ query (list): contains tuples of (img_path(s), pid, camid).
+ gallery (list): contains tuples of (img_path(s), pid, camid).
+ transform: transform function.
+ k_tfm (int): number of times to apply augmentation to an image
+ independently. If k_tfm > 1, the transform function will be
+ applied k_tfm times to an image. This variable will only be
+ useful for training and is currently valid for image datasets only.
+ mode (str): 'train', 'query' or 'gallery'.
+ combineall (bool): combines train, query and gallery in a
+ dataset for training.
+ verbose (bool): show information.
+ """
+
+ # junk_pids contains useless person IDs, e.g. background,
+ # false detections, distractors. These IDs will be ignored
+ # when combining all images in a dataset for training, i.e.
+ # combineall=True
+ _junk_pids = []
+
+ # Some datasets are only used for training, like CUHK-SYSU
+ # In this case, "combineall=True" is not used for them
+ _train_only = False
+
+ def __init__(
+ self,
+ train,
+ query,
+ gallery,
+ transform=None,
+ k_tfm=1,
+ mode='train',
+ combineall=False,
+ verbose=True,
+ **kwargs
+ ):
+ # extend 3-tuple (img_path(s), pid, camid) to
+ # 4-tuple (img_path(s), pid, camid, dsetid) by
+ # adding a dataset indicator "dsetid"
+ if len(train[0]) == 3:
+ train = [(*items, 0) for items in train]
+ if len(query[0]) == 3:
+ query = [(*items, 0) for items in query]
+ if len(gallery[0]) == 3:
+ gallery = [(*items, 0) for items in gallery]
+
+ self.train = train
+ self.query = query
+ self.gallery = gallery
+ self.transform = transform
+ self.k_tfm = k_tfm
+ self.mode = mode
+ self.combineall = combineall
+ self.verbose = verbose
+
+ self.num_train_pids = self.get_num_pids(self.train)
+ self.num_train_cams = self.get_num_cams(self.train)
+ self.num_datasets = self.get_num_datasets(self.train)
+
+ if self.combineall:
+ self.combine_all()
+
+ if self.mode == 'train':
+ self.data = self.train
+ elif self.mode == 'query':
+ self.data = self.query
+ elif self.mode == 'gallery':
+ self.data = self.gallery
+ else:
+ raise ValueError(
+ 'Invalid mode. Got {}, but expected to be '
+ 'one of [train | query | gallery]'.format(self.mode)
+ )
+
+ if self.verbose:
+ self.show_summary()
+
+ def __getitem__(self, index):
+ raise NotImplementedError
+
+ def __len__(self):
+ return len(self.data)
+
+ def __add__(self, other):
+ """Adds two datasets together (only the train set)."""
+ train = copy.deepcopy(self.train)
+
+ for img_path, pid, camid, dsetid in other.train:
+ pid += self.num_train_pids
+ camid += self.num_train_cams
+ dsetid += self.num_datasets
+ train.append((img_path, pid, camid, dsetid))
+
+ ###################################
+ # Note that
+ # 1. set verbose=False to avoid unnecessary print
+ # 2. set combineall=False because combineall would have been applied
+ # if it was True for a specific dataset; setting it to True will
+ # create new IDs that should have already been included
+ ###################################
+ if isinstance(train[0][0], str):
+ return ImageDataset(
+ train,
+ self.query,
+ self.gallery,
+ transform=self.transform,
+ mode=self.mode,
+ combineall=False,
+ verbose=False
+ )
+ else:
+ return VideoDataset(
+ train,
+ self.query,
+ self.gallery,
+ transform=self.transform,
+ mode=self.mode,
+ combineall=False,
+ verbose=False,
+ seq_len=self.seq_len,
+ sample_method=self.sample_method
+ )
+
+ def __radd__(self, other):
+ """Supports sum([dataset1, dataset2, dataset3])."""
+ if other == 0:
+ return self
+ else:
+ return self.__add__(other)
+
+ def get_num_pids(self, data):
+ """Returns the number of training person identities.
+
+ Each tuple in data contains (img_path(s), pid, camid, dsetid).
+ """
+ pids = set()
+ for items in data:
+ pid = items[1]
+ pids.add(pid)
+ return len(pids)
+
+ def get_num_cams(self, data):
+ """Returns the number of training cameras.
+
+ Each tuple in data contains (img_path(s), pid, camid, dsetid).
+ """
+ cams = set()
+ for items in data:
+ camid = items[2]
+ cams.add(camid)
+ return len(cams)
+
+ def get_num_datasets(self, data):
+ """Returns the number of datasets included.
+
+ Each tuple in data contains (img_path(s), pid, camid, dsetid).
+ """
+ dsets = set()
+ for items in data:
+ dsetid = items[3]
+ dsets.add(dsetid)
+ return len(dsets)
+
+ def show_summary(self):
+ """Shows dataset statistics."""
+ pass
+
+ def combine_all(self):
+ """Combines train, query and gallery in a dataset for training."""
+ if self._train_only:
+ return
+
+ combined = copy.deepcopy(self.train)
+
+ # relabel pids in gallery (query shares the same scope)
+ g_pids = set()
+ for items in self.gallery:
+ pid = items[1]
+ if pid in self._junk_pids:
+ continue
+ g_pids.add(pid)
+ pid2label = {pid: i for i, pid in enumerate(g_pids)}
+
+ def _combine_data(data):
+ for img_path, pid, camid, dsetid in data:
+ if pid in self._junk_pids:
+ continue
+ pid = pid2label[pid] + self.num_train_pids
+ combined.append((img_path, pid, camid, dsetid))
+
+ _combine_data(self.query)
+ _combine_data(self.gallery)
+
+ self.train = combined
+ self.num_train_pids = self.get_num_pids(self.train)
+
+ def download_dataset(self, dataset_dir, dataset_url):
+ """Downloads and extracts dataset.
+
+ Args:
+ dataset_dir (str): dataset directory.
+ dataset_url (str): url to download dataset.
+ """
+ if osp.exists(dataset_dir):
+ return
+
+ if dataset_url is None:
+ raise RuntimeError(
+ '{} dataset needs to be manually '
+ 'prepared, please follow the '
+ 'document to prepare this dataset'.format(
+ self.__class__.__name__
+ )
+ )
+
+ print('Creating directory "{}"'.format(dataset_dir))
+ mkdir_if_missing(dataset_dir)
+ fpath = osp.join(dataset_dir, osp.basename(dataset_url))
+
+ print(
+ 'Downloading {} dataset to "{}"'.format(
+ self.__class__.__name__, dataset_dir
+ )
+ )
+ download_url(dataset_url, fpath)
+
+ print('Extracting "{}"'.format(fpath))
+ try:
+ tar = tarfile.open(fpath)
+ tar.extractall(path=dataset_dir)
+ tar.close()
+ except:
+ zip_ref = zipfile.ZipFile(fpath, 'r')
+ zip_ref.extractall(dataset_dir)
+ zip_ref.close()
+
+ print('{} dataset is ready'.format(self.__class__.__name__))
+
+ def check_before_run(self, required_files):
+ """Checks if required files exist before going deeper.
+
+ Args:
+ required_files (str or list): string file name(s).
+ """
+ if isinstance(required_files, str):
+ required_files = [required_files]
+
+ for fpath in required_files:
+ if not osp.exists(fpath):
+ raise RuntimeError('"{}" is not found'.format(fpath))
+
+ def __repr__(self):
+ num_train_pids = self.get_num_pids(self.train)
+ num_train_cams = self.get_num_cams(self.train)
+
+ num_query_pids = self.get_num_pids(self.query)
+ num_query_cams = self.get_num_cams(self.query)
+
+ num_gallery_pids = self.get_num_pids(self.gallery)
+ num_gallery_cams = self.get_num_cams(self.gallery)
+
+ msg = ' ----------------------------------------\n' \
+ ' subset | # ids | # items | # cameras\n' \
+ ' ----------------------------------------\n' \
+ ' train | {:5d} | {:7d} | {:9d}\n' \
+ ' query | {:5d} | {:7d} | {:9d}\n' \
+ ' gallery | {:5d} | {:7d} | {:9d}\n' \
+ ' ----------------------------------------\n' \
+ ' items: images/tracklets for image/video dataset\n'.format(
+ num_train_pids, len(self.train), num_train_cams,
+ num_query_pids, len(self.query), num_query_cams,
+ num_gallery_pids, len(self.gallery), num_gallery_cams
+ )
+
+ return msg
+
+ def _transform_image(self, tfm, k_tfm, img0):
+ """Transforms a raw image (img0) k_tfm times with
+ the transform function tfm.
+ """
+ img_list = []
+
+ for k in range(k_tfm):
+ img_list.append(tfm(img0))
+
+ img = img_list
+ if len(img) == 1:
+ img = img[0]
+
+ return img
+
+
+class ImageDataset(Dataset):
+ """A base class representing ImageDataset.
+
+ All other image datasets should subclass it.
+
+ ``__getitem__`` returns an image given index.
+ It will return ``img``, ``pid``, ``camid`` and ``img_path``
+ where ``img`` has shape (channel, height, width). As a result,
+ data in each batch has shape (batch_size, channel, height, width).
+ """
+
+ def __init__(self, train, query, gallery, **kwargs):
+ super(ImageDataset, self).__init__(train, query, gallery, **kwargs)
+
+ def __getitem__(self, index):
+ img_path, pid, camid, dsetid = self.data[index]
+ img = read_image(img_path)
+ if self.transform is not None:
+ img = self._transform_image(self.transform, self.k_tfm, img)
+ item = {
+ 'img': img,
+ 'pid': pid,
+ 'camid': camid,
+ 'impath': img_path,
+ 'dsetid': dsetid
+ }
+ return item
+
+ def show_summary(self):
+ num_train_pids = self.get_num_pids(self.train)
+ num_train_cams = self.get_num_cams(self.train)
+
+ num_query_pids = self.get_num_pids(self.query)
+ num_query_cams = self.get_num_cams(self.query)
+
+ num_gallery_pids = self.get_num_pids(self.gallery)
+ num_gallery_cams = self.get_num_cams(self.gallery)
+
+ print('=> Loaded {}'.format(self.__class__.__name__))
+ print(' ----------------------------------------')
+ print(' subset | # ids | # images | # cameras')
+ print(' ----------------------------------------')
+ print(
+ ' train | {:5d} | {:8d} | {:9d}'.format(
+ num_train_pids, len(self.train), num_train_cams
+ )
+ )
+ print(
+ ' query | {:5d} | {:8d} | {:9d}'.format(
+ num_query_pids, len(self.query), num_query_cams
+ )
+ )
+ print(
+ ' gallery | {:5d} | {:8d} | {:9d}'.format(
+ num_gallery_pids, len(self.gallery), num_gallery_cams
+ )
+ )
+ print(' ----------------------------------------')
+
+
+class VideoDataset(Dataset):
+ """A base class representing VideoDataset.
+
+ All other video datasets should subclass it.
+
+ ``__getitem__`` returns an image given index.
+ It will return ``imgs``, ``pid`` and ``camid``
+ where ``imgs`` has shape (seq_len, channel, height, width). As a result,
+ data in each batch has shape (batch_size, seq_len, channel, height, width).
+ """
+
+ def __init__(
+ self,
+ train,
+ query,
+ gallery,
+ seq_len=15,
+ sample_method='evenly',
+ **kwargs
+ ):
+ super(VideoDataset, self).__init__(train, query, gallery, **kwargs)
+ self.seq_len = seq_len
+ self.sample_method = sample_method
+
+ if self.transform is None:
+ raise RuntimeError('transform must not be None')
+
+ def __getitem__(self, index):
+ img_paths, pid, camid, dsetid = self.data[index]
+ num_imgs = len(img_paths)
+
+ if self.sample_method == 'random':
+ # Randomly samples seq_len images from a tracklet of length num_imgs,
+ # if num_imgs is smaller than seq_len, then replicates images
+ indices = np.arange(num_imgs)
+ replace = False if num_imgs >= self.seq_len else True
+ indices = np.random.choice(
+ indices, size=self.seq_len, replace=replace
+ )
+ # sort indices to keep temporal order (comment it to be order-agnostic)
+ indices = np.sort(indices)
+
+ elif self.sample_method == 'evenly':
+ # Evenly samples seq_len images from a tracklet
+ if num_imgs >= self.seq_len:
+ num_imgs -= num_imgs % self.seq_len
+ indices = np.arange(0, num_imgs, num_imgs / self.seq_len)
+ else:
+ # if num_imgs is smaller than seq_len, simply replicate the last image
+ # until the seq_len requirement is satisfied
+ indices = np.arange(0, num_imgs)
+ num_pads = self.seq_len - num_imgs
+ indices = np.concatenate(
+ [
+ indices,
+ np.ones(num_pads).astype(np.int32) * (num_imgs-1)
+ ]
+ )
+ assert len(indices) == self.seq_len
+
+ elif self.sample_method == 'all':
+ # Samples all images in a tracklet. batch_size must be set to 1
+ indices = np.arange(num_imgs)
+
+ else:
+ raise ValueError(
+ 'Unknown sample method: {}'.format(self.sample_method)
+ )
+
+ imgs = []
+ for index in indices:
+ img_path = img_paths[int(index)]
+ img = read_image(img_path)
+ if self.transform is not None:
+ img = self.transform(img)
+ img = img.unsqueeze(0) # img must be torch.Tensor
+ imgs.append(img)
+ imgs = torch.cat(imgs, dim=0)
+
+ item = {'img': imgs, 'pid': pid, 'camid': camid, 'dsetid': dsetid}
+
+ return item
+
+ def show_summary(self):
+ num_train_pids = self.get_num_pids(self.train)
+ num_train_cams = self.get_num_cams(self.train)
+
+ num_query_pids = self.get_num_pids(self.query)
+ num_query_cams = self.get_num_cams(self.query)
+
+ num_gallery_pids = self.get_num_pids(self.gallery)
+ num_gallery_cams = self.get_num_cams(self.gallery)
+
+ print('=> Loaded {}'.format(self.__class__.__name__))
+ print(' -------------------------------------------')
+ print(' subset | # ids | # tracklets | # cameras')
+ print(' -------------------------------------------')
+ print(
+ ' train | {:5d} | {:11d} | {:9d}'.format(
+ num_train_pids, len(self.train), num_train_cams
+ )
+ )
+ print(
+ ' query | {:5d} | {:11d} | {:9d}'.format(
+ num_query_pids, len(self.query), num_query_cams
+ )
+ )
+ print(
+ ' gallery | {:5d} | {:11d} | {:9d}'.format(
+ num_gallery_pids, len(self.gallery), num_gallery_cams
+ )
+ )
+ print(' -------------------------------------------')
diff --git a/trackers/strong_sort/deep/reid/torchreid/data/datasets/image/__init__.py b/trackers/strong_sort/deep/reid/torchreid/data/datasets/image/__init__.py
new file mode 100644
index 0000000000000000000000000000000000000000..f2216e96db061ee38f7172147778a495d3124db0
--- /dev/null
+++ b/trackers/strong_sort/deep/reid/torchreid/data/datasets/image/__init__.py
@@ -0,0 +1,15 @@
+from __future__ import print_function, absolute_import
+
+from .grid import GRID
+from .prid import PRID
+from .ilids import iLIDS
+from .viper import VIPeR
+from .cuhk01 import CUHK01
+from .cuhk02 import CUHK02
+from .cuhk03 import CUHK03
+from .msmt17 import MSMT17
+from .cuhksysu import CUHKSYSU
+from .sensereid import SenseReID
+from .market1501 import Market1501
+from .dukemtmcreid import DukeMTMCreID
+from .university1652 import University1652
diff --git a/trackers/strong_sort/deep/reid/torchreid/data/datasets/image/cuhk01.py b/trackers/strong_sort/deep/reid/torchreid/data/datasets/image/cuhk01.py
new file mode 100644
index 0000000000000000000000000000000000000000..c4c332ef7921a8d9f501ccb6d57aa56d78a9a98a
--- /dev/null
+++ b/trackers/strong_sort/deep/reid/torchreid/data/datasets/image/cuhk01.py
@@ -0,0 +1,137 @@
+from __future__ import division, print_function, absolute_import
+import glob
+import numpy as np
+import os.path as osp
+import zipfile
+
+from torchreid.utils import read_json, write_json
+
+from ..dataset import ImageDataset
+
+
+class CUHK01(ImageDataset):
+ """CUHK01.
+
+ Reference:
+ Li et al. Human Reidentification with Transferred Metric Learning. ACCV 2012.
+
+ URL: ``_
+
+ Dataset statistics:
+ - identities: 971.
+ - images: 3884.
+ - cameras: 4.
+
+ Note: CUHK01 and CUHK02 overlap.
+ """
+ dataset_dir = 'cuhk01'
+ dataset_url = None
+
+ def __init__(self, root='', split_id=0, **kwargs):
+ self.root = osp.abspath(osp.expanduser(root))
+ self.dataset_dir = osp.join(self.root, self.dataset_dir)
+ self.download_dataset(self.dataset_dir, self.dataset_url)
+
+ self.zip_path = osp.join(self.dataset_dir, 'CUHK01.zip')
+ self.campus_dir = osp.join(self.dataset_dir, 'campus')
+ self.split_path = osp.join(self.dataset_dir, 'splits.json')
+
+ self.extract_file()
+
+ required_files = [self.dataset_dir, self.campus_dir]
+ self.check_before_run(required_files)
+
+ self.prepare_split()
+ splits = read_json(self.split_path)
+ if split_id >= len(splits):
+ raise ValueError(
+ 'split_id exceeds range, received {}, but expected between 0 and {}'
+ .format(split_id,
+ len(splits) - 1)
+ )
+ split = splits[split_id]
+
+ train = split['train']
+ query = split['query']
+ gallery = split['gallery']
+
+ train = [tuple(item) for item in train]
+ query = [tuple(item) for item in query]
+ gallery = [tuple(item) for item in gallery]
+
+ super(CUHK01, self).__init__(train, query, gallery, **kwargs)
+
+ def extract_file(self):
+ if not osp.exists(self.campus_dir):
+ print('Extracting files')
+ zip_ref = zipfile.ZipFile(self.zip_path, 'r')
+ zip_ref.extractall(self.dataset_dir)
+ zip_ref.close()
+
+ def prepare_split(self):
+ """
+ Image name format: 0001001.png, where first four digits represent identity
+ and last four digits represent cameras. Camera 1&2 are considered the same
+ view and camera 3&4 are considered the same view.
+ """
+ if not osp.exists(self.split_path):
+ print('Creating 10 random splits of train ids and test ids')
+ img_paths = sorted(glob.glob(osp.join(self.campus_dir, '*.png')))
+ img_list = []
+ pid_container = set()
+ for img_path in img_paths:
+ img_name = osp.basename(img_path)
+ pid = int(img_name[:4]) - 1
+ camid = (int(img_name[4:7]) - 1) // 2 # result is either 0 or 1
+ img_list.append((img_path, pid, camid))
+ pid_container.add(pid)
+
+ num_pids = len(pid_container)
+ num_train_pids = num_pids // 2
+
+ splits = []
+ for _ in range(10):
+ order = np.arange(num_pids)
+ np.random.shuffle(order)
+ train_idxs = order[:num_train_pids]
+ train_idxs = np.sort(train_idxs)
+ idx2label = {
+ idx: label
+ for label, idx in enumerate(train_idxs)
+ }
+
+ train, test_a, test_b = [], [], []
+ for img_path, pid, camid in img_list:
+ if pid in train_idxs:
+ train.append((img_path, idx2label[pid], camid))
+ else:
+ if camid == 0:
+ test_a.append((img_path, pid, camid))
+ else:
+ test_b.append((img_path, pid, camid))
+
+ # use cameraA as query and cameraB as gallery
+ split = {
+ 'train': train,
+ 'query': test_a,
+ 'gallery': test_b,
+ 'num_train_pids': num_train_pids,
+ 'num_query_pids': num_pids - num_train_pids,
+ 'num_gallery_pids': num_pids - num_train_pids
+ }
+ splits.append(split)
+
+ # use cameraB as query and cameraA as gallery
+ split = {
+ 'train': train,
+ 'query': test_b,
+ 'gallery': test_a,
+ 'num_train_pids': num_train_pids,
+ 'num_query_pids': num_pids - num_train_pids,
+ 'num_gallery_pids': num_pids - num_train_pids
+ }
+ splits.append(split)
+
+ print('Totally {} splits are created'.format(len(splits)))
+ write_json(splits, self.split_path)
+ print('Split file saved to {}'.format(self.split_path))
diff --git a/trackers/strong_sort/deep/reid/torchreid/data/datasets/image/cuhk02.py b/trackers/strong_sort/deep/reid/torchreid/data/datasets/image/cuhk02.py
new file mode 100644
index 0000000000000000000000000000000000000000..dd92588d21fe6d48ca56e51e41d1e78f31095b38
--- /dev/null
+++ b/trackers/strong_sort/deep/reid/torchreid/data/datasets/image/cuhk02.py
@@ -0,0 +1,97 @@
+from __future__ import division, print_function, absolute_import
+import glob
+import os.path as osp
+
+from ..dataset import ImageDataset
+
+
+class CUHK02(ImageDataset):
+ """CUHK02.
+
+ Reference:
+ Li and Wang. Locally Aligned Feature Transforms across Views. CVPR 2013.
+
+ URL: ``_
+
+ Dataset statistics:
+ - 5 camera view pairs each with two cameras
+ - 971, 306, 107, 193 and 239 identities from P1 - P5
+ - totally 1,816 identities
+ - image format is png
+
+ Protocol: Use P1 - P4 for training and P5 for evaluation.
+
+ Note: CUHK01 and CUHK02 overlap.
+ """
+ dataset_dir = 'cuhk02'
+ cam_pairs = ['P1', 'P2', 'P3', 'P4', 'P5']
+ test_cam_pair = 'P5'
+
+ def __init__(self, root='', **kwargs):
+ self.root = osp.abspath(osp.expanduser(root))
+ self.dataset_dir = osp.join(self.root, self.dataset_dir, 'Dataset')
+
+ required_files = [self.dataset_dir]
+ self.check_before_run(required_files)
+
+ train, query, gallery = self.get_data_list()
+
+ super(CUHK02, self).__init__(train, query, gallery, **kwargs)
+
+ def get_data_list(self):
+ num_train_pids, camid = 0, 0
+ train, query, gallery = [], [], []
+
+ for cam_pair in self.cam_pairs:
+ cam_pair_dir = osp.join(self.dataset_dir, cam_pair)
+
+ cam1_dir = osp.join(cam_pair_dir, 'cam1')
+ cam2_dir = osp.join(cam_pair_dir, 'cam2')
+
+ impaths1 = glob.glob(osp.join(cam1_dir, '*.png'))
+ impaths2 = glob.glob(osp.join(cam2_dir, '*.png'))
+
+ if cam_pair == self.test_cam_pair:
+ # add images to query
+ for impath in impaths1:
+ pid = osp.basename(impath).split('_')[0]
+ pid = int(pid)
+ query.append((impath, pid, camid))
+ camid += 1
+
+ # add images to gallery
+ for impath in impaths2:
+ pid = osp.basename(impath).split('_')[0]
+ pid = int(pid)
+ gallery.append((impath, pid, camid))
+ camid += 1
+
+ else:
+ pids1 = [
+ osp.basename(impath).split('_')[0] for impath in impaths1
+ ]
+ pids2 = [
+ osp.basename(impath).split('_')[0] for impath in impaths2
+ ]
+ pids = set(pids1 + pids2)
+ pid2label = {
+ pid: label + num_train_pids
+ for label, pid in enumerate(pids)
+ }
+
+ # add images to train from cam1
+ for impath in impaths1:
+ pid = osp.basename(impath).split('_')[0]
+ pid = pid2label[pid]
+ train.append((impath, pid, camid))
+ camid += 1
+
+ # add images to train from cam2
+ for impath in impaths2:
+ pid = osp.basename(impath).split('_')[0]
+ pid = pid2label[pid]
+ train.append((impath, pid, camid))
+ camid += 1
+ num_train_pids += len(pids)
+
+ return train, query, gallery
diff --git a/trackers/strong_sort/deep/reid/torchreid/data/datasets/image/cuhk03.py b/trackers/strong_sort/deep/reid/torchreid/data/datasets/image/cuhk03.py
new file mode 100644
index 0000000000000000000000000000000000000000..cd27bc2fbd5dd345352559087aa27c1d7c088fd9
--- /dev/null
+++ b/trackers/strong_sort/deep/reid/torchreid/data/datasets/image/cuhk03.py
@@ -0,0 +1,307 @@
+from __future__ import division, print_function, absolute_import
+import os.path as osp
+
+from torchreid.utils import read_json, write_json, mkdir_if_missing
+
+from ..dataset import ImageDataset
+
+
+class CUHK03(ImageDataset):
+ """CUHK03.
+
+ Reference:
+ Li et al. DeepReID: Deep Filter Pairing Neural Network for Person Re-identification. CVPR 2014.
+
+ URL: ``_
+
+ Dataset statistics:
+ - identities: 1360.
+ - images: 13164.
+ - cameras: 6.
+ - splits: 20 (classic).
+ """
+ dataset_dir = 'cuhk03'
+ dataset_url = None
+
+ def __init__(
+ self,
+ root='',
+ split_id=0,
+ cuhk03_labeled=False,
+ cuhk03_classic_split=False,
+ **kwargs
+ ):
+ self.root = osp.abspath(osp.expanduser(root))
+ self.dataset_dir = osp.join(self.root, self.dataset_dir)
+ self.download_dataset(self.dataset_dir, self.dataset_url)
+
+ self.data_dir = osp.join(self.dataset_dir, 'cuhk03_release')
+ self.raw_mat_path = osp.join(self.data_dir, 'cuhk-03.mat')
+
+ self.imgs_detected_dir = osp.join(self.dataset_dir, 'images_detected')
+ self.imgs_labeled_dir = osp.join(self.dataset_dir, 'images_labeled')
+
+ self.split_classic_det_json_path = osp.join(
+ self.dataset_dir, 'splits_classic_detected.json'
+ )
+ self.split_classic_lab_json_path = osp.join(
+ self.dataset_dir, 'splits_classic_labeled.json'
+ )
+
+ self.split_new_det_json_path = osp.join(
+ self.dataset_dir, 'splits_new_detected.json'
+ )
+ self.split_new_lab_json_path = osp.join(
+ self.dataset_dir, 'splits_new_labeled.json'
+ )
+
+ self.split_new_det_mat_path = osp.join(
+ self.dataset_dir, 'cuhk03_new_protocol_config_detected.mat'
+ )
+ self.split_new_lab_mat_path = osp.join(
+ self.dataset_dir, 'cuhk03_new_protocol_config_labeled.mat'
+ )
+
+ required_files = [
+ self.dataset_dir, self.data_dir, self.raw_mat_path,
+ self.split_new_det_mat_path, self.split_new_lab_mat_path
+ ]
+ self.check_before_run(required_files)
+
+ self.preprocess_split()
+
+ if cuhk03_labeled:
+ split_path = self.split_classic_lab_json_path if cuhk03_classic_split else self.split_new_lab_json_path
+ else:
+ split_path = self.split_classic_det_json_path if cuhk03_classic_split else self.split_new_det_json_path
+
+ splits = read_json(split_path)
+ assert split_id < len(
+ splits
+ ), 'Condition split_id ({}) < len(splits) ({}) is false'.format(
+ split_id, len(splits)
+ )
+ split = splits[split_id]
+
+ train = split['train']
+ query = split['query']
+ gallery = split['gallery']
+
+ super(CUHK03, self).__init__(train, query, gallery, **kwargs)
+
+ def preprocess_split(self):
+ # This function is a bit complex and ugly, what it does is
+ # 1. extract data from cuhk-03.mat and save as png images
+ # 2. create 20 classic splits (Li et al. CVPR'14)
+ # 3. create new split (Zhong et al. CVPR'17)
+ if osp.exists(self.imgs_labeled_dir) \
+ and osp.exists(self.imgs_detected_dir) \
+ and osp.exists(self.split_classic_det_json_path) \
+ and osp.exists(self.split_classic_lab_json_path) \
+ and osp.exists(self.split_new_det_json_path) \
+ and osp.exists(self.split_new_lab_json_path):
+ return
+
+ import h5py
+ import imageio
+ from scipy.io import loadmat
+
+ mkdir_if_missing(self.imgs_detected_dir)
+ mkdir_if_missing(self.imgs_labeled_dir)
+
+ print(
+ 'Extract image data from "{}" and save as png'.format(
+ self.raw_mat_path
+ )
+ )
+ mat = h5py.File(self.raw_mat_path, 'r')
+
+ def _deref(ref):
+ return mat[ref][:].T
+
+ def _process_images(img_refs, campid, pid, save_dir):
+ img_paths = [] # Note: some persons only have images for one view
+ for imgid, img_ref in enumerate(img_refs):
+ img = _deref(img_ref)
+ if img.size == 0 or img.ndim < 3:
+ continue # skip empty cell
+ # images are saved with the following format, index-1 (ensure uniqueness)
+ # campid: index of camera pair (1-5)
+ # pid: index of person in 'campid'-th camera pair
+ # viewid: index of view, {1, 2}
+ # imgid: index of image, (1-10)
+ viewid = 1 if imgid < 5 else 2
+ img_name = '{:01d}_{:03d}_{:01d}_{:02d}.png'.format(
+ campid + 1, pid + 1, viewid, imgid + 1
+ )
+ img_path = osp.join(save_dir, img_name)
+ if not osp.isfile(img_path):
+ imageio.imwrite(img_path, img)
+ img_paths.append(img_path)
+ return img_paths
+
+ def _extract_img(image_type):
+ print('Processing {} images ...'.format(image_type))
+ meta_data = []
+ imgs_dir = self.imgs_detected_dir if image_type == 'detected' else self.imgs_labeled_dir
+ for campid, camp_ref in enumerate(mat[image_type][0]):
+ camp = _deref(camp_ref)
+ num_pids = camp.shape[0]
+ for pid in range(num_pids):
+ img_paths = _process_images(
+ camp[pid, :], campid, pid, imgs_dir
+ )
+ assert len(img_paths) > 0, \
+ 'campid{}-pid{} has no images'.format(campid, pid)
+ meta_data.append((campid + 1, pid + 1, img_paths))
+ print(
+ '- done camera pair {} with {} identities'.format(
+ campid + 1, num_pids
+ )
+ )
+ return meta_data
+
+ meta_detected = _extract_img('detected')
+ meta_labeled = _extract_img('labeled')
+
+ def _extract_classic_split(meta_data, test_split):
+ train, test = [], []
+ num_train_pids, num_test_pids = 0, 0
+ num_train_imgs, num_test_imgs = 0, 0
+ for i, (campid, pid, img_paths) in enumerate(meta_data):
+
+ if [campid, pid] in test_split:
+ for img_path in img_paths:
+ camid = int(
+ osp.basename(img_path).split('_')[2]
+ ) - 1 # make it 0-based
+ test.append((img_path, num_test_pids, camid))
+ num_test_pids += 1
+ num_test_imgs += len(img_paths)
+ else:
+ for img_path in img_paths:
+ camid = int(
+ osp.basename(img_path).split('_')[2]
+ ) - 1 # make it 0-based
+ train.append((img_path, num_train_pids, camid))
+ num_train_pids += 1
+ num_train_imgs += len(img_paths)
+ return train, num_train_pids, num_train_imgs, test, num_test_pids, num_test_imgs
+
+ print('Creating classic splits (# = 20) ...')
+ splits_classic_det, splits_classic_lab = [], []
+ for split_ref in mat['testsets'][0]:
+ test_split = _deref(split_ref).tolist()
+
+ # create split for detected images
+ train, num_train_pids, num_train_imgs, test, num_test_pids, num_test_imgs = \
+ _extract_classic_split(meta_detected, test_split)
+ splits_classic_det.append(
+ {
+ 'train': train,
+ 'query': test,
+ 'gallery': test,
+ 'num_train_pids': num_train_pids,
+ 'num_train_imgs': num_train_imgs,
+ 'num_query_pids': num_test_pids,
+ 'num_query_imgs': num_test_imgs,
+ 'num_gallery_pids': num_test_pids,
+ 'num_gallery_imgs': num_test_imgs
+ }
+ )
+
+ # create split for labeled images
+ train, num_train_pids, num_train_imgs, test, num_test_pids, num_test_imgs = \
+ _extract_classic_split(meta_labeled, test_split)
+ splits_classic_lab.append(
+ {
+ 'train': train,
+ 'query': test,
+ 'gallery': test,
+ 'num_train_pids': num_train_pids,
+ 'num_train_imgs': num_train_imgs,
+ 'num_query_pids': num_test_pids,
+ 'num_query_imgs': num_test_imgs,
+ 'num_gallery_pids': num_test_pids,
+ 'num_gallery_imgs': num_test_imgs
+ }
+ )
+
+ write_json(splits_classic_det, self.split_classic_det_json_path)
+ write_json(splits_classic_lab, self.split_classic_lab_json_path)
+
+ def _extract_set(filelist, pids, pid2label, idxs, img_dir, relabel):
+ tmp_set = []
+ unique_pids = set()
+ for idx in idxs:
+ img_name = filelist[idx][0]
+ camid = int(img_name.split('_')[2]) - 1 # make it 0-based
+ pid = pids[idx]
+ if relabel:
+ pid = pid2label[pid]
+ img_path = osp.join(img_dir, img_name)
+ tmp_set.append((img_path, int(pid), camid))
+ unique_pids.add(pid)
+ return tmp_set, len(unique_pids), len(idxs)
+
+ def _extract_new_split(split_dict, img_dir):
+ train_idxs = split_dict['train_idx'].flatten() - 1 # index-0
+ pids = split_dict['labels'].flatten()
+ train_pids = set(pids[train_idxs])
+ pid2label = {pid: label for label, pid in enumerate(train_pids)}
+ query_idxs = split_dict['query_idx'].flatten() - 1
+ gallery_idxs = split_dict['gallery_idx'].flatten() - 1
+ filelist = split_dict['filelist'].flatten()
+ train_info = _extract_set(
+ filelist, pids, pid2label, train_idxs, img_dir, relabel=True
+ )
+ query_info = _extract_set(
+ filelist, pids, pid2label, query_idxs, img_dir, relabel=False
+ )
+ gallery_info = _extract_set(
+ filelist,
+ pids,
+ pid2label,
+ gallery_idxs,
+ img_dir,
+ relabel=False
+ )
+ return train_info, query_info, gallery_info
+
+ print('Creating new split for detected images (767/700) ...')
+ train_info, query_info, gallery_info = _extract_new_split(
+ loadmat(self.split_new_det_mat_path), self.imgs_detected_dir
+ )
+ split = [
+ {
+ 'train': train_info[0],
+ 'query': query_info[0],
+ 'gallery': gallery_info[0],
+ 'num_train_pids': train_info[1],
+ 'num_train_imgs': train_info[2],
+ 'num_query_pids': query_info[1],
+ 'num_query_imgs': query_info[2],
+ 'num_gallery_pids': gallery_info[1],
+ 'num_gallery_imgs': gallery_info[2]
+ }
+ ]
+ write_json(split, self.split_new_det_json_path)
+
+ print('Creating new split for labeled images (767/700) ...')
+ train_info, query_info, gallery_info = _extract_new_split(
+ loadmat(self.split_new_lab_mat_path), self.imgs_labeled_dir
+ )
+ split = [
+ {
+ 'train': train_info[0],
+ 'query': query_info[0],
+ 'gallery': gallery_info[0],
+ 'num_train_pids': train_info[1],
+ 'num_train_imgs': train_info[2],
+ 'num_query_pids': query_info[1],
+ 'num_query_imgs': query_info[2],
+ 'num_gallery_pids': gallery_info[1],
+ 'num_gallery_imgs': gallery_info[2]
+ }
+ ]
+ write_json(split, self.split_new_lab_json_path)
diff --git a/trackers/strong_sort/deep/reid/torchreid/data/datasets/image/cuhksysu.py b/trackers/strong_sort/deep/reid/torchreid/data/datasets/image/cuhksysu.py
new file mode 100644
index 0000000000000000000000000000000000000000..f6c9edd74d23732be4477c7c5b9a5e2fe1464c96
--- /dev/null
+++ b/trackers/strong_sort/deep/reid/torchreid/data/datasets/image/cuhksysu.py
@@ -0,0 +1,60 @@
+from __future__ import division, print_function, absolute_import
+import copy
+import glob
+import os.path as osp
+
+from ..dataset import ImageDataset
+
+
+class CUHKSYSU(ImageDataset):
+ """CUHKSYSU.
+
+ This dataset can only be used for model training.
+
+ Reference:
+ Xiao et al. End-to-end deep learning for person search.
+
+ URL: ``_
+
+ Dataset statistics:
+ - identities: 11,934
+ - images: 34,574
+ """
+ _train_only = True
+ dataset_dir = 'cuhksysu'
+
+ def __init__(self, root='', **kwargs):
+ self.root = osp.abspath(osp.expanduser(root))
+ self.dataset_dir = osp.join(self.root, self.dataset_dir)
+ self.data_dir = osp.join(self.dataset_dir, 'cropped_images')
+
+ # image name format: p11422_s16929_1.jpg
+ train = self.process_dir(self.data_dir)
+ query = [copy.deepcopy(train[0])]
+ gallery = [copy.deepcopy(train[0])]
+
+ super(CUHKSYSU, self).__init__(train, query, gallery, **kwargs)
+
+ def process_dir(self, dirname):
+ img_paths = glob.glob(osp.join(dirname, '*.jpg'))
+ # num_imgs = len(img_paths)
+
+ # get all identities:
+ pid_container = set()
+ for img_path in img_paths:
+ img_name = osp.basename(img_path)
+ pid = img_name.split('_')[0]
+ pid_container.add(pid)
+ pid2label = {pid: label for label, pid in enumerate(pid_container)}
+
+ # num_pids = len(pid_container)
+
+ # extract data
+ data = []
+ for img_path in img_paths:
+ img_name = osp.basename(img_path)
+ pid = img_name.split('_')[0]
+ label = pid2label[pid]
+ data.append((img_path, label, 0)) # dummy camera id
+
+ return data
diff --git a/trackers/strong_sort/deep/reid/torchreid/data/datasets/image/dukemtmcreid.py b/trackers/strong_sort/deep/reid/torchreid/data/datasets/image/dukemtmcreid.py
new file mode 100644
index 0000000000000000000000000000000000000000..5915da51bf831824e5b2207d8938fbc3707c4e61
--- /dev/null
+++ b/trackers/strong_sort/deep/reid/torchreid/data/datasets/image/dukemtmcreid.py
@@ -0,0 +1,68 @@
+from __future__ import division, print_function, absolute_import
+import re
+import glob
+import os.path as osp
+
+from ..dataset import ImageDataset
+
+
+class DukeMTMCreID(ImageDataset):
+ """DukeMTMC-reID.
+
+ Reference:
+ - Ristani et al. Performance Measures and a Data Set for Multi-Target, Multi-Camera Tracking. ECCVW 2016.
+ - Zheng et al. Unlabeled Samples Generated by GAN Improve the Person Re-identification Baseline in vitro. ICCV 2017.
+
+ URL: ``_
+
+ Dataset statistics:
+ - identities: 1404 (train + query).
+ - images:16522 (train) + 2228 (query) + 17661 (gallery).
+ - cameras: 8.
+ """
+ dataset_dir = 'dukemtmc-reid'
+ dataset_url = 'http://vision.cs.duke.edu/DukeMTMC/data/misc/DukeMTMC-reID.zip'
+
+ def __init__(self, root='', **kwargs):
+ self.root = osp.abspath(osp.expanduser(root))
+ self.dataset_dir = osp.join(self.root, self.dataset_dir)
+ self.download_dataset(self.dataset_dir, self.dataset_url)
+ self.train_dir = osp.join(
+ self.dataset_dir, 'DukeMTMC-reID/bounding_box_train'
+ )
+ self.query_dir = osp.join(self.dataset_dir, 'DukeMTMC-reID/query')
+ self.gallery_dir = osp.join(
+ self.dataset_dir, 'DukeMTMC-reID/bounding_box_test'
+ )
+
+ required_files = [
+ self.dataset_dir, self.train_dir, self.query_dir, self.gallery_dir
+ ]
+ self.check_before_run(required_files)
+
+ train = self.process_dir(self.train_dir, relabel=True)
+ query = self.process_dir(self.query_dir, relabel=False)
+ gallery = self.process_dir(self.gallery_dir, relabel=False)
+
+ super(DukeMTMCreID, self).__init__(train, query, gallery, **kwargs)
+
+ def process_dir(self, dir_path, relabel=False):
+ img_paths = glob.glob(osp.join(dir_path, '*.jpg'))
+ pattern = re.compile(r'([-\d]+)_c(\d)')
+
+ pid_container = set()
+ for img_path in img_paths:
+ pid, _ = map(int, pattern.search(img_path).groups())
+ pid_container.add(pid)
+ pid2label = {pid: label for label, pid in enumerate(pid_container)}
+
+ data = []
+ for img_path in img_paths:
+ pid, camid = map(int, pattern.search(img_path).groups())
+ assert 1 <= camid <= 8
+ camid -= 1 # index starts from 0
+ if relabel:
+ pid = pid2label[pid]
+ data.append((img_path, pid, camid))
+
+ return data
diff --git a/trackers/strong_sort/deep/reid/torchreid/data/datasets/image/grid.py b/trackers/strong_sort/deep/reid/torchreid/data/datasets/image/grid.py
new file mode 100644
index 0000000000000000000000000000000000000000..96023d62ea1cbb4eae2172184e6fd48c941c81d1
--- /dev/null
+++ b/trackers/strong_sort/deep/reid/torchreid/data/datasets/image/grid.py
@@ -0,0 +1,131 @@
+from __future__ import division, print_function, absolute_import
+import glob
+import os.path as osp
+from scipy.io import loadmat
+
+from torchreid.utils import read_json, write_json
+
+from ..dataset import ImageDataset
+
+
+class GRID(ImageDataset):
+ """GRID.
+
+ Reference:
+ Loy et al. Multi-camera activity correlation analysis. CVPR 2009.
+
+ URL: ``_
+
+ Dataset statistics:
+ - identities: 250.
+ - images: 1275.
+ - cameras: 8.
+ """
+ dataset_dir = 'grid'
+ dataset_url = 'http://personal.ie.cuhk.edu.hk/~ccloy/files/datasets/underground_reid.zip'
+ _junk_pids = [0]
+
+ def __init__(self, root='', split_id=0, **kwargs):
+ self.root = osp.abspath(osp.expanduser(root))
+ self.dataset_dir = osp.join(self.root, self.dataset_dir)
+ self.download_dataset(self.dataset_dir, self.dataset_url)
+
+ self.probe_path = osp.join(
+ self.dataset_dir, 'underground_reid', 'probe'
+ )
+ self.gallery_path = osp.join(
+ self.dataset_dir, 'underground_reid', 'gallery'
+ )
+ self.split_mat_path = osp.join(
+ self.dataset_dir, 'underground_reid', 'features_and_partitions.mat'
+ )
+ self.split_path = osp.join(self.dataset_dir, 'splits.json')
+
+ required_files = [
+ self.dataset_dir, self.probe_path, self.gallery_path,
+ self.split_mat_path
+ ]
+ self.check_before_run(required_files)
+
+ self.prepare_split()
+ splits = read_json(self.split_path)
+ if split_id >= len(splits):
+ raise ValueError(
+ 'split_id exceeds range, received {}, '
+ 'but expected between 0 and {}'.format(
+ split_id,
+ len(splits) - 1
+ )
+ )
+ split = splits[split_id]
+
+ train = split['train']
+ query = split['query']
+ gallery = split['gallery']
+
+ train = [tuple(item) for item in train]
+ query = [tuple(item) for item in query]
+ gallery = [tuple(item) for item in gallery]
+
+ super(GRID, self).__init__(train, query, gallery, **kwargs)
+
+ def prepare_split(self):
+ if not osp.exists(self.split_path):
+ print('Creating 10 random splits')
+ split_mat = loadmat(self.split_mat_path)
+ trainIdxAll = split_mat['trainIdxAll'][0] # length = 10
+ probe_img_paths = sorted(
+ glob.glob(osp.join(self.probe_path, '*.jpeg'))
+ )
+ gallery_img_paths = sorted(
+ glob.glob(osp.join(self.gallery_path, '*.jpeg'))
+ )
+
+ splits = []
+ for split_idx in range(10):
+ train_idxs = trainIdxAll[split_idx][0][0][2][0].tolist()
+ assert len(train_idxs) == 125
+ idx2label = {
+ idx: label
+ for label, idx in enumerate(train_idxs)
+ }
+
+ train, query, gallery = [], [], []
+
+ # processing probe folder
+ for img_path in probe_img_paths:
+ img_name = osp.basename(img_path)
+ img_idx = int(img_name.split('_')[0])
+ camid = int(
+ img_name.split('_')[1]
+ ) - 1 # index starts from 0
+ if img_idx in train_idxs:
+ train.append((img_path, idx2label[img_idx], camid))
+ else:
+ query.append((img_path, img_idx, camid))
+
+ # process gallery folder
+ for img_path in gallery_img_paths:
+ img_name = osp.basename(img_path)
+ img_idx = int(img_name.split('_')[0])
+ camid = int(
+ img_name.split('_')[1]
+ ) - 1 # index starts from 0
+ if img_idx in train_idxs:
+ train.append((img_path, idx2label[img_idx], camid))
+ else:
+ gallery.append((img_path, img_idx, camid))
+
+ split = {
+ 'train': train,
+ 'query': query,
+ 'gallery': gallery,
+ 'num_train_pids': 125,
+ 'num_query_pids': 125,
+ 'num_gallery_pids': 900
+ }
+ splits.append(split)
+
+ print('Totally {} splits are created'.format(len(splits)))
+ write_json(splits, self.split_path)
+ print('Split file saved to {}'.format(self.split_path))
diff --git a/trackers/strong_sort/deep/reid/torchreid/data/datasets/image/ilids.py b/trackers/strong_sort/deep/reid/torchreid/data/datasets/image/ilids.py
new file mode 100644
index 0000000000000000000000000000000000000000..42971b03c020fb42c23e74a64ee20699220be962
--- /dev/null
+++ b/trackers/strong_sort/deep/reid/torchreid/data/datasets/image/ilids.py
@@ -0,0 +1,135 @@
+from __future__ import division, print_function, absolute_import
+import copy
+import glob
+import random
+import os.path as osp
+from collections import defaultdict
+
+from torchreid.utils import read_json, write_json
+
+from ..dataset import ImageDataset
+
+
+class iLIDS(ImageDataset):
+ """QMUL-iLIDS.
+
+ Reference:
+ Zheng et al. Associating Groups of People. BMVC 2009.
+
+ Dataset statistics:
+ - identities: 119.
+ - images: 476.
+ - cameras: 8 (not explicitly provided).
+ """
+ dataset_dir = 'ilids'
+ dataset_url = 'http://www.eecs.qmul.ac.uk/~jason/data/i-LIDS_Pedestrian.tgz'
+
+ def __init__(self, root='', split_id=0, **kwargs):
+ self.root = osp.abspath(osp.expanduser(root))
+ self.dataset_dir = osp.join(self.root, self.dataset_dir)
+ self.download_dataset(self.dataset_dir, self.dataset_url)
+
+ self.data_dir = osp.join(self.dataset_dir, 'i-LIDS_Pedestrian/Persons')
+ self.split_path = osp.join(self.dataset_dir, 'splits.json')
+
+ required_files = [self.dataset_dir, self.data_dir]
+ self.check_before_run(required_files)
+
+ self.prepare_split()
+ splits = read_json(self.split_path)
+ if split_id >= len(splits):
+ raise ValueError(
+ 'split_id exceeds range, received {}, but '
+ 'expected between 0 and {}'.format(split_id,
+ len(splits) - 1)
+ )
+ split = splits[split_id]
+
+ train, query, gallery = self.process_split(split)
+
+ super(iLIDS, self).__init__(train, query, gallery, **kwargs)
+
+ def prepare_split(self):
+ if not osp.exists(self.split_path):
+ print('Creating splits ...')
+
+ paths = glob.glob(osp.join(self.data_dir, '*.jpg'))
+ img_names = [osp.basename(path) for path in paths]
+ num_imgs = len(img_names)
+ assert num_imgs == 476, 'There should be 476 images, but ' \
+ 'got {}, please check the data'.format(num_imgs)
+
+ # store image names
+ # image naming format:
+ # the first four digits denote the person ID
+ # the last four digits denote the sequence index
+ pid_dict = defaultdict(list)
+ for img_name in img_names:
+ pid = int(img_name[:4])
+ pid_dict[pid].append(img_name)
+ pids = list(pid_dict.keys())
+ num_pids = len(pids)
+ assert num_pids == 119, 'There should be 119 identities, ' \
+ 'but got {}, please check the data'.format(num_pids)
+
+ num_train_pids = int(num_pids * 0.5)
+
+ splits = []
+ for _ in range(10):
+ # randomly choose num_train_pids train IDs and the rest for test IDs
+ pids_copy = copy.deepcopy(pids)
+ random.shuffle(pids_copy)
+ train_pids = pids_copy[:num_train_pids]
+ test_pids = pids_copy[num_train_pids:]
+
+ train = []
+ query = []
+ gallery = []
+
+ # for train IDs, all images are used in the train set.
+ for pid in train_pids:
+ img_names = pid_dict[pid]
+ train.extend(img_names)
+
+ # for each test ID, randomly choose two images, one for
+ # query and the other one for gallery.
+ for pid in test_pids:
+ img_names = pid_dict[pid]
+ samples = random.sample(img_names, 2)
+ query.append(samples[0])
+ gallery.append(samples[1])
+
+ split = {'train': train, 'query': query, 'gallery': gallery}
+ splits.append(split)
+
+ print('Totally {} splits are created'.format(len(splits)))
+ write_json(splits, self.split_path)
+ print('Split file is saved to {}'.format(self.split_path))
+
+ def get_pid2label(self, img_names):
+ pid_container = set()
+ for img_name in img_names:
+ pid = int(img_name[:4])
+ pid_container.add(pid)
+ pid2label = {pid: label for label, pid in enumerate(pid_container)}
+ return pid2label
+
+ def parse_img_names(self, img_names, pid2label=None):
+ data = []
+
+ for img_name in img_names:
+ pid = int(img_name[:4])
+ if pid2label is not None:
+ pid = pid2label[pid]
+ camid = int(img_name[4:7]) - 1 # 0-based
+ img_path = osp.join(self.data_dir, img_name)
+ data.append((img_path, pid, camid))
+
+ return data
+
+ def process_split(self, split):
+ train_pid2label = self.get_pid2label(split['train'])
+ train = self.parse_img_names(split['train'], train_pid2label)
+ query = self.parse_img_names(split['query'])
+ gallery = self.parse_img_names(split['gallery'])
+ return train, query, gallery
diff --git a/trackers/strong_sort/deep/reid/torchreid/data/datasets/image/market1501.py b/trackers/strong_sort/deep/reid/torchreid/data/datasets/image/market1501.py
new file mode 100644
index 0000000000000000000000000000000000000000..7d138d1119cf2811a2570c265849d9f74f6a721d
--- /dev/null
+++ b/trackers/strong_sort/deep/reid/torchreid/data/datasets/image/market1501.py
@@ -0,0 +1,88 @@
+from __future__ import division, print_function, absolute_import
+import re
+import glob
+import os.path as osp
+import warnings
+
+from ..dataset import ImageDataset
+
+
+class Market1501(ImageDataset):
+ """Market1501.
+
+ Reference:
+ Zheng et al. Scalable Person Re-identification: A Benchmark. ICCV 2015.
+
+ URL: ``_
+
+ Dataset statistics:
+ - identities: 1501 (+1 for background).
+ - images: 12936 (train) + 3368 (query) + 15913 (gallery).
+ """
+ _junk_pids = [0, -1]
+ dataset_dir = 'market1501'
+ dataset_url = 'http://188.138.127.15:81/Datasets/Market-1501-v15.09.15.zip'
+
+ def __init__(self, root='', market1501_500k=False, **kwargs):
+ self.root = osp.abspath(osp.expanduser(root))
+ self.dataset_dir = osp.join(self.root, self.dataset_dir)
+ self.download_dataset(self.dataset_dir, self.dataset_url)
+
+ # allow alternative directory structure
+ self.data_dir = self.dataset_dir
+ data_dir = osp.join(self.data_dir, 'Market-1501-v15.09.15')
+ if osp.isdir(data_dir):
+ self.data_dir = data_dir
+ else:
+ warnings.warn(
+ 'The current data structure is deprecated. Please '
+ 'put data folders such as "bounding_box_train" under '
+ '"Market-1501-v15.09.15".'
+ )
+
+ self.train_dir = osp.join(self.data_dir, 'bounding_box_train')
+ self.query_dir = osp.join(self.data_dir, 'query')
+ self.gallery_dir = osp.join(self.data_dir, 'bounding_box_test')
+ self.extra_gallery_dir = osp.join(self.data_dir, 'images')
+ self.market1501_500k = market1501_500k
+
+ required_files = [
+ self.data_dir, self.train_dir, self.query_dir, self.gallery_dir
+ ]
+ if self.market1501_500k:
+ required_files.append(self.extra_gallery_dir)
+ self.check_before_run(required_files)
+
+ train = self.process_dir(self.train_dir, relabel=True)
+ query = self.process_dir(self.query_dir, relabel=False)
+ gallery = self.process_dir(self.gallery_dir, relabel=False)
+ if self.market1501_500k:
+ gallery += self.process_dir(self.extra_gallery_dir, relabel=False)
+
+ super(Market1501, self).__init__(train, query, gallery, **kwargs)
+
+ def process_dir(self, dir_path, relabel=False):
+ img_paths = glob.glob(osp.join(dir_path, '*.jpg'))
+ pattern = re.compile(r'([-\d]+)_c(\d)')
+
+ pid_container = set()
+ for img_path in img_paths:
+ pid, _ = map(int, pattern.search(img_path).groups())
+ if pid == -1:
+ continue # junk images are just ignored
+ pid_container.add(pid)
+ pid2label = {pid: label for label, pid in enumerate(pid_container)}
+
+ data = []
+ for img_path in img_paths:
+ pid, camid = map(int, pattern.search(img_path).groups())
+ if pid == -1:
+ continue # junk images are just ignored
+ assert 0 <= pid <= 1501 # pid == 0 means background
+ assert 1 <= camid <= 6
+ camid -= 1 # index starts from 0
+ if relabel:
+ pid = pid2label[pid]
+ data.append((img_path, pid, camid))
+
+ return data
diff --git a/trackers/strong_sort/deep/reid/torchreid/data/datasets/image/msmt17.py b/trackers/strong_sort/deep/reid/torchreid/data/datasets/image/msmt17.py
new file mode 100644
index 0000000000000000000000000000000000000000..c4741e61ea02f0fc58e6941f4058a76b9b4ccefc
--- /dev/null
+++ b/trackers/strong_sort/deep/reid/torchreid/data/datasets/image/msmt17.py
@@ -0,0 +1,98 @@
+from __future__ import division, print_function, absolute_import
+import os.path as osp
+
+from ..dataset import ImageDataset
+
+# Log
+# 22.01.2019
+# - add v2
+# - v1 and v2 differ in dir names
+# - note that faces in v2 are blurred
+TRAIN_DIR_KEY = 'train_dir'
+TEST_DIR_KEY = 'test_dir'
+VERSION_DICT = {
+ 'MSMT17_V1': {
+ TRAIN_DIR_KEY: 'train',
+ TEST_DIR_KEY: 'test',
+ },
+ 'MSMT17_V2': {
+ TRAIN_DIR_KEY: 'mask_train_v2',
+ TEST_DIR_KEY: 'mask_test_v2',
+ }
+}
+
+
+class MSMT17(ImageDataset):
+ """MSMT17.
+
+ Reference:
+ Wei et al. Person Transfer GAN to Bridge Domain Gap for Person Re-Identification. CVPR 2018.
+
+ URL: ``_
+
+ Dataset statistics:
+ - identities: 4101.
+ - images: 32621 (train) + 11659 (query) + 82161 (gallery).
+ - cameras: 15.
+ """
+ dataset_dir = 'msmt17'
+ dataset_url = None
+
+ def __init__(self, root='', **kwargs):
+ self.root = osp.abspath(osp.expanduser(root))
+ self.dataset_dir = osp.join(self.root, self.dataset_dir)
+ self.download_dataset(self.dataset_dir, self.dataset_url)
+
+ has_main_dir = False
+ for main_dir in VERSION_DICT:
+ if osp.exists(osp.join(self.dataset_dir, main_dir)):
+ train_dir = VERSION_DICT[main_dir][TRAIN_DIR_KEY]
+ test_dir = VERSION_DICT[main_dir][TEST_DIR_KEY]
+ has_main_dir = True
+ break
+ assert has_main_dir, 'Dataset folder not found'
+
+ self.train_dir = osp.join(self.dataset_dir, main_dir, train_dir)
+ self.test_dir = osp.join(self.dataset_dir, main_dir, test_dir)
+ self.list_train_path = osp.join(
+ self.dataset_dir, main_dir, 'list_train.txt'
+ )
+ self.list_val_path = osp.join(
+ self.dataset_dir, main_dir, 'list_val.txt'
+ )
+ self.list_query_path = osp.join(
+ self.dataset_dir, main_dir, 'list_query.txt'
+ )
+ self.list_gallery_path = osp.join(
+ self.dataset_dir, main_dir, 'list_gallery.txt'
+ )
+
+ required_files = [self.dataset_dir, self.train_dir, self.test_dir]
+ self.check_before_run(required_files)
+
+ train = self.process_dir(self.train_dir, self.list_train_path)
+ val = self.process_dir(self.train_dir, self.list_val_path)
+ query = self.process_dir(self.test_dir, self.list_query_path)
+ gallery = self.process_dir(self.test_dir, self.list_gallery_path)
+
+ # Note: to fairly compare with published methods on the conventional ReID setting,
+ # do not add val images to the training set.
+ if 'combineall' in kwargs and kwargs['combineall']:
+ train += val
+
+ super(MSMT17, self).__init__(train, query, gallery, **kwargs)
+
+ def process_dir(self, dir_path, list_path):
+ with open(list_path, 'r') as txt:
+ lines = txt.readlines()
+
+ data = []
+
+ for img_idx, img_info in enumerate(lines):
+ img_path, pid = img_info.split(' ')
+ pid = int(pid) # no need to relabel
+ camid = int(img_path.split('_')[2]) - 1 # index starts from 0
+ img_path = osp.join(dir_path, img_path)
+ data.append((img_path, pid, camid))
+
+ return data
diff --git a/trackers/strong_sort/deep/reid/torchreid/data/datasets/image/prid.py b/trackers/strong_sort/deep/reid/torchreid/data/datasets/image/prid.py
new file mode 100644
index 0000000000000000000000000000000000000000..d6d6c2058ac48ccb2e793680ee34e6d8d25bcede
--- /dev/null
+++ b/trackers/strong_sort/deep/reid/torchreid/data/datasets/image/prid.py
@@ -0,0 +1,107 @@
+from __future__ import division, print_function, absolute_import
+import random
+import os.path as osp
+
+from torchreid.utils import read_json, write_json
+
+from ..dataset import ImageDataset
+
+
+class PRID(ImageDataset):
+ """PRID (single-shot version of prid-2011)
+
+ Reference:
+ Hirzer et al. Person Re-Identification by Descriptive and Discriminative
+ Classification. SCIA 2011.
+
+ URL: ``_
+
+ Dataset statistics:
+ - Two views.
+ - View A captures 385 identities.
+ - View B captures 749 identities.
+ - 200 identities appear in both views (index starts from 1 to 200).
+ """
+ dataset_dir = 'prid2011'
+ dataset_url = None
+ _junk_pids = list(range(201, 750))
+
+ def __init__(self, root='', split_id=0, **kwargs):
+ self.root = osp.abspath(osp.expanduser(root))
+ self.dataset_dir = osp.join(self.root, self.dataset_dir)
+ self.download_dataset(self.dataset_dir, self.dataset_url)
+
+ self.cam_a_dir = osp.join(
+ self.dataset_dir, 'prid_2011', 'single_shot', 'cam_a'
+ )
+ self.cam_b_dir = osp.join(
+ self.dataset_dir, 'prid_2011', 'single_shot', 'cam_b'
+ )
+ self.split_path = osp.join(self.dataset_dir, 'splits_single_shot.json')
+
+ required_files = [self.dataset_dir, self.cam_a_dir, self.cam_b_dir]
+ self.check_before_run(required_files)
+
+ self.prepare_split()
+ splits = read_json(self.split_path)
+ if split_id >= len(splits):
+ raise ValueError(
+ 'split_id exceeds range, received {}, but expected between 0 and {}'
+ .format(split_id,
+ len(splits) - 1)
+ )
+ split = splits[split_id]
+
+ train, query, gallery = self.process_split(split)
+
+ super(PRID, self).__init__(train, query, gallery, **kwargs)
+
+ def prepare_split(self):
+ if not osp.exists(self.split_path):
+ print('Creating splits ...')
+
+ splits = []
+ for _ in range(10):
+ # randomly sample 100 IDs for train and use the rest 100 IDs for test
+ # (note: there are only 200 IDs appearing in both views)
+ pids = [i for i in range(1, 201)]
+ train_pids = random.sample(pids, 100)
+ train_pids.sort()
+ test_pids = [i for i in pids if i not in train_pids]
+ split = {'train': train_pids, 'test': test_pids}
+ splits.append(split)
+
+ print('Totally {} splits are created'.format(len(splits)))
+ write_json(splits, self.split_path)
+ print('Split file is saved to {}'.format(self.split_path))
+
+ def process_split(self, split):
+ train_pids = split['train']
+ test_pids = split['test']
+
+ train_pid2label = {pid: label for label, pid in enumerate(train_pids)}
+
+ # train
+ train = []
+ for pid in train_pids:
+ img_name = 'person_' + str(pid).zfill(4) + '.png'
+ pid = train_pid2label[pid]
+ img_a_path = osp.join(self.cam_a_dir, img_name)
+ train.append((img_a_path, pid, 0))
+ img_b_path = osp.join(self.cam_b_dir, img_name)
+ train.append((img_b_path, pid, 1))
+
+ # query and gallery
+ query, gallery = [], []
+ for pid in test_pids:
+ img_name = 'person_' + str(pid).zfill(4) + '.png'
+ img_a_path = osp.join(self.cam_a_dir, img_name)
+ query.append((img_a_path, pid, 0))
+ img_b_path = osp.join(self.cam_b_dir, img_name)
+ gallery.append((img_b_path, pid, 1))
+ for pid in range(201, 750):
+ img_name = 'person_' + str(pid).zfill(4) + '.png'
+ img_b_path = osp.join(self.cam_b_dir, img_name)
+ gallery.append((img_b_path, pid, 1))
+
+ return train, query, gallery
diff --git a/trackers/strong_sort/deep/reid/torchreid/data/datasets/image/sensereid.py b/trackers/strong_sort/deep/reid/torchreid/data/datasets/image/sensereid.py
new file mode 100644
index 0000000000000000000000000000000000000000..7cf5f3246665a4b4f45275a8b2c7351b0cd4ad48
--- /dev/null
+++ b/trackers/strong_sort/deep/reid/torchreid/data/datasets/image/sensereid.py
@@ -0,0 +1,70 @@
+from __future__ import division, print_function, absolute_import
+import copy
+import glob
+import os.path as osp
+
+from ..dataset import ImageDataset
+
+
+class SenseReID(ImageDataset):
+ """SenseReID.
+
+ This dataset is used for test purpose only.
+
+ Reference:
+ Zhao et al. Spindle Net: Person Re-identification with Human Body
+ Region Guided Feature Decomposition and Fusion. CVPR 2017.
+
+ URL: ``_
+
+ Dataset statistics:
+ - query: 522 ids, 1040 images.
+ - gallery: 1717 ids, 3388 images.
+ """
+ dataset_dir = 'sensereid'
+ dataset_url = None
+
+ def __init__(self, root='', **kwargs):
+ self.root = osp.abspath(osp.expanduser(root))
+ self.dataset_dir = osp.join(self.root, self.dataset_dir)
+ self.download_dataset(self.dataset_dir, self.dataset_url)
+
+ self.query_dir = osp.join(self.dataset_dir, 'SenseReID', 'test_probe')
+ self.gallery_dir = osp.join(
+ self.dataset_dir, 'SenseReID', 'test_gallery'
+ )
+
+ required_files = [self.dataset_dir, self.query_dir, self.gallery_dir]
+ self.check_before_run(required_files)
+
+ query = self.process_dir(self.query_dir)
+ gallery = self.process_dir(self.gallery_dir)
+
+ # relabel
+ g_pids = set()
+ for _, pid, _ in gallery:
+ g_pids.add(pid)
+ pid2label = {pid: i for i, pid in enumerate(g_pids)}
+
+ query = [
+ (img_path, pid2label[pid], camid) for img_path, pid, camid in query
+ ]
+ gallery = [
+ (img_path, pid2label[pid], camid)
+ for img_path, pid, camid in gallery
+ ]
+ train = copy.deepcopy(query) + copy.deepcopy(gallery) # dummy variable
+
+ super(SenseReID, self).__init__(train, query, gallery, **kwargs)
+
+ def process_dir(self, dir_path):
+ img_paths = glob.glob(osp.join(dir_path, '*.jpg'))
+ data = []
+
+ for img_path in img_paths:
+ img_name = osp.splitext(osp.basename(img_path))[0]
+ pid, camid = img_name.split('_')
+ pid, camid = int(pid), int(camid)
+ data.append((img_path, pid, camid))
+
+ return data
diff --git a/trackers/strong_sort/deep/reid/torchreid/data/datasets/image/university1652.py b/trackers/strong_sort/deep/reid/torchreid/data/datasets/image/university1652.py
new file mode 100644
index 0000000000000000000000000000000000000000..ce1e386b04b904dca17fb5c0b1373e648cc995ec
--- /dev/null
+++ b/trackers/strong_sort/deep/reid/torchreid/data/datasets/image/university1652.py
@@ -0,0 +1,110 @@
+from __future__ import division, print_function, absolute_import
+import os
+import glob
+import os.path as osp
+import gdown
+
+from ..dataset import ImageDataset
+
+
+class University1652(ImageDataset):
+ """University-1652.
+
+ Reference:
+ - Zheng et al. University-1652: A Multi-view Multi-source Benchmark for Drone-based Geo-localization. ACM MM 2020.
+
+ URL: ``_
+ OneDrive:
+ https://studentutsedu-my.sharepoint.com/:u:/g/personal/12639605_student_uts_edu_au/Ecrz6xK-PcdCjFdpNb0T0s8B_9J5ynaUy3q63_XumjJyrA?e=z4hpcz
+ [Backup] GoogleDrive:
+ https://drive.google.com/file/d/1iVnP4gjw-iHXa0KerZQ1IfIO0i1jADsR/view?usp=sharing
+ [Backup] Baidu Yun:
+ https://pan.baidu.com/s/1H_wBnWwikKbaBY1pMPjoqQ password: hrqp
+
+ Dataset statistics:
+ - buildings: 1652 (train + query).
+ - The dataset split is as follows:
+ | Split | #imgs | #buildings | #universities|
+ | -------- | ----- | ----| ----|
+ | Training | 50,218 | 701 | 33 |
+ | Query_drone | 37,855 | 701 | 39 |
+ | Query_satellite | 701 | 701 | 39|
+ | Query_ground | 2,579 | 701 | 39|
+ | Gallery_drone | 51,355 | 951 | 39|
+ | Gallery_satellite | 951 | 951 | 39|
+ | Gallery_ground | 2,921 | 793 | 39|
+ - cameras: None.
+
+ datamanager = torchreid.data.ImageDataManager(
+ root='reid-data',
+ sources='university1652',
+ targets='university1652',
+ height=256,
+ width=256,
+ batch_size_train=32,
+ batch_size_test=100,
+ transforms=['random_flip', 'random_crop']
+ )
+ """
+ dataset_dir = 'university1652'
+ dataset_url = 'https://drive.google.com/uc?id=1iVnP4gjw-iHXa0KerZQ1IfIO0i1jADsR'
+
+ def __init__(self, root='', **kwargs):
+ self.root = osp.abspath(osp.expanduser(root))
+ self.dataset_dir = osp.join(self.root, self.dataset_dir)
+ print(self.dataset_dir)
+ if not os.path.isdir(self.dataset_dir):
+ os.mkdir(self.dataset_dir)
+ gdown.download(
+ self.dataset_url, self.dataset_dir + 'data.zip', quiet=False
+ )
+ os.system('unzip %s' % (self.dataset_dir + 'data.zip'))
+ self.train_dir = osp.join(
+ self.dataset_dir, 'University-Release/train/'
+ )
+ self.query_dir = osp.join(
+ self.dataset_dir, 'University-Release/test/query_drone'
+ )
+ self.gallery_dir = osp.join(
+ self.dataset_dir, 'University-Release/test/gallery_satellite'
+ )
+
+ required_files = [
+ self.dataset_dir, self.train_dir, self.query_dir, self.gallery_dir
+ ]
+ self.check_before_run(required_files)
+
+ self.fake_camid = 0
+ train = self.process_dir(self.train_dir, relabel=True, train=True)
+ query = self.process_dir(self.query_dir, relabel=False)
+ gallery = self.process_dir(self.gallery_dir, relabel=False)
+
+ super(University1652, self).__init__(train, query, gallery, **kwargs)
+
+ def process_dir(self, dir_path, relabel=False, train=False):
+ IMG_EXTENSIONS = (
+ '.jpg', '.jpeg', '.png', '.ppm', '.bmp', '.pgm', '.tif', '.tiff',
+ '.webp'
+ )
+ if train:
+ img_paths = glob.glob(osp.join(dir_path, '*/*/*'))
+ else:
+ img_paths = glob.glob(osp.join(dir_path, '*/*'))
+ pid_container = set()
+ for img_path in img_paths:
+ if not img_path.lower().endswith(IMG_EXTENSIONS):
+ continue
+ pid = int(os.path.basename(os.path.dirname(img_path)))
+ pid_container.add(pid)
+ pid2label = {pid: label for label, pid in enumerate(pid_container)}
+ data = []
+ # no camera for university
+ for img_path in img_paths:
+ if not img_path.lower().endswith(IMG_EXTENSIONS):
+ continue
+ pid = int(os.path.basename(os.path.dirname(img_path)))
+ if relabel:
+ pid = pid2label[pid]
+ data.append((img_path, pid, self.fake_camid))
+ self.fake_camid += 1
+ return data
diff --git a/trackers/strong_sort/deep/reid/torchreid/data/datasets/image/viper.py b/trackers/strong_sort/deep/reid/torchreid/data/datasets/image/viper.py
new file mode 100644
index 0000000000000000000000000000000000000000..161dd99e654b43b93dcd4b46646b2d0f85a2ab1f
--- /dev/null
+++ b/trackers/strong_sort/deep/reid/torchreid/data/datasets/image/viper.py
@@ -0,0 +1,128 @@
+from __future__ import division, print_function, absolute_import
+import glob
+import numpy as np
+import os.path as osp
+
+from torchreid.utils import read_json, write_json
+
+from ..dataset import ImageDataset
+
+
+class VIPeR(ImageDataset):
+ """VIPeR.
+
+ Reference:
+ Gray et al. Evaluating appearance models for recognition, reacquisition, and tracking. PETS 2007.
+
+ URL: ``_
+
+ Dataset statistics:
+ - identities: 632.
+ - images: 632 x 2 = 1264.
+ - cameras: 2.
+ """
+ dataset_dir = 'viper'
+ dataset_url = 'http://users.soe.ucsc.edu/~manduchi/VIPeR.v1.0.zip'
+
+ def __init__(self, root='', split_id=0, **kwargs):
+ self.root = osp.abspath(osp.expanduser(root))
+ self.dataset_dir = osp.join(self.root, self.dataset_dir)
+ self.download_dataset(self.dataset_dir, self.dataset_url)
+
+ self.cam_a_dir = osp.join(self.dataset_dir, 'VIPeR', 'cam_a')
+ self.cam_b_dir = osp.join(self.dataset_dir, 'VIPeR', 'cam_b')
+ self.split_path = osp.join(self.dataset_dir, 'splits.json')
+
+ required_files = [self.dataset_dir, self.cam_a_dir, self.cam_b_dir]
+ self.check_before_run(required_files)
+
+ self.prepare_split()
+ splits = read_json(self.split_path)
+ if split_id >= len(splits):
+ raise ValueError(
+ 'split_id exceeds range, received {}, '
+ 'but expected between 0 and {}'.format(
+ split_id,
+ len(splits) - 1
+ )
+ )
+ split = splits[split_id]
+
+ train = split['train']
+ query = split['query'] # query and gallery share the same images
+ gallery = split['gallery']
+
+ train = [tuple(item) for item in train]
+ query = [tuple(item) for item in query]
+ gallery = [tuple(item) for item in gallery]
+
+ super(VIPeR, self).__init__(train, query, gallery, **kwargs)
+
+ def prepare_split(self):
+ if not osp.exists(self.split_path):
+ print('Creating 10 random splits of train ids and test ids')
+
+ cam_a_imgs = sorted(glob.glob(osp.join(self.cam_a_dir, '*.bmp')))
+ cam_b_imgs = sorted(glob.glob(osp.join(self.cam_b_dir, '*.bmp')))
+ assert len(cam_a_imgs) == len(cam_b_imgs)
+ num_pids = len(cam_a_imgs)
+ print('Number of identities: {}'.format(num_pids))
+ num_train_pids = num_pids // 2
+ """
+ In total, there will be 20 splits because each random split creates two
+ sub-splits, one using cameraA as query and cameraB as gallery
+ while the other using cameraB as query and cameraA as gallery.
+ Therefore, results should be averaged over 20 splits (split_id=0~19).
+
+ In practice, a model trained on split_id=0 can be applied to split_id=0&1
+ as split_id=0&1 share the same training data (so on and so forth).
+ """
+ splits = []
+ for _ in range(10):
+ order = np.arange(num_pids)
+ np.random.shuffle(order)
+ train_idxs = order[:num_train_pids]
+ test_idxs = order[num_train_pids:]
+ assert not bool(set(train_idxs) & set(test_idxs)), \
+ 'Error: train and test overlap'
+
+ train = []
+ for pid, idx in enumerate(train_idxs):
+ cam_a_img = cam_a_imgs[idx]
+ cam_b_img = cam_b_imgs[idx]
+ train.append((cam_a_img, pid, 0))
+ train.append((cam_b_img, pid, 1))
+
+ test_a = []
+ test_b = []
+ for pid, idx in enumerate(test_idxs):
+ cam_a_img = cam_a_imgs[idx]
+ cam_b_img = cam_b_imgs[idx]
+ test_a.append((cam_a_img, pid, 0))
+ test_b.append((cam_b_img, pid, 1))
+
+ # use cameraA as query and cameraB as gallery
+ split = {
+ 'train': train,
+ 'query': test_a,
+ 'gallery': test_b,
+ 'num_train_pids': num_train_pids,
+ 'num_query_pids': num_pids - num_train_pids,
+ 'num_gallery_pids': num_pids - num_train_pids
+ }
+ splits.append(split)
+
+ # use cameraB as query and cameraA as gallery
+ split = {
+ 'train': train,
+ 'query': test_b,
+ 'gallery': test_a,
+ 'num_train_pids': num_train_pids,
+ 'num_query_pids': num_pids - num_train_pids,
+ 'num_gallery_pids': num_pids - num_train_pids
+ }
+ splits.append(split)
+
+ print('Totally {} splits are created'.format(len(splits)))
+ write_json(splits, self.split_path)
+ print('Split file saved to {}'.format(self.split_path))
diff --git a/trackers/strong_sort/deep/reid/torchreid/data/datasets/video/__init__.py b/trackers/strong_sort/deep/reid/torchreid/data/datasets/video/__init__.py
new file mode 100644
index 0000000000000000000000000000000000000000..f4e75d316d4b1763c82848490b6766bf7c662654
--- /dev/null
+++ b/trackers/strong_sort/deep/reid/torchreid/data/datasets/video/__init__.py
@@ -0,0 +1,6 @@
+from __future__ import print_function, absolute_import
+
+from .mars import Mars
+from .ilidsvid import iLIDSVID
+from .prid2011 import PRID2011
+from .dukemtmcvidreid import DukeMTMCVidReID
diff --git a/trackers/strong_sort/deep/reid/torchreid/data/datasets/video/dukemtmcvidreid.py b/trackers/strong_sort/deep/reid/torchreid/data/datasets/video/dukemtmcvidreid.py
new file mode 100644
index 0000000000000000000000000000000000000000..4b4c82f9e92008bdfeb6c56b79bd24b916f83922
--- /dev/null
+++ b/trackers/strong_sort/deep/reid/torchreid/data/datasets/video/dukemtmcvidreid.py
@@ -0,0 +1,128 @@
+from __future__ import division, print_function, absolute_import
+import glob
+import os.path as osp
+import warnings
+
+from torchreid.utils import read_json, write_json
+
+from ..dataset import VideoDataset
+
+
+class DukeMTMCVidReID(VideoDataset):
+ """DukeMTMCVidReID.
+
+ Reference:
+ - Ristani et al. Performance Measures and a Data Set for Multi-Target,
+ Multi-Camera Tracking. ECCVW 2016.
+ - Wu et al. Exploit the Unknown Gradually: One-Shot Video-Based Person
+ Re-Identification by Stepwise Learning. CVPR 2018.
+
+ URL: ``_
+
+ Dataset statistics:
+ - identities: 702 (train) + 702 (test).
+ - tracklets: 2196 (train) + 2636 (test).
+ """
+ dataset_dir = 'dukemtmc-vidreid'
+ dataset_url = 'http://vision.cs.duke.edu/DukeMTMC/data/misc/DukeMTMC-VideoReID.zip'
+
+ def __init__(self, root='', min_seq_len=0, **kwargs):
+ self.root = osp.abspath(osp.expanduser(root))
+ self.dataset_dir = osp.join(self.root, self.dataset_dir)
+ self.download_dataset(self.dataset_dir, self.dataset_url)
+
+ self.train_dir = osp.join(self.dataset_dir, 'DukeMTMC-VideoReID/train')
+ self.query_dir = osp.join(self.dataset_dir, 'DukeMTMC-VideoReID/query')
+ self.gallery_dir = osp.join(
+ self.dataset_dir, 'DukeMTMC-VideoReID/gallery'
+ )
+ self.split_train_json_path = osp.join(
+ self.dataset_dir, 'split_train.json'
+ )
+ self.split_query_json_path = osp.join(
+ self.dataset_dir, 'split_query.json'
+ )
+ self.split_gallery_json_path = osp.join(
+ self.dataset_dir, 'split_gallery.json'
+ )
+ self.min_seq_len = min_seq_len
+
+ required_files = [
+ self.dataset_dir, self.train_dir, self.query_dir, self.gallery_dir
+ ]
+ self.check_before_run(required_files)
+
+ train = self.process_dir(
+ self.train_dir, self.split_train_json_path, relabel=True
+ )
+ query = self.process_dir(
+ self.query_dir, self.split_query_json_path, relabel=False
+ )
+ gallery = self.process_dir(
+ self.gallery_dir, self.split_gallery_json_path, relabel=False
+ )
+
+ super(DukeMTMCVidReID, self).__init__(train, query, gallery, **kwargs)
+
+ def process_dir(self, dir_path, json_path, relabel):
+ if osp.exists(json_path):
+ split = read_json(json_path)
+ return split['tracklets']
+
+ print('=> Generating split json file (** this might take a while **)')
+ pdirs = glob.glob(osp.join(dir_path, '*')) # avoid .DS_Store
+ print(
+ 'Processing "{}" with {} person identities'.format(
+ dir_path, len(pdirs)
+ )
+ )
+
+ pid_container = set()
+ for pdir in pdirs:
+ pid = int(osp.basename(pdir))
+ pid_container.add(pid)
+ pid2label = {pid: label for label, pid in enumerate(pid_container)}
+
+ tracklets = []
+ for pdir in pdirs:
+ pid = int(osp.basename(pdir))
+ if relabel:
+ pid = pid2label[pid]
+ tdirs = glob.glob(osp.join(pdir, '*'))
+ for tdir in tdirs:
+ raw_img_paths = glob.glob(osp.join(tdir, '*.jpg'))
+ num_imgs = len(raw_img_paths)
+
+ if num_imgs < self.min_seq_len:
+ continue
+
+ img_paths = []
+ for img_idx in range(num_imgs):
+ # some tracklet starts from 0002 instead of 0001
+ img_idx_name = 'F' + str(img_idx + 1).zfill(4)
+ res = glob.glob(
+ osp.join(tdir, '*' + img_idx_name + '*.jpg')
+ )
+ if len(res) == 0:
+ warnings.warn(
+ 'Index name {} in {} is missing, skip'.format(
+ img_idx_name, tdir
+ )
+ )
+ continue
+ img_paths.append(res[0])
+ img_name = osp.basename(img_paths[0])
+ if img_name.find('_') == -1:
+ # old naming format: 0001C6F0099X30823.jpg
+ camid = int(img_name[5]) - 1
+ else:
+ # new naming format: 0001_C6_F0099_X30823.jpg
+ camid = int(img_name[6]) - 1
+ img_paths = tuple(img_paths)
+ tracklets.append((img_paths, pid, camid))
+
+ print('Saving split to {}'.format(json_path))
+ split_dict = {'tracklets': tracklets}
+ write_json(split_dict, json_path)
+
+ return tracklets
diff --git a/trackers/strong_sort/deep/reid/torchreid/data/datasets/video/ilidsvid.py b/trackers/strong_sort/deep/reid/torchreid/data/datasets/video/ilidsvid.py
new file mode 100644
index 0000000000000000000000000000000000000000..c3ac1bbe6f182301f726fb8027efab6f142808c9
--- /dev/null
+++ b/trackers/strong_sort/deep/reid/torchreid/data/datasets/video/ilidsvid.py
@@ -0,0 +1,143 @@
+from __future__ import division, print_function, absolute_import
+import glob
+import os.path as osp
+from scipy.io import loadmat
+
+from torchreid.utils import read_json, write_json
+
+from ..dataset import VideoDataset
+
+
+class iLIDSVID(VideoDataset):
+ """iLIDS-VID.
+
+ Reference:
+ Wang et al. Person Re-Identification by Video Ranking. ECCV 2014.
+
+ URL: ``_
+
+ Dataset statistics:
+ - identities: 300.
+ - tracklets: 600.
+ - cameras: 2.
+ """
+ dataset_dir = 'ilids-vid'
+ dataset_url = 'http://www.eecs.qmul.ac.uk/~xiatian/iLIDS-VID/iLIDS-VID.tar'
+
+ def __init__(self, root='', split_id=0, **kwargs):
+ self.root = osp.abspath(osp.expanduser(root))
+ self.dataset_dir = osp.join(self.root, self.dataset_dir)
+ self.download_dataset(self.dataset_dir, self.dataset_url)
+
+ self.data_dir = osp.join(self.dataset_dir, 'i-LIDS-VID')
+ self.split_dir = osp.join(self.dataset_dir, 'train-test people splits')
+ self.split_mat_path = osp.join(
+ self.split_dir, 'train_test_splits_ilidsvid.mat'
+ )
+ self.split_path = osp.join(self.dataset_dir, 'splits.json')
+ self.cam_1_path = osp.join(
+ self.dataset_dir, 'i-LIDS-VID/sequences/cam1'
+ )
+ self.cam_2_path = osp.join(
+ self.dataset_dir, 'i-LIDS-VID/sequences/cam2'
+ )
+
+ required_files = [self.dataset_dir, self.data_dir, self.split_dir]
+ self.check_before_run(required_files)
+
+ self.prepare_split()
+ splits = read_json(self.split_path)
+ if split_id >= len(splits):
+ raise ValueError(
+ 'split_id exceeds range, received {}, but expected between 0 and {}'
+ .format(split_id,
+ len(splits) - 1)
+ )
+ split = splits[split_id]
+ train_dirs, test_dirs = split['train'], split['test']
+
+ train = self.process_data(train_dirs, cam1=True, cam2=True)
+ query = self.process_data(test_dirs, cam1=True, cam2=False)
+ gallery = self.process_data(test_dirs, cam1=False, cam2=True)
+
+ super(iLIDSVID, self).__init__(train, query, gallery, **kwargs)
+
+ def prepare_split(self):
+ if not osp.exists(self.split_path):
+ print('Creating splits ...')
+ mat_split_data = loadmat(self.split_mat_path)['ls_set']
+
+ num_splits = mat_split_data.shape[0]
+ num_total_ids = mat_split_data.shape[1]
+ assert num_splits == 10
+ assert num_total_ids == 300
+ num_ids_each = num_total_ids // 2
+
+ # pids in mat_split_data are indices, so we need to transform them
+ # to real pids
+ person_cam1_dirs = sorted(
+ glob.glob(osp.join(self.cam_1_path, '*'))
+ )
+ person_cam2_dirs = sorted(
+ glob.glob(osp.join(self.cam_2_path, '*'))
+ )
+
+ person_cam1_dirs = [
+ osp.basename(item) for item in person_cam1_dirs
+ ]
+ person_cam2_dirs = [
+ osp.basename(item) for item in person_cam2_dirs
+ ]
+
+ # make sure persons in one camera view can be found in the other camera view
+ assert set(person_cam1_dirs) == set(person_cam2_dirs)
+
+ splits = []
+ for i_split in range(num_splits):
+ # first 50% for testing and the remaining for training, following Wang et al. ECCV'14.
+ train_idxs = sorted(
+ list(mat_split_data[i_split, num_ids_each:])
+ )
+ test_idxs = sorted(
+ list(mat_split_data[i_split, :num_ids_each])
+ )
+
+ train_idxs = [int(i) - 1 for i in train_idxs]
+ test_idxs = [int(i) - 1 for i in test_idxs]
+
+ # transform pids to person dir names
+ train_dirs = [person_cam1_dirs[i] for i in train_idxs]
+ test_dirs = [person_cam1_dirs[i] for i in test_idxs]
+
+ split = {'train': train_dirs, 'test': test_dirs}
+ splits.append(split)
+
+ print(
+ 'Totally {} splits are created, following Wang et al. ECCV\'14'
+ .format(len(splits))
+ )
+ print('Split file is saved to {}'.format(self.split_path))
+ write_json(splits, self.split_path)
+
+ def process_data(self, dirnames, cam1=True, cam2=True):
+ tracklets = []
+ dirname2pid = {dirname: i for i, dirname in enumerate(dirnames)}
+
+ for dirname in dirnames:
+ if cam1:
+ person_dir = osp.join(self.cam_1_path, dirname)
+ img_names = glob.glob(osp.join(person_dir, '*.png'))
+ assert len(img_names) > 0
+ img_names = tuple(img_names)
+ pid = dirname2pid[dirname]
+ tracklets.append((img_names, pid, 0))
+
+ if cam2:
+ person_dir = osp.join(self.cam_2_path, dirname)
+ img_names = glob.glob(osp.join(person_dir, '*.png'))
+ assert len(img_names) > 0
+ img_names = tuple(img_names)
+ pid = dirname2pid[dirname]
+ tracklets.append((img_names, pid, 1))
+
+ return tracklets
diff --git a/trackers/strong_sort/deep/reid/torchreid/data/datasets/video/mars.py b/trackers/strong_sort/deep/reid/torchreid/data/datasets/video/mars.py
new file mode 100644
index 0000000000000000000000000000000000000000..4128e1cbf53ca39fad8e468eed90c3d80c9310a5
--- /dev/null
+++ b/trackers/strong_sort/deep/reid/torchreid/data/datasets/video/mars.py
@@ -0,0 +1,133 @@
+from __future__ import division, print_function, absolute_import
+import os.path as osp
+import warnings
+from scipy.io import loadmat
+
+from ..dataset import VideoDataset
+
+
+class Mars(VideoDataset):
+ """MARS.
+
+ Reference:
+ Zheng et al. MARS: A Video Benchmark for Large-Scale Person Re-identification. ECCV 2016.
+
+ URL: ``_
+
+ Dataset statistics:
+ - identities: 1261.
+ - tracklets: 8298 (train) + 1980 (query) + 9330 (gallery).
+ - cameras: 6.
+ """
+ dataset_dir = 'mars'
+ dataset_url = None
+
+ def __init__(self, root='', **kwargs):
+ self.root = osp.abspath(osp.expanduser(root))
+ self.dataset_dir = osp.join(self.root, self.dataset_dir)
+ self.download_dataset(self.dataset_dir, self.dataset_url)
+
+ self.train_name_path = osp.join(
+ self.dataset_dir, 'info/train_name.txt'
+ )
+ self.test_name_path = osp.join(self.dataset_dir, 'info/test_name.txt')
+ self.track_train_info_path = osp.join(
+ self.dataset_dir, 'info/tracks_train_info.mat'
+ )
+ self.track_test_info_path = osp.join(
+ self.dataset_dir, 'info/tracks_test_info.mat'
+ )
+ self.query_IDX_path = osp.join(self.dataset_dir, 'info/query_IDX.mat')
+
+ required_files = [
+ self.dataset_dir, self.train_name_path, self.test_name_path,
+ self.track_train_info_path, self.track_test_info_path,
+ self.query_IDX_path
+ ]
+ self.check_before_run(required_files)
+
+ train_names = self.get_names(self.train_name_path)
+ test_names = self.get_names(self.test_name_path)
+ track_train = loadmat(self.track_train_info_path
+ )['track_train_info'] # numpy.ndarray (8298, 4)
+ track_test = loadmat(self.track_test_info_path
+ )['track_test_info'] # numpy.ndarray (12180, 4)
+ query_IDX = loadmat(self.query_IDX_path
+ )['query_IDX'].squeeze() # numpy.ndarray (1980,)
+ query_IDX -= 1 # index from 0
+ track_query = track_test[query_IDX, :]
+ gallery_IDX = [
+ i for i in range(track_test.shape[0]) if i not in query_IDX
+ ]
+ track_gallery = track_test[gallery_IDX, :]
+
+ train = self.process_data(
+ train_names, track_train, home_dir='bbox_train', relabel=True
+ )
+ query = self.process_data(
+ test_names, track_query, home_dir='bbox_test', relabel=False
+ )
+ gallery = self.process_data(
+ test_names, track_gallery, home_dir='bbox_test', relabel=False
+ )
+
+ super(Mars, self).__init__(train, query, gallery, **kwargs)
+
+ def get_names(self, fpath):
+ names = []
+ with open(fpath, 'r') as f:
+ for line in f:
+ new_line = line.rstrip()
+ names.append(new_line)
+ return names
+
+ def process_data(
+ self, names, meta_data, home_dir=None, relabel=False, min_seq_len=0
+ ):
+ assert home_dir in ['bbox_train', 'bbox_test']
+ num_tracklets = meta_data.shape[0]
+ pid_list = list(set(meta_data[:, 2].tolist()))
+
+ if relabel:
+ pid2label = {pid: label for label, pid in enumerate(pid_list)}
+ tracklets = []
+
+ for tracklet_idx in range(num_tracklets):
+ data = meta_data[tracklet_idx, ...]
+ start_index, end_index, pid, camid = data
+ if pid == -1:
+ continue # junk images are just ignored
+ assert 1 <= camid <= 6
+ if relabel:
+ pid = pid2label[pid]
+ camid -= 1 # index starts from 0
+ img_names = names[start_index - 1:end_index]
+
+ # make sure image names correspond to the same person
+ pnames = [img_name[:4] for img_name in img_names]
+ assert len(
+ set(pnames)
+ ) == 1, 'Error: a single tracklet contains different person images'
+
+ # make sure all images are captured under the same camera
+ camnames = [img_name[5] for img_name in img_names]
+ assert len(
+ set(camnames)
+ ) == 1, 'Error: images are captured under different cameras!'
+
+ # append image names with directory information
+ img_paths = [
+ osp.join(self.dataset_dir, home_dir, img_name[:4], img_name)
+ for img_name in img_names
+ ]
+ if len(img_paths) >= min_seq_len:
+ img_paths = tuple(img_paths)
+ tracklets.append((img_paths, pid, camid))
+
+ return tracklets
+
+ def combine_all(self):
+ warnings.warn(
+ 'Some query IDs do not appear in gallery. Therefore, combineall '
+ 'does not make any difference to Mars'
+ )
diff --git a/trackers/strong_sort/deep/reid/torchreid/data/datasets/video/prid2011.py b/trackers/strong_sort/deep/reid/torchreid/data/datasets/video/prid2011.py
new file mode 100644
index 0000000000000000000000000000000000000000..3af2e4d1ffd6ccc71f2b5705090c5931602f1e3e
--- /dev/null
+++ b/trackers/strong_sort/deep/reid/torchreid/data/datasets/video/prid2011.py
@@ -0,0 +1,80 @@
+from __future__ import division, print_function, absolute_import
+import glob
+import os.path as osp
+
+from torchreid.utils import read_json
+
+from ..dataset import VideoDataset
+
+
+class PRID2011(VideoDataset):
+ """PRID2011.
+
+ Reference:
+ Hirzer et al. Person Re-Identification by Descriptive and
+ Discriminative Classification. SCIA 2011.
+
+ URL: ``_
+
+ Dataset statistics:
+ - identities: 200.
+ - tracklets: 400.
+ - cameras: 2.
+ """
+ dataset_dir = 'prid2011'
+ dataset_url = None
+
+ def __init__(self, root='', split_id=0, **kwargs):
+ self.root = osp.abspath(osp.expanduser(root))
+ self.dataset_dir = osp.join(self.root, self.dataset_dir)
+ self.download_dataset(self.dataset_dir, self.dataset_url)
+
+ self.split_path = osp.join(self.dataset_dir, 'splits_prid2011.json')
+ self.cam_a_dir = osp.join(
+ self.dataset_dir, 'prid_2011', 'multi_shot', 'cam_a'
+ )
+ self.cam_b_dir = osp.join(
+ self.dataset_dir, 'prid_2011', 'multi_shot', 'cam_b'
+ )
+
+ required_files = [self.dataset_dir, self.cam_a_dir, self.cam_b_dir]
+ self.check_before_run(required_files)
+
+ splits = read_json(self.split_path)
+ if split_id >= len(splits):
+ raise ValueError(
+ 'split_id exceeds range, received {}, but expected between 0 and {}'
+ .format(split_id,
+ len(splits) - 1)
+ )
+ split = splits[split_id]
+ train_dirs, test_dirs = split['train'], split['test']
+
+ train = self.process_dir(train_dirs, cam1=True, cam2=True)
+ query = self.process_dir(test_dirs, cam1=True, cam2=False)
+ gallery = self.process_dir(test_dirs, cam1=False, cam2=True)
+
+ super(PRID2011, self).__init__(train, query, gallery, **kwargs)
+
+ def process_dir(self, dirnames, cam1=True, cam2=True):
+ tracklets = []
+ dirname2pid = {dirname: i for i, dirname in enumerate(dirnames)}
+
+ for dirname in dirnames:
+ if cam1:
+ person_dir = osp.join(self.cam_a_dir, dirname)
+ img_names = glob.glob(osp.join(person_dir, '*.png'))
+ assert len(img_names) > 0
+ img_names = tuple(img_names)
+ pid = dirname2pid[dirname]
+ tracklets.append((img_names, pid, 0))
+
+ if cam2:
+ person_dir = osp.join(self.cam_b_dir, dirname)
+ img_names = glob.glob(osp.join(person_dir, '*.png'))
+ assert len(img_names) > 0
+ img_names = tuple(img_names)
+ pid = dirname2pid[dirname]
+ tracklets.append((img_names, pid, 1))
+
+ return tracklets
diff --git a/trackers/strong_sort/deep/reid/torchreid/data/sampler.py b/trackers/strong_sort/deep/reid/torchreid/data/sampler.py
new file mode 100644
index 0000000000000000000000000000000000000000..f69b3e02a7f111bc88595dae7a6fe64b25e0703d
--- /dev/null
+++ b/trackers/strong_sort/deep/reid/torchreid/data/sampler.py
@@ -0,0 +1,245 @@
+from __future__ import division, absolute_import
+import copy
+import numpy as np
+import random
+from collections import defaultdict
+from torch.utils.data.sampler import Sampler, RandomSampler, SequentialSampler
+
+AVAI_SAMPLERS = [
+ 'RandomIdentitySampler', 'SequentialSampler', 'RandomSampler',
+ 'RandomDomainSampler', 'RandomDatasetSampler'
+]
+
+
+class RandomIdentitySampler(Sampler):
+ """Randomly samples N identities each with K instances.
+
+ Args:
+ data_source (list): contains tuples of (img_path(s), pid, camid, dsetid).
+ batch_size (int): batch size.
+ num_instances (int): number of instances per identity in a batch.
+ """
+
+ def __init__(self, data_source, batch_size, num_instances):
+ if batch_size < num_instances:
+ raise ValueError(
+ 'batch_size={} must be no less '
+ 'than num_instances={}'.format(batch_size, num_instances)
+ )
+
+ self.data_source = data_source
+ self.batch_size = batch_size
+ self.num_instances = num_instances
+ self.num_pids_per_batch = self.batch_size // self.num_instances
+ self.index_dic = defaultdict(list)
+ for index, items in enumerate(data_source):
+ pid = items[1]
+ self.index_dic[pid].append(index)
+ self.pids = list(self.index_dic.keys())
+ assert len(self.pids) >= self.num_pids_per_batch
+
+ # estimate number of examples in an epoch
+ # TODO: improve precision
+ self.length = 0
+ for pid in self.pids:
+ idxs = self.index_dic[pid]
+ num = len(idxs)
+ if num < self.num_instances:
+ num = self.num_instances
+ self.length += num - num % self.num_instances
+
+ def __iter__(self):
+ batch_idxs_dict = defaultdict(list)
+
+ for pid in self.pids:
+ idxs = copy.deepcopy(self.index_dic[pid])
+ if len(idxs) < self.num_instances:
+ idxs = np.random.choice(
+ idxs, size=self.num_instances, replace=True
+ )
+ random.shuffle(idxs)
+ batch_idxs = []
+ for idx in idxs:
+ batch_idxs.append(idx)
+ if len(batch_idxs) == self.num_instances:
+ batch_idxs_dict[pid].append(batch_idxs)
+ batch_idxs = []
+
+ avai_pids = copy.deepcopy(self.pids)
+ final_idxs = []
+
+ while len(avai_pids) >= self.num_pids_per_batch:
+ selected_pids = random.sample(avai_pids, self.num_pids_per_batch)
+ for pid in selected_pids:
+ batch_idxs = batch_idxs_dict[pid].pop(0)
+ final_idxs.extend(batch_idxs)
+ if len(batch_idxs_dict[pid]) == 0:
+ avai_pids.remove(pid)
+
+ return iter(final_idxs)
+
+ def __len__(self):
+ return self.length
+
+
+class RandomDomainSampler(Sampler):
+ """Random domain sampler.
+
+ We consider each camera as a visual domain.
+
+ How does the sampling work:
+ 1. Randomly sample N cameras (based on the "camid" label).
+ 2. From each camera, randomly sample K images.
+
+ Args:
+ data_source (list): contains tuples of (img_path(s), pid, camid, dsetid).
+ batch_size (int): batch size.
+ n_domain (int): number of cameras to sample in a batch.
+ """
+
+ def __init__(self, data_source, batch_size, n_domain):
+ self.data_source = data_source
+
+ # Keep track of image indices for each domain
+ self.domain_dict = defaultdict(list)
+ for i, items in enumerate(data_source):
+ camid = items[2]
+ self.domain_dict[camid].append(i)
+ self.domains = list(self.domain_dict.keys())
+
+ # Make sure each domain can be assigned an equal number of images
+ if n_domain is None or n_domain <= 0:
+ n_domain = len(self.domains)
+ assert batch_size % n_domain == 0
+ self.n_img_per_domain = batch_size // n_domain
+
+ self.batch_size = batch_size
+ self.n_domain = n_domain
+ self.length = len(list(self.__iter__()))
+
+ def __iter__(self):
+ domain_dict = copy.deepcopy(self.domain_dict)
+ final_idxs = []
+ stop_sampling = False
+
+ while not stop_sampling:
+ selected_domains = random.sample(self.domains, self.n_domain)
+
+ for domain in selected_domains:
+ idxs = domain_dict[domain]
+ selected_idxs = random.sample(idxs, self.n_img_per_domain)
+ final_idxs.extend(selected_idxs)
+
+ for idx in selected_idxs:
+ domain_dict[domain].remove(idx)
+
+ remaining = len(domain_dict[domain])
+ if remaining < self.n_img_per_domain:
+ stop_sampling = True
+
+ return iter(final_idxs)
+
+ def __len__(self):
+ return self.length
+
+
+class RandomDatasetSampler(Sampler):
+ """Random dataset sampler.
+
+ How does the sampling work:
+ 1. Randomly sample N datasets (based on the "dsetid" label).
+ 2. From each dataset, randomly sample K images.
+
+ Args:
+ data_source (list): contains tuples of (img_path(s), pid, camid, dsetid).
+ batch_size (int): batch size.
+ n_dataset (int): number of datasets to sample in a batch.
+ """
+
+ def __init__(self, data_source, batch_size, n_dataset):
+ self.data_source = data_source
+
+ # Keep track of image indices for each dataset
+ self.dataset_dict = defaultdict(list)
+ for i, items in enumerate(data_source):
+ dsetid = items[3]
+ self.dataset_dict[dsetid].append(i)
+ self.datasets = list(self.dataset_dict.keys())
+
+ # Make sure each dataset can be assigned an equal number of images
+ if n_dataset is None or n_dataset <= 0:
+ n_dataset = len(self.datasets)
+ assert batch_size % n_dataset == 0
+ self.n_img_per_dset = batch_size // n_dataset
+
+ self.batch_size = batch_size
+ self.n_dataset = n_dataset
+ self.length = len(list(self.__iter__()))
+
+ def __iter__(self):
+ dataset_dict = copy.deepcopy(self.dataset_dict)
+ final_idxs = []
+ stop_sampling = False
+
+ while not stop_sampling:
+ selected_datasets = random.sample(self.datasets, self.n_dataset)
+
+ for dset in selected_datasets:
+ idxs = dataset_dict[dset]
+ selected_idxs = random.sample(idxs, self.n_img_per_dset)
+ final_idxs.extend(selected_idxs)
+
+ for idx in selected_idxs:
+ dataset_dict[dset].remove(idx)
+
+ remaining = len(dataset_dict[dset])
+ if remaining < self.n_img_per_dset:
+ stop_sampling = True
+
+ return iter(final_idxs)
+
+ def __len__(self):
+ return self.length
+
+
+def build_train_sampler(
+ data_source,
+ train_sampler,
+ batch_size=32,
+ num_instances=4,
+ num_cams=1,
+ num_datasets=1,
+ **kwargs
+):
+ """Builds a training sampler.
+
+ Args:
+ data_source (list): contains tuples of (img_path(s), pid, camid).
+ train_sampler (str): sampler name (default: ``RandomSampler``).
+ batch_size (int, optional): batch size. Default is 32.
+ num_instances (int, optional): number of instances per identity in a
+ batch (when using ``RandomIdentitySampler``). Default is 4.
+ num_cams (int, optional): number of cameras to sample in a batch (when using
+ ``RandomDomainSampler``). Default is 1.
+ num_datasets (int, optional): number of datasets to sample in a batch (when
+ using ``RandomDatasetSampler``). Default is 1.
+ """
+ assert train_sampler in AVAI_SAMPLERS, \
+ 'train_sampler must be one of {}, but got {}'.format(AVAI_SAMPLERS, train_sampler)
+
+ if train_sampler == 'RandomIdentitySampler':
+ sampler = RandomIdentitySampler(data_source, batch_size, num_instances)
+
+ elif train_sampler == 'RandomDomainSampler':
+ sampler = RandomDomainSampler(data_source, batch_size, num_cams)
+
+ elif train_sampler == 'RandomDatasetSampler':
+ sampler = RandomDatasetSampler(data_source, batch_size, num_datasets)
+
+ elif train_sampler == 'SequentialSampler':
+ sampler = SequentialSampler(data_source)
+
+ elif train_sampler == 'RandomSampler':
+ sampler = RandomSampler(data_source)
+
+ return sampler
diff --git a/trackers/strong_sort/deep/reid/torchreid/data/transforms.py b/trackers/strong_sort/deep/reid/torchreid/data/transforms.py
new file mode 100644
index 0000000000000000000000000000000000000000..0c09ca0ed0a0316c3a3087f1ca137fdde0c48be1
--- /dev/null
+++ b/trackers/strong_sort/deep/reid/torchreid/data/transforms.py
@@ -0,0 +1,326 @@
+from __future__ import division, print_function, absolute_import
+import math
+import random
+from collections import deque
+import torch
+from PIL import Image
+from torchvision.transforms import (
+ Resize, Compose, ToTensor, Normalize, ColorJitter, RandomHorizontalFlip
+)
+
+
+class Random2DTranslation(object):
+ """Randomly translates the input image with a probability.
+
+ Specifically, given a predefined shape (height, width), the input is first
+ resized with a factor of 1.125, leading to (height*1.125, width*1.125), then
+ a random crop is performed. Such operation is done with a probability.
+
+ Args:
+ height (int): target image height.
+ width (int): target image width.
+ p (float, optional): probability that this operation takes place.
+ Default is 0.5.
+ interpolation (int, optional): desired interpolation. Default is
+ ``PIL.Image.BILINEAR``
+ """
+
+ def __init__(self, height, width, p=0.5, interpolation=Image.BILINEAR):
+ self.height = height
+ self.width = width
+ self.p = p
+ self.interpolation = interpolation
+
+ def __call__(self, img):
+ if random.uniform(0, 1) > self.p:
+ return img.resize((self.width, self.height), self.interpolation)
+
+ new_width, new_height = int(round(self.width * 1.125)
+ ), int(round(self.height * 1.125))
+ resized_img = img.resize((new_width, new_height), self.interpolation)
+ x_maxrange = new_width - self.width
+ y_maxrange = new_height - self.height
+ x1 = int(round(random.uniform(0, x_maxrange)))
+ y1 = int(round(random.uniform(0, y_maxrange)))
+ croped_img = resized_img.crop(
+ (x1, y1, x1 + self.width, y1 + self.height)
+ )
+ return croped_img
+
+
+class RandomErasing(object):
+ """Randomly erases an image patch.
+
+ Origin: ``_
+
+ Reference:
+ Zhong et al. Random Erasing Data Augmentation.
+
+ Args:
+ probability (float, optional): probability that this operation takes place.
+ Default is 0.5.
+ sl (float, optional): min erasing area.
+ sh (float, optional): max erasing area.
+ r1 (float, optional): min aspect ratio.
+ mean (list, optional): erasing value.
+ """
+
+ def __init__(
+ self,
+ probability=0.5,
+ sl=0.02,
+ sh=0.4,
+ r1=0.3,
+ mean=[0.4914, 0.4822, 0.4465]
+ ):
+ self.probability = probability
+ self.mean = mean
+ self.sl = sl
+ self.sh = sh
+ self.r1 = r1
+
+ def __call__(self, img):
+ if random.uniform(0, 1) > self.probability:
+ return img
+
+ for attempt in range(100):
+ area = img.size()[1] * img.size()[2]
+
+ target_area = random.uniform(self.sl, self.sh) * area
+ aspect_ratio = random.uniform(self.r1, 1 / self.r1)
+
+ h = int(round(math.sqrt(target_area * aspect_ratio)))
+ w = int(round(math.sqrt(target_area / aspect_ratio)))
+
+ if w < img.size()[2] and h < img.size()[1]:
+ x1 = random.randint(0, img.size()[1] - h)
+ y1 = random.randint(0, img.size()[2] - w)
+ if img.size()[0] == 3:
+ img[0, x1:x1 + h, y1:y1 + w] = self.mean[0]
+ img[1, x1:x1 + h, y1:y1 + w] = self.mean[1]
+ img[2, x1:x1 + h, y1:y1 + w] = self.mean[2]
+ else:
+ img[0, x1:x1 + h, y1:y1 + w] = self.mean[0]
+ return img
+
+ return img
+
+
+class ColorAugmentation(object):
+ """Randomly alters the intensities of RGB channels.
+
+ Reference:
+ Krizhevsky et al. ImageNet Classification with Deep ConvolutionalNeural
+ Networks. NIPS 2012.
+
+ Args:
+ p (float, optional): probability that this operation takes place.
+ Default is 0.5.
+ """
+
+ def __init__(self, p=0.5):
+ self.p = p
+ self.eig_vec = torch.Tensor(
+ [
+ [0.4009, 0.7192, -0.5675],
+ [-0.8140, -0.0045, -0.5808],
+ [0.4203, -0.6948, -0.5836],
+ ]
+ )
+ self.eig_val = torch.Tensor([[0.2175, 0.0188, 0.0045]])
+
+ def _check_input(self, tensor):
+ assert tensor.dim() == 3 and tensor.size(0) == 3
+
+ def __call__(self, tensor):
+ if random.uniform(0, 1) > self.p:
+ return tensor
+ alpha = torch.normal(mean=torch.zeros_like(self.eig_val)) * 0.1
+ quatity = torch.mm(self.eig_val * alpha, self.eig_vec)
+ tensor = tensor + quatity.view(3, 1, 1)
+ return tensor
+
+
+class RandomPatch(object):
+ """Random patch data augmentation.
+
+ There is a patch pool that stores randomly extracted pathces from person images.
+
+ For each input image, RandomPatch
+ 1) extracts a random patch and stores the patch in the patch pool;
+ 2) randomly selects a patch from the patch pool and pastes it on the
+ input (at random position) to simulate occlusion.
+
+ Reference:
+ - Zhou et al. Omni-Scale Feature Learning for Person Re-Identification. ICCV, 2019.
+ - Zhou et al. Learning Generalisable Omni-Scale Representations
+ for Person Re-Identification. TPAMI, 2021.
+ """
+
+ def __init__(
+ self,
+ prob_happen=0.5,
+ pool_capacity=50000,
+ min_sample_size=100,
+ patch_min_area=0.01,
+ patch_max_area=0.5,
+ patch_min_ratio=0.1,
+ prob_rotate=0.5,
+ prob_flip_leftright=0.5,
+ ):
+ self.prob_happen = prob_happen
+
+ self.patch_min_area = patch_min_area
+ self.patch_max_area = patch_max_area
+ self.patch_min_ratio = patch_min_ratio
+
+ self.prob_rotate = prob_rotate
+ self.prob_flip_leftright = prob_flip_leftright
+
+ self.patchpool = deque(maxlen=pool_capacity)
+ self.min_sample_size = min_sample_size
+
+ def generate_wh(self, W, H):
+ area = W * H
+ for attempt in range(100):
+ target_area = random.uniform(
+ self.patch_min_area, self.patch_max_area
+ ) * area
+ aspect_ratio = random.uniform(
+ self.patch_min_ratio, 1. / self.patch_min_ratio
+ )
+ h = int(round(math.sqrt(target_area * aspect_ratio)))
+ w = int(round(math.sqrt(target_area / aspect_ratio)))
+ if w < W and h < H:
+ return w, h
+ return None, None
+
+ def transform_patch(self, patch):
+ if random.uniform(0, 1) > self.prob_flip_leftright:
+ patch = patch.transpose(Image.FLIP_LEFT_RIGHT)
+ if random.uniform(0, 1) > self.prob_rotate:
+ patch = patch.rotate(random.randint(-10, 10))
+ return patch
+
+ def __call__(self, img):
+ W, H = img.size # original image size
+
+ # collect new patch
+ w, h = self.generate_wh(W, H)
+ if w is not None and h is not None:
+ x1 = random.randint(0, W - w)
+ y1 = random.randint(0, H - h)
+ new_patch = img.crop((x1, y1, x1 + w, y1 + h))
+ self.patchpool.append(new_patch)
+
+ if len(self.patchpool) < self.min_sample_size:
+ return img
+
+ if random.uniform(0, 1) > self.prob_happen:
+ return img
+
+ # paste a randomly selected patch on a random position
+ patch = random.sample(self.patchpool, 1)[0]
+ patchW, patchH = patch.size
+ x1 = random.randint(0, W - patchW)
+ y1 = random.randint(0, H - patchH)
+ patch = self.transform_patch(patch)
+ img.paste(patch, (x1, y1))
+
+ return img
+
+
+def build_transforms(
+ height,
+ width,
+ transforms='random_flip',
+ norm_mean=[0.485, 0.456, 0.406],
+ norm_std=[0.229, 0.224, 0.225],
+ **kwargs
+):
+ """Builds train and test transform functions.
+
+ Args:
+ height (int): target image height.
+ width (int): target image width.
+ transforms (str or list of str, optional): transformations applied to model training.
+ Default is 'random_flip'.
+ norm_mean (list or None, optional): normalization mean values. Default is ImageNet means.
+ norm_std (list or None, optional): normalization standard deviation values. Default is
+ ImageNet standard deviation values.
+ """
+ if transforms is None:
+ transforms = []
+
+ if isinstance(transforms, str):
+ transforms = [transforms]
+
+ if not isinstance(transforms, list):
+ raise ValueError(
+ 'transforms must be a list of strings, but found to be {}'.format(
+ type(transforms)
+ )
+ )
+
+ if len(transforms) > 0:
+ transforms = [t.lower() for t in transforms]
+
+ if norm_mean is None or norm_std is None:
+ norm_mean = [0.485, 0.456, 0.406] # imagenet mean
+ norm_std = [0.229, 0.224, 0.225] # imagenet std
+ normalize = Normalize(mean=norm_mean, std=norm_std)
+
+ print('Building train transforms ...')
+ transform_tr = []
+
+ print('+ resize to {}x{}'.format(height, width))
+ transform_tr += [Resize((height, width))]
+
+ if 'random_flip' in transforms:
+ print('+ random flip')
+ transform_tr += [RandomHorizontalFlip()]
+
+ if 'random_crop' in transforms:
+ print(
+ '+ random crop (enlarge to {}x{} and '
+ 'crop {}x{})'.format(
+ int(round(height * 1.125)), int(round(width * 1.125)), height,
+ width
+ )
+ )
+ transform_tr += [Random2DTranslation(height, width)]
+
+ if 'random_patch' in transforms:
+ print('+ random patch')
+ transform_tr += [RandomPatch()]
+
+ if 'color_jitter' in transforms:
+ print('+ color jitter')
+ transform_tr += [
+ ColorJitter(brightness=0.2, contrast=0.15, saturation=0, hue=0)
+ ]
+
+ print('+ to torch tensor of range [0, 1]')
+ transform_tr += [ToTensor()]
+
+ print('+ normalization (mean={}, std={})'.format(norm_mean, norm_std))
+ transform_tr += [normalize]
+
+ if 'random_erase' in transforms:
+ print('+ random erase')
+ transform_tr += [RandomErasing(mean=norm_mean)]
+
+ transform_tr = Compose(transform_tr)
+
+ print('Building test transforms ...')
+ print('+ resize to {}x{}'.format(height, width))
+ print('+ to torch tensor of range [0, 1]')
+ print('+ normalization (mean={}, std={})'.format(norm_mean, norm_std))
+
+ transform_te = Compose([
+ Resize((height, width)),
+ ToTensor(),
+ normalize,
+ ])
+
+ return transform_tr, transform_te
diff --git a/trackers/strong_sort/deep/reid/torchreid/engine/__init__.py b/trackers/strong_sort/deep/reid/torchreid/engine/__init__.py
new file mode 100644
index 0000000000000000000000000000000000000000..a39cc7f767e5350132b5e165a87624c7ca80bf80
--- /dev/null
+++ b/trackers/strong_sort/deep/reid/torchreid/engine/__init__.py
@@ -0,0 +1,5 @@
+from __future__ import print_function, absolute_import
+
+from .image import ImageSoftmaxEngine, ImageTripletEngine
+from .video import VideoSoftmaxEngine, VideoTripletEngine
+from .engine import Engine
diff --git a/trackers/strong_sort/deep/reid/torchreid/engine/engine.py b/trackers/strong_sort/deep/reid/torchreid/engine/engine.py
new file mode 100644
index 0000000000000000000000000000000000000000..bbc01e09ea542c4f2c08b49a78e3610627a0143a
--- /dev/null
+++ b/trackers/strong_sort/deep/reid/torchreid/engine/engine.py
@@ -0,0 +1,478 @@
+from __future__ import division, print_function, absolute_import
+import time
+import numpy as np
+import os.path as osp
+import datetime
+from collections import OrderedDict
+import torch
+from torch.nn import functional as F
+from torch.utils.tensorboard import SummaryWriter
+
+from torchreid import metrics
+from torchreid.utils import (
+ MetricMeter, AverageMeter, re_ranking, open_all_layers, save_checkpoint,
+ open_specified_layers, visualize_ranked_results
+)
+from torchreid.losses import DeepSupervision
+
+
+class Engine(object):
+ r"""A generic base Engine class for both image- and video-reid.
+
+ Args:
+ datamanager (DataManager): an instance of ``torchreid.data.ImageDataManager``
+ or ``torchreid.data.VideoDataManager``.
+ use_gpu (bool, optional): use gpu. Default is True.
+ """
+
+ def __init__(self, datamanager, use_gpu=True):
+ self.datamanager = datamanager
+ self.train_loader = self.datamanager.train_loader
+ self.test_loader = self.datamanager.test_loader
+ self.use_gpu = (torch.cuda.is_available() and use_gpu)
+ self.writer = None
+ self.epoch = 0
+
+ self.model = None
+ self.optimizer = None
+ self.scheduler = None
+
+ self._models = OrderedDict()
+ self._optims = OrderedDict()
+ self._scheds = OrderedDict()
+
+ def register_model(self, name='model', model=None, optim=None, sched=None):
+ if self.__dict__.get('_models') is None:
+ raise AttributeError(
+ 'Cannot assign model before super().__init__() call'
+ )
+
+ if self.__dict__.get('_optims') is None:
+ raise AttributeError(
+ 'Cannot assign optim before super().__init__() call'
+ )
+
+ if self.__dict__.get('_scheds') is None:
+ raise AttributeError(
+ 'Cannot assign sched before super().__init__() call'
+ )
+
+ self._models[name] = model
+ self._optims[name] = optim
+ self._scheds[name] = sched
+
+ def get_model_names(self, names=None):
+ names_real = list(self._models.keys())
+ if names is not None:
+ if not isinstance(names, list):
+ names = [names]
+ for name in names:
+ assert name in names_real
+ return names
+ else:
+ return names_real
+
+ def save_model(self, epoch, rank1, save_dir, is_best=False):
+ names = self.get_model_names()
+
+ for name in names:
+ save_checkpoint(
+ {
+ 'state_dict': self._models[name].state_dict(),
+ 'epoch': epoch + 1,
+ 'rank1': rank1,
+ 'optimizer': self._optims[name].state_dict(),
+ 'scheduler': self._scheds[name].state_dict()
+ },
+ osp.join(save_dir, name),
+ is_best=is_best
+ )
+
+ def set_model_mode(self, mode='train', names=None):
+ assert mode in ['train', 'eval', 'test']
+ names = self.get_model_names(names)
+
+ for name in names:
+ if mode == 'train':
+ self._models[name].train()
+ else:
+ self._models[name].eval()
+
+ def get_current_lr(self, names=None):
+ names = self.get_model_names(names)
+ name = names[0]
+ return self._optims[name].param_groups[-1]['lr']
+
+ def update_lr(self, names=None):
+ names = self.get_model_names(names)
+
+ for name in names:
+ if self._scheds[name] is not None:
+ self._scheds[name].step()
+
+ def run(
+ self,
+ save_dir='log',
+ max_epoch=0,
+ start_epoch=0,
+ print_freq=10,
+ fixbase_epoch=0,
+ open_layers=None,
+ start_eval=0,
+ eval_freq=-1,
+ test_only=False,
+ dist_metric='euclidean',
+ normalize_feature=False,
+ visrank=False,
+ visrank_topk=10,
+ use_metric_cuhk03=False,
+ ranks=[1, 5, 10, 20],
+ rerank=False
+ ):
+ r"""A unified pipeline for training and evaluating a model.
+
+ Args:
+ save_dir (str): directory to save model.
+ max_epoch (int): maximum epoch.
+ start_epoch (int, optional): starting epoch. Default is 0.
+ print_freq (int, optional): print_frequency. Default is 10.
+ fixbase_epoch (int, optional): number of epochs to train ``open_layers`` (new layers)
+ while keeping base layers frozen. Default is 0. ``fixbase_epoch`` is counted
+ in ``max_epoch``.
+ open_layers (str or list, optional): layers (attribute names) open for training.
+ start_eval (int, optional): from which epoch to start evaluation. Default is 0.
+ eval_freq (int, optional): evaluation frequency. Default is -1 (meaning evaluation
+ is only performed at the end of training).
+ test_only (bool, optional): if True, only runs evaluation on test datasets.
+ Default is False.
+ dist_metric (str, optional): distance metric used to compute distance matrix
+ between query and gallery. Default is "euclidean".
+ normalize_feature (bool, optional): performs L2 normalization on feature vectors before
+ computing feature distance. Default is False.
+ visrank (bool, optional): visualizes ranked results. Default is False. It is recommended to
+ enable ``visrank`` when ``test_only`` is True. The ranked images will be saved to
+ "save_dir/visrank_dataset", e.g. "save_dir/visrank_market1501".
+ visrank_topk (int, optional): top-k ranked images to be visualized. Default is 10.
+ use_metric_cuhk03 (bool, optional): use single-gallery-shot setting for cuhk03.
+ Default is False. This should be enabled when using cuhk03 classic split.
+ ranks (list, optional): cmc ranks to be computed. Default is [1, 5, 10, 20].
+ rerank (bool, optional): uses person re-ranking (by Zhong et al. CVPR'17).
+ Default is False. This is only enabled when test_only=True.
+ """
+
+ if visrank and not test_only:
+ raise ValueError(
+ 'visrank can be set to True only if test_only=True'
+ )
+
+ if test_only:
+ self.test(
+ dist_metric=dist_metric,
+ normalize_feature=normalize_feature,
+ visrank=visrank,
+ visrank_topk=visrank_topk,
+ save_dir=save_dir,
+ use_metric_cuhk03=use_metric_cuhk03,
+ ranks=ranks,
+ rerank=rerank
+ )
+ return
+
+ if self.writer is None:
+ self.writer = SummaryWriter(log_dir=save_dir)
+
+ time_start = time.time()
+ self.start_epoch = start_epoch
+ self.max_epoch = max_epoch
+ print('=> Start training')
+
+ for self.epoch in range(self.start_epoch, self.max_epoch):
+ self.train(
+ print_freq=print_freq,
+ fixbase_epoch=fixbase_epoch,
+ open_layers=open_layers
+ )
+
+ if (self.epoch + 1) >= start_eval \
+ and eval_freq > 0 \
+ and (self.epoch+1) % eval_freq == 0 \
+ and (self.epoch + 1) != self.max_epoch:
+ rank1 = self.test(
+ dist_metric=dist_metric,
+ normalize_feature=normalize_feature,
+ visrank=visrank,
+ visrank_topk=visrank_topk,
+ save_dir=save_dir,
+ use_metric_cuhk03=use_metric_cuhk03,
+ ranks=ranks
+ )
+ self.save_model(self.epoch, rank1, save_dir)
+
+ if self.max_epoch > 0:
+ print('=> Final test')
+ rank1 = self.test(
+ dist_metric=dist_metric,
+ normalize_feature=normalize_feature,
+ visrank=visrank,
+ visrank_topk=visrank_topk,
+ save_dir=save_dir,
+ use_metric_cuhk03=use_metric_cuhk03,
+ ranks=ranks
+ )
+ self.save_model(self.epoch, rank1, save_dir)
+
+ elapsed = round(time.time() - time_start)
+ elapsed = str(datetime.timedelta(seconds=elapsed))
+ print('Elapsed {}'.format(elapsed))
+ if self.writer is not None:
+ self.writer.close()
+
+ def train(self, print_freq=10, fixbase_epoch=0, open_layers=None):
+ losses = MetricMeter()
+ batch_time = AverageMeter()
+ data_time = AverageMeter()
+
+ self.set_model_mode('train')
+
+ self.two_stepped_transfer_learning(
+ self.epoch, fixbase_epoch, open_layers
+ )
+
+ self.num_batches = len(self.train_loader)
+ end = time.time()
+ for self.batch_idx, data in enumerate(self.train_loader):
+ data_time.update(time.time() - end)
+ loss_summary = self.forward_backward(data)
+ batch_time.update(time.time() - end)
+ losses.update(loss_summary)
+
+ if (self.batch_idx + 1) % print_freq == 0:
+ nb_this_epoch = self.num_batches - (self.batch_idx + 1)
+ nb_future_epochs = (
+ self.max_epoch - (self.epoch + 1)
+ ) * self.num_batches
+ eta_seconds = batch_time.avg * (nb_this_epoch+nb_future_epochs)
+ eta_str = str(datetime.timedelta(seconds=int(eta_seconds)))
+ print(
+ 'epoch: [{0}/{1}][{2}/{3}]\t'
+ 'time {batch_time.val:.3f} ({batch_time.avg:.3f})\t'
+ 'data {data_time.val:.3f} ({data_time.avg:.3f})\t'
+ 'eta {eta}\t'
+ '{losses}\t'
+ 'lr {lr:.6f}'.format(
+ self.epoch + 1,
+ self.max_epoch,
+ self.batch_idx + 1,
+ self.num_batches,
+ batch_time=batch_time,
+ data_time=data_time,
+ eta=eta_str,
+ losses=losses,
+ lr=self.get_current_lr()
+ )
+ )
+
+ if self.writer is not None:
+ n_iter = self.epoch * self.num_batches + self.batch_idx
+ self.writer.add_scalar('Train/time', batch_time.avg, n_iter)
+ self.writer.add_scalar('Train/data', data_time.avg, n_iter)
+ for name, meter in losses.meters.items():
+ self.writer.add_scalar('Train/' + name, meter.avg, n_iter)
+ self.writer.add_scalar(
+ 'Train/lr', self.get_current_lr(), n_iter
+ )
+
+ end = time.time()
+
+ self.update_lr()
+
+ def forward_backward(self, data):
+ raise NotImplementedError
+
+ def test(
+ self,
+ dist_metric='euclidean',
+ normalize_feature=False,
+ visrank=False,
+ visrank_topk=10,
+ save_dir='',
+ use_metric_cuhk03=False,
+ ranks=[1, 5, 10, 20],
+ rerank=False
+ ):
+ r"""Tests model on target datasets.
+
+ .. note::
+
+ This function has been called in ``run()``.
+
+ .. note::
+
+ The test pipeline implemented in this function suits both image- and
+ video-reid. In general, a subclass of Engine only needs to re-implement
+ ``extract_features()`` and ``parse_data_for_eval()`` (most of the time),
+ but not a must. Please refer to the source code for more details.
+ """
+ self.set_model_mode('eval')
+ targets = list(self.test_loader.keys())
+
+ for name in targets:
+ domain = 'source' if name in self.datamanager.sources else 'target'
+ print('##### Evaluating {} ({}) #####'.format(name, domain))
+ query_loader = self.test_loader[name]['query']
+ gallery_loader = self.test_loader[name]['gallery']
+ rank1, mAP = self._evaluate(
+ dataset_name=name,
+ query_loader=query_loader,
+ gallery_loader=gallery_loader,
+ dist_metric=dist_metric,
+ normalize_feature=normalize_feature,
+ visrank=visrank,
+ visrank_topk=visrank_topk,
+ save_dir=save_dir,
+ use_metric_cuhk03=use_metric_cuhk03,
+ ranks=ranks,
+ rerank=rerank
+ )
+
+ if self.writer is not None:
+ self.writer.add_scalar(f'Test/{name}/rank1', rank1, self.epoch)
+ self.writer.add_scalar(f'Test/{name}/mAP', mAP, self.epoch)
+
+ return rank1
+
+ @torch.no_grad()
+ def _evaluate(
+ self,
+ dataset_name='',
+ query_loader=None,
+ gallery_loader=None,
+ dist_metric='euclidean',
+ normalize_feature=False,
+ visrank=False,
+ visrank_topk=10,
+ save_dir='',
+ use_metric_cuhk03=False,
+ ranks=[1, 5, 10, 20],
+ rerank=False
+ ):
+ batch_time = AverageMeter()
+
+ def _feature_extraction(data_loader):
+ f_, pids_, camids_ = [], [], []
+ for batch_idx, data in enumerate(data_loader):
+ imgs, pids, camids = self.parse_data_for_eval(data)
+ if self.use_gpu:
+ imgs = imgs.cuda()
+ end = time.time()
+ features = self.extract_features(imgs)
+ batch_time.update(time.time() - end)
+ features = features.cpu()
+ f_.append(features)
+ pids_.extend(pids.tolist())
+ camids_.extend(camids.tolist())
+ f_ = torch.cat(f_, 0)
+ pids_ = np.asarray(pids_)
+ camids_ = np.asarray(camids_)
+ return f_, pids_, camids_
+
+ print('Extracting features from query set ...')
+ qf, q_pids, q_camids = _feature_extraction(query_loader)
+ print('Done, obtained {}-by-{} matrix'.format(qf.size(0), qf.size(1)))
+
+ print('Extracting features from gallery set ...')
+ gf, g_pids, g_camids = _feature_extraction(gallery_loader)
+ print('Done, obtained {}-by-{} matrix'.format(gf.size(0), gf.size(1)))
+
+ print('Speed: {:.4f} sec/batch'.format(batch_time.avg))
+
+ if normalize_feature:
+ print('Normalzing features with L2 norm ...')
+ qf = F.normalize(qf, p=2, dim=1)
+ gf = F.normalize(gf, p=2, dim=1)
+
+ print(
+ 'Computing distance matrix with metric={} ...'.format(dist_metric)
+ )
+ distmat = metrics.compute_distance_matrix(qf, gf, dist_metric)
+ distmat = distmat.numpy()
+
+ if rerank:
+ print('Applying person re-ranking ...')
+ distmat_qq = metrics.compute_distance_matrix(qf, qf, dist_metric)
+ distmat_gg = metrics.compute_distance_matrix(gf, gf, dist_metric)
+ distmat = re_ranking(distmat, distmat_qq, distmat_gg)
+
+ print('Computing CMC and mAP ...')
+ cmc, mAP = metrics.evaluate_rank(
+ distmat,
+ q_pids,
+ g_pids,
+ q_camids,
+ g_camids,
+ use_metric_cuhk03=use_metric_cuhk03
+ )
+
+ print('** Results **')
+ print('mAP: {:.1%}'.format(mAP))
+ print('CMC curve')
+ for r in ranks:
+ print('Rank-{:<3}: {:.1%}'.format(r, cmc[r - 1]))
+
+ if visrank:
+ visualize_ranked_results(
+ distmat,
+ self.datamanager.fetch_test_loaders(dataset_name),
+ self.datamanager.data_type,
+ width=self.datamanager.width,
+ height=self.datamanager.height,
+ save_dir=osp.join(save_dir, 'visrank_' + dataset_name),
+ topk=visrank_topk
+ )
+
+ return cmc[0], mAP
+
+ def compute_loss(self, criterion, outputs, targets):
+ if isinstance(outputs, (tuple, list)):
+ loss = DeepSupervision(criterion, outputs, targets)
+ else:
+ loss = criterion(outputs, targets)
+ return loss
+
+ def extract_features(self, input):
+ return self.model(input)
+
+ def parse_data_for_train(self, data):
+ imgs = data['img']
+ pids = data['pid']
+ return imgs, pids
+
+ def parse_data_for_eval(self, data):
+ imgs = data['img']
+ pids = data['pid']
+ camids = data['camid']
+ return imgs, pids, camids
+
+ def two_stepped_transfer_learning(
+ self, epoch, fixbase_epoch, open_layers, model=None
+ ):
+ """Two-stepped transfer learning.
+
+ The idea is to freeze base layers for a certain number of epochs
+ and then open all layers for training.
+
+ Reference: https://arxiv.org/abs/1611.05244
+ """
+ model = self.model if model is None else model
+ if model is None:
+ return
+
+ if (epoch + 1) <= fixbase_epoch and open_layers is not None:
+ print(
+ '* Only train {} (epoch: {}/{})'.format(
+ open_layers, epoch + 1, fixbase_epoch
+ )
+ )
+ open_specified_layers(model, open_layers)
+ else:
+ open_all_layers(model)
diff --git a/trackers/strong_sort/deep/reid/torchreid/engine/image/__init__.py b/trackers/strong_sort/deep/reid/torchreid/engine/image/__init__.py
new file mode 100644
index 0000000000000000000000000000000000000000..08d313a53f99f1524f973f3d998f182c59950df1
--- /dev/null
+++ b/trackers/strong_sort/deep/reid/torchreid/engine/image/__init__.py
@@ -0,0 +1,4 @@
+from __future__ import absolute_import
+
+from .softmax import ImageSoftmaxEngine
+from .triplet import ImageTripletEngine
diff --git a/trackers/strong_sort/deep/reid/torchreid/engine/image/softmax.py b/trackers/strong_sort/deep/reid/torchreid/engine/image/softmax.py
new file mode 100644
index 0000000000000000000000000000000000000000..5785d4f8c612de60b82de37dfdac2820c793545e
--- /dev/null
+++ b/trackers/strong_sort/deep/reid/torchreid/engine/image/softmax.py
@@ -0,0 +1,97 @@
+from __future__ import division, print_function, absolute_import
+
+from torchreid import metrics
+from torchreid.losses import CrossEntropyLoss
+
+from ..engine import Engine
+
+
+class ImageSoftmaxEngine(Engine):
+ r"""Softmax-loss engine for image-reid.
+
+ Args:
+ datamanager (DataManager): an instance of ``torchreid.data.ImageDataManager``
+ or ``torchreid.data.VideoDataManager``.
+ model (nn.Module): model instance.
+ optimizer (Optimizer): an Optimizer.
+ scheduler (LRScheduler, optional): if None, no learning rate decay will be performed.
+ use_gpu (bool, optional): use gpu. Default is True.
+ label_smooth (bool, optional): use label smoothing regularizer. Default is True.
+
+ Examples::
+
+ import torchreid
+ datamanager = torchreid.data.ImageDataManager(
+ root='path/to/reid-data',
+ sources='market1501',
+ height=256,
+ width=128,
+ combineall=False,
+ batch_size=32
+ )
+ model = torchreid.models.build_model(
+ name='resnet50',
+ num_classes=datamanager.num_train_pids,
+ loss='softmax'
+ )
+ model = model.cuda()
+ optimizer = torchreid.optim.build_optimizer(
+ model, optim='adam', lr=0.0003
+ )
+ scheduler = torchreid.optim.build_lr_scheduler(
+ optimizer,
+ lr_scheduler='single_step',
+ stepsize=20
+ )
+ engine = torchreid.engine.ImageSoftmaxEngine(
+ datamanager, model, optimizer, scheduler=scheduler
+ )
+ engine.run(
+ max_epoch=60,
+ save_dir='log/resnet50-softmax-market1501',
+ print_freq=10
+ )
+ """
+
+ def __init__(
+ self,
+ datamanager,
+ model,
+ optimizer,
+ scheduler=None,
+ use_gpu=True,
+ label_smooth=True
+ ):
+ super(ImageSoftmaxEngine, self).__init__(datamanager, use_gpu)
+
+ self.model = model
+ self.optimizer = optimizer
+ self.scheduler = scheduler
+ self.register_model('model', model, optimizer, scheduler)
+
+ self.criterion = CrossEntropyLoss(
+ num_classes=self.datamanager.num_train_pids,
+ use_gpu=self.use_gpu,
+ label_smooth=label_smooth
+ )
+
+ def forward_backward(self, data):
+ imgs, pids = self.parse_data_for_train(data)
+
+ if self.use_gpu:
+ imgs = imgs.cuda()
+ pids = pids.cuda()
+
+ outputs = self.model(imgs)
+ loss = self.compute_loss(self.criterion, outputs, pids)
+
+ self.optimizer.zero_grad()
+ loss.backward()
+ self.optimizer.step()
+
+ loss_summary = {
+ 'loss': loss.item(),
+ 'acc': metrics.accuracy(outputs, pids)[0].item()
+ }
+
+ return loss_summary
diff --git a/trackers/strong_sort/deep/reid/torchreid/engine/image/triplet.py b/trackers/strong_sort/deep/reid/torchreid/engine/image/triplet.py
new file mode 100644
index 0000000000000000000000000000000000000000..cd15cfb203cbb18244b440ac7e74f253bd1db8a8
--- /dev/null
+++ b/trackers/strong_sort/deep/reid/torchreid/engine/image/triplet.py
@@ -0,0 +1,122 @@
+from __future__ import division, print_function, absolute_import
+
+from torchreid import metrics
+from torchreid.losses import TripletLoss, CrossEntropyLoss
+
+from ..engine import Engine
+
+
+class ImageTripletEngine(Engine):
+ r"""Triplet-loss engine for image-reid.
+
+ Args:
+ datamanager (DataManager): an instance of ``torchreid.data.ImageDataManager``
+ or ``torchreid.data.VideoDataManager``.
+ model (nn.Module): model instance.
+ optimizer (Optimizer): an Optimizer.
+ margin (float, optional): margin for triplet loss. Default is 0.3.
+ weight_t (float, optional): weight for triplet loss. Default is 1.
+ weight_x (float, optional): weight for softmax loss. Default is 1.
+ scheduler (LRScheduler, optional): if None, no learning rate decay will be performed.
+ use_gpu (bool, optional): use gpu. Default is True.
+ label_smooth (bool, optional): use label smoothing regularizer. Default is True.
+
+ Examples::
+
+ import torchreid
+ datamanager = torchreid.data.ImageDataManager(
+ root='path/to/reid-data',
+ sources='market1501',
+ height=256,
+ width=128,
+ combineall=False,
+ batch_size=32,
+ num_instances=4,
+ train_sampler='RandomIdentitySampler' # this is important
+ )
+ model = torchreid.models.build_model(
+ name='resnet50',
+ num_classes=datamanager.num_train_pids,
+ loss='triplet'
+ )
+ model = model.cuda()
+ optimizer = torchreid.optim.build_optimizer(
+ model, optim='adam', lr=0.0003
+ )
+ scheduler = torchreid.optim.build_lr_scheduler(
+ optimizer,
+ lr_scheduler='single_step',
+ stepsize=20
+ )
+ engine = torchreid.engine.ImageTripletEngine(
+ datamanager, model, optimizer, margin=0.3,
+ weight_t=0.7, weight_x=1, scheduler=scheduler
+ )
+ engine.run(
+ max_epoch=60,
+ save_dir='log/resnet50-triplet-market1501',
+ print_freq=10
+ )
+ """
+
+ def __init__(
+ self,
+ datamanager,
+ model,
+ optimizer,
+ margin=0.3,
+ weight_t=1,
+ weight_x=1,
+ scheduler=None,
+ use_gpu=True,
+ label_smooth=True
+ ):
+ super(ImageTripletEngine, self).__init__(datamanager, use_gpu)
+
+ self.model = model
+ self.optimizer = optimizer
+ self.scheduler = scheduler
+ self.register_model('model', model, optimizer, scheduler)
+
+ assert weight_t >= 0 and weight_x >= 0
+ assert weight_t + weight_x > 0
+ self.weight_t = weight_t
+ self.weight_x = weight_x
+
+ self.criterion_t = TripletLoss(margin=margin)
+ self.criterion_x = CrossEntropyLoss(
+ num_classes=self.datamanager.num_train_pids,
+ use_gpu=self.use_gpu,
+ label_smooth=label_smooth
+ )
+
+ def forward_backward(self, data):
+ imgs, pids = self.parse_data_for_train(data)
+
+ if self.use_gpu:
+ imgs = imgs.cuda()
+ pids = pids.cuda()
+
+ outputs, features = self.model(imgs)
+
+ loss = 0
+ loss_summary = {}
+
+ if self.weight_t > 0:
+ loss_t = self.compute_loss(self.criterion_t, features, pids)
+ loss += self.weight_t * loss_t
+ loss_summary['loss_t'] = loss_t.item()
+
+ if self.weight_x > 0:
+ loss_x = self.compute_loss(self.criterion_x, outputs, pids)
+ loss += self.weight_x * loss_x
+ loss_summary['loss_x'] = loss_x.item()
+ loss_summary['acc'] = metrics.accuracy(outputs, pids)[0].item()
+
+ assert loss_summary
+
+ self.optimizer.zero_grad()
+ loss.backward()
+ self.optimizer.step()
+
+ return loss_summary
diff --git a/trackers/strong_sort/deep/reid/torchreid/engine/video/__init__.py b/trackers/strong_sort/deep/reid/torchreid/engine/video/__init__.py
new file mode 100644
index 0000000000000000000000000000000000000000..b818bf4d32cbb3eba1545f1900749a2dd3636af1
--- /dev/null
+++ b/trackers/strong_sort/deep/reid/torchreid/engine/video/__init__.py
@@ -0,0 +1,4 @@
+from __future__ import absolute_import
+
+from .softmax import VideoSoftmaxEngine
+from .triplet import VideoTripletEngine
diff --git a/trackers/strong_sort/deep/reid/torchreid/engine/video/softmax.py b/trackers/strong_sort/deep/reid/torchreid/engine/video/softmax.py
new file mode 100644
index 0000000000000000000000000000000000000000..fe92feba11935900f3c01e8591ce3eaa19bf6862
--- /dev/null
+++ b/trackers/strong_sort/deep/reid/torchreid/engine/video/softmax.py
@@ -0,0 +1,109 @@
+from __future__ import division, print_function, absolute_import
+import torch
+
+from torchreid.engine.image import ImageSoftmaxEngine
+
+
+class VideoSoftmaxEngine(ImageSoftmaxEngine):
+ """Softmax-loss engine for video-reid.
+
+ Args:
+ datamanager (DataManager): an instance of ``torchreid.data.ImageDataManager``
+ or ``torchreid.data.VideoDataManager``.
+ model (nn.Module): model instance.
+ optimizer (Optimizer): an Optimizer.
+ scheduler (LRScheduler, optional): if None, no learning rate decay will be performed.
+ use_gpu (bool, optional): use gpu. Default is True.
+ label_smooth (bool, optional): use label smoothing regularizer. Default is True.
+ pooling_method (str, optional): how to pool features for a tracklet.
+ Default is "avg" (average). Choices are ["avg", "max"].
+
+ Examples::
+
+ import torch
+ import torchreid
+ # Each batch contains batch_size*seq_len images
+ datamanager = torchreid.data.VideoDataManager(
+ root='path/to/reid-data',
+ sources='mars',
+ height=256,
+ width=128,
+ combineall=False,
+ batch_size=8, # number of tracklets
+ seq_len=15 # number of images in each tracklet
+ )
+ model = torchreid.models.build_model(
+ name='resnet50',
+ num_classes=datamanager.num_train_pids,
+ loss='softmax'
+ )
+ model = model.cuda()
+ optimizer = torchreid.optim.build_optimizer(
+ model, optim='adam', lr=0.0003
+ )
+ scheduler = torchreid.optim.build_lr_scheduler(
+ optimizer,
+ lr_scheduler='single_step',
+ stepsize=20
+ )
+ engine = torchreid.engine.VideoSoftmaxEngine(
+ datamanager, model, optimizer, scheduler=scheduler,
+ pooling_method='avg'
+ )
+ engine.run(
+ max_epoch=60,
+ save_dir='log/resnet50-softmax-mars',
+ print_freq=10
+ )
+ """
+
+ def __init__(
+ self,
+ datamanager,
+ model,
+ optimizer,
+ scheduler=None,
+ use_gpu=True,
+ label_smooth=True,
+ pooling_method='avg'
+ ):
+ super(VideoSoftmaxEngine, self).__init__(
+ datamanager,
+ model,
+ optimizer,
+ scheduler=scheduler,
+ use_gpu=use_gpu,
+ label_smooth=label_smooth
+ )
+ self.pooling_method = pooling_method
+
+ def parse_data_for_train(self, data):
+ imgs = data['img']
+ pids = data['pid']
+ if imgs.dim() == 5:
+ # b: batch size
+ # s: sqeuence length
+ # c: channel depth
+ # h: height
+ # w: width
+ b, s, c, h, w = imgs.size()
+ imgs = imgs.view(b * s, c, h, w)
+ pids = pids.view(b, 1).expand(b, s)
+ pids = pids.contiguous().view(b * s)
+ return imgs, pids
+
+ def extract_features(self, input):
+ # b: batch size
+ # s: sqeuence length
+ # c: channel depth
+ # h: height
+ # w: width
+ b, s, c, h, w = input.size()
+ input = input.view(b * s, c, h, w)
+ features = self.model(input)
+ features = features.view(b, s, -1)
+ if self.pooling_method == 'avg':
+ features = torch.mean(features, 1)
+ else:
+ features = torch.max(features, 1)[0]
+ return features
diff --git a/trackers/strong_sort/deep/reid/torchreid/engine/video/triplet.py b/trackers/strong_sort/deep/reid/torchreid/engine/video/triplet.py
new file mode 100644
index 0000000000000000000000000000000000000000..b2778db9b3b1cd4c44e167735062c662884e3b52
--- /dev/null
+++ b/trackers/strong_sort/deep/reid/torchreid/engine/video/triplet.py
@@ -0,0 +1,122 @@
+from __future__ import division, print_function, absolute_import
+import torch
+
+from torchreid.engine.image import ImageTripletEngine
+
+
+class VideoTripletEngine(ImageTripletEngine):
+ """Triplet-loss engine for video-reid.
+
+ Args:
+ datamanager (DataManager): an instance of ``torchreid.data.ImageDataManager``
+ or ``torchreid.data.VideoDataManager``.
+ model (nn.Module): model instance.
+ optimizer (Optimizer): an Optimizer.
+ margin (float, optional): margin for triplet loss. Default is 0.3.
+ weight_t (float, optional): weight for triplet loss. Default is 1.
+ weight_x (float, optional): weight for softmax loss. Default is 1.
+ scheduler (LRScheduler, optional): if None, no learning rate decay will be performed.
+ use_gpu (bool, optional): use gpu. Default is True.
+ label_smooth (bool, optional): use label smoothing regularizer. Default is True.
+ pooling_method (str, optional): how to pool features for a tracklet.
+ Default is "avg" (average). Choices are ["avg", "max"].
+
+ Examples::
+
+ import torch
+ import torchreid
+ # Each batch contains batch_size*seq_len images
+ # Each identity is sampled with num_instances tracklets
+ datamanager = torchreid.data.VideoDataManager(
+ root='path/to/reid-data',
+ sources='mars',
+ height=256,
+ width=128,
+ combineall=False,
+ num_instances=4,
+ train_sampler='RandomIdentitySampler'
+ batch_size=8, # number of tracklets
+ seq_len=15 # number of images in each tracklet
+ )
+ model = torchreid.models.build_model(
+ name='resnet50',
+ num_classes=datamanager.num_train_pids,
+ loss='triplet'
+ )
+ model = model.cuda()
+ optimizer = torchreid.optim.build_optimizer(
+ model, optim='adam', lr=0.0003
+ )
+ scheduler = torchreid.optim.build_lr_scheduler(
+ optimizer,
+ lr_scheduler='single_step',
+ stepsize=20
+ )
+ engine = torchreid.engine.VideoTripletEngine(
+ datamanager, model, optimizer, margin=0.3,
+ weight_t=0.7, weight_x=1, scheduler=scheduler,
+ pooling_method='avg'
+ )
+ engine.run(
+ max_epoch=60,
+ save_dir='log/resnet50-triplet-mars',
+ print_freq=10
+ )
+ """
+
+ def __init__(
+ self,
+ datamanager,
+ model,
+ optimizer,
+ margin=0.3,
+ weight_t=1,
+ weight_x=1,
+ scheduler=None,
+ use_gpu=True,
+ label_smooth=True,
+ pooling_method='avg'
+ ):
+ super(VideoTripletEngine, self).__init__(
+ datamanager,
+ model,
+ optimizer,
+ margin=margin,
+ weight_t=weight_t,
+ weight_x=weight_x,
+ scheduler=scheduler,
+ use_gpu=use_gpu,
+ label_smooth=label_smooth
+ )
+ self.pooling_method = pooling_method
+
+ def parse_data_for_train(self, data):
+ imgs = data['img']
+ pids = data['pid']
+ if imgs.dim() == 5:
+ # b: batch size
+ # s: sqeuence length
+ # c: channel depth
+ # h: height
+ # w: width
+ b, s, c, h, w = imgs.size()
+ imgs = imgs.view(b * s, c, h, w)
+ pids = pids.view(b, 1).expand(b, s)
+ pids = pids.contiguous().view(b * s)
+ return imgs, pids
+
+ def extract_features(self, input):
+ # b: batch size
+ # s: sqeuence length
+ # c: channel depth
+ # h: height
+ # w: width
+ b, s, c, h, w = input.size()
+ input = input.view(b * s, c, h, w)
+ features = self.model(input)
+ features = features.view(b, s, -1)
+ if self.pooling_method == 'avg':
+ features = torch.mean(features, 1)
+ else:
+ features = torch.max(features, 1)[0]
+ return features
diff --git a/trackers/strong_sort/deep/reid/torchreid/losses/__init__.py b/trackers/strong_sort/deep/reid/torchreid/losses/__init__.py
new file mode 100644
index 0000000000000000000000000000000000000000..16257499c736c9f7c9ee46abd2f75c24ef10370b
--- /dev/null
+++ b/trackers/strong_sort/deep/reid/torchreid/losses/__init__.py
@@ -0,0 +1,21 @@
+from __future__ import division, print_function, absolute_import
+
+from .cross_entropy_loss import CrossEntropyLoss
+from .hard_mine_triplet_loss import TripletLoss
+
+
+def DeepSupervision(criterion, xs, y):
+ """DeepSupervision
+
+ Applies criterion to each element in a list.
+
+ Args:
+ criterion: loss function
+ xs: tuple of inputs
+ y: ground truth
+ """
+ loss = 0.
+ for x in xs:
+ loss += criterion(x, y)
+ loss /= len(xs)
+ return loss
diff --git a/trackers/strong_sort/deep/reid/torchreid/losses/cross_entropy_loss.py b/trackers/strong_sort/deep/reid/torchreid/losses/cross_entropy_loss.py
new file mode 100644
index 0000000000000000000000000000000000000000..4cfa5d46e41b7c7d11b95a8bd62c04903981d0c0
--- /dev/null
+++ b/trackers/strong_sort/deep/reid/torchreid/losses/cross_entropy_loss.py
@@ -0,0 +1,50 @@
+from __future__ import division, absolute_import
+import torch
+import torch.nn as nn
+
+
+class CrossEntropyLoss(nn.Module):
+ r"""Cross entropy loss with label smoothing regularizer.
+
+ Reference:
+ Szegedy et al. Rethinking the Inception Architecture for Computer Vision. CVPR 2016.
+
+ With label smoothing, the label :math:`y` for a class is computed by
+
+ .. math::
+ \begin{equation}
+ (1 - \eps) \times y + \frac{\eps}{K},
+ \end{equation}
+
+ where :math:`K` denotes the number of classes and :math:`\eps` is a weight. When
+ :math:`\eps = 0`, the loss function reduces to the normal cross entropy.
+
+ Args:
+ num_classes (int): number of classes.
+ eps (float, optional): weight. Default is 0.1.
+ use_gpu (bool, optional): whether to use gpu devices. Default is True.
+ label_smooth (bool, optional): whether to apply label smoothing. Default is True.
+ """
+
+ def __init__(self, num_classes, eps=0.1, use_gpu=True, label_smooth=True):
+ super(CrossEntropyLoss, self).__init__()
+ self.num_classes = num_classes
+ self.eps = eps if label_smooth else 0
+ self.use_gpu = use_gpu
+ self.logsoftmax = nn.LogSoftmax(dim=1)
+
+ def forward(self, inputs, targets):
+ """
+ Args:
+ inputs (torch.Tensor): prediction matrix (before softmax) with
+ shape (batch_size, num_classes).
+ targets (torch.LongTensor): ground truth labels with shape (batch_size).
+ Each position contains the label index.
+ """
+ log_probs = self.logsoftmax(inputs)
+ zeros = torch.zeros(log_probs.size())
+ targets = zeros.scatter_(1, targets.unsqueeze(1).data.cpu(), 1)
+ if self.use_gpu:
+ targets = targets.cuda()
+ targets = (1 - self.eps) * targets + self.eps / self.num_classes
+ return (-targets * log_probs).mean(0).sum()
diff --git a/trackers/strong_sort/deep/reid/torchreid/losses/hard_mine_triplet_loss.py b/trackers/strong_sort/deep/reid/torchreid/losses/hard_mine_triplet_loss.py
new file mode 100644
index 0000000000000000000000000000000000000000..ef9019bdcc19015b690b2b4b48674edd20c5b949
--- /dev/null
+++ b/trackers/strong_sort/deep/reid/torchreid/losses/hard_mine_triplet_loss.py
@@ -0,0 +1,48 @@
+from __future__ import division, absolute_import
+import torch
+import torch.nn as nn
+
+
+class TripletLoss(nn.Module):
+ """Triplet loss with hard positive/negative mining.
+
+ Reference:
+ Hermans et al. In Defense of the Triplet Loss for Person Re-Identification. arXiv:1703.07737.
+
+ Imported from ``_.
+
+ Args:
+ margin (float, optional): margin for triplet. Default is 0.3.
+ """
+
+ def __init__(self, margin=0.3):
+ super(TripletLoss, self).__init__()
+ self.margin = margin
+ self.ranking_loss = nn.MarginRankingLoss(margin=margin)
+
+ def forward(self, inputs, targets):
+ """
+ Args:
+ inputs (torch.Tensor): feature matrix with shape (batch_size, feat_dim).
+ targets (torch.LongTensor): ground truth labels with shape (num_classes).
+ """
+ n = inputs.size(0)
+
+ # Compute pairwise distance, replace by the official when merged
+ dist = torch.pow(inputs, 2).sum(dim=1, keepdim=True).expand(n, n)
+ dist = dist + dist.t()
+ dist.addmm_(inputs, inputs.t(), beta=1, alpha=-2)
+ dist = dist.clamp(min=1e-12).sqrt() # for numerical stability
+
+ # For each anchor, find the hardest positive and negative
+ mask = targets.expand(n, n).eq(targets.expand(n, n).t())
+ dist_ap, dist_an = [], []
+ for i in range(n):
+ dist_ap.append(dist[i][mask[i]].max().unsqueeze(0))
+ dist_an.append(dist[i][mask[i] == 0].min().unsqueeze(0))
+ dist_ap = torch.cat(dist_ap)
+ dist_an = torch.cat(dist_an)
+
+ # Compute ranking hinge loss
+ y = torch.ones_like(dist_an)
+ return self.ranking_loss(dist_an, dist_ap, y)
diff --git a/trackers/strong_sort/deep/reid/torchreid/metrics/__init__.py b/trackers/strong_sort/deep/reid/torchreid/metrics/__init__.py
new file mode 100644
index 0000000000000000000000000000000000000000..5159e1e9aa07d972d2a07ef00feac341349c66b8
--- /dev/null
+++ b/trackers/strong_sort/deep/reid/torchreid/metrics/__init__.py
@@ -0,0 +1,5 @@
+from __future__ import absolute_import
+
+from .rank import evaluate_rank
+from .accuracy import accuracy
+from .distance import compute_distance_matrix
diff --git a/trackers/strong_sort/deep/reid/torchreid/metrics/accuracy.py b/trackers/strong_sort/deep/reid/torchreid/metrics/accuracy.py
new file mode 100644
index 0000000000000000000000000000000000000000..3161f7bff08acbd8f598bc1712e693009feb60c9
--- /dev/null
+++ b/trackers/strong_sort/deep/reid/torchreid/metrics/accuracy.py
@@ -0,0 +1,37 @@
+from __future__ import division, print_function, absolute_import
+
+
+def accuracy(output, target, topk=(1, )):
+ """Computes the accuracy over the k top predictions for
+ the specified values of k.
+
+ Args:
+ output (torch.Tensor): prediction matrix with shape (batch_size, num_classes).
+ target (torch.LongTensor): ground truth labels with shape (batch_size).
+ topk (tuple, optional): accuracy at top-k will be computed. For example,
+ topk=(1, 5) means accuracy at top-1 and top-5 will be computed.
+
+ Returns:
+ list: accuracy at top-k.
+
+ Examples::
+ >>> from torchreid import metrics
+ >>> metrics.accuracy(output, target)
+ """
+ maxk = max(topk)
+ batch_size = target.size(0)
+
+ if isinstance(output, (tuple, list)):
+ output = output[0]
+
+ _, pred = output.topk(maxk, 1, True, True)
+ pred = pred.t()
+ correct = pred.eq(target.view(1, -1).expand_as(pred))
+
+ res = []
+ for k in topk:
+ correct_k = correct[:k].view(-1).float().sum(0, keepdim=True)
+ acc = correct_k.mul_(100.0 / batch_size)
+ res.append(acc)
+
+ return res
diff --git a/trackers/strong_sort/deep/reid/torchreid/metrics/distance.py b/trackers/strong_sort/deep/reid/torchreid/metrics/distance.py
new file mode 100644
index 0000000000000000000000000000000000000000..f4fb38316c2af8ec13a5cf734eec64734ddf715f
--- /dev/null
+++ b/trackers/strong_sort/deep/reid/torchreid/metrics/distance.py
@@ -0,0 +1,80 @@
+from __future__ import division, print_function, absolute_import
+import torch
+from torch.nn import functional as F
+
+
+def compute_distance_matrix(input1, input2, metric='euclidean'):
+ """A wrapper function for computing distance matrix.
+
+ Args:
+ input1 (torch.Tensor): 2-D feature matrix.
+ input2 (torch.Tensor): 2-D feature matrix.
+ metric (str, optional): "euclidean" or "cosine".
+ Default is "euclidean".
+
+ Returns:
+ torch.Tensor: distance matrix.
+
+ Examples::
+ >>> from torchreid import metrics
+ >>> input1 = torch.rand(10, 2048)
+ >>> input2 = torch.rand(100, 2048)
+ >>> distmat = metrics.compute_distance_matrix(input1, input2)
+ >>> distmat.size() # (10, 100)
+ """
+ # check input
+ assert isinstance(input1, torch.Tensor)
+ assert isinstance(input2, torch.Tensor)
+ assert input1.dim() == 2, 'Expected 2-D tensor, but got {}-D'.format(
+ input1.dim()
+ )
+ assert input2.dim() == 2, 'Expected 2-D tensor, but got {}-D'.format(
+ input2.dim()
+ )
+ assert input1.size(1) == input2.size(1)
+
+ if metric == 'euclidean':
+ distmat = euclidean_squared_distance(input1, input2)
+ elif metric == 'cosine':
+ distmat = cosine_distance(input1, input2)
+ else:
+ raise ValueError(
+ 'Unknown distance metric: {}. '
+ 'Please choose either "euclidean" or "cosine"'.format(metric)
+ )
+
+ return distmat
+
+
+def euclidean_squared_distance(input1, input2):
+ """Computes euclidean squared distance.
+
+ Args:
+ input1 (torch.Tensor): 2-D feature matrix.
+ input2 (torch.Tensor): 2-D feature matrix.
+
+ Returns:
+ torch.Tensor: distance matrix.
+ """
+ m, n = input1.size(0), input2.size(0)
+ mat1 = torch.pow(input1, 2).sum(dim=1, keepdim=True).expand(m, n)
+ mat2 = torch.pow(input2, 2).sum(dim=1, keepdim=True).expand(n, m).t()
+ distmat = mat1 + mat2
+ distmat.addmm_(input1, input2.t(), beta=1, alpha=-2)
+ return distmat
+
+
+def cosine_distance(input1, input2):
+ """Computes cosine distance.
+
+ Args:
+ input1 (torch.Tensor): 2-D feature matrix.
+ input2 (torch.Tensor): 2-D feature matrix.
+
+ Returns:
+ torch.Tensor: distance matrix.
+ """
+ input1_normed = F.normalize(input1, p=2, dim=1)
+ input2_normed = F.normalize(input2, p=2, dim=1)
+ distmat = 1 - torch.mm(input1_normed, input2_normed.t())
+ return distmat
diff --git a/trackers/strong_sort/deep/reid/torchreid/metrics/rank.py b/trackers/strong_sort/deep/reid/torchreid/metrics/rank.py
new file mode 100644
index 0000000000000000000000000000000000000000..bf6205b32807fcbe4ea658f28705fdc821b22feb
--- /dev/null
+++ b/trackers/strong_sort/deep/reid/torchreid/metrics/rank.py
@@ -0,0 +1,207 @@
+from __future__ import division, print_function, absolute_import
+import numpy as np
+import warnings
+from collections import defaultdict
+
+try:
+ from torchreid.metrics.rank_cylib.rank_cy import evaluate_cy
+ IS_CYTHON_AVAI = True
+except ImportError:
+ IS_CYTHON_AVAI = False
+ warnings.warn(
+ 'Cython evaluation (very fast so highly recommended) is '
+ 'unavailable, now use python evaluation.'
+ )
+
+
+def eval_cuhk03(distmat, q_pids, g_pids, q_camids, g_camids, max_rank):
+ """Evaluation with cuhk03 metric
+ Key: one image for each gallery identity is randomly sampled for each query identity.
+ Random sampling is performed num_repeats times.
+ """
+ num_repeats = 10
+ num_q, num_g = distmat.shape
+
+ if num_g < max_rank:
+ max_rank = num_g
+ print(
+ 'Note: number of gallery samples is quite small, got {}'.
+ format(num_g)
+ )
+
+ indices = np.argsort(distmat, axis=1)
+ matches = (g_pids[indices] == q_pids[:, np.newaxis]).astype(np.int32)
+
+ # compute cmc curve for each query
+ all_cmc = []
+ all_AP = []
+ num_valid_q = 0. # number of valid query
+
+ for q_idx in range(num_q):
+ # get query pid and camid
+ q_pid = q_pids[q_idx]
+ q_camid = q_camids[q_idx]
+
+ # remove gallery samples that have the same pid and camid with query
+ order = indices[q_idx]
+ remove = (g_pids[order] == q_pid) & (g_camids[order] == q_camid)
+ keep = np.invert(remove)
+
+ # compute cmc curve
+ raw_cmc = matches[q_idx][
+ keep] # binary vector, positions with value 1 are correct matches
+ if not np.any(raw_cmc):
+ # this condition is true when query identity does not appear in gallery
+ continue
+
+ kept_g_pids = g_pids[order][keep]
+ g_pids_dict = defaultdict(list)
+ for idx, pid in enumerate(kept_g_pids):
+ g_pids_dict[pid].append(idx)
+
+ cmc = 0.
+ for repeat_idx in range(num_repeats):
+ mask = np.zeros(len(raw_cmc), dtype=np.bool)
+ for _, idxs in g_pids_dict.items():
+ # randomly sample one image for each gallery person
+ rnd_idx = np.random.choice(idxs)
+ mask[rnd_idx] = True
+ masked_raw_cmc = raw_cmc[mask]
+ _cmc = masked_raw_cmc.cumsum()
+ _cmc[_cmc > 1] = 1
+ cmc += _cmc[:max_rank].astype(np.float32)
+
+ cmc /= num_repeats
+ all_cmc.append(cmc)
+ # compute AP
+ num_rel = raw_cmc.sum()
+ tmp_cmc = raw_cmc.cumsum()
+ tmp_cmc = [x / (i+1.) for i, x in enumerate(tmp_cmc)]
+ tmp_cmc = np.asarray(tmp_cmc) * raw_cmc
+ AP = tmp_cmc.sum() / num_rel
+ all_AP.append(AP)
+ num_valid_q += 1.
+
+ assert num_valid_q > 0, 'Error: all query identities do not appear in gallery'
+
+ all_cmc = np.asarray(all_cmc).astype(np.float32)
+ all_cmc = all_cmc.sum(0) / num_valid_q
+ mAP = np.mean(all_AP)
+
+ return all_cmc, mAP
+
+
+def eval_market1501(distmat, q_pids, g_pids, q_camids, g_camids, max_rank):
+ """Evaluation with market1501 metric
+ Key: for each query identity, its gallery images from the same camera view are discarded.
+ """
+ num_q, num_g = distmat.shape
+
+ if num_g < max_rank:
+ max_rank = num_g
+ print(
+ 'Note: number of gallery samples is quite small, got {}'.
+ format(num_g)
+ )
+
+ indices = np.argsort(distmat, axis=1)
+ matches = (g_pids[indices] == q_pids[:, np.newaxis]).astype(np.int32)
+
+ # compute cmc curve for each query
+ all_cmc = []
+ all_AP = []
+ num_valid_q = 0. # number of valid query
+
+ for q_idx in range(num_q):
+ # get query pid and camid
+ q_pid = q_pids[q_idx]
+ q_camid = q_camids[q_idx]
+
+ # remove gallery samples that have the same pid and camid with query
+ order = indices[q_idx]
+ remove = (g_pids[order] == q_pid) & (g_camids[order] == q_camid)
+ keep = np.invert(remove)
+
+ # compute cmc curve
+ raw_cmc = matches[q_idx][
+ keep] # binary vector, positions with value 1 are correct matches
+ if not np.any(raw_cmc):
+ # this condition is true when query identity does not appear in gallery
+ continue
+
+ cmc = raw_cmc.cumsum()
+ cmc[cmc > 1] = 1
+
+ all_cmc.append(cmc[:max_rank])
+ num_valid_q += 1.
+
+ # compute average precision
+ # reference: https://en.wikipedia.org/wiki/Evaluation_measures_(information_retrieval)#Average_precision
+ num_rel = raw_cmc.sum()
+ tmp_cmc = raw_cmc.cumsum()
+ tmp_cmc = [x / (i+1.) for i, x in enumerate(tmp_cmc)]
+ tmp_cmc = np.asarray(tmp_cmc) * raw_cmc
+ AP = tmp_cmc.sum() / num_rel
+ all_AP.append(AP)
+
+ assert num_valid_q > 0, 'Error: all query identities do not appear in gallery'
+
+ all_cmc = np.asarray(all_cmc).astype(np.float32)
+ all_cmc = all_cmc.sum(0) / num_valid_q
+ mAP = np.mean(all_AP)
+
+ return all_cmc, mAP
+
+
+def evaluate_py(
+ distmat, q_pids, g_pids, q_camids, g_camids, max_rank, use_metric_cuhk03
+):
+ if use_metric_cuhk03:
+ return eval_cuhk03(
+ distmat, q_pids, g_pids, q_camids, g_camids, max_rank
+ )
+ else:
+ return eval_market1501(
+ distmat, q_pids, g_pids, q_camids, g_camids, max_rank
+ )
+
+
+def evaluate_rank(
+ distmat,
+ q_pids,
+ g_pids,
+ q_camids,
+ g_camids,
+ max_rank=50,
+ use_metric_cuhk03=False,
+ use_cython=True
+):
+ """Evaluates CMC rank.
+
+ Args:
+ distmat (numpy.ndarray): distance matrix of shape (num_query, num_gallery).
+ q_pids (numpy.ndarray): 1-D array containing person identities
+ of each query instance.
+ g_pids (numpy.ndarray): 1-D array containing person identities
+ of each gallery instance.
+ q_camids (numpy.ndarray): 1-D array containing camera views under
+ which each query instance is captured.
+ g_camids (numpy.ndarray): 1-D array containing camera views under
+ which each gallery instance is captured.
+ max_rank (int, optional): maximum CMC rank to be computed. Default is 50.
+ use_metric_cuhk03 (bool, optional): use single-gallery-shot setting for cuhk03.
+ Default is False. This should be enabled when using cuhk03 classic split.
+ use_cython (bool, optional): use cython code for evaluation. Default is True.
+ This is highly recommended as the cython code can speed up the cmc computation
+ by more than 10x. This requires Cython to be installed.
+ """
+ if use_cython and IS_CYTHON_AVAI:
+ return evaluate_cy(
+ distmat, q_pids, g_pids, q_camids, g_camids, max_rank,
+ use_metric_cuhk03
+ )
+ else:
+ return evaluate_py(
+ distmat, q_pids, g_pids, q_camids, g_camids, max_rank,
+ use_metric_cuhk03
+ )
diff --git a/trackers/strong_sort/deep/reid/torchreid/metrics/rank_cylib/Makefile b/trackers/strong_sort/deep/reid/torchreid/metrics/rank_cylib/Makefile
new file mode 100644
index 0000000000000000000000000000000000000000..d49e655f85f829cb8ccda5bad6fe2c65cccf2bf2
--- /dev/null
+++ b/trackers/strong_sort/deep/reid/torchreid/metrics/rank_cylib/Makefile
@@ -0,0 +1,6 @@
+all:
+ $(PYTHON) setup.py build_ext --inplace
+ rm -rf build
+clean:
+ rm -rf build
+ rm -f rank_cy.c *.so
\ No newline at end of file
diff --git a/trackers/strong_sort/deep/reid/torchreid/metrics/rank_cylib/__init__.py b/trackers/strong_sort/deep/reid/torchreid/metrics/rank_cylib/__init__.py
new file mode 100644
index 0000000000000000000000000000000000000000..e69de29bb2d1d6434b8b29ae775ad8c2e48c5391
diff --git a/trackers/strong_sort/deep/reid/torchreid/metrics/rank_cylib/rank_cy.pyx b/trackers/strong_sort/deep/reid/torchreid/metrics/rank_cylib/rank_cy.pyx
new file mode 100644
index 0000000000000000000000000000000000000000..b4a8690e57fce4a286b49a7e822c1c6d014ba30d
--- /dev/null
+++ b/trackers/strong_sort/deep/reid/torchreid/metrics/rank_cylib/rank_cy.pyx
@@ -0,0 +1,251 @@
+# cython: boundscheck=False, wraparound=False, nonecheck=False, cdivision=True
+
+from __future__ import print_function
+import numpy as np
+from libc.stdint cimport int64_t, uint64_t
+
+import cython
+
+cimport numpy as np
+
+import random
+from collections import defaultdict
+
+"""
+Compiler directives:
+https://github.com/cython/cython/wiki/enhancements-compilerdirectives
+
+Cython tutorial:
+https://cython.readthedocs.io/en/latest/src/userguide/numpy_tutorial.html
+
+Credit to https://github.com/luzai
+"""
+
+
+# Main interface
+cpdef evaluate_cy(distmat, q_pids, g_pids, q_camids, g_camids, max_rank, use_metric_cuhk03=False):
+ distmat = np.asarray(distmat, dtype=np.float32)
+ q_pids = np.asarray(q_pids, dtype=np.int64)
+ g_pids = np.asarray(g_pids, dtype=np.int64)
+ q_camids = np.asarray(q_camids, dtype=np.int64)
+ g_camids = np.asarray(g_camids, dtype=np.int64)
+ if use_metric_cuhk03:
+ return eval_cuhk03_cy(distmat, q_pids, g_pids, q_camids, g_camids, max_rank)
+ return eval_market1501_cy(distmat, q_pids, g_pids, q_camids, g_camids, max_rank)
+
+
+cpdef eval_cuhk03_cy(float[:,:] distmat, int64_t[:] q_pids, int64_t[:]g_pids,
+ int64_t[:]q_camids, int64_t[:]g_camids, int64_t max_rank):
+
+ cdef int64_t num_q = distmat.shape[0]
+ cdef int64_t num_g = distmat.shape[1]
+
+ if num_g < max_rank:
+ max_rank = num_g
+ print('Note: number of gallery samples is quite small, got {}'.format(num_g))
+
+ cdef:
+ int64_t num_repeats = 10
+ int64_t[:,:] indices = np.argsort(distmat, axis=1)
+ int64_t[:,:] matches = (np.asarray(g_pids)[np.asarray(indices)] == np.asarray(q_pids)[:, np.newaxis]).astype(np.int64)
+
+ float[:,:] all_cmc = np.zeros((num_q, max_rank), dtype=np.float32)
+ float[:] all_AP = np.zeros(num_q, dtype=np.float32)
+ float num_valid_q = 0. # number of valid query
+
+ int64_t q_idx, q_pid, q_camid, g_idx
+ int64_t[:] order = np.zeros(num_g, dtype=np.int64)
+ int64_t keep
+
+ float[:] raw_cmc = np.zeros(num_g, dtype=np.float32) # binary vector, positions with value 1 are correct matches
+ float[:] masked_raw_cmc = np.zeros(num_g, dtype=np.float32)
+ float[:] cmc, masked_cmc
+ int64_t num_g_real, num_g_real_masked, rank_idx, rnd_idx
+ uint64_t meet_condition
+ float AP
+ int64_t[:] kept_g_pids, mask
+
+ float num_rel
+ float[:] tmp_cmc = np.zeros(num_g, dtype=np.float32)
+ float tmp_cmc_sum
+
+ for q_idx in range(num_q):
+ # get query pid and camid
+ q_pid = q_pids[q_idx]
+ q_camid = q_camids[q_idx]
+
+ # remove gallery samples that have the same pid and camid with query
+ for g_idx in range(num_g):
+ order[g_idx] = indices[q_idx, g_idx]
+ num_g_real = 0
+ meet_condition = 0
+ kept_g_pids = np.zeros(num_g, dtype=np.int64)
+
+ for g_idx in range(num_g):
+ if (g_pids[order[g_idx]] != q_pid) or (g_camids[order[g_idx]] != q_camid):
+ raw_cmc[num_g_real] = matches[q_idx][g_idx]
+ kept_g_pids[num_g_real] = g_pids[order[g_idx]]
+ num_g_real += 1
+ if matches[q_idx][g_idx] > 1e-31:
+ meet_condition = 1
+
+ if not meet_condition:
+ # this condition is true when query identity does not appear in gallery
+ continue
+
+ # cuhk03-specific setting
+ g_pids_dict = defaultdict(list) # overhead!
+ for g_idx in range(num_g_real):
+ g_pids_dict[kept_g_pids[g_idx]].append(g_idx)
+
+ cmc = np.zeros(max_rank, dtype=np.float32)
+ for _ in range(num_repeats):
+ mask = np.zeros(num_g_real, dtype=np.int64)
+
+ for _, idxs in g_pids_dict.items():
+ # randomly sample one image for each gallery person
+ rnd_idx = np.random.choice(idxs)
+ #rnd_idx = idxs[0] # use deterministic for debugging
+ mask[rnd_idx] = 1
+
+ num_g_real_masked = 0
+ for g_idx in range(num_g_real):
+ if mask[g_idx] == 1:
+ masked_raw_cmc[num_g_real_masked] = raw_cmc[g_idx]
+ num_g_real_masked += 1
+
+ masked_cmc = np.zeros(num_g, dtype=np.float32)
+ function_cumsum(masked_raw_cmc, masked_cmc, num_g_real_masked)
+ for g_idx in range(num_g_real_masked):
+ if masked_cmc[g_idx] > 1:
+ masked_cmc[g_idx] = 1
+
+ for rank_idx in range(max_rank):
+ cmc[rank_idx] += masked_cmc[rank_idx] / num_repeats
+
+ for rank_idx in range(max_rank):
+ all_cmc[q_idx, rank_idx] = cmc[rank_idx]
+ # compute average precision
+ # reference: https://en.wikipedia.org/wiki/Evaluation_measures_(information_retrieval)#Average_precision
+ function_cumsum(raw_cmc, tmp_cmc, num_g_real)
+ num_rel = 0
+ tmp_cmc_sum = 0
+ for g_idx in range(num_g_real):
+ tmp_cmc_sum += (tmp_cmc[g_idx] / (g_idx + 1.)) * raw_cmc[g_idx]
+ num_rel += raw_cmc[g_idx]
+ all_AP[q_idx] = tmp_cmc_sum / num_rel
+ num_valid_q += 1.
+
+ assert num_valid_q > 0, 'Error: all query identities do not appear in gallery'
+
+ # compute averaged cmc
+ cdef float[:] avg_cmc = np.zeros(max_rank, dtype=np.float32)
+ for rank_idx in range(max_rank):
+ for q_idx in range(num_q):
+ avg_cmc[rank_idx] += all_cmc[q_idx, rank_idx]
+ avg_cmc[rank_idx] /= num_valid_q
+
+ cdef float mAP = 0
+ for q_idx in range(num_q):
+ mAP += all_AP[q_idx]
+ mAP /= num_valid_q
+
+ return np.asarray(avg_cmc).astype(np.float32), mAP
+
+
+cpdef eval_market1501_cy(float[:,:] distmat, int64_t[:] q_pids, int64_t[:]g_pids,
+ int64_t[:]q_camids, int64_t[:]g_camids, int64_t max_rank):
+
+ cdef int64_t num_q = distmat.shape[0]
+ cdef int64_t num_g = distmat.shape[1]
+
+ if num_g < max_rank:
+ max_rank = num_g
+ print('Note: number of gallery samples is quite small, got {}'.format(num_g))
+
+ cdef:
+ int64_t[:,:] indices = np.argsort(distmat, axis=1)
+ int64_t[:,:] matches = (np.asarray(g_pids)[np.asarray(indices)] == np.asarray(q_pids)[:, np.newaxis]).astype(np.int64)
+
+ float[:,:] all_cmc = np.zeros((num_q, max_rank), dtype=np.float32)
+ float[:] all_AP = np.zeros(num_q, dtype=np.float32)
+ float num_valid_q = 0. # number of valid query
+
+ int64_t q_idx, q_pid, q_camid, g_idx
+ int64_t[:] order = np.zeros(num_g, dtype=np.int64)
+ int64_t keep
+
+ float[:] raw_cmc = np.zeros(num_g, dtype=np.float32) # binary vector, positions with value 1 are correct matches
+ float[:] cmc = np.zeros(num_g, dtype=np.float32)
+ int64_t num_g_real, rank_idx
+ uint64_t meet_condition
+
+ float num_rel
+ float[:] tmp_cmc = np.zeros(num_g, dtype=np.float32)
+ float tmp_cmc_sum
+
+ for q_idx in range(num_q):
+ # get query pid and camid
+ q_pid = q_pids[q_idx]
+ q_camid = q_camids[q_idx]
+
+ # remove gallery samples that have the same pid and camid with query
+ for g_idx in range(num_g):
+ order[g_idx] = indices[q_idx, g_idx]
+ num_g_real = 0
+ meet_condition = 0
+
+ for g_idx in range(num_g):
+ if (g_pids[order[g_idx]] != q_pid) or (g_camids[order[g_idx]] != q_camid):
+ raw_cmc[num_g_real] = matches[q_idx][g_idx]
+ num_g_real += 1
+ if matches[q_idx][g_idx] > 1e-31:
+ meet_condition = 1
+
+ if not meet_condition:
+ # this condition is true when query identity does not appear in gallery
+ continue
+
+ # compute cmc
+ function_cumsum(raw_cmc, cmc, num_g_real)
+ for g_idx in range(num_g_real):
+ if cmc[g_idx] > 1:
+ cmc[g_idx] = 1
+
+ for rank_idx in range(max_rank):
+ all_cmc[q_idx, rank_idx] = cmc[rank_idx]
+ num_valid_q += 1.
+
+ # compute average precision
+ # reference: https://en.wikipedia.org/wiki/Evaluation_measures_(information_retrieval)#Average_precision
+ function_cumsum(raw_cmc, tmp_cmc, num_g_real)
+ num_rel = 0
+ tmp_cmc_sum = 0
+ for g_idx in range(num_g_real):
+ tmp_cmc_sum += (tmp_cmc[g_idx] / (g_idx + 1.)) * raw_cmc[g_idx]
+ num_rel += raw_cmc[g_idx]
+ all_AP[q_idx] = tmp_cmc_sum / num_rel
+
+ assert num_valid_q > 0, 'Error: all query identities do not appear in gallery'
+
+ # compute averaged cmc
+ cdef float[:] avg_cmc = np.zeros(max_rank, dtype=np.float32)
+ for rank_idx in range(max_rank):
+ for q_idx in range(num_q):
+ avg_cmc[rank_idx] += all_cmc[q_idx, rank_idx]
+ avg_cmc[rank_idx] /= num_valid_q
+
+ cdef float mAP = 0
+ for q_idx in range(num_q):
+ mAP += all_AP[q_idx]
+ mAP /= num_valid_q
+
+ return np.asarray(avg_cmc).astype(np.float32), mAP
+
+
+# Compute the cumulative sum
+cdef void function_cumsum(cython.numeric[:] src, cython.numeric[:] dst, int64_t n):
+ cdef int64_t i
+ dst[0] = src[0]
+ for i in range(1, n):
+ dst[i] = src[i] + dst[i - 1]
\ No newline at end of file
diff --git a/trackers/strong_sort/deep/reid/torchreid/metrics/rank_cylib/setup.py b/trackers/strong_sort/deep/reid/torchreid/metrics/rank_cylib/setup.py
new file mode 100644
index 0000000000000000000000000000000000000000..ce2aeb7376092aa53ee32671a133bb57ee5941a2
--- /dev/null
+++ b/trackers/strong_sort/deep/reid/torchreid/metrics/rank_cylib/setup.py
@@ -0,0 +1,26 @@
+import numpy as np
+from distutils.core import setup
+from distutils.extension import Extension
+from Cython.Build import cythonize
+
+
+def numpy_include():
+ try:
+ numpy_include = np.get_include()
+ except AttributeError:
+ numpy_include = np.get_numpy_include()
+ return numpy_include
+
+
+ext_modules = [
+ Extension(
+ 'rank_cy',
+ ['rank_cy.pyx'],
+ include_dirs=[numpy_include()],
+ )
+]
+
+setup(
+ name='Cython-based reid evaluation code',
+ ext_modules=cythonize(ext_modules)
+)
diff --git a/trackers/strong_sort/deep/reid/torchreid/metrics/rank_cylib/test_cython.py b/trackers/strong_sort/deep/reid/torchreid/metrics/rank_cylib/test_cython.py
new file mode 100644
index 0000000000000000000000000000000000000000..5d1175d70bbc22e8c98fa8c5f89e2f5e88dc9c0f
--- /dev/null
+++ b/trackers/strong_sort/deep/reid/torchreid/metrics/rank_cylib/test_cython.py
@@ -0,0 +1,83 @@
+from __future__ import print_function
+import sys
+import numpy as np
+import timeit
+import os.path as osp
+
+from torchreid import metrics
+
+sys.path.insert(0, osp.dirname(osp.abspath(__file__)) + '/../../..')
+"""
+Test the speed of cython-based evaluation code. The speed improvements
+can be much bigger when using the real reid data, which contains a larger
+amount of query and gallery images.
+
+Note: you might encounter the following error:
+ 'AssertionError: Error: all query identities do not appear in gallery'.
+This is normal because the inputs are random numbers. Just try again.
+"""
+
+print('*** Compare running time ***')
+
+setup = '''
+import sys
+import os.path as osp
+import numpy as np
+sys.path.insert(0, osp.dirname(osp.abspath(__file__)) + '/../../..')
+from torchreid import metrics
+num_q = 30
+num_g = 300
+max_rank = 5
+distmat = np.random.rand(num_q, num_g) * 20
+q_pids = np.random.randint(0, num_q, size=num_q)
+g_pids = np.random.randint(0, num_g, size=num_g)
+q_camids = np.random.randint(0, 5, size=num_q)
+g_camids = np.random.randint(0, 5, size=num_g)
+'''
+
+print('=> Using market1501\'s metric')
+pytime = timeit.timeit(
+ 'metrics.evaluate_rank(distmat, q_pids, g_pids, q_camids, g_camids, max_rank, use_cython=False)',
+ setup=setup,
+ number=20
+)
+cytime = timeit.timeit(
+ 'metrics.evaluate_rank(distmat, q_pids, g_pids, q_camids, g_camids, max_rank, use_cython=True)',
+ setup=setup,
+ number=20
+)
+print('Python time: {} s'.format(pytime))
+print('Cython time: {} s'.format(cytime))
+print('Cython is {} times faster than python\n'.format(pytime / cytime))
+
+print('=> Using cuhk03\'s metric')
+pytime = timeit.timeit(
+ 'metrics.evaluate_rank(distmat, q_pids, g_pids, q_camids, g_camids, max_rank, use_metric_cuhk03=True, use_cython=False)',
+ setup=setup,
+ number=20
+)
+cytime = timeit.timeit(
+ 'metrics.evaluate_rank(distmat, q_pids, g_pids, q_camids, g_camids, max_rank, use_metric_cuhk03=True, use_cython=True)',
+ setup=setup,
+ number=20
+)
+print('Python time: {} s'.format(pytime))
+print('Cython time: {} s'.format(cytime))
+print('Cython is {} times faster than python\n'.format(pytime / cytime))
+"""
+print("=> Check precision")
+
+num_q = 30
+num_g = 300
+max_rank = 5
+distmat = np.random.rand(num_q, num_g) * 20
+q_pids = np.random.randint(0, num_q, size=num_q)
+g_pids = np.random.randint(0, num_g, size=num_g)
+q_camids = np.random.randint(0, 5, size=num_q)
+g_camids = np.random.randint(0, 5, size=num_g)
+
+cmc, mAP = evaluate(distmat, q_pids, g_pids, q_camids, g_camids, max_rank, use_cython=False)
+print("Python:\nmAP = {} \ncmc = {}\n".format(mAP, cmc))
+cmc, mAP = evaluate(distmat, q_pids, g_pids, q_camids, g_camids, max_rank, use_cython=True)
+print("Cython:\nmAP = {} \ncmc = {}\n".format(mAP, cmc))
+"""
diff --git a/trackers/strong_sort/deep/reid/torchreid/models/__init__.py b/trackers/strong_sort/deep/reid/torchreid/models/__init__.py
new file mode 100644
index 0000000000000000000000000000000000000000..3c60ba6f59ca7fa5ff9f3c6a4dcef1357c353dde
--- /dev/null
+++ b/trackers/strong_sort/deep/reid/torchreid/models/__init__.py
@@ -0,0 +1,122 @@
+from __future__ import absolute_import
+import torch
+
+from .pcb import *
+from .mlfn import *
+from .hacnn import *
+from .osnet import *
+from .senet import *
+from .mudeep import *
+from .nasnet import *
+from .resnet import *
+from .densenet import *
+from .xception import *
+from .osnet_ain import *
+from .resnetmid import *
+from .shufflenet import *
+from .squeezenet import *
+from .inceptionv4 import *
+from .mobilenetv2 import *
+from .resnet_ibn_a import *
+from .resnet_ibn_b import *
+from .shufflenetv2 import *
+from .inceptionresnetv2 import *
+
+__model_factory = {
+ # image classification models
+ 'resnet18': resnet18,
+ 'resnet34': resnet34,
+ 'resnet50': resnet50,
+ 'resnet101': resnet101,
+ 'resnet152': resnet152,
+ 'resnext50_32x4d': resnext50_32x4d,
+ 'resnext101_32x8d': resnext101_32x8d,
+ 'resnet50_fc512': resnet50_fc512,
+ 'se_resnet50': se_resnet50,
+ 'se_resnet50_fc512': se_resnet50_fc512,
+ 'se_resnet101': se_resnet101,
+ 'se_resnext50_32x4d': se_resnext50_32x4d,
+ 'se_resnext101_32x4d': se_resnext101_32x4d,
+ 'densenet121': densenet121,
+ 'densenet169': densenet169,
+ 'densenet201': densenet201,
+ 'densenet161': densenet161,
+ 'densenet121_fc512': densenet121_fc512,
+ 'inceptionresnetv2': inceptionresnetv2,
+ 'inceptionv4': inceptionv4,
+ 'xception': xception,
+ 'resnet50_ibn_a': resnet50_ibn_a,
+ 'resnet50_ibn_b': resnet50_ibn_b,
+ # lightweight models
+ 'nasnsetmobile': nasnetamobile,
+ 'mobilenetv2_x1_0': mobilenetv2_x1_0,
+ 'mobilenetv2_x1_4': mobilenetv2_x1_4,
+ 'shufflenet': shufflenet,
+ 'squeezenet1_0': squeezenet1_0,
+ 'squeezenet1_0_fc512': squeezenet1_0_fc512,
+ 'squeezenet1_1': squeezenet1_1,
+ 'shufflenet_v2_x0_5': shufflenet_v2_x0_5,
+ 'shufflenet_v2_x1_0': shufflenet_v2_x1_0,
+ 'shufflenet_v2_x1_5': shufflenet_v2_x1_5,
+ 'shufflenet_v2_x2_0': shufflenet_v2_x2_0,
+ # reid-specific models
+ 'mudeep': MuDeep,
+ 'resnet50mid': resnet50mid,
+ 'hacnn': HACNN,
+ 'pcb_p6': pcb_p6,
+ 'pcb_p4': pcb_p4,
+ 'mlfn': mlfn,
+ 'osnet_x1_0': osnet_x1_0,
+ 'osnet_x0_75': osnet_x0_75,
+ 'osnet_x0_5': osnet_x0_5,
+ 'osnet_x0_25': osnet_x0_25,
+ 'osnet_ibn_x1_0': osnet_ibn_x1_0,
+ 'osnet_ain_x1_0': osnet_ain_x1_0,
+ 'osnet_ain_x0_75': osnet_ain_x0_75,
+ 'osnet_ain_x0_5': osnet_ain_x0_5,
+ 'osnet_ain_x0_25': osnet_ain_x0_25
+}
+
+
+def show_avai_models():
+ """Displays available models.
+
+ Examples::
+ >>> from torchreid import models
+ >>> models.show_avai_models()
+ """
+ print(list(__model_factory.keys()))
+
+
+def build_model(
+ name, num_classes, loss='softmax', pretrained=True, use_gpu=True
+):
+ """A function wrapper for building a model.
+
+ Args:
+ name (str): model name.
+ num_classes (int): number of training identities.
+ loss (str, optional): loss function to optimize the model. Currently
+ supports "softmax" and "triplet". Default is "softmax".
+ pretrained (bool, optional): whether to load ImageNet-pretrained weights.
+ Default is True.
+ use_gpu (bool, optional): whether to use gpu. Default is True.
+
+ Returns:
+ nn.Module
+
+ Examples::
+ >>> from torchreid import models
+ >>> model = models.build_model('resnet50', 751, loss='softmax')
+ """
+ avai_models = list(__model_factory.keys())
+ if name not in avai_models:
+ raise KeyError(
+ 'Unknown model: {}. Must be one of {}'.format(name, avai_models)
+ )
+ return __model_factory[name](
+ num_classes=num_classes,
+ loss=loss,
+ pretrained=pretrained,
+ use_gpu=use_gpu
+ )
diff --git a/trackers/strong_sort/deep/reid/torchreid/models/densenet.py b/trackers/strong_sort/deep/reid/torchreid/models/densenet.py
new file mode 100644
index 0000000000000000000000000000000000000000..a1d9b7ef85a79cbc4c4e8a81840935531df636b8
--- /dev/null
+++ b/trackers/strong_sort/deep/reid/torchreid/models/densenet.py
@@ -0,0 +1,380 @@
+"""
+Code source: https://github.com/pytorch/vision
+"""
+from __future__ import division, absolute_import
+import re
+from collections import OrderedDict
+import torch
+import torch.nn as nn
+from torch.nn import functional as F
+from torch.utils import model_zoo
+
+__all__ = [
+ 'densenet121', 'densenet169', 'densenet201', 'densenet161',
+ 'densenet121_fc512'
+]
+
+model_urls = {
+ 'densenet121':
+ 'https://download.pytorch.org/models/densenet121-a639ec97.pth',
+ 'densenet169':
+ 'https://download.pytorch.org/models/densenet169-b2777c0a.pth',
+ 'densenet201':
+ 'https://download.pytorch.org/models/densenet201-c1103571.pth',
+ 'densenet161':
+ 'https://download.pytorch.org/models/densenet161-8d451a50.pth',
+}
+
+
+class _DenseLayer(nn.Sequential):
+
+ def __init__(self, num_input_features, growth_rate, bn_size, drop_rate):
+ super(_DenseLayer, self).__init__()
+ self.add_module('norm1', nn.BatchNorm2d(num_input_features)),
+ self.add_module('relu1', nn.ReLU(inplace=True)),
+ self.add_module(
+ 'conv1',
+ nn.Conv2d(
+ num_input_features,
+ bn_size * growth_rate,
+ kernel_size=1,
+ stride=1,
+ bias=False
+ )
+ ),
+ self.add_module('norm2', nn.BatchNorm2d(bn_size * growth_rate)),
+ self.add_module('relu2', nn.ReLU(inplace=True)),
+ self.add_module(
+ 'conv2',
+ nn.Conv2d(
+ bn_size * growth_rate,
+ growth_rate,
+ kernel_size=3,
+ stride=1,
+ padding=1,
+ bias=False
+ )
+ ),
+ self.drop_rate = drop_rate
+
+ def forward(self, x):
+ new_features = super(_DenseLayer, self).forward(x)
+ if self.drop_rate > 0:
+ new_features = F.dropout(
+ new_features, p=self.drop_rate, training=self.training
+ )
+ return torch.cat([x, new_features], 1)
+
+
+class _DenseBlock(nn.Sequential):
+
+ def __init__(
+ self, num_layers, num_input_features, bn_size, growth_rate, drop_rate
+ ):
+ super(_DenseBlock, self).__init__()
+ for i in range(num_layers):
+ layer = _DenseLayer(
+ num_input_features + i*growth_rate, growth_rate, bn_size,
+ drop_rate
+ )
+ self.add_module('denselayer%d' % (i+1), layer)
+
+
+class _Transition(nn.Sequential):
+
+ def __init__(self, num_input_features, num_output_features):
+ super(_Transition, self).__init__()
+ self.add_module('norm', nn.BatchNorm2d(num_input_features))
+ self.add_module('relu', nn.ReLU(inplace=True))
+ self.add_module(
+ 'conv',
+ nn.Conv2d(
+ num_input_features,
+ num_output_features,
+ kernel_size=1,
+ stride=1,
+ bias=False
+ )
+ )
+ self.add_module('pool', nn.AvgPool2d(kernel_size=2, stride=2))
+
+
+class DenseNet(nn.Module):
+ """Densely connected network.
+
+ Reference:
+ Huang et al. Densely Connected Convolutional Networks. CVPR 2017.
+
+ Public keys:
+ - ``densenet121``: DenseNet121.
+ - ``densenet169``: DenseNet169.
+ - ``densenet201``: DenseNet201.
+ - ``densenet161``: DenseNet161.
+ - ``densenet121_fc512``: DenseNet121 + FC.
+ """
+
+ def __init__(
+ self,
+ num_classes,
+ loss,
+ growth_rate=32,
+ block_config=(6, 12, 24, 16),
+ num_init_features=64,
+ bn_size=4,
+ drop_rate=0,
+ fc_dims=None,
+ dropout_p=None,
+ **kwargs
+ ):
+
+ super(DenseNet, self).__init__()
+ self.loss = loss
+
+ # First convolution
+ self.features = nn.Sequential(
+ OrderedDict(
+ [
+ (
+ 'conv0',
+ nn.Conv2d(
+ 3,
+ num_init_features,
+ kernel_size=7,
+ stride=2,
+ padding=3,
+ bias=False
+ )
+ ),
+ ('norm0', nn.BatchNorm2d(num_init_features)),
+ ('relu0', nn.ReLU(inplace=True)),
+ (
+ 'pool0',
+ nn.MaxPool2d(kernel_size=3, stride=2, padding=1)
+ ),
+ ]
+ )
+ )
+
+ # Each denseblock
+ num_features = num_init_features
+ for i, num_layers in enumerate(block_config):
+ block = _DenseBlock(
+ num_layers=num_layers,
+ num_input_features=num_features,
+ bn_size=bn_size,
+ growth_rate=growth_rate,
+ drop_rate=drop_rate
+ )
+ self.features.add_module('denseblock%d' % (i+1), block)
+ num_features = num_features + num_layers*growth_rate
+ if i != len(block_config) - 1:
+ trans = _Transition(
+ num_input_features=num_features,
+ num_output_features=num_features // 2
+ )
+ self.features.add_module('transition%d' % (i+1), trans)
+ num_features = num_features // 2
+
+ # Final batch norm
+ self.features.add_module('norm5', nn.BatchNorm2d(num_features))
+
+ self.global_avgpool = nn.AdaptiveAvgPool2d(1)
+ self.feature_dim = num_features
+ self.fc = self._construct_fc_layer(fc_dims, num_features, dropout_p)
+
+ # Linear layer
+ self.classifier = nn.Linear(self.feature_dim, num_classes)
+
+ self._init_params()
+
+ def _construct_fc_layer(self, fc_dims, input_dim, dropout_p=None):
+ """Constructs fully connected layer.
+
+ Args:
+ fc_dims (list or tuple): dimensions of fc layers, if None, no fc layers are constructed
+ input_dim (int): input dimension
+ dropout_p (float): dropout probability, if None, dropout is unused
+ """
+ if fc_dims is None:
+ self.feature_dim = input_dim
+ return None
+
+ assert isinstance(
+ fc_dims, (list, tuple)
+ ), 'fc_dims must be either list or tuple, but got {}'.format(
+ type(fc_dims)
+ )
+
+ layers = []
+ for dim in fc_dims:
+ layers.append(nn.Linear(input_dim, dim))
+ layers.append(nn.BatchNorm1d(dim))
+ layers.append(nn.ReLU(inplace=True))
+ if dropout_p is not None:
+ layers.append(nn.Dropout(p=dropout_p))
+ input_dim = dim
+
+ self.feature_dim = fc_dims[-1]
+
+ return nn.Sequential(*layers)
+
+ def _init_params(self):
+ for m in self.modules():
+ if isinstance(m, nn.Conv2d):
+ nn.init.kaiming_normal_(
+ m.weight, mode='fan_out', nonlinearity='relu'
+ )
+ if m.bias is not None:
+ nn.init.constant_(m.bias, 0)
+ elif isinstance(m, nn.BatchNorm2d):
+ nn.init.constant_(m.weight, 1)
+ nn.init.constant_(m.bias, 0)
+ elif isinstance(m, nn.BatchNorm1d):
+ nn.init.constant_(m.weight, 1)
+ nn.init.constant_(m.bias, 0)
+ elif isinstance(m, nn.Linear):
+ nn.init.normal_(m.weight, 0, 0.01)
+ if m.bias is not None:
+ nn.init.constant_(m.bias, 0)
+
+ def forward(self, x):
+ f = self.features(x)
+ f = F.relu(f, inplace=True)
+ v = self.global_avgpool(f)
+ v = v.view(v.size(0), -1)
+
+ if self.fc is not None:
+ v = self.fc(v)
+
+ if not self.training:
+ return v
+
+ y = self.classifier(v)
+
+ if self.loss == 'softmax':
+ return y
+ elif self.loss == 'triplet':
+ return y, v
+ else:
+ raise KeyError('Unsupported loss: {}'.format(self.loss))
+
+
+def init_pretrained_weights(model, model_url):
+ """Initializes model with pretrained weights.
+
+ Layers that don't match with pretrained layers in name or size are kept unchanged.
+ """
+ pretrain_dict = model_zoo.load_url(model_url)
+
+ # '.'s are no longer allowed in module names, but pervious _DenseLayer
+ # has keys 'norm.1', 'relu.1', 'conv.1', 'norm.2', 'relu.2', 'conv.2'.
+ # They are also in the checkpoints in model_urls. This pattern is used
+ # to find such keys.
+ pattern = re.compile(
+ r'^(.*denselayer\d+\.(?:norm|relu|conv))\.((?:[12])\.(?:weight|bias|running_mean|running_var))$'
+ )
+ for key in list(pretrain_dict.keys()):
+ res = pattern.match(key)
+ if res:
+ new_key = res.group(1) + res.group(2)
+ pretrain_dict[new_key] = pretrain_dict[key]
+ del pretrain_dict[key]
+
+ model_dict = model.state_dict()
+ pretrain_dict = {
+ k: v
+ for k, v in pretrain_dict.items()
+ if k in model_dict and model_dict[k].size() == v.size()
+ }
+ model_dict.update(pretrain_dict)
+ model.load_state_dict(model_dict)
+
+
+"""
+Dense network configurations:
+--
+densenet121: num_init_features=64, growth_rate=32, block_config=(6, 12, 24, 16)
+densenet169: num_init_features=64, growth_rate=32, block_config=(6, 12, 32, 32)
+densenet201: num_init_features=64, growth_rate=32, block_config=(6, 12, 48, 32)
+densenet161: num_init_features=96, growth_rate=48, block_config=(6, 12, 36, 24)
+"""
+
+
+def densenet121(num_classes, loss='softmax', pretrained=True, **kwargs):
+ model = DenseNet(
+ num_classes=num_classes,
+ loss=loss,
+ num_init_features=64,
+ growth_rate=32,
+ block_config=(6, 12, 24, 16),
+ fc_dims=None,
+ dropout_p=None,
+ **kwargs
+ )
+ if pretrained:
+ init_pretrained_weights(model, model_urls['densenet121'])
+ return model
+
+
+def densenet169(num_classes, loss='softmax', pretrained=True, **kwargs):
+ model = DenseNet(
+ num_classes=num_classes,
+ loss=loss,
+ num_init_features=64,
+ growth_rate=32,
+ block_config=(6, 12, 32, 32),
+ fc_dims=None,
+ dropout_p=None,
+ **kwargs
+ )
+ if pretrained:
+ init_pretrained_weights(model, model_urls['densenet169'])
+ return model
+
+
+def densenet201(num_classes, loss='softmax', pretrained=True, **kwargs):
+ model = DenseNet(
+ num_classes=num_classes,
+ loss=loss,
+ num_init_features=64,
+ growth_rate=32,
+ block_config=(6, 12, 48, 32),
+ fc_dims=None,
+ dropout_p=None,
+ **kwargs
+ )
+ if pretrained:
+ init_pretrained_weights(model, model_urls['densenet201'])
+ return model
+
+
+def densenet161(num_classes, loss='softmax', pretrained=True, **kwargs):
+ model = DenseNet(
+ num_classes=num_classes,
+ loss=loss,
+ num_init_features=96,
+ growth_rate=48,
+ block_config=(6, 12, 36, 24),
+ fc_dims=None,
+ dropout_p=None,
+ **kwargs
+ )
+ if pretrained:
+ init_pretrained_weights(model, model_urls['densenet161'])
+ return model
+
+
+def densenet121_fc512(num_classes, loss='softmax', pretrained=True, **kwargs):
+ model = DenseNet(
+ num_classes=num_classes,
+ loss=loss,
+ num_init_features=64,
+ growth_rate=32,
+ block_config=(6, 12, 24, 16),
+ fc_dims=[512],
+ dropout_p=None,
+ **kwargs
+ )
+ if pretrained:
+ init_pretrained_weights(model, model_urls['densenet121'])
+ return model
diff --git a/trackers/strong_sort/deep/reid/torchreid/models/hacnn.py b/trackers/strong_sort/deep/reid/torchreid/models/hacnn.py
new file mode 100644
index 0000000000000000000000000000000000000000..f21cc82f42fe181317f9a0d89cdede95699f45a9
--- /dev/null
+++ b/trackers/strong_sort/deep/reid/torchreid/models/hacnn.py
@@ -0,0 +1,414 @@
+from __future__ import division, absolute_import
+import torch
+from torch import nn
+from torch.nn import functional as F
+
+__all__ = ['HACNN']
+
+
+class ConvBlock(nn.Module):
+ """Basic convolutional block.
+
+ convolution + batch normalization + relu.
+
+ Args:
+ in_c (int): number of input channels.
+ out_c (int): number of output channels.
+ k (int or tuple): kernel size.
+ s (int or tuple): stride.
+ p (int or tuple): padding.
+ """
+
+ def __init__(self, in_c, out_c, k, s=1, p=0):
+ super(ConvBlock, self).__init__()
+ self.conv = nn.Conv2d(in_c, out_c, k, stride=s, padding=p)
+ self.bn = nn.BatchNorm2d(out_c)
+
+ def forward(self, x):
+ return F.relu(self.bn(self.conv(x)))
+
+
+class InceptionA(nn.Module):
+
+ def __init__(self, in_channels, out_channels):
+ super(InceptionA, self).__init__()
+ mid_channels = out_channels // 4
+
+ self.stream1 = nn.Sequential(
+ ConvBlock(in_channels, mid_channels, 1),
+ ConvBlock(mid_channels, mid_channels, 3, p=1),
+ )
+ self.stream2 = nn.Sequential(
+ ConvBlock(in_channels, mid_channels, 1),
+ ConvBlock(mid_channels, mid_channels, 3, p=1),
+ )
+ self.stream3 = nn.Sequential(
+ ConvBlock(in_channels, mid_channels, 1),
+ ConvBlock(mid_channels, mid_channels, 3, p=1),
+ )
+ self.stream4 = nn.Sequential(
+ nn.AvgPool2d(3, stride=1, padding=1),
+ ConvBlock(in_channels, mid_channels, 1),
+ )
+
+ def forward(self, x):
+ s1 = self.stream1(x)
+ s2 = self.stream2(x)
+ s3 = self.stream3(x)
+ s4 = self.stream4(x)
+ y = torch.cat([s1, s2, s3, s4], dim=1)
+ return y
+
+
+class InceptionB(nn.Module):
+
+ def __init__(self, in_channels, out_channels):
+ super(InceptionB, self).__init__()
+ mid_channels = out_channels // 4
+
+ self.stream1 = nn.Sequential(
+ ConvBlock(in_channels, mid_channels, 1),
+ ConvBlock(mid_channels, mid_channels, 3, s=2, p=1),
+ )
+ self.stream2 = nn.Sequential(
+ ConvBlock(in_channels, mid_channels, 1),
+ ConvBlock(mid_channels, mid_channels, 3, p=1),
+ ConvBlock(mid_channels, mid_channels, 3, s=2, p=1),
+ )
+ self.stream3 = nn.Sequential(
+ nn.MaxPool2d(3, stride=2, padding=1),
+ ConvBlock(in_channels, mid_channels * 2, 1),
+ )
+
+ def forward(self, x):
+ s1 = self.stream1(x)
+ s2 = self.stream2(x)
+ s3 = self.stream3(x)
+ y = torch.cat([s1, s2, s3], dim=1)
+ return y
+
+
+class SpatialAttn(nn.Module):
+ """Spatial Attention (Sec. 3.1.I.1)"""
+
+ def __init__(self):
+ super(SpatialAttn, self).__init__()
+ self.conv1 = ConvBlock(1, 1, 3, s=2, p=1)
+ self.conv2 = ConvBlock(1, 1, 1)
+
+ def forward(self, x):
+ # global cross-channel averaging
+ x = x.mean(1, keepdim=True)
+ # 3-by-3 conv
+ x = self.conv1(x)
+ # bilinear resizing
+ x = F.upsample(
+ x, (x.size(2) * 2, x.size(3) * 2),
+ mode='bilinear',
+ align_corners=True
+ )
+ # scaling conv
+ x = self.conv2(x)
+ return x
+
+
+class ChannelAttn(nn.Module):
+ """Channel Attention (Sec. 3.1.I.2)"""
+
+ def __init__(self, in_channels, reduction_rate=16):
+ super(ChannelAttn, self).__init__()
+ assert in_channels % reduction_rate == 0
+ self.conv1 = ConvBlock(in_channels, in_channels // reduction_rate, 1)
+ self.conv2 = ConvBlock(in_channels // reduction_rate, in_channels, 1)
+
+ def forward(self, x):
+ # squeeze operation (global average pooling)
+ x = F.avg_pool2d(x, x.size()[2:])
+ # excitation operation (2 conv layers)
+ x = self.conv1(x)
+ x = self.conv2(x)
+ return x
+
+
+class SoftAttn(nn.Module):
+ """Soft Attention (Sec. 3.1.I)
+
+ Aim: Spatial Attention + Channel Attention
+
+ Output: attention maps with shape identical to input.
+ """
+
+ def __init__(self, in_channels):
+ super(SoftAttn, self).__init__()
+ self.spatial_attn = SpatialAttn()
+ self.channel_attn = ChannelAttn(in_channels)
+ self.conv = ConvBlock(in_channels, in_channels, 1)
+
+ def forward(self, x):
+ y_spatial = self.spatial_attn(x)
+ y_channel = self.channel_attn(x)
+ y = y_spatial * y_channel
+ y = torch.sigmoid(self.conv(y))
+ return y
+
+
+class HardAttn(nn.Module):
+ """Hard Attention (Sec. 3.1.II)"""
+
+ def __init__(self, in_channels):
+ super(HardAttn, self).__init__()
+ self.fc = nn.Linear(in_channels, 4 * 2)
+ self.init_params()
+
+ def init_params(self):
+ self.fc.weight.data.zero_()
+ self.fc.bias.data.copy_(
+ torch.tensor(
+ [0, -0.75, 0, -0.25, 0, 0.25, 0, 0.75], dtype=torch.float
+ )
+ )
+
+ def forward(self, x):
+ # squeeze operation (global average pooling)
+ x = F.avg_pool2d(x, x.size()[2:]).view(x.size(0), x.size(1))
+ # predict transformation parameters
+ theta = torch.tanh(self.fc(x))
+ theta = theta.view(-1, 4, 2)
+ return theta
+
+
+class HarmAttn(nn.Module):
+ """Harmonious Attention (Sec. 3.1)"""
+
+ def __init__(self, in_channels):
+ super(HarmAttn, self).__init__()
+ self.soft_attn = SoftAttn(in_channels)
+ self.hard_attn = HardAttn(in_channels)
+
+ def forward(self, x):
+ y_soft_attn = self.soft_attn(x)
+ theta = self.hard_attn(x)
+ return y_soft_attn, theta
+
+
+class HACNN(nn.Module):
+ """Harmonious Attention Convolutional Neural Network.
+
+ Reference:
+ Li et al. Harmonious Attention Network for Person Re-identification. CVPR 2018.
+
+ Public keys:
+ - ``hacnn``: HACNN.
+ """
+
+ # Args:
+ # num_classes (int): number of classes to predict
+ # nchannels (list): number of channels AFTER concatenation
+ # feat_dim (int): feature dimension for a single stream
+ # learn_region (bool): whether to learn region features (i.e. local branch)
+
+ def __init__(
+ self,
+ num_classes,
+ loss='softmax',
+ nchannels=[128, 256, 384],
+ feat_dim=512,
+ learn_region=True,
+ use_gpu=True,
+ **kwargs
+ ):
+ super(HACNN, self).__init__()
+ self.loss = loss
+ self.learn_region = learn_region
+ self.use_gpu = use_gpu
+
+ self.conv = ConvBlock(3, 32, 3, s=2, p=1)
+
+ # Construct Inception + HarmAttn blocks
+ # ============== Block 1 ==============
+ self.inception1 = nn.Sequential(
+ InceptionA(32, nchannels[0]),
+ InceptionB(nchannels[0], nchannels[0]),
+ )
+ self.ha1 = HarmAttn(nchannels[0])
+
+ # ============== Block 2 ==============
+ self.inception2 = nn.Sequential(
+ InceptionA(nchannels[0], nchannels[1]),
+ InceptionB(nchannels[1], nchannels[1]),
+ )
+ self.ha2 = HarmAttn(nchannels[1])
+
+ # ============== Block 3 ==============
+ self.inception3 = nn.Sequential(
+ InceptionA(nchannels[1], nchannels[2]),
+ InceptionB(nchannels[2], nchannels[2]),
+ )
+ self.ha3 = HarmAttn(nchannels[2])
+
+ self.fc_global = nn.Sequential(
+ nn.Linear(nchannels[2], feat_dim),
+ nn.BatchNorm1d(feat_dim),
+ nn.ReLU(),
+ )
+ self.classifier_global = nn.Linear(feat_dim, num_classes)
+
+ if self.learn_region:
+ self.init_scale_factors()
+ self.local_conv1 = InceptionB(32, nchannels[0])
+ self.local_conv2 = InceptionB(nchannels[0], nchannels[1])
+ self.local_conv3 = InceptionB(nchannels[1], nchannels[2])
+ self.fc_local = nn.Sequential(
+ nn.Linear(nchannels[2] * 4, feat_dim),
+ nn.BatchNorm1d(feat_dim),
+ nn.ReLU(),
+ )
+ self.classifier_local = nn.Linear(feat_dim, num_classes)
+ self.feat_dim = feat_dim * 2
+ else:
+ self.feat_dim = feat_dim
+
+ def init_scale_factors(self):
+ # initialize scale factors (s_w, s_h) for four regions
+ self.scale_factors = []
+ self.scale_factors.append(
+ torch.tensor([[1, 0], [0, 0.25]], dtype=torch.float)
+ )
+ self.scale_factors.append(
+ torch.tensor([[1, 0], [0, 0.25]], dtype=torch.float)
+ )
+ self.scale_factors.append(
+ torch.tensor([[1, 0], [0, 0.25]], dtype=torch.float)
+ )
+ self.scale_factors.append(
+ torch.tensor([[1, 0], [0, 0.25]], dtype=torch.float)
+ )
+
+ def stn(self, x, theta):
+ """Performs spatial transform
+
+ x: (batch, channel, height, width)
+ theta: (batch, 2, 3)
+ """
+ grid = F.affine_grid(theta, x.size())
+ x = F.grid_sample(x, grid)
+ return x
+
+ def transform_theta(self, theta_i, region_idx):
+ """Transforms theta to include (s_w, s_h), resulting in (batch, 2, 3)"""
+ scale_factors = self.scale_factors[region_idx]
+ theta = torch.zeros(theta_i.size(0), 2, 3)
+ theta[:, :, :2] = scale_factors
+ theta[:, :, -1] = theta_i
+ if self.use_gpu:
+ theta = theta.cuda()
+ return theta
+
+ def forward(self, x):
+ assert x.size(2) == 160 and x.size(3) == 64, \
+ 'Input size does not match, expected (160, 64) but got ({}, {})'.format(x.size(2), x.size(3))
+ x = self.conv(x)
+
+ # ============== Block 1 ==============
+ # global branch
+ x1 = self.inception1(x)
+ x1_attn, x1_theta = self.ha1(x1)
+ x1_out = x1 * x1_attn
+ # local branch
+ if self.learn_region:
+ x1_local_list = []
+ for region_idx in range(4):
+ x1_theta_i = x1_theta[:, region_idx, :]
+ x1_theta_i = self.transform_theta(x1_theta_i, region_idx)
+ x1_trans_i = self.stn(x, x1_theta_i)
+ x1_trans_i = F.upsample(
+ x1_trans_i, (24, 28), mode='bilinear', align_corners=True
+ )
+ x1_local_i = self.local_conv1(x1_trans_i)
+ x1_local_list.append(x1_local_i)
+
+ # ============== Block 2 ==============
+ # Block 2
+ # global branch
+ x2 = self.inception2(x1_out)
+ x2_attn, x2_theta = self.ha2(x2)
+ x2_out = x2 * x2_attn
+ # local branch
+ if self.learn_region:
+ x2_local_list = []
+ for region_idx in range(4):
+ x2_theta_i = x2_theta[:, region_idx, :]
+ x2_theta_i = self.transform_theta(x2_theta_i, region_idx)
+ x2_trans_i = self.stn(x1_out, x2_theta_i)
+ x2_trans_i = F.upsample(
+ x2_trans_i, (12, 14), mode='bilinear', align_corners=True
+ )
+ x2_local_i = x2_trans_i + x1_local_list[region_idx]
+ x2_local_i = self.local_conv2(x2_local_i)
+ x2_local_list.append(x2_local_i)
+
+ # ============== Block 3 ==============
+ # Block 3
+ # global branch
+ x3 = self.inception3(x2_out)
+ x3_attn, x3_theta = self.ha3(x3)
+ x3_out = x3 * x3_attn
+ # local branch
+ if self.learn_region:
+ x3_local_list = []
+ for region_idx in range(4):
+ x3_theta_i = x3_theta[:, region_idx, :]
+ x3_theta_i = self.transform_theta(x3_theta_i, region_idx)
+ x3_trans_i = self.stn(x2_out, x3_theta_i)
+ x3_trans_i = F.upsample(
+ x3_trans_i, (6, 7), mode='bilinear', align_corners=True
+ )
+ x3_local_i = x3_trans_i + x2_local_list[region_idx]
+ x3_local_i = self.local_conv3(x3_local_i)
+ x3_local_list.append(x3_local_i)
+
+ # ============== Feature generation ==============
+ # global branch
+ x_global = F.avg_pool2d(x3_out,
+ x3_out.size()[2:]
+ ).view(x3_out.size(0), x3_out.size(1))
+ x_global = self.fc_global(x_global)
+ # local branch
+ if self.learn_region:
+ x_local_list = []
+ for region_idx in range(4):
+ x_local_i = x3_local_list[region_idx]
+ x_local_i = F.avg_pool2d(x_local_i,
+ x_local_i.size()[2:]
+ ).view(x_local_i.size(0), -1)
+ x_local_list.append(x_local_i)
+ x_local = torch.cat(x_local_list, 1)
+ x_local = self.fc_local(x_local)
+
+ if not self.training:
+ # l2 normalization before concatenation
+ if self.learn_region:
+ x_global = x_global / x_global.norm(p=2, dim=1, keepdim=True)
+ x_local = x_local / x_local.norm(p=2, dim=1, keepdim=True)
+ return torch.cat([x_global, x_local], 1)
+ else:
+ return x_global
+
+ prelogits_global = self.classifier_global(x_global)
+ if self.learn_region:
+ prelogits_local = self.classifier_local(x_local)
+
+ if self.loss == 'softmax':
+ if self.learn_region:
+ return (prelogits_global, prelogits_local)
+ else:
+ return prelogits_global
+
+ elif self.loss == 'triplet':
+ if self.learn_region:
+ return (prelogits_global, prelogits_local), (x_global, x_local)
+ else:
+ return prelogits_global, x_global
+
+ else:
+ raise KeyError("Unsupported loss: {}".format(self.loss))
diff --git a/trackers/strong_sort/deep/reid/torchreid/models/inceptionresnetv2.py b/trackers/strong_sort/deep/reid/torchreid/models/inceptionresnetv2.py
new file mode 100644
index 0000000000000000000000000000000000000000..03e40348425a2b1bc73e6f336efae8e5525cc45c
--- /dev/null
+++ b/trackers/strong_sort/deep/reid/torchreid/models/inceptionresnetv2.py
@@ -0,0 +1,361 @@
+"""
+Code imported from https://github.com/Cadene/pretrained-models.pytorch
+"""
+from __future__ import division, absolute_import
+import torch
+import torch.nn as nn
+import torch.utils.model_zoo as model_zoo
+
+__all__ = ['inceptionresnetv2']
+
+pretrained_settings = {
+ 'inceptionresnetv2': {
+ 'imagenet': {
+ 'url':
+ 'http://data.lip6.fr/cadene/pretrainedmodels/inceptionresnetv2-520b38e4.pth',
+ 'input_space': 'RGB',
+ 'input_size': [3, 299, 299],
+ 'input_range': [0, 1],
+ 'mean': [0.5, 0.5, 0.5],
+ 'std': [0.5, 0.5, 0.5],
+ 'num_classes': 1000
+ },
+ 'imagenet+background': {
+ 'url':
+ 'http://data.lip6.fr/cadene/pretrainedmodels/inceptionresnetv2-520b38e4.pth',
+ 'input_space': 'RGB',
+ 'input_size': [3, 299, 299],
+ 'input_range': [0, 1],
+ 'mean': [0.5, 0.5, 0.5],
+ 'std': [0.5, 0.5, 0.5],
+ 'num_classes': 1001
+ }
+ }
+}
+
+
+class BasicConv2d(nn.Module):
+
+ def __init__(self, in_planes, out_planes, kernel_size, stride, padding=0):
+ super(BasicConv2d, self).__init__()
+ self.conv = nn.Conv2d(
+ in_planes,
+ out_planes,
+ kernel_size=kernel_size,
+ stride=stride,
+ padding=padding,
+ bias=False
+ ) # verify bias false
+ self.bn = nn.BatchNorm2d(
+ out_planes,
+ eps=0.001, # value found in tensorflow
+ momentum=0.1, # default pytorch value
+ affine=True
+ )
+ self.relu = nn.ReLU(inplace=False)
+
+ def forward(self, x):
+ x = self.conv(x)
+ x = self.bn(x)
+ x = self.relu(x)
+ return x
+
+
+class Mixed_5b(nn.Module):
+
+ def __init__(self):
+ super(Mixed_5b, self).__init__()
+
+ self.branch0 = BasicConv2d(192, 96, kernel_size=1, stride=1)
+
+ self.branch1 = nn.Sequential(
+ BasicConv2d(192, 48, kernel_size=1, stride=1),
+ BasicConv2d(48, 64, kernel_size=5, stride=1, padding=2)
+ )
+
+ self.branch2 = nn.Sequential(
+ BasicConv2d(192, 64, kernel_size=1, stride=1),
+ BasicConv2d(64, 96, kernel_size=3, stride=1, padding=1),
+ BasicConv2d(96, 96, kernel_size=3, stride=1, padding=1)
+ )
+
+ self.branch3 = nn.Sequential(
+ nn.AvgPool2d(3, stride=1, padding=1, count_include_pad=False),
+ BasicConv2d(192, 64, kernel_size=1, stride=1)
+ )
+
+ def forward(self, x):
+ x0 = self.branch0(x)
+ x1 = self.branch1(x)
+ x2 = self.branch2(x)
+ x3 = self.branch3(x)
+ out = torch.cat((x0, x1, x2, x3), 1)
+ return out
+
+
+class Block35(nn.Module):
+
+ def __init__(self, scale=1.0):
+ super(Block35, self).__init__()
+
+ self.scale = scale
+
+ self.branch0 = BasicConv2d(320, 32, kernel_size=1, stride=1)
+
+ self.branch1 = nn.Sequential(
+ BasicConv2d(320, 32, kernel_size=1, stride=1),
+ BasicConv2d(32, 32, kernel_size=3, stride=1, padding=1)
+ )
+
+ self.branch2 = nn.Sequential(
+ BasicConv2d(320, 32, kernel_size=1, stride=1),
+ BasicConv2d(32, 48, kernel_size=3, stride=1, padding=1),
+ BasicConv2d(48, 64, kernel_size=3, stride=1, padding=1)
+ )
+
+ self.conv2d = nn.Conv2d(128, 320, kernel_size=1, stride=1)
+ self.relu = nn.ReLU(inplace=False)
+
+ def forward(self, x):
+ x0 = self.branch0(x)
+ x1 = self.branch1(x)
+ x2 = self.branch2(x)
+ out = torch.cat((x0, x1, x2), 1)
+ out = self.conv2d(out)
+ out = out * self.scale + x
+ out = self.relu(out)
+ return out
+
+
+class Mixed_6a(nn.Module):
+
+ def __init__(self):
+ super(Mixed_6a, self).__init__()
+
+ self.branch0 = BasicConv2d(320, 384, kernel_size=3, stride=2)
+
+ self.branch1 = nn.Sequential(
+ BasicConv2d(320, 256, kernel_size=1, stride=1),
+ BasicConv2d(256, 256, kernel_size=3, stride=1, padding=1),
+ BasicConv2d(256, 384, kernel_size=3, stride=2)
+ )
+
+ self.branch2 = nn.MaxPool2d(3, stride=2)
+
+ def forward(self, x):
+ x0 = self.branch0(x)
+ x1 = self.branch1(x)
+ x2 = self.branch2(x)
+ out = torch.cat((x0, x1, x2), 1)
+ return out
+
+
+class Block17(nn.Module):
+
+ def __init__(self, scale=1.0):
+ super(Block17, self).__init__()
+
+ self.scale = scale
+
+ self.branch0 = BasicConv2d(1088, 192, kernel_size=1, stride=1)
+
+ self.branch1 = nn.Sequential(
+ BasicConv2d(1088, 128, kernel_size=1, stride=1),
+ BasicConv2d(
+ 128, 160, kernel_size=(1, 7), stride=1, padding=(0, 3)
+ ),
+ BasicConv2d(
+ 160, 192, kernel_size=(7, 1), stride=1, padding=(3, 0)
+ )
+ )
+
+ self.conv2d = nn.Conv2d(384, 1088, kernel_size=1, stride=1)
+ self.relu = nn.ReLU(inplace=False)
+
+ def forward(self, x):
+ x0 = self.branch0(x)
+ x1 = self.branch1(x)
+ out = torch.cat((x0, x1), 1)
+ out = self.conv2d(out)
+ out = out * self.scale + x
+ out = self.relu(out)
+ return out
+
+
+class Mixed_7a(nn.Module):
+
+ def __init__(self):
+ super(Mixed_7a, self).__init__()
+
+ self.branch0 = nn.Sequential(
+ BasicConv2d(1088, 256, kernel_size=1, stride=1),
+ BasicConv2d(256, 384, kernel_size=3, stride=2)
+ )
+
+ self.branch1 = nn.Sequential(
+ BasicConv2d(1088, 256, kernel_size=1, stride=1),
+ BasicConv2d(256, 288, kernel_size=3, stride=2)
+ )
+
+ self.branch2 = nn.Sequential(
+ BasicConv2d(1088, 256, kernel_size=1, stride=1),
+ BasicConv2d(256, 288, kernel_size=3, stride=1, padding=1),
+ BasicConv2d(288, 320, kernel_size=3, stride=2)
+ )
+
+ self.branch3 = nn.MaxPool2d(3, stride=2)
+
+ def forward(self, x):
+ x0 = self.branch0(x)
+ x1 = self.branch1(x)
+ x2 = self.branch2(x)
+ x3 = self.branch3(x)
+ out = torch.cat((x0, x1, x2, x3), 1)
+ return out
+
+
+class Block8(nn.Module):
+
+ def __init__(self, scale=1.0, noReLU=False):
+ super(Block8, self).__init__()
+
+ self.scale = scale
+ self.noReLU = noReLU
+
+ self.branch0 = BasicConv2d(2080, 192, kernel_size=1, stride=1)
+
+ self.branch1 = nn.Sequential(
+ BasicConv2d(2080, 192, kernel_size=1, stride=1),
+ BasicConv2d(
+ 192, 224, kernel_size=(1, 3), stride=1, padding=(0, 1)
+ ),
+ BasicConv2d(
+ 224, 256, kernel_size=(3, 1), stride=1, padding=(1, 0)
+ )
+ )
+
+ self.conv2d = nn.Conv2d(448, 2080, kernel_size=1, stride=1)
+ if not self.noReLU:
+ self.relu = nn.ReLU(inplace=False)
+
+ def forward(self, x):
+ x0 = self.branch0(x)
+ x1 = self.branch1(x)
+ out = torch.cat((x0, x1), 1)
+ out = self.conv2d(out)
+ out = out * self.scale + x
+ if not self.noReLU:
+ out = self.relu(out)
+ return out
+
+
+# ----------------
+# Model Definition
+# ----------------
+class InceptionResNetV2(nn.Module):
+ """Inception-ResNet-V2.
+
+ Reference:
+ Szegedy et al. Inception-v4, Inception-ResNet and the Impact of Residual
+ Connections on Learning. AAAI 2017.
+
+ Public keys:
+ - ``inceptionresnetv2``: Inception-ResNet-V2.
+ """
+
+ def __init__(self, num_classes, loss='softmax', **kwargs):
+ super(InceptionResNetV2, self).__init__()
+ self.loss = loss
+
+ # Modules
+ self.conv2d_1a = BasicConv2d(3, 32, kernel_size=3, stride=2)
+ self.conv2d_2a = BasicConv2d(32, 32, kernel_size=3, stride=1)
+ self.conv2d_2b = BasicConv2d(
+ 32, 64, kernel_size=3, stride=1, padding=1
+ )
+ self.maxpool_3a = nn.MaxPool2d(3, stride=2)
+ self.conv2d_3b = BasicConv2d(64, 80, kernel_size=1, stride=1)
+ self.conv2d_4a = BasicConv2d(80, 192, kernel_size=3, stride=1)
+ self.maxpool_5a = nn.MaxPool2d(3, stride=2)
+ self.mixed_5b = Mixed_5b()
+ self.repeat = nn.Sequential(
+ Block35(scale=0.17), Block35(scale=0.17), Block35(scale=0.17),
+ Block35(scale=0.17), Block35(scale=0.17), Block35(scale=0.17),
+ Block35(scale=0.17), Block35(scale=0.17), Block35(scale=0.17),
+ Block35(scale=0.17)
+ )
+ self.mixed_6a = Mixed_6a()
+ self.repeat_1 = nn.Sequential(
+ Block17(scale=0.10), Block17(scale=0.10), Block17(scale=0.10),
+ Block17(scale=0.10), Block17(scale=0.10), Block17(scale=0.10),
+ Block17(scale=0.10), Block17(scale=0.10), Block17(scale=0.10),
+ Block17(scale=0.10), Block17(scale=0.10), Block17(scale=0.10),
+ Block17(scale=0.10), Block17(scale=0.10), Block17(scale=0.10),
+ Block17(scale=0.10), Block17(scale=0.10), Block17(scale=0.10),
+ Block17(scale=0.10), Block17(scale=0.10)
+ )
+ self.mixed_7a = Mixed_7a()
+ self.repeat_2 = nn.Sequential(
+ Block8(scale=0.20), Block8(scale=0.20), Block8(scale=0.20),
+ Block8(scale=0.20), Block8(scale=0.20), Block8(scale=0.20),
+ Block8(scale=0.20), Block8(scale=0.20), Block8(scale=0.20)
+ )
+
+ self.block8 = Block8(noReLU=True)
+ self.conv2d_7b = BasicConv2d(2080, 1536, kernel_size=1, stride=1)
+ self.global_avgpool = nn.AdaptiveAvgPool2d(1)
+ self.classifier = nn.Linear(1536, num_classes)
+
+ def load_imagenet_weights(self):
+ settings = pretrained_settings['inceptionresnetv2']['imagenet']
+ pretrain_dict = model_zoo.load_url(settings['url'])
+ model_dict = self.state_dict()
+ pretrain_dict = {
+ k: v
+ for k, v in pretrain_dict.items()
+ if k in model_dict and model_dict[k].size() == v.size()
+ }
+ model_dict.update(pretrain_dict)
+ self.load_state_dict(model_dict)
+
+ def featuremaps(self, x):
+ x = self.conv2d_1a(x)
+ x = self.conv2d_2a(x)
+ x = self.conv2d_2b(x)
+ x = self.maxpool_3a(x)
+ x = self.conv2d_3b(x)
+ x = self.conv2d_4a(x)
+ x = self.maxpool_5a(x)
+ x = self.mixed_5b(x)
+ x = self.repeat(x)
+ x = self.mixed_6a(x)
+ x = self.repeat_1(x)
+ x = self.mixed_7a(x)
+ x = self.repeat_2(x)
+ x = self.block8(x)
+ x = self.conv2d_7b(x)
+ return x
+
+ def forward(self, x):
+ f = self.featuremaps(x)
+ v = self.global_avgpool(f)
+ v = v.view(v.size(0), -1)
+
+ if not self.training:
+ return v
+
+ y = self.classifier(v)
+
+ if self.loss == 'softmax':
+ return y
+ elif self.loss == 'triplet':
+ return y, v
+ else:
+ raise KeyError('Unsupported loss: {}'.format(self.loss))
+
+
+def inceptionresnetv2(num_classes, loss='softmax', pretrained=True, **kwargs):
+ model = InceptionResNetV2(num_classes=num_classes, loss=loss, **kwargs)
+ if pretrained:
+ model.load_imagenet_weights()
+ return model
diff --git a/trackers/strong_sort/deep/reid/torchreid/models/inceptionv4.py b/trackers/strong_sort/deep/reid/torchreid/models/inceptionv4.py
new file mode 100644
index 0000000000000000000000000000000000000000..b14916f140712298866c943ebdb4ebad67d72fc4
--- /dev/null
+++ b/trackers/strong_sort/deep/reid/torchreid/models/inceptionv4.py
@@ -0,0 +1,381 @@
+from __future__ import division, absolute_import
+import torch
+import torch.nn as nn
+import torch.utils.model_zoo as model_zoo
+
+__all__ = ['inceptionv4']
+"""
+Code imported from https://github.com/Cadene/pretrained-models.pytorch
+"""
+
+pretrained_settings = {
+ 'inceptionv4': {
+ 'imagenet': {
+ 'url':
+ 'http://data.lip6.fr/cadene/pretrainedmodels/inceptionv4-8e4777a0.pth',
+ 'input_space': 'RGB',
+ 'input_size': [3, 299, 299],
+ 'input_range': [0, 1],
+ 'mean': [0.5, 0.5, 0.5],
+ 'std': [0.5, 0.5, 0.5],
+ 'num_classes': 1000
+ },
+ 'imagenet+background': {
+ 'url':
+ 'http://data.lip6.fr/cadene/pretrainedmodels/inceptionv4-8e4777a0.pth',
+ 'input_space': 'RGB',
+ 'input_size': [3, 299, 299],
+ 'input_range': [0, 1],
+ 'mean': [0.5, 0.5, 0.5],
+ 'std': [0.5, 0.5, 0.5],
+ 'num_classes': 1001
+ }
+ }
+}
+
+
+class BasicConv2d(nn.Module):
+
+ def __init__(self, in_planes, out_planes, kernel_size, stride, padding=0):
+ super(BasicConv2d, self).__init__()
+ self.conv = nn.Conv2d(
+ in_planes,
+ out_planes,
+ kernel_size=kernel_size,
+ stride=stride,
+ padding=padding,
+ bias=False
+ ) # verify bias false
+ self.bn = nn.BatchNorm2d(
+ out_planes,
+ eps=0.001, # value found in tensorflow
+ momentum=0.1, # default pytorch value
+ affine=True
+ )
+ self.relu = nn.ReLU(inplace=True)
+
+ def forward(self, x):
+ x = self.conv(x)
+ x = self.bn(x)
+ x = self.relu(x)
+ return x
+
+
+class Mixed_3a(nn.Module):
+
+ def __init__(self):
+ super(Mixed_3a, self).__init__()
+ self.maxpool = nn.MaxPool2d(3, stride=2)
+ self.conv = BasicConv2d(64, 96, kernel_size=3, stride=2)
+
+ def forward(self, x):
+ x0 = self.maxpool(x)
+ x1 = self.conv(x)
+ out = torch.cat((x0, x1), 1)
+ return out
+
+
+class Mixed_4a(nn.Module):
+
+ def __init__(self):
+ super(Mixed_4a, self).__init__()
+
+ self.branch0 = nn.Sequential(
+ BasicConv2d(160, 64, kernel_size=1, stride=1),
+ BasicConv2d(64, 96, kernel_size=3, stride=1)
+ )
+
+ self.branch1 = nn.Sequential(
+ BasicConv2d(160, 64, kernel_size=1, stride=1),
+ BasicConv2d(64, 64, kernel_size=(1, 7), stride=1, padding=(0, 3)),
+ BasicConv2d(64, 64, kernel_size=(7, 1), stride=1, padding=(3, 0)),
+ BasicConv2d(64, 96, kernel_size=(3, 3), stride=1)
+ )
+
+ def forward(self, x):
+ x0 = self.branch0(x)
+ x1 = self.branch1(x)
+ out = torch.cat((x0, x1), 1)
+ return out
+
+
+class Mixed_5a(nn.Module):
+
+ def __init__(self):
+ super(Mixed_5a, self).__init__()
+ self.conv = BasicConv2d(192, 192, kernel_size=3, stride=2)
+ self.maxpool = nn.MaxPool2d(3, stride=2)
+
+ def forward(self, x):
+ x0 = self.conv(x)
+ x1 = self.maxpool(x)
+ out = torch.cat((x0, x1), 1)
+ return out
+
+
+class Inception_A(nn.Module):
+
+ def __init__(self):
+ super(Inception_A, self).__init__()
+ self.branch0 = BasicConv2d(384, 96, kernel_size=1, stride=1)
+
+ self.branch1 = nn.Sequential(
+ BasicConv2d(384, 64, kernel_size=1, stride=1),
+ BasicConv2d(64, 96, kernel_size=3, stride=1, padding=1)
+ )
+
+ self.branch2 = nn.Sequential(
+ BasicConv2d(384, 64, kernel_size=1, stride=1),
+ BasicConv2d(64, 96, kernel_size=3, stride=1, padding=1),
+ BasicConv2d(96, 96, kernel_size=3, stride=1, padding=1)
+ )
+
+ self.branch3 = nn.Sequential(
+ nn.AvgPool2d(3, stride=1, padding=1, count_include_pad=False),
+ BasicConv2d(384, 96, kernel_size=1, stride=1)
+ )
+
+ def forward(self, x):
+ x0 = self.branch0(x)
+ x1 = self.branch1(x)
+ x2 = self.branch2(x)
+ x3 = self.branch3(x)
+ out = torch.cat((x0, x1, x2, x3), 1)
+ return out
+
+
+class Reduction_A(nn.Module):
+
+ def __init__(self):
+ super(Reduction_A, self).__init__()
+ self.branch0 = BasicConv2d(384, 384, kernel_size=3, stride=2)
+
+ self.branch1 = nn.Sequential(
+ BasicConv2d(384, 192, kernel_size=1, stride=1),
+ BasicConv2d(192, 224, kernel_size=3, stride=1, padding=1),
+ BasicConv2d(224, 256, kernel_size=3, stride=2)
+ )
+
+ self.branch2 = nn.MaxPool2d(3, stride=2)
+
+ def forward(self, x):
+ x0 = self.branch0(x)
+ x1 = self.branch1(x)
+ x2 = self.branch2(x)
+ out = torch.cat((x0, x1, x2), 1)
+ return out
+
+
+class Inception_B(nn.Module):
+
+ def __init__(self):
+ super(Inception_B, self).__init__()
+ self.branch0 = BasicConv2d(1024, 384, kernel_size=1, stride=1)
+
+ self.branch1 = nn.Sequential(
+ BasicConv2d(1024, 192, kernel_size=1, stride=1),
+ BasicConv2d(
+ 192, 224, kernel_size=(1, 7), stride=1, padding=(0, 3)
+ ),
+ BasicConv2d(
+ 224, 256, kernel_size=(7, 1), stride=1, padding=(3, 0)
+ )
+ )
+
+ self.branch2 = nn.Sequential(
+ BasicConv2d(1024, 192, kernel_size=1, stride=1),
+ BasicConv2d(
+ 192, 192, kernel_size=(7, 1), stride=1, padding=(3, 0)
+ ),
+ BasicConv2d(
+ 192, 224, kernel_size=(1, 7), stride=1, padding=(0, 3)
+ ),
+ BasicConv2d(
+ 224, 224, kernel_size=(7, 1), stride=1, padding=(3, 0)
+ ),
+ BasicConv2d(
+ 224, 256, kernel_size=(1, 7), stride=1, padding=(0, 3)
+ )
+ )
+
+ self.branch3 = nn.Sequential(
+ nn.AvgPool2d(3, stride=1, padding=1, count_include_pad=False),
+ BasicConv2d(1024, 128, kernel_size=1, stride=1)
+ )
+
+ def forward(self, x):
+ x0 = self.branch0(x)
+ x1 = self.branch1(x)
+ x2 = self.branch2(x)
+ x3 = self.branch3(x)
+ out = torch.cat((x0, x1, x2, x3), 1)
+ return out
+
+
+class Reduction_B(nn.Module):
+
+ def __init__(self):
+ super(Reduction_B, self).__init__()
+
+ self.branch0 = nn.Sequential(
+ BasicConv2d(1024, 192, kernel_size=1, stride=1),
+ BasicConv2d(192, 192, kernel_size=3, stride=2)
+ )
+
+ self.branch1 = nn.Sequential(
+ BasicConv2d(1024, 256, kernel_size=1, stride=1),
+ BasicConv2d(
+ 256, 256, kernel_size=(1, 7), stride=1, padding=(0, 3)
+ ),
+ BasicConv2d(
+ 256, 320, kernel_size=(7, 1), stride=1, padding=(3, 0)
+ ), BasicConv2d(320, 320, kernel_size=3, stride=2)
+ )
+
+ self.branch2 = nn.MaxPool2d(3, stride=2)
+
+ def forward(self, x):
+ x0 = self.branch0(x)
+ x1 = self.branch1(x)
+ x2 = self.branch2(x)
+ out = torch.cat((x0, x1, x2), 1)
+ return out
+
+
+class Inception_C(nn.Module):
+
+ def __init__(self):
+ super(Inception_C, self).__init__()
+
+ self.branch0 = BasicConv2d(1536, 256, kernel_size=1, stride=1)
+
+ self.branch1_0 = BasicConv2d(1536, 384, kernel_size=1, stride=1)
+ self.branch1_1a = BasicConv2d(
+ 384, 256, kernel_size=(1, 3), stride=1, padding=(0, 1)
+ )
+ self.branch1_1b = BasicConv2d(
+ 384, 256, kernel_size=(3, 1), stride=1, padding=(1, 0)
+ )
+
+ self.branch2_0 = BasicConv2d(1536, 384, kernel_size=1, stride=1)
+ self.branch2_1 = BasicConv2d(
+ 384, 448, kernel_size=(3, 1), stride=1, padding=(1, 0)
+ )
+ self.branch2_2 = BasicConv2d(
+ 448, 512, kernel_size=(1, 3), stride=1, padding=(0, 1)
+ )
+ self.branch2_3a = BasicConv2d(
+ 512, 256, kernel_size=(1, 3), stride=1, padding=(0, 1)
+ )
+ self.branch2_3b = BasicConv2d(
+ 512, 256, kernel_size=(3, 1), stride=1, padding=(1, 0)
+ )
+
+ self.branch3 = nn.Sequential(
+ nn.AvgPool2d(3, stride=1, padding=1, count_include_pad=False),
+ BasicConv2d(1536, 256, kernel_size=1, stride=1)
+ )
+
+ def forward(self, x):
+ x0 = self.branch0(x)
+
+ x1_0 = self.branch1_0(x)
+ x1_1a = self.branch1_1a(x1_0)
+ x1_1b = self.branch1_1b(x1_0)
+ x1 = torch.cat((x1_1a, x1_1b), 1)
+
+ x2_0 = self.branch2_0(x)
+ x2_1 = self.branch2_1(x2_0)
+ x2_2 = self.branch2_2(x2_1)
+ x2_3a = self.branch2_3a(x2_2)
+ x2_3b = self.branch2_3b(x2_2)
+ x2 = torch.cat((x2_3a, x2_3b), 1)
+
+ x3 = self.branch3(x)
+
+ out = torch.cat((x0, x1, x2, x3), 1)
+ return out
+
+
+class InceptionV4(nn.Module):
+ """Inception-v4.
+
+ Reference:
+ Szegedy et al. Inception-v4, Inception-ResNet and the Impact of Residual
+ Connections on Learning. AAAI 2017.
+
+ Public keys:
+ - ``inceptionv4``: InceptionV4.
+ """
+
+ def __init__(self, num_classes, loss, **kwargs):
+ super(InceptionV4, self).__init__()
+ self.loss = loss
+
+ self.features = nn.Sequential(
+ BasicConv2d(3, 32, kernel_size=3, stride=2),
+ BasicConv2d(32, 32, kernel_size=3, stride=1),
+ BasicConv2d(32, 64, kernel_size=3, stride=1, padding=1),
+ Mixed_3a(),
+ Mixed_4a(),
+ Mixed_5a(),
+ Inception_A(),
+ Inception_A(),
+ Inception_A(),
+ Inception_A(),
+ Reduction_A(), # Mixed_6a
+ Inception_B(),
+ Inception_B(),
+ Inception_B(),
+ Inception_B(),
+ Inception_B(),
+ Inception_B(),
+ Inception_B(),
+ Reduction_B(), # Mixed_7a
+ Inception_C(),
+ Inception_C(),
+ Inception_C()
+ )
+ self.global_avgpool = nn.AdaptiveAvgPool2d(1)
+ self.classifier = nn.Linear(1536, num_classes)
+
+ def forward(self, x):
+ f = self.features(x)
+ v = self.global_avgpool(f)
+ v = v.view(v.size(0), -1)
+
+ if not self.training:
+ return v
+
+ y = self.classifier(v)
+
+ if self.loss == 'softmax':
+ return y
+ elif self.loss == 'triplet':
+ return y, v
+ else:
+ raise KeyError('Unsupported loss: {}'.format(self.loss))
+
+
+def init_pretrained_weights(model, model_url):
+ """Initializes model with pretrained weights.
+
+ Layers that don't match with pretrained layers in name or size are kept unchanged.
+ """
+ pretrain_dict = model_zoo.load_url(model_url)
+ model_dict = model.state_dict()
+ pretrain_dict = {
+ k: v
+ for k, v in pretrain_dict.items()
+ if k in model_dict and model_dict[k].size() == v.size()
+ }
+ model_dict.update(pretrain_dict)
+ model.load_state_dict(model_dict)
+
+
+def inceptionv4(num_classes, loss='softmax', pretrained=True, **kwargs):
+ model = InceptionV4(num_classes, loss, **kwargs)
+ if pretrained:
+ model_url = pretrained_settings['inceptionv4']['imagenet']['url']
+ init_pretrained_weights(model, model_url)
+ return model
diff --git a/trackers/strong_sort/deep/reid/torchreid/models/mlfn.py b/trackers/strong_sort/deep/reid/torchreid/models/mlfn.py
new file mode 100644
index 0000000000000000000000000000000000000000..ac7e126b073db6a710fc41e62624127ca91ec131
--- /dev/null
+++ b/trackers/strong_sort/deep/reid/torchreid/models/mlfn.py
@@ -0,0 +1,269 @@
+from __future__ import division, absolute_import
+import torch
+import torch.utils.model_zoo as model_zoo
+from torch import nn
+from torch.nn import functional as F
+
+__all__ = ['mlfn']
+
+model_urls = {
+ # training epoch = 5, top1 = 51.6
+ 'imagenet':
+ 'https://mega.nz/#!YHxAhaxC!yu9E6zWl0x5zscSouTdbZu8gdFFytDdl-RAdD2DEfpk',
+}
+
+
+class MLFNBlock(nn.Module):
+
+ def __init__(
+ self, in_channels, out_channels, stride, fsm_channels, groups=32
+ ):
+ super(MLFNBlock, self).__init__()
+ self.groups = groups
+ mid_channels = out_channels // 2
+
+ # Factor Modules
+ self.fm_conv1 = nn.Conv2d(in_channels, mid_channels, 1, bias=False)
+ self.fm_bn1 = nn.BatchNorm2d(mid_channels)
+ self.fm_conv2 = nn.Conv2d(
+ mid_channels,
+ mid_channels,
+ 3,
+ stride=stride,
+ padding=1,
+ bias=False,
+ groups=self.groups
+ )
+ self.fm_bn2 = nn.BatchNorm2d(mid_channels)
+ self.fm_conv3 = nn.Conv2d(mid_channels, out_channels, 1, bias=False)
+ self.fm_bn3 = nn.BatchNorm2d(out_channels)
+
+ # Factor Selection Module
+ self.fsm = nn.Sequential(
+ nn.AdaptiveAvgPool2d(1),
+ nn.Conv2d(in_channels, fsm_channels[0], 1),
+ nn.BatchNorm2d(fsm_channels[0]),
+ nn.ReLU(inplace=True),
+ nn.Conv2d(fsm_channels[0], fsm_channels[1], 1),
+ nn.BatchNorm2d(fsm_channels[1]),
+ nn.ReLU(inplace=True),
+ nn.Conv2d(fsm_channels[1], self.groups, 1),
+ nn.BatchNorm2d(self.groups),
+ nn.Sigmoid(),
+ )
+
+ self.downsample = None
+ if in_channels != out_channels or stride > 1:
+ self.downsample = nn.Sequential(
+ nn.Conv2d(
+ in_channels, out_channels, 1, stride=stride, bias=False
+ ),
+ nn.BatchNorm2d(out_channels),
+ )
+
+ def forward(self, x):
+ residual = x
+ s = self.fsm(x)
+
+ # reduce dimension
+ x = self.fm_conv1(x)
+ x = self.fm_bn1(x)
+ x = F.relu(x, inplace=True)
+
+ # group convolution
+ x = self.fm_conv2(x)
+ x = self.fm_bn2(x)
+ x = F.relu(x, inplace=True)
+
+ # factor selection
+ b, c = x.size(0), x.size(1)
+ n = c // self.groups
+ ss = s.repeat(1, n, 1, 1) # from (b, g, 1, 1) to (b, g*n=c, 1, 1)
+ ss = ss.view(b, n, self.groups, 1, 1)
+ ss = ss.permute(0, 2, 1, 3, 4).contiguous()
+ ss = ss.view(b, c, 1, 1)
+ x = ss * x
+
+ # recover dimension
+ x = self.fm_conv3(x)
+ x = self.fm_bn3(x)
+ x = F.relu(x, inplace=True)
+
+ if self.downsample is not None:
+ residual = self.downsample(residual)
+
+ return F.relu(residual + x, inplace=True), s
+
+
+class MLFN(nn.Module):
+ """Multi-Level Factorisation Net.
+
+ Reference:
+ Chang et al. Multi-Level Factorisation Net for
+ Person Re-Identification. CVPR 2018.
+
+ Public keys:
+ - ``mlfn``: MLFN (Multi-Level Factorisation Net).
+ """
+
+ def __init__(
+ self,
+ num_classes,
+ loss='softmax',
+ groups=32,
+ channels=[64, 256, 512, 1024, 2048],
+ embed_dim=1024,
+ **kwargs
+ ):
+ super(MLFN, self).__init__()
+ self.loss = loss
+ self.groups = groups
+
+ # first convolutional layer
+ self.conv1 = nn.Conv2d(3, channels[0], 7, stride=2, padding=3)
+ self.bn1 = nn.BatchNorm2d(channels[0])
+ self.maxpool = nn.MaxPool2d(3, stride=2, padding=1)
+
+ # main body
+ self.feature = nn.ModuleList(
+ [
+ # layer 1-3
+ MLFNBlock(channels[0], channels[1], 1, [128, 64], self.groups),
+ MLFNBlock(channels[1], channels[1], 1, [128, 64], self.groups),
+ MLFNBlock(channels[1], channels[1], 1, [128, 64], self.groups),
+ # layer 4-7
+ MLFNBlock(
+ channels[1], channels[2], 2, [256, 128], self.groups
+ ),
+ MLFNBlock(
+ channels[2], channels[2], 1, [256, 128], self.groups
+ ),
+ MLFNBlock(
+ channels[2], channels[2], 1, [256, 128], self.groups
+ ),
+ MLFNBlock(
+ channels[2], channels[2], 1, [256, 128], self.groups
+ ),
+ # layer 8-13
+ MLFNBlock(
+ channels[2], channels[3], 2, [512, 128], self.groups
+ ),
+ MLFNBlock(
+ channels[3], channels[3], 1, [512, 128], self.groups
+ ),
+ MLFNBlock(
+ channels[3], channels[3], 1, [512, 128], self.groups
+ ),
+ MLFNBlock(
+ channels[3], channels[3], 1, [512, 128], self.groups
+ ),
+ MLFNBlock(
+ channels[3], channels[3], 1, [512, 128], self.groups
+ ),
+ MLFNBlock(
+ channels[3], channels[3], 1, [512, 128], self.groups
+ ),
+ # layer 14-16
+ MLFNBlock(
+ channels[3], channels[4], 2, [512, 128], self.groups
+ ),
+ MLFNBlock(
+ channels[4], channels[4], 1, [512, 128], self.groups
+ ),
+ MLFNBlock(
+ channels[4], channels[4], 1, [512, 128], self.groups
+ ),
+ ]
+ )
+ self.global_avgpool = nn.AdaptiveAvgPool2d(1)
+
+ # projection functions
+ self.fc_x = nn.Sequential(
+ nn.Conv2d(channels[4], embed_dim, 1, bias=False),
+ nn.BatchNorm2d(embed_dim),
+ nn.ReLU(inplace=True),
+ )
+ self.fc_s = nn.Sequential(
+ nn.Conv2d(self.groups * 16, embed_dim, 1, bias=False),
+ nn.BatchNorm2d(embed_dim),
+ nn.ReLU(inplace=True),
+ )
+
+ self.classifier = nn.Linear(embed_dim, num_classes)
+
+ self.init_params()
+
+ def init_params(self):
+ for m in self.modules():
+ if isinstance(m, nn.Conv2d):
+ nn.init.kaiming_normal_(
+ m.weight, mode='fan_out', nonlinearity='relu'
+ )
+ if m.bias is not None:
+ nn.init.constant_(m.bias, 0)
+ elif isinstance(m, nn.BatchNorm2d):
+ nn.init.constant_(m.weight, 1)
+ nn.init.constant_(m.bias, 0)
+ elif isinstance(m, nn.Linear):
+ nn.init.normal_(m.weight, 0, 0.01)
+ if m.bias is not None:
+ nn.init.constant_(m.bias, 0)
+
+ def forward(self, x):
+ x = self.conv1(x)
+ x = self.bn1(x)
+ x = F.relu(x, inplace=True)
+ x = self.maxpool(x)
+
+ s_hat = []
+ for block in self.feature:
+ x, s = block(x)
+ s_hat.append(s)
+ s_hat = torch.cat(s_hat, 1)
+
+ x = self.global_avgpool(x)
+ x = self.fc_x(x)
+ s_hat = self.fc_s(s_hat)
+
+ v = (x+s_hat) * 0.5
+ v = v.view(v.size(0), -1)
+
+ if not self.training:
+ return v
+
+ y = self.classifier(v)
+
+ if self.loss == 'softmax':
+ return y
+ elif self.loss == 'triplet':
+ return y, v
+ else:
+ raise KeyError('Unsupported loss: {}'.format(self.loss))
+
+
+def init_pretrained_weights(model, model_url):
+ """Initializes model with pretrained weights.
+
+ Layers that don't match with pretrained layers in name or size are kept unchanged.
+ """
+ pretrain_dict = model_zoo.load_url(model_url)
+ model_dict = model.state_dict()
+ pretrain_dict = {
+ k: v
+ for k, v in pretrain_dict.items()
+ if k in model_dict and model_dict[k].size() == v.size()
+ }
+ model_dict.update(pretrain_dict)
+ model.load_state_dict(model_dict)
+
+
+def mlfn(num_classes, loss='softmax', pretrained=True, **kwargs):
+ model = MLFN(num_classes, loss, **kwargs)
+ if pretrained:
+ # init_pretrained_weights(model, model_urls['imagenet'])
+ import warnings
+ warnings.warn(
+ 'The imagenet pretrained weights need to be manually downloaded from {}'
+ .format(model_urls['imagenet'])
+ )
+ return model
diff --git a/trackers/strong_sort/deep/reid/torchreid/models/mobilenetv2.py b/trackers/strong_sort/deep/reid/torchreid/models/mobilenetv2.py
new file mode 100644
index 0000000000000000000000000000000000000000..c451ef84e726ebc8d4c8e47253f335494eb801c9
--- /dev/null
+++ b/trackers/strong_sort/deep/reid/torchreid/models/mobilenetv2.py
@@ -0,0 +1,274 @@
+from __future__ import division, absolute_import
+import torch.utils.model_zoo as model_zoo
+from torch import nn
+from torch.nn import functional as F
+
+__all__ = ['mobilenetv2_x1_0', 'mobilenetv2_x1_4']
+
+model_urls = {
+ # 1.0: top-1 71.3
+ 'mobilenetv2_x1_0':
+ 'https://mega.nz/#!NKp2wAIA!1NH1pbNzY_M2hVk_hdsxNM1NUOWvvGPHhaNr-fASF6c',
+ # 1.4: top-1 73.9
+ 'mobilenetv2_x1_4':
+ 'https://mega.nz/#!RGhgEIwS!xN2s2ZdyqI6vQ3EwgmRXLEW3khr9tpXg96G9SUJugGk',
+}
+
+
+class ConvBlock(nn.Module):
+ """Basic convolutional block.
+
+ convolution (bias discarded) + batch normalization + relu6.
+
+ Args:
+ in_c (int): number of input channels.
+ out_c (int): number of output channels.
+ k (int or tuple): kernel size.
+ s (int or tuple): stride.
+ p (int or tuple): padding.
+ g (int): number of blocked connections from input channels
+ to output channels (default: 1).
+ """
+
+ def __init__(self, in_c, out_c, k, s=1, p=0, g=1):
+ super(ConvBlock, self).__init__()
+ self.conv = nn.Conv2d(
+ in_c, out_c, k, stride=s, padding=p, bias=False, groups=g
+ )
+ self.bn = nn.BatchNorm2d(out_c)
+
+ def forward(self, x):
+ return F.relu6(self.bn(self.conv(x)))
+
+
+class Bottleneck(nn.Module):
+
+ def __init__(self, in_channels, out_channels, expansion_factor, stride=1):
+ super(Bottleneck, self).__init__()
+ mid_channels = in_channels * expansion_factor
+ self.use_residual = stride == 1 and in_channels == out_channels
+ self.conv1 = ConvBlock(in_channels, mid_channels, 1)
+ self.dwconv2 = ConvBlock(
+ mid_channels, mid_channels, 3, stride, 1, g=mid_channels
+ )
+ self.conv3 = nn.Sequential(
+ nn.Conv2d(mid_channels, out_channels, 1, bias=False),
+ nn.BatchNorm2d(out_channels),
+ )
+
+ def forward(self, x):
+ m = self.conv1(x)
+ m = self.dwconv2(m)
+ m = self.conv3(m)
+ if self.use_residual:
+ return x + m
+ else:
+ return m
+
+
+class MobileNetV2(nn.Module):
+ """MobileNetV2.
+
+ Reference:
+ Sandler et al. MobileNetV2: Inverted Residuals and
+ Linear Bottlenecks. CVPR 2018.
+
+ Public keys:
+ - ``mobilenetv2_x1_0``: MobileNetV2 x1.0.
+ - ``mobilenetv2_x1_4``: MobileNetV2 x1.4.
+ """
+
+ def __init__(
+ self,
+ num_classes,
+ width_mult=1,
+ loss='softmax',
+ fc_dims=None,
+ dropout_p=None,
+ **kwargs
+ ):
+ super(MobileNetV2, self).__init__()
+ self.loss = loss
+ self.in_channels = int(32 * width_mult)
+ self.feature_dim = int(1280 * width_mult) if width_mult > 1 else 1280
+
+ # construct layers
+ self.conv1 = ConvBlock(3, self.in_channels, 3, s=2, p=1)
+ self.conv2 = self._make_layer(
+ Bottleneck, 1, int(16 * width_mult), 1, 1
+ )
+ self.conv3 = self._make_layer(
+ Bottleneck, 6, int(24 * width_mult), 2, 2
+ )
+ self.conv4 = self._make_layer(
+ Bottleneck, 6, int(32 * width_mult), 3, 2
+ )
+ self.conv5 = self._make_layer(
+ Bottleneck, 6, int(64 * width_mult), 4, 2
+ )
+ self.conv6 = self._make_layer(
+ Bottleneck, 6, int(96 * width_mult), 3, 1
+ )
+ self.conv7 = self._make_layer(
+ Bottleneck, 6, int(160 * width_mult), 3, 2
+ )
+ self.conv8 = self._make_layer(
+ Bottleneck, 6, int(320 * width_mult), 1, 1
+ )
+ self.conv9 = ConvBlock(self.in_channels, self.feature_dim, 1)
+
+ self.global_avgpool = nn.AdaptiveAvgPool2d(1)
+ self.fc = self._construct_fc_layer(
+ fc_dims, self.feature_dim, dropout_p
+ )
+ self.classifier = nn.Linear(self.feature_dim, num_classes)
+
+ self._init_params()
+
+ def _make_layer(self, block, t, c, n, s):
+ # t: expansion factor
+ # c: output channels
+ # n: number of blocks
+ # s: stride for first layer
+ layers = []
+ layers.append(block(self.in_channels, c, t, s))
+ self.in_channels = c
+ for i in range(1, n):
+ layers.append(block(self.in_channels, c, t))
+ return nn.Sequential(*layers)
+
+ def _construct_fc_layer(self, fc_dims, input_dim, dropout_p=None):
+ """Constructs fully connected layer.
+
+ Args:
+ fc_dims (list or tuple): dimensions of fc layers, if None, no fc layers are constructed
+ input_dim (int): input dimension
+ dropout_p (float): dropout probability, if None, dropout is unused
+ """
+ if fc_dims is None:
+ self.feature_dim = input_dim
+ return None
+
+ assert isinstance(
+ fc_dims, (list, tuple)
+ ), 'fc_dims must be either list or tuple, but got {}'.format(
+ type(fc_dims)
+ )
+
+ layers = []
+ for dim in fc_dims:
+ layers.append(nn.Linear(input_dim, dim))
+ layers.append(nn.BatchNorm1d(dim))
+ layers.append(nn.ReLU(inplace=True))
+ if dropout_p is not None:
+ layers.append(nn.Dropout(p=dropout_p))
+ input_dim = dim
+
+ self.feature_dim = fc_dims[-1]
+
+ return nn.Sequential(*layers)
+
+ def _init_params(self):
+ for m in self.modules():
+ if isinstance(m, nn.Conv2d):
+ nn.init.kaiming_normal_(
+ m.weight, mode='fan_out', nonlinearity='relu'
+ )
+ if m.bias is not None:
+ nn.init.constant_(m.bias, 0)
+ elif isinstance(m, nn.BatchNorm2d):
+ nn.init.constant_(m.weight, 1)
+ nn.init.constant_(m.bias, 0)
+ elif isinstance(m, nn.BatchNorm1d):
+ nn.init.constant_(m.weight, 1)
+ nn.init.constant_(m.bias, 0)
+ elif isinstance(m, nn.Linear):
+ nn.init.normal_(m.weight, 0, 0.01)
+ if m.bias is not None:
+ nn.init.constant_(m.bias, 0)
+
+ def featuremaps(self, x):
+ x = self.conv1(x)
+ x = self.conv2(x)
+ x = self.conv3(x)
+ x = self.conv4(x)
+ x = self.conv5(x)
+ x = self.conv6(x)
+ x = self.conv7(x)
+ x = self.conv8(x)
+ x = self.conv9(x)
+ return x
+
+ def forward(self, x):
+ f = self.featuremaps(x)
+ v = self.global_avgpool(f)
+ v = v.view(v.size(0), -1)
+
+ if self.fc is not None:
+ v = self.fc(v)
+
+ if not self.training:
+ return v
+
+ y = self.classifier(v)
+
+ if self.loss == 'softmax':
+ return y
+ elif self.loss == 'triplet':
+ return y, v
+ else:
+ raise KeyError("Unsupported loss: {}".format(self.loss))
+
+
+def init_pretrained_weights(model, model_url):
+ """Initializes model with pretrained weights.
+
+ Layers that don't match with pretrained layers in name or size are kept unchanged.
+ """
+ pretrain_dict = model_zoo.load_url(model_url)
+ model_dict = model.state_dict()
+ pretrain_dict = {
+ k: v
+ for k, v in pretrain_dict.items()
+ if k in model_dict and model_dict[k].size() == v.size()
+ }
+ model_dict.update(pretrain_dict)
+ model.load_state_dict(model_dict)
+
+
+def mobilenetv2_x1_0(num_classes, loss, pretrained=True, **kwargs):
+ model = MobileNetV2(
+ num_classes,
+ loss=loss,
+ width_mult=1,
+ fc_dims=None,
+ dropout_p=None,
+ **kwargs
+ )
+ if pretrained:
+ # init_pretrained_weights(model, model_urls['mobilenetv2_x1_0'])
+ import warnings
+ warnings.warn(
+ 'The imagenet pretrained weights need to be manually downloaded from {}'
+ .format(model_urls['mobilenetv2_x1_0'])
+ )
+ return model
+
+
+def mobilenetv2_x1_4(num_classes, loss, pretrained=True, **kwargs):
+ model = MobileNetV2(
+ num_classes,
+ loss=loss,
+ width_mult=1.4,
+ fc_dims=None,
+ dropout_p=None,
+ **kwargs
+ )
+ if pretrained:
+ # init_pretrained_weights(model, model_urls['mobilenetv2_x1_4'])
+ import warnings
+ warnings.warn(
+ 'The imagenet pretrained weights need to be manually downloaded from {}'
+ .format(model_urls['mobilenetv2_x1_4'])
+ )
+ return model
diff --git a/trackers/strong_sort/deep/reid/torchreid/models/mudeep.py b/trackers/strong_sort/deep/reid/torchreid/models/mudeep.py
new file mode 100644
index 0000000000000000000000000000000000000000..ddbca675b69fcf38523d8687d8c7b279ededd8d1
--- /dev/null
+++ b/trackers/strong_sort/deep/reid/torchreid/models/mudeep.py
@@ -0,0 +1,206 @@
+from __future__ import division, absolute_import
+import torch
+from torch import nn
+from torch.nn import functional as F
+
+__all__ = ['MuDeep']
+
+
+class ConvBlock(nn.Module):
+ """Basic convolutional block.
+
+ convolution + batch normalization + relu.
+
+ Args:
+ in_c (int): number of input channels.
+ out_c (int): number of output channels.
+ k (int or tuple): kernel size.
+ s (int or tuple): stride.
+ p (int or tuple): padding.
+ """
+
+ def __init__(self, in_c, out_c, k, s, p):
+ super(ConvBlock, self).__init__()
+ self.conv = nn.Conv2d(in_c, out_c, k, stride=s, padding=p)
+ self.bn = nn.BatchNorm2d(out_c)
+
+ def forward(self, x):
+ return F.relu(self.bn(self.conv(x)))
+
+
+class ConvLayers(nn.Module):
+ """Preprocessing layers."""
+
+ def __init__(self):
+ super(ConvLayers, self).__init__()
+ self.conv1 = ConvBlock(3, 48, k=3, s=1, p=1)
+ self.conv2 = ConvBlock(48, 96, k=3, s=1, p=1)
+ self.maxpool = nn.MaxPool2d(kernel_size=3, stride=2, padding=1)
+
+ def forward(self, x):
+ x = self.conv1(x)
+ x = self.conv2(x)
+ x = self.maxpool(x)
+ return x
+
+
+class MultiScaleA(nn.Module):
+ """Multi-scale stream layer A (Sec.3.1)"""
+
+ def __init__(self):
+ super(MultiScaleA, self).__init__()
+ self.stream1 = nn.Sequential(
+ ConvBlock(96, 96, k=1, s=1, p=0),
+ ConvBlock(96, 24, k=3, s=1, p=1),
+ )
+ self.stream2 = nn.Sequential(
+ nn.AvgPool2d(kernel_size=3, stride=1, padding=1),
+ ConvBlock(96, 24, k=1, s=1, p=0),
+ )
+ self.stream3 = ConvBlock(96, 24, k=1, s=1, p=0)
+ self.stream4 = nn.Sequential(
+ ConvBlock(96, 16, k=1, s=1, p=0),
+ ConvBlock(16, 24, k=3, s=1, p=1),
+ ConvBlock(24, 24, k=3, s=1, p=1),
+ )
+
+ def forward(self, x):
+ s1 = self.stream1(x)
+ s2 = self.stream2(x)
+ s3 = self.stream3(x)
+ s4 = self.stream4(x)
+ y = torch.cat([s1, s2, s3, s4], dim=1)
+ return y
+
+
+class Reduction(nn.Module):
+ """Reduction layer (Sec.3.1)"""
+
+ def __init__(self):
+ super(Reduction, self).__init__()
+ self.stream1 = nn.MaxPool2d(kernel_size=3, stride=2, padding=1)
+ self.stream2 = ConvBlock(96, 96, k=3, s=2, p=1)
+ self.stream3 = nn.Sequential(
+ ConvBlock(96, 48, k=1, s=1, p=0),
+ ConvBlock(48, 56, k=3, s=1, p=1),
+ ConvBlock(56, 64, k=3, s=2, p=1),
+ )
+
+ def forward(self, x):
+ s1 = self.stream1(x)
+ s2 = self.stream2(x)
+ s3 = self.stream3(x)
+ y = torch.cat([s1, s2, s3], dim=1)
+ return y
+
+
+class MultiScaleB(nn.Module):
+ """Multi-scale stream layer B (Sec.3.1)"""
+
+ def __init__(self):
+ super(MultiScaleB, self).__init__()
+ self.stream1 = nn.Sequential(
+ nn.AvgPool2d(kernel_size=3, stride=1, padding=1),
+ ConvBlock(256, 256, k=1, s=1, p=0),
+ )
+ self.stream2 = nn.Sequential(
+ ConvBlock(256, 64, k=1, s=1, p=0),
+ ConvBlock(64, 128, k=(1, 3), s=1, p=(0, 1)),
+ ConvBlock(128, 256, k=(3, 1), s=1, p=(1, 0)),
+ )
+ self.stream3 = ConvBlock(256, 256, k=1, s=1, p=0)
+ self.stream4 = nn.Sequential(
+ ConvBlock(256, 64, k=1, s=1, p=0),
+ ConvBlock(64, 64, k=(1, 3), s=1, p=(0, 1)),
+ ConvBlock(64, 128, k=(3, 1), s=1, p=(1, 0)),
+ ConvBlock(128, 128, k=(1, 3), s=1, p=(0, 1)),
+ ConvBlock(128, 256, k=(3, 1), s=1, p=(1, 0)),
+ )
+
+ def forward(self, x):
+ s1 = self.stream1(x)
+ s2 = self.stream2(x)
+ s3 = self.stream3(x)
+ s4 = self.stream4(x)
+ return s1, s2, s3, s4
+
+
+class Fusion(nn.Module):
+ """Saliency-based learning fusion layer (Sec.3.2)"""
+
+ def __init__(self):
+ super(Fusion, self).__init__()
+ self.a1 = nn.Parameter(torch.rand(1, 256, 1, 1))
+ self.a2 = nn.Parameter(torch.rand(1, 256, 1, 1))
+ self.a3 = nn.Parameter(torch.rand(1, 256, 1, 1))
+ self.a4 = nn.Parameter(torch.rand(1, 256, 1, 1))
+
+ # We add an average pooling layer to reduce the spatial dimension
+ # of feature maps, which differs from the original paper.
+ self.avgpool = nn.AvgPool2d(kernel_size=4, stride=4, padding=0)
+
+ def forward(self, x1, x2, x3, x4):
+ s1 = self.a1.expand_as(x1) * x1
+ s2 = self.a2.expand_as(x2) * x2
+ s3 = self.a3.expand_as(x3) * x3
+ s4 = self.a4.expand_as(x4) * x4
+ y = self.avgpool(s1 + s2 + s3 + s4)
+ return y
+
+
+class MuDeep(nn.Module):
+ """Multiscale deep neural network.
+
+ Reference:
+ Qian et al. Multi-scale Deep Learning Architectures
+ for Person Re-identification. ICCV 2017.
+
+ Public keys:
+ - ``mudeep``: Multiscale deep neural network.
+ """
+
+ def __init__(self, num_classes, loss='softmax', **kwargs):
+ super(MuDeep, self).__init__()
+ self.loss = loss
+
+ self.block1 = ConvLayers()
+ self.block2 = MultiScaleA()
+ self.block3 = Reduction()
+ self.block4 = MultiScaleB()
+ self.block5 = Fusion()
+
+ # Due to this fully connected layer, input image has to be fixed
+ # in shape, i.e. (3, 256, 128), such that the last convolutional feature
+ # maps are of shape (256, 16, 8). If input shape is changed,
+ # the input dimension of this layer has to be changed accordingly.
+ self.fc = nn.Sequential(
+ nn.Linear(256 * 16 * 8, 4096),
+ nn.BatchNorm1d(4096),
+ nn.ReLU(),
+ )
+ self.classifier = nn.Linear(4096, num_classes)
+ self.feat_dim = 4096
+
+ def featuremaps(self, x):
+ x = self.block1(x)
+ x = self.block2(x)
+ x = self.block3(x)
+ x = self.block4(x)
+ x = self.block5(*x)
+ return x
+
+ def forward(self, x):
+ x = self.featuremaps(x)
+ x = x.view(x.size(0), -1)
+ x = self.fc(x)
+ y = self.classifier(x)
+
+ if not self.training:
+ return x
+
+ if self.loss == 'softmax':
+ return y
+ elif self.loss == 'triplet':
+ return y, x
+ else:
+ raise KeyError('Unsupported loss: {}'.format(self.loss))
diff --git a/trackers/strong_sort/deep/reid/torchreid/models/nasnet.py b/trackers/strong_sort/deep/reid/torchreid/models/nasnet.py
new file mode 100644
index 0000000000000000000000000000000000000000..b1f31def5515c3ba464c86cde471328b50c55b14
--- /dev/null
+++ b/trackers/strong_sort/deep/reid/torchreid/models/nasnet.py
@@ -0,0 +1,1131 @@
+from __future__ import division, absolute_import
+import torch
+import torch.nn as nn
+import torch.nn.functional as F
+import torch.utils.model_zoo as model_zoo
+
+__all__ = ['nasnetamobile']
+"""
+NASNet Mobile
+Thanks to Anastasiia (https://github.com/DagnyT) for the great help, support and motivation!
+
+
+------------------------------------------------------------------------------------
+ Architecture | Top-1 Acc | Top-5 Acc | Multiply-Adds | Params (M)
+------------------------------------------------------------------------------------
+| NASNet-A (4 @ 1056) | 74.08% | 91.74% | 564 M | 5.3 |
+------------------------------------------------------------------------------------
+# References:
+ - [Learning Transferable Architectures for Scalable Image Recognition]
+ (https://arxiv.org/abs/1707.07012)
+"""
+"""
+Code imported from https://github.com/Cadene/pretrained-models.pytorch
+"""
+
+pretrained_settings = {
+ 'nasnetamobile': {
+ 'imagenet': {
+ # 'url': 'https://github.com/veronikayurchuk/pretrained-models.pytorch/releases/download/v1.0/nasnetmobile-7e03cead.pth.tar',
+ 'url':
+ 'http://data.lip6.fr/cadene/pretrainedmodels/nasnetamobile-7e03cead.pth',
+ 'input_space': 'RGB',
+ 'input_size': [3, 224, 224], # resize 256
+ 'input_range': [0, 1],
+ 'mean': [0.5, 0.5, 0.5],
+ 'std': [0.5, 0.5, 0.5],
+ 'num_classes': 1000
+ },
+ # 'imagenet+background': {
+ # # 'url': 'http://data.lip6.fr/cadene/pretrainedmodels/nasnetalarge-a1897284.pth',
+ # 'input_space': 'RGB',
+ # 'input_size': [3, 224, 224], # resize 256
+ # 'input_range': [0, 1],
+ # 'mean': [0.5, 0.5, 0.5],
+ # 'std': [0.5, 0.5, 0.5],
+ # 'num_classes': 1001
+ # }
+ }
+}
+
+
+class MaxPoolPad(nn.Module):
+
+ def __init__(self):
+ super(MaxPoolPad, self).__init__()
+ self.pad = nn.ZeroPad2d((1, 0, 1, 0))
+ self.pool = nn.MaxPool2d(3, stride=2, padding=1)
+
+ def forward(self, x):
+ x = self.pad(x)
+ x = self.pool(x)
+ x = x[:, :, 1:, 1:].contiguous()
+ return x
+
+
+class AvgPoolPad(nn.Module):
+
+ def __init__(self, stride=2, padding=1):
+ super(AvgPoolPad, self).__init__()
+ self.pad = nn.ZeroPad2d((1, 0, 1, 0))
+ self.pool = nn.AvgPool2d(
+ 3, stride=stride, padding=padding, count_include_pad=False
+ )
+
+ def forward(self, x):
+ x = self.pad(x)
+ x = self.pool(x)
+ x = x[:, :, 1:, 1:].contiguous()
+ return x
+
+
+class SeparableConv2d(nn.Module):
+
+ def __init__(
+ self,
+ in_channels,
+ out_channels,
+ dw_kernel,
+ dw_stride,
+ dw_padding,
+ bias=False
+ ):
+ super(SeparableConv2d, self).__init__()
+ self.depthwise_conv2d = nn.Conv2d(
+ in_channels,
+ in_channels,
+ dw_kernel,
+ stride=dw_stride,
+ padding=dw_padding,
+ bias=bias,
+ groups=in_channels
+ )
+ self.pointwise_conv2d = nn.Conv2d(
+ in_channels, out_channels, 1, stride=1, bias=bias
+ )
+
+ def forward(self, x):
+ x = self.depthwise_conv2d(x)
+ x = self.pointwise_conv2d(x)
+ return x
+
+
+class BranchSeparables(nn.Module):
+
+ def __init__(
+ self,
+ in_channels,
+ out_channels,
+ kernel_size,
+ stride,
+ padding,
+ name=None,
+ bias=False
+ ):
+ super(BranchSeparables, self).__init__()
+ self.relu = nn.ReLU()
+ self.separable_1 = SeparableConv2d(
+ in_channels, in_channels, kernel_size, stride, padding, bias=bias
+ )
+ self.bn_sep_1 = nn.BatchNorm2d(
+ in_channels, eps=0.001, momentum=0.1, affine=True
+ )
+ self.relu1 = nn.ReLU()
+ self.separable_2 = SeparableConv2d(
+ in_channels, out_channels, kernel_size, 1, padding, bias=bias
+ )
+ self.bn_sep_2 = nn.BatchNorm2d(
+ out_channels, eps=0.001, momentum=0.1, affine=True
+ )
+ self.name = name
+
+ def forward(self, x):
+ x = self.relu(x)
+ if self.name == 'specific':
+ x = nn.ZeroPad2d((1, 0, 1, 0))(x)
+ x = self.separable_1(x)
+ if self.name == 'specific':
+ x = x[:, :, 1:, 1:].contiguous()
+
+ x = self.bn_sep_1(x)
+ x = self.relu1(x)
+ x = self.separable_2(x)
+ x = self.bn_sep_2(x)
+ return x
+
+
+class BranchSeparablesStem(nn.Module):
+
+ def __init__(
+ self,
+ in_channels,
+ out_channels,
+ kernel_size,
+ stride,
+ padding,
+ bias=False
+ ):
+ super(BranchSeparablesStem, self).__init__()
+ self.relu = nn.ReLU()
+ self.separable_1 = SeparableConv2d(
+ in_channels, out_channels, kernel_size, stride, padding, bias=bias
+ )
+ self.bn_sep_1 = nn.BatchNorm2d(
+ out_channels, eps=0.001, momentum=0.1, affine=True
+ )
+ self.relu1 = nn.ReLU()
+ self.separable_2 = SeparableConv2d(
+ out_channels, out_channels, kernel_size, 1, padding, bias=bias
+ )
+ self.bn_sep_2 = nn.BatchNorm2d(
+ out_channels, eps=0.001, momentum=0.1, affine=True
+ )
+
+ def forward(self, x):
+ x = self.relu(x)
+ x = self.separable_1(x)
+ x = self.bn_sep_1(x)
+ x = self.relu1(x)
+ x = self.separable_2(x)
+ x = self.bn_sep_2(x)
+ return x
+
+
+class BranchSeparablesReduction(BranchSeparables):
+
+ def __init__(
+ self,
+ in_channels,
+ out_channels,
+ kernel_size,
+ stride,
+ padding,
+ z_padding=1,
+ bias=False
+ ):
+ BranchSeparables.__init__(
+ self, in_channels, out_channels, kernel_size, stride, padding, bias
+ )
+ self.padding = nn.ZeroPad2d((z_padding, 0, z_padding, 0))
+
+ def forward(self, x):
+ x = self.relu(x)
+ x = self.padding(x)
+ x = self.separable_1(x)
+ x = x[:, :, 1:, 1:].contiguous()
+ x = self.bn_sep_1(x)
+ x = self.relu1(x)
+ x = self.separable_2(x)
+ x = self.bn_sep_2(x)
+ return x
+
+
+class CellStem0(nn.Module):
+
+ def __init__(self, stem_filters, num_filters=42):
+ super(CellStem0, self).__init__()
+ self.num_filters = num_filters
+ self.stem_filters = stem_filters
+ self.conv_1x1 = nn.Sequential()
+ self.conv_1x1.add_module('relu', nn.ReLU())
+ self.conv_1x1.add_module(
+ 'conv',
+ nn.Conv2d(
+ self.stem_filters, self.num_filters, 1, stride=1, bias=False
+ )
+ )
+ self.conv_1x1.add_module(
+ 'bn',
+ nn.BatchNorm2d(
+ self.num_filters, eps=0.001, momentum=0.1, affine=True
+ )
+ )
+
+ self.comb_iter_0_left = BranchSeparables(
+ self.num_filters, self.num_filters, 5, 2, 2
+ )
+ self.comb_iter_0_right = BranchSeparablesStem(
+ self.stem_filters, self.num_filters, 7, 2, 3, bias=False
+ )
+
+ self.comb_iter_1_left = nn.MaxPool2d(3, stride=2, padding=1)
+ self.comb_iter_1_right = BranchSeparablesStem(
+ self.stem_filters, self.num_filters, 7, 2, 3, bias=False
+ )
+
+ self.comb_iter_2_left = nn.AvgPool2d(
+ 3, stride=2, padding=1, count_include_pad=False
+ )
+ self.comb_iter_2_right = BranchSeparablesStem(
+ self.stem_filters, self.num_filters, 5, 2, 2, bias=False
+ )
+
+ self.comb_iter_3_right = nn.AvgPool2d(
+ 3, stride=1, padding=1, count_include_pad=False
+ )
+
+ self.comb_iter_4_left = BranchSeparables(
+ self.num_filters, self.num_filters, 3, 1, 1, bias=False
+ )
+ self.comb_iter_4_right = nn.MaxPool2d(3, stride=2, padding=1)
+
+ def forward(self, x):
+ x1 = self.conv_1x1(x)
+
+ x_comb_iter_0_left = self.comb_iter_0_left(x1)
+ x_comb_iter_0_right = self.comb_iter_0_right(x)
+ x_comb_iter_0 = x_comb_iter_0_left + x_comb_iter_0_right
+
+ x_comb_iter_1_left = self.comb_iter_1_left(x1)
+ x_comb_iter_1_right = self.comb_iter_1_right(x)
+ x_comb_iter_1 = x_comb_iter_1_left + x_comb_iter_1_right
+
+ x_comb_iter_2_left = self.comb_iter_2_left(x1)
+ x_comb_iter_2_right = self.comb_iter_2_right(x)
+ x_comb_iter_2 = x_comb_iter_2_left + x_comb_iter_2_right
+
+ x_comb_iter_3_right = self.comb_iter_3_right(x_comb_iter_0)
+ x_comb_iter_3 = x_comb_iter_3_right + x_comb_iter_1
+
+ x_comb_iter_4_left = self.comb_iter_4_left(x_comb_iter_0)
+ x_comb_iter_4_right = self.comb_iter_4_right(x1)
+ x_comb_iter_4 = x_comb_iter_4_left + x_comb_iter_4_right
+
+ x_out = torch.cat(
+ [x_comb_iter_1, x_comb_iter_2, x_comb_iter_3, x_comb_iter_4], 1
+ )
+ return x_out
+
+
+class CellStem1(nn.Module):
+
+ def __init__(self, stem_filters, num_filters):
+ super(CellStem1, self).__init__()
+ self.num_filters = num_filters
+ self.stem_filters = stem_filters
+ self.conv_1x1 = nn.Sequential()
+ self.conv_1x1.add_module('relu', nn.ReLU())
+ self.conv_1x1.add_module(
+ 'conv',
+ nn.Conv2d(
+ 2 * self.num_filters,
+ self.num_filters,
+ 1,
+ stride=1,
+ bias=False
+ )
+ )
+ self.conv_1x1.add_module(
+ 'bn',
+ nn.BatchNorm2d(
+ self.num_filters, eps=0.001, momentum=0.1, affine=True
+ )
+ )
+
+ self.relu = nn.ReLU()
+ self.path_1 = nn.Sequential()
+ self.path_1.add_module(
+ 'avgpool', nn.AvgPool2d(1, stride=2, count_include_pad=False)
+ )
+ self.path_1.add_module(
+ 'conv',
+ nn.Conv2d(
+ self.stem_filters,
+ self.num_filters // 2,
+ 1,
+ stride=1,
+ bias=False
+ )
+ )
+ self.path_2 = nn.ModuleList()
+ self.path_2.add_module('pad', nn.ZeroPad2d((0, 1, 0, 1)))
+ self.path_2.add_module(
+ 'avgpool', nn.AvgPool2d(1, stride=2, count_include_pad=False)
+ )
+ self.path_2.add_module(
+ 'conv',
+ nn.Conv2d(
+ self.stem_filters,
+ self.num_filters // 2,
+ 1,
+ stride=1,
+ bias=False
+ )
+ )
+
+ self.final_path_bn = nn.BatchNorm2d(
+ self.num_filters, eps=0.001, momentum=0.1, affine=True
+ )
+
+ self.comb_iter_0_left = BranchSeparables(
+ self.num_filters,
+ self.num_filters,
+ 5,
+ 2,
+ 2,
+ name='specific',
+ bias=False
+ )
+ self.comb_iter_0_right = BranchSeparables(
+ self.num_filters,
+ self.num_filters,
+ 7,
+ 2,
+ 3,
+ name='specific',
+ bias=False
+ )
+
+ # self.comb_iter_1_left = nn.MaxPool2d(3, stride=2, padding=1)
+ self.comb_iter_1_left = MaxPoolPad()
+ self.comb_iter_1_right = BranchSeparables(
+ self.num_filters,
+ self.num_filters,
+ 7,
+ 2,
+ 3,
+ name='specific',
+ bias=False
+ )
+
+ # self.comb_iter_2_left = nn.AvgPool2d(3, stride=2, padding=1, count_include_pad=False)
+ self.comb_iter_2_left = AvgPoolPad()
+ self.comb_iter_2_right = BranchSeparables(
+ self.num_filters,
+ self.num_filters,
+ 5,
+ 2,
+ 2,
+ name='specific',
+ bias=False
+ )
+
+ self.comb_iter_3_right = nn.AvgPool2d(
+ 3, stride=1, padding=1, count_include_pad=False
+ )
+
+ self.comb_iter_4_left = BranchSeparables(
+ self.num_filters,
+ self.num_filters,
+ 3,
+ 1,
+ 1,
+ name='specific',
+ bias=False
+ )
+ # self.comb_iter_4_right = nn.MaxPool2d(3, stride=2, padding=1)
+ self.comb_iter_4_right = MaxPoolPad()
+
+ def forward(self, x_conv0, x_stem_0):
+ x_left = self.conv_1x1(x_stem_0)
+
+ x_relu = self.relu(x_conv0)
+ # path 1
+ x_path1 = self.path_1(x_relu)
+ # path 2
+ x_path2 = self.path_2.pad(x_relu)
+ x_path2 = x_path2[:, :, 1:, 1:]
+ x_path2 = self.path_2.avgpool(x_path2)
+ x_path2 = self.path_2.conv(x_path2)
+ # final path
+ x_right = self.final_path_bn(torch.cat([x_path1, x_path2], 1))
+
+ x_comb_iter_0_left = self.comb_iter_0_left(x_left)
+ x_comb_iter_0_right = self.comb_iter_0_right(x_right)
+ x_comb_iter_0 = x_comb_iter_0_left + x_comb_iter_0_right
+
+ x_comb_iter_1_left = self.comb_iter_1_left(x_left)
+ x_comb_iter_1_right = self.comb_iter_1_right(x_right)
+ x_comb_iter_1 = x_comb_iter_1_left + x_comb_iter_1_right
+
+ x_comb_iter_2_left = self.comb_iter_2_left(x_left)
+ x_comb_iter_2_right = self.comb_iter_2_right(x_right)
+ x_comb_iter_2 = x_comb_iter_2_left + x_comb_iter_2_right
+
+ x_comb_iter_3_right = self.comb_iter_3_right(x_comb_iter_0)
+ x_comb_iter_3 = x_comb_iter_3_right + x_comb_iter_1
+
+ x_comb_iter_4_left = self.comb_iter_4_left(x_comb_iter_0)
+ x_comb_iter_4_right = self.comb_iter_4_right(x_left)
+ x_comb_iter_4 = x_comb_iter_4_left + x_comb_iter_4_right
+
+ x_out = torch.cat(
+ [x_comb_iter_1, x_comb_iter_2, x_comb_iter_3, x_comb_iter_4], 1
+ )
+ return x_out
+
+
+class FirstCell(nn.Module):
+
+ def __init__(
+ self, in_channels_left, out_channels_left, in_channels_right,
+ out_channels_right
+ ):
+ super(FirstCell, self).__init__()
+ self.conv_1x1 = nn.Sequential()
+ self.conv_1x1.add_module('relu', nn.ReLU())
+ self.conv_1x1.add_module(
+ 'conv',
+ nn.Conv2d(
+ in_channels_right, out_channels_right, 1, stride=1, bias=False
+ )
+ )
+ self.conv_1x1.add_module(
+ 'bn',
+ nn.BatchNorm2d(
+ out_channels_right, eps=0.001, momentum=0.1, affine=True
+ )
+ )
+
+ self.relu = nn.ReLU()
+ self.path_1 = nn.Sequential()
+ self.path_1.add_module(
+ 'avgpool', nn.AvgPool2d(1, stride=2, count_include_pad=False)
+ )
+ self.path_1.add_module(
+ 'conv',
+ nn.Conv2d(
+ in_channels_left, out_channels_left, 1, stride=1, bias=False
+ )
+ )
+ self.path_2 = nn.ModuleList()
+ self.path_2.add_module('pad', nn.ZeroPad2d((0, 1, 0, 1)))
+ self.path_2.add_module(
+ 'avgpool', nn.AvgPool2d(1, stride=2, count_include_pad=False)
+ )
+ self.path_2.add_module(
+ 'conv',
+ nn.Conv2d(
+ in_channels_left, out_channels_left, 1, stride=1, bias=False
+ )
+ )
+
+ self.final_path_bn = nn.BatchNorm2d(
+ out_channels_left * 2, eps=0.001, momentum=0.1, affine=True
+ )
+
+ self.comb_iter_0_left = BranchSeparables(
+ out_channels_right, out_channels_right, 5, 1, 2, bias=False
+ )
+ self.comb_iter_0_right = BranchSeparables(
+ out_channels_right, out_channels_right, 3, 1, 1, bias=False
+ )
+
+ self.comb_iter_1_left = BranchSeparables(
+ out_channels_right, out_channels_right, 5, 1, 2, bias=False
+ )
+ self.comb_iter_1_right = BranchSeparables(
+ out_channels_right, out_channels_right, 3, 1, 1, bias=False
+ )
+
+ self.comb_iter_2_left = nn.AvgPool2d(
+ 3, stride=1, padding=1, count_include_pad=False
+ )
+
+ self.comb_iter_3_left = nn.AvgPool2d(
+ 3, stride=1, padding=1, count_include_pad=False
+ )
+ self.comb_iter_3_right = nn.AvgPool2d(
+ 3, stride=1, padding=1, count_include_pad=False
+ )
+
+ self.comb_iter_4_left = BranchSeparables(
+ out_channels_right, out_channels_right, 3, 1, 1, bias=False
+ )
+
+ def forward(self, x, x_prev):
+ x_relu = self.relu(x_prev)
+ # path 1
+ x_path1 = self.path_1(x_relu)
+ # path 2
+ x_path2 = self.path_2.pad(x_relu)
+ x_path2 = x_path2[:, :, 1:, 1:]
+ x_path2 = self.path_2.avgpool(x_path2)
+ x_path2 = self.path_2.conv(x_path2)
+ # final path
+ x_left = self.final_path_bn(torch.cat([x_path1, x_path2], 1))
+
+ x_right = self.conv_1x1(x)
+
+ x_comb_iter_0_left = self.comb_iter_0_left(x_right)
+ x_comb_iter_0_right = self.comb_iter_0_right(x_left)
+ x_comb_iter_0 = x_comb_iter_0_left + x_comb_iter_0_right
+
+ x_comb_iter_1_left = self.comb_iter_1_left(x_left)
+ x_comb_iter_1_right = self.comb_iter_1_right(x_left)
+ x_comb_iter_1 = x_comb_iter_1_left + x_comb_iter_1_right
+
+ x_comb_iter_2_left = self.comb_iter_2_left(x_right)
+ x_comb_iter_2 = x_comb_iter_2_left + x_left
+
+ x_comb_iter_3_left = self.comb_iter_3_left(x_left)
+ x_comb_iter_3_right = self.comb_iter_3_right(x_left)
+ x_comb_iter_3 = x_comb_iter_3_left + x_comb_iter_3_right
+
+ x_comb_iter_4_left = self.comb_iter_4_left(x_right)
+ x_comb_iter_4 = x_comb_iter_4_left + x_right
+
+ x_out = torch.cat(
+ [
+ x_left, x_comb_iter_0, x_comb_iter_1, x_comb_iter_2,
+ x_comb_iter_3, x_comb_iter_4
+ ], 1
+ )
+ return x_out
+
+
+class NormalCell(nn.Module):
+
+ def __init__(
+ self, in_channels_left, out_channels_left, in_channels_right,
+ out_channels_right
+ ):
+ super(NormalCell, self).__init__()
+ self.conv_prev_1x1 = nn.Sequential()
+ self.conv_prev_1x1.add_module('relu', nn.ReLU())
+ self.conv_prev_1x1.add_module(
+ 'conv',
+ nn.Conv2d(
+ in_channels_left, out_channels_left, 1, stride=1, bias=False
+ )
+ )
+ self.conv_prev_1x1.add_module(
+ 'bn',
+ nn.BatchNorm2d(
+ out_channels_left, eps=0.001, momentum=0.1, affine=True
+ )
+ )
+
+ self.conv_1x1 = nn.Sequential()
+ self.conv_1x1.add_module('relu', nn.ReLU())
+ self.conv_1x1.add_module(
+ 'conv',
+ nn.Conv2d(
+ in_channels_right, out_channels_right, 1, stride=1, bias=False
+ )
+ )
+ self.conv_1x1.add_module(
+ 'bn',
+ nn.BatchNorm2d(
+ out_channels_right, eps=0.001, momentum=0.1, affine=True
+ )
+ )
+
+ self.comb_iter_0_left = BranchSeparables(
+ out_channels_right, out_channels_right, 5, 1, 2, bias=False
+ )
+ self.comb_iter_0_right = BranchSeparables(
+ out_channels_left, out_channels_left, 3, 1, 1, bias=False
+ )
+
+ self.comb_iter_1_left = BranchSeparables(
+ out_channels_left, out_channels_left, 5, 1, 2, bias=False
+ )
+ self.comb_iter_1_right = BranchSeparables(
+ out_channels_left, out_channels_left, 3, 1, 1, bias=False
+ )
+
+ self.comb_iter_2_left = nn.AvgPool2d(
+ 3, stride=1, padding=1, count_include_pad=False
+ )
+
+ self.comb_iter_3_left = nn.AvgPool2d(
+ 3, stride=1, padding=1, count_include_pad=False
+ )
+ self.comb_iter_3_right = nn.AvgPool2d(
+ 3, stride=1, padding=1, count_include_pad=False
+ )
+
+ self.comb_iter_4_left = BranchSeparables(
+ out_channels_right, out_channels_right, 3, 1, 1, bias=False
+ )
+
+ def forward(self, x, x_prev):
+ x_left = self.conv_prev_1x1(x_prev)
+ x_right = self.conv_1x1(x)
+
+ x_comb_iter_0_left = self.comb_iter_0_left(x_right)
+ x_comb_iter_0_right = self.comb_iter_0_right(x_left)
+ x_comb_iter_0 = x_comb_iter_0_left + x_comb_iter_0_right
+
+ x_comb_iter_1_left = self.comb_iter_1_left(x_left)
+ x_comb_iter_1_right = self.comb_iter_1_right(x_left)
+ x_comb_iter_1 = x_comb_iter_1_left + x_comb_iter_1_right
+
+ x_comb_iter_2_left = self.comb_iter_2_left(x_right)
+ x_comb_iter_2 = x_comb_iter_2_left + x_left
+
+ x_comb_iter_3_left = self.comb_iter_3_left(x_left)
+ x_comb_iter_3_right = self.comb_iter_3_right(x_left)
+ x_comb_iter_3 = x_comb_iter_3_left + x_comb_iter_3_right
+
+ x_comb_iter_4_left = self.comb_iter_4_left(x_right)
+ x_comb_iter_4 = x_comb_iter_4_left + x_right
+
+ x_out = torch.cat(
+ [
+ x_left, x_comb_iter_0, x_comb_iter_1, x_comb_iter_2,
+ x_comb_iter_3, x_comb_iter_4
+ ], 1
+ )
+ return x_out
+
+
+class ReductionCell0(nn.Module):
+
+ def __init__(
+ self, in_channels_left, out_channels_left, in_channels_right,
+ out_channels_right
+ ):
+ super(ReductionCell0, self).__init__()
+ self.conv_prev_1x1 = nn.Sequential()
+ self.conv_prev_1x1.add_module('relu', nn.ReLU())
+ self.conv_prev_1x1.add_module(
+ 'conv',
+ nn.Conv2d(
+ in_channels_left, out_channels_left, 1, stride=1, bias=False
+ )
+ )
+ self.conv_prev_1x1.add_module(
+ 'bn',
+ nn.BatchNorm2d(
+ out_channels_left, eps=0.001, momentum=0.1, affine=True
+ )
+ )
+
+ self.conv_1x1 = nn.Sequential()
+ self.conv_1x1.add_module('relu', nn.ReLU())
+ self.conv_1x1.add_module(
+ 'conv',
+ nn.Conv2d(
+ in_channels_right, out_channels_right, 1, stride=1, bias=False
+ )
+ )
+ self.conv_1x1.add_module(
+ 'bn',
+ nn.BatchNorm2d(
+ out_channels_right, eps=0.001, momentum=0.1, affine=True
+ )
+ )
+
+ self.comb_iter_0_left = BranchSeparablesReduction(
+ out_channels_right, out_channels_right, 5, 2, 2, bias=False
+ )
+ self.comb_iter_0_right = BranchSeparablesReduction(
+ out_channels_right, out_channels_right, 7, 2, 3, bias=False
+ )
+
+ self.comb_iter_1_left = MaxPoolPad()
+ self.comb_iter_1_right = BranchSeparablesReduction(
+ out_channels_right, out_channels_right, 7, 2, 3, bias=False
+ )
+
+ self.comb_iter_2_left = AvgPoolPad()
+ self.comb_iter_2_right = BranchSeparablesReduction(
+ out_channels_right, out_channels_right, 5, 2, 2, bias=False
+ )
+
+ self.comb_iter_3_right = nn.AvgPool2d(
+ 3, stride=1, padding=1, count_include_pad=False
+ )
+
+ self.comb_iter_4_left = BranchSeparablesReduction(
+ out_channels_right, out_channels_right, 3, 1, 1, bias=False
+ )
+ self.comb_iter_4_right = MaxPoolPad()
+
+ def forward(self, x, x_prev):
+ x_left = self.conv_prev_1x1(x_prev)
+ x_right = self.conv_1x1(x)
+
+ x_comb_iter_0_left = self.comb_iter_0_left(x_right)
+ x_comb_iter_0_right = self.comb_iter_0_right(x_left)
+ x_comb_iter_0 = x_comb_iter_0_left + x_comb_iter_0_right
+
+ x_comb_iter_1_left = self.comb_iter_1_left(x_right)
+ x_comb_iter_1_right = self.comb_iter_1_right(x_left)
+ x_comb_iter_1 = x_comb_iter_1_left + x_comb_iter_1_right
+
+ x_comb_iter_2_left = self.comb_iter_2_left(x_right)
+ x_comb_iter_2_right = self.comb_iter_2_right(x_left)
+ x_comb_iter_2 = x_comb_iter_2_left + x_comb_iter_2_right
+
+ x_comb_iter_3_right = self.comb_iter_3_right(x_comb_iter_0)
+ x_comb_iter_3 = x_comb_iter_3_right + x_comb_iter_1
+
+ x_comb_iter_4_left = self.comb_iter_4_left(x_comb_iter_0)
+ x_comb_iter_4_right = self.comb_iter_4_right(x_right)
+ x_comb_iter_4 = x_comb_iter_4_left + x_comb_iter_4_right
+
+ x_out = torch.cat(
+ [x_comb_iter_1, x_comb_iter_2, x_comb_iter_3, x_comb_iter_4], 1
+ )
+ return x_out
+
+
+class ReductionCell1(nn.Module):
+
+ def __init__(
+ self, in_channels_left, out_channels_left, in_channels_right,
+ out_channels_right
+ ):
+ super(ReductionCell1, self).__init__()
+ self.conv_prev_1x1 = nn.Sequential()
+ self.conv_prev_1x1.add_module('relu', nn.ReLU())
+ self.conv_prev_1x1.add_module(
+ 'conv',
+ nn.Conv2d(
+ in_channels_left, out_channels_left, 1, stride=1, bias=False
+ )
+ )
+ self.conv_prev_1x1.add_module(
+ 'bn',
+ nn.BatchNorm2d(
+ out_channels_left, eps=0.001, momentum=0.1, affine=True
+ )
+ )
+
+ self.conv_1x1 = nn.Sequential()
+ self.conv_1x1.add_module('relu', nn.ReLU())
+ self.conv_1x1.add_module(
+ 'conv',
+ nn.Conv2d(
+ in_channels_right, out_channels_right, 1, stride=1, bias=False
+ )
+ )
+ self.conv_1x1.add_module(
+ 'bn',
+ nn.BatchNorm2d(
+ out_channels_right, eps=0.001, momentum=0.1, affine=True
+ )
+ )
+
+ self.comb_iter_0_left = BranchSeparables(
+ out_channels_right,
+ out_channels_right,
+ 5,
+ 2,
+ 2,
+ name='specific',
+ bias=False
+ )
+ self.comb_iter_0_right = BranchSeparables(
+ out_channels_right,
+ out_channels_right,
+ 7,
+ 2,
+ 3,
+ name='specific',
+ bias=False
+ )
+
+ # self.comb_iter_1_left = nn.MaxPool2d(3, stride=2, padding=1)
+ self.comb_iter_1_left = MaxPoolPad()
+ self.comb_iter_1_right = BranchSeparables(
+ out_channels_right,
+ out_channels_right,
+ 7,
+ 2,
+ 3,
+ name='specific',
+ bias=False
+ )
+
+ # self.comb_iter_2_left = nn.AvgPool2d(3, stride=2, padding=1, count_include_pad=False)
+ self.comb_iter_2_left = AvgPoolPad()
+ self.comb_iter_2_right = BranchSeparables(
+ out_channels_right,
+ out_channels_right,
+ 5,
+ 2,
+ 2,
+ name='specific',
+ bias=False
+ )
+
+ self.comb_iter_3_right = nn.AvgPool2d(
+ 3, stride=1, padding=1, count_include_pad=False
+ )
+
+ self.comb_iter_4_left = BranchSeparables(
+ out_channels_right,
+ out_channels_right,
+ 3,
+ 1,
+ 1,
+ name='specific',
+ bias=False
+ )
+ # self.comb_iter_4_right = nn.MaxPool2d(3, stride=2, padding=1)
+ self.comb_iter_4_right = MaxPoolPad()
+
+ def forward(self, x, x_prev):
+ x_left = self.conv_prev_1x1(x_prev)
+ x_right = self.conv_1x1(x)
+
+ x_comb_iter_0_left = self.comb_iter_0_left(x_right)
+ x_comb_iter_0_right = self.comb_iter_0_right(x_left)
+ x_comb_iter_0 = x_comb_iter_0_left + x_comb_iter_0_right
+
+ x_comb_iter_1_left = self.comb_iter_1_left(x_right)
+ x_comb_iter_1_right = self.comb_iter_1_right(x_left)
+ x_comb_iter_1 = x_comb_iter_1_left + x_comb_iter_1_right
+
+ x_comb_iter_2_left = self.comb_iter_2_left(x_right)
+ x_comb_iter_2_right = self.comb_iter_2_right(x_left)
+ x_comb_iter_2 = x_comb_iter_2_left + x_comb_iter_2_right
+
+ x_comb_iter_3_right = self.comb_iter_3_right(x_comb_iter_0)
+ x_comb_iter_3 = x_comb_iter_3_right + x_comb_iter_1
+
+ x_comb_iter_4_left = self.comb_iter_4_left(x_comb_iter_0)
+ x_comb_iter_4_right = self.comb_iter_4_right(x_right)
+ x_comb_iter_4 = x_comb_iter_4_left + x_comb_iter_4_right
+
+ x_out = torch.cat(
+ [x_comb_iter_1, x_comb_iter_2, x_comb_iter_3, x_comb_iter_4], 1
+ )
+ return x_out
+
+
+class NASNetAMobile(nn.Module):
+ """Neural Architecture Search (NAS).
+
+ Reference:
+ Zoph et al. Learning Transferable Architectures
+ for Scalable Image Recognition. CVPR 2018.
+
+ Public keys:
+ - ``nasnetamobile``: NASNet-A Mobile.
+ """
+
+ def __init__(
+ self,
+ num_classes,
+ loss,
+ stem_filters=32,
+ penultimate_filters=1056,
+ filters_multiplier=2,
+ **kwargs
+ ):
+ super(NASNetAMobile, self).__init__()
+ self.stem_filters = stem_filters
+ self.penultimate_filters = penultimate_filters
+ self.filters_multiplier = filters_multiplier
+ self.loss = loss
+
+ filters = self.penultimate_filters // 24
+ # 24 is default value for the architecture
+
+ self.conv0 = nn.Sequential()
+ self.conv0.add_module(
+ 'conv',
+ nn.Conv2d(
+ in_channels=3,
+ out_channels=self.stem_filters,
+ kernel_size=3,
+ padding=0,
+ stride=2,
+ bias=False
+ )
+ )
+ self.conv0.add_module(
+ 'bn',
+ nn.BatchNorm2d(
+ self.stem_filters, eps=0.001, momentum=0.1, affine=True
+ )
+ )
+
+ self.cell_stem_0 = CellStem0(
+ self.stem_filters, num_filters=filters // (filters_multiplier**2)
+ )
+ self.cell_stem_1 = CellStem1(
+ self.stem_filters, num_filters=filters // filters_multiplier
+ )
+
+ self.cell_0 = FirstCell(
+ in_channels_left=filters,
+ out_channels_left=filters // 2, # 1, 0.5
+ in_channels_right=2 * filters,
+ out_channels_right=filters
+ ) # 2, 1
+ self.cell_1 = NormalCell(
+ in_channels_left=2 * filters,
+ out_channels_left=filters, # 2, 1
+ in_channels_right=6 * filters,
+ out_channels_right=filters
+ ) # 6, 1
+ self.cell_2 = NormalCell(
+ in_channels_left=6 * filters,
+ out_channels_left=filters, # 6, 1
+ in_channels_right=6 * filters,
+ out_channels_right=filters
+ ) # 6, 1
+ self.cell_3 = NormalCell(
+ in_channels_left=6 * filters,
+ out_channels_left=filters, # 6, 1
+ in_channels_right=6 * filters,
+ out_channels_right=filters
+ ) # 6, 1
+
+ self.reduction_cell_0 = ReductionCell0(
+ in_channels_left=6 * filters,
+ out_channels_left=2 * filters, # 6, 2
+ in_channels_right=6 * filters,
+ out_channels_right=2 * filters
+ ) # 6, 2
+
+ self.cell_6 = FirstCell(
+ in_channels_left=6 * filters,
+ out_channels_left=filters, # 6, 1
+ in_channels_right=8 * filters,
+ out_channels_right=2 * filters
+ ) # 8, 2
+ self.cell_7 = NormalCell(
+ in_channels_left=8 * filters,
+ out_channels_left=2 * filters, # 8, 2
+ in_channels_right=12 * filters,
+ out_channels_right=2 * filters
+ ) # 12, 2
+ self.cell_8 = NormalCell(
+ in_channels_left=12 * filters,
+ out_channels_left=2 * filters, # 12, 2
+ in_channels_right=12 * filters,
+ out_channels_right=2 * filters
+ ) # 12, 2
+ self.cell_9 = NormalCell(
+ in_channels_left=12 * filters,
+ out_channels_left=2 * filters, # 12, 2
+ in_channels_right=12 * filters,
+ out_channels_right=2 * filters
+ ) # 12, 2
+
+ self.reduction_cell_1 = ReductionCell1(
+ in_channels_left=12 * filters,
+ out_channels_left=4 * filters, # 12, 4
+ in_channels_right=12 * filters,
+ out_channels_right=4 * filters
+ ) # 12, 4
+
+ self.cell_12 = FirstCell(
+ in_channels_left=12 * filters,
+ out_channels_left=2 * filters, # 12, 2
+ in_channels_right=16 * filters,
+ out_channels_right=4 * filters
+ ) # 16, 4
+ self.cell_13 = NormalCell(
+ in_channels_left=16 * filters,
+ out_channels_left=4 * filters, # 16, 4
+ in_channels_right=24 * filters,
+ out_channels_right=4 * filters
+ ) # 24, 4
+ self.cell_14 = NormalCell(
+ in_channels_left=24 * filters,
+ out_channels_left=4 * filters, # 24, 4
+ in_channels_right=24 * filters,
+ out_channels_right=4 * filters
+ ) # 24, 4
+ self.cell_15 = NormalCell(
+ in_channels_left=24 * filters,
+ out_channels_left=4 * filters, # 24, 4
+ in_channels_right=24 * filters,
+ out_channels_right=4 * filters
+ ) # 24, 4
+
+ self.relu = nn.ReLU()
+ self.dropout = nn.Dropout()
+ self.classifier = nn.Linear(24 * filters, num_classes)
+
+ self._init_params()
+
+ def _init_params(self):
+ for m in self.modules():
+ if isinstance(m, nn.Conv2d):
+ nn.init.kaiming_normal_(
+ m.weight, mode='fan_out', nonlinearity='relu'
+ )
+ if m.bias is not None:
+ nn.init.constant_(m.bias, 0)
+ elif isinstance(m, nn.BatchNorm2d):
+ nn.init.constant_(m.weight, 1)
+ nn.init.constant_(m.bias, 0)
+ elif isinstance(m, nn.BatchNorm1d):
+ nn.init.constant_(m.weight, 1)
+ nn.init.constant_(m.bias, 0)
+ elif isinstance(m, nn.Linear):
+ nn.init.normal_(m.weight, 0, 0.01)
+ if m.bias is not None:
+ nn.init.constant_(m.bias, 0)
+
+ def features(self, input):
+ x_conv0 = self.conv0(input)
+ x_stem_0 = self.cell_stem_0(x_conv0)
+ x_stem_1 = self.cell_stem_1(x_conv0, x_stem_0)
+
+ x_cell_0 = self.cell_0(x_stem_1, x_stem_0)
+ x_cell_1 = self.cell_1(x_cell_0, x_stem_1)
+ x_cell_2 = self.cell_2(x_cell_1, x_cell_0)
+ x_cell_3 = self.cell_3(x_cell_2, x_cell_1)
+
+ x_reduction_cell_0 = self.reduction_cell_0(x_cell_3, x_cell_2)
+
+ x_cell_6 = self.cell_6(x_reduction_cell_0, x_cell_3)
+ x_cell_7 = self.cell_7(x_cell_6, x_reduction_cell_0)
+ x_cell_8 = self.cell_8(x_cell_7, x_cell_6)
+ x_cell_9 = self.cell_9(x_cell_8, x_cell_7)
+
+ x_reduction_cell_1 = self.reduction_cell_1(x_cell_9, x_cell_8)
+
+ x_cell_12 = self.cell_12(x_reduction_cell_1, x_cell_9)
+ x_cell_13 = self.cell_13(x_cell_12, x_reduction_cell_1)
+ x_cell_14 = self.cell_14(x_cell_13, x_cell_12)
+ x_cell_15 = self.cell_15(x_cell_14, x_cell_13)
+
+ x_cell_15 = self.relu(x_cell_15)
+ x_cell_15 = F.avg_pool2d(
+ x_cell_15,
+ x_cell_15.size()[2:]
+ ) # global average pool
+ x_cell_15 = x_cell_15.view(x_cell_15.size(0), -1)
+ x_cell_15 = self.dropout(x_cell_15)
+
+ return x_cell_15
+
+ def forward(self, input):
+ v = self.features(input)
+
+ if not self.training:
+ return v
+
+ y = self.classifier(v)
+
+ if self.loss == 'softmax':
+ return y
+ elif self.loss == 'triplet':
+ return y, v
+ else:
+ raise KeyError('Unsupported loss: {}'.format(self.loss))
+
+
+def init_pretrained_weights(model, model_url):
+ """Initializes model with pretrained weights.
+
+ Layers that don't match with pretrained layers in name or size are kept unchanged.
+ """
+ pretrain_dict = model_zoo.load_url(model_url)
+ model_dict = model.state_dict()
+ pretrain_dict = {
+ k: v
+ for k, v in pretrain_dict.items()
+ if k in model_dict and model_dict[k].size() == v.size()
+ }
+ model_dict.update(pretrain_dict)
+ model.load_state_dict(model_dict)
+
+
+def nasnetamobile(num_classes, loss='softmax', pretrained=True, **kwargs):
+ model = NASNetAMobile(num_classes, loss, **kwargs)
+ if pretrained:
+ model_url = pretrained_settings['nasnetamobile']['imagenet']['url']
+ init_pretrained_weights(model, model_url)
+ return model
diff --git a/trackers/strong_sort/deep/reid/torchreid/models/osnet.py b/trackers/strong_sort/deep/reid/torchreid/models/osnet.py
new file mode 100644
index 0000000000000000000000000000000000000000..b77388f13289f050da2bf2bdebd40ab4fce6f976
--- /dev/null
+++ b/trackers/strong_sort/deep/reid/torchreid/models/osnet.py
@@ -0,0 +1,598 @@
+from __future__ import division, absolute_import
+import warnings
+import torch
+from torch import nn
+from torch.nn import functional as F
+
+__all__ = [
+ 'osnet_x1_0', 'osnet_x0_75', 'osnet_x0_5', 'osnet_x0_25', 'osnet_ibn_x1_0'
+]
+
+pretrained_urls = {
+ 'osnet_x1_0':
+ 'https://drive.google.com/uc?id=1LaG1EJpHrxdAxKnSCJ_i0u-nbxSAeiFY',
+ 'osnet_x0_75':
+ 'https://drive.google.com/uc?id=1uwA9fElHOk3ZogwbeY5GkLI6QPTX70Hq',
+ 'osnet_x0_5':
+ 'https://drive.google.com/uc?id=16DGLbZukvVYgINws8u8deSaOqjybZ83i',
+ 'osnet_x0_25':
+ 'https://drive.google.com/uc?id=1rb8UN5ZzPKRc_xvtHlyDh-cSz88YX9hs',
+ 'osnet_ibn_x1_0':
+ 'https://drive.google.com/uc?id=1sr90V6irlYYDd4_4ISU2iruoRG8J__6l'
+}
+
+
+##########
+# Basic layers
+##########
+class ConvLayer(nn.Module):
+ """Convolution layer (conv + bn + relu)."""
+
+ def __init__(
+ self,
+ in_channels,
+ out_channels,
+ kernel_size,
+ stride=1,
+ padding=0,
+ groups=1,
+ IN=False
+ ):
+ super(ConvLayer, self).__init__()
+ self.conv = nn.Conv2d(
+ in_channels,
+ out_channels,
+ kernel_size,
+ stride=stride,
+ padding=padding,
+ bias=False,
+ groups=groups
+ )
+ if IN:
+ self.bn = nn.InstanceNorm2d(out_channels, affine=True)
+ else:
+ self.bn = nn.BatchNorm2d(out_channels)
+ self.relu = nn.ReLU(inplace=True)
+
+ def forward(self, x):
+ x = self.conv(x)
+ x = self.bn(x)
+ x = self.relu(x)
+ return x
+
+
+class Conv1x1(nn.Module):
+ """1x1 convolution + bn + relu."""
+
+ def __init__(self, in_channels, out_channels, stride=1, groups=1):
+ super(Conv1x1, self).__init__()
+ self.conv = nn.Conv2d(
+ in_channels,
+ out_channels,
+ 1,
+ stride=stride,
+ padding=0,
+ bias=False,
+ groups=groups
+ )
+ self.bn = nn.BatchNorm2d(out_channels)
+ self.relu = nn.ReLU(inplace=True)
+
+ def forward(self, x):
+ x = self.conv(x)
+ x = self.bn(x)
+ x = self.relu(x)
+ return x
+
+
+class Conv1x1Linear(nn.Module):
+ """1x1 convolution + bn (w/o non-linearity)."""
+
+ def __init__(self, in_channels, out_channels, stride=1):
+ super(Conv1x1Linear, self).__init__()
+ self.conv = nn.Conv2d(
+ in_channels, out_channels, 1, stride=stride, padding=0, bias=False
+ )
+ self.bn = nn.BatchNorm2d(out_channels)
+
+ def forward(self, x):
+ x = self.conv(x)
+ x = self.bn(x)
+ return x
+
+
+class Conv3x3(nn.Module):
+ """3x3 convolution + bn + relu."""
+
+ def __init__(self, in_channels, out_channels, stride=1, groups=1):
+ super(Conv3x3, self).__init__()
+ self.conv = nn.Conv2d(
+ in_channels,
+ out_channels,
+ 3,
+ stride=stride,
+ padding=1,
+ bias=False,
+ groups=groups
+ )
+ self.bn = nn.BatchNorm2d(out_channels)
+ self.relu = nn.ReLU(inplace=True)
+
+ def forward(self, x):
+ x = self.conv(x)
+ x = self.bn(x)
+ x = self.relu(x)
+ return x
+
+
+class LightConv3x3(nn.Module):
+ """Lightweight 3x3 convolution.
+
+ 1x1 (linear) + dw 3x3 (nonlinear).
+ """
+
+ def __init__(self, in_channels, out_channels):
+ super(LightConv3x3, self).__init__()
+ self.conv1 = nn.Conv2d(
+ in_channels, out_channels, 1, stride=1, padding=0, bias=False
+ )
+ self.conv2 = nn.Conv2d(
+ out_channels,
+ out_channels,
+ 3,
+ stride=1,
+ padding=1,
+ bias=False,
+ groups=out_channels
+ )
+ self.bn = nn.BatchNorm2d(out_channels)
+ self.relu = nn.ReLU(inplace=True)
+
+ def forward(self, x):
+ x = self.conv1(x)
+ x = self.conv2(x)
+ x = self.bn(x)
+ x = self.relu(x)
+ return x
+
+
+##########
+# Building blocks for omni-scale feature learning
+##########
+class ChannelGate(nn.Module):
+ """A mini-network that generates channel-wise gates conditioned on input tensor."""
+
+ def __init__(
+ self,
+ in_channels,
+ num_gates=None,
+ return_gates=False,
+ gate_activation='sigmoid',
+ reduction=16,
+ layer_norm=False
+ ):
+ super(ChannelGate, self).__init__()
+ if num_gates is None:
+ num_gates = in_channels
+ self.return_gates = return_gates
+ self.global_avgpool = nn.AdaptiveAvgPool2d(1)
+ self.fc1 = nn.Conv2d(
+ in_channels,
+ in_channels // reduction,
+ kernel_size=1,
+ bias=True,
+ padding=0
+ )
+ self.norm1 = None
+ if layer_norm:
+ self.norm1 = nn.LayerNorm((in_channels // reduction, 1, 1))
+ self.relu = nn.ReLU(inplace=True)
+ self.fc2 = nn.Conv2d(
+ in_channels // reduction,
+ num_gates,
+ kernel_size=1,
+ bias=True,
+ padding=0
+ )
+ if gate_activation == 'sigmoid':
+ self.gate_activation = nn.Sigmoid()
+ elif gate_activation == 'relu':
+ self.gate_activation = nn.ReLU(inplace=True)
+ elif gate_activation == 'linear':
+ self.gate_activation = None
+ else:
+ raise RuntimeError(
+ "Unknown gate activation: {}".format(gate_activation)
+ )
+
+ def forward(self, x):
+ input = x
+ x = self.global_avgpool(x)
+ x = self.fc1(x)
+ if self.norm1 is not None:
+ x = self.norm1(x)
+ x = self.relu(x)
+ x = self.fc2(x)
+ if self.gate_activation is not None:
+ x = self.gate_activation(x)
+ if self.return_gates:
+ return x
+ return input * x
+
+
+class OSBlock(nn.Module):
+ """Omni-scale feature learning block."""
+
+ def __init__(
+ self,
+ in_channels,
+ out_channels,
+ IN=False,
+ bottleneck_reduction=4,
+ **kwargs
+ ):
+ super(OSBlock, self).__init__()
+ mid_channels = out_channels // bottleneck_reduction
+ self.conv1 = Conv1x1(in_channels, mid_channels)
+ self.conv2a = LightConv3x3(mid_channels, mid_channels)
+ self.conv2b = nn.Sequential(
+ LightConv3x3(mid_channels, mid_channels),
+ LightConv3x3(mid_channels, mid_channels),
+ )
+ self.conv2c = nn.Sequential(
+ LightConv3x3(mid_channels, mid_channels),
+ LightConv3x3(mid_channels, mid_channels),
+ LightConv3x3(mid_channels, mid_channels),
+ )
+ self.conv2d = nn.Sequential(
+ LightConv3x3(mid_channels, mid_channels),
+ LightConv3x3(mid_channels, mid_channels),
+ LightConv3x3(mid_channels, mid_channels),
+ LightConv3x3(mid_channels, mid_channels),
+ )
+ self.gate = ChannelGate(mid_channels)
+ self.conv3 = Conv1x1Linear(mid_channels, out_channels)
+ self.downsample = None
+ if in_channels != out_channels:
+ self.downsample = Conv1x1Linear(in_channels, out_channels)
+ self.IN = None
+ if IN:
+ self.IN = nn.InstanceNorm2d(out_channels, affine=True)
+
+ def forward(self, x):
+ identity = x
+ x1 = self.conv1(x)
+ x2a = self.conv2a(x1)
+ x2b = self.conv2b(x1)
+ x2c = self.conv2c(x1)
+ x2d = self.conv2d(x1)
+ x2 = self.gate(x2a) + self.gate(x2b) + self.gate(x2c) + self.gate(x2d)
+ x3 = self.conv3(x2)
+ if self.downsample is not None:
+ identity = self.downsample(identity)
+ out = x3 + identity
+ if self.IN is not None:
+ out = self.IN(out)
+ return F.relu(out)
+
+
+##########
+# Network architecture
+##########
+class OSNet(nn.Module):
+ """Omni-Scale Network.
+
+ Reference:
+ - Zhou et al. Omni-Scale Feature Learning for Person Re-Identification. ICCV, 2019.
+ - Zhou et al. Learning Generalisable Omni-Scale Representations
+ for Person Re-Identification. TPAMI, 2021.
+ """
+
+ def __init__(
+ self,
+ num_classes,
+ blocks,
+ layers,
+ channels,
+ feature_dim=512,
+ loss='softmax',
+ IN=False,
+ **kwargs
+ ):
+ super(OSNet, self).__init__()
+ num_blocks = len(blocks)
+ assert num_blocks == len(layers)
+ assert num_blocks == len(channels) - 1
+ self.loss = loss
+ self.feature_dim = feature_dim
+
+ # convolutional backbone
+ self.conv1 = ConvLayer(3, channels[0], 7, stride=2, padding=3, IN=IN)
+ self.maxpool = nn.MaxPool2d(3, stride=2, padding=1)
+ self.conv2 = self._make_layer(
+ blocks[0],
+ layers[0],
+ channels[0],
+ channels[1],
+ reduce_spatial_size=True,
+ IN=IN
+ )
+ self.conv3 = self._make_layer(
+ blocks[1],
+ layers[1],
+ channels[1],
+ channels[2],
+ reduce_spatial_size=True
+ )
+ self.conv4 = self._make_layer(
+ blocks[2],
+ layers[2],
+ channels[2],
+ channels[3],
+ reduce_spatial_size=False
+ )
+ self.conv5 = Conv1x1(channels[3], channels[3])
+ self.global_avgpool = nn.AdaptiveAvgPool2d(1)
+ # fully connected layer
+ self.fc = self._construct_fc_layer(
+ self.feature_dim, channels[3], dropout_p=None
+ )
+ # identity classification layer
+ self.classifier = nn.Linear(self.feature_dim, num_classes)
+
+ self._init_params()
+
+ def _make_layer(
+ self,
+ block,
+ layer,
+ in_channels,
+ out_channels,
+ reduce_spatial_size,
+ IN=False
+ ):
+ layers = []
+
+ layers.append(block(in_channels, out_channels, IN=IN))
+ for i in range(1, layer):
+ layers.append(block(out_channels, out_channels, IN=IN))
+
+ if reduce_spatial_size:
+ layers.append(
+ nn.Sequential(
+ Conv1x1(out_channels, out_channels),
+ nn.AvgPool2d(2, stride=2)
+ )
+ )
+
+ return nn.Sequential(*layers)
+
+ def _construct_fc_layer(self, fc_dims, input_dim, dropout_p=None):
+ if fc_dims is None or fc_dims < 0:
+ self.feature_dim = input_dim
+ return None
+
+ if isinstance(fc_dims, int):
+ fc_dims = [fc_dims]
+
+ layers = []
+ for dim in fc_dims:
+ layers.append(nn.Linear(input_dim, dim))
+ layers.append(nn.BatchNorm1d(dim))
+ layers.append(nn.ReLU(inplace=True))
+ if dropout_p is not None:
+ layers.append(nn.Dropout(p=dropout_p))
+ input_dim = dim
+
+ self.feature_dim = fc_dims[-1]
+
+ return nn.Sequential(*layers)
+
+ def _init_params(self):
+ for m in self.modules():
+ if isinstance(m, nn.Conv2d):
+ nn.init.kaiming_normal_(
+ m.weight, mode='fan_out', nonlinearity='relu'
+ )
+ if m.bias is not None:
+ nn.init.constant_(m.bias, 0)
+
+ elif isinstance(m, nn.BatchNorm2d):
+ nn.init.constant_(m.weight, 1)
+ nn.init.constant_(m.bias, 0)
+
+ elif isinstance(m, nn.BatchNorm1d):
+ nn.init.constant_(m.weight, 1)
+ nn.init.constant_(m.bias, 0)
+
+ elif isinstance(m, nn.Linear):
+ nn.init.normal_(m.weight, 0, 0.01)
+ if m.bias is not None:
+ nn.init.constant_(m.bias, 0)
+
+ def featuremaps(self, x):
+ x = self.conv1(x)
+ x = self.maxpool(x)
+ x = self.conv2(x)
+ x = self.conv3(x)
+ x = self.conv4(x)
+ x = self.conv5(x)
+ return x
+
+ def forward(self, x, return_featuremaps=False):
+ x = self.featuremaps(x)
+ if return_featuremaps:
+ return x
+ v = self.global_avgpool(x)
+ v = v.view(v.size(0), -1)
+ if self.fc is not None:
+ v = self.fc(v)
+ if not self.training:
+ return v
+ y = self.classifier(v)
+ if self.loss == 'softmax':
+ return y
+ elif self.loss == 'triplet':
+ return y, v
+ else:
+ raise KeyError("Unsupported loss: {}".format(self.loss))
+
+
+def init_pretrained_weights(model, key=''):
+ """Initializes model with pretrained weights.
+
+ Layers that don't match with pretrained layers in name or size are kept unchanged.
+ """
+ import os
+ import errno
+ import gdown
+ from collections import OrderedDict
+
+ def _get_torch_home():
+ ENV_TORCH_HOME = 'TORCH_HOME'
+ ENV_XDG_CACHE_HOME = 'XDG_CACHE_HOME'
+ DEFAULT_CACHE_DIR = '~/.cache'
+ torch_home = os.path.expanduser(
+ os.getenv(
+ ENV_TORCH_HOME,
+ os.path.join(
+ os.getenv(ENV_XDG_CACHE_HOME, DEFAULT_CACHE_DIR), 'torch'
+ )
+ )
+ )
+ return torch_home
+
+ torch_home = _get_torch_home()
+ model_dir = os.path.join(torch_home, 'checkpoints')
+ try:
+ os.makedirs(model_dir)
+ except OSError as e:
+ if e.errno == errno.EEXIST:
+ # Directory already exists, ignore.
+ pass
+ else:
+ # Unexpected OSError, re-raise.
+ raise
+ filename = key + '_imagenet.pth'
+ cached_file = os.path.join(model_dir, filename)
+
+ if not os.path.exists(cached_file):
+ gdown.download(pretrained_urls[key], cached_file, quiet=False)
+
+ state_dict = torch.load(cached_file)
+ model_dict = model.state_dict()
+ new_state_dict = OrderedDict()
+ matched_layers, discarded_layers = [], []
+
+ for k, v in state_dict.items():
+ if k.startswith('module.'):
+ k = k[7:] # discard module.
+
+ if k in model_dict and model_dict[k].size() == v.size():
+ new_state_dict[k] = v
+ matched_layers.append(k)
+ else:
+ discarded_layers.append(k)
+
+ model_dict.update(new_state_dict)
+ model.load_state_dict(model_dict)
+
+ if len(matched_layers) == 0:
+ warnings.warn(
+ 'The pretrained weights from "{}" cannot be loaded, '
+ 'please check the key names manually '
+ '(** ignored and continue **)'.format(cached_file)
+ )
+ else:
+ print(
+ 'Successfully loaded imagenet pretrained weights from "{}"'.
+ format(cached_file)
+ )
+ if len(discarded_layers) > 0:
+ print(
+ '** The following layers are discarded '
+ 'due to unmatched keys or layer size: {}'.
+ format(discarded_layers)
+ )
+
+
+##########
+# Instantiation
+##########
+def osnet_x1_0(num_classes=1000, pretrained=True, loss='softmax', **kwargs):
+ # standard size (width x1.0)
+ model = OSNet(
+ num_classes,
+ blocks=[OSBlock, OSBlock, OSBlock],
+ layers=[2, 2, 2],
+ channels=[64, 256, 384, 512],
+ loss=loss,
+ **kwargs
+ )
+ if pretrained:
+ init_pretrained_weights(model, key='osnet_x1_0')
+ return model
+
+
+def osnet_x0_75(num_classes=1000, pretrained=True, loss='softmax', **kwargs):
+ # medium size (width x0.75)
+ model = OSNet(
+ num_classes,
+ blocks=[OSBlock, OSBlock, OSBlock],
+ layers=[2, 2, 2],
+ channels=[48, 192, 288, 384],
+ loss=loss,
+ **kwargs
+ )
+ if pretrained:
+ init_pretrained_weights(model, key='osnet_x0_75')
+ return model
+
+
+def osnet_x0_5(num_classes=1000, pretrained=True, loss='softmax', **kwargs):
+ # tiny size (width x0.5)
+ model = OSNet(
+ num_classes,
+ blocks=[OSBlock, OSBlock, OSBlock],
+ layers=[2, 2, 2],
+ channels=[32, 128, 192, 256],
+ loss=loss,
+ **kwargs
+ )
+ if pretrained:
+ init_pretrained_weights(model, key='osnet_x0_5')
+ return model
+
+
+def osnet_x0_25(num_classes=1000, pretrained=True, loss='softmax', **kwargs):
+ # very tiny size (width x0.25)
+ model = OSNet(
+ num_classes,
+ blocks=[OSBlock, OSBlock, OSBlock],
+ layers=[2, 2, 2],
+ channels=[16, 64, 96, 128],
+ loss=loss,
+ **kwargs
+ )
+ if pretrained:
+ init_pretrained_weights(model, key='osnet_x0_25')
+ return model
+
+
+def osnet_ibn_x1_0(
+ num_classes=1000, pretrained=True, loss='softmax', **kwargs
+):
+ # standard size (width x1.0) + IBN layer
+ # Ref: Pan et al. Two at Once: Enhancing Learning and Generalization Capacities via IBN-Net. ECCV, 2018.
+ model = OSNet(
+ num_classes,
+ blocks=[OSBlock, OSBlock, OSBlock],
+ layers=[2, 2, 2],
+ channels=[64, 256, 384, 512],
+ loss=loss,
+ IN=True,
+ **kwargs
+ )
+ if pretrained:
+ init_pretrained_weights(model, key='osnet_ibn_x1_0')
+ return model
diff --git a/trackers/strong_sort/deep/reid/torchreid/models/osnet_ain.py b/trackers/strong_sort/deep/reid/torchreid/models/osnet_ain.py
new file mode 100644
index 0000000000000000000000000000000000000000..3f9f7bd0704502401d499fd2bfdb802522b99efe
--- /dev/null
+++ b/trackers/strong_sort/deep/reid/torchreid/models/osnet_ain.py
@@ -0,0 +1,609 @@
+from __future__ import division, absolute_import
+import warnings
+import torch
+from torch import nn
+from torch.nn import functional as F
+
+__all__ = [
+ 'osnet_ain_x1_0', 'osnet_ain_x0_75', 'osnet_ain_x0_5', 'osnet_ain_x0_25'
+]
+
+pretrained_urls = {
+ 'osnet_ain_x1_0':
+ 'https://drive.google.com/uc?id=1-CaioD9NaqbHK_kzSMW8VE4_3KcsRjEo',
+ 'osnet_ain_x0_75':
+ 'https://drive.google.com/uc?id=1apy0hpsMypqstfencdH-jKIUEFOW4xoM',
+ 'osnet_ain_x0_5':
+ 'https://drive.google.com/uc?id=1KusKvEYyKGDTUBVRxRiz55G31wkihB6l',
+ 'osnet_ain_x0_25':
+ 'https://drive.google.com/uc?id=1SxQt2AvmEcgWNhaRb2xC4rP6ZwVDP0Wt'
+}
+
+
+##########
+# Basic layers
+##########
+class ConvLayer(nn.Module):
+ """Convolution layer (conv + bn + relu)."""
+
+ def __init__(
+ self,
+ in_channels,
+ out_channels,
+ kernel_size,
+ stride=1,
+ padding=0,
+ groups=1,
+ IN=False
+ ):
+ super(ConvLayer, self).__init__()
+ self.conv = nn.Conv2d(
+ in_channels,
+ out_channels,
+ kernel_size,
+ stride=stride,
+ padding=padding,
+ bias=False,
+ groups=groups
+ )
+ if IN:
+ self.bn = nn.InstanceNorm2d(out_channels, affine=True)
+ else:
+ self.bn = nn.BatchNorm2d(out_channels)
+ self.relu = nn.ReLU()
+
+ def forward(self, x):
+ x = self.conv(x)
+ x = self.bn(x)
+ return self.relu(x)
+
+
+class Conv1x1(nn.Module):
+ """1x1 convolution + bn + relu."""
+
+ def __init__(self, in_channels, out_channels, stride=1, groups=1):
+ super(Conv1x1, self).__init__()
+ self.conv = nn.Conv2d(
+ in_channels,
+ out_channels,
+ 1,
+ stride=stride,
+ padding=0,
+ bias=False,
+ groups=groups
+ )
+ self.bn = nn.BatchNorm2d(out_channels)
+ self.relu = nn.ReLU()
+
+ def forward(self, x):
+ x = self.conv(x)
+ x = self.bn(x)
+ return self.relu(x)
+
+
+class Conv1x1Linear(nn.Module):
+ """1x1 convolution + bn (w/o non-linearity)."""
+
+ def __init__(self, in_channels, out_channels, stride=1, bn=True):
+ super(Conv1x1Linear, self).__init__()
+ self.conv = nn.Conv2d(
+ in_channels, out_channels, 1, stride=stride, padding=0, bias=False
+ )
+ self.bn = None
+ if bn:
+ self.bn = nn.BatchNorm2d(out_channels)
+
+ def forward(self, x):
+ x = self.conv(x)
+ if self.bn is not None:
+ x = self.bn(x)
+ return x
+
+
+class Conv3x3(nn.Module):
+ """3x3 convolution + bn + relu."""
+
+ def __init__(self, in_channels, out_channels, stride=1, groups=1):
+ super(Conv3x3, self).__init__()
+ self.conv = nn.Conv2d(
+ in_channels,
+ out_channels,
+ 3,
+ stride=stride,
+ padding=1,
+ bias=False,
+ groups=groups
+ )
+ self.bn = nn.BatchNorm2d(out_channels)
+ self.relu = nn.ReLU()
+
+ def forward(self, x):
+ x = self.conv(x)
+ x = self.bn(x)
+ return self.relu(x)
+
+
+class LightConv3x3(nn.Module):
+ """Lightweight 3x3 convolution.
+
+ 1x1 (linear) + dw 3x3 (nonlinear).
+ """
+
+ def __init__(self, in_channels, out_channels):
+ super(LightConv3x3, self).__init__()
+ self.conv1 = nn.Conv2d(
+ in_channels, out_channels, 1, stride=1, padding=0, bias=False
+ )
+ self.conv2 = nn.Conv2d(
+ out_channels,
+ out_channels,
+ 3,
+ stride=1,
+ padding=1,
+ bias=False,
+ groups=out_channels
+ )
+ self.bn = nn.BatchNorm2d(out_channels)
+ self.relu = nn.ReLU()
+
+ def forward(self, x):
+ x = self.conv1(x)
+ x = self.conv2(x)
+ x = self.bn(x)
+ return self.relu(x)
+
+
+class LightConvStream(nn.Module):
+ """Lightweight convolution stream."""
+
+ def __init__(self, in_channels, out_channels, depth):
+ super(LightConvStream, self).__init__()
+ assert depth >= 1, 'depth must be equal to or larger than 1, but got {}'.format(
+ depth
+ )
+ layers = []
+ layers += [LightConv3x3(in_channels, out_channels)]
+ for i in range(depth - 1):
+ layers += [LightConv3x3(out_channels, out_channels)]
+ self.layers = nn.Sequential(*layers)
+
+ def forward(self, x):
+ return self.layers(x)
+
+
+##########
+# Building blocks for omni-scale feature learning
+##########
+class ChannelGate(nn.Module):
+ """A mini-network that generates channel-wise gates conditioned on input tensor."""
+
+ def __init__(
+ self,
+ in_channels,
+ num_gates=None,
+ return_gates=False,
+ gate_activation='sigmoid',
+ reduction=16,
+ layer_norm=False
+ ):
+ super(ChannelGate, self).__init__()
+ if num_gates is None:
+ num_gates = in_channels
+ self.return_gates = return_gates
+ self.global_avgpool = nn.AdaptiveAvgPool2d(1)
+ self.fc1 = nn.Conv2d(
+ in_channels,
+ in_channels // reduction,
+ kernel_size=1,
+ bias=True,
+ padding=0
+ )
+ self.norm1 = None
+ if layer_norm:
+ self.norm1 = nn.LayerNorm((in_channels // reduction, 1, 1))
+ self.relu = nn.ReLU()
+ self.fc2 = nn.Conv2d(
+ in_channels // reduction,
+ num_gates,
+ kernel_size=1,
+ bias=True,
+ padding=0
+ )
+ if gate_activation == 'sigmoid':
+ self.gate_activation = nn.Sigmoid()
+ elif gate_activation == 'relu':
+ self.gate_activation = nn.ReLU()
+ elif gate_activation == 'linear':
+ self.gate_activation = None
+ else:
+ raise RuntimeError(
+ "Unknown gate activation: {}".format(gate_activation)
+ )
+
+ def forward(self, x):
+ input = x
+ x = self.global_avgpool(x)
+ x = self.fc1(x)
+ if self.norm1 is not None:
+ x = self.norm1(x)
+ x = self.relu(x)
+ x = self.fc2(x)
+ if self.gate_activation is not None:
+ x = self.gate_activation(x)
+ if self.return_gates:
+ return x
+ return input * x
+
+
+class OSBlock(nn.Module):
+ """Omni-scale feature learning block."""
+
+ def __init__(self, in_channels, out_channels, reduction=4, T=4, **kwargs):
+ super(OSBlock, self).__init__()
+ assert T >= 1
+ assert out_channels >= reduction and out_channels % reduction == 0
+ mid_channels = out_channels // reduction
+
+ self.conv1 = Conv1x1(in_channels, mid_channels)
+ self.conv2 = nn.ModuleList()
+ for t in range(1, T + 1):
+ self.conv2 += [LightConvStream(mid_channels, mid_channels, t)]
+ self.gate = ChannelGate(mid_channels)
+ self.conv3 = Conv1x1Linear(mid_channels, out_channels)
+ self.downsample = None
+ if in_channels != out_channels:
+ self.downsample = Conv1x1Linear(in_channels, out_channels)
+
+ def forward(self, x):
+ identity = x
+ x1 = self.conv1(x)
+ x2 = 0
+ for conv2_t in self.conv2:
+ x2_t = conv2_t(x1)
+ x2 = x2 + self.gate(x2_t)
+ x3 = self.conv3(x2)
+ if self.downsample is not None:
+ identity = self.downsample(identity)
+ out = x3 + identity
+ return F.relu(out)
+
+
+class OSBlockINin(nn.Module):
+ """Omni-scale feature learning block with instance normalization."""
+
+ def __init__(self, in_channels, out_channels, reduction=4, T=4, **kwargs):
+ super(OSBlockINin, self).__init__()
+ assert T >= 1
+ assert out_channels >= reduction and out_channels % reduction == 0
+ mid_channels = out_channels // reduction
+
+ self.conv1 = Conv1x1(in_channels, mid_channels)
+ self.conv2 = nn.ModuleList()
+ for t in range(1, T + 1):
+ self.conv2 += [LightConvStream(mid_channels, mid_channels, t)]
+ self.gate = ChannelGate(mid_channels)
+ self.conv3 = Conv1x1Linear(mid_channels, out_channels, bn=False)
+ self.downsample = None
+ if in_channels != out_channels:
+ self.downsample = Conv1x1Linear(in_channels, out_channels)
+ self.IN = nn.InstanceNorm2d(out_channels, affine=True)
+
+ def forward(self, x):
+ identity = x
+ x1 = self.conv1(x)
+ x2 = 0
+ for conv2_t in self.conv2:
+ x2_t = conv2_t(x1)
+ x2 = x2 + self.gate(x2_t)
+ x3 = self.conv3(x2)
+ x3 = self.IN(x3) # IN inside residual
+ if self.downsample is not None:
+ identity = self.downsample(identity)
+ out = x3 + identity
+ return F.relu(out)
+
+
+##########
+# Network architecture
+##########
+class OSNet(nn.Module):
+ """Omni-Scale Network.
+
+ Reference:
+ - Zhou et al. Omni-Scale Feature Learning for Person Re-Identification. ICCV, 2019.
+ - Zhou et al. Learning Generalisable Omni-Scale Representations
+ for Person Re-Identification. TPAMI, 2021.
+ """
+
+ def __init__(
+ self,
+ num_classes,
+ blocks,
+ layers,
+ channels,
+ feature_dim=512,
+ loss='softmax',
+ conv1_IN=False,
+ **kwargs
+ ):
+ super(OSNet, self).__init__()
+ num_blocks = len(blocks)
+ assert num_blocks == len(layers)
+ assert num_blocks == len(channels) - 1
+ self.loss = loss
+ self.feature_dim = feature_dim
+
+ # convolutional backbone
+ self.conv1 = ConvLayer(
+ 3, channels[0], 7, stride=2, padding=3, IN=conv1_IN
+ )
+ self.maxpool = nn.MaxPool2d(3, stride=2, padding=1)
+ self.conv2 = self._make_layer(
+ blocks[0], layers[0], channels[0], channels[1]
+ )
+ self.pool2 = nn.Sequential(
+ Conv1x1(channels[1], channels[1]), nn.AvgPool2d(2, stride=2)
+ )
+ self.conv3 = self._make_layer(
+ blocks[1], layers[1], channels[1], channels[2]
+ )
+ self.pool3 = nn.Sequential(
+ Conv1x1(channels[2], channels[2]), nn.AvgPool2d(2, stride=2)
+ )
+ self.conv4 = self._make_layer(
+ blocks[2], layers[2], channels[2], channels[3]
+ )
+ self.conv5 = Conv1x1(channels[3], channels[3])
+ self.global_avgpool = nn.AdaptiveAvgPool2d(1)
+ # fully connected layer
+ self.fc = self._construct_fc_layer(
+ self.feature_dim, channels[3], dropout_p=None
+ )
+ # identity classification layer
+ self.classifier = nn.Linear(self.feature_dim, num_classes)
+
+ self._init_params()
+
+ def _make_layer(self, blocks, layer, in_channels, out_channels):
+ layers = []
+ layers += [blocks[0](in_channels, out_channels)]
+ for i in range(1, len(blocks)):
+ layers += [blocks[i](out_channels, out_channels)]
+ return nn.Sequential(*layers)
+
+ def _construct_fc_layer(self, fc_dims, input_dim, dropout_p=None):
+ if fc_dims is None or fc_dims < 0:
+ self.feature_dim = input_dim
+ return None
+
+ if isinstance(fc_dims, int):
+ fc_dims = [fc_dims]
+
+ layers = []
+ for dim in fc_dims:
+ layers.append(nn.Linear(input_dim, dim))
+ layers.append(nn.BatchNorm1d(dim))
+ layers.append(nn.ReLU())
+ if dropout_p is not None:
+ layers.append(nn.Dropout(p=dropout_p))
+ input_dim = dim
+
+ self.feature_dim = fc_dims[-1]
+
+ return nn.Sequential(*layers)
+
+ def _init_params(self):
+ for m in self.modules():
+ if isinstance(m, nn.Conv2d):
+ nn.init.kaiming_normal_(
+ m.weight, mode='fan_out', nonlinearity='relu'
+ )
+ if m.bias is not None:
+ nn.init.constant_(m.bias, 0)
+
+ elif isinstance(m, nn.BatchNorm2d):
+ nn.init.constant_(m.weight, 1)
+ nn.init.constant_(m.bias, 0)
+
+ elif isinstance(m, nn.BatchNorm1d):
+ nn.init.constant_(m.weight, 1)
+ nn.init.constant_(m.bias, 0)
+
+ elif isinstance(m, nn.InstanceNorm2d):
+ nn.init.constant_(m.weight, 1)
+ nn.init.constant_(m.bias, 0)
+
+ elif isinstance(m, nn.Linear):
+ nn.init.normal_(m.weight, 0, 0.01)
+ if m.bias is not None:
+ nn.init.constant_(m.bias, 0)
+
+ def featuremaps(self, x):
+ x = self.conv1(x)
+ x = self.maxpool(x)
+ x = self.conv2(x)
+ x = self.pool2(x)
+ x = self.conv3(x)
+ x = self.pool3(x)
+ x = self.conv4(x)
+ x = self.conv5(x)
+ return x
+
+ def forward(self, x, return_featuremaps=False):
+ x = self.featuremaps(x)
+ if return_featuremaps:
+ return x
+ v = self.global_avgpool(x)
+ v = v.view(v.size(0), -1)
+ if self.fc is not None:
+ v = self.fc(v)
+ if not self.training:
+ return v
+ y = self.classifier(v)
+ if self.loss == 'softmax':
+ return y
+ elif self.loss == 'triplet':
+ return y, v
+ else:
+ raise KeyError("Unsupported loss: {}".format(self.loss))
+
+
+def init_pretrained_weights(model, key=''):
+ """Initializes model with pretrained weights.
+
+ Layers that don't match with pretrained layers in name or size are kept unchanged.
+ """
+ import os
+ import errno
+ import gdown
+ from collections import OrderedDict
+
+ def _get_torch_home():
+ ENV_TORCH_HOME = 'TORCH_HOME'
+ ENV_XDG_CACHE_HOME = 'XDG_CACHE_HOME'
+ DEFAULT_CACHE_DIR = '~/.cache'
+ torch_home = os.path.expanduser(
+ os.getenv(
+ ENV_TORCH_HOME,
+ os.path.join(
+ os.getenv(ENV_XDG_CACHE_HOME, DEFAULT_CACHE_DIR), 'torch'
+ )
+ )
+ )
+ return torch_home
+
+ torch_home = _get_torch_home()
+ model_dir = os.path.join(torch_home, 'checkpoints')
+ try:
+ os.makedirs(model_dir)
+ except OSError as e:
+ if e.errno == errno.EEXIST:
+ # Directory already exists, ignore.
+ pass
+ else:
+ # Unexpected OSError, re-raise.
+ raise
+ filename = key + '_imagenet.pth'
+ cached_file = os.path.join(model_dir, filename)
+
+ if not os.path.exists(cached_file):
+ gdown.download(pretrained_urls[key], cached_file, quiet=False)
+
+ state_dict = torch.load(cached_file)
+ model_dict = model.state_dict()
+ new_state_dict = OrderedDict()
+ matched_layers, discarded_layers = [], []
+
+ for k, v in state_dict.items():
+ if k.startswith('module.'):
+ k = k[7:] # discard module.
+
+ if k in model_dict and model_dict[k].size() == v.size():
+ new_state_dict[k] = v
+ matched_layers.append(k)
+ else:
+ discarded_layers.append(k)
+
+ model_dict.update(new_state_dict)
+ model.load_state_dict(model_dict)
+
+ if len(matched_layers) == 0:
+ warnings.warn(
+ 'The pretrained weights from "{}" cannot be loaded, '
+ 'please check the key names manually '
+ '(** ignored and continue **)'.format(cached_file)
+ )
+ else:
+ print(
+ 'Successfully loaded imagenet pretrained weights from "{}"'.
+ format(cached_file)
+ )
+ if len(discarded_layers) > 0:
+ print(
+ '** The following layers are discarded '
+ 'due to unmatched keys or layer size: {}'.
+ format(discarded_layers)
+ )
+
+
+##########
+# Instantiation
+##########
+def osnet_ain_x1_0(
+ num_classes=1000, pretrained=True, loss='softmax', **kwargs
+):
+ model = OSNet(
+ num_classes,
+ blocks=[
+ [OSBlockINin, OSBlockINin], [OSBlock, OSBlockINin],
+ [OSBlockINin, OSBlock]
+ ],
+ layers=[2, 2, 2],
+ channels=[64, 256, 384, 512],
+ loss=loss,
+ conv1_IN=True,
+ **kwargs
+ )
+ if pretrained:
+ init_pretrained_weights(model, key='osnet_ain_x1_0')
+ return model
+
+
+def osnet_ain_x0_75(
+ num_classes=1000, pretrained=True, loss='softmax', **kwargs
+):
+ model = OSNet(
+ num_classes,
+ blocks=[
+ [OSBlockINin, OSBlockINin], [OSBlock, OSBlockINin],
+ [OSBlockINin, OSBlock]
+ ],
+ layers=[2, 2, 2],
+ channels=[48, 192, 288, 384],
+ loss=loss,
+ conv1_IN=True,
+ **kwargs
+ )
+ if pretrained:
+ init_pretrained_weights(model, key='osnet_ain_x0_75')
+ return model
+
+
+def osnet_ain_x0_5(
+ num_classes=1000, pretrained=True, loss='softmax', **kwargs
+):
+ model = OSNet(
+ num_classes,
+ blocks=[
+ [OSBlockINin, OSBlockINin], [OSBlock, OSBlockINin],
+ [OSBlockINin, OSBlock]
+ ],
+ layers=[2, 2, 2],
+ channels=[32, 128, 192, 256],
+ loss=loss,
+ conv1_IN=True,
+ **kwargs
+ )
+ if pretrained:
+ init_pretrained_weights(model, key='osnet_ain_x0_5')
+ return model
+
+
+def osnet_ain_x0_25(
+ num_classes=1000, pretrained=True, loss='softmax', **kwargs
+):
+ model = OSNet(
+ num_classes,
+ blocks=[
+ [OSBlockINin, OSBlockINin], [OSBlock, OSBlockINin],
+ [OSBlockINin, OSBlock]
+ ],
+ layers=[2, 2, 2],
+ channels=[16, 64, 96, 128],
+ loss=loss,
+ conv1_IN=True,
+ **kwargs
+ )
+ if pretrained:
+ init_pretrained_weights(model, key='osnet_ain_x0_25')
+ return model
diff --git a/trackers/strong_sort/deep/reid/torchreid/models/pcb.py b/trackers/strong_sort/deep/reid/torchreid/models/pcb.py
new file mode 100644
index 0000000000000000000000000000000000000000..92c74148763a600ed331bb0e361588fbf3b09189
--- /dev/null
+++ b/trackers/strong_sort/deep/reid/torchreid/models/pcb.py
@@ -0,0 +1,314 @@
+from __future__ import division, absolute_import
+import torch.utils.model_zoo as model_zoo
+from torch import nn
+from torch.nn import functional as F
+
+__all__ = ['pcb_p6', 'pcb_p4']
+
+model_urls = {
+ 'resnet18': 'https://download.pytorch.org/models/resnet18-5c106cde.pth',
+ 'resnet34': 'https://download.pytorch.org/models/resnet34-333f7ec4.pth',
+ 'resnet50': 'https://download.pytorch.org/models/resnet50-19c8e357.pth',
+ 'resnet101': 'https://download.pytorch.org/models/resnet101-5d3b4d8f.pth',
+ 'resnet152': 'https://download.pytorch.org/models/resnet152-b121ed2d.pth',
+}
+
+
+def conv3x3(in_planes, out_planes, stride=1):
+ """3x3 convolution with padding"""
+ return nn.Conv2d(
+ in_planes,
+ out_planes,
+ kernel_size=3,
+ stride=stride,
+ padding=1,
+ bias=False
+ )
+
+
+class BasicBlock(nn.Module):
+ expansion = 1
+
+ def __init__(self, inplanes, planes, stride=1, downsample=None):
+ super(BasicBlock, self).__init__()
+ self.conv1 = conv3x3(inplanes, planes, stride)
+ self.bn1 = nn.BatchNorm2d(planes)
+ self.relu = nn.ReLU(inplace=True)
+ self.conv2 = conv3x3(planes, planes)
+ self.bn2 = nn.BatchNorm2d(planes)
+ self.downsample = downsample
+ self.stride = stride
+
+ def forward(self, x):
+ residual = x
+
+ out = self.conv1(x)
+ out = self.bn1(out)
+ out = self.relu(out)
+
+ out = self.conv2(out)
+ out = self.bn2(out)
+
+ if self.downsample is not None:
+ residual = self.downsample(x)
+
+ out += residual
+ out = self.relu(out)
+
+ return out
+
+
+class Bottleneck(nn.Module):
+ expansion = 4
+
+ def __init__(self, inplanes, planes, stride=1, downsample=None):
+ super(Bottleneck, self).__init__()
+ self.conv1 = nn.Conv2d(inplanes, planes, kernel_size=1, bias=False)
+ self.bn1 = nn.BatchNorm2d(planes)
+ self.conv2 = nn.Conv2d(
+ planes,
+ planes,
+ kernel_size=3,
+ stride=stride,
+ padding=1,
+ bias=False
+ )
+ self.bn2 = nn.BatchNorm2d(planes)
+ self.conv3 = nn.Conv2d(
+ planes, planes * self.expansion, kernel_size=1, bias=False
+ )
+ self.bn3 = nn.BatchNorm2d(planes * self.expansion)
+ self.relu = nn.ReLU(inplace=True)
+ self.downsample = downsample
+ self.stride = stride
+
+ def forward(self, x):
+ residual = x
+
+ out = self.conv1(x)
+ out = self.bn1(out)
+ out = self.relu(out)
+
+ out = self.conv2(out)
+ out = self.bn2(out)
+ out = self.relu(out)
+
+ out = self.conv3(out)
+ out = self.bn3(out)
+
+ if self.downsample is not None:
+ residual = self.downsample(x)
+
+ out += residual
+ out = self.relu(out)
+
+ return out
+
+
+class DimReduceLayer(nn.Module):
+
+ def __init__(self, in_channels, out_channels, nonlinear):
+ super(DimReduceLayer, self).__init__()
+ layers = []
+ layers.append(
+ nn.Conv2d(
+ in_channels, out_channels, 1, stride=1, padding=0, bias=False
+ )
+ )
+ layers.append(nn.BatchNorm2d(out_channels))
+
+ if nonlinear == 'relu':
+ layers.append(nn.ReLU(inplace=True))
+ elif nonlinear == 'leakyrelu':
+ layers.append(nn.LeakyReLU(0.1))
+
+ self.layers = nn.Sequential(*layers)
+
+ def forward(self, x):
+ return self.layers(x)
+
+
+class PCB(nn.Module):
+ """Part-based Convolutional Baseline.
+
+ Reference:
+ Sun et al. Beyond Part Models: Person Retrieval with Refined
+ Part Pooling (and A Strong Convolutional Baseline). ECCV 2018.
+
+ Public keys:
+ - ``pcb_p4``: PCB with 4-part strips.
+ - ``pcb_p6``: PCB with 6-part strips.
+ """
+
+ def __init__(
+ self,
+ num_classes,
+ loss,
+ block,
+ layers,
+ parts=6,
+ reduced_dim=256,
+ nonlinear='relu',
+ **kwargs
+ ):
+ self.inplanes = 64
+ super(PCB, self).__init__()
+ self.loss = loss
+ self.parts = parts
+ self.feature_dim = 512 * block.expansion
+
+ # backbone network
+ self.conv1 = nn.Conv2d(
+ 3, 64, kernel_size=7, stride=2, padding=3, bias=False
+ )
+ self.bn1 = nn.BatchNorm2d(64)
+ self.relu = nn.ReLU(inplace=True)
+ self.maxpool = nn.MaxPool2d(kernel_size=3, stride=2, padding=1)
+ self.layer1 = self._make_layer(block, 64, layers[0])
+ self.layer2 = self._make_layer(block, 128, layers[1], stride=2)
+ self.layer3 = self._make_layer(block, 256, layers[2], stride=2)
+ self.layer4 = self._make_layer(block, 512, layers[3], stride=1)
+
+ # pcb layers
+ self.parts_avgpool = nn.AdaptiveAvgPool2d((self.parts, 1))
+ self.dropout = nn.Dropout(p=0.5)
+ self.conv5 = DimReduceLayer(
+ 512 * block.expansion, reduced_dim, nonlinear=nonlinear
+ )
+ self.feature_dim = reduced_dim
+ self.classifier = nn.ModuleList(
+ [
+ nn.Linear(self.feature_dim, num_classes)
+ for _ in range(self.parts)
+ ]
+ )
+
+ self._init_params()
+
+ def _make_layer(self, block, planes, blocks, stride=1):
+ downsample = None
+ if stride != 1 or self.inplanes != planes * block.expansion:
+ downsample = nn.Sequential(
+ nn.Conv2d(
+ self.inplanes,
+ planes * block.expansion,
+ kernel_size=1,
+ stride=stride,
+ bias=False
+ ),
+ nn.BatchNorm2d(planes * block.expansion),
+ )
+
+ layers = []
+ layers.append(block(self.inplanes, planes, stride, downsample))
+ self.inplanes = planes * block.expansion
+ for i in range(1, blocks):
+ layers.append(block(self.inplanes, planes))
+
+ return nn.Sequential(*layers)
+
+ def _init_params(self):
+ for m in self.modules():
+ if isinstance(m, nn.Conv2d):
+ nn.init.kaiming_normal_(
+ m.weight, mode='fan_out', nonlinearity='relu'
+ )
+ if m.bias is not None:
+ nn.init.constant_(m.bias, 0)
+ elif isinstance(m, nn.BatchNorm2d):
+ nn.init.constant_(m.weight, 1)
+ nn.init.constant_(m.bias, 0)
+ elif isinstance(m, nn.BatchNorm1d):
+ nn.init.constant_(m.weight, 1)
+ nn.init.constant_(m.bias, 0)
+ elif isinstance(m, nn.Linear):
+ nn.init.normal_(m.weight, 0, 0.01)
+ if m.bias is not None:
+ nn.init.constant_(m.bias, 0)
+
+ def featuremaps(self, x):
+ x = self.conv1(x)
+ x = self.bn1(x)
+ x = self.relu(x)
+ x = self.maxpool(x)
+ x = self.layer1(x)
+ x = self.layer2(x)
+ x = self.layer3(x)
+ x = self.layer4(x)
+ return x
+
+ def forward(self, x):
+ f = self.featuremaps(x)
+ v_g = self.parts_avgpool(f)
+
+ if not self.training:
+ v_g = F.normalize(v_g, p=2, dim=1)
+ return v_g.view(v_g.size(0), -1)
+
+ v_g = self.dropout(v_g)
+ v_h = self.conv5(v_g)
+
+ y = []
+ for i in range(self.parts):
+ v_h_i = v_h[:, :, i, :]
+ v_h_i = v_h_i.view(v_h_i.size(0), -1)
+ y_i = self.classifier[i](v_h_i)
+ y.append(y_i)
+
+ if self.loss == 'softmax':
+ return y
+ elif self.loss == 'triplet':
+ v_g = F.normalize(v_g, p=2, dim=1)
+ return y, v_g.view(v_g.size(0), -1)
+ else:
+ raise KeyError('Unsupported loss: {}'.format(self.loss))
+
+
+def init_pretrained_weights(model, model_url):
+ """Initializes model with pretrained weights.
+
+ Layers that don't match with pretrained layers in name or size are kept unchanged.
+ """
+ pretrain_dict = model_zoo.load_url(model_url)
+ model_dict = model.state_dict()
+ pretrain_dict = {
+ k: v
+ for k, v in pretrain_dict.items()
+ if k in model_dict and model_dict[k].size() == v.size()
+ }
+ model_dict.update(pretrain_dict)
+ model.load_state_dict(model_dict)
+
+
+def pcb_p6(num_classes, loss='softmax', pretrained=True, **kwargs):
+ model = PCB(
+ num_classes=num_classes,
+ loss=loss,
+ block=Bottleneck,
+ layers=[3, 4, 6, 3],
+ last_stride=1,
+ parts=6,
+ reduced_dim=256,
+ nonlinear='relu',
+ **kwargs
+ )
+ if pretrained:
+ init_pretrained_weights(model, model_urls['resnet50'])
+ return model
+
+
+def pcb_p4(num_classes, loss='softmax', pretrained=True, **kwargs):
+ model = PCB(
+ num_classes=num_classes,
+ loss=loss,
+ block=Bottleneck,
+ layers=[3, 4, 6, 3],
+ last_stride=1,
+ parts=4,
+ reduced_dim=256,
+ nonlinear='relu',
+ **kwargs
+ )
+ if pretrained:
+ init_pretrained_weights(model, model_urls['resnet50'])
+ return model
diff --git a/trackers/strong_sort/deep/reid/torchreid/models/resnet.py b/trackers/strong_sort/deep/reid/torchreid/models/resnet.py
new file mode 100644
index 0000000000000000000000000000000000000000..63d7f43ff43373d28c45de3930da0bdbee817b61
--- /dev/null
+++ b/trackers/strong_sort/deep/reid/torchreid/models/resnet.py
@@ -0,0 +1,530 @@
+"""
+Code source: https://github.com/pytorch/vision
+"""
+from __future__ import division, absolute_import
+import torch.utils.model_zoo as model_zoo
+from torch import nn
+
+__all__ = [
+ 'resnet18', 'resnet34', 'resnet50', 'resnet101', 'resnet152',
+ 'resnext50_32x4d', 'resnext101_32x8d', 'resnet50_fc512'
+]
+
+model_urls = {
+ 'resnet18':
+ 'https://download.pytorch.org/models/resnet18-5c106cde.pth',
+ 'resnet34':
+ 'https://download.pytorch.org/models/resnet34-333f7ec4.pth',
+ 'resnet50':
+ 'https://download.pytorch.org/models/resnet50-19c8e357.pth',
+ 'resnet101':
+ 'https://download.pytorch.org/models/resnet101-5d3b4d8f.pth',
+ 'resnet152':
+ 'https://download.pytorch.org/models/resnet152-b121ed2d.pth',
+ 'resnext50_32x4d':
+ 'https://download.pytorch.org/models/resnext50_32x4d-7cdf4587.pth',
+ 'resnext101_32x8d':
+ 'https://download.pytorch.org/models/resnext101_32x8d-8ba56ff5.pth',
+}
+
+
+def conv3x3(in_planes, out_planes, stride=1, groups=1, dilation=1):
+ """3x3 convolution with padding"""
+ return nn.Conv2d(
+ in_planes,
+ out_planes,
+ kernel_size=3,
+ stride=stride,
+ padding=dilation,
+ groups=groups,
+ bias=False,
+ dilation=dilation
+ )
+
+
+def conv1x1(in_planes, out_planes, stride=1):
+ """1x1 convolution"""
+ return nn.Conv2d(
+ in_planes, out_planes, kernel_size=1, stride=stride, bias=False
+ )
+
+
+class BasicBlock(nn.Module):
+ expansion = 1
+
+ def __init__(
+ self,
+ inplanes,
+ planes,
+ stride=1,
+ downsample=None,
+ groups=1,
+ base_width=64,
+ dilation=1,
+ norm_layer=None
+ ):
+ super(BasicBlock, self).__init__()
+ if norm_layer is None:
+ norm_layer = nn.BatchNorm2d
+ if groups != 1 or base_width != 64:
+ raise ValueError(
+ 'BasicBlock only supports groups=1 and base_width=64'
+ )
+ if dilation > 1:
+ raise NotImplementedError(
+ "Dilation > 1 not supported in BasicBlock"
+ )
+ # Both self.conv1 and self.downsample layers downsample the input when stride != 1
+ self.conv1 = conv3x3(inplanes, planes, stride)
+ self.bn1 = norm_layer(planes)
+ self.relu = nn.ReLU(inplace=True)
+ self.conv2 = conv3x3(planes, planes)
+ self.bn2 = norm_layer(planes)
+ self.downsample = downsample
+ self.stride = stride
+
+ def forward(self, x):
+ identity = x
+
+ out = self.conv1(x)
+ out = self.bn1(out)
+ out = self.relu(out)
+
+ out = self.conv2(out)
+ out = self.bn2(out)
+
+ if self.downsample is not None:
+ identity = self.downsample(x)
+
+ out += identity
+ out = self.relu(out)
+
+ return out
+
+
+class Bottleneck(nn.Module):
+ expansion = 4
+
+ def __init__(
+ self,
+ inplanes,
+ planes,
+ stride=1,
+ downsample=None,
+ groups=1,
+ base_width=64,
+ dilation=1,
+ norm_layer=None
+ ):
+ super(Bottleneck, self).__init__()
+ if norm_layer is None:
+ norm_layer = nn.BatchNorm2d
+ width = int(planes * (base_width/64.)) * groups
+ # Both self.conv2 and self.downsample layers downsample the input when stride != 1
+ self.conv1 = conv1x1(inplanes, width)
+ self.bn1 = norm_layer(width)
+ self.conv2 = conv3x3(width, width, stride, groups, dilation)
+ self.bn2 = norm_layer(width)
+ self.conv3 = conv1x1(width, planes * self.expansion)
+ self.bn3 = norm_layer(planes * self.expansion)
+ self.relu = nn.ReLU(inplace=True)
+ self.downsample = downsample
+ self.stride = stride
+
+ def forward(self, x):
+ identity = x
+
+ out = self.conv1(x)
+ out = self.bn1(out)
+ out = self.relu(out)
+
+ out = self.conv2(out)
+ out = self.bn2(out)
+ out = self.relu(out)
+
+ out = self.conv3(out)
+ out = self.bn3(out)
+
+ if self.downsample is not None:
+ identity = self.downsample(x)
+
+ out += identity
+ out = self.relu(out)
+
+ return out
+
+
+class ResNet(nn.Module):
+ """Residual network.
+
+ Reference:
+ - He et al. Deep Residual Learning for Image Recognition. CVPR 2016.
+ - Xie et al. Aggregated Residual Transformations for Deep Neural Networks. CVPR 2017.
+
+ Public keys:
+ - ``resnet18``: ResNet18.
+ - ``resnet34``: ResNet34.
+ - ``resnet50``: ResNet50.
+ - ``resnet101``: ResNet101.
+ - ``resnet152``: ResNet152.
+ - ``resnext50_32x4d``: ResNeXt50.
+ - ``resnext101_32x8d``: ResNeXt101.
+ - ``resnet50_fc512``: ResNet50 + FC.
+ """
+
+ def __init__(
+ self,
+ num_classes,
+ loss,
+ block,
+ layers,
+ zero_init_residual=False,
+ groups=1,
+ width_per_group=64,
+ replace_stride_with_dilation=None,
+ norm_layer=None,
+ last_stride=2,
+ fc_dims=None,
+ dropout_p=None,
+ **kwargs
+ ):
+ super(ResNet, self).__init__()
+ if norm_layer is None:
+ norm_layer = nn.BatchNorm2d
+ self._norm_layer = norm_layer
+ self.loss = loss
+ self.feature_dim = 512 * block.expansion
+ self.inplanes = 64
+ self.dilation = 1
+ if replace_stride_with_dilation is None:
+ # each element in the tuple indicates if we should replace
+ # the 2x2 stride with a dilated convolution instead
+ replace_stride_with_dilation = [False, False, False]
+ if len(replace_stride_with_dilation) != 3:
+ raise ValueError(
+ "replace_stride_with_dilation should be None "
+ "or a 3-element tuple, got {}".
+ format(replace_stride_with_dilation)
+ )
+ self.groups = groups
+ self.base_width = width_per_group
+ self.conv1 = nn.Conv2d(
+ 3, self.inplanes, kernel_size=7, stride=2, padding=3, bias=False
+ )
+ self.bn1 = norm_layer(self.inplanes)
+ self.relu = nn.ReLU(inplace=True)
+ self.maxpool = nn.MaxPool2d(kernel_size=3, stride=2, padding=1)
+ self.layer1 = self._make_layer(block, 64, layers[0])
+ self.layer2 = self._make_layer(
+ block,
+ 128,
+ layers[1],
+ stride=2,
+ dilate=replace_stride_with_dilation[0]
+ )
+ self.layer3 = self._make_layer(
+ block,
+ 256,
+ layers[2],
+ stride=2,
+ dilate=replace_stride_with_dilation[1]
+ )
+ self.layer4 = self._make_layer(
+ block,
+ 512,
+ layers[3],
+ stride=last_stride,
+ dilate=replace_stride_with_dilation[2]
+ )
+ self.global_avgpool = nn.AdaptiveAvgPool2d((1, 1))
+ self.fc = self._construct_fc_layer(
+ fc_dims, 512 * block.expansion, dropout_p
+ )
+ self.classifier = nn.Linear(self.feature_dim, num_classes)
+
+ self._init_params()
+
+ # Zero-initialize the last BN in each residual branch,
+ # so that the residual branch starts with zeros, and each residual block behaves like an identity.
+ # This improves the model by 0.2~0.3% according to https://arxiv.org/abs/1706.02677
+ if zero_init_residual:
+ for m in self.modules():
+ if isinstance(m, Bottleneck):
+ nn.init.constant_(m.bn3.weight, 0)
+ elif isinstance(m, BasicBlock):
+ nn.init.constant_(m.bn2.weight, 0)
+
+ def _make_layer(self, block, planes, blocks, stride=1, dilate=False):
+ norm_layer = self._norm_layer
+ downsample = None
+ previous_dilation = self.dilation
+ if dilate:
+ self.dilation *= stride
+ stride = 1
+ if stride != 1 or self.inplanes != planes * block.expansion:
+ downsample = nn.Sequential(
+ conv1x1(self.inplanes, planes * block.expansion, stride),
+ norm_layer(planes * block.expansion),
+ )
+
+ layers = []
+ layers.append(
+ block(
+ self.inplanes, planes, stride, downsample, self.groups,
+ self.base_width, previous_dilation, norm_layer
+ )
+ )
+ self.inplanes = planes * block.expansion
+ for _ in range(1, blocks):
+ layers.append(
+ block(
+ self.inplanes,
+ planes,
+ groups=self.groups,
+ base_width=self.base_width,
+ dilation=self.dilation,
+ norm_layer=norm_layer
+ )
+ )
+
+ return nn.Sequential(*layers)
+
+ def _construct_fc_layer(self, fc_dims, input_dim, dropout_p=None):
+ """Constructs fully connected layer
+
+ Args:
+ fc_dims (list or tuple): dimensions of fc layers, if None, no fc layers are constructed
+ input_dim (int): input dimension
+ dropout_p (float): dropout probability, if None, dropout is unused
+ """
+ if fc_dims is None:
+ self.feature_dim = input_dim
+ return None
+
+ assert isinstance(
+ fc_dims, (list, tuple)
+ ), 'fc_dims must be either list or tuple, but got {}'.format(
+ type(fc_dims)
+ )
+
+ layers = []
+ for dim in fc_dims:
+ layers.append(nn.Linear(input_dim, dim))
+ layers.append(nn.BatchNorm1d(dim))
+ layers.append(nn.ReLU(inplace=True))
+ if dropout_p is not None:
+ layers.append(nn.Dropout(p=dropout_p))
+ input_dim = dim
+
+ self.feature_dim = fc_dims[-1]
+
+ return nn.Sequential(*layers)
+
+ def _init_params(self):
+ for m in self.modules():
+ if isinstance(m, nn.Conv2d):
+ nn.init.kaiming_normal_(
+ m.weight, mode='fan_out', nonlinearity='relu'
+ )
+ if m.bias is not None:
+ nn.init.constant_(m.bias, 0)
+ elif isinstance(m, nn.BatchNorm2d):
+ nn.init.constant_(m.weight, 1)
+ nn.init.constant_(m.bias, 0)
+ elif isinstance(m, nn.BatchNorm1d):
+ nn.init.constant_(m.weight, 1)
+ nn.init.constant_(m.bias, 0)
+ elif isinstance(m, nn.Linear):
+ nn.init.normal_(m.weight, 0, 0.01)
+ if m.bias is not None:
+ nn.init.constant_(m.bias, 0)
+
+ def featuremaps(self, x):
+ x = self.conv1(x)
+ x = self.bn1(x)
+ x = self.relu(x)
+ x = self.maxpool(x)
+ x = self.layer1(x)
+ x = self.layer2(x)
+ x = self.layer3(x)
+ x = self.layer4(x)
+ return x
+
+ def forward(self, x):
+ f = self.featuremaps(x)
+ v = self.global_avgpool(f)
+ v = v.view(v.size(0), -1)
+
+ if self.fc is not None:
+ v = self.fc(v)
+
+ if not self.training:
+ return v
+
+ y = self.classifier(v)
+
+ if self.loss == 'softmax':
+ return y
+ elif self.loss == 'triplet':
+ return y, v
+ else:
+ raise KeyError("Unsupported loss: {}".format(self.loss))
+
+
+def init_pretrained_weights(model, model_url):
+ """Initializes model with pretrained weights.
+
+ Layers that don't match with pretrained layers in name or size are kept unchanged.
+ """
+ pretrain_dict = model_zoo.load_url(model_url)
+ model_dict = model.state_dict()
+ pretrain_dict = {
+ k: v
+ for k, v in pretrain_dict.items()
+ if k in model_dict and model_dict[k].size() == v.size()
+ }
+ model_dict.update(pretrain_dict)
+ model.load_state_dict(model_dict)
+
+
+"""ResNet"""
+
+
+def resnet18(num_classes, loss='softmax', pretrained=True, **kwargs):
+ model = ResNet(
+ num_classes=num_classes,
+ loss=loss,
+ block=BasicBlock,
+ layers=[2, 2, 2, 2],
+ last_stride=2,
+ fc_dims=None,
+ dropout_p=None,
+ **kwargs
+ )
+ if pretrained:
+ init_pretrained_weights(model, model_urls['resnet18'])
+ return model
+
+
+def resnet34(num_classes, loss='softmax', pretrained=True, **kwargs):
+ model = ResNet(
+ num_classes=num_classes,
+ loss=loss,
+ block=BasicBlock,
+ layers=[3, 4, 6, 3],
+ last_stride=2,
+ fc_dims=None,
+ dropout_p=None,
+ **kwargs
+ )
+ if pretrained:
+ init_pretrained_weights(model, model_urls['resnet34'])
+ return model
+
+
+def resnet50(num_classes, loss='softmax', pretrained=True, **kwargs):
+ model = ResNet(
+ num_classes=num_classes,
+ loss=loss,
+ block=Bottleneck,
+ layers=[3, 4, 6, 3],
+ last_stride=2,
+ fc_dims=None,
+ dropout_p=None,
+ **kwargs
+ )
+ if pretrained:
+ init_pretrained_weights(model, model_urls['resnet50'])
+ return model
+
+
+def resnet101(num_classes, loss='softmax', pretrained=True, **kwargs):
+ model = ResNet(
+ num_classes=num_classes,
+ loss=loss,
+ block=Bottleneck,
+ layers=[3, 4, 23, 3],
+ last_stride=2,
+ fc_dims=None,
+ dropout_p=None,
+ **kwargs
+ )
+ if pretrained:
+ init_pretrained_weights(model, model_urls['resnet101'])
+ return model
+
+
+def resnet152(num_classes, loss='softmax', pretrained=True, **kwargs):
+ model = ResNet(
+ num_classes=num_classes,
+ loss=loss,
+ block=Bottleneck,
+ layers=[3, 8, 36, 3],
+ last_stride=2,
+ fc_dims=None,
+ dropout_p=None,
+ **kwargs
+ )
+ if pretrained:
+ init_pretrained_weights(model, model_urls['resnet152'])
+ return model
+
+
+"""ResNeXt"""
+
+
+def resnext50_32x4d(num_classes, loss='softmax', pretrained=True, **kwargs):
+ model = ResNet(
+ num_classes=num_classes,
+ loss=loss,
+ block=Bottleneck,
+ layers=[3, 4, 6, 3],
+ last_stride=2,
+ fc_dims=None,
+ dropout_p=None,
+ groups=32,
+ width_per_group=4,
+ **kwargs
+ )
+ if pretrained:
+ init_pretrained_weights(model, model_urls['resnext50_32x4d'])
+ return model
+
+
+def resnext101_32x8d(num_classes, loss='softmax', pretrained=True, **kwargs):
+ model = ResNet(
+ num_classes=num_classes,
+ loss=loss,
+ block=Bottleneck,
+ layers=[3, 4, 23, 3],
+ last_stride=2,
+ fc_dims=None,
+ dropout_p=None,
+ groups=32,
+ width_per_group=8,
+ **kwargs
+ )
+ if pretrained:
+ init_pretrained_weights(model, model_urls['resnext101_32x8d'])
+ return model
+
+
+"""
+ResNet + FC
+"""
+
+
+def resnet50_fc512(num_classes, loss='softmax', pretrained=True, **kwargs):
+ model = ResNet(
+ num_classes=num_classes,
+ loss=loss,
+ block=Bottleneck,
+ layers=[3, 4, 6, 3],
+ last_stride=1,
+ fc_dims=[512],
+ dropout_p=None,
+ **kwargs
+ )
+ if pretrained:
+ init_pretrained_weights(model, model_urls['resnet50'])
+ return model
diff --git a/trackers/strong_sort/deep/reid/torchreid/models/resnet_ibn_a.py b/trackers/strong_sort/deep/reid/torchreid/models/resnet_ibn_a.py
new file mode 100644
index 0000000000000000000000000000000000000000..d198e7c9e361c40d25bc7eb1f352b971596ee124
--- /dev/null
+++ b/trackers/strong_sort/deep/reid/torchreid/models/resnet_ibn_a.py
@@ -0,0 +1,289 @@
+"""
+Credit to https://github.com/XingangPan/IBN-Net.
+"""
+from __future__ import division, absolute_import
+import math
+import torch
+import torch.nn as nn
+import torch.utils.model_zoo as model_zoo
+
+__all__ = ['resnet50_ibn_a']
+
+model_urls = {
+ 'resnet50': 'https://download.pytorch.org/models/resnet50-19c8e357.pth',
+ 'resnet101': 'https://download.pytorch.org/models/resnet101-5d3b4d8f.pth',
+ 'resnet152': 'https://download.pytorch.org/models/resnet152-b121ed2d.pth',
+}
+
+
+def conv3x3(in_planes, out_planes, stride=1):
+ "3x3 convolution with padding"
+ return nn.Conv2d(
+ in_planes,
+ out_planes,
+ kernel_size=3,
+ stride=stride,
+ padding=1,
+ bias=False
+ )
+
+
+class BasicBlock(nn.Module):
+ expansion = 1
+
+ def __init__(self, inplanes, planes, stride=1, downsample=None):
+ super(BasicBlock, self).__init__()
+ self.conv1 = conv3x3(inplanes, planes, stride)
+ self.bn1 = nn.BatchNorm2d(planes)
+ self.relu = nn.ReLU(inplace=True)
+ self.conv2 = conv3x3(planes, planes)
+ self.bn2 = nn.BatchNorm2d(planes)
+ self.downsample = downsample
+ self.stride = stride
+
+ def forward(self, x):
+ residual = x
+
+ out = self.conv1(x)
+ out = self.bn1(out)
+ out = self.relu(out)
+
+ out = self.conv2(out)
+ out = self.bn2(out)
+
+ if self.downsample is not None:
+ residual = self.downsample(x)
+
+ out += residual
+ out = self.relu(out)
+
+ return out
+
+
+class IBN(nn.Module):
+
+ def __init__(self, planes):
+ super(IBN, self).__init__()
+ half1 = int(planes / 2)
+ self.half = half1
+ half2 = planes - half1
+ self.IN = nn.InstanceNorm2d(half1, affine=True)
+ self.BN = nn.BatchNorm2d(half2)
+
+ def forward(self, x):
+ split = torch.split(x, self.half, 1)
+ out1 = self.IN(split[0].contiguous())
+ out2 = self.BN(split[1].contiguous())
+ out = torch.cat((out1, out2), 1)
+ return out
+
+
+class Bottleneck(nn.Module):
+ expansion = 4
+
+ def __init__(self, inplanes, planes, ibn=False, stride=1, downsample=None):
+ super(Bottleneck, self).__init__()
+ self.conv1 = nn.Conv2d(inplanes, planes, kernel_size=1, bias=False)
+ if ibn:
+ self.bn1 = IBN(planes)
+ else:
+ self.bn1 = nn.BatchNorm2d(planes)
+ self.conv2 = nn.Conv2d(
+ planes,
+ planes,
+ kernel_size=3,
+ stride=stride,
+ padding=1,
+ bias=False
+ )
+ self.bn2 = nn.BatchNorm2d(planes)
+ self.conv3 = nn.Conv2d(
+ planes, planes * self.expansion, kernel_size=1, bias=False
+ )
+ self.bn3 = nn.BatchNorm2d(planes * self.expansion)
+ self.relu = nn.ReLU(inplace=True)
+ self.downsample = downsample
+ self.stride = stride
+
+ def forward(self, x):
+ residual = x
+
+ out = self.conv1(x)
+ out = self.bn1(out)
+ out = self.relu(out)
+
+ out = self.conv2(out)
+ out = self.bn2(out)
+ out = self.relu(out)
+
+ out = self.conv3(out)
+ out = self.bn3(out)
+
+ if self.downsample is not None:
+ residual = self.downsample(x)
+
+ out += residual
+ out = self.relu(out)
+
+ return out
+
+
+class ResNet(nn.Module):
+ """Residual network + IBN layer.
+
+ Reference:
+ - He et al. Deep Residual Learning for Image Recognition. CVPR 2016.
+ - Pan et al. Two at Once: Enhancing Learning and Generalization
+ Capacities via IBN-Net. ECCV 2018.
+ """
+
+ def __init__(
+ self,
+ block,
+ layers,
+ num_classes=1000,
+ loss='softmax',
+ fc_dims=None,
+ dropout_p=None,
+ **kwargs
+ ):
+ scale = 64
+ self.inplanes = scale
+ super(ResNet, self).__init__()
+ self.loss = loss
+ self.feature_dim = scale * 8 * block.expansion
+
+ self.conv1 = nn.Conv2d(
+ 3, scale, kernel_size=7, stride=2, padding=3, bias=False
+ )
+ self.bn1 = nn.BatchNorm2d(scale)
+ self.relu = nn.ReLU(inplace=True)
+ self.maxpool = nn.MaxPool2d(kernel_size=3, stride=2, padding=1)
+ self.layer1 = self._make_layer(block, scale, layers[0])
+ self.layer2 = self._make_layer(block, scale * 2, layers[1], stride=2)
+ self.layer3 = self._make_layer(block, scale * 4, layers[2], stride=2)
+ self.layer4 = self._make_layer(block, scale * 8, layers[3], stride=2)
+ self.avgpool = nn.AdaptiveAvgPool2d((1, 1))
+ self.fc = self._construct_fc_layer(
+ fc_dims, scale * 8 * block.expansion, dropout_p
+ )
+ self.classifier = nn.Linear(self.feature_dim, num_classes)
+
+ for m in self.modules():
+ if isinstance(m, nn.Conv2d):
+ n = m.kernel_size[0] * m.kernel_size[1] * m.out_channels
+ m.weight.data.normal_(0, math.sqrt(2. / n))
+ elif isinstance(m, nn.BatchNorm2d):
+ m.weight.data.fill_(1)
+ m.bias.data.zero_()
+ elif isinstance(m, nn.InstanceNorm2d):
+ m.weight.data.fill_(1)
+ m.bias.data.zero_()
+
+ def _make_layer(self, block, planes, blocks, stride=1):
+ downsample = None
+ if stride != 1 or self.inplanes != planes * block.expansion:
+ downsample = nn.Sequential(
+ nn.Conv2d(
+ self.inplanes,
+ planes * block.expansion,
+ kernel_size=1,
+ stride=stride,
+ bias=False
+ ),
+ nn.BatchNorm2d(planes * block.expansion),
+ )
+
+ layers = []
+ ibn = True
+ if planes == 512:
+ ibn = False
+ layers.append(block(self.inplanes, planes, ibn, stride, downsample))
+ self.inplanes = planes * block.expansion
+ for i in range(1, blocks):
+ layers.append(block(self.inplanes, planes, ibn))
+
+ return nn.Sequential(*layers)
+
+ def _construct_fc_layer(self, fc_dims, input_dim, dropout_p=None):
+ """Constructs fully connected layer
+
+ Args:
+ fc_dims (list or tuple): dimensions of fc layers, if None, no fc layers are constructed
+ input_dim (int): input dimension
+ dropout_p (float): dropout probability, if None, dropout is unused
+ """
+ if fc_dims is None:
+ self.feature_dim = input_dim
+ return None
+
+ assert isinstance(
+ fc_dims, (list, tuple)
+ ), 'fc_dims must be either list or tuple, but got {}'.format(
+ type(fc_dims)
+ )
+
+ layers = []
+ for dim in fc_dims:
+ layers.append(nn.Linear(input_dim, dim))
+ layers.append(nn.BatchNorm1d(dim))
+ layers.append(nn.ReLU(inplace=True))
+ if dropout_p is not None:
+ layers.append(nn.Dropout(p=dropout_p))
+ input_dim = dim
+
+ self.feature_dim = fc_dims[-1]
+
+ return nn.Sequential(*layers)
+
+ def featuremaps(self, x):
+ x = self.conv1(x)
+ x = self.bn1(x)
+ x = self.relu(x)
+ x = self.maxpool(x)
+ x = self.layer1(x)
+ x = self.layer2(x)
+ x = self.layer3(x)
+ x = self.layer4(x)
+ return x
+
+ def forward(self, x):
+ f = self.featuremaps(x)
+ v = self.avgpool(f)
+ v = v.view(v.size(0), -1)
+ if self.fc is not None:
+ v = self.fc(v)
+ if not self.training:
+ return v
+ y = self.classifier(v)
+ if self.loss == 'softmax':
+ return y
+ elif self.loss == 'triplet':
+ return y, v
+ else:
+ raise KeyError("Unsupported loss: {}".format(self.loss))
+
+
+def init_pretrained_weights(model, model_url):
+ """Initializes model with pretrained weights.
+
+ Layers that don't match with pretrained layers in name or size are kept unchanged.
+ """
+ pretrain_dict = model_zoo.load_url(model_url)
+ model_dict = model.state_dict()
+ pretrain_dict = {
+ k: v
+ for k, v in pretrain_dict.items()
+ if k in model_dict and model_dict[k].size() == v.size()
+ }
+ model_dict.update(pretrain_dict)
+ model.load_state_dict(model_dict)
+
+
+def resnet50_ibn_a(num_classes, loss='softmax', pretrained=False, **kwargs):
+ model = ResNet(
+ Bottleneck, [3, 4, 6, 3], num_classes=num_classes, loss=loss, **kwargs
+ )
+ if pretrained:
+ init_pretrained_weights(model, model_urls['resnet50'])
+ return model
diff --git a/trackers/strong_sort/deep/reid/torchreid/models/resnet_ibn_b.py b/trackers/strong_sort/deep/reid/torchreid/models/resnet_ibn_b.py
new file mode 100644
index 0000000000000000000000000000000000000000..9881cc7d64e97a74bab35e6145197d6d740689ad
--- /dev/null
+++ b/trackers/strong_sort/deep/reid/torchreid/models/resnet_ibn_b.py
@@ -0,0 +1,274 @@
+"""
+Credit to https://github.com/XingangPan/IBN-Net.
+"""
+from __future__ import division, absolute_import
+import math
+import torch.nn as nn
+import torch.utils.model_zoo as model_zoo
+
+__all__ = ['resnet50_ibn_b']
+
+model_urls = {
+ 'resnet50': 'https://download.pytorch.org/models/resnet50-19c8e357.pth',
+ 'resnet101': 'https://download.pytorch.org/models/resnet101-5d3b4d8f.pth',
+ 'resnet152': 'https://download.pytorch.org/models/resnet152-b121ed2d.pth',
+}
+
+
+def conv3x3(in_planes, out_planes, stride=1):
+ "3x3 convolution with padding"
+ return nn.Conv2d(
+ in_planes,
+ out_planes,
+ kernel_size=3,
+ stride=stride,
+ padding=1,
+ bias=False
+ )
+
+
+class BasicBlock(nn.Module):
+ expansion = 1
+
+ def __init__(self, inplanes, planes, stride=1, downsample=None):
+ super(BasicBlock, self).__init__()
+ self.conv1 = conv3x3(inplanes, planes, stride)
+ self.bn1 = nn.BatchNorm2d(planes)
+ self.relu = nn.ReLU(inplace=True)
+ self.conv2 = conv3x3(planes, planes)
+ self.bn2 = nn.BatchNorm2d(planes)
+ self.downsample = downsample
+ self.stride = stride
+
+ def forward(self, x):
+ residual = x
+
+ out = self.conv1(x)
+ out = self.bn1(out)
+ out = self.relu(out)
+
+ out = self.conv2(out)
+ out = self.bn2(out)
+
+ if self.downsample is not None:
+ residual = self.downsample(x)
+
+ out += residual
+ out = self.relu(out)
+
+ return out
+
+
+class Bottleneck(nn.Module):
+ expansion = 4
+
+ def __init__(self, inplanes, planes, stride=1, downsample=None, IN=False):
+ super(Bottleneck, self).__init__()
+ self.conv1 = nn.Conv2d(inplanes, planes, kernel_size=1, bias=False)
+ self.bn1 = nn.BatchNorm2d(planes)
+ self.conv2 = nn.Conv2d(
+ planes,
+ planes,
+ kernel_size=3,
+ stride=stride,
+ padding=1,
+ bias=False
+ )
+ self.bn2 = nn.BatchNorm2d(planes)
+ self.conv3 = nn.Conv2d(
+ planes, planes * self.expansion, kernel_size=1, bias=False
+ )
+ self.bn3 = nn.BatchNorm2d(planes * self.expansion)
+ self.IN = None
+ if IN:
+ self.IN = nn.InstanceNorm2d(planes * 4, affine=True)
+ self.relu = nn.ReLU(inplace=True)
+ self.downsample = downsample
+ self.stride = stride
+
+ def forward(self, x):
+ residual = x
+
+ out = self.conv1(x)
+ out = self.bn1(out)
+ out = self.relu(out)
+
+ out = self.conv2(out)
+ out = self.bn2(out)
+ out = self.relu(out)
+
+ out = self.conv3(out)
+ out = self.bn3(out)
+
+ if self.downsample is not None:
+ residual = self.downsample(x)
+
+ out += residual
+ if self.IN is not None:
+ out = self.IN(out)
+ out = self.relu(out)
+
+ return out
+
+
+class ResNet(nn.Module):
+ """Residual network + IBN layer.
+
+ Reference:
+ - He et al. Deep Residual Learning for Image Recognition. CVPR 2016.
+ - Pan et al. Two at Once: Enhancing Learning and Generalization
+ Capacities via IBN-Net. ECCV 2018.
+ """
+
+ def __init__(
+ self,
+ block,
+ layers,
+ num_classes=1000,
+ loss='softmax',
+ fc_dims=None,
+ dropout_p=None,
+ **kwargs
+ ):
+ scale = 64
+ self.inplanes = scale
+ super(ResNet, self).__init__()
+ self.loss = loss
+ self.feature_dim = scale * 8 * block.expansion
+
+ self.conv1 = nn.Conv2d(
+ 3, scale, kernel_size=7, stride=2, padding=3, bias=False
+ )
+ self.bn1 = nn.InstanceNorm2d(scale, affine=True)
+ self.relu = nn.ReLU(inplace=True)
+ self.maxpool = nn.MaxPool2d(kernel_size=3, stride=2, padding=1)
+ self.layer1 = self._make_layer(
+ block, scale, layers[0], stride=1, IN=True
+ )
+ self.layer2 = self._make_layer(
+ block, scale * 2, layers[1], stride=2, IN=True
+ )
+ self.layer3 = self._make_layer(block, scale * 4, layers[2], stride=2)
+ self.layer4 = self._make_layer(block, scale * 8, layers[3], stride=2)
+ self.avgpool = nn.AdaptiveAvgPool2d((1, 1))
+ self.fc = self._construct_fc_layer(
+ fc_dims, scale * 8 * block.expansion, dropout_p
+ )
+ self.classifier = nn.Linear(self.feature_dim, num_classes)
+
+ for m in self.modules():
+ if isinstance(m, nn.Conv2d):
+ n = m.kernel_size[0] * m.kernel_size[1] * m.out_channels
+ m.weight.data.normal_(0, math.sqrt(2. / n))
+ elif isinstance(m, nn.BatchNorm2d):
+ m.weight.data.fill_(1)
+ m.bias.data.zero_()
+ elif isinstance(m, nn.InstanceNorm2d):
+ m.weight.data.fill_(1)
+ m.bias.data.zero_()
+
+ def _make_layer(self, block, planes, blocks, stride=1, IN=False):
+ downsample = None
+ if stride != 1 or self.inplanes != planes * block.expansion:
+ downsample = nn.Sequential(
+ nn.Conv2d(
+ self.inplanes,
+ planes * block.expansion,
+ kernel_size=1,
+ stride=stride,
+ bias=False
+ ),
+ nn.BatchNorm2d(planes * block.expansion),
+ )
+
+ layers = []
+ layers.append(block(self.inplanes, planes, stride, downsample))
+ self.inplanes = planes * block.expansion
+ for i in range(1, blocks - 1):
+ layers.append(block(self.inplanes, planes))
+ layers.append(block(self.inplanes, planes, IN=IN))
+
+ return nn.Sequential(*layers)
+
+ def _construct_fc_layer(self, fc_dims, input_dim, dropout_p=None):
+ """Constructs fully connected layer
+
+ Args:
+ fc_dims (list or tuple): dimensions of fc layers, if None, no fc layers are constructed
+ input_dim (int): input dimension
+ dropout_p (float): dropout probability, if None, dropout is unused
+ """
+ if fc_dims is None:
+ self.feature_dim = input_dim
+ return None
+
+ assert isinstance(
+ fc_dims, (list, tuple)
+ ), 'fc_dims must be either list or tuple, but got {}'.format(
+ type(fc_dims)
+ )
+
+ layers = []
+ for dim in fc_dims:
+ layers.append(nn.Linear(input_dim, dim))
+ layers.append(nn.BatchNorm1d(dim))
+ layers.append(nn.ReLU(inplace=True))
+ if dropout_p is not None:
+ layers.append(nn.Dropout(p=dropout_p))
+ input_dim = dim
+
+ self.feature_dim = fc_dims[-1]
+
+ return nn.Sequential(*layers)
+
+ def featuremaps(self, x):
+ x = self.conv1(x)
+ x = self.bn1(x)
+ x = self.relu(x)
+ x = self.maxpool(x)
+ x = self.layer1(x)
+ x = self.layer2(x)
+ x = self.layer3(x)
+ x = self.layer4(x)
+ return x
+
+ def forward(self, x):
+ f = self.featuremaps(x)
+ v = self.avgpool(f)
+ v = v.view(v.size(0), -1)
+ if self.fc is not None:
+ v = self.fc(v)
+ if not self.training:
+ return v
+ y = self.classifier(v)
+ if self.loss == 'softmax':
+ return y
+ elif self.loss == 'triplet':
+ return y, v
+ else:
+ raise KeyError("Unsupported loss: {}".format(self.loss))
+
+
+def init_pretrained_weights(model, model_url):
+ """Initializes model with pretrained weights.
+
+ Layers that don't match with pretrained layers in name or size are kept unchanged.
+ """
+ pretrain_dict = model_zoo.load_url(model_url)
+ model_dict = model.state_dict()
+ pretrain_dict = {
+ k: v
+ for k, v in pretrain_dict.items()
+ if k in model_dict and model_dict[k].size() == v.size()
+ }
+ model_dict.update(pretrain_dict)
+ model.load_state_dict(model_dict)
+
+
+def resnet50_ibn_b(num_classes, loss='softmax', pretrained=False, **kwargs):
+ model = ResNet(
+ Bottleneck, [3, 4, 6, 3], num_classes=num_classes, loss=loss, **kwargs
+ )
+ if pretrained:
+ init_pretrained_weights(model, model_urls['resnet50'])
+ return model
diff --git a/trackers/strong_sort/deep/reid/torchreid/models/resnetmid.py b/trackers/strong_sort/deep/reid/torchreid/models/resnetmid.py
new file mode 100644
index 0000000000000000000000000000000000000000..017f6c62653535a7b04566227d893cb4dfa2a34c
--- /dev/null
+++ b/trackers/strong_sort/deep/reid/torchreid/models/resnetmid.py
@@ -0,0 +1,307 @@
+from __future__ import division, absolute_import
+import torch
+import torch.utils.model_zoo as model_zoo
+from torch import nn
+
+__all__ = ['resnet50mid']
+
+model_urls = {
+ 'resnet18': 'https://download.pytorch.org/models/resnet18-5c106cde.pth',
+ 'resnet34': 'https://download.pytorch.org/models/resnet34-333f7ec4.pth',
+ 'resnet50': 'https://download.pytorch.org/models/resnet50-19c8e357.pth',
+ 'resnet101': 'https://download.pytorch.org/models/resnet101-5d3b4d8f.pth',
+ 'resnet152': 'https://download.pytorch.org/models/resnet152-b121ed2d.pth',
+}
+
+
+def conv3x3(in_planes, out_planes, stride=1):
+ """3x3 convolution with padding"""
+ return nn.Conv2d(
+ in_planes,
+ out_planes,
+ kernel_size=3,
+ stride=stride,
+ padding=1,
+ bias=False
+ )
+
+
+class BasicBlock(nn.Module):
+ expansion = 1
+
+ def __init__(self, inplanes, planes, stride=1, downsample=None):
+ super(BasicBlock, self).__init__()
+ self.conv1 = conv3x3(inplanes, planes, stride)
+ self.bn1 = nn.BatchNorm2d(planes)
+ self.relu = nn.ReLU(inplace=True)
+ self.conv2 = conv3x3(planes, planes)
+ self.bn2 = nn.BatchNorm2d(planes)
+ self.downsample = downsample
+ self.stride = stride
+
+ def forward(self, x):
+ residual = x
+
+ out = self.conv1(x)
+ out = self.bn1(out)
+ out = self.relu(out)
+
+ out = self.conv2(out)
+ out = self.bn2(out)
+
+ if self.downsample is not None:
+ residual = self.downsample(x)
+
+ out += residual
+ out = self.relu(out)
+
+ return out
+
+
+class Bottleneck(nn.Module):
+ expansion = 4
+
+ def __init__(self, inplanes, planes, stride=1, downsample=None):
+ super(Bottleneck, self).__init__()
+ self.conv1 = nn.Conv2d(inplanes, planes, kernel_size=1, bias=False)
+ self.bn1 = nn.BatchNorm2d(planes)
+ self.conv2 = nn.Conv2d(
+ planes,
+ planes,
+ kernel_size=3,
+ stride=stride,
+ padding=1,
+ bias=False
+ )
+ self.bn2 = nn.BatchNorm2d(planes)
+ self.conv3 = nn.Conv2d(
+ planes, planes * self.expansion, kernel_size=1, bias=False
+ )
+ self.bn3 = nn.BatchNorm2d(planes * self.expansion)
+ self.relu = nn.ReLU(inplace=True)
+ self.downsample = downsample
+ self.stride = stride
+
+ def forward(self, x):
+ residual = x
+
+ out = self.conv1(x)
+ out = self.bn1(out)
+ out = self.relu(out)
+
+ out = self.conv2(out)
+ out = self.bn2(out)
+ out = self.relu(out)
+
+ out = self.conv3(out)
+ out = self.bn3(out)
+
+ if self.downsample is not None:
+ residual = self.downsample(x)
+
+ out += residual
+ out = self.relu(out)
+
+ return out
+
+
+class ResNetMid(nn.Module):
+ """Residual network + mid-level features.
+
+ Reference:
+ Yu et al. The Devil is in the Middle: Exploiting Mid-level Representations for
+ Cross-Domain Instance Matching. arXiv:1711.08106.
+
+ Public keys:
+ - ``resnet50mid``: ResNet50 + mid-level feature fusion.
+ """
+
+ def __init__(
+ self,
+ num_classes,
+ loss,
+ block,
+ layers,
+ last_stride=2,
+ fc_dims=None,
+ **kwargs
+ ):
+ self.inplanes = 64
+ super(ResNetMid, self).__init__()
+ self.loss = loss
+ self.feature_dim = 512 * block.expansion
+
+ # backbone network
+ self.conv1 = nn.Conv2d(
+ 3, 64, kernel_size=7, stride=2, padding=3, bias=False
+ )
+ self.bn1 = nn.BatchNorm2d(64)
+ self.relu = nn.ReLU(inplace=True)
+ self.maxpool = nn.MaxPool2d(kernel_size=3, stride=2, padding=1)
+ self.layer1 = self._make_layer(block, 64, layers[0])
+ self.layer2 = self._make_layer(block, 128, layers[1], stride=2)
+ self.layer3 = self._make_layer(block, 256, layers[2], stride=2)
+ self.layer4 = self._make_layer(
+ block, 512, layers[3], stride=last_stride
+ )
+
+ self.global_avgpool = nn.AdaptiveAvgPool2d(1)
+ assert fc_dims is not None
+ self.fc_fusion = self._construct_fc_layer(
+ fc_dims, 512 * block.expansion * 2
+ )
+ self.feature_dim += 512 * block.expansion
+ self.classifier = nn.Linear(self.feature_dim, num_classes)
+
+ self._init_params()
+
+ def _make_layer(self, block, planes, blocks, stride=1):
+ downsample = None
+ if stride != 1 or self.inplanes != planes * block.expansion:
+ downsample = nn.Sequential(
+ nn.Conv2d(
+ self.inplanes,
+ planes * block.expansion,
+ kernel_size=1,
+ stride=stride,
+ bias=False
+ ),
+ nn.BatchNorm2d(planes * block.expansion),
+ )
+
+ layers = []
+ layers.append(block(self.inplanes, planes, stride, downsample))
+ self.inplanes = planes * block.expansion
+ for i in range(1, blocks):
+ layers.append(block(self.inplanes, planes))
+
+ return nn.Sequential(*layers)
+
+ def _construct_fc_layer(self, fc_dims, input_dim, dropout_p=None):
+ """Constructs fully connected layer
+
+ Args:
+ fc_dims (list or tuple): dimensions of fc layers, if None, no fc layers are constructed
+ input_dim (int): input dimension
+ dropout_p (float): dropout probability, if None, dropout is unused
+ """
+ if fc_dims is None:
+ self.feature_dim = input_dim
+ return None
+
+ assert isinstance(
+ fc_dims, (list, tuple)
+ ), 'fc_dims must be either list or tuple, but got {}'.format(
+ type(fc_dims)
+ )
+
+ layers = []
+ for dim in fc_dims:
+ layers.append(nn.Linear(input_dim, dim))
+ layers.append(nn.BatchNorm1d(dim))
+ layers.append(nn.ReLU(inplace=True))
+ if dropout_p is not None:
+ layers.append(nn.Dropout(p=dropout_p))
+ input_dim = dim
+
+ self.feature_dim = fc_dims[-1]
+
+ return nn.Sequential(*layers)
+
+ def _init_params(self):
+ for m in self.modules():
+ if isinstance(m, nn.Conv2d):
+ nn.init.kaiming_normal_(
+ m.weight, mode='fan_out', nonlinearity='relu'
+ )
+ if m.bias is not None:
+ nn.init.constant_(m.bias, 0)
+ elif isinstance(m, nn.BatchNorm2d):
+ nn.init.constant_(m.weight, 1)
+ nn.init.constant_(m.bias, 0)
+ elif isinstance(m, nn.BatchNorm1d):
+ nn.init.constant_(m.weight, 1)
+ nn.init.constant_(m.bias, 0)
+ elif isinstance(m, nn.Linear):
+ nn.init.normal_(m.weight, 0, 0.01)
+ if m.bias is not None:
+ nn.init.constant_(m.bias, 0)
+
+ def featuremaps(self, x):
+ x = self.conv1(x)
+ x = self.bn1(x)
+ x = self.relu(x)
+ x = self.maxpool(x)
+ x = self.layer1(x)
+ x = self.layer2(x)
+ x = self.layer3(x)
+ x4a = self.layer4[0](x)
+ x4b = self.layer4[1](x4a)
+ x4c = self.layer4[2](x4b)
+ return x4a, x4b, x4c
+
+ def forward(self, x):
+ x4a, x4b, x4c = self.featuremaps(x)
+
+ v4a = self.global_avgpool(x4a)
+ v4b = self.global_avgpool(x4b)
+ v4c = self.global_avgpool(x4c)
+ v4ab = torch.cat([v4a, v4b], 1)
+ v4ab = v4ab.view(v4ab.size(0), -1)
+ v4ab = self.fc_fusion(v4ab)
+ v4c = v4c.view(v4c.size(0), -1)
+ v = torch.cat([v4ab, v4c], 1)
+
+ if not self.training:
+ return v
+
+ y = self.classifier(v)
+
+ if self.loss == 'softmax':
+ return y
+ elif self.loss == 'triplet':
+ return y, v
+ else:
+ raise KeyError('Unsupported loss: {}'.format(self.loss))
+
+
+def init_pretrained_weights(model, model_url):
+ """Initializes model with pretrained weights.
+
+ Layers that don't match with pretrained layers in name or size are kept unchanged.
+ """
+ pretrain_dict = model_zoo.load_url(model_url)
+ model_dict = model.state_dict()
+ pretrain_dict = {
+ k: v
+ for k, v in pretrain_dict.items()
+ if k in model_dict and model_dict[k].size() == v.size()
+ }
+ model_dict.update(pretrain_dict)
+ model.load_state_dict(model_dict)
+
+
+"""
+Residual network configurations:
+--
+resnet18: block=BasicBlock, layers=[2, 2, 2, 2]
+resnet34: block=BasicBlock, layers=[3, 4, 6, 3]
+resnet50: block=Bottleneck, layers=[3, 4, 6, 3]
+resnet101: block=Bottleneck, layers=[3, 4, 23, 3]
+resnet152: block=Bottleneck, layers=[3, 8, 36, 3]
+"""
+
+
+def resnet50mid(num_classes, loss='softmax', pretrained=True, **kwargs):
+ model = ResNetMid(
+ num_classes=num_classes,
+ loss=loss,
+ block=Bottleneck,
+ layers=[3, 4, 6, 3],
+ last_stride=2,
+ fc_dims=[1024],
+ **kwargs
+ )
+ if pretrained:
+ init_pretrained_weights(model, model_urls['resnet50'])
+ return model
diff --git a/trackers/strong_sort/deep/reid/torchreid/models/senet.py b/trackers/strong_sort/deep/reid/torchreid/models/senet.py
new file mode 100644
index 0000000000000000000000000000000000000000..baaf9b0acbe8577bd5e574de47d3f9ef935946db
--- /dev/null
+++ b/trackers/strong_sort/deep/reid/torchreid/models/senet.py
@@ -0,0 +1,688 @@
+from __future__ import division, absolute_import
+import math
+from collections import OrderedDict
+import torch.nn as nn
+from torch.utils import model_zoo
+
+__all__ = [
+ 'senet154', 'se_resnet50', 'se_resnet101', 'se_resnet152',
+ 'se_resnext50_32x4d', 'se_resnext101_32x4d', 'se_resnet50_fc512'
+]
+"""
+Code imported from https://github.com/Cadene/pretrained-models.pytorch
+"""
+
+pretrained_settings = {
+ 'senet154': {
+ 'imagenet': {
+ 'url':
+ 'http://data.lip6.fr/cadene/pretrainedmodels/senet154-c7b49a05.pth',
+ 'input_space': 'RGB',
+ 'input_size': [3, 224, 224],
+ 'input_range': [0, 1],
+ 'mean': [0.485, 0.456, 0.406],
+ 'std': [0.229, 0.224, 0.225],
+ 'num_classes': 1000
+ }
+ },
+ 'se_resnet50': {
+ 'imagenet': {
+ 'url':
+ 'http://data.lip6.fr/cadene/pretrainedmodels/se_resnet50-ce0d4300.pth',
+ 'input_space': 'RGB',
+ 'input_size': [3, 224, 224],
+ 'input_range': [0, 1],
+ 'mean': [0.485, 0.456, 0.406],
+ 'std': [0.229, 0.224, 0.225],
+ 'num_classes': 1000
+ }
+ },
+ 'se_resnet101': {
+ 'imagenet': {
+ 'url':
+ 'http://data.lip6.fr/cadene/pretrainedmodels/se_resnet101-7e38fcc6.pth',
+ 'input_space': 'RGB',
+ 'input_size': [3, 224, 224],
+ 'input_range': [0, 1],
+ 'mean': [0.485, 0.456, 0.406],
+ 'std': [0.229, 0.224, 0.225],
+ 'num_classes': 1000
+ }
+ },
+ 'se_resnet152': {
+ 'imagenet': {
+ 'url':
+ 'http://data.lip6.fr/cadene/pretrainedmodels/se_resnet152-d17c99b7.pth',
+ 'input_space': 'RGB',
+ 'input_size': [3, 224, 224],
+ 'input_range': [0, 1],
+ 'mean': [0.485, 0.456, 0.406],
+ 'std': [0.229, 0.224, 0.225],
+ 'num_classes': 1000
+ }
+ },
+ 'se_resnext50_32x4d': {
+ 'imagenet': {
+ 'url':
+ 'http://data.lip6.fr/cadene/pretrainedmodels/se_resnext50_32x4d-a260b3a4.pth',
+ 'input_space': 'RGB',
+ 'input_size': [3, 224, 224],
+ 'input_range': [0, 1],
+ 'mean': [0.485, 0.456, 0.406],
+ 'std': [0.229, 0.224, 0.225],
+ 'num_classes': 1000
+ }
+ },
+ 'se_resnext101_32x4d': {
+ 'imagenet': {
+ 'url':
+ 'http://data.lip6.fr/cadene/pretrainedmodels/se_resnext101_32x4d-3b2fe3d8.pth',
+ 'input_space': 'RGB',
+ 'input_size': [3, 224, 224],
+ 'input_range': [0, 1],
+ 'mean': [0.485, 0.456, 0.406],
+ 'std': [0.229, 0.224, 0.225],
+ 'num_classes': 1000
+ }
+ },
+}
+
+
+class SEModule(nn.Module):
+
+ def __init__(self, channels, reduction):
+ super(SEModule, self).__init__()
+ self.avg_pool = nn.AdaptiveAvgPool2d(1)
+ self.fc1 = nn.Conv2d(
+ channels, channels // reduction, kernel_size=1, padding=0
+ )
+ self.relu = nn.ReLU(inplace=True)
+ self.fc2 = nn.Conv2d(
+ channels // reduction, channels, kernel_size=1, padding=0
+ )
+ self.sigmoid = nn.Sigmoid()
+
+ def forward(self, x):
+ module_input = x
+ x = self.avg_pool(x)
+ x = self.fc1(x)
+ x = self.relu(x)
+ x = self.fc2(x)
+ x = self.sigmoid(x)
+ return module_input * x
+
+
+class Bottleneck(nn.Module):
+ """
+ Base class for bottlenecks that implements `forward()` method.
+ """
+
+ def forward(self, x):
+ residual = x
+
+ out = self.conv1(x)
+ out = self.bn1(out)
+ out = self.relu(out)
+
+ out = self.conv2(out)
+ out = self.bn2(out)
+ out = self.relu(out)
+
+ out = self.conv3(out)
+ out = self.bn3(out)
+
+ if self.downsample is not None:
+ residual = self.downsample(x)
+
+ out = self.se_module(out) + residual
+ out = self.relu(out)
+
+ return out
+
+
+class SEBottleneck(Bottleneck):
+ """
+ Bottleneck for SENet154.
+ """
+ expansion = 4
+
+ def __init__(
+ self, inplanes, planes, groups, reduction, stride=1, downsample=None
+ ):
+ super(SEBottleneck, self).__init__()
+ self.conv1 = nn.Conv2d(inplanes, planes * 2, kernel_size=1, bias=False)
+ self.bn1 = nn.BatchNorm2d(planes * 2)
+ self.conv2 = nn.Conv2d(
+ planes * 2,
+ planes * 4,
+ kernel_size=3,
+ stride=stride,
+ padding=1,
+ groups=groups,
+ bias=False
+ )
+ self.bn2 = nn.BatchNorm2d(planes * 4)
+ self.conv3 = nn.Conv2d(
+ planes * 4, planes * 4, kernel_size=1, bias=False
+ )
+ self.bn3 = nn.BatchNorm2d(planes * 4)
+ self.relu = nn.ReLU(inplace=True)
+ self.se_module = SEModule(planes * 4, reduction=reduction)
+ self.downsample = downsample
+ self.stride = stride
+
+
+class SEResNetBottleneck(Bottleneck):
+ """
+ ResNet bottleneck with a Squeeze-and-Excitation module. It follows Caffe
+ implementation and uses `stride=stride` in `conv1` and not in `conv2`
+ (the latter is used in the torchvision implementation of ResNet).
+ """
+ expansion = 4
+
+ def __init__(
+ self, inplanes, planes, groups, reduction, stride=1, downsample=None
+ ):
+ super(SEResNetBottleneck, self).__init__()
+ self.conv1 = nn.Conv2d(
+ inplanes, planes, kernel_size=1, bias=False, stride=stride
+ )
+ self.bn1 = nn.BatchNorm2d(planes)
+ self.conv2 = nn.Conv2d(
+ planes,
+ planes,
+ kernel_size=3,
+ padding=1,
+ groups=groups,
+ bias=False
+ )
+ self.bn2 = nn.BatchNorm2d(planes)
+ self.conv3 = nn.Conv2d(planes, planes * 4, kernel_size=1, bias=False)
+ self.bn3 = nn.BatchNorm2d(planes * 4)
+ self.relu = nn.ReLU(inplace=True)
+ self.se_module = SEModule(planes * 4, reduction=reduction)
+ self.downsample = downsample
+ self.stride = stride
+
+
+class SEResNeXtBottleneck(Bottleneck):
+ """ResNeXt bottleneck type C with a Squeeze-and-Excitation module"""
+ expansion = 4
+
+ def __init__(
+ self,
+ inplanes,
+ planes,
+ groups,
+ reduction,
+ stride=1,
+ downsample=None,
+ base_width=4
+ ):
+ super(SEResNeXtBottleneck, self).__init__()
+ width = int(math.floor(planes * (base_width/64.)) * groups)
+ self.conv1 = nn.Conv2d(
+ inplanes, width, kernel_size=1, bias=False, stride=1
+ )
+ self.bn1 = nn.BatchNorm2d(width)
+ self.conv2 = nn.Conv2d(
+ width,
+ width,
+ kernel_size=3,
+ stride=stride,
+ padding=1,
+ groups=groups,
+ bias=False
+ )
+ self.bn2 = nn.BatchNorm2d(width)
+ self.conv3 = nn.Conv2d(width, planes * 4, kernel_size=1, bias=False)
+ self.bn3 = nn.BatchNorm2d(planes * 4)
+ self.relu = nn.ReLU(inplace=True)
+ self.se_module = SEModule(planes * 4, reduction=reduction)
+ self.downsample = downsample
+ self.stride = stride
+
+
+class SENet(nn.Module):
+ """Squeeze-and-excitation network.
+
+ Reference:
+ Hu et al. Squeeze-and-Excitation Networks. CVPR 2018.
+
+ Public keys:
+ - ``senet154``: SENet154.
+ - ``se_resnet50``: ResNet50 + SE.
+ - ``se_resnet101``: ResNet101 + SE.
+ - ``se_resnet152``: ResNet152 + SE.
+ - ``se_resnext50_32x4d``: ResNeXt50 (groups=32, width=4) + SE.
+ - ``se_resnext101_32x4d``: ResNeXt101 (groups=32, width=4) + SE.
+ - ``se_resnet50_fc512``: (ResNet50 + SE) + FC.
+ """
+
+ def __init__(
+ self,
+ num_classes,
+ loss,
+ block,
+ layers,
+ groups,
+ reduction,
+ dropout_p=0.2,
+ inplanes=128,
+ input_3x3=True,
+ downsample_kernel_size=3,
+ downsample_padding=1,
+ last_stride=2,
+ fc_dims=None,
+ **kwargs
+ ):
+ """
+ Parameters
+ ----------
+ block (nn.Module): Bottleneck class.
+ - For SENet154: SEBottleneck
+ - For SE-ResNet models: SEResNetBottleneck
+ - For SE-ResNeXt models: SEResNeXtBottleneck
+ layers (list of ints): Number of residual blocks for 4 layers of the
+ network (layer1...layer4).
+ groups (int): Number of groups for the 3x3 convolution in each
+ bottleneck block.
+ - For SENet154: 64
+ - For SE-ResNet models: 1
+ - For SE-ResNeXt models: 32
+ reduction (int): Reduction ratio for Squeeze-and-Excitation modules.
+ - For all models: 16
+ dropout_p (float or None): Drop probability for the Dropout layer.
+ If `None` the Dropout layer is not used.
+ - For SENet154: 0.2
+ - For SE-ResNet models: None
+ - For SE-ResNeXt models: None
+ inplanes (int): Number of input channels for layer1.
+ - For SENet154: 128
+ - For SE-ResNet models: 64
+ - For SE-ResNeXt models: 64
+ input_3x3 (bool): If `True`, use three 3x3 convolutions instead of
+ a single 7x7 convolution in layer0.
+ - For SENet154: True
+ - For SE-ResNet models: False
+ - For SE-ResNeXt models: False
+ downsample_kernel_size (int): Kernel size for downsampling convolutions
+ in layer2, layer3 and layer4.
+ - For SENet154: 3
+ - For SE-ResNet models: 1
+ - For SE-ResNeXt models: 1
+ downsample_padding (int): Padding for downsampling convolutions in
+ layer2, layer3 and layer4.
+ - For SENet154: 1
+ - For SE-ResNet models: 0
+ - For SE-ResNeXt models: 0
+ num_classes (int): Number of outputs in `classifier` layer.
+ """
+ super(SENet, self).__init__()
+ self.inplanes = inplanes
+ self.loss = loss
+
+ if input_3x3:
+ layer0_modules = [
+ (
+ 'conv1',
+ nn.Conv2d(3, 64, 3, stride=2, padding=1, bias=False)
+ ),
+ ('bn1', nn.BatchNorm2d(64)),
+ ('relu1', nn.ReLU(inplace=True)),
+ (
+ 'conv2',
+ nn.Conv2d(64, 64, 3, stride=1, padding=1, bias=False)
+ ),
+ ('bn2', nn.BatchNorm2d(64)),
+ ('relu2', nn.ReLU(inplace=True)),
+ (
+ 'conv3',
+ nn.Conv2d(
+ 64, inplanes, 3, stride=1, padding=1, bias=False
+ )
+ ),
+ ('bn3', nn.BatchNorm2d(inplanes)),
+ ('relu3', nn.ReLU(inplace=True)),
+ ]
+ else:
+ layer0_modules = [
+ (
+ 'conv1',
+ nn.Conv2d(
+ 3,
+ inplanes,
+ kernel_size=7,
+ stride=2,
+ padding=3,
+ bias=False
+ )
+ ),
+ ('bn1', nn.BatchNorm2d(inplanes)),
+ ('relu1', nn.ReLU(inplace=True)),
+ ]
+ # To preserve compatibility with Caffe weights `ceil_mode=True`
+ # is used instead of `padding=1`.
+ layer0_modules.append(
+ ('pool', nn.MaxPool2d(3, stride=2, ceil_mode=True))
+ )
+ self.layer0 = nn.Sequential(OrderedDict(layer0_modules))
+ self.layer1 = self._make_layer(
+ block,
+ planes=64,
+ blocks=layers[0],
+ groups=groups,
+ reduction=reduction,
+ downsample_kernel_size=1,
+ downsample_padding=0
+ )
+ self.layer2 = self._make_layer(
+ block,
+ planes=128,
+ blocks=layers[1],
+ stride=2,
+ groups=groups,
+ reduction=reduction,
+ downsample_kernel_size=downsample_kernel_size,
+ downsample_padding=downsample_padding
+ )
+ self.layer3 = self._make_layer(
+ block,
+ planes=256,
+ blocks=layers[2],
+ stride=2,
+ groups=groups,
+ reduction=reduction,
+ downsample_kernel_size=downsample_kernel_size,
+ downsample_padding=downsample_padding
+ )
+ self.layer4 = self._make_layer(
+ block,
+ planes=512,
+ blocks=layers[3],
+ stride=last_stride,
+ groups=groups,
+ reduction=reduction,
+ downsample_kernel_size=downsample_kernel_size,
+ downsample_padding=downsample_padding
+ )
+
+ self.global_avgpool = nn.AdaptiveAvgPool2d(1)
+ self.fc = self._construct_fc_layer(
+ fc_dims, 512 * block.expansion, dropout_p
+ )
+ self.classifier = nn.Linear(self.feature_dim, num_classes)
+
+ def _make_layer(
+ self,
+ block,
+ planes,
+ blocks,
+ groups,
+ reduction,
+ stride=1,
+ downsample_kernel_size=1,
+ downsample_padding=0
+ ):
+ downsample = None
+ if stride != 1 or self.inplanes != planes * block.expansion:
+ downsample = nn.Sequential(
+ nn.Conv2d(
+ self.inplanes,
+ planes * block.expansion,
+ kernel_size=downsample_kernel_size,
+ stride=stride,
+ padding=downsample_padding,
+ bias=False
+ ),
+ nn.BatchNorm2d(planes * block.expansion),
+ )
+
+ layers = []
+ layers.append(
+ block(
+ self.inplanes, planes, groups, reduction, stride, downsample
+ )
+ )
+ self.inplanes = planes * block.expansion
+ for i in range(1, blocks):
+ layers.append(block(self.inplanes, planes, groups, reduction))
+
+ return nn.Sequential(*layers)
+
+ def _construct_fc_layer(self, fc_dims, input_dim, dropout_p=None):
+ """
+ Construct fully connected layer
+
+ - fc_dims (list or tuple): dimensions of fc layers, if None,
+ no fc layers are constructed
+ - input_dim (int): input dimension
+ - dropout_p (float): dropout probability, if None, dropout is unused
+ """
+ if fc_dims is None:
+ self.feature_dim = input_dim
+ return None
+
+ assert isinstance(
+ fc_dims, (list, tuple)
+ ), 'fc_dims must be either list or tuple, but got {}'.format(
+ type(fc_dims)
+ )
+
+ layers = []
+ for dim in fc_dims:
+ layers.append(nn.Linear(input_dim, dim))
+ layers.append(nn.BatchNorm1d(dim))
+ layers.append(nn.ReLU(inplace=True))
+ if dropout_p is not None:
+ layers.append(nn.Dropout(p=dropout_p))
+ input_dim = dim
+
+ self.feature_dim = fc_dims[-1]
+
+ return nn.Sequential(*layers)
+
+ def featuremaps(self, x):
+ x = self.layer0(x)
+ x = self.layer1(x)
+ x = self.layer2(x)
+ x = self.layer3(x)
+ x = self.layer4(x)
+ return x
+
+ def forward(self, x):
+ f = self.featuremaps(x)
+ v = self.global_avgpool(f)
+ v = v.view(v.size(0), -1)
+
+ if self.fc is not None:
+ v = self.fc(v)
+
+ if not self.training:
+ return v
+
+ y = self.classifier(v)
+
+ if self.loss == 'softmax':
+ return y
+ elif self.loss == 'triplet':
+ return y, v
+ else:
+ raise KeyError("Unsupported loss: {}".format(self.loss))
+
+
+def init_pretrained_weights(model, model_url):
+ """Initializes model with pretrained weights.
+
+ Layers that don't match with pretrained layers in name or size are kept unchanged.
+ """
+ pretrain_dict = model_zoo.load_url(model_url)
+ model_dict = model.state_dict()
+ pretrain_dict = {
+ k: v
+ for k, v in pretrain_dict.items()
+ if k in model_dict and model_dict[k].size() == v.size()
+ }
+ model_dict.update(pretrain_dict)
+ model.load_state_dict(model_dict)
+
+
+def senet154(num_classes, loss='softmax', pretrained=True, **kwargs):
+ model = SENet(
+ num_classes=num_classes,
+ loss=loss,
+ block=SEBottleneck,
+ layers=[3, 8, 36, 3],
+ groups=64,
+ reduction=16,
+ dropout_p=0.2,
+ last_stride=2,
+ fc_dims=None,
+ **kwargs
+ )
+ if pretrained:
+ model_url = pretrained_settings['senet154']['imagenet']['url']
+ init_pretrained_weights(model, model_url)
+ return model
+
+
+def se_resnet50(num_classes, loss='softmax', pretrained=True, **kwargs):
+ model = SENet(
+ num_classes=num_classes,
+ loss=loss,
+ block=SEResNetBottleneck,
+ layers=[3, 4, 6, 3],
+ groups=1,
+ reduction=16,
+ dropout_p=None,
+ inplanes=64,
+ input_3x3=False,
+ downsample_kernel_size=1,
+ downsample_padding=0,
+ last_stride=2,
+ fc_dims=None,
+ **kwargs
+ )
+ if pretrained:
+ model_url = pretrained_settings['se_resnet50']['imagenet']['url']
+ init_pretrained_weights(model, model_url)
+ return model
+
+
+def se_resnet50_fc512(num_classes, loss='softmax', pretrained=True, **kwargs):
+ model = SENet(
+ num_classes=num_classes,
+ loss=loss,
+ block=SEResNetBottleneck,
+ layers=[3, 4, 6, 3],
+ groups=1,
+ reduction=16,
+ dropout_p=None,
+ inplanes=64,
+ input_3x3=False,
+ downsample_kernel_size=1,
+ downsample_padding=0,
+ last_stride=1,
+ fc_dims=[512],
+ **kwargs
+ )
+ if pretrained:
+ model_url = pretrained_settings['se_resnet50']['imagenet']['url']
+ init_pretrained_weights(model, model_url)
+ return model
+
+
+def se_resnet101(num_classes, loss='softmax', pretrained=True, **kwargs):
+ model = SENet(
+ num_classes=num_classes,
+ loss=loss,
+ block=SEResNetBottleneck,
+ layers=[3, 4, 23, 3],
+ groups=1,
+ reduction=16,
+ dropout_p=None,
+ inplanes=64,
+ input_3x3=False,
+ downsample_kernel_size=1,
+ downsample_padding=0,
+ last_stride=2,
+ fc_dims=None,
+ **kwargs
+ )
+ if pretrained:
+ model_url = pretrained_settings['se_resnet101']['imagenet']['url']
+ init_pretrained_weights(model, model_url)
+ return model
+
+
+def se_resnet152(num_classes, loss='softmax', pretrained=True, **kwargs):
+ model = SENet(
+ num_classes=num_classes,
+ loss=loss,
+ block=SEResNetBottleneck,
+ layers=[3, 8, 36, 3],
+ groups=1,
+ reduction=16,
+ dropout_p=None,
+ inplanes=64,
+ input_3x3=False,
+ downsample_kernel_size=1,
+ downsample_padding=0,
+ last_stride=2,
+ fc_dims=None,
+ **kwargs
+ )
+ if pretrained:
+ model_url = pretrained_settings['se_resnet152']['imagenet']['url']
+ init_pretrained_weights(model, model_url)
+ return model
+
+
+def se_resnext50_32x4d(num_classes, loss='softmax', pretrained=True, **kwargs):
+ model = SENet(
+ num_classes=num_classes,
+ loss=loss,
+ block=SEResNeXtBottleneck,
+ layers=[3, 4, 6, 3],
+ groups=32,
+ reduction=16,
+ dropout_p=None,
+ inplanes=64,
+ input_3x3=False,
+ downsample_kernel_size=1,
+ downsample_padding=0,
+ last_stride=2,
+ fc_dims=None,
+ **kwargs
+ )
+ if pretrained:
+ model_url = pretrained_settings['se_resnext50_32x4d']['imagenet']['url'
+ ]
+ init_pretrained_weights(model, model_url)
+ return model
+
+
+def se_resnext101_32x4d(
+ num_classes, loss='softmax', pretrained=True, **kwargs
+):
+ model = SENet(
+ num_classes=num_classes,
+ loss=loss,
+ block=SEResNeXtBottleneck,
+ layers=[3, 4, 23, 3],
+ groups=32,
+ reduction=16,
+ dropout_p=None,
+ inplanes=64,
+ input_3x3=False,
+ downsample_kernel_size=1,
+ downsample_padding=0,
+ last_stride=2,
+ fc_dims=None,
+ **kwargs
+ )
+ if pretrained:
+ model_url = pretrained_settings['se_resnext101_32x4d']['imagenet'][
+ 'url']
+ init_pretrained_weights(model, model_url)
+ return model
diff --git a/trackers/strong_sort/deep/reid/torchreid/models/shufflenet.py b/trackers/strong_sort/deep/reid/torchreid/models/shufflenet.py
new file mode 100644
index 0000000000000000000000000000000000000000..bc4d34f1c4a631aa981cfb1797b036f23aed4503
--- /dev/null
+++ b/trackers/strong_sort/deep/reid/torchreid/models/shufflenet.py
@@ -0,0 +1,198 @@
+from __future__ import division, absolute_import
+import torch
+import torch.utils.model_zoo as model_zoo
+from torch import nn
+from torch.nn import functional as F
+
+__all__ = ['shufflenet']
+
+model_urls = {
+ # training epoch = 90, top1 = 61.8
+ 'imagenet':
+ 'https://mega.nz/#!RDpUlQCY!tr_5xBEkelzDjveIYBBcGcovNCOrgfiJO9kiidz9fZM',
+}
+
+
+class ChannelShuffle(nn.Module):
+
+ def __init__(self, num_groups):
+ super(ChannelShuffle, self).__init__()
+ self.g = num_groups
+
+ def forward(self, x):
+ b, c, h, w = x.size()
+ n = c // self.g
+ # reshape
+ x = x.view(b, self.g, n, h, w)
+ # transpose
+ x = x.permute(0, 2, 1, 3, 4).contiguous()
+ # flatten
+ x = x.view(b, c, h, w)
+ return x
+
+
+class Bottleneck(nn.Module):
+
+ def __init__(
+ self,
+ in_channels,
+ out_channels,
+ stride,
+ num_groups,
+ group_conv1x1=True
+ ):
+ super(Bottleneck, self).__init__()
+ assert stride in [1, 2], 'Warning: stride must be either 1 or 2'
+ self.stride = stride
+ mid_channels = out_channels // 4
+ if stride == 2:
+ out_channels -= in_channels
+ # group conv is not applied to first conv1x1 at stage 2
+ num_groups_conv1x1 = num_groups if group_conv1x1 else 1
+ self.conv1 = nn.Conv2d(
+ in_channels,
+ mid_channels,
+ 1,
+ groups=num_groups_conv1x1,
+ bias=False
+ )
+ self.bn1 = nn.BatchNorm2d(mid_channels)
+ self.shuffle1 = ChannelShuffle(num_groups)
+ self.conv2 = nn.Conv2d(
+ mid_channels,
+ mid_channels,
+ 3,
+ stride=stride,
+ padding=1,
+ groups=mid_channels,
+ bias=False
+ )
+ self.bn2 = nn.BatchNorm2d(mid_channels)
+ self.conv3 = nn.Conv2d(
+ mid_channels, out_channels, 1, groups=num_groups, bias=False
+ )
+ self.bn3 = nn.BatchNorm2d(out_channels)
+ if stride == 2:
+ self.shortcut = nn.AvgPool2d(3, stride=2, padding=1)
+
+ def forward(self, x):
+ out = F.relu(self.bn1(self.conv1(x)))
+ out = self.shuffle1(out)
+ out = self.bn2(self.conv2(out))
+ out = self.bn3(self.conv3(out))
+ if self.stride == 2:
+ res = self.shortcut(x)
+ out = F.relu(torch.cat([res, out], 1))
+ else:
+ out = F.relu(x + out)
+ return out
+
+
+# configuration of (num_groups: #out_channels) based on Table 1 in the paper
+cfg = {
+ 1: [144, 288, 576],
+ 2: [200, 400, 800],
+ 3: [240, 480, 960],
+ 4: [272, 544, 1088],
+ 8: [384, 768, 1536],
+}
+
+
+class ShuffleNet(nn.Module):
+ """ShuffleNet.
+
+ Reference:
+ Zhang et al. ShuffleNet: An Extremely Efficient Convolutional Neural
+ Network for Mobile Devices. CVPR 2018.
+
+ Public keys:
+ - ``shufflenet``: ShuffleNet (groups=3).
+ """
+
+ def __init__(self, num_classes, loss='softmax', num_groups=3, **kwargs):
+ super(ShuffleNet, self).__init__()
+ self.loss = loss
+
+ self.conv1 = nn.Sequential(
+ nn.Conv2d(3, 24, 3, stride=2, padding=1, bias=False),
+ nn.BatchNorm2d(24),
+ nn.ReLU(),
+ nn.MaxPool2d(3, stride=2, padding=1),
+ )
+
+ self.stage2 = nn.Sequential(
+ Bottleneck(
+ 24, cfg[num_groups][0], 2, num_groups, group_conv1x1=False
+ ),
+ Bottleneck(cfg[num_groups][0], cfg[num_groups][0], 1, num_groups),
+ Bottleneck(cfg[num_groups][0], cfg[num_groups][0], 1, num_groups),
+ Bottleneck(cfg[num_groups][0], cfg[num_groups][0], 1, num_groups),
+ )
+
+ self.stage3 = nn.Sequential(
+ Bottleneck(cfg[num_groups][0], cfg[num_groups][1], 2, num_groups),
+ Bottleneck(cfg[num_groups][1], cfg[num_groups][1], 1, num_groups),
+ Bottleneck(cfg[num_groups][1], cfg[num_groups][1], 1, num_groups),
+ Bottleneck(cfg[num_groups][1], cfg[num_groups][1], 1, num_groups),
+ Bottleneck(cfg[num_groups][1], cfg[num_groups][1], 1, num_groups),
+ Bottleneck(cfg[num_groups][1], cfg[num_groups][1], 1, num_groups),
+ Bottleneck(cfg[num_groups][1], cfg[num_groups][1], 1, num_groups),
+ Bottleneck(cfg[num_groups][1], cfg[num_groups][1], 1, num_groups),
+ )
+
+ self.stage4 = nn.Sequential(
+ Bottleneck(cfg[num_groups][1], cfg[num_groups][2], 2, num_groups),
+ Bottleneck(cfg[num_groups][2], cfg[num_groups][2], 1, num_groups),
+ Bottleneck(cfg[num_groups][2], cfg[num_groups][2], 1, num_groups),
+ Bottleneck(cfg[num_groups][2], cfg[num_groups][2], 1, num_groups),
+ )
+
+ self.classifier = nn.Linear(cfg[num_groups][2], num_classes)
+ self.feat_dim = cfg[num_groups][2]
+
+ def forward(self, x):
+ x = self.conv1(x)
+ x = self.stage2(x)
+ x = self.stage3(x)
+ x = self.stage4(x)
+ x = F.avg_pool2d(x, x.size()[2:]).view(x.size(0), -1)
+
+ if not self.training:
+ return x
+
+ y = self.classifier(x)
+
+ if self.loss == 'softmax':
+ return y
+ elif self.loss == 'triplet':
+ return y, x
+ else:
+ raise KeyError('Unsupported loss: {}'.format(self.loss))
+
+
+def init_pretrained_weights(model, model_url):
+ """Initializes model with pretrained weights.
+
+ Layers that don't match with pretrained layers in name or size are kept unchanged.
+ """
+ pretrain_dict = model_zoo.load_url(model_url)
+ model_dict = model.state_dict()
+ pretrain_dict = {
+ k: v
+ for k, v in pretrain_dict.items()
+ if k in model_dict and model_dict[k].size() == v.size()
+ }
+ model_dict.update(pretrain_dict)
+ model.load_state_dict(model_dict)
+
+
+def shufflenet(num_classes, loss='softmax', pretrained=True, **kwargs):
+ model = ShuffleNet(num_classes, loss, **kwargs)
+ if pretrained:
+ # init_pretrained_weights(model, model_urls['imagenet'])
+ import warnings
+ warnings.warn(
+ 'The imagenet pretrained weights need to be manually downloaded from {}'
+ .format(model_urls['imagenet'])
+ )
+ return model
diff --git a/trackers/strong_sort/deep/reid/torchreid/models/shufflenetv2.py b/trackers/strong_sort/deep/reid/torchreid/models/shufflenetv2.py
new file mode 100644
index 0000000000000000000000000000000000000000..3ff879e8d731b4cb16a77cfa6892035656405f71
--- /dev/null
+++ b/trackers/strong_sort/deep/reid/torchreid/models/shufflenetv2.py
@@ -0,0 +1,262 @@
+"""
+Code source: https://github.com/pytorch/vision
+"""
+from __future__ import division, absolute_import
+import torch
+import torch.utils.model_zoo as model_zoo
+from torch import nn
+
+__all__ = [
+ 'shufflenet_v2_x0_5', 'shufflenet_v2_x1_0', 'shufflenet_v2_x1_5',
+ 'shufflenet_v2_x2_0'
+]
+
+model_urls = {
+ 'shufflenetv2_x0.5':
+ 'https://download.pytorch.org/models/shufflenetv2_x0.5-f707e7126e.pth',
+ 'shufflenetv2_x1.0':
+ 'https://download.pytorch.org/models/shufflenetv2_x1-5666bf0f80.pth',
+ 'shufflenetv2_x1.5': None,
+ 'shufflenetv2_x2.0': None,
+}
+
+
+def channel_shuffle(x, groups):
+ batchsize, num_channels, height, width = x.data.size()
+ channels_per_group = num_channels // groups
+
+ # reshape
+ x = x.view(batchsize, groups, channels_per_group, height, width)
+
+ x = torch.transpose(x, 1, 2).contiguous()
+
+ # flatten
+ x = x.view(batchsize, -1, height, width)
+
+ return x
+
+
+class InvertedResidual(nn.Module):
+
+ def __init__(self, inp, oup, stride):
+ super(InvertedResidual, self).__init__()
+
+ if not (1 <= stride <= 3):
+ raise ValueError('illegal stride value')
+ self.stride = stride
+
+ branch_features = oup // 2
+ assert (self.stride != 1) or (inp == branch_features << 1)
+
+ if self.stride > 1:
+ self.branch1 = nn.Sequential(
+ self.depthwise_conv(
+ inp, inp, kernel_size=3, stride=self.stride, padding=1
+ ),
+ nn.BatchNorm2d(inp),
+ nn.Conv2d(
+ inp,
+ branch_features,
+ kernel_size=1,
+ stride=1,
+ padding=0,
+ bias=False
+ ),
+ nn.BatchNorm2d(branch_features),
+ nn.ReLU(inplace=True),
+ )
+
+ self.branch2 = nn.Sequential(
+ nn.Conv2d(
+ inp if (self.stride > 1) else branch_features,
+ branch_features,
+ kernel_size=1,
+ stride=1,
+ padding=0,
+ bias=False
+ ),
+ nn.BatchNorm2d(branch_features),
+ nn.ReLU(inplace=True),
+ self.depthwise_conv(
+ branch_features,
+ branch_features,
+ kernel_size=3,
+ stride=self.stride,
+ padding=1
+ ),
+ nn.BatchNorm2d(branch_features),
+ nn.Conv2d(
+ branch_features,
+ branch_features,
+ kernel_size=1,
+ stride=1,
+ padding=0,
+ bias=False
+ ),
+ nn.BatchNorm2d(branch_features),
+ nn.ReLU(inplace=True),
+ )
+
+ @staticmethod
+ def depthwise_conv(i, o, kernel_size, stride=1, padding=0, bias=False):
+ return nn.Conv2d(
+ i, o, kernel_size, stride, padding, bias=bias, groups=i
+ )
+
+ def forward(self, x):
+ if self.stride == 1:
+ x1, x2 = x.chunk(2, dim=1)
+ out = torch.cat((x1, self.branch2(x2)), dim=1)
+ else:
+ out = torch.cat((self.branch1(x), self.branch2(x)), dim=1)
+
+ out = channel_shuffle(out, 2)
+
+ return out
+
+
+class ShuffleNetV2(nn.Module):
+ """ShuffleNetV2.
+
+ Reference:
+ Ma et al. ShuffleNet V2: Practical Guidelines for Efficient CNN Architecture Design. ECCV 2018.
+
+ Public keys:
+ - ``shufflenet_v2_x0_5``: ShuffleNetV2 x0.5.
+ - ``shufflenet_v2_x1_0``: ShuffleNetV2 x1.0.
+ - ``shufflenet_v2_x1_5``: ShuffleNetV2 x1.5.
+ - ``shufflenet_v2_x2_0``: ShuffleNetV2 x2.0.
+ """
+
+ def __init__(
+ self, num_classes, loss, stages_repeats, stages_out_channels, **kwargs
+ ):
+ super(ShuffleNetV2, self).__init__()
+ self.loss = loss
+
+ if len(stages_repeats) != 3:
+ raise ValueError(
+ 'expected stages_repeats as list of 3 positive ints'
+ )
+ if len(stages_out_channels) != 5:
+ raise ValueError(
+ 'expected stages_out_channels as list of 5 positive ints'
+ )
+ self._stage_out_channels = stages_out_channels
+
+ input_channels = 3
+ output_channels = self._stage_out_channels[0]
+ self.conv1 = nn.Sequential(
+ nn.Conv2d(input_channels, output_channels, 3, 2, 1, bias=False),
+ nn.BatchNorm2d(output_channels),
+ nn.ReLU(inplace=True),
+ )
+ input_channels = output_channels
+
+ self.maxpool = nn.MaxPool2d(kernel_size=3, stride=2, padding=1)
+
+ stage_names = ['stage{}'.format(i) for i in [2, 3, 4]]
+ for name, repeats, output_channels in zip(
+ stage_names, stages_repeats, self._stage_out_channels[1:]
+ ):
+ seq = [InvertedResidual(input_channels, output_channels, 2)]
+ for i in range(repeats - 1):
+ seq.append(
+ InvertedResidual(output_channels, output_channels, 1)
+ )
+ setattr(self, name, nn.Sequential(*seq))
+ input_channels = output_channels
+
+ output_channels = self._stage_out_channels[-1]
+ self.conv5 = nn.Sequential(
+ nn.Conv2d(input_channels, output_channels, 1, 1, 0, bias=False),
+ nn.BatchNorm2d(output_channels),
+ nn.ReLU(inplace=True),
+ )
+ self.global_avgpool = nn.AdaptiveAvgPool2d((1, 1))
+
+ self.classifier = nn.Linear(output_channels, num_classes)
+
+ def featuremaps(self, x):
+ x = self.conv1(x)
+ x = self.maxpool(x)
+ x = self.stage2(x)
+ x = self.stage3(x)
+ x = self.stage4(x)
+ x = self.conv5(x)
+ return x
+
+ def forward(self, x):
+ f = self.featuremaps(x)
+ v = self.global_avgpool(f)
+ v = v.view(v.size(0), -1)
+
+ if not self.training:
+ return v
+
+ y = self.classifier(v)
+
+ if self.loss == 'softmax':
+ return y
+ elif self.loss == 'triplet':
+ return y, v
+ else:
+ raise KeyError("Unsupported loss: {}".format(self.loss))
+
+
+def init_pretrained_weights(model, model_url):
+ """Initializes model with pretrained weights.
+
+ Layers that don't match with pretrained layers in name or size are kept unchanged.
+ """
+ if model_url is None:
+ import warnings
+ warnings.warn(
+ 'ImageNet pretrained weights are unavailable for this model'
+ )
+ return
+ pretrain_dict = model_zoo.load_url(model_url)
+ model_dict = model.state_dict()
+ pretrain_dict = {
+ k: v
+ for k, v in pretrain_dict.items()
+ if k in model_dict and model_dict[k].size() == v.size()
+ }
+ model_dict.update(pretrain_dict)
+ model.load_state_dict(model_dict)
+
+
+def shufflenet_v2_x0_5(num_classes, loss='softmax', pretrained=True, **kwargs):
+ model = ShuffleNetV2(
+ num_classes, loss, [4, 8, 4], [24, 48, 96, 192, 1024], **kwargs
+ )
+ if pretrained:
+ init_pretrained_weights(model, model_urls['shufflenetv2_x0.5'])
+ return model
+
+
+def shufflenet_v2_x1_0(num_classes, loss='softmax', pretrained=True, **kwargs):
+ model = ShuffleNetV2(
+ num_classes, loss, [4, 8, 4], [24, 116, 232, 464, 1024], **kwargs
+ )
+ if pretrained:
+ init_pretrained_weights(model, model_urls['shufflenetv2_x1.0'])
+ return model
+
+
+def shufflenet_v2_x1_5(num_classes, loss='softmax', pretrained=True, **kwargs):
+ model = ShuffleNetV2(
+ num_classes, loss, [4, 8, 4], [24, 176, 352, 704, 1024], **kwargs
+ )
+ if pretrained:
+ init_pretrained_weights(model, model_urls['shufflenetv2_x1.5'])
+ return model
+
+
+def shufflenet_v2_x2_0(num_classes, loss='softmax', pretrained=True, **kwargs):
+ model = ShuffleNetV2(
+ num_classes, loss, [4, 8, 4], [24, 244, 488, 976, 2048], **kwargs
+ )
+ if pretrained:
+ init_pretrained_weights(model, model_urls['shufflenetv2_x2.0'])
+ return model
diff --git a/trackers/strong_sort/deep/reid/torchreid/models/squeezenet.py b/trackers/strong_sort/deep/reid/torchreid/models/squeezenet.py
new file mode 100644
index 0000000000000000000000000000000000000000..83e8dc9fc46b4e76304bf1b681a14ce5b865b993
--- /dev/null
+++ b/trackers/strong_sort/deep/reid/torchreid/models/squeezenet.py
@@ -0,0 +1,236 @@
+"""
+Code source: https://github.com/pytorch/vision
+"""
+from __future__ import division, absolute_import
+import torch
+import torch.nn as nn
+import torch.utils.model_zoo as model_zoo
+
+__all__ = ['squeezenet1_0', 'squeezenet1_1', 'squeezenet1_0_fc512']
+
+model_urls = {
+ 'squeezenet1_0':
+ 'https://download.pytorch.org/models/squeezenet1_0-a815701f.pth',
+ 'squeezenet1_1':
+ 'https://download.pytorch.org/models/squeezenet1_1-f364aa15.pth',
+}
+
+
+class Fire(nn.Module):
+
+ def __init__(
+ self, inplanes, squeeze_planes, expand1x1_planes, expand3x3_planes
+ ):
+ super(Fire, self).__init__()
+ self.inplanes = inplanes
+ self.squeeze = nn.Conv2d(inplanes, squeeze_planes, kernel_size=1)
+ self.squeeze_activation = nn.ReLU(inplace=True)
+ self.expand1x1 = nn.Conv2d(
+ squeeze_planes, expand1x1_planes, kernel_size=1
+ )
+ self.expand1x1_activation = nn.ReLU(inplace=True)
+ self.expand3x3 = nn.Conv2d(
+ squeeze_planes, expand3x3_planes, kernel_size=3, padding=1
+ )
+ self.expand3x3_activation = nn.ReLU(inplace=True)
+
+ def forward(self, x):
+ x = self.squeeze_activation(self.squeeze(x))
+ return torch.cat(
+ [
+ self.expand1x1_activation(self.expand1x1(x)),
+ self.expand3x3_activation(self.expand3x3(x))
+ ], 1
+ )
+
+
+class SqueezeNet(nn.Module):
+ """SqueezeNet.
+
+ Reference:
+ Iandola et al. SqueezeNet: AlexNet-level accuracy with 50x fewer parameters
+ and< 0.5 MB model size. arXiv:1602.07360.
+
+ Public keys:
+ - ``squeezenet1_0``: SqueezeNet (version=1.0).
+ - ``squeezenet1_1``: SqueezeNet (version=1.1).
+ - ``squeezenet1_0_fc512``: SqueezeNet (version=1.0) + FC.
+ """
+
+ def __init__(
+ self,
+ num_classes,
+ loss,
+ version=1.0,
+ fc_dims=None,
+ dropout_p=None,
+ **kwargs
+ ):
+ super(SqueezeNet, self).__init__()
+ self.loss = loss
+ self.feature_dim = 512
+
+ if version not in [1.0, 1.1]:
+ raise ValueError(
+ 'Unsupported SqueezeNet version {version}:'
+ '1.0 or 1.1 expected'.format(version=version)
+ )
+
+ if version == 1.0:
+ self.features = nn.Sequential(
+ nn.Conv2d(3, 96, kernel_size=7, stride=2),
+ nn.ReLU(inplace=True),
+ nn.MaxPool2d(kernel_size=3, stride=2, ceil_mode=True),
+ Fire(96, 16, 64, 64),
+ Fire(128, 16, 64, 64),
+ Fire(128, 32, 128, 128),
+ nn.MaxPool2d(kernel_size=3, stride=2, ceil_mode=True),
+ Fire(256, 32, 128, 128),
+ Fire(256, 48, 192, 192),
+ Fire(384, 48, 192, 192),
+ Fire(384, 64, 256, 256),
+ nn.MaxPool2d(kernel_size=3, stride=2, ceil_mode=True),
+ Fire(512, 64, 256, 256),
+ )
+ else:
+ self.features = nn.Sequential(
+ nn.Conv2d(3, 64, kernel_size=3, stride=2),
+ nn.ReLU(inplace=True),
+ nn.MaxPool2d(kernel_size=3, stride=2, ceil_mode=True),
+ Fire(64, 16, 64, 64),
+ Fire(128, 16, 64, 64),
+ nn.MaxPool2d(kernel_size=3, stride=2, ceil_mode=True),
+ Fire(128, 32, 128, 128),
+ Fire(256, 32, 128, 128),
+ nn.MaxPool2d(kernel_size=3, stride=2, ceil_mode=True),
+ Fire(256, 48, 192, 192),
+ Fire(384, 48, 192, 192),
+ Fire(384, 64, 256, 256),
+ Fire(512, 64, 256, 256),
+ )
+
+ self.global_avgpool = nn.AdaptiveAvgPool2d(1)
+ self.fc = self._construct_fc_layer(fc_dims, 512, dropout_p)
+ self.classifier = nn.Linear(self.feature_dim, num_classes)
+
+ self._init_params()
+
+ def _construct_fc_layer(self, fc_dims, input_dim, dropout_p=None):
+ """Constructs fully connected layer
+
+ Args:
+ fc_dims (list or tuple): dimensions of fc layers, if None, no fc layers are constructed
+ input_dim (int): input dimension
+ dropout_p (float): dropout probability, if None, dropout is unused
+ """
+ if fc_dims is None:
+ self.feature_dim = input_dim
+ return None
+
+ assert isinstance(
+ fc_dims, (list, tuple)
+ ), 'fc_dims must be either list or tuple, but got {}'.format(
+ type(fc_dims)
+ )
+
+ layers = []
+ for dim in fc_dims:
+ layers.append(nn.Linear(input_dim, dim))
+ layers.append(nn.BatchNorm1d(dim))
+ layers.append(nn.ReLU(inplace=True))
+ if dropout_p is not None:
+ layers.append(nn.Dropout(p=dropout_p))
+ input_dim = dim
+
+ self.feature_dim = fc_dims[-1]
+
+ return nn.Sequential(*layers)
+
+ def _init_params(self):
+ for m in self.modules():
+ if isinstance(m, nn.Conv2d):
+ nn.init.kaiming_normal_(
+ m.weight, mode='fan_out', nonlinearity='relu'
+ )
+ if m.bias is not None:
+ nn.init.constant_(m.bias, 0)
+ elif isinstance(m, nn.BatchNorm2d):
+ nn.init.constant_(m.weight, 1)
+ nn.init.constant_(m.bias, 0)
+ elif isinstance(m, nn.BatchNorm1d):
+ nn.init.constant_(m.weight, 1)
+ nn.init.constant_(m.bias, 0)
+ elif isinstance(m, nn.Linear):
+ nn.init.normal_(m.weight, 0, 0.01)
+ if m.bias is not None:
+ nn.init.constant_(m.bias, 0)
+
+ def forward(self, x):
+ f = self.features(x)
+ v = self.global_avgpool(f)
+ v = v.view(v.size(0), -1)
+
+ if self.fc is not None:
+ v = self.fc(v)
+
+ if not self.training:
+ return v
+
+ y = self.classifier(v)
+
+ if self.loss == 'softmax':
+ return y
+ elif self.loss == 'triplet':
+ return y, v
+ else:
+ raise KeyError('Unsupported loss: {}'.format(self.loss))
+
+
+def init_pretrained_weights(model, model_url):
+ """Initializes model with pretrained weights.
+
+ Layers that don't match with pretrained layers in name or size are kept unchanged.
+ """
+ pretrain_dict = model_zoo.load_url(model_url, map_location=None)
+ model_dict = model.state_dict()
+ pretrain_dict = {
+ k: v
+ for k, v in pretrain_dict.items()
+ if k in model_dict and model_dict[k].size() == v.size()
+ }
+ model_dict.update(pretrain_dict)
+ model.load_state_dict(model_dict)
+
+
+def squeezenet1_0(num_classes, loss='softmax', pretrained=True, **kwargs):
+ model = SqueezeNet(
+ num_classes, loss, version=1.0, fc_dims=None, dropout_p=None, **kwargs
+ )
+ if pretrained:
+ init_pretrained_weights(model, model_urls['squeezenet1_0'])
+ return model
+
+
+def squeezenet1_0_fc512(
+ num_classes, loss='softmax', pretrained=True, **kwargs
+):
+ model = SqueezeNet(
+ num_classes,
+ loss,
+ version=1.0,
+ fc_dims=[512],
+ dropout_p=None,
+ **kwargs
+ )
+ if pretrained:
+ init_pretrained_weights(model, model_urls['squeezenet1_0'])
+ return model
+
+
+def squeezenet1_1(num_classes, loss='softmax', pretrained=True, **kwargs):
+ model = SqueezeNet(
+ num_classes, loss, version=1.1, fc_dims=None, dropout_p=None, **kwargs
+ )
+ if pretrained:
+ init_pretrained_weights(model, model_urls['squeezenet1_1'])
+ return model
diff --git a/trackers/strong_sort/deep/reid/torchreid/models/xception.py b/trackers/strong_sort/deep/reid/torchreid/models/xception.py
new file mode 100644
index 0000000000000000000000000000000000000000..43db4ab53283daf1267f2f4cc5f7d778daf4076a
--- /dev/null
+++ b/trackers/strong_sort/deep/reid/torchreid/models/xception.py
@@ -0,0 +1,344 @@
+from __future__ import division, absolute_import
+import torch.nn as nn
+import torch.nn.functional as F
+import torch.utils.model_zoo as model_zoo
+
+__all__ = ['xception']
+
+pretrained_settings = {
+ 'xception': {
+ 'imagenet': {
+ 'url':
+ 'http://data.lip6.fr/cadene/pretrainedmodels/xception-43020ad28.pth',
+ 'input_space': 'RGB',
+ 'input_size': [3, 299, 299],
+ 'input_range': [0, 1],
+ 'mean': [0.5, 0.5, 0.5],
+ 'std': [0.5, 0.5, 0.5],
+ 'num_classes': 1000,
+ 'scale':
+ 0.8975 # The resize parameter of the validation transform should be 333, and make sure to center crop at 299x299
+ }
+ }
+}
+
+
+class SeparableConv2d(nn.Module):
+
+ def __init__(
+ self,
+ in_channels,
+ out_channels,
+ kernel_size=1,
+ stride=1,
+ padding=0,
+ dilation=1,
+ bias=False
+ ):
+ super(SeparableConv2d, self).__init__()
+
+ self.conv1 = nn.Conv2d(
+ in_channels,
+ in_channels,
+ kernel_size,
+ stride,
+ padding,
+ dilation,
+ groups=in_channels,
+ bias=bias
+ )
+ self.pointwise = nn.Conv2d(
+ in_channels, out_channels, 1, 1, 0, 1, 1, bias=bias
+ )
+
+ def forward(self, x):
+ x = self.conv1(x)
+ x = self.pointwise(x)
+ return x
+
+
+class Block(nn.Module):
+
+ def __init__(
+ self,
+ in_filters,
+ out_filters,
+ reps,
+ strides=1,
+ start_with_relu=True,
+ grow_first=True
+ ):
+ super(Block, self).__init__()
+
+ if out_filters != in_filters or strides != 1:
+ self.skip = nn.Conv2d(
+ in_filters, out_filters, 1, stride=strides, bias=False
+ )
+ self.skipbn = nn.BatchNorm2d(out_filters)
+ else:
+ self.skip = None
+
+ self.relu = nn.ReLU(inplace=True)
+ rep = []
+
+ filters = in_filters
+ if grow_first:
+ rep.append(self.relu)
+ rep.append(
+ SeparableConv2d(
+ in_filters,
+ out_filters,
+ 3,
+ stride=1,
+ padding=1,
+ bias=False
+ )
+ )
+ rep.append(nn.BatchNorm2d(out_filters))
+ filters = out_filters
+
+ for i in range(reps - 1):
+ rep.append(self.relu)
+ rep.append(
+ SeparableConv2d(
+ filters, filters, 3, stride=1, padding=1, bias=False
+ )
+ )
+ rep.append(nn.BatchNorm2d(filters))
+
+ if not grow_first:
+ rep.append(self.relu)
+ rep.append(
+ SeparableConv2d(
+ in_filters,
+ out_filters,
+ 3,
+ stride=1,
+ padding=1,
+ bias=False
+ )
+ )
+ rep.append(nn.BatchNorm2d(out_filters))
+
+ if not start_with_relu:
+ rep = rep[1:]
+ else:
+ rep[0] = nn.ReLU(inplace=False)
+
+ if strides != 1:
+ rep.append(nn.MaxPool2d(3, strides, 1))
+ self.rep = nn.Sequential(*rep)
+
+ def forward(self, inp):
+ x = self.rep(inp)
+
+ if self.skip is not None:
+ skip = self.skip(inp)
+ skip = self.skipbn(skip)
+ else:
+ skip = inp
+
+ x += skip
+ return x
+
+
+class Xception(nn.Module):
+ """Xception.
+
+ Reference:
+ Chollet. Xception: Deep Learning with Depthwise
+ Separable Convolutions. CVPR 2017.
+
+ Public keys:
+ - ``xception``: Xception.
+ """
+
+ def __init__(
+ self, num_classes, loss, fc_dims=None, dropout_p=None, **kwargs
+ ):
+ super(Xception, self).__init__()
+ self.loss = loss
+
+ self.conv1 = nn.Conv2d(3, 32, 3, 2, 0, bias=False)
+ self.bn1 = nn.BatchNorm2d(32)
+
+ self.conv2 = nn.Conv2d(32, 64, 3, bias=False)
+ self.bn2 = nn.BatchNorm2d(64)
+
+ self.block1 = Block(
+ 64, 128, 2, 2, start_with_relu=False, grow_first=True
+ )
+ self.block2 = Block(
+ 128, 256, 2, 2, start_with_relu=True, grow_first=True
+ )
+ self.block3 = Block(
+ 256, 728, 2, 2, start_with_relu=True, grow_first=True
+ )
+
+ self.block4 = Block(
+ 728, 728, 3, 1, start_with_relu=True, grow_first=True
+ )
+ self.block5 = Block(
+ 728, 728, 3, 1, start_with_relu=True, grow_first=True
+ )
+ self.block6 = Block(
+ 728, 728, 3, 1, start_with_relu=True, grow_first=True
+ )
+ self.block7 = Block(
+ 728, 728, 3, 1, start_with_relu=True, grow_first=True
+ )
+
+ self.block8 = Block(
+ 728, 728, 3, 1, start_with_relu=True, grow_first=True
+ )
+ self.block9 = Block(
+ 728, 728, 3, 1, start_with_relu=True, grow_first=True
+ )
+ self.block10 = Block(
+ 728, 728, 3, 1, start_with_relu=True, grow_first=True
+ )
+ self.block11 = Block(
+ 728, 728, 3, 1, start_with_relu=True, grow_first=True
+ )
+
+ self.block12 = Block(
+ 728, 1024, 2, 2, start_with_relu=True, grow_first=False
+ )
+
+ self.conv3 = SeparableConv2d(1024, 1536, 3, 1, 1)
+ self.bn3 = nn.BatchNorm2d(1536)
+
+ self.conv4 = SeparableConv2d(1536, 2048, 3, 1, 1)
+ self.bn4 = nn.BatchNorm2d(2048)
+
+ self.global_avgpool = nn.AdaptiveAvgPool2d(1)
+ self.feature_dim = 2048
+ self.fc = self._construct_fc_layer(fc_dims, 2048, dropout_p)
+ self.classifier = nn.Linear(self.feature_dim, num_classes)
+
+ self._init_params()
+
+ def _construct_fc_layer(self, fc_dims, input_dim, dropout_p=None):
+ """Constructs fully connected layer.
+
+ Args:
+ fc_dims (list or tuple): dimensions of fc layers, if None, no fc layers are constructed
+ input_dim (int): input dimension
+ dropout_p (float): dropout probability, if None, dropout is unused
+ """
+ if fc_dims is None:
+ self.feature_dim = input_dim
+ return None
+
+ assert isinstance(
+ fc_dims, (list, tuple)
+ ), 'fc_dims must be either list or tuple, but got {}'.format(
+ type(fc_dims)
+ )
+
+ layers = []
+ for dim in fc_dims:
+ layers.append(nn.Linear(input_dim, dim))
+ layers.append(nn.BatchNorm1d(dim))
+ layers.append(nn.ReLU(inplace=True))
+ if dropout_p is not None:
+ layers.append(nn.Dropout(p=dropout_p))
+ input_dim = dim
+
+ self.feature_dim = fc_dims[-1]
+
+ return nn.Sequential(*layers)
+
+ def _init_params(self):
+ for m in self.modules():
+ if isinstance(m, nn.Conv2d):
+ nn.init.kaiming_normal_(
+ m.weight, mode='fan_out', nonlinearity='relu'
+ )
+ if m.bias is not None:
+ nn.init.constant_(m.bias, 0)
+ elif isinstance(m, nn.BatchNorm2d):
+ nn.init.constant_(m.weight, 1)
+ nn.init.constant_(m.bias, 0)
+ elif isinstance(m, nn.BatchNorm1d):
+ nn.init.constant_(m.weight, 1)
+ nn.init.constant_(m.bias, 0)
+ elif isinstance(m, nn.Linear):
+ nn.init.normal_(m.weight, 0, 0.01)
+ if m.bias is not None:
+ nn.init.constant_(m.bias, 0)
+
+ def featuremaps(self, input):
+ x = self.conv1(input)
+ x = self.bn1(x)
+ x = F.relu(x, inplace=True)
+
+ x = self.conv2(x)
+ x = self.bn2(x)
+ x = F.relu(x, inplace=True)
+
+ x = self.block1(x)
+ x = self.block2(x)
+ x = self.block3(x)
+ x = self.block4(x)
+ x = self.block5(x)
+ x = self.block6(x)
+ x = self.block7(x)
+ x = self.block8(x)
+ x = self.block9(x)
+ x = self.block10(x)
+ x = self.block11(x)
+ x = self.block12(x)
+
+ x = self.conv3(x)
+ x = self.bn3(x)
+ x = F.relu(x, inplace=True)
+
+ x = self.conv4(x)
+ x = self.bn4(x)
+ x = F.relu(x, inplace=True)
+ return x
+
+ def forward(self, x):
+ f = self.featuremaps(x)
+ v = self.global_avgpool(f)
+ v = v.view(v.size(0), -1)
+
+ if self.fc is not None:
+ v = self.fc(v)
+
+ if not self.training:
+ return v
+
+ y = self.classifier(v)
+
+ if self.loss == 'softmax':
+ return y
+ elif self.loss == 'triplet':
+ return y, v
+ else:
+ raise KeyError('Unsupported loss: {}'.format(self.loss))
+
+
+def init_pretrained_weights(model, model_url):
+ """Initialize models with pretrained weights.
+
+ Layers that don't match with pretrained layers in name or size are kept unchanged.
+ """
+ pretrain_dict = model_zoo.load_url(model_url)
+ model_dict = model.state_dict()
+ pretrain_dict = {
+ k: v
+ for k, v in pretrain_dict.items()
+ if k in model_dict and model_dict[k].size() == v.size()
+ }
+ model_dict.update(pretrain_dict)
+ model.load_state_dict(model_dict)
+
+
+def xception(num_classes, loss='softmax', pretrained=True, **kwargs):
+ model = Xception(num_classes, loss, fc_dims=None, dropout_p=None, **kwargs)
+ if pretrained:
+ model_url = pretrained_settings['xception']['imagenet']['url']
+ init_pretrained_weights(model, model_url)
+ return model
diff --git a/trackers/strong_sort/deep/reid/torchreid/optim/__init__.py b/trackers/strong_sort/deep/reid/torchreid/optim/__init__.py
new file mode 100644
index 0000000000000000000000000000000000000000..1813e465741cf723c570f9dccf221bd186172dd4
--- /dev/null
+++ b/trackers/strong_sort/deep/reid/torchreid/optim/__init__.py
@@ -0,0 +1,4 @@
+from __future__ import absolute_import
+
+from .optimizer import build_optimizer
+from .lr_scheduler import build_lr_scheduler
diff --git a/trackers/strong_sort/deep/reid/torchreid/optim/lr_scheduler.py b/trackers/strong_sort/deep/reid/torchreid/optim/lr_scheduler.py
new file mode 100644
index 0000000000000000000000000000000000000000..d60bd1dc237372b068f80d6867d4ca3b1709ad07
--- /dev/null
+++ b/trackers/strong_sort/deep/reid/torchreid/optim/lr_scheduler.py
@@ -0,0 +1,68 @@
+from __future__ import print_function, absolute_import
+import torch
+
+AVAI_SCH = ['single_step', 'multi_step', 'cosine']
+
+
+def build_lr_scheduler(
+ optimizer, lr_scheduler='single_step', stepsize=1, gamma=0.1, max_epoch=1
+):
+ """A function wrapper for building a learning rate scheduler.
+
+ Args:
+ optimizer (Optimizer): an Optimizer.
+ lr_scheduler (str, optional): learning rate scheduler method. Default is single_step.
+ stepsize (int or list, optional): step size to decay learning rate. When ``lr_scheduler``
+ is "single_step", ``stepsize`` should be an integer. When ``lr_scheduler`` is
+ "multi_step", ``stepsize`` is a list. Default is 1.
+ gamma (float, optional): decay rate. Default is 0.1.
+ max_epoch (int, optional): maximum epoch (for cosine annealing). Default is 1.
+
+ Examples::
+ >>> # Decay learning rate by every 20 epochs.
+ >>> scheduler = torchreid.optim.build_lr_scheduler(
+ >>> optimizer, lr_scheduler='single_step', stepsize=20
+ >>> )
+ >>> # Decay learning rate at 30, 50 and 55 epochs.
+ >>> scheduler = torchreid.optim.build_lr_scheduler(
+ >>> optimizer, lr_scheduler='multi_step', stepsize=[30, 50, 55]
+ >>> )
+ """
+ if lr_scheduler not in AVAI_SCH:
+ raise ValueError(
+ 'Unsupported scheduler: {}. Must be one of {}'.format(
+ lr_scheduler, AVAI_SCH
+ )
+ )
+
+ if lr_scheduler == 'single_step':
+ if isinstance(stepsize, list):
+ stepsize = stepsize[-1]
+
+ if not isinstance(stepsize, int):
+ raise TypeError(
+ 'For single_step lr_scheduler, stepsize must '
+ 'be an integer, but got {}'.format(type(stepsize))
+ )
+
+ scheduler = torch.optim.lr_scheduler.StepLR(
+ optimizer, step_size=stepsize, gamma=gamma
+ )
+
+ elif lr_scheduler == 'multi_step':
+ if not isinstance(stepsize, list):
+ raise TypeError(
+ 'For multi_step lr_scheduler, stepsize must '
+ 'be a list, but got {}'.format(type(stepsize))
+ )
+
+ scheduler = torch.optim.lr_scheduler.MultiStepLR(
+ optimizer, milestones=stepsize, gamma=gamma
+ )
+
+ elif lr_scheduler == 'cosine':
+ scheduler = torch.optim.lr_scheduler.CosineAnnealingLR(
+ optimizer, float(max_epoch)
+ )
+
+ return scheduler
diff --git a/trackers/strong_sort/deep/reid/torchreid/optim/optimizer.py b/trackers/strong_sort/deep/reid/torchreid/optim/optimizer.py
new file mode 100644
index 0000000000000000000000000000000000000000..f57b03a0537b9596c3e072eeb8800cd6e54051ca
--- /dev/null
+++ b/trackers/strong_sort/deep/reid/torchreid/optim/optimizer.py
@@ -0,0 +1,157 @@
+from __future__ import print_function, absolute_import
+import warnings
+import torch
+import torch.nn as nn
+
+from .radam import RAdam
+
+AVAI_OPTIMS = ['adam', 'amsgrad', 'sgd', 'rmsprop', 'radam']
+
+
+def build_optimizer(
+ model,
+ optim='adam',
+ lr=0.0003,
+ weight_decay=5e-04,
+ momentum=0.9,
+ sgd_dampening=0,
+ sgd_nesterov=False,
+ rmsprop_alpha=0.99,
+ adam_beta1=0.9,
+ adam_beta2=0.99,
+ staged_lr=False,
+ new_layers='',
+ base_lr_mult=0.1
+):
+ """A function wrapper for building an optimizer.
+
+ Args:
+ model (nn.Module): model.
+ optim (str, optional): optimizer. Default is "adam".
+ lr (float, optional): learning rate. Default is 0.0003.
+ weight_decay (float, optional): weight decay (L2 penalty). Default is 5e-04.
+ momentum (float, optional): momentum factor in sgd. Default is 0.9,
+ sgd_dampening (float, optional): dampening for momentum. Default is 0.
+ sgd_nesterov (bool, optional): enables Nesterov momentum. Default is False.
+ rmsprop_alpha (float, optional): smoothing constant for rmsprop. Default is 0.99.
+ adam_beta1 (float, optional): beta-1 value in adam. Default is 0.9.
+ adam_beta2 (float, optional): beta-2 value in adam. Default is 0.99,
+ staged_lr (bool, optional): uses different learning rates for base and new layers. Base
+ layers are pretrained layers while new layers are randomly initialized, e.g. the
+ identity classification layer. Enabling ``staged_lr`` can allow the base layers to
+ be trained with a smaller learning rate determined by ``base_lr_mult``, while the new
+ layers will take the ``lr``. Default is False.
+ new_layers (str or list): attribute names in ``model``. Default is empty.
+ base_lr_mult (float, optional): learning rate multiplier for base layers. Default is 0.1.
+
+ Examples::
+ >>> # A normal optimizer can be built by
+ >>> optimizer = torchreid.optim.build_optimizer(model, optim='sgd', lr=0.01)
+ >>> # If you want to use a smaller learning rate for pretrained layers
+ >>> # and the attribute name for the randomly initialized layer is 'classifier',
+ >>> # you can do
+ >>> optimizer = torchreid.optim.build_optimizer(
+ >>> model, optim='sgd', lr=0.01, staged_lr=True,
+ >>> new_layers='classifier', base_lr_mult=0.1
+ >>> )
+ >>> # Now the `classifier` has learning rate 0.01 but the base layers
+ >>> # have learning rate 0.01 * 0.1.
+ >>> # new_layers can also take multiple attribute names. Say the new layers
+ >>> # are 'fc' and 'classifier', you can do
+ >>> optimizer = torchreid.optim.build_optimizer(
+ >>> model, optim='sgd', lr=0.01, staged_lr=True,
+ >>> new_layers=['fc', 'classifier'], base_lr_mult=0.1
+ >>> )
+ """
+ if optim not in AVAI_OPTIMS:
+ raise ValueError(
+ 'Unsupported optim: {}. Must be one of {}'.format(
+ optim, AVAI_OPTIMS
+ )
+ )
+
+ if not isinstance(model, nn.Module):
+ raise TypeError(
+ 'model given to build_optimizer must be an instance of nn.Module'
+ )
+
+ if staged_lr:
+ if isinstance(new_layers, str):
+ if new_layers is None:
+ warnings.warn(
+ 'new_layers is empty, therefore, staged_lr is useless'
+ )
+ new_layers = [new_layers]
+
+ if isinstance(model, nn.DataParallel):
+ model = model.module
+
+ base_params = []
+ base_layers = []
+ new_params = []
+
+ for name, module in model.named_children():
+ if name in new_layers:
+ new_params += [p for p in module.parameters()]
+ else:
+ base_params += [p for p in module.parameters()]
+ base_layers.append(name)
+
+ param_groups = [
+ {
+ 'params': base_params,
+ 'lr': lr * base_lr_mult
+ },
+ {
+ 'params': new_params
+ },
+ ]
+
+ else:
+ param_groups = model.parameters()
+
+ if optim == 'adam':
+ optimizer = torch.optim.Adam(
+ param_groups,
+ lr=lr,
+ weight_decay=weight_decay,
+ betas=(adam_beta1, adam_beta2),
+ )
+
+ elif optim == 'amsgrad':
+ optimizer = torch.optim.Adam(
+ param_groups,
+ lr=lr,
+ weight_decay=weight_decay,
+ betas=(adam_beta1, adam_beta2),
+ amsgrad=True,
+ )
+
+ elif optim == 'sgd':
+ optimizer = torch.optim.SGD(
+ param_groups,
+ lr=lr,
+ momentum=momentum,
+ weight_decay=weight_decay,
+ dampening=sgd_dampening,
+ nesterov=sgd_nesterov,
+ )
+
+ elif optim == 'rmsprop':
+ optimizer = torch.optim.RMSprop(
+ param_groups,
+ lr=lr,
+ momentum=momentum,
+ weight_decay=weight_decay,
+ alpha=rmsprop_alpha,
+ )
+
+ elif optim == 'radam':
+ optimizer = RAdam(
+ param_groups,
+ lr=lr,
+ weight_decay=weight_decay,
+ betas=(adam_beta1, adam_beta2)
+ )
+
+ return optimizer
diff --git a/trackers/strong_sort/deep/reid/torchreid/optim/radam.py b/trackers/strong_sort/deep/reid/torchreid/optim/radam.py
new file mode 100644
index 0000000000000000000000000000000000000000..f066c573f8b650a6162f0b54a1c2c100b2679f3b
--- /dev/null
+++ b/trackers/strong_sort/deep/reid/torchreid/optim/radam.py
@@ -0,0 +1,330 @@
+"""
+Imported from: https://github.com/LiyuanLucasLiu/RAdam
+
+Paper: https://arxiv.org/abs/1908.03265
+
+@article{liu2019radam,
+ title={On the Variance of the Adaptive Learning Rate and Beyond},
+ author={Liu, Liyuan and Jiang, Haoming and He, Pengcheng and Chen, Weizhu and Liu, Xiaodong and Gao, Jianfeng and Han, Jiawei},
+ journal={arXiv preprint arXiv:1908.03265},
+ year={2019}
+}
+"""
+from __future__ import print_function, absolute_import
+import math
+import torch
+from torch.optim.optimizer import Optimizer
+
+
+class RAdam(Optimizer):
+
+ def __init__(
+ self,
+ params,
+ lr=1e-3,
+ betas=(0.9, 0.999),
+ eps=1e-8,
+ weight_decay=0,
+ degenerated_to_sgd=True
+ ):
+ if not 0.0 <= lr:
+ raise ValueError("Invalid learning rate: {}".format(lr))
+ if not 0.0 <= eps:
+ raise ValueError("Invalid epsilon value: {}".format(eps))
+ if not 0.0 <= betas[0] < 1.0:
+ raise ValueError(
+ "Invalid beta parameter at index 0: {}".format(betas[0])
+ )
+ if not 0.0 <= betas[1] < 1.0:
+ raise ValueError(
+ "Invalid beta parameter at index 1: {}".format(betas[1])
+ )
+
+ self.degenerated_to_sgd = degenerated_to_sgd
+ defaults = dict(lr=lr, betas=betas, eps=eps, weight_decay=weight_decay)
+ self.buffer = [[None, None, None] for ind in range(10)]
+ super(RAdam, self).__init__(params, defaults)
+
+ def __setstate__(self, state):
+ super(RAdam, self).__setstate__(state)
+
+ def step(self, closure=None):
+
+ loss = None
+ if closure is not None:
+ loss = closure()
+
+ for group in self.param_groups:
+
+ for p in group['params']:
+ if p.grad is None:
+ continue
+ grad = p.grad.data.float()
+ if grad.is_sparse:
+ raise RuntimeError(
+ 'RAdam does not support sparse gradients'
+ )
+
+ p_data_fp32 = p.data.float()
+
+ state = self.state[p]
+
+ if len(state) == 0:
+ state['step'] = 0
+ state['exp_avg'] = torch.zeros_like(p_data_fp32)
+ state['exp_avg_sq'] = torch.zeros_like(p_data_fp32)
+ else:
+ state['exp_avg'] = state['exp_avg'].type_as(p_data_fp32)
+ state['exp_avg_sq'] = state['exp_avg_sq'].type_as(
+ p_data_fp32
+ )
+
+ exp_avg, exp_avg_sq = state['exp_avg'], state['exp_avg_sq']
+ beta1, beta2 = group['betas']
+
+ exp_avg_sq.mul_(beta2).addcmul_(1 - beta2, grad, grad)
+ exp_avg.mul_(beta1).add_(1 - beta1, grad)
+
+ state['step'] += 1
+ buffered = self.buffer[int(state['step'] % 10)]
+ if state['step'] == buffered[0]:
+ N_sma, step_size = buffered[1], buffered[2]
+ else:
+ buffered[0] = state['step']
+ beta2_t = beta2**state['step']
+ N_sma_max = 2 / (1-beta2) - 1
+ N_sma = N_sma_max - 2 * state['step'
+ ] * beta2_t / (1-beta2_t)
+ buffered[1] = N_sma
+
+ # more conservative since it's an approximated value
+ if N_sma >= 5:
+ step_size = math.sqrt(
+ (1-beta2_t) * (N_sma-4) / (N_sma_max-4) *
+ (N_sma-2) / N_sma * N_sma_max / (N_sma_max-2)
+ ) / (1 - beta1**state['step'])
+ elif self.degenerated_to_sgd:
+ step_size = 1.0 / (1 - beta1**state['step'])
+ else:
+ step_size = -1
+ buffered[2] = step_size
+
+ # more conservative since it's an approximated value
+ if N_sma >= 5:
+ if group['weight_decay'] != 0:
+ p_data_fp32.add_(
+ -group['weight_decay'] * group['lr'], p_data_fp32
+ )
+ denom = exp_avg_sq.sqrt().add_(group['eps'])
+ p_data_fp32.addcdiv_(
+ -step_size * group['lr'], exp_avg, denom
+ )
+ p.data.copy_(p_data_fp32)
+ elif step_size > 0:
+ if group['weight_decay'] != 0:
+ p_data_fp32.add_(
+ -group['weight_decay'] * group['lr'], p_data_fp32
+ )
+ p_data_fp32.add_(-step_size * group['lr'], exp_avg)
+ p.data.copy_(p_data_fp32)
+
+ return loss
+
+
+class PlainRAdam(Optimizer):
+
+ def __init__(
+ self,
+ params,
+ lr=1e-3,
+ betas=(0.9, 0.999),
+ eps=1e-8,
+ weight_decay=0,
+ degenerated_to_sgd=True
+ ):
+ if not 0.0 <= lr:
+ raise ValueError("Invalid learning rate: {}".format(lr))
+ if not 0.0 <= eps:
+ raise ValueError("Invalid epsilon value: {}".format(eps))
+ if not 0.0 <= betas[0] < 1.0:
+ raise ValueError(
+ "Invalid beta parameter at index 0: {}".format(betas[0])
+ )
+ if not 0.0 <= betas[1] < 1.0:
+ raise ValueError(
+ "Invalid beta parameter at index 1: {}".format(betas[1])
+ )
+
+ self.degenerated_to_sgd = degenerated_to_sgd
+ defaults = dict(lr=lr, betas=betas, eps=eps, weight_decay=weight_decay)
+
+ super(PlainRAdam, self).__init__(params, defaults)
+
+ def __setstate__(self, state):
+ super(PlainRAdam, self).__setstate__(state)
+
+ def step(self, closure=None):
+
+ loss = None
+ if closure is not None:
+ loss = closure()
+
+ for group in self.param_groups:
+
+ for p in group['params']:
+ if p.grad is None:
+ continue
+ grad = p.grad.data.float()
+ if grad.is_sparse:
+ raise RuntimeError(
+ 'RAdam does not support sparse gradients'
+ )
+
+ p_data_fp32 = p.data.float()
+
+ state = self.state[p]
+
+ if len(state) == 0:
+ state['step'] = 0
+ state['exp_avg'] = torch.zeros_like(p_data_fp32)
+ state['exp_avg_sq'] = torch.zeros_like(p_data_fp32)
+ else:
+ state['exp_avg'] = state['exp_avg'].type_as(p_data_fp32)
+ state['exp_avg_sq'] = state['exp_avg_sq'].type_as(
+ p_data_fp32
+ )
+
+ exp_avg, exp_avg_sq = state['exp_avg'], state['exp_avg_sq']
+ beta1, beta2 = group['betas']
+
+ exp_avg_sq.mul_(beta2).addcmul_(1 - beta2, grad, grad)
+ exp_avg.mul_(beta1).add_(1 - beta1, grad)
+
+ state['step'] += 1
+ beta2_t = beta2**state['step']
+ N_sma_max = 2 / (1-beta2) - 1
+ N_sma = N_sma_max - 2 * state['step'] * beta2_t / (1-beta2_t)
+
+ # more conservative since it's an approximated value
+ if N_sma >= 5:
+ if group['weight_decay'] != 0:
+ p_data_fp32.add_(
+ -group['weight_decay'] * group['lr'], p_data_fp32
+ )
+ step_size = group['lr'] * math.sqrt(
+ (1-beta2_t) * (N_sma-4) / (N_sma_max-4) *
+ (N_sma-2) / N_sma * N_sma_max / (N_sma_max-2)
+ ) / (1 - beta1**state['step'])
+ denom = exp_avg_sq.sqrt().add_(group['eps'])
+ p_data_fp32.addcdiv_(-step_size, exp_avg, denom)
+ p.data.copy_(p_data_fp32)
+ elif self.degenerated_to_sgd:
+ if group['weight_decay'] != 0:
+ p_data_fp32.add_(
+ -group['weight_decay'] * group['lr'], p_data_fp32
+ )
+ step_size = group['lr'] / (1 - beta1**state['step'])
+ p_data_fp32.add_(-step_size, exp_avg)
+ p.data.copy_(p_data_fp32)
+
+ return loss
+
+
+class AdamW(Optimizer):
+
+ def __init__(
+ self,
+ params,
+ lr=1e-3,
+ betas=(0.9, 0.999),
+ eps=1e-8,
+ weight_decay=0,
+ warmup=0
+ ):
+ if not 0.0 <= lr:
+ raise ValueError("Invalid learning rate: {}".format(lr))
+ if not 0.0 <= eps:
+ raise ValueError("Invalid epsilon value: {}".format(eps))
+ if not 0.0 <= betas[0] < 1.0:
+ raise ValueError(
+ "Invalid beta parameter at index 0: {}".format(betas[0])
+ )
+ if not 0.0 <= betas[1] < 1.0:
+ raise ValueError(
+ "Invalid beta parameter at index 1: {}".format(betas[1])
+ )
+
+ defaults = dict(
+ lr=lr,
+ betas=betas,
+ eps=eps,
+ weight_decay=weight_decay,
+ warmup=warmup
+ )
+ super(AdamW, self).__init__(params, defaults)
+
+ def __setstate__(self, state):
+ super(AdamW, self).__setstate__(state)
+
+ def step(self, closure=None):
+ loss = None
+ if closure is not None:
+ loss = closure()
+
+ for group in self.param_groups:
+
+ for p in group['params']:
+ if p.grad is None:
+ continue
+ grad = p.grad.data.float()
+ if grad.is_sparse:
+ raise RuntimeError(
+ 'Adam does not support sparse gradients, please consider SparseAdam instead'
+ )
+
+ p_data_fp32 = p.data.float()
+
+ state = self.state[p]
+
+ if len(state) == 0:
+ state['step'] = 0
+ state['exp_avg'] = torch.zeros_like(p_data_fp32)
+ state['exp_avg_sq'] = torch.zeros_like(p_data_fp32)
+ else:
+ state['exp_avg'] = state['exp_avg'].type_as(p_data_fp32)
+ state['exp_avg_sq'] = state['exp_avg_sq'].type_as(
+ p_data_fp32
+ )
+
+ exp_avg, exp_avg_sq = state['exp_avg'], state['exp_avg_sq']
+ beta1, beta2 = group['betas']
+
+ state['step'] += 1
+
+ exp_avg_sq.mul_(beta2).addcmul_(1 - beta2, grad, grad)
+ exp_avg.mul_(beta1).add_(1 - beta1, grad)
+
+ denom = exp_avg_sq.sqrt().add_(group['eps'])
+ bias_correction1 = 1 - beta1**state['step']
+ bias_correction2 = 1 - beta2**state['step']
+
+ if group['warmup'] > state['step']:
+ scheduled_lr = 1e-8 + state['step'] * group['lr'] / group[
+ 'warmup']
+ else:
+ scheduled_lr = group['lr']
+
+ step_size = scheduled_lr * math.sqrt(
+ bias_correction2
+ ) / bias_correction1
+
+ if group['weight_decay'] != 0:
+ p_data_fp32.add_(
+ -group['weight_decay'] * scheduled_lr, p_data_fp32
+ )
+
+ p_data_fp32.addcdiv_(-step_size, exp_avg, denom)
+
+ p.data.copy_(p_data_fp32)
+
+ return loss
diff --git a/trackers/strong_sort/deep/reid/torchreid/utils/GPU-Re-Ranking/README.md b/trackers/strong_sort/deep/reid/torchreid/utils/GPU-Re-Ranking/README.md
new file mode 100644
index 0000000000000000000000000000000000000000..349a9ef637309852f0628151f010058bf4f1a4cc
--- /dev/null
+++ b/trackers/strong_sort/deep/reid/torchreid/utils/GPU-Re-Ranking/README.md
@@ -0,0 +1,37 @@
+# Understanding Image Retrieval Re-Ranking: A Graph Neural Network Perspective
+
+[[Paper]](https://arxiv.org/abs/2012.07620v2)
+
+On the Market-1501 dataset, we accelerate the re-ranking processing from **89.2s** to **9.4ms** with one K40m GPU, facilitating the real-time post-processing.
+Similarly, we observe that our method achieves comparable or even better retrieval results on the other four image retrieval benchmarks,
+i.e., VeRi-776, Oxford-5k, Paris-6k and University-1652, with limited time cost.
+
+## Prerequisites
+
+The code was mainly developed and tested with python 3.7, PyTorch 1.4.1, CUDA 10.2, and CentOS release 6.10.
+
+The code has been included in `/extension`. To compile it:
+
+```shell
+cd extension
+sh make.sh
+```
+
+## Demo
+
+The demo script `main.py` provides the gnn re-ranking method using the prepared feature.
+
+```shell
+python main.py --data_path PATH_TO_DATA --k1 26 --k2 7
+```
+
+## Citation
+```bibtex
+@article{zhang2020understanding,
+ title={Understanding Image Retrieval Re-Ranking: A Graph Neural Network Perspective},
+ author={Xuanmeng Zhang, Minyue Jiang, Zhedong Zheng, Xiao Tan, Errui Ding, Yi Yang},
+ journal={arXiv preprint arXiv:2012.07620},
+ year={2020}
+}
+```
+
diff --git a/trackers/strong_sort/deep/reid/torchreid/utils/GPU-Re-Ranking/extension/adjacency_matrix/build_adjacency_matrix.cpp b/trackers/strong_sort/deep/reid/torchreid/utils/GPU-Re-Ranking/extension/adjacency_matrix/build_adjacency_matrix.cpp
new file mode 100644
index 0000000000000000000000000000000000000000..4c496041e37e525af728794f11627a7e0027a267
--- /dev/null
+++ b/trackers/strong_sort/deep/reid/torchreid/utils/GPU-Re-Ranking/extension/adjacency_matrix/build_adjacency_matrix.cpp
@@ -0,0 +1,19 @@
+#include
+#include
+#include
+
+at::Tensor build_adjacency_matrix_forward(torch::Tensor initial_rank);
+
+
+#define CHECK_CUDA(x) AT_ASSERTM(x.type().is_cuda(), #x " must be a CUDA tensor")
+#define CHECK_CONTIGUOUS(x) AT_ASSERTM(x.is_contiguous(), #x " must be contiguous")
+#define CHECK_INPUT(x) CHECK_CUDA(x); CHECK_CONTIGUOUS(x)
+
+at::Tensor build_adjacency_matrix(at::Tensor initial_rank) {
+ CHECK_INPUT(initial_rank);
+ return build_adjacency_matrix_forward(initial_rank);
+}
+
+PYBIND11_MODULE(TORCH_EXTENSION_NAME, m) {
+ m.def("forward", &build_adjacency_matrix, "build_adjacency_matrix (CUDA)");
+}
diff --git a/trackers/strong_sort/deep/reid/torchreid/utils/GPU-Re-Ranking/extension/adjacency_matrix/build_adjacency_matrix_kernel.cu b/trackers/strong_sort/deep/reid/torchreid/utils/GPU-Re-Ranking/extension/adjacency_matrix/build_adjacency_matrix_kernel.cu
new file mode 100644
index 0000000000000000000000000000000000000000..4973ddefeaa4253291bb9d34fbbbb50a46c32b8a
--- /dev/null
+++ b/trackers/strong_sort/deep/reid/torchreid/utils/GPU-Re-Ranking/extension/adjacency_matrix/build_adjacency_matrix_kernel.cu
@@ -0,0 +1,31 @@
+#include
+
+#include
+#include
+#include
+
+#define CUDA_1D_KERNEL_LOOP(i, n) for (int i = blockIdx.x * blockDim.x + threadIdx.x; i < n; i += blockDim.x * gridDim.x)
+
+
+__global__ void build_adjacency_matrix_kernel(float* initial_rank, float* A, const int total_num, const int topk, const int nthreads, const int all_num) {
+ int index = blockIdx.x * blockDim.x + threadIdx.x;
+ int stride = blockDim.x * gridDim.x;
+ for (int i = index; i < all_num; i += stride) {
+ int ii = i / topk;
+ A[ii * total_num + int(initial_rank[i])] = float(1.0);
+ }
+}
+
+at::Tensor build_adjacency_matrix_forward(at::Tensor initial_rank) {
+ const auto total_num = initial_rank.size(0);
+ const auto topk = initial_rank.size(1);
+ const auto all_num = total_num * topk;
+ auto A = torch::zeros({total_num, total_num}, at::device(initial_rank.device()).dtype(at::ScalarType::Float));
+
+ const int threads = 1024;
+ const int blocks = (all_num + threads - 1) / threads;
+
+ build_adjacency_matrix_kernel<<>>(initial_rank.data_ptr(), A.data_ptr(), total_num, topk, threads, all_num);
+ return A;
+
+}
diff --git a/trackers/strong_sort/deep/reid/torchreid/utils/GPU-Re-Ranking/extension/adjacency_matrix/setup.py b/trackers/strong_sort/deep/reid/torchreid/utils/GPU-Re-Ranking/extension/adjacency_matrix/setup.py
new file mode 100644
index 0000000000000000000000000000000000000000..abd3e3a4e1fbd1db7b4bdd1d080d48381082c60a
--- /dev/null
+++ b/trackers/strong_sort/deep/reid/torchreid/utils/GPU-Re-Ranking/extension/adjacency_matrix/setup.py
@@ -0,0 +1,36 @@
+"""
+ Understanding Image Retrieval Re-Ranking: A Graph Neural Network Perspective
+
+ Xuanmeng Zhang, Minyue Jiang, Zhedong Zheng, Xiao Tan, Errui Ding, Yi Yang
+
+ Project Page : https://github.com/Xuanmeng-Zhang/gnn-re-ranking
+
+ Paper: https://arxiv.org/abs/2012.07620v2
+
+ ======================================================================
+
+ On the Market-1501 dataset, we accelerate the re-ranking processing from 89.2s to 9.4ms
+ with one K40m GPU, facilitating the real-time post-processing. Similarly, we observe
+ that our method achieves comparable or even better retrieval results on the other four
+ image retrieval benchmarks, i.e., VeRi-776, Oxford-5k, Paris-6k and University-1652,
+ with limited time cost.
+"""
+
+from setuptools import Extension, setup
+import torch
+import torch.nn as nn
+from torch.autograd import Function
+from torch.utils.cpp_extension import CUDAExtension, BuildExtension
+
+setup(
+ name='build_adjacency_matrix',
+ ext_modules=[
+ CUDAExtension(
+ 'build_adjacency_matrix', [
+ 'build_adjacency_matrix.cpp',
+ 'build_adjacency_matrix_kernel.cu',
+ ]
+ ),
+ ],
+ cmdclass={'build_ext': BuildExtension}
+)
diff --git a/trackers/strong_sort/deep/reid/torchreid/utils/GPU-Re-Ranking/extension/make.sh b/trackers/strong_sort/deep/reid/torchreid/utils/GPU-Re-Ranking/extension/make.sh
new file mode 100644
index 0000000000000000000000000000000000000000..f0197ff9c964cfa7b6496a29b8411da01d323568
--- /dev/null
+++ b/trackers/strong_sort/deep/reid/torchreid/utils/GPU-Re-Ranking/extension/make.sh
@@ -0,0 +1,4 @@
+cd adjacency_matrix
+python setup.py install
+cd ../propagation
+python setup.py install
\ No newline at end of file
diff --git a/trackers/strong_sort/deep/reid/torchreid/utils/GPU-Re-Ranking/extension/propagation/gnn_propagate.cpp b/trackers/strong_sort/deep/reid/torchreid/utils/GPU-Re-Ranking/extension/propagation/gnn_propagate.cpp
new file mode 100644
index 0000000000000000000000000000000000000000..10a939ffe7fb0ed73dd450d6bfc667132448dfca
--- /dev/null
+++ b/trackers/strong_sort/deep/reid/torchreid/utils/GPU-Re-Ranking/extension/propagation/gnn_propagate.cpp
@@ -0,0 +1,21 @@
+#include
+#include
+#include
+
+at::Tensor gnn_propagate_forward(at::Tensor A, at::Tensor initial_rank, at::Tensor S);
+
+
+#define CHECK_CUDA(x) AT_ASSERTM(x.type().is_cuda(), #x " must be a CUDA tensor")
+#define CHECK_CONTIGUOUS(x) AT_ASSERTM(x.is_contiguous(), #x " must be contiguous")
+#define CHECK_INPUT(x) CHECK_CUDA(x); CHECK_CONTIGUOUS(x)
+
+at::Tensor gnn_propagate(at::Tensor A ,at::Tensor initial_rank, at::Tensor S) {
+ CHECK_INPUT(A);
+ CHECK_INPUT(initial_rank);
+ CHECK_INPUT(S);
+ return gnn_propagate_forward(A, initial_rank, S);
+}
+
+PYBIND11_MODULE(TORCH_EXTENSION_NAME, m) {
+ m.def("forward", &gnn_propagate, "gnn propagate (CUDA)");
+}
\ No newline at end of file
diff --git a/trackers/strong_sort/deep/reid/torchreid/utils/GPU-Re-Ranking/extension/propagation/gnn_propagate_kernel.cu b/trackers/strong_sort/deep/reid/torchreid/utils/GPU-Re-Ranking/extension/propagation/gnn_propagate_kernel.cu
new file mode 100644
index 0000000000000000000000000000000000000000..8bdebf1666e16f3fbdd294d374e6438e667f96a1
--- /dev/null
+++ b/trackers/strong_sort/deep/reid/torchreid/utils/GPU-Re-Ranking/extension/propagation/gnn_propagate_kernel.cu
@@ -0,0 +1,36 @@
+#include
+
+#include
+#include
+#include
+#include
+
+__global__ void gnn_propagate_forward_kernel(float* initial_rank, float* A, float* A_qe, float* S, const int sample_num, const int topk, const int total_num) {
+ int index = blockIdx.x * blockDim.x + threadIdx.x;
+ int stride = blockDim.x * gridDim.x;
+ for (int i = index; i < total_num; i += stride) {
+ int fea = i % sample_num;
+ int sample_index = i / sample_num;
+ float sum = 0.0;
+ for (int j = 0; j < topk ; j++) {
+ int topk_fea_index = int(initial_rank[sample_index*topk+j]) * sample_num + fea;
+ sum += A[ topk_fea_index] * S[sample_index*topk+j];
+ }
+ A_qe[i] = sum;
+ }
+}
+
+at::Tensor gnn_propagate_forward(at::Tensor A, at::Tensor initial_rank, at::Tensor S) {
+ const auto sample_num = A.size(0);
+ const auto topk = initial_rank.size(1);
+
+ const auto total_num = sample_num * sample_num ;
+ auto A_qe = torch::zeros({sample_num, sample_num}, at::device(initial_rank.device()).dtype(at::ScalarType::Float));
+
+ const int threads = 1024;
+ const int blocks = (total_num + threads - 1) / threads;
+
+ gnn_propagate_forward_kernel<<>>(initial_rank.data_ptr(), A.data_ptr(), A_qe.data_ptr(), S.data_ptr(), sample_num, topk, total_num);
+ return A_qe;
+
+}
\ No newline at end of file
diff --git a/trackers/strong_sort/deep/reid/torchreid/utils/GPU-Re-Ranking/extension/propagation/setup.py b/trackers/strong_sort/deep/reid/torchreid/utils/GPU-Re-Ranking/extension/propagation/setup.py
new file mode 100644
index 0000000000000000000000000000000000000000..1f7b43b42150dd8bf7ea839c51b2d8be29263310
--- /dev/null
+++ b/trackers/strong_sort/deep/reid/torchreid/utils/GPU-Re-Ranking/extension/propagation/setup.py
@@ -0,0 +1,36 @@
+"""
+ Understanding Image Retrieval Re-Ranking: A Graph Neural Network Perspective
+
+ Xuanmeng Zhang, Minyue Jiang, Zhedong Zheng, Xiao Tan, Errui Ding, Yi Yang
+
+ Project Page : https://github.com/Xuanmeng-Zhang/gnn-re-ranking
+
+ Paper: https://arxiv.org/abs/2012.07620v2
+
+ ======================================================================
+
+ On the Market-1501 dataset, we accelerate the re-ranking processing from 89.2s to 9.4ms
+ with one K40m GPU, facilitating the real-time post-processing. Similarly, we observe
+ that our method achieves comparable or even better retrieval results on the other four
+ image retrieval benchmarks, i.e., VeRi-776, Oxford-5k, Paris-6k and University-1652,
+ with limited time cost.
+"""
+
+from setuptools import Extension, setup
+import torch
+import torch.nn as nn
+from torch.autograd import Function
+from torch.utils.cpp_extension import CUDAExtension, BuildExtension
+
+setup(
+ name='gnn_propagate',
+ ext_modules=[
+ CUDAExtension(
+ 'gnn_propagate', [
+ 'gnn_propagate.cpp',
+ 'gnn_propagate_kernel.cu',
+ ]
+ ),
+ ],
+ cmdclass={'build_ext': BuildExtension}
+)
diff --git a/trackers/strong_sort/deep/reid/torchreid/utils/GPU-Re-Ranking/gnn_reranking.py b/trackers/strong_sort/deep/reid/torchreid/utils/GPU-Re-Ranking/gnn_reranking.py
new file mode 100644
index 0000000000000000000000000000000000000000..2c8cc53b91d4e2a384881825839b44910556ee11
--- /dev/null
+++ b/trackers/strong_sort/deep/reid/torchreid/utils/GPU-Re-Ranking/gnn_reranking.py
@@ -0,0 +1,59 @@
+"""
+ Understanding Image Retrieval Re-Ranking: A Graph Neural Network Perspective
+
+ Xuanmeng Zhang, Minyue Jiang, Zhedong Zheng, Xiao Tan, Errui Ding, Yi Yang
+
+ Project Page : https://github.com/Xuanmeng-Zhang/gnn-re-ranking
+
+ Paper: https://arxiv.org/abs/2012.07620v2
+
+ ======================================================================
+
+ On the Market-1501 dataset, we accelerate the re-ranking processing from 89.2s to 9.4ms
+ with one K40m GPU, facilitating the real-time post-processing. Similarly, we observe
+ that our method achieves comparable or even better retrieval results on the other four
+ image retrieval benchmarks, i.e., VeRi-776, Oxford-5k, Paris-6k and University-1652,
+ with limited time cost.
+"""
+
+import numpy as np
+import torch
+
+import gnn_propagate
+import build_adjacency_matrix
+from utils import *
+
+
+def gnn_reranking(X_q, X_g, k1, k2):
+ query_num, gallery_num = X_q.shape[0], X_g.shape[0]
+
+ X_u = torch.cat((X_q, X_g), axis=0)
+ original_score = torch.mm(X_u, X_u.t())
+ del X_u, X_q, X_g
+
+ # initial ranking list
+ S, initial_rank = original_score.topk(
+ k=k1, dim=-1, largest=True, sorted=True
+ )
+
+ # stage 1
+ A = build_adjacency_matrix.forward(initial_rank.float())
+ S = S * S
+
+ # stage 2
+ if k2 != 1:
+ for i in range(2):
+ A = A + A.T
+ A = gnn_propagate.forward(
+ A, initial_rank[:, :k2].contiguous().float(),
+ S[:, :k2].contiguous().float()
+ )
+ A_norm = torch.norm(A, p=2, dim=1, keepdim=True)
+ A = A.div(A_norm.expand_as(A))
+
+ cosine_similarity = torch.mm(A[:query_num, ], A[query_num:, ].t())
+ del A, S
+
+ L = torch.sort(-cosine_similarity, dim=1)[1]
+ L = L.data.cpu().numpy()
+ return L
diff --git a/trackers/strong_sort/deep/reid/torchreid/utils/GPU-Re-Ranking/main.py b/trackers/strong_sort/deep/reid/torchreid/utils/GPU-Re-Ranking/main.py
new file mode 100644
index 0000000000000000000000000000000000000000..53ef6ac570194a136023c8b602759bc3792691db
--- /dev/null
+++ b/trackers/strong_sort/deep/reid/torchreid/utils/GPU-Re-Ranking/main.py
@@ -0,0 +1,72 @@
+"""
+ Understanding Image Retrieval Re-Ranking: A Graph Neural Network Perspective
+
+ Xuanmeng Zhang, Minyue Jiang, Zhedong Zheng, Xiao Tan, Errui Ding, Yi Yang
+
+ Project Page : https://github.com/Xuanmeng-Zhang/gnn-re-ranking
+
+ Paper: https://arxiv.org/abs/2012.07620v2
+
+ ======================================================================
+
+ On the Market-1501 dataset, we accelerate the re-ranking processing from 89.2s to 9.4ms
+ with one K40m GPU, facilitating the real-time post-processing. Similarly, we observe
+ that our method achieves comparable or even better retrieval results on the other four
+ image retrieval benchmarks, i.e., VeRi-776, Oxford-5k, Paris-6k and University-1652,
+ with limited time cost.
+"""
+
+import os
+import numpy as np
+import argparse
+import torch
+
+from utils import *
+from gnn_reranking import *
+
+parser = argparse.ArgumentParser(description='Reranking_is_GNN')
+parser.add_argument(
+ '--data_path',
+ type=str,
+ default='../xm_rerank_gpu_2/features/market_88_test.pkl',
+ help='path to dataset'
+)
+parser.add_argument(
+ '--k1',
+ type=int,
+ default=26, # Market-1501
+ # default=60, # Veri-776
+ help='parameter k1'
+)
+parser.add_argument(
+ '--k2',
+ type=int,
+ default=7, # Market-1501
+ # default=10, # Veri-776
+ help='parameter k2'
+)
+
+args = parser.parse_args()
+
+
+def main():
+ data = load_pickle(args.data_path)
+
+ query_cam = data['query_cam']
+ query_label = data['query_label']
+ gallery_cam = data['gallery_cam']
+ gallery_label = data['gallery_label']
+
+ gallery_feature = torch.FloatTensor(data['gallery_f'])
+ query_feature = torch.FloatTensor(data['query_f'])
+ query_feature = query_feature.cuda()
+ gallery_feature = gallery_feature.cuda()
+
+ indices = gnn_reranking(query_feature, gallery_feature, args.k1, args.k2)
+ evaluate_ranking_list(
+ indices, query_label, query_cam, gallery_label, gallery_cam
+ )
+
+
+if __name__ == '__main__':
+ main()
diff --git a/trackers/strong_sort/deep/reid/torchreid/utils/GPU-Re-Ranking/utils.py b/trackers/strong_sort/deep/reid/torchreid/utils/GPU-Re-Ranking/utils.py
new file mode 100644
index 0000000000000000000000000000000000000000..5f1ed9f88a3c53a1c8ceb14c0cf3959f332fe271
--- /dev/null
+++ b/trackers/strong_sort/deep/reid/torchreid/utils/GPU-Re-Ranking/utils.py
@@ -0,0 +1,137 @@
+"""
+ Understanding Image Retrieval Re-Ranking: A Graph Neural Network Perspective
+
+ Xuanmeng Zhang, Minyue Jiang, Zhedong Zheng, Xiao Tan, Errui Ding, Yi Yang
+
+ Project Page : https://github.com/Xuanmeng-Zhang/gnn-re-ranking
+
+ Paper: https://arxiv.org/abs/2012.07620v2
+
+ ======================================================================
+
+ On the Market-1501 dataset, we accelerate the re-ranking processing from 89.2s to 9.4ms
+ with one K40m GPU, facilitating the real-time post-processing. Similarly, we observe
+ that our method achieves comparable or even better retrieval results on the other four
+ image retrieval benchmarks, i.e., VeRi-776, Oxford-5k, Paris-6k and University-1652,
+ with limited time cost.
+"""
+
+import os
+import numpy as np
+import pickle
+import torch
+
+
+def load_pickle(pickle_path):
+ with open(pickle_path, 'rb') as f:
+ data = pickle.load(f)
+ return data
+
+
+def save_pickle(pickle_path, data):
+ with open(pickle_path, 'wb') as f:
+ pickle.dump(data, f, protocol=pickle.HIGHEST_PROTOCOL)
+
+
+def pairwise_squared_distance(x):
+ '''
+ x : (n_samples, n_points, dims)
+ return : (n_samples, n_points, n_points)
+ '''
+ x2s = (x * x).sum(-1, keepdim=True)
+ return x2s + x2s.transpose(-1, -2) - 2 * x @ x.transpose(-1, -2)
+
+
+def pairwise_distance(x, y):
+ m, n = x.size(0), y.size(0)
+
+ x = x.view(m, -1)
+ y = y.view(n, -1)
+
+ dist = torch.pow(x, 2).sum(
+ dim=1, keepdim=True
+ ).expand(m, n) + torch.pow(y, 2).sum(
+ dim=1, keepdim=True
+ ).expand(n, m).t()
+ dist.addmm_(1, -2, x, y.t())
+
+ return dist
+
+
+def cosine_similarity(x, y):
+ m, n = x.size(0), y.size(0)
+
+ x = x.view(m, -1)
+ y = y.view(n, -1)
+
+ y = y.t()
+ score = torch.mm(x, y)
+
+ return score
+
+
+def evaluate_ranking_list(
+ indices, query_label, query_cam, gallery_label, gallery_cam
+):
+ CMC = np.zeros((len(gallery_label)), dtype=np.int)
+ ap = 0.0
+
+ for i in range(len(query_label)):
+ ap_tmp, CMC_tmp = evaluate(
+ indices[i], query_label[i], query_cam[i], gallery_label,
+ gallery_cam
+ )
+ if CMC_tmp[0] == -1:
+ continue
+ CMC = CMC + CMC_tmp
+ ap += ap_tmp
+
+ CMC = CMC.astype(np.float32)
+ CMC = CMC / len(query_label) #average CMC
+ print(
+ 'Rank@1:%f Rank@5:%f Rank@10:%f mAP:%f' %
+ (CMC[0], CMC[4], CMC[9], ap / len(query_label))
+ )
+
+
+def evaluate(index, ql, qc, gl, gc):
+ query_index = np.argwhere(gl == ql)
+ camera_index = np.argwhere(gc == qc)
+
+ good_index = np.setdiff1d(query_index, camera_index, assume_unique=True)
+ junk_index1 = np.argwhere(gl == -1)
+ junk_index2 = np.intersect1d(query_index, camera_index)
+ junk_index = np.append(junk_index2, junk_index1) #.flatten())
+
+ CMC_tmp = compute_mAP(index, good_index, junk_index)
+ return CMC_tmp
+
+
+def compute_mAP(index, good_index, junk_index):
+ ap = 0
+ cmc = np.zeros((len(index)), dtype=np.int)
+ if good_index.size == 0: # if empty
+ cmc[0] = -1
+ return ap, cmc
+
+ # remove junk_index
+ mask = np.in1d(index, junk_index, invert=True)
+ index = index[mask]
+
+ # find good_index index
+ ngood = len(good_index)
+ mask = np.in1d(index, good_index)
+ rows_good = np.argwhere(mask == True)
+ rows_good = rows_good.flatten()
+
+ cmc[rows_good[0]:] = 1
+ for i in range(ngood):
+ d_recall = 1.0 / ngood
+ precision = (i+1) * 1.0 / (rows_good[i] + 1)
+ if rows_good[i] != 0:
+ old_precision = i * 1.0 / rows_good[i]
+ else:
+ old_precision = 1.0
+ ap = ap + d_recall * (old_precision+precision) / 2
+
+ return ap, cmc
diff --git a/trackers/strong_sort/deep/reid/torchreid/utils/__init__.py b/trackers/strong_sort/deep/reid/torchreid/utils/__init__.py
new file mode 100644
index 0000000000000000000000000000000000000000..50167c3f05779b327f88e0450048730a1224e473
--- /dev/null
+++ b/trackers/strong_sort/deep/reid/torchreid/utils/__init__.py
@@ -0,0 +1,10 @@
+from __future__ import absolute_import
+
+from .tools import *
+from .rerank import re_ranking
+from .loggers import *
+from .avgmeter import *
+from .reidtools import *
+from .torchtools import *
+from .model_complexity import compute_model_complexity
+from .feature_extractor import FeatureExtractor
diff --git a/trackers/strong_sort/deep/reid/torchreid/utils/avgmeter.py b/trackers/strong_sort/deep/reid/torchreid/utils/avgmeter.py
new file mode 100644
index 0000000000000000000000000000000000000000..b62d26d4ec7d8cbe8d21a209f9af53ff16b1cc7d
--- /dev/null
+++ b/trackers/strong_sort/deep/reid/torchreid/utils/avgmeter.py
@@ -0,0 +1,73 @@
+from __future__ import division, absolute_import
+from collections import defaultdict
+import torch
+
+__all__ = ['AverageMeter', 'MetricMeter']
+
+
+class AverageMeter(object):
+ """Computes and stores the average and current value.
+
+ Examples::
+ >>> # Initialize a meter to record loss
+ >>> losses = AverageMeter()
+ >>> # Update meter after every minibatch update
+ >>> losses.update(loss_value, batch_size)
+ """
+
+ def __init__(self):
+ self.reset()
+
+ def reset(self):
+ self.val = 0
+ self.avg = 0
+ self.sum = 0
+ self.count = 0
+
+ def update(self, val, n=1):
+ self.val = val
+ self.sum += val * n
+ self.count += n
+ self.avg = self.sum / self.count
+
+
+class MetricMeter(object):
+ """A collection of metrics.
+
+ Source: https://github.com/KaiyangZhou/Dassl.pytorch
+
+ Examples::
+ >>> # 1. Create an instance of MetricMeter
+ >>> metric = MetricMeter()
+ >>> # 2. Update using a dictionary as input
+ >>> input_dict = {'loss_1': value_1, 'loss_2': value_2}
+ >>> metric.update(input_dict)
+ >>> # 3. Convert to string and print
+ >>> print(str(metric))
+ """
+
+ def __init__(self, delimiter='\t'):
+ self.meters = defaultdict(AverageMeter)
+ self.delimiter = delimiter
+
+ def update(self, input_dict):
+ if input_dict is None:
+ return
+
+ if not isinstance(input_dict, dict):
+ raise TypeError(
+ 'Input to MetricMeter.update() must be a dictionary'
+ )
+
+ for k, v in input_dict.items():
+ if isinstance(v, torch.Tensor):
+ v = v.item()
+ self.meters[k].update(v)
+
+ def __str__(self):
+ output_str = []
+ for name, meter in self.meters.items():
+ output_str.append(
+ '{} {:.4f} ({:.4f})'.format(name, meter.val, meter.avg)
+ )
+ return self.delimiter.join(output_str)
diff --git a/trackers/strong_sort/deep/reid/torchreid/utils/feature_extractor.py b/trackers/strong_sort/deep/reid/torchreid/utils/feature_extractor.py
new file mode 100644
index 0000000000000000000000000000000000000000..83635fd3fe3419d54fbe1d0c6e9b7114deb8bdf5
--- /dev/null
+++ b/trackers/strong_sort/deep/reid/torchreid/utils/feature_extractor.py
@@ -0,0 +1,152 @@
+from __future__ import absolute_import
+import numpy as np
+import torch
+import torchvision.transforms as T
+from PIL import Image
+
+from torchreid.utils import (
+ check_isfile, load_pretrained_weights, compute_model_complexity
+)
+from torchreid.models import build_model
+
+
+class FeatureExtractor(object):
+ """A simple API for feature extraction.
+
+ FeatureExtractor can be used like a python function, which
+ accepts input of the following types:
+ - a list of strings (image paths)
+ - a list of numpy.ndarray each with shape (H, W, C)
+ - a single string (image path)
+ - a single numpy.ndarray with shape (H, W, C)
+ - a torch.Tensor with shape (B, C, H, W) or (C, H, W)
+
+ Returned is a torch tensor with shape (B, D) where D is the
+ feature dimension.
+
+ Args:
+ model_name (str): model name.
+ model_path (str): path to model weights.
+ image_size (sequence or int): image height and width.
+ pixel_mean (list): pixel mean for normalization.
+ pixel_std (list): pixel std for normalization.
+ pixel_norm (bool): whether to normalize pixels.
+ device (str): 'cpu' or 'cuda' (could be specific gpu devices).
+ verbose (bool): show model details.
+
+ Examples::
+
+ from torchreid.utils import FeatureExtractor
+
+ extractor = FeatureExtractor(
+ model_name='osnet_x1_0',
+ model_path='a/b/c/model.pth.tar',
+ device='cuda'
+ )
+
+ image_list = [
+ 'a/b/c/image001.jpg',
+ 'a/b/c/image002.jpg',
+ 'a/b/c/image003.jpg',
+ 'a/b/c/image004.jpg',
+ 'a/b/c/image005.jpg'
+ ]
+
+ features = extractor(image_list)
+ print(features.shape) # output (5, 512)
+ """
+
+ def __init__(
+ self,
+ model_name='',
+ model_path='',
+ image_size=(256, 128),
+ pixel_mean=[0.485, 0.456, 0.406],
+ pixel_std=[0.229, 0.224, 0.225],
+ pixel_norm=True,
+ device='cuda',
+ verbose=True
+ ):
+ # Build model
+ model = build_model(
+ model_name,
+ num_classes=1,
+ pretrained=not (model_path and check_isfile(model_path)),
+ use_gpu=device.startswith('cuda')
+ )
+ model.eval()
+
+ if verbose:
+ num_params, flops = compute_model_complexity(
+ model, (1, 3, image_size[0], image_size[1])
+ )
+ print('Model: {}'.format(model_name))
+ print('- params: {:,}'.format(num_params))
+ print('- flops: {:,}'.format(flops))
+
+ if model_path and check_isfile(model_path):
+ load_pretrained_weights(model, model_path)
+
+ # Build transform functions
+ transforms = []
+ transforms += [T.Resize(image_size)]
+ transforms += [T.ToTensor()]
+ if pixel_norm:
+ transforms += [T.Normalize(mean=pixel_mean, std=pixel_std)]
+ preprocess = T.Compose(transforms)
+
+ to_pil = T.ToPILImage()
+
+ device = torch.device(device)
+ model.to(device)
+
+ # Class attributes
+ self.model = model
+ self.preprocess = preprocess
+ self.to_pil = to_pil
+ self.device = device
+
+ def __call__(self, input):
+ if isinstance(input, list):
+ images = []
+
+ for element in input:
+ if isinstance(element, str):
+ image = Image.open(element).convert('RGB')
+
+ elif isinstance(element, np.ndarray):
+ image = self.to_pil(element)
+
+ else:
+ raise TypeError(
+ 'Type of each element must belong to [str | numpy.ndarray]'
+ )
+
+ image = self.preprocess(image)
+ images.append(image)
+
+ images = torch.stack(images, dim=0)
+ images = images.to(self.device)
+
+ elif isinstance(input, str):
+ image = Image.open(input).convert('RGB')
+ image = self.preprocess(image)
+ images = image.unsqueeze(0).to(self.device)
+
+ elif isinstance(input, np.ndarray):
+ image = self.to_pil(input)
+ image = self.preprocess(image)
+ images = image.unsqueeze(0).to(self.device)
+
+ elif isinstance(input, torch.Tensor):
+ if input.dim() == 3:
+ input = input.unsqueeze(0)
+ images = input.to(self.device)
+
+ else:
+ raise NotImplementedError
+
+ with torch.no_grad():
+ features = self.model(images)
+
+ return features
diff --git a/trackers/strong_sort/deep/reid/torchreid/utils/loggers.py b/trackers/strong_sort/deep/reid/torchreid/utils/loggers.py
new file mode 100644
index 0000000000000000000000000000000000000000..f7fae3c57b300b76afd8e5a485439aee9fefdbbe
--- /dev/null
+++ b/trackers/strong_sort/deep/reid/torchreid/utils/loggers.py
@@ -0,0 +1,146 @@
+from __future__ import absolute_import
+import os
+import sys
+import os.path as osp
+
+from .tools import mkdir_if_missing
+
+__all__ = ['Logger', 'RankLogger']
+
+
+class Logger(object):
+ """Writes console output to external text file.
+
+ Imported from ``_
+
+ Args:
+ fpath (str): directory to save logging file.
+
+ Examples::
+ >>> import sys
+ >>> import os
+ >>> import os.path as osp
+ >>> from torchreid.utils import Logger
+ >>> save_dir = 'log/resnet50-softmax-market1501'
+ >>> log_name = 'train.log'
+ >>> sys.stdout = Logger(osp.join(args.save_dir, log_name))
+ """
+
+ def __init__(self, fpath=None):
+ self.console = sys.stdout
+ self.file = None
+ if fpath is not None:
+ mkdir_if_missing(osp.dirname(fpath))
+ self.file = open(fpath, 'w')
+
+ def __del__(self):
+ self.close()
+
+ def __enter__(self):
+ pass
+
+ def __exit__(self, *args):
+ self.close()
+
+ def write(self, msg):
+ self.console.write(msg)
+ if self.file is not None:
+ self.file.write(msg)
+
+ def flush(self):
+ self.console.flush()
+ if self.file is not None:
+ self.file.flush()
+ os.fsync(self.file.fileno())
+
+ def close(self):
+ self.console.close()
+ if self.file is not None:
+ self.file.close()
+
+
+class RankLogger(object):
+ """Records the rank1 matching accuracy obtained for each
+ test dataset at specified evaluation steps and provides a function
+ to show the summarized results, which are convenient for analysis.
+
+ Args:
+ sources (str or list): source dataset name(s).
+ targets (str or list): target dataset name(s).
+
+ Examples::
+ >>> from torchreid.utils import RankLogger
+ >>> s = 'market1501'
+ >>> t = 'market1501'
+ >>> ranklogger = RankLogger(s, t)
+ >>> ranklogger.write(t, 10, 0.5)
+ >>> ranklogger.write(t, 20, 0.7)
+ >>> ranklogger.write(t, 30, 0.9)
+ >>> ranklogger.show_summary()
+ >>> # You will see:
+ >>> # => Show performance summary
+ >>> # market1501 (source)
+ >>> # - epoch 10 rank1 50.0%
+ >>> # - epoch 20 rank1 70.0%
+ >>> # - epoch 30 rank1 90.0%
+ >>> # If there are multiple test datasets
+ >>> t = ['market1501', 'dukemtmcreid']
+ >>> ranklogger = RankLogger(s, t)
+ >>> ranklogger.write(t[0], 10, 0.5)
+ >>> ranklogger.write(t[0], 20, 0.7)
+ >>> ranklogger.write(t[0], 30, 0.9)
+ >>> ranklogger.write(t[1], 10, 0.1)
+ >>> ranklogger.write(t[1], 20, 0.2)
+ >>> ranklogger.write(t[1], 30, 0.3)
+ >>> ranklogger.show_summary()
+ >>> # You can see:
+ >>> # => Show performance summary
+ >>> # market1501 (source)
+ >>> # - epoch 10 rank1 50.0%
+ >>> # - epoch 20 rank1 70.0%
+ >>> # - epoch 30 rank1 90.0%
+ >>> # dukemtmcreid (target)
+ >>> # - epoch 10 rank1 10.0%
+ >>> # - epoch 20 rank1 20.0%
+ >>> # - epoch 30 rank1 30.0%
+ """
+
+ def __init__(self, sources, targets):
+ self.sources = sources
+ self.targets = targets
+
+ if isinstance(self.sources, str):
+ self.sources = [self.sources]
+
+ if isinstance(self.targets, str):
+ self.targets = [self.targets]
+
+ self.logger = {
+ name: {
+ 'epoch': [],
+ 'rank1': []
+ }
+ for name in self.targets
+ }
+
+ def write(self, name, epoch, rank1):
+ """Writes result.
+
+ Args:
+ name (str): dataset name.
+ epoch (int): current epoch.
+ rank1 (float): rank1 result.
+ """
+ self.logger[name]['epoch'].append(epoch)
+ self.logger[name]['rank1'].append(rank1)
+
+ def show_summary(self):
+ """Shows saved results."""
+ print('=> Show performance summary')
+ for name in self.targets:
+ from_where = 'source' if name in self.sources else 'target'
+ print('{} ({})'.format(name, from_where))
+ for epoch, rank1 in zip(
+ self.logger[name]['epoch'], self.logger[name]['rank1']
+ ):
+ print('- epoch {}\t rank1 {:.1%}'.format(epoch, rank1))
diff --git a/trackers/strong_sort/deep/reid/torchreid/utils/model_complexity.py b/trackers/strong_sort/deep/reid/torchreid/utils/model_complexity.py
new file mode 100644
index 0000000000000000000000000000000000000000..7d1dc1ed13ba747b510030a5690b81cd0d570822
--- /dev/null
+++ b/trackers/strong_sort/deep/reid/torchreid/utils/model_complexity.py
@@ -0,0 +1,363 @@
+from __future__ import division, print_function, absolute_import
+import math
+import numpy as np
+from itertools import repeat
+from collections import namedtuple, defaultdict
+import torch
+
+__all__ = ['compute_model_complexity']
+"""
+Utility
+"""
+
+
+def _ntuple(n):
+
+ def parse(x):
+ if isinstance(x, int):
+ return tuple(repeat(x, n))
+ return x
+
+ return parse
+
+
+_single = _ntuple(1)
+_pair = _ntuple(2)
+_triple = _ntuple(3)
+"""
+Convolution
+"""
+
+
+def hook_convNd(m, x, y):
+ k = torch.prod(torch.Tensor(m.kernel_size)).item()
+ cin = m.in_channels
+ flops_per_ele = k * cin # + (k*cin-1)
+ if m.bias is not None:
+ flops_per_ele += 1
+ flops = flops_per_ele * y.numel() / m.groups
+ return int(flops)
+
+
+"""
+Pooling
+"""
+
+
+def hook_maxpool1d(m, x, y):
+ flops_per_ele = m.kernel_size - 1
+ flops = flops_per_ele * y.numel()
+ return int(flops)
+
+
+def hook_maxpool2d(m, x, y):
+ k = _pair(m.kernel_size)
+ k = torch.prod(torch.Tensor(k)).item()
+ # ops: compare
+ flops_per_ele = k - 1
+ flops = flops_per_ele * y.numel()
+ return int(flops)
+
+
+def hook_maxpool3d(m, x, y):
+ k = _triple(m.kernel_size)
+ k = torch.prod(torch.Tensor(k)).item()
+ flops_per_ele = k - 1
+ flops = flops_per_ele * y.numel()
+ return int(flops)
+
+
+def hook_avgpool1d(m, x, y):
+ flops_per_ele = m.kernel_size
+ flops = flops_per_ele * y.numel()
+ return int(flops)
+
+
+def hook_avgpool2d(m, x, y):
+ k = _pair(m.kernel_size)
+ k = torch.prod(torch.Tensor(k)).item()
+ flops_per_ele = k
+ flops = flops_per_ele * y.numel()
+ return int(flops)
+
+
+def hook_avgpool3d(m, x, y):
+ k = _triple(m.kernel_size)
+ k = torch.prod(torch.Tensor(k)).item()
+ flops_per_ele = k
+ flops = flops_per_ele * y.numel()
+ return int(flops)
+
+
+def hook_adapmaxpool1d(m, x, y):
+ x = x[0]
+ out_size = m.output_size
+ k = math.ceil(x.size(2) / out_size)
+ flops_per_ele = k - 1
+ flops = flops_per_ele * y.numel()
+ return int(flops)
+
+
+def hook_adapmaxpool2d(m, x, y):
+ x = x[0]
+ out_size = _pair(m.output_size)
+ k = torch.Tensor(list(x.size()[2:])) / torch.Tensor(out_size)
+ k = torch.prod(torch.ceil(k)).item()
+ flops_per_ele = k - 1
+ flops = flops_per_ele * y.numel()
+ return int(flops)
+
+
+def hook_adapmaxpool3d(m, x, y):
+ x = x[0]
+ out_size = _triple(m.output_size)
+ k = torch.Tensor(list(x.size()[2:])) / torch.Tensor(out_size)
+ k = torch.prod(torch.ceil(k)).item()
+ flops_per_ele = k - 1
+ flops = flops_per_ele * y.numel()
+ return int(flops)
+
+
+def hook_adapavgpool1d(m, x, y):
+ x = x[0]
+ out_size = m.output_size
+ k = math.ceil(x.size(2) / out_size)
+ flops_per_ele = k
+ flops = flops_per_ele * y.numel()
+ return int(flops)
+
+
+def hook_adapavgpool2d(m, x, y):
+ x = x[0]
+ out_size = _pair(m.output_size)
+ k = torch.Tensor(list(x.size()[2:])) / torch.Tensor(out_size)
+ k = torch.prod(torch.ceil(k)).item()
+ flops_per_ele = k
+ flops = flops_per_ele * y.numel()
+ return int(flops)
+
+
+def hook_adapavgpool3d(m, x, y):
+ x = x[0]
+ out_size = _triple(m.output_size)
+ k = torch.Tensor(list(x.size()[2:])) / torch.Tensor(out_size)
+ k = torch.prod(torch.ceil(k)).item()
+ flops_per_ele = k
+ flops = flops_per_ele * y.numel()
+ return int(flops)
+
+
+"""
+Non-linear activations
+"""
+
+
+def hook_relu(m, x, y):
+ # eq: max(0, x)
+ num_ele = y.numel()
+ return int(num_ele)
+
+
+def hook_leakyrelu(m, x, y):
+ # eq: max(0, x) + negative_slope*min(0, x)
+ num_ele = y.numel()
+ flops = 3 * num_ele
+ return int(flops)
+
+
+"""
+Normalization
+"""
+
+
+def hook_batchnormNd(m, x, y):
+ num_ele = y.numel()
+ flops = 2 * num_ele # mean and std
+ if m.affine:
+ flops += 2 * num_ele # gamma and beta
+ return int(flops)
+
+
+def hook_instancenormNd(m, x, y):
+ return hook_batchnormNd(m, x, y)
+
+
+def hook_groupnorm(m, x, y):
+ return hook_batchnormNd(m, x, y)
+
+
+def hook_layernorm(m, x, y):
+ num_ele = y.numel()
+ flops = 2 * num_ele # mean and std
+ if m.elementwise_affine:
+ flops += 2 * num_ele # gamma and beta
+ return int(flops)
+
+
+"""
+Linear
+"""
+
+
+def hook_linear(m, x, y):
+ flops_per_ele = m.in_features # + (m.in_features-1)
+ if m.bias is not None:
+ flops_per_ele += 1
+ flops = flops_per_ele * y.numel()
+ return int(flops)
+
+
+__generic_flops_counter = {
+ # Convolution
+ 'Conv1d': hook_convNd,
+ 'Conv2d': hook_convNd,
+ 'Conv3d': hook_convNd,
+ # Pooling
+ 'MaxPool1d': hook_maxpool1d,
+ 'MaxPool2d': hook_maxpool2d,
+ 'MaxPool3d': hook_maxpool3d,
+ 'AvgPool1d': hook_avgpool1d,
+ 'AvgPool2d': hook_avgpool2d,
+ 'AvgPool3d': hook_avgpool3d,
+ 'AdaptiveMaxPool1d': hook_adapmaxpool1d,
+ 'AdaptiveMaxPool2d': hook_adapmaxpool2d,
+ 'AdaptiveMaxPool3d': hook_adapmaxpool3d,
+ 'AdaptiveAvgPool1d': hook_adapavgpool1d,
+ 'AdaptiveAvgPool2d': hook_adapavgpool2d,
+ 'AdaptiveAvgPool3d': hook_adapavgpool3d,
+ # Non-linear activations
+ 'ReLU': hook_relu,
+ 'ReLU6': hook_relu,
+ 'LeakyReLU': hook_leakyrelu,
+ # Normalization
+ 'BatchNorm1d': hook_batchnormNd,
+ 'BatchNorm2d': hook_batchnormNd,
+ 'BatchNorm3d': hook_batchnormNd,
+ 'InstanceNorm1d': hook_instancenormNd,
+ 'InstanceNorm2d': hook_instancenormNd,
+ 'InstanceNorm3d': hook_instancenormNd,
+ 'GroupNorm': hook_groupnorm,
+ 'LayerNorm': hook_layernorm,
+ # Linear
+ 'Linear': hook_linear,
+}
+
+__conv_linear_flops_counter = {
+ # Convolution
+ 'Conv1d': hook_convNd,
+ 'Conv2d': hook_convNd,
+ 'Conv3d': hook_convNd,
+ # Linear
+ 'Linear': hook_linear,
+}
+
+
+def _get_flops_counter(only_conv_linear):
+ if only_conv_linear:
+ return __conv_linear_flops_counter
+ return __generic_flops_counter
+
+
+def compute_model_complexity(
+ model, input_size, verbose=False, only_conv_linear=True
+):
+ """Returns number of parameters and FLOPs.
+
+ .. note::
+ (1) this function only provides an estimate of the theoretical time complexity
+ rather than the actual running time which depends on implementations and hardware,
+ and (2) the FLOPs is only counted for layers that are used at test time. This means
+ that redundant layers such as person ID classification layer will be ignored as it
+ is discarded when doing feature extraction. Note that the inference graph depends on
+ how you construct the computations in ``forward()``.
+
+ Args:
+ model (nn.Module): network model.
+ input_size (tuple): input size, e.g. (1, 3, 256, 128).
+ verbose (bool, optional): shows detailed complexity of
+ each module. Default is False.
+ only_conv_linear (bool, optional): only considers convolution
+ and linear layers when counting flops. Default is True.
+ If set to False, flops of all layers will be counted.
+
+ Examples::
+ >>> from torchreid import models, utils
+ >>> model = models.build_model(name='resnet50', num_classes=1000)
+ >>> num_params, flops = utils.compute_model_complexity(model, (1, 3, 256, 128), verbose=True)
+ """
+ registered_handles = []
+ layer_list = []
+ layer = namedtuple('layer', ['class_name', 'params', 'flops'])
+
+ def _add_hooks(m):
+
+ def _has_submodule(m):
+ return len(list(m.children())) > 0
+
+ def _hook(m, x, y):
+ params = sum(p.numel() for p in m.parameters())
+ class_name = str(m.__class__.__name__)
+ flops_counter = _get_flops_counter(only_conv_linear)
+ if class_name in flops_counter:
+ flops = flops_counter[class_name](m, x, y)
+ else:
+ flops = 0
+ layer_list.append(
+ layer(class_name=class_name, params=params, flops=flops)
+ )
+
+ # only consider the very basic nn layer
+ if _has_submodule(m):
+ return
+
+ handle = m.register_forward_hook(_hook)
+ registered_handles.append(handle)
+
+ default_train_mode = model.training
+
+ model.eval().apply(_add_hooks)
+ input = torch.rand(input_size)
+ if next(model.parameters()).is_cuda:
+ input = input.cuda()
+ model(input) # forward
+
+ for handle in registered_handles:
+ handle.remove()
+
+ model.train(default_train_mode)
+
+ if verbose:
+ per_module_params = defaultdict(list)
+ per_module_flops = defaultdict(list)
+
+ total_params, total_flops = 0, 0
+
+ for layer in layer_list:
+ total_params += layer.params
+ total_flops += layer.flops
+ if verbose:
+ per_module_params[layer.class_name].append(layer.params)
+ per_module_flops[layer.class_name].append(layer.flops)
+
+ if verbose:
+ num_udscore = 55
+ print(' {}'.format('-' * num_udscore))
+ print(' Model complexity with input size {}'.format(input_size))
+ print(' {}'.format('-' * num_udscore))
+ for class_name in per_module_params:
+ params = int(np.sum(per_module_params[class_name]))
+ flops = int(np.sum(per_module_flops[class_name]))
+ print(
+ ' {} (params={:,}, flops={:,})'.format(
+ class_name, params, flops
+ )
+ )
+ print(' {}'.format('-' * num_udscore))
+ print(
+ ' Total (params={:,}, flops={:,})'.format(
+ total_params, total_flops
+ )
+ )
+ print(' {}'.format('-' * num_udscore))
+
+ return total_params, total_flops
diff --git a/trackers/strong_sort/deep/reid/torchreid/utils/reidtools.py b/trackers/strong_sort/deep/reid/torchreid/utils/reidtools.py
new file mode 100644
index 0000000000000000000000000000000000000000..acb87602ba905062424a9ebbe524f9cad83384e3
--- /dev/null
+++ b/trackers/strong_sort/deep/reid/torchreid/utils/reidtools.py
@@ -0,0 +1,154 @@
+from __future__ import print_function, absolute_import
+import numpy as np
+import shutil
+import os.path as osp
+import cv2
+
+from .tools import mkdir_if_missing
+
+__all__ = ['visualize_ranked_results']
+
+GRID_SPACING = 10
+QUERY_EXTRA_SPACING = 90
+BW = 5 # border width
+GREEN = (0, 255, 0)
+RED = (0, 0, 255)
+
+
+def visualize_ranked_results(
+ distmat, dataset, data_type, width=128, height=256, save_dir='', topk=10
+):
+ """Visualizes ranked results.
+
+ Supports both image-reid and video-reid.
+
+ For image-reid, ranks will be plotted in a single figure. For video-reid, ranks will be
+ saved in folders each containing a tracklet.
+
+ Args:
+ distmat (numpy.ndarray): distance matrix of shape (num_query, num_gallery).
+ dataset (tuple): a 2-tuple containing (query, gallery), each of which contains
+ tuples of (img_path(s), pid, camid, dsetid).
+ data_type (str): "image" or "video".
+ width (int, optional): resized image width. Default is 128.
+ height (int, optional): resized image height. Default is 256.
+ save_dir (str): directory to save output images.
+ topk (int, optional): denoting top-k images in the rank list to be visualized.
+ Default is 10.
+ """
+ num_q, num_g = distmat.shape
+ mkdir_if_missing(save_dir)
+
+ print('# query: {}\n# gallery {}'.format(num_q, num_g))
+ print('Visualizing top-{} ranks ...'.format(topk))
+
+ query, gallery = dataset
+ assert num_q == len(query)
+ assert num_g == len(gallery)
+
+ indices = np.argsort(distmat, axis=1)
+
+ def _cp_img_to(src, dst, rank, prefix, matched=False):
+ """
+ Args:
+ src: image path or tuple (for vidreid)
+ dst: target directory
+ rank: int, denoting ranked position, starting from 1
+ prefix: string
+ matched: bool
+ """
+ if isinstance(src, (tuple, list)):
+ if prefix == 'gallery':
+ suffix = 'TRUE' if matched else 'FALSE'
+ dst = osp.join(
+ dst, prefix + '_top' + str(rank).zfill(3)
+ ) + '_' + suffix
+ else:
+ dst = osp.join(dst, prefix + '_top' + str(rank).zfill(3))
+ mkdir_if_missing(dst)
+ for img_path in src:
+ shutil.copy(img_path, dst)
+ else:
+ dst = osp.join(
+ dst, prefix + '_top' + str(rank).zfill(3) + '_name_' +
+ osp.basename(src)
+ )
+ shutil.copy(src, dst)
+
+ for q_idx in range(num_q):
+ qimg_path, qpid, qcamid = query[q_idx][:3]
+ qimg_path_name = qimg_path[0] if isinstance(
+ qimg_path, (tuple, list)
+ ) else qimg_path
+
+ if data_type == 'image':
+ qimg = cv2.imread(qimg_path)
+ qimg = cv2.resize(qimg, (width, height))
+ qimg = cv2.copyMakeBorder(
+ qimg, BW, BW, BW, BW, cv2.BORDER_CONSTANT, value=(0, 0, 0)
+ )
+ # resize twice to ensure that the border width is consistent across images
+ qimg = cv2.resize(qimg, (width, height))
+ num_cols = topk + 1
+ grid_img = 255 * np.ones(
+ (
+ height,
+ num_cols*width + topk*GRID_SPACING + QUERY_EXTRA_SPACING, 3
+ ),
+ dtype=np.uint8
+ )
+ grid_img[:, :width, :] = qimg
+ else:
+ qdir = osp.join(
+ save_dir, osp.basename(osp.splitext(qimg_path_name)[0])
+ )
+ mkdir_if_missing(qdir)
+ _cp_img_to(qimg_path, qdir, rank=0, prefix='query')
+
+ rank_idx = 1
+ for g_idx in indices[q_idx, :]:
+ gimg_path, gpid, gcamid = gallery[g_idx][:3]
+ invalid = (qpid == gpid) & (qcamid == gcamid)
+
+ if not invalid:
+ matched = gpid == qpid
+ if data_type == 'image':
+ border_color = GREEN if matched else RED
+ gimg = cv2.imread(gimg_path)
+ gimg = cv2.resize(gimg, (width, height))
+ gimg = cv2.copyMakeBorder(
+ gimg,
+ BW,
+ BW,
+ BW,
+ BW,
+ cv2.BORDER_CONSTANT,
+ value=border_color
+ )
+ gimg = cv2.resize(gimg, (width, height))
+ start = rank_idx*width + rank_idx*GRID_SPACING + QUERY_EXTRA_SPACING
+ end = (
+ rank_idx+1
+ ) * width + rank_idx*GRID_SPACING + QUERY_EXTRA_SPACING
+ grid_img[:, start:end, :] = gimg
+ else:
+ _cp_img_to(
+ gimg_path,
+ qdir,
+ rank=rank_idx,
+ prefix='gallery',
+ matched=matched
+ )
+
+ rank_idx += 1
+ if rank_idx > topk:
+ break
+
+ if data_type == 'image':
+ imname = osp.basename(osp.splitext(qimg_path_name)[0])
+ cv2.imwrite(osp.join(save_dir, imname + '.jpg'), grid_img)
+
+ if (q_idx+1) % 100 == 0:
+ print('- done {}/{}'.format(q_idx + 1, num_q))
+
+ print('Done. Images have been saved to "{}" ...'.format(save_dir))
diff --git a/trackers/strong_sort/deep/reid/torchreid/utils/rerank.py b/trackers/strong_sort/deep/reid/torchreid/utils/rerank.py
new file mode 100644
index 0000000000000000000000000000000000000000..efadf5afe26ec057bd66e161a34dea637849e7ab
--- /dev/null
+++ b/trackers/strong_sort/deep/reid/torchreid/utils/rerank.py
@@ -0,0 +1,113 @@
+#!/usr/bin/env python2/python3
+# -*- coding: utf-8 -*-
+"""
+Source: https://github.com/zhunzhong07/person-re-ranking
+
+Created on Mon Jun 26 14:46:56 2017
+@author: luohao
+Modified by Houjing Huang, 2017-12-22.
+- This version accepts distance matrix instead of raw features.
+- The difference of `/` division between python 2 and 3 is handled.
+- numpy.float16 is replaced by numpy.float32 for numerical precision.
+
+CVPR2017 paper:Zhong Z, Zheng L, Cao D, et al. Re-ranking Person Re-identification with k-reciprocal Encoding[J]. 2017.
+url:http://openaccess.thecvf.com/content_cvpr_2017/papers/Zhong_Re-Ranking_Person_Re-Identification_CVPR_2017_paper.pdf
+Matlab version: https://github.com/zhunzhong07/person-re-ranking
+
+API
+q_g_dist: query-gallery distance matrix, numpy array, shape [num_query, num_gallery]
+q_q_dist: query-query distance matrix, numpy array, shape [num_query, num_query]
+g_g_dist: gallery-gallery distance matrix, numpy array, shape [num_gallery, num_gallery]
+k1, k2, lambda_value: parameters, the original paper is (k1=20, k2=6, lambda_value=0.3)
+Returns:
+ final_dist: re-ranked distance, numpy array, shape [num_query, num_gallery]
+"""
+from __future__ import division, print_function, absolute_import
+import numpy as np
+
+__all__ = ['re_ranking']
+
+
+def re_ranking(q_g_dist, q_q_dist, g_g_dist, k1=20, k2=6, lambda_value=0.3):
+
+ # The following naming, e.g. gallery_num, is different from outer scope.
+ # Don't care about it.
+
+ original_dist = np.concatenate(
+ [
+ np.concatenate([q_q_dist, q_g_dist], axis=1),
+ np.concatenate([q_g_dist.T, g_g_dist], axis=1)
+ ],
+ axis=0
+ )
+ original_dist = np.power(original_dist, 2).astype(np.float32)
+ original_dist = np.transpose(
+ 1. * original_dist / np.max(original_dist, axis=0)
+ )
+ V = np.zeros_like(original_dist).astype(np.float32)
+ initial_rank = np.argsort(original_dist).astype(np.int32)
+
+ query_num = q_g_dist.shape[0]
+ gallery_num = q_g_dist.shape[0] + q_g_dist.shape[1]
+ all_num = gallery_num
+
+ for i in range(all_num):
+ # k-reciprocal neighbors
+ forward_k_neigh_index = initial_rank[i, :k1 + 1]
+ backward_k_neigh_index = initial_rank[forward_k_neigh_index, :k1 + 1]
+ fi = np.where(backward_k_neigh_index == i)[0]
+ k_reciprocal_index = forward_k_neigh_index[fi]
+ k_reciprocal_expansion_index = k_reciprocal_index
+ for j in range(len(k_reciprocal_index)):
+ candidate = k_reciprocal_index[j]
+ candidate_forward_k_neigh_index = initial_rank[
+ candidate, :int(np.around(k1 / 2.)) + 1]
+ candidate_backward_k_neigh_index = initial_rank[
+ candidate_forward_k_neigh_index, :int(np.around(k1 / 2.)) + 1]
+ fi_candidate = np.where(
+ candidate_backward_k_neigh_index == candidate
+ )[0]
+ candidate_k_reciprocal_index = candidate_forward_k_neigh_index[
+ fi_candidate]
+ if len(
+ np.
+ intersect1d(candidate_k_reciprocal_index, k_reciprocal_index)
+ ) > 2. / 3 * len(candidate_k_reciprocal_index):
+ k_reciprocal_expansion_index = np.append(
+ k_reciprocal_expansion_index, candidate_k_reciprocal_index
+ )
+
+ k_reciprocal_expansion_index = np.unique(k_reciprocal_expansion_index)
+ weight = np.exp(-original_dist[i, k_reciprocal_expansion_index])
+ V[i, k_reciprocal_expansion_index] = 1. * weight / np.sum(weight)
+ original_dist = original_dist[:query_num, ]
+ if k2 != 1:
+ V_qe = np.zeros_like(V, dtype=np.float32)
+ for i in range(all_num):
+ V_qe[i, :] = np.mean(V[initial_rank[i, :k2], :], axis=0)
+ V = V_qe
+ del V_qe
+ del initial_rank
+ invIndex = []
+ for i in range(gallery_num):
+ invIndex.append(np.where(V[:, i] != 0)[0])
+
+ jaccard_dist = np.zeros_like(original_dist, dtype=np.float32)
+
+ for i in range(query_num):
+ temp_min = np.zeros(shape=[1, gallery_num], dtype=np.float32)
+ indNonZero = np.where(V[i, :] != 0)[0]
+ indImages = []
+ indImages = [invIndex[ind] for ind in indNonZero]
+ for j in range(len(indNonZero)):
+ temp_min[0, indImages[j]] = temp_min[0, indImages[j]] + np.minimum(
+ V[i, indNonZero[j]], V[indImages[j], indNonZero[j]]
+ )
+ jaccard_dist[i] = 1 - temp_min / (2.-temp_min)
+
+ final_dist = jaccard_dist * (1-lambda_value) + original_dist*lambda_value
+ del original_dist
+ del V
+ del jaccard_dist
+ final_dist = final_dist[:query_num, query_num:]
+ return final_dist
diff --git a/trackers/strong_sort/deep/reid/torchreid/utils/tools.py b/trackers/strong_sort/deep/reid/torchreid/utils/tools.py
new file mode 100644
index 0000000000000000000000000000000000000000..2ccf63ab066fdc8132a8f2f7f7b3266de2edc845
--- /dev/null
+++ b/trackers/strong_sort/deep/reid/torchreid/utils/tools.py
@@ -0,0 +1,143 @@
+from __future__ import division, print_function, absolute_import
+import os
+import sys
+import json
+import time
+import errno
+import numpy as np
+import random
+import os.path as osp
+import warnings
+import PIL
+import torch
+from PIL import Image
+
+__all__ = [
+ 'mkdir_if_missing', 'check_isfile', 'read_json', 'write_json',
+ 'set_random_seed', 'download_url', 'read_image', 'collect_env_info',
+ 'listdir_nohidden'
+]
+
+
+def mkdir_if_missing(dirname):
+ """Creates dirname if it is missing."""
+ if not osp.exists(dirname):
+ try:
+ os.makedirs(dirname)
+ except OSError as e:
+ if e.errno != errno.EEXIST:
+ raise
+
+
+def check_isfile(fpath):
+ """Checks if the given path is a file.
+
+ Args:
+ fpath (str): file path.
+
+ Returns:
+ bool
+ """
+ isfile = osp.isfile(fpath)
+ if not isfile:
+ warnings.warn('No file found at "{}"'.format(fpath))
+ return isfile
+
+
+def read_json(fpath):
+ """Reads json file from a path."""
+ with open(fpath, 'r') as f:
+ obj = json.load(f)
+ return obj
+
+
+def write_json(obj, fpath):
+ """Writes to a json file."""
+ mkdir_if_missing(osp.dirname(fpath))
+ with open(fpath, 'w') as f:
+ json.dump(obj, f, indent=4, separators=(',', ': '))
+
+
+def set_random_seed(seed):
+ random.seed(seed)
+ np.random.seed(seed)
+ torch.manual_seed(seed)
+ torch.cuda.manual_seed_all(seed)
+
+
+def download_url(url, dst):
+ """Downloads file from a url to a destination.
+
+ Args:
+ url (str): url to download file.
+ dst (str): destination path.
+ """
+ from six.moves import urllib
+ print('* url="{}"'.format(url))
+ print('* destination="{}"'.format(dst))
+
+ def _reporthook(count, block_size, total_size):
+ global start_time
+ if count == 0:
+ start_time = time.time()
+ return
+ duration = time.time() - start_time
+ progress_size = int(count * block_size)
+ speed = 0 if duration == 0 else int(progress_size / (1024*duration))
+ percent = int(count * block_size * 100 / total_size)
+ sys.stdout.write(
+ '\r...%d%%, %d MB, %d KB/s, %d seconds passed' %
+ (percent, progress_size / (1024*1024), speed, duration)
+ )
+ sys.stdout.flush()
+
+ urllib.request.urlretrieve(url, dst, _reporthook)
+ sys.stdout.write('\n')
+
+
+def read_image(path):
+ """Reads image from path using ``PIL.Image``.
+
+ Args:
+ path (str): path to an image.
+
+ Returns:
+ PIL image
+ """
+ got_img = False
+ if not osp.exists(path):
+ raise IOError('"{}" does not exist'.format(path))
+ while not got_img:
+ try:
+ img = Image.open(path).convert('RGB')
+ got_img = True
+ except IOError:
+ print(
+ 'IOError incurred when reading "{}". Will redo. Don\'t worry. Just chill.'
+ .format(path)
+ )
+ return img
+
+
+def collect_env_info():
+ """Returns env info as a string.
+
+ Code source: github.com/facebookresearch/maskrcnn-benchmark
+ """
+ from torch.utils.collect_env import get_pretty_env_info
+ env_str = get_pretty_env_info()
+ env_str += '\n Pillow ({})'.format(PIL.__version__)
+ return env_str
+
+
+def listdir_nohidden(path, sort=False):
+ """List non-hidden items in a directory.
+
+ Args:
+ path (str): directory path.
+ sort (bool): sort the items.
+ """
+ items = [f for f in os.listdir(path) if not f.startswith('.')]
+ if sort:
+ items.sort()
+ return items
diff --git a/trackers/strong_sort/deep/reid/torchreid/utils/torchtools.py b/trackers/strong_sort/deep/reid/torchreid/utils/torchtools.py
new file mode 100644
index 0000000000000000000000000000000000000000..e854278d90106e64c0f0822b2fa699da05221f7c
--- /dev/null
+++ b/trackers/strong_sort/deep/reid/torchreid/utils/torchtools.py
@@ -0,0 +1,312 @@
+from __future__ import division, print_function, absolute_import
+import pickle
+import shutil
+import os.path as osp
+import warnings
+from functools import partial
+from collections import OrderedDict
+import torch
+import torch.nn as nn
+
+from .tools import mkdir_if_missing
+
+__all__ = [
+ 'save_checkpoint', 'load_checkpoint', 'resume_from_checkpoint',
+ 'open_all_layers', 'open_specified_layers', 'count_num_param',
+ 'load_pretrained_weights'
+]
+
+
+def save_checkpoint(
+ state, save_dir, is_best=False, remove_module_from_keys=False
+):
+ r"""Saves checkpoint.
+
+ Args:
+ state (dict): dictionary.
+ save_dir (str): directory to save checkpoint.
+ is_best (bool, optional): if True, this checkpoint will be copied and named
+ ``model-best.pth.tar``. Default is False.
+ remove_module_from_keys (bool, optional): whether to remove "module."
+ from layer names. Default is False.
+
+ Examples::
+ >>> state = {
+ >>> 'state_dict': model.state_dict(),
+ >>> 'epoch': 10,
+ >>> 'rank1': 0.5,
+ >>> 'optimizer': optimizer.state_dict()
+ >>> }
+ >>> save_checkpoint(state, 'log/my_model')
+ """
+ mkdir_if_missing(save_dir)
+ if remove_module_from_keys:
+ # remove 'module.' in state_dict's keys
+ state_dict = state['state_dict']
+ new_state_dict = OrderedDict()
+ for k, v in state_dict.items():
+ if k.startswith('module.'):
+ k = k[7:]
+ new_state_dict[k] = v
+ state['state_dict'] = new_state_dict
+ # save
+ epoch = state['epoch']
+ fpath = osp.join(save_dir, 'model.pth.tar-' + str(epoch))
+ torch.save(state, fpath)
+ print('Checkpoint saved to "{}"'.format(fpath))
+ if is_best:
+ shutil.copy(fpath, osp.join(osp.dirname(fpath), 'model-best.pth.tar'))
+
+
+def load_checkpoint(fpath):
+ r"""Loads checkpoint.
+
+ ``UnicodeDecodeError`` can be well handled, which means
+ python2-saved files can be read from python3.
+
+ Args:
+ fpath (str): path to checkpoint.
+
+ Returns:
+ dict
+
+ Examples::
+ >>> from torchreid.utils import load_checkpoint
+ >>> fpath = 'log/my_model/model.pth.tar-10'
+ >>> checkpoint = load_checkpoint(fpath)
+ """
+ if fpath is None:
+ raise ValueError('File path is None')
+ fpath = osp.abspath(osp.expanduser(fpath))
+ if not osp.exists(fpath):
+ raise FileNotFoundError('File is not found at "{}"'.format(fpath))
+ map_location = None if torch.cuda.is_available() else 'cpu'
+ try:
+ checkpoint = torch.load(fpath, map_location=map_location)
+ except UnicodeDecodeError:
+ pickle.load = partial(pickle.load, encoding="latin1")
+ pickle.Unpickler = partial(pickle.Unpickler, encoding="latin1")
+ checkpoint = torch.load(
+ fpath, pickle_module=pickle, map_location=map_location
+ )
+ except Exception:
+ print('Unable to load checkpoint from "{}"'.format(fpath))
+ raise
+ return checkpoint
+
+
+def resume_from_checkpoint(fpath, model, optimizer=None, scheduler=None):
+ r"""Resumes training from a checkpoint.
+
+ This will load (1) model weights and (2) ``state_dict``
+ of optimizer if ``optimizer`` is not None.
+
+ Args:
+ fpath (str): path to checkpoint.
+ model (nn.Module): model.
+ optimizer (Optimizer, optional): an Optimizer.
+ scheduler (LRScheduler, optional): an LRScheduler.
+
+ Returns:
+ int: start_epoch.
+
+ Examples::
+ >>> from torchreid.utils import resume_from_checkpoint
+ >>> fpath = 'log/my_model/model.pth.tar-10'
+ >>> start_epoch = resume_from_checkpoint(
+ >>> fpath, model, optimizer, scheduler
+ >>> )
+ """
+ print('Loading checkpoint from "{}"'.format(fpath))
+ checkpoint = load_checkpoint(fpath)
+ model.load_state_dict(checkpoint['state_dict'])
+ print('Loaded model weights')
+ if optimizer is not None and 'optimizer' in checkpoint.keys():
+ optimizer.load_state_dict(checkpoint['optimizer'])
+ print('Loaded optimizer')
+ if scheduler is not None and 'scheduler' in checkpoint.keys():
+ scheduler.load_state_dict(checkpoint['scheduler'])
+ print('Loaded scheduler')
+ start_epoch = checkpoint['epoch']
+ print('Last epoch = {}'.format(start_epoch))
+ if 'rank1' in checkpoint.keys():
+ print('Last rank1 = {:.1%}'.format(checkpoint['rank1']))
+ return start_epoch
+
+
+def adjust_learning_rate(
+ optimizer,
+ base_lr,
+ epoch,
+ stepsize=20,
+ gamma=0.1,
+ linear_decay=False,
+ final_lr=0,
+ max_epoch=100
+):
+ r"""Adjusts learning rate.
+
+ Deprecated.
+ """
+ if linear_decay:
+ # linearly decay learning rate from base_lr to final_lr
+ frac_done = epoch / max_epoch
+ lr = frac_done*final_lr + (1.-frac_done) * base_lr
+ else:
+ # decay learning rate by gamma for every stepsize
+ lr = base_lr * (gamma**(epoch // stepsize))
+
+ for param_group in optimizer.param_groups:
+ param_group['lr'] = lr
+
+
+def set_bn_to_eval(m):
+ r"""Sets BatchNorm layers to eval mode."""
+ # 1. no update for running mean and var
+ # 2. scale and shift parameters are still trainable
+ classname = m.__class__.__name__
+ if classname.find('BatchNorm') != -1:
+ m.eval()
+
+
+def open_all_layers(model):
+ r"""Opens all layers in model for training.
+
+ Examples::
+ >>> from torchreid.utils import open_all_layers
+ >>> open_all_layers(model)
+ """
+ model.train()
+ for p in model.parameters():
+ p.requires_grad = True
+
+
+def open_specified_layers(model, open_layers):
+ r"""Opens specified layers in model for training while keeping
+ other layers frozen.
+
+ Args:
+ model (nn.Module): neural net model.
+ open_layers (str or list): layers open for training.
+
+ Examples::
+ >>> from torchreid.utils import open_specified_layers
+ >>> # Only model.classifier will be updated.
+ >>> open_layers = 'classifier'
+ >>> open_specified_layers(model, open_layers)
+ >>> # Only model.fc and model.classifier will be updated.
+ >>> open_layers = ['fc', 'classifier']
+ >>> open_specified_layers(model, open_layers)
+ """
+ if isinstance(model, nn.DataParallel):
+ model = model.module
+
+ if isinstance(open_layers, str):
+ open_layers = [open_layers]
+
+ for layer in open_layers:
+ assert hasattr(
+ model, layer
+ ), '"{}" is not an attribute of the model, please provide the correct name'.format(
+ layer
+ )
+
+ for name, module in model.named_children():
+ if name in open_layers:
+ module.train()
+ for p in module.parameters():
+ p.requires_grad = True
+ else:
+ module.eval()
+ for p in module.parameters():
+ p.requires_grad = False
+
+
+def count_num_param(model):
+ r"""Counts number of parameters in a model while ignoring ``self.classifier``.
+
+ Args:
+ model (nn.Module): network model.
+
+ Examples::
+ >>> from torchreid.utils import count_num_param
+ >>> model_size = count_num_param(model)
+
+ .. warning::
+
+ This method is deprecated in favor of
+ ``torchreid.utils.compute_model_complexity``.
+ """
+ warnings.warn(
+ 'This method is deprecated and will be removed in the future.'
+ )
+
+ num_param = sum(p.numel() for p in model.parameters())
+
+ if isinstance(model, nn.DataParallel):
+ model = model.module
+
+ if hasattr(model,
+ 'classifier') and isinstance(model.classifier, nn.Module):
+ # we ignore the classifier because it is unused at test time
+ num_param -= sum(p.numel() for p in model.classifier.parameters())
+
+ return num_param
+
+
+def load_pretrained_weights(model, weight_path):
+ r"""Loads pretrianed weights to model.
+
+ Features::
+ - Incompatible layers (unmatched in name or size) will be ignored.
+ - Can automatically deal with keys containing "module.".
+
+ Args:
+ model (nn.Module): network model.
+ weight_path (str): path to pretrained weights.
+
+ Examples::
+ >>> from torchreid.utils import load_pretrained_weights
+ >>> weight_path = 'log/my_model/model-best.pth.tar'
+ >>> load_pretrained_weights(model, weight_path)
+ """
+ checkpoint = load_checkpoint(weight_path)
+ if 'state_dict' in checkpoint:
+ state_dict = checkpoint['state_dict']
+ else:
+ state_dict = checkpoint
+
+ model_dict = model.state_dict()
+ new_state_dict = OrderedDict()
+ matched_layers, discarded_layers = [], []
+
+ for k, v in state_dict.items():
+ if k.startswith('module.'):
+ k = k[7:] # discard module.
+
+ if k in model_dict and model_dict[k].size() == v.size():
+ new_state_dict[k] = v
+ matched_layers.append(k)
+ else:
+ discarded_layers.append(k)
+
+ model_dict.update(new_state_dict)
+ model.load_state_dict(model_dict)
+
+ if len(matched_layers) == 0:
+ warnings.warn(
+ 'The pretrained weights "{}" cannot be loaded, '
+ 'please check the key names manually '
+ '(** ignored and continue **)'.format(weight_path)
+ )
+ else:
+ print(
+ 'Successfully loaded pretrained weights from "{}"'.
+ format(weight_path)
+ )
+ if len(discarded_layers) > 0:
+ print(
+ '** The following layers are discarded '
+ 'due to unmatched keys or layer size: {}'.
+ format(discarded_layers)
+ )
diff --git a/trackers/strong_sort/deep/reid_model_factory.py b/trackers/strong_sort/deep/reid_model_factory.py
new file mode 100644
index 0000000000000000000000000000000000000000..ed0542dd6269397c962f3285f3e61b15a7fb1fa4
--- /dev/null
+++ b/trackers/strong_sort/deep/reid_model_factory.py
@@ -0,0 +1,215 @@
+import torch
+from collections import OrderedDict
+
+
+
+__model_types = [
+ 'resnet50', 'mlfn', 'hacnn', 'mobilenetv2_x1_0', 'mobilenetv2_x1_4',
+ 'osnet_x1_0', 'osnet_x0_75', 'osnet_x0_5', 'osnet_x0_25',
+ 'osnet_ibn_x1_0', 'osnet_ain_x1_0']
+
+__trained_urls = {
+
+ # market1501 models ########################################################
+ 'resnet50_market1501.pt':
+ 'https://drive.google.com/uc?id=1dUUZ4rHDWohmsQXCRe2C_HbYkzz94iBV',
+ 'resnet50_dukemtmcreid.pt':
+ 'https://drive.google.com/uc?id=17ymnLglnc64NRvGOitY3BqMRS9UWd1wg',
+ 'resnet50_msmt17.pt':
+ 'https://drive.google.com/uc?id=1ep7RypVDOthCRIAqDnn4_N-UhkkFHJsj',
+
+ 'resnet50_fc512_market1501.pt':
+ 'https://drive.google.com/uc?id=1kv8l5laX_YCdIGVCetjlNdzKIA3NvsSt',
+ 'resnet50_fc512_dukemtmcreid.pt':
+ 'https://drive.google.com/uc?id=13QN8Mp3XH81GK4BPGXobKHKyTGH50Rtx',
+ 'resnet50_fc512_msmt17.pt':
+ 'https://drive.google.com/uc?id=1fDJLcz4O5wxNSUvImIIjoaIF9u1Rwaud',
+
+ 'mlfn_market1501.pt':
+ 'https://drive.google.com/uc?id=1wXcvhA_b1kpDfrt9s2Pma-MHxtj9pmvS',
+ 'mlfn_dukemtmcreid.pt':
+ 'https://drive.google.com/uc?id=1rExgrTNb0VCIcOnXfMsbwSUW1h2L1Bum',
+ 'mlfn_msmt17.pt':
+ 'https://drive.google.com/uc?id=18JzsZlJb3Wm7irCbZbZ07TN4IFKvR6p-',
+
+ 'hacnn_market1501.pt':
+ 'https://drive.google.com/uc?id=1LRKIQduThwGxMDQMiVkTScBwR7WidmYF',
+ 'hacnn_dukemtmcreid.pt':
+ 'https://drive.google.com/uc?id=1zNm6tP4ozFUCUQ7Sv1Z98EAJWXJEhtYH',
+ 'hacnn_msmt17.pt':
+ 'https://drive.google.com/uc?id=1MsKRtPM5WJ3_Tk2xC0aGOO7pM3VaFDNZ',
+
+ 'mobilenetv2_x1_0_market1501.pt':
+ 'https://drive.google.com/uc?id=18DgHC2ZJkjekVoqBWszD8_Xiikz-fewp',
+ 'mobilenetv2_x1_0_dukemtmcreid.pt':
+ 'https://drive.google.com/uc?id=1q1WU2FETRJ3BXcpVtfJUuqq4z3psetds',
+ 'mobilenetv2_x1_0_msmt17.pt':
+ 'https://drive.google.com/uc?id=1j50Hv14NOUAg7ZeB3frzfX-WYLi7SrhZ',
+
+ 'mobilenetv2_x1_4_market1501.pt':
+ 'https://drive.google.com/uc?id=1t6JCqphJG-fwwPVkRLmGGyEBhGOf2GO5',
+ 'mobilenetv2_x1_4_dukemtmcreid.pt':
+ 'https://drive.google.com/uc?id=12uD5FeVqLg9-AFDju2L7SQxjmPb4zpBN',
+ 'mobilenetv2_x1_4_msmt17.pt':
+ 'https://drive.google.com/uc?id=1ZY5P2Zgm-3RbDpbXM0kIBMPvspeNIbXz',
+
+ 'osnet_x1_0_market1501.pt':
+ 'https://drive.google.com/uc?id=1vduhq5DpN2q1g4fYEZfPI17MJeh9qyrA',
+ 'osnet_x1_0_dukemtmcreid.pt':
+ 'https://drive.google.com/uc?id=1QZO_4sNf4hdOKKKzKc-TZU9WW1v6zQbq',
+ 'osnet_x1_0_msmt17.pt':
+ 'https://drive.google.com/uc?id=112EMUfBPYeYg70w-syK6V6Mx8-Qb9Q1M',
+
+ 'osnet_x0_75_market1501.pt':
+ 'https://drive.google.com/uc?id=1ozRaDSQw_EQ8_93OUmjDbvLXw9TnfPer',
+ 'osnet_x0_75_dukemtmcreid.pt':
+ 'https://drive.google.com/uc?id=1IE3KRaTPp4OUa6PGTFL_d5_KQSJbP0Or',
+ 'osnet_x0_75_msmt17.pt':
+ 'https://drive.google.com/uc?id=1QEGO6WnJ-BmUzVPd3q9NoaO_GsPNlmWc',
+
+ 'osnet_x0_5_market1501.pt':
+ 'https://drive.google.com/uc?id=1PLB9rgqrUM7blWrg4QlprCuPT7ILYGKT',
+ 'osnet_x0_5_dukemtmcreid.pt':
+ 'https://drive.google.com/uc?id=1KoUVqmiST175hnkALg9XuTi1oYpqcyTu',
+ 'osnet_x0_5_msmt17.pt':
+ 'https://drive.google.com/uc?id=1UT3AxIaDvS2PdxzZmbkLmjtiqq7AIKCv',
+
+ 'osnet_x0_25_market1501.pt':
+ 'https://drive.google.com/uc?id=1z1UghYvOTtjx7kEoRfmqSMu-z62J6MAj',
+ 'osnet_x0_25_dukemtmcreid.pt':
+ 'https://drive.google.com/uc?id=1eumrtiXT4NOspjyEV4j8cHmlOaaCGk5l',
+ 'osnet_x0_25_msmt17.pt':
+ 'https://drive.google.com/uc?id=1sSwXSUlj4_tHZequ_iZ8w_Jh0VaRQMqF',
+
+ ####### market1501 models ##################################################
+ 'resnet50_msmt17.pt':
+ 'https://drive.google.com/uc?id=1yiBteqgIZoOeywE8AhGmEQl7FTVwrQmf',
+ 'osnet_x1_0_msmt17.pt':
+ 'https://drive.google.com/uc?id=1IosIFlLiulGIjwW3H8uMRmx3MzPwf86x',
+ 'osnet_x0_75_msmt17.pt':
+ 'https://drive.google.com/uc?id=1fhjSS_7SUGCioIf2SWXaRGPqIY9j7-uw',
+
+ 'osnet_x0_5_msmt17.pt':
+ 'https://drive.google.com/uc?id=1DHgmb6XV4fwG3n-CnCM0zdL9nMsZ9_RF',
+ 'osnet_x0_25_msmt17.pt':
+ 'https://drive.google.com/uc?id=1Kkx2zW89jq_NETu4u42CFZTMVD5Hwm6e',
+ 'osnet_ibn_x1_0_msmt17.pt':
+ 'https://drive.google.com/uc?id=1q3Sj2ii34NlfxA4LvmHdWO_75NDRmECJ',
+ 'osnet_ain_x1_0_msmt17.pt':
+ 'https://drive.google.com/uc?id=1SigwBE6mPdqiJMqhuIY4aqC7--5CsMal',
+}
+
+
+def show_downloadeable_models():
+ print('\nAvailable .pt ReID models for automatic download')
+ print(list(__trained_urls.keys()))
+
+
+def get_model_url(model):
+ if model.name in __trained_urls:
+ return __trained_urls[model.name]
+ else:
+ None
+
+
+def is_model_in_model_types(model):
+ if model.name in __model_types:
+ return True
+ else:
+ return False
+
+
+def get_model_name(model):
+ for x in __model_types:
+ if x in model.name:
+ return x
+ return None
+
+
+def download_url(url, dst):
+ """Downloads file from a url to a destination.
+
+ Args:
+ url (str): url to download file.
+ dst (str): destination path.
+ """
+ from six.moves import urllib
+ print('* url="{}"'.format(url))
+ print('* destination="{}"'.format(dst))
+
+ def _reporthook(count, block_size, total_size):
+ global start_time
+ if count == 0:
+ start_time = time.time()
+ return
+ duration = time.time() - start_time
+ progress_size = int(count * block_size)
+ speed = int(progress_size / (1024*duration))
+ percent = int(count * block_size * 100 / total_size)
+ sys.stdout.write(
+ '\r...%d%%, %d MB, %d KB/s, %d seconds passed' %
+ (percent, progress_size / (1024*1024), speed, duration)
+ )
+ sys.stdout.flush()
+
+ urllib.request.urlretrieve(url, dst, _reporthook)
+ sys.stdout.write('\n')
+
+
+def load_pretrained_weights(model, weight_path):
+ r"""Loads pretrianed weights to model.
+
+ Features::
+ - Incompatible layers (unmatched in name or size) will be ignored.
+ - Can automatically deal with keys containing "module.".
+
+ Args:
+ model (nn.Module): network model.
+ weight_path (str): path to pretrained weights.
+
+ Examples::
+ >>> from torchreid.utils import load_pretrained_weights
+ >>> weight_path = 'log/my_model/model-best.pth.tar'
+ >>> load_pretrained_weights(model, weight_path)
+ """
+ checkpoint = torch.load(weight_path)
+ if 'state_dict' in checkpoint:
+ state_dict = checkpoint['state_dict']
+ else:
+ state_dict = checkpoint
+
+ model_dict = model.state_dict()
+ new_state_dict = OrderedDict()
+ matched_layers, discarded_layers = [], []
+
+ for k, v in state_dict.items():
+ if k.startswith('module.'):
+ k = k[7:] # discard module.
+
+ if k in model_dict and model_dict[k].size() == v.size():
+ new_state_dict[k] = v
+ matched_layers.append(k)
+ else:
+ discarded_layers.append(k)
+
+ model_dict.update(new_state_dict)
+ model.load_state_dict(model_dict)
+
+ if len(matched_layers) == 0:
+ warnings.warn(
+ 'The pretrained weights "{}" cannot be loaded, '
+ 'please check the key names manually '
+ '(** ignored and continue **)'.format(weight_path)
+ )
+ else:
+ print(
+ 'Successfully loaded pretrained weights from "{}"'.
+ format(weight_path)
+ )
+ if len(discarded_layers) > 0:
+ print(
+ '** The following layers are discarded '
+ 'due to unmatched keys or layer size: {}'.
+ format(discarded_layers)
+ )
+
diff --git a/trackers/strong_sort/reid_multibackend.py b/trackers/strong_sort/reid_multibackend.py
new file mode 100644
index 0000000000000000000000000000000000000000..8439971e9b5345d803a2695056b536baee9063ad
--- /dev/null
+++ b/trackers/strong_sort/reid_multibackend.py
@@ -0,0 +1,237 @@
+import torch.nn as nn
+import torch
+from pathlib import Path
+import numpy as np
+from itertools import islice
+import torchvision.transforms as transforms
+import cv2
+import sys
+import torchvision.transforms as T
+from collections import OrderedDict, namedtuple
+import gdown
+from os.path import exists as file_exists
+
+from yolov5.utils.general import LOGGER, check_version, check_requirements
+from trackers.strong_sort.deep.reid_model_factory import (show_downloadeable_models, get_model_url, get_model_name,
+ download_url, load_pretrained_weights)
+from trackers.strong_sort.deep.models import build_model
+
+
+def check_suffix(file='yolov5s.pt', suffix=('.pt',), msg=''):
+ # Check file(s) for acceptable suffix
+ if file and suffix:
+ if isinstance(suffix, str):
+ suffix = [suffix]
+ for f in file if isinstance(file, (list, tuple)) else [file]:
+ s = Path(f).suffix.lower() # file suffix
+ if len(s):
+ assert s in suffix, f"{msg}{f} acceptable suffix is {suffix}"
+
+
+class ReIDDetectMultiBackend(nn.Module):
+ # ReID models MultiBackend class for python inference on various backends
+ def __init__(self, weights='osnet_x0_25_msmt17.pt', device=torch.device('cpu'), fp16=False):
+ super().__init__()
+
+ w = weights[0] if isinstance(weights, list) else weights
+ self.pt, self.jit, self.onnx, self.xml, self.engine, self.coreml, self.saved_model, \
+ self.pb, self.tflite, self.edgetpu, self.tfjs, self.paddle = self.model_type(w) # get backend
+ self.fp16 = fp16
+ self.fp16 &= self.pt or self.jit or self.engine # FP16
+
+ # Build transform functions
+ self.device = device
+ self.image_size=(256, 128)
+ self.pixel_mean=[0.485, 0.456, 0.406]
+ self.pixel_std=[0.229, 0.224, 0.225]
+ self.transforms = []
+ self.transforms += [T.Resize(self.image_size)]
+ self.transforms += [T.ToTensor()]
+ self.transforms += [T.Normalize(mean=self.pixel_mean, std=self.pixel_std)]
+ self.preprocess = T.Compose(self.transforms)
+ self.to_pil = T.ToPILImage()
+
+ model_name = get_model_name(w)
+
+ if w.suffix == '.pt':
+ model_url = get_model_url(w)
+ if not file_exists(w) and model_url is not None:
+ gdown.download(model_url, str(w), quiet=False)
+ elif file_exists(w):
+ pass
+ else:
+ print(f'No URL associated to the chosen StrongSORT weights ({w}). Choose between:')
+ show_downloadeable_models()
+ exit()
+
+ # Build model
+ self.model = build_model(
+ model_name,
+ num_classes=1,
+ pretrained=not (w and w.is_file()),
+ use_gpu=device
+ )
+
+ if self.pt: # PyTorch
+ # populate model arch with weights
+ if w and w.is_file() and w.suffix == '.pt':
+ load_pretrained_weights(self.model, w)
+
+ self.model.to(device).eval()
+ self.model.half() if self.fp16 else self.model.float()
+ elif self.jit:
+ LOGGER.info(f'Loading {w} for TorchScript inference...')
+ self.model = torch.jit.load(w)
+ self.model.half() if self.fp16 else self.model.float()
+ elif self.onnx: # ONNX Runtime
+ LOGGER.info(f'Loading {w} for ONNX Runtime inference...')
+ cuda = torch.cuda.is_available() and device.type != 'cpu'
+ #check_requirements(('onnx', 'onnxruntime-gpu' if cuda else 'onnxruntime'))
+ import onnxruntime
+ providers = ['CUDAExecutionProvider', 'CPUExecutionProvider'] if cuda else ['CPUExecutionProvider']
+ self.session = onnxruntime.InferenceSession(str(w), providers=providers)
+ elif self.engine: # TensorRT
+ LOGGER.info(f'Loading {w} for TensorRT inference...')
+ import tensorrt as trt # https://developer.nvidia.com/nvidia-tensorrt-download
+ check_version(trt.__version__, '7.0.0', hard=True) # require tensorrt>=7.0.0
+ if device.type == 'cpu':
+ device = torch.device('cuda:0')
+ Binding = namedtuple('Binding', ('name', 'dtype', 'shape', 'data', 'ptr'))
+ logger = trt.Logger(trt.Logger.INFO)
+ with open(w, 'rb') as f, trt.Runtime(logger) as runtime:
+ self.model_ = runtime.deserialize_cuda_engine(f.read())
+ self.context = self.model_.create_execution_context()
+ self.bindings = OrderedDict()
+ self.fp16 = False # default updated below
+ dynamic = False
+ for index in range(self.model_.num_bindings):
+ name = self.model_.get_binding_name(index)
+ dtype = trt.nptype(self.model_.get_binding_dtype(index))
+ if self.model_.binding_is_input(index):
+ if -1 in tuple(self.model_.get_binding_shape(index)): # dynamic
+ dynamic = True
+ self.context.set_binding_shape(index, tuple(self.model_.get_profile_shape(0, index)[2]))
+ if dtype == np.float16:
+ self.fp16 = True
+ shape = tuple(self.context.get_binding_shape(index))
+ im = torch.from_numpy(np.empty(shape, dtype=dtype)).to(device)
+ self.bindings[name] = Binding(name, dtype, shape, im, int(im.data_ptr()))
+ self.binding_addrs = OrderedDict((n, d.ptr) for n, d in self.bindings.items())
+ batch_size = self.bindings['images'].shape[0] # if dynamic, this is instead max batch size
+ elif self.xml: # OpenVINO
+ LOGGER.info(f'Loading {w} for OpenVINO inference...')
+ check_requirements(('openvino',)) # requires openvino-dev: https://pypi.org/project/openvino-dev/
+ from openvino.runtime import Core, Layout, get_batch
+ ie = Core()
+ if not Path(w).is_file(): # if not *.xml
+ w = next(Path(w).glob('*.xml')) # get *.xml file from *_openvino_model dir
+ network = ie.read_model(model=w, weights=Path(w).with_suffix('.bin'))
+ if network.get_parameters()[0].get_layout().empty:
+ network.get_parameters()[0].set_layout(Layout("NCWH"))
+ batch_dim = get_batch(network)
+ if batch_dim.is_static:
+ batch_size = batch_dim.get_length()
+ self.executable_network = ie.compile_model(network, device_name="CPU") # device_name="MYRIAD" for Intel NCS2
+ self.output_layer = next(iter(self.executable_network.outputs))
+
+ elif self.tflite:
+ LOGGER.info(f'Loading {w} for TensorFlow Lite inference...')
+ try: # https://coral.ai/docs/edgetpu/tflite-python/#update-existing-tf-lite-code-for-the-edge-tpu
+ from tflite_runtime.interpreter import Interpreter, load_delegate
+ except ImportError:
+ import tensorflow as tf
+ Interpreter, load_delegate = tf.lite.Interpreter, tf.lite.experimental.load_delegate,
+ self.interpreter = tf.lite.Interpreter(model_path=w)
+ self.interpreter.allocate_tensors()
+ # Get input and output tensors.
+ self.input_details = self.interpreter.get_input_details()
+ self.output_details = self.interpreter.get_output_details()
+
+ # Test model on random input data.
+ input_data = np.array(np.random.random_sample((1,256,128,3)), dtype=np.float32)
+ self.interpreter.set_tensor(self.input_details[0]['index'], input_data)
+
+ self.interpreter.invoke()
+
+ # The function `get_tensor()` returns a copy of the tensor data.
+ output_data = self.interpreter.get_tensor(self.output_details[0]['index'])
+ else:
+ print('This model framework is not supported yet!')
+ exit()
+
+
+ @staticmethod
+ def model_type(p='path/to/model.pt'):
+ # Return model type from model path, i.e. path='path/to/model.onnx' -> type=onnx
+ from export import export_formats
+ sf = list(export_formats().Suffix) # export suffixes
+ check_suffix(p, sf) # checks
+ types = [s in Path(p).name for s in sf]
+ types[8] &= not types[9] # tflite &= not edgetpu
+ return types
+
+ def _preprocess(self, im_batch):
+
+ images = []
+ for element in im_batch:
+ image = self.to_pil(element)
+ image = self.preprocess(image)
+ images.append(image)
+
+ images = torch.stack(images, dim=0)
+ images = images.to(self.device)
+
+ return images
+
+
+ def forward(self, im_batch):
+
+ # preprocess batch
+ im_batch = self._preprocess(im_batch)
+
+ # batch to half
+ if self.fp16 and im_batch.dtype != torch.float16:
+ im_batch = im_batch.half()
+
+ # batch processing
+ features = []
+ if self.pt:
+ features = self.model(im_batch)
+ elif self.jit: # TorchScript
+ features = self.model(im_batch)
+ elif self.onnx: # ONNX Runtime
+ im_batch = im_batch.cpu().numpy() # torch to numpy
+ features = self.session.run([self.session.get_outputs()[0].name], {self.session.get_inputs()[0].name: im_batch})[0]
+ elif self.engine: # TensorRT
+ if True and im_batch.shape != self.bindings['images'].shape:
+ i_in, i_out = (self.model_.get_binding_index(x) for x in ('images', 'output'))
+ self.context.set_binding_shape(i_in, im_batch.shape) # reshape if dynamic
+ self.bindings['images'] = self.bindings['images']._replace(shape=im_batch.shape)
+ self.bindings['output'].data.resize_(tuple(self.context.get_binding_shape(i_out)))
+ s = self.bindings['images'].shape
+ assert im_batch.shape == s, f"input size {im_batch.shape} {'>' if self.dynamic else 'not equal to'} max model size {s}"
+ self.binding_addrs['images'] = int(im_batch.data_ptr())
+ self.context.execute_v2(list(self.binding_addrs.values()))
+ features = self.bindings['output'].data
+ elif self.xml: # OpenVINO
+ im_batch = im_batch.cpu().numpy() # FP32
+ features = self.executable_network([im_batch])[self.output_layer]
+ else:
+ print('Framework not supported at the moment, we are working on it...')
+ exit()
+
+ if isinstance(features, (list, tuple)):
+ return self.from_numpy(features[0]) if len(features) == 1 else [self.from_numpy(x) for x in features]
+ else:
+ return self.from_numpy(features)
+
+ def from_numpy(self, x):
+ return torch.from_numpy(x).to(self.device) if isinstance(x, np.ndarray) else x
+
+ def warmup(self, imgsz=[(256, 128, 3)]):
+ # Warmup model by running inference once
+ warmup_types = self.pt, self.jit, self.onnx, self.engine, self.saved_model, self.pb
+ if any(warmup_types) and self.device.type != 'cpu':
+ im = [np.empty(*imgsz).astype(np.uint8)] # input
+ for _ in range(2 if self.jit else 1): #
+ self.forward(im) # warmup
\ No newline at end of file
diff --git a/trackers/strong_sort/sort/__init__.py b/trackers/strong_sort/sort/__init__.py
new file mode 100644
index 0000000000000000000000000000000000000000..e69de29bb2d1d6434b8b29ae775ad8c2e48c5391
diff --git a/trackers/strong_sort/sort/__pycache__/__init__.cpython-38.pyc b/trackers/strong_sort/sort/__pycache__/__init__.cpython-38.pyc
new file mode 100644
index 0000000000000000000000000000000000000000..7ae39dd88e0e5fb991e8b13cb1be8003203f151f
Binary files /dev/null and b/trackers/strong_sort/sort/__pycache__/__init__.cpython-38.pyc differ
diff --git a/trackers/strong_sort/sort/__pycache__/__init__.cpython-39.pyc b/trackers/strong_sort/sort/__pycache__/__init__.cpython-39.pyc
new file mode 100644
index 0000000000000000000000000000000000000000..5b2ff7e72b9e3fcd1361a2102c9ad4f84ee5de9d
Binary files /dev/null and b/trackers/strong_sort/sort/__pycache__/__init__.cpython-39.pyc differ
diff --git a/trackers/strong_sort/sort/__pycache__/detection.cpython-38.pyc b/trackers/strong_sort/sort/__pycache__/detection.cpython-38.pyc
new file mode 100644
index 0000000000000000000000000000000000000000..ab5e8ba335d94f870844f5ee6ce62c94b938fb8c
Binary files /dev/null and b/trackers/strong_sort/sort/__pycache__/detection.cpython-38.pyc differ
diff --git a/trackers/strong_sort/sort/__pycache__/detection.cpython-39.pyc b/trackers/strong_sort/sort/__pycache__/detection.cpython-39.pyc
new file mode 100644
index 0000000000000000000000000000000000000000..e25123863cbd1daf1d749dca220a0b0b853fcdbf
Binary files /dev/null and b/trackers/strong_sort/sort/__pycache__/detection.cpython-39.pyc differ
diff --git a/trackers/strong_sort/sort/__pycache__/iou_matching.cpython-38.pyc b/trackers/strong_sort/sort/__pycache__/iou_matching.cpython-38.pyc
new file mode 100644
index 0000000000000000000000000000000000000000..ddca35d7dd1cdcbb2406487637eb8cf61890b82c
Binary files /dev/null and b/trackers/strong_sort/sort/__pycache__/iou_matching.cpython-38.pyc differ
diff --git a/trackers/strong_sort/sort/__pycache__/iou_matching.cpython-39.pyc b/trackers/strong_sort/sort/__pycache__/iou_matching.cpython-39.pyc
new file mode 100644
index 0000000000000000000000000000000000000000..7380c7e06825c8e0864bed735b226825a9cf191a
Binary files /dev/null and b/trackers/strong_sort/sort/__pycache__/iou_matching.cpython-39.pyc differ
diff --git a/trackers/strong_sort/sort/__pycache__/kalman_filter.cpython-38.pyc b/trackers/strong_sort/sort/__pycache__/kalman_filter.cpython-38.pyc
new file mode 100644
index 0000000000000000000000000000000000000000..74272c1d8f30546f7136e18a09ca002a7d5181e8
Binary files /dev/null and b/trackers/strong_sort/sort/__pycache__/kalman_filter.cpython-38.pyc differ
diff --git a/trackers/strong_sort/sort/__pycache__/kalman_filter.cpython-39.pyc b/trackers/strong_sort/sort/__pycache__/kalman_filter.cpython-39.pyc
new file mode 100644
index 0000000000000000000000000000000000000000..8a585b1dfb0bde830dd599de61b9d043aed049b9
Binary files /dev/null and b/trackers/strong_sort/sort/__pycache__/kalman_filter.cpython-39.pyc differ
diff --git a/trackers/strong_sort/sort/__pycache__/linear_assignment.cpython-38.pyc b/trackers/strong_sort/sort/__pycache__/linear_assignment.cpython-38.pyc
new file mode 100644
index 0000000000000000000000000000000000000000..b5cce4b6834f554ae58efaf77207c024109fb4fe
Binary files /dev/null and b/trackers/strong_sort/sort/__pycache__/linear_assignment.cpython-38.pyc differ
diff --git a/trackers/strong_sort/sort/__pycache__/linear_assignment.cpython-39.pyc b/trackers/strong_sort/sort/__pycache__/linear_assignment.cpython-39.pyc
new file mode 100644
index 0000000000000000000000000000000000000000..3b4cda18025b190901dff02758827ac7846295f4
Binary files /dev/null and b/trackers/strong_sort/sort/__pycache__/linear_assignment.cpython-39.pyc differ
diff --git a/trackers/strong_sort/sort/__pycache__/nn_matching.cpython-38.pyc b/trackers/strong_sort/sort/__pycache__/nn_matching.cpython-38.pyc
new file mode 100644
index 0000000000000000000000000000000000000000..46e01296e481e5d596de8dec5b6e1be6027a6200
Binary files /dev/null and b/trackers/strong_sort/sort/__pycache__/nn_matching.cpython-38.pyc differ
diff --git a/trackers/strong_sort/sort/__pycache__/nn_matching.cpython-39.pyc b/trackers/strong_sort/sort/__pycache__/nn_matching.cpython-39.pyc
new file mode 100644
index 0000000000000000000000000000000000000000..a0aac7b4f959bd2b6e7b4987a1505493fc150919
Binary files /dev/null and b/trackers/strong_sort/sort/__pycache__/nn_matching.cpython-39.pyc differ
diff --git a/trackers/strong_sort/sort/__pycache__/track.cpython-38.pyc b/trackers/strong_sort/sort/__pycache__/track.cpython-38.pyc
new file mode 100644
index 0000000000000000000000000000000000000000..6444bd9fd1118691d69d8870064362b216d46f05
Binary files /dev/null and b/trackers/strong_sort/sort/__pycache__/track.cpython-38.pyc differ
diff --git a/trackers/strong_sort/sort/__pycache__/track.cpython-39.pyc b/trackers/strong_sort/sort/__pycache__/track.cpython-39.pyc
new file mode 100644
index 0000000000000000000000000000000000000000..637c0798c40b10de973a39996714de34f5ef0f31
Binary files /dev/null and b/trackers/strong_sort/sort/__pycache__/track.cpython-39.pyc differ
diff --git a/trackers/strong_sort/sort/__pycache__/tracker.cpython-38.pyc b/trackers/strong_sort/sort/__pycache__/tracker.cpython-38.pyc
new file mode 100644
index 0000000000000000000000000000000000000000..a0cb8c6c303ad58be545175b580beab1e32f1176
Binary files /dev/null and b/trackers/strong_sort/sort/__pycache__/tracker.cpython-38.pyc differ
diff --git a/trackers/strong_sort/sort/__pycache__/tracker.cpython-39.pyc b/trackers/strong_sort/sort/__pycache__/tracker.cpython-39.pyc
new file mode 100644
index 0000000000000000000000000000000000000000..dc1cc649d50a339ae97c4942f4659d6f3d17f6d4
Binary files /dev/null and b/trackers/strong_sort/sort/__pycache__/tracker.cpython-39.pyc differ
diff --git a/trackers/strong_sort/sort/detection.py b/trackers/strong_sort/sort/detection.py
new file mode 100644
index 0000000000000000000000000000000000000000..22fbd603a65341569ed70a82a079fa532d3dae6f
--- /dev/null
+++ b/trackers/strong_sort/sort/detection.py
@@ -0,0 +1,49 @@
+# vim: expandtab:ts=4:sw=4
+import numpy as np
+
+
+class Detection(object):
+ """
+ This class represents a bounding box detection in a single image.
+
+ Parameters
+ ----------
+ tlwh : array_like
+ Bounding box in format `(x, y, w, h)`.
+ confidence : float
+ Detector confidence score.
+ feature : array_like
+ A feature vector that describes the object contained in this image.
+
+ Attributes
+ ----------
+ tlwh : ndarray
+ Bounding box in format `(top left x, top left y, width, height)`.
+ confidence : ndarray
+ Detector confidence score.
+ feature : ndarray | NoneType
+ A feature vector that describes the object contained in this image.
+
+ """
+
+ def __init__(self, tlwh, confidence, feature):
+ self.tlwh = np.asarray(tlwh, dtype=np.float)
+ self.confidence = float(confidence)
+ self.feature = np.asarray(feature.cpu(), dtype=np.float32)
+
+ def to_tlbr(self):
+ """Convert bounding box to format `(min x, min y, max x, max y)`, i.e.,
+ `(top left, bottom right)`.
+ """
+ ret = self.tlwh.copy()
+ ret[2:] += ret[:2]
+ return ret
+
+ def to_xyah(self):
+ """Convert bounding box to format `(center x, center y, aspect ratio,
+ height)`, where the aspect ratio is `width / height`.
+ """
+ ret = self.tlwh.copy()
+ ret[:2] += ret[2:] / 2
+ ret[2] /= ret[3]
+ return ret
diff --git a/trackers/strong_sort/sort/iou_matching.py b/trackers/strong_sort/sort/iou_matching.py
new file mode 100644
index 0000000000000000000000000000000000000000..62d5a3f63b70db5e322b6f8766444dd824c010ae
--- /dev/null
+++ b/trackers/strong_sort/sort/iou_matching.py
@@ -0,0 +1,82 @@
+# vim: expandtab:ts=4:sw=4
+from __future__ import absolute_import
+import numpy as np
+from . import linear_assignment
+
+
+def iou(bbox, candidates):
+ """Computer intersection over union.
+
+ Parameters
+ ----------
+ bbox : ndarray
+ A bounding box in format `(top left x, top left y, width, height)`.
+ candidates : ndarray
+ A matrix of candidate bounding boxes (one per row) in the same format
+ as `bbox`.
+
+ Returns
+ -------
+ ndarray
+ The intersection over union in [0, 1] between the `bbox` and each
+ candidate. A higher score means a larger fraction of the `bbox` is
+ occluded by the candidate.
+
+ """
+ bbox_tl, bbox_br = bbox[:2], bbox[:2] + bbox[2:]
+ candidates_tl = candidates[:, :2]
+ candidates_br = candidates[:, :2] + candidates[:, 2:]
+
+ tl = np.c_[np.maximum(bbox_tl[0], candidates_tl[:, 0])[:, np.newaxis],
+ np.maximum(bbox_tl[1], candidates_tl[:, 1])[:, np.newaxis]]
+ br = np.c_[np.minimum(bbox_br[0], candidates_br[:, 0])[:, np.newaxis],
+ np.minimum(bbox_br[1], candidates_br[:, 1])[:, np.newaxis]]
+ wh = np.maximum(0., br - tl)
+
+ area_intersection = wh.prod(axis=1)
+ area_bbox = bbox[2:].prod()
+ area_candidates = candidates[:, 2:].prod(axis=1)
+ return area_intersection / (area_bbox + area_candidates - area_intersection)
+
+
+def iou_cost(tracks, detections, track_indices=None,
+ detection_indices=None):
+ """An intersection over union distance metric.
+
+ Parameters
+ ----------
+ tracks : List[deep_sort.track.Track]
+ A list of tracks.
+ detections : List[deep_sort.detection.Detection]
+ A list of detections.
+ track_indices : Optional[List[int]]
+ A list of indices to tracks that should be matched. Defaults to
+ all `tracks`.
+ detection_indices : Optional[List[int]]
+ A list of indices to detections that should be matched. Defaults
+ to all `detections`.
+
+ Returns
+ -------
+ ndarray
+ Returns a cost matrix of shape
+ len(track_indices), len(detection_indices) where entry (i, j) is
+ `1 - iou(tracks[track_indices[i]], detections[detection_indices[j]])`.
+
+ """
+ if track_indices is None:
+ track_indices = np.arange(len(tracks))
+ if detection_indices is None:
+ detection_indices = np.arange(len(detections))
+
+ cost_matrix = np.zeros((len(track_indices), len(detection_indices)))
+ for row, track_idx in enumerate(track_indices):
+ if tracks[track_idx].time_since_update > 1:
+ cost_matrix[row, :] = linear_assignment.INFTY_COST
+ continue
+
+ bbox = tracks[track_idx].to_tlwh()
+ candidates = np.asarray(
+ [detections[i].tlwh for i in detection_indices])
+ cost_matrix[row, :] = 1. - iou(bbox, candidates)
+ return cost_matrix
diff --git a/trackers/strong_sort/sort/kalman_filter.py b/trackers/strong_sort/sort/kalman_filter.py
new file mode 100644
index 0000000000000000000000000000000000000000..87c48d7e332bef5f8feab8abf7936409abbf5d03
--- /dev/null
+++ b/trackers/strong_sort/sort/kalman_filter.py
@@ -0,0 +1,214 @@
+# vim: expandtab:ts=4:sw=4
+import numpy as np
+import scipy.linalg
+"""
+Table for the 0.95 quantile of the chi-square distribution with N degrees of
+freedom (contains values for N=1, ..., 9). Taken from MATLAB/Octave's chi2inv
+function and used as Mahalanobis gating threshold.
+"""
+chi2inv95 = {
+ 1: 3.8415,
+ 2: 5.9915,
+ 3: 7.8147,
+ 4: 9.4877,
+ 5: 11.070,
+ 6: 12.592,
+ 7: 14.067,
+ 8: 15.507,
+ 9: 16.919}
+
+
+class KalmanFilter(object):
+ """
+ A simple Kalman filter for tracking bounding boxes in image space.
+ The 8-dimensional state space
+ x, y, a, h, vx, vy, va, vh
+ contains the bounding box center position (x, y), aspect ratio a, height h,
+ and their respective velocities.
+ Object motion follows a constant velocity model. The bounding box location
+ (x, y, a, h) is taken as direct observation of the state space (linear
+ observation model).
+ """
+
+ def __init__(self):
+ ndim, dt = 4, 1.
+
+ # Create Kalman filter model matrices.
+ self._motion_mat = np.eye(2 * ndim, 2 * ndim)
+ for i in range(ndim):
+ self._motion_mat[i, ndim + i] = dt
+
+ self._update_mat = np.eye(ndim, 2 * ndim)
+
+ # Motion and observation uncertainty are chosen relative to the current
+ # state estimate. These weights control the amount of uncertainty in
+ # the model. This is a bit hacky.
+ self._std_weight_position = 1. / 20
+ self._std_weight_velocity = 1. / 160
+
+ def initiate(self, measurement):
+ """Create track from unassociated measurement.
+ Parameters
+ ----------
+ measurement : ndarray
+ Bounding box coordinates (x, y, a, h) with center position (x, y),
+ aspect ratio a, and height h.
+ Returns
+ -------
+ (ndarray, ndarray)
+ Returns the mean vector (8 dimensional) and covariance matrix (8x8
+ dimensional) of the new track. Unobserved velocities are initialized
+ to 0 mean.
+ """
+ mean_pos = measurement
+ mean_vel = np.zeros_like(mean_pos)
+ mean = np.r_[mean_pos, mean_vel]
+
+ std = [
+ 2 * self._std_weight_position * measurement[0], # the center point x
+ 2 * self._std_weight_position * measurement[1], # the center point y
+ 1 * measurement[2], # the ratio of width/height
+ 2 * self._std_weight_position * measurement[3], # the height
+ 10 * self._std_weight_velocity * measurement[0],
+ 10 * self._std_weight_velocity * measurement[1],
+ 0.1 * measurement[2],
+ 10 * self._std_weight_velocity * measurement[3]]
+ covariance = np.diag(np.square(std))
+ return mean, covariance
+
+ def predict(self, mean, covariance):
+ """Run Kalman filter prediction step.
+ Parameters
+ ----------
+ mean : ndarray
+ The 8 dimensional mean vector of the object state at the previous
+ time step.
+ covariance : ndarray
+ The 8x8 dimensional covariance matrix of the object state at the
+ previous time step.
+ Returns
+ -------
+ (ndarray, ndarray)
+ Returns the mean vector and covariance matrix of the predicted
+ state. Unobserved velocities are initialized to 0 mean.
+ """
+ std_pos = [
+ self._std_weight_position * mean[0],
+ self._std_weight_position * mean[1],
+ 1 * mean[2],
+ self._std_weight_position * mean[3]]
+ std_vel = [
+ self._std_weight_velocity * mean[0],
+ self._std_weight_velocity * mean[1],
+ 0.1 * mean[2],
+ self._std_weight_velocity * mean[3]]
+ motion_cov = np.diag(np.square(np.r_[std_pos, std_vel]))
+
+ mean = np.dot(self._motion_mat, mean)
+ covariance = np.linalg.multi_dot((
+ self._motion_mat, covariance, self._motion_mat.T)) + motion_cov
+
+ return mean, covariance
+
+ def project(self, mean, covariance, confidence=.0):
+ """Project state distribution to measurement space.
+ Parameters
+ ----------
+ mean : ndarray
+ The state's mean vector (8 dimensional array).
+ covariance : ndarray
+ The state's covariance matrix (8x8 dimensional).
+ confidence: (dyh) 检测框置信度
+ Returns
+ -------
+ (ndarray, ndarray)
+ Returns the projected mean and covariance matrix of the given state
+ estimate.
+ """
+ std = [
+ self._std_weight_position * mean[3],
+ self._std_weight_position * mean[3],
+ 1e-1,
+ self._std_weight_position * mean[3]]
+
+
+ std = [(1 - confidence) * x for x in std]
+
+ innovation_cov = np.diag(np.square(std))
+
+ mean = np.dot(self._update_mat, mean)
+ covariance = np.linalg.multi_dot((
+ self._update_mat, covariance, self._update_mat.T))
+ return mean, covariance + innovation_cov
+
+ def update(self, mean, covariance, measurement, confidence=.0):
+ """Run Kalman filter correction step.
+ Parameters
+ ----------
+ mean : ndarray
+ The predicted state's mean vector (8 dimensional).
+ covariance : ndarray
+ The state's covariance matrix (8x8 dimensional).
+ measurement : ndarray
+ The 4 dimensional measurement vector (x, y, a, h), where (x, y)
+ is the center position, a the aspect ratio, and h the height of the
+ bounding box.
+ confidence: (dyh)检测框置信度
+ Returns
+ -------
+ (ndarray, ndarray)
+ Returns the measurement-corrected state distribution.
+ """
+ projected_mean, projected_cov = self.project(mean, covariance, confidence)
+
+ chol_factor, lower = scipy.linalg.cho_factor(
+ projected_cov, lower=True, check_finite=False)
+ kalman_gain = scipy.linalg.cho_solve(
+ (chol_factor, lower), np.dot(covariance, self._update_mat.T).T,
+ check_finite=False).T
+ innovation = measurement - projected_mean
+
+ new_mean = mean + np.dot(innovation, kalman_gain.T)
+ new_covariance = covariance - np.linalg.multi_dot((
+ kalman_gain, projected_cov, kalman_gain.T))
+ return new_mean, new_covariance
+
+ def gating_distance(self, mean, covariance, measurements,
+ only_position=False):
+ """Compute gating distance between state distribution and measurements.
+ A suitable distance threshold can be obtained from `chi2inv95`. If
+ `only_position` is False, the chi-square distribution has 4 degrees of
+ freedom, otherwise 2.
+ Parameters
+ ----------
+ mean : ndarray
+ Mean vector over the state distribution (8 dimensional).
+ covariance : ndarray
+ Covariance of the state distribution (8x8 dimensional).
+ measurements : ndarray
+ An Nx4 dimensional matrix of N measurements, each in
+ format (x, y, a, h) where (x, y) is the bounding box center
+ position, a the aspect ratio, and h the height.
+ only_position : Optional[bool]
+ If True, distance computation is done with respect to the bounding
+ box center position only.
+ Returns
+ -------
+ ndarray
+ Returns an array of length N, where the i-th element contains the
+ squared Mahalanobis distance between (mean, covariance) and
+ `measurements[i]`.
+ """
+ mean, covariance = self.project(mean, covariance)
+
+ if only_position:
+ mean, covariance = mean[:2], covariance[:2, :2]
+ measurements = measurements[:, :2]
+
+ cholesky_factor = np.linalg.cholesky(covariance)
+ d = measurements - mean
+ z = scipy.linalg.solve_triangular(
+ cholesky_factor, d.T, lower=True, check_finite=False,
+ overwrite_b=True)
+ squared_maha = np.sum(z * z, axis=0)
+ return squared_maha
\ No newline at end of file
diff --git a/trackers/strong_sort/sort/linear_assignment.py b/trackers/strong_sort/sort/linear_assignment.py
new file mode 100644
index 0000000000000000000000000000000000000000..924895fd3a0b8243a6eb691624883feccaa937cc
--- /dev/null
+++ b/trackers/strong_sort/sort/linear_assignment.py
@@ -0,0 +1,174 @@
+# vim: expandtab:ts=4:sw=4
+from __future__ import absolute_import
+import numpy as np
+from scipy.optimize import linear_sum_assignment
+from . import kalman_filter
+
+
+INFTY_COST = 1e+5
+
+
+def min_cost_matching(
+ distance_metric, max_distance, tracks, detections, track_indices=None,
+ detection_indices=None):
+ """Solve linear assignment problem.
+ Parameters
+ ----------
+ distance_metric : Callable[List[Track], List[Detection], List[int], List[int]) -> ndarray
+ The distance metric is given a list of tracks and detections as well as
+ a list of N track indices and M detection indices. The metric should
+ return the NxM dimensional cost matrix, where element (i, j) is the
+ association cost between the i-th track in the given track indices and
+ the j-th detection in the given detection_indices.
+ max_distance : float
+ Gating threshold. Associations with cost larger than this value are
+ disregarded.
+ tracks : List[track.Track]
+ A list of predicted tracks at the current time step.
+ detections : List[detection.Detection]
+ A list of detections at the current time step.
+ track_indices : List[int]
+ List of track indices that maps rows in `cost_matrix` to tracks in
+ `tracks` (see description above).
+ detection_indices : List[int]
+ List of detection indices that maps columns in `cost_matrix` to
+ detections in `detections` (see description above).
+ Returns
+ -------
+ (List[(int, int)], List[int], List[int])
+ Returns a tuple with the following three entries:
+ * A list of matched track and detection indices.
+ * A list of unmatched track indices.
+ * A list of unmatched detection indices.
+ """
+ if track_indices is None:
+ track_indices = np.arange(len(tracks))
+ if detection_indices is None:
+ detection_indices = np.arange(len(detections))
+
+ if len(detection_indices) == 0 or len(track_indices) == 0:
+ return [], track_indices, detection_indices # Nothing to match.
+
+ cost_matrix = distance_metric(
+ tracks, detections, track_indices, detection_indices)
+ cost_matrix[cost_matrix > max_distance] = max_distance + 1e-5
+ row_indices, col_indices = linear_sum_assignment(cost_matrix)
+
+ matches, unmatched_tracks, unmatched_detections = [], [], []
+ for col, detection_idx in enumerate(detection_indices):
+ if col not in col_indices:
+ unmatched_detections.append(detection_idx)
+ for row, track_idx in enumerate(track_indices):
+ if row not in row_indices:
+ unmatched_tracks.append(track_idx)
+ for row, col in zip(row_indices, col_indices):
+ track_idx = track_indices[row]
+ detection_idx = detection_indices[col]
+ if cost_matrix[row, col] > max_distance:
+ unmatched_tracks.append(track_idx)
+ unmatched_detections.append(detection_idx)
+ else:
+ matches.append((track_idx, detection_idx))
+ return matches, unmatched_tracks, unmatched_detections
+
+
+def matching_cascade(
+ distance_metric, max_distance, cascade_depth, tracks, detections,
+ track_indices=None, detection_indices=None):
+ """Run matching cascade.
+ Parameters
+ ----------
+ distance_metric : Callable[List[Track], List[Detection], List[int], List[int]) -> ndarray
+ The distance metric is given a list of tracks and detections as well as
+ a list of N track indices and M detection indices. The metric should
+ return the NxM dimensional cost matrix, where element (i, j) is the
+ association cost between the i-th track in the given track indices and
+ the j-th detection in the given detection indices.
+ max_distance : float
+ Gating threshold. Associations with cost larger than this value are
+ disregarded.
+ cascade_depth: int
+ The cascade depth, should be se to the maximum track age.
+ tracks : List[track.Track]
+ A list of predicted tracks at the current time step.
+ detections : List[detection.Detection]
+ A list of detections at the current time step.
+ track_indices : Optional[List[int]]
+ List of track indices that maps rows in `cost_matrix` to tracks in
+ `tracks` (see description above). Defaults to all tracks.
+ detection_indices : Optional[List[int]]
+ List of detection indices that maps columns in `cost_matrix` to
+ detections in `detections` (see description above). Defaults to all
+ detections.
+ Returns
+ -------
+ (List[(int, int)], List[int], List[int])
+ Returns a tuple with the following three entries:
+ * A list of matched track and detection indices.
+ * A list of unmatched track indices.
+ * A list of unmatched detection indices.
+ """
+ if track_indices is None:
+ track_indices = list(range(len(tracks)))
+ if detection_indices is None:
+ detection_indices = list(range(len(detections)))
+
+ unmatched_detections = detection_indices
+ matches = []
+ track_indices_l = [
+ k for k in track_indices
+ # if tracks[k].time_since_update == 1 + level
+ ]
+ matches_l, _, unmatched_detections = \
+ min_cost_matching(
+ distance_metric, max_distance, tracks, detections,
+ track_indices_l, unmatched_detections)
+ matches += matches_l
+ unmatched_tracks = list(set(track_indices) - set(k for k, _ in matches))
+ return matches, unmatched_tracks, unmatched_detections
+
+
+def gate_cost_matrix(
+ cost_matrix, tracks, detections, track_indices, detection_indices,
+ gated_cost=INFTY_COST, only_position=False):
+ """Invalidate infeasible entries in cost matrix based on the state
+ distributions obtained by Kalman filtering.
+ Parameters
+ ----------
+ kf : The Kalman filter.
+ cost_matrix : ndarray
+ The NxM dimensional cost matrix, where N is the number of track indices
+ and M is the number of detection indices, such that entry (i, j) is the
+ association cost between `tracks[track_indices[i]]` and
+ `detections[detection_indices[j]]`.
+ tracks : List[track.Track]
+ A list of predicted tracks at the current time step.
+ detections : List[detection.Detection]
+ A list of detections at the current time step.
+ track_indices : List[int]
+ List of track indices that maps rows in `cost_matrix` to tracks in
+ `tracks` (see description above).
+ detection_indices : List[int]
+ List of detection indices that maps columns in `cost_matrix` to
+ detections in `detections` (see description above).
+ gated_cost : Optional[float]
+ Entries in the cost matrix corresponding to infeasible associations are
+ set this value. Defaults to a very large value.
+ only_position : Optional[bool]
+ If True, only the x, y position of the state distribution is considered
+ during gating. Defaults to False.
+ Returns
+ -------
+ ndarray
+ Returns the modified cost matrix.
+ """
+ gating_dim = 2 if only_position else 4
+ gating_threshold = kalman_filter.chi2inv95[gating_dim]
+ measurements = np.asarray(
+ [detections[i].to_xyah() for i in detection_indices])
+ for row, track_idx in enumerate(track_indices):
+ track = tracks[track_idx]
+ gating_distance = track.kf.gating_distance(track.mean, track.covariance, measurements, only_position)
+ cost_matrix[row, gating_distance > gating_threshold] = gated_cost
+ cost_matrix[row] = 0.995 * cost_matrix[row] + (1 - 0.995) * gating_distance
+ return cost_matrix
\ No newline at end of file
diff --git a/trackers/strong_sort/sort/nn_matching.py b/trackers/strong_sort/sort/nn_matching.py
new file mode 100644
index 0000000000000000000000000000000000000000..154f8546c3fb1ac30b46b0fe405bbffce1b99ce0
--- /dev/null
+++ b/trackers/strong_sort/sort/nn_matching.py
@@ -0,0 +1,162 @@
+# vim: expandtab:ts=4:sw=4
+import numpy as np
+import sys
+import torch
+
+
+def _pdist(a, b):
+ """Compute pair-wise squared distance between points in `a` and `b`.
+ Parameters
+ ----------
+ a : array_like
+ An NxM matrix of N samples of dimensionality M.
+ b : array_like
+ An LxM matrix of L samples of dimensionality M.
+ Returns
+ -------
+ ndarray
+ Returns a matrix of size len(a), len(b) such that eleement (i, j)
+ contains the squared distance between `a[i]` and `b[j]`.
+ """
+ a, b = np.asarray(a), np.asarray(b)
+ if len(a) == 0 or len(b) == 0:
+ return np.zeros((len(a), len(b)))
+ a2, b2 = np.square(a).sum(axis=1), np.square(b).sum(axis=1)
+ r2 = -2. * np.dot(a, b.T) + a2[:, None] + b2[None, :]
+ r2 = np.clip(r2, 0., float(np.inf))
+ return r2
+
+
+def _cosine_distance(a, b, data_is_normalized=False):
+ """Compute pair-wise cosine distance between points in `a` and `b`.
+ Parameters
+ ----------
+ a : array_like
+ An NxM matrix of N samples of dimensionality M.
+ b : array_like
+ An LxM matrix of L samples of dimensionality M.
+ data_is_normalized : Optional[bool]
+ If True, assumes rows in a and b are unit length vectors.
+ Otherwise, a and b are explicitly normalized to lenght 1.
+ Returns
+ -------
+ ndarray
+ Returns a matrix of size len(a), len(b) such that eleement (i, j)
+ contains the squared distance between `a[i]` and `b[j]`.
+ """
+ if not data_is_normalized:
+ a = np.asarray(a) / np.linalg.norm(a, axis=1, keepdims=True)
+ b = np.asarray(b) / np.linalg.norm(b, axis=1, keepdims=True)
+ return 1. - np.dot(a, b.T)
+
+
+def _nn_euclidean_distance(x, y):
+ """ Helper function for nearest neighbor distance metric (Euclidean).
+ Parameters
+ ----------
+ x : ndarray
+ A matrix of N row-vectors (sample points).
+ y : ndarray
+ A matrix of M row-vectors (query points).
+ Returns
+ -------
+ ndarray
+ A vector of length M that contains for each entry in `y` the
+ smallest Euclidean distance to a sample in `x`.
+ """
+ # x_ = torch.from_numpy(np.asarray(x) / np.linalg.norm(x, axis=1, keepdims=True))
+ # y_ = torch.from_numpy(np.asarray(y) / np.linalg.norm(y, axis=1, keepdims=True))
+ distances = distances = _pdist(x, y)
+ return np.maximum(0.0, torch.min(distances, axis=0)[0].numpy())
+
+
+def _nn_cosine_distance(x, y):
+ """ Helper function for nearest neighbor distance metric (cosine).
+ Parameters
+ ----------
+ x : ndarray
+ A matrix of N row-vectors (sample points).
+ y : ndarray
+ A matrix of M row-vectors (query points).
+ Returns
+ -------
+ ndarray
+ A vector of length M that contains for each entry in `y` the
+ smallest cosine distance to a sample in `x`.
+ """
+ x_ = torch.from_numpy(np.asarray(x))
+ y_ = torch.from_numpy(np.asarray(y))
+ distances = _cosine_distance(x_, y_)
+ distances = distances
+ return distances.min(axis=0)
+
+
+class NearestNeighborDistanceMetric(object):
+ """
+ A nearest neighbor distance metric that, for each target, returns
+ the closest distance to any sample that has been observed so far.
+ Parameters
+ ----------
+ metric : str
+ Either "euclidean" or "cosine".
+ matching_threshold: float
+ The matching threshold. Samples with larger distance are considered an
+ invalid match.
+ budget : Optional[int]
+ If not None, fix samples per class to at most this number. Removes
+ the oldest samples when the budget is reached.
+ Attributes
+ ----------
+ samples : Dict[int -> List[ndarray]]
+ A dictionary that maps from target identities to the list of samples
+ that have been observed so far.
+ """
+
+ def __init__(self, metric, matching_threshold, budget=None):
+ if metric == "euclidean":
+ self._metric = _nn_euclidean_distance
+ elif metric == "cosine":
+ self._metric = _nn_cosine_distance
+ else:
+ raise ValueError(
+ "Invalid metric; must be either 'euclidean' or 'cosine'")
+ self.matching_threshold = matching_threshold
+ self.budget = budget
+ self.samples = {}
+
+ def partial_fit(self, features, targets, active_targets):
+ """Update the distance metric with new data.
+ Parameters
+ ----------
+ features : ndarray
+ An NxM matrix of N features of dimensionality M.
+ targets : ndarray
+ An integer array of associated target identities.
+ active_targets : List[int]
+ A list of targets that are currently present in the scene.
+ """
+ for feature, target in zip(features, targets):
+ self.samples.setdefault(target, []).append(feature)
+ if self.budget is not None:
+ self.samples[target] = self.samples[target][-self.budget:]
+ self.samples = {k: self.samples[k] for k in active_targets}
+
+ def distance(self, features, targets):
+ """Compute distance between features and targets.
+ Parameters
+ ----------
+ features : ndarray
+ An NxM matrix of N features of dimensionality M.
+ targets : List[int]
+ A list of targets to match the given `features` against.
+ Returns
+ -------
+ ndarray
+ Returns a cost matrix of shape len(targets), len(features), where
+ element (i, j) contains the closest squared distance between
+ `targets[i]` and `features[j]`.
+ """
+ cost_matrix = np.zeros((len(targets), len(features)))
+ for i, target in enumerate(targets):
+ cost_matrix[i, :] = self._metric(self.samples[target], features)
+ return cost_matrix
\ No newline at end of file
diff --git a/trackers/strong_sort/sort/preprocessing.py b/trackers/strong_sort/sort/preprocessing.py
new file mode 100644
index 0000000000000000000000000000000000000000..5493b127f602dec398efac4269c00d31a3650ce9
--- /dev/null
+++ b/trackers/strong_sort/sort/preprocessing.py
@@ -0,0 +1,73 @@
+# vim: expandtab:ts=4:sw=4
+import numpy as np
+import cv2
+
+
+def non_max_suppression(boxes, max_bbox_overlap, scores=None):
+ """Suppress overlapping detections.
+
+ Original code from [1]_ has been adapted to include confidence score.
+
+ .. [1] http://www.pyimagesearch.com/2015/02/16/
+ faster-non-maximum-suppression-python/
+
+ Examples
+ --------
+
+ >>> boxes = [d.roi for d in detections]
+ >>> scores = [d.confidence for d in detections]
+ >>> indices = non_max_suppression(boxes, max_bbox_overlap, scores)
+ >>> detections = [detections[i] for i in indices]
+
+ Parameters
+ ----------
+ boxes : ndarray
+ Array of ROIs (x, y, width, height).
+ max_bbox_overlap : float
+ ROIs that overlap more than this values are suppressed.
+ scores : Optional[array_like]
+ Detector confidence score.
+
+ Returns
+ -------
+ List[int]
+ Returns indices of detections that have survived non-maxima suppression.
+
+ """
+ if len(boxes) == 0:
+ return []
+
+ boxes = boxes.astype(np.float)
+ pick = []
+
+ x1 = boxes[:, 0]
+ y1 = boxes[:, 1]
+ x2 = boxes[:, 2] + boxes[:, 0]
+ y2 = boxes[:, 3] + boxes[:, 1]
+
+ area = (x2 - x1 + 1) * (y2 - y1 + 1)
+ if scores is not None:
+ idxs = np.argsort(scores)
+ else:
+ idxs = np.argsort(y2)
+
+ while len(idxs) > 0:
+ last = len(idxs) - 1
+ i = idxs[last]
+ pick.append(i)
+
+ xx1 = np.maximum(x1[i], x1[idxs[:last]])
+ yy1 = np.maximum(y1[i], y1[idxs[:last]])
+ xx2 = np.minimum(x2[i], x2[idxs[:last]])
+ yy2 = np.minimum(y2[i], y2[idxs[:last]])
+
+ w = np.maximum(0, xx2 - xx1 + 1)
+ h = np.maximum(0, yy2 - yy1 + 1)
+
+ overlap = (w * h) / area[idxs[:last]]
+
+ idxs = np.delete(
+ idxs, np.concatenate(
+ ([last], np.where(overlap > max_bbox_overlap)[0])))
+
+ return pick
diff --git a/trackers/strong_sort/sort/track.py b/trackers/strong_sort/sort/track.py
new file mode 100644
index 0000000000000000000000000000000000000000..b6388b616c66e6112fff298f973c3e3e0c61306d
--- /dev/null
+++ b/trackers/strong_sort/sort/track.py
@@ -0,0 +1,298 @@
+# vim: expandtab:ts=4:sw=4
+import cv2
+import numpy as np
+from trackers.strong_sort.sort.kalman_filter import KalmanFilter
+
+
+class TrackState:
+ """
+ Enumeration type for the single target track state. Newly created tracks are
+ classified as `tentative` until enough evidence has been collected. Then,
+ the track state is changed to `confirmed`. Tracks that are no longer alive
+ are classified as `deleted` to mark them for removal from the set of active
+ tracks.
+
+ """
+
+ Tentative = 1
+ Confirmed = 2
+ Deleted = 3
+
+
+class Track:
+ """
+ A single target track with state space `(x, y, a, h)` and associated
+ velocities, where `(x, y)` is the center of the bounding box, `a` is the
+ aspect ratio and `h` is the height.
+
+ Parameters
+ ----------
+ mean : ndarray
+ Mean vector of the initial state distribution.
+ covariance : ndarray
+ Covariance matrix of the initial state distribution.
+ track_id : int
+ A unique track identifier.
+ n_init : int
+ Number of consecutive detections before the track is confirmed. The
+ track state is set to `Deleted` if a miss occurs within the first
+ `n_init` frames.
+ max_age : int
+ The maximum number of consecutive misses before the track state is
+ set to `Deleted`.
+ feature : Optional[ndarray]
+ Feature vector of the detection this track originates from. If not None,
+ this feature is added to the `features` cache.
+
+ Attributes
+ ----------
+ mean : ndarray
+ Mean vector of the initial state distribution.
+ covariance : ndarray
+ Covariance matrix of the initial state distribution.
+ track_id : int
+ A unique track identifier.
+ hits : int
+ Total number of measurement updates.
+ age : int
+ Total number of frames since first occurance.
+ time_since_update : int
+ Total number of frames since last measurement update.
+ state : TrackState
+ The current track state.
+ features : List[ndarray]
+ A cache of features. On each measurement update, the associated feature
+ vector is added to this list.
+
+ """
+
+ def __init__(self, detection, track_id, class_id, conf, n_init, max_age, ema_alpha,
+ feature=None):
+ self.track_id = track_id
+ self.class_id = int(class_id)
+ self.hits = 1
+ self.age = 1
+ self.time_since_update = 0
+ self.ema_alpha = ema_alpha
+
+ self.state = TrackState.Tentative
+ self.features = []
+ if feature is not None:
+ feature /= np.linalg.norm(feature)
+ self.features.append(feature)
+
+ self.conf = conf
+ self._n_init = n_init
+ self._max_age = max_age
+
+ self.kf = KalmanFilter()
+ self.mean, self.covariance = self.kf.initiate(detection)
+
+ def to_tlwh(self):
+ """Get current position in bounding box format `(top left x, top left y,
+ width, height)`.
+
+ Returns
+ -------
+ ndarray
+ The bounding box.
+
+ """
+ ret = self.mean[:4].copy()
+ ret[2] *= ret[3]
+ ret[:2] -= ret[2:] / 2
+ return ret
+
+ def to_tlbr(self):
+ """Get kf estimated current position in bounding box format `(min x, miny, max x,
+ max y)`.
+
+ Returns
+ -------
+ ndarray
+ The predicted kf bounding box.
+
+ """
+ ret = self.to_tlwh()
+ ret[2:] = ret[:2] + ret[2:]
+ return ret
+
+
+ def ECC(self, src, dst, warp_mode = cv2.MOTION_EUCLIDEAN, eps = 1e-5,
+ max_iter = 100, scale = 0.1, align = False):
+ """Compute the warp matrix from src to dst.
+ Parameters
+ ----------
+ src : ndarray
+ An NxM matrix of source img(BGR or Gray), it must be the same format as dst.
+ dst : ndarray
+ An NxM matrix of target img(BGR or Gray).
+ warp_mode: flags of opencv
+ translation: cv2.MOTION_TRANSLATION
+ rotated and shifted: cv2.MOTION_EUCLIDEAN
+ affine(shift,rotated,shear): cv2.MOTION_AFFINE
+ homography(3d): cv2.MOTION_HOMOGRAPHY
+ eps: float
+ the threshold of the increment in the correlation coefficient between two iterations
+ max_iter: int
+ the number of iterations.
+ scale: float or [int, int]
+ scale_ratio: float
+ scale_size: [W, H]
+ align: bool
+ whether to warp affine or perspective transforms to the source image
+ Returns
+ -------
+ warp matrix : ndarray
+ Returns the warp matrix from src to dst.
+ if motion models is homography, the warp matrix will be 3x3, otherwise 2x3
+ src_aligned: ndarray
+ aligned source image of gray
+ """
+
+ # BGR2GRAY
+ if src.ndim == 3:
+ # Convert images to grayscale
+ src = cv2.cvtColor(src, cv2.COLOR_BGR2GRAY)
+ dst = cv2.cvtColor(dst, cv2.COLOR_BGR2GRAY)
+
+ # make the imgs smaller to speed up
+ if scale is not None:
+ if isinstance(scale, float) or isinstance(scale, int):
+ if scale != 1:
+ src_r = cv2.resize(src, (0, 0), fx = scale, fy = scale,interpolation = cv2.INTER_LINEAR)
+ dst_r = cv2.resize(dst, (0, 0), fx = scale, fy = scale,interpolation = cv2.INTER_LINEAR)
+ scale = [scale, scale]
+ else:
+ src_r, dst_r = src, dst
+ scale = None
+ else:
+ if scale[0] != src.shape[1] and scale[1] != src.shape[0]:
+ src_r = cv2.resize(src, (scale[0], scale[1]), interpolation = cv2.INTER_LINEAR)
+ dst_r = cv2.resize(dst, (scale[0], scale[1]), interpolation=cv2.INTER_LINEAR)
+ scale = [scale[0] / src.shape[1], scale[1] / src.shape[0]]
+ else:
+ src_r, dst_r = src, dst
+ scale = None
+ else:
+ src_r, dst_r = src, dst
+
+ # Define 2x3 or 3x3 matrices and initialize the matrix to identity
+ if warp_mode == cv2.MOTION_HOMOGRAPHY :
+ warp_matrix = np.eye(3, 3, dtype=np.float32)
+ else :
+ warp_matrix = np.eye(2, 3, dtype=np.float32)
+
+ # Define termination criteria
+ criteria = (cv2.TERM_CRITERIA_EPS | cv2.TERM_CRITERIA_COUNT, max_iter, eps)
+
+ # Run the ECC algorithm. The results are stored in warp_matrix.
+ try:
+ (cc, warp_matrix) = cv2.findTransformECC (src_r, dst_r, warp_matrix, warp_mode, criteria, None, 1)
+ except cv2.error as e:
+ print('ecc transform failed')
+ return None, None
+
+ if scale is not None:
+ warp_matrix[0, 2] = warp_matrix[0, 2] / scale[0]
+ warp_matrix[1, 2] = warp_matrix[1, 2] / scale[1]
+
+ if align:
+ sz = src.shape
+ if warp_mode == cv2.MOTION_HOMOGRAPHY:
+ # Use warpPerspective for Homography
+ src_aligned = cv2.warpPerspective(src, warp_matrix, (sz[1],sz[0]), flags=cv2.INTER_LINEAR)
+ else :
+ # Use warpAffine for Translation, Euclidean and Affine
+ src_aligned = cv2.warpAffine(src, warp_matrix, (sz[1],sz[0]), flags=cv2.INTER_LINEAR)
+ return warp_matrix, src_aligned
+ else:
+ return warp_matrix, None
+
+
+ def get_matrix(self, matrix):
+ eye = np.eye(3)
+ dist = np.linalg.norm(eye - matrix)
+ if dist < 100:
+ return matrix
+ else:
+ return eye
+
+ def camera_update(self, previous_frame, next_frame):
+ warp_matrix, src_aligned = self.ECC(previous_frame, next_frame)
+ if warp_matrix is None and src_aligned is None:
+ return
+ [a,b] = warp_matrix
+ warp_matrix=np.array([a,b,[0,0,1]])
+ warp_matrix = warp_matrix.tolist()
+ matrix = self.get_matrix(warp_matrix)
+
+ x1, y1, x2, y2 = self.to_tlbr()
+ x1_, y1_, _ = matrix @ np.array([x1, y1, 1]).T
+ x2_, y2_, _ = matrix @ np.array([x2, y2, 1]).T
+ w, h = x2_ - x1_, y2_ - y1_
+ cx, cy = x1_ + w / 2, y1_ + h / 2
+ self.mean[:4] = [cx, cy, w / h, h]
+
+
+ def increment_age(self):
+ self.age += 1
+ self.time_since_update += 1
+
+ def predict(self, kf):
+ """Propagate the state distribution to the current time step using a
+ Kalman filter prediction step.
+
+ Parameters
+ ----------
+ kf : kalman_filter.KalmanFilter
+ The Kalman filter.
+
+ """
+ self.mean, self.covariance = self.kf.predict(self.mean, self.covariance)
+ self.age += 1
+ self.time_since_update += 1
+
+ def update(self, detection, class_id, conf):
+ """Perform Kalman filter measurement update step and update the feature
+ cache.
+ Parameters
+ ----------
+ detection : Detection
+ The associated detection.
+ """
+ self.conf = conf
+ self.class_id = class_id.int()
+ self.mean, self.covariance = self.kf.update(self.mean, self.covariance, detection.to_xyah(), detection.confidence)
+
+ feature = detection.feature / np.linalg.norm(detection.feature)
+
+ smooth_feat = self.ema_alpha * self.features[-1] + (1 - self.ema_alpha) * feature
+ smooth_feat /= np.linalg.norm(smooth_feat)
+ self.features = [smooth_feat]
+
+ self.hits += 1
+ self.time_since_update = 0
+ if self.state == TrackState.Tentative and self.hits >= self._n_init:
+ self.state = TrackState.Confirmed
+
+ def mark_missed(self):
+ """Mark this track as missed (no association at the current time step).
+ """
+ if self.state == TrackState.Tentative:
+ self.state = TrackState.Deleted
+ elif self.time_since_update > self._max_age:
+ self.state = TrackState.Deleted
+
+ def is_tentative(self):
+ """Returns True if this track is tentative (unconfirmed).
+ """
+ return self.state == TrackState.Tentative
+
+ def is_confirmed(self):
+ """Returns True if this track is confirmed."""
+ return self.state == TrackState.Confirmed
+
+ def is_deleted(self):
+ """Returns True if this track is dead and should be deleted."""
+ return self.state == TrackState.Deleted
diff --git a/trackers/strong_sort/sort/tracker.py b/trackers/strong_sort/sort/tracker.py
new file mode 100644
index 0000000000000000000000000000000000000000..e0bcce6c7b48e4535f21710abf226c107d5a0f99
--- /dev/null
+++ b/trackers/strong_sort/sort/tracker.py
@@ -0,0 +1,177 @@
+# vim: expandtab:ts=4:sw=4
+from __future__ import absolute_import
+import numpy as np
+from . import kalman_filter
+from . import linear_assignment
+from . import iou_matching
+from .track import Track
+
+
+class Tracker:
+ """
+ This is the multi-target tracker.
+ Parameters
+ ----------
+ metric : nn_matching.NearestNeighborDistanceMetric
+ A distance metric for measurement-to-track association.
+ max_age : int
+ Maximum number of missed misses before a track is deleted.
+ n_init : int
+ Number of consecutive detections before the track is confirmed. The
+ track state is set to `Deleted` if a miss occurs within the first
+ `n_init` frames.
+ Attributes
+ ----------
+ metric : nn_matching.NearestNeighborDistanceMetric
+ The distance metric used for measurement to track association.
+ max_age : int
+ Maximum number of missed misses before a track is deleted.
+ n_init : int
+ Number of frames that a track remains in initialization phase.
+ kf : kalman_filter.KalmanFilter
+ A Kalman filter to filter target trajectories in image space.
+ tracks : List[Track]
+ The list of active tracks at the current time step.
+ """
+ GATING_THRESHOLD = np.sqrt(kalman_filter.chi2inv95[4])
+
+ def __init__(self, metric, max_iou_distance=0.9, max_age=30, n_init=3, _lambda=0, ema_alpha=0.9, mc_lambda=0.995):
+ self.metric = metric
+ self.max_iou_distance = max_iou_distance
+ self.max_age = max_age
+ self.n_init = n_init
+ self._lambda = _lambda
+ self.ema_alpha = ema_alpha
+ self.mc_lambda = mc_lambda
+
+ self.kf = kalman_filter.KalmanFilter()
+ self.tracks = []
+ self._next_id = 1
+
+ def predict(self):
+ """Propagate track state distributions one time step forward.
+
+ This function should be called once every time step, before `update`.
+ """
+ for track in self.tracks:
+ track.predict(self.kf)
+
+ def increment_ages(self):
+ for track in self.tracks:
+ track.increment_age()
+ track.mark_missed()
+
+ def camera_update(self, previous_img, current_img):
+ for track in self.tracks:
+ track.camera_update(previous_img, current_img)
+
+ def update(self, detections, classes, confidences):
+ """Perform measurement update and track management.
+
+ Parameters
+ ----------
+ detections : List[deep_sort.detection.Detection]
+ A list of detections at the current time step.
+
+ """
+ # Run matching cascade.
+ matches, unmatched_tracks, unmatched_detections = \
+ self._match(detections)
+
+ # Update track set.
+ for track_idx, detection_idx in matches:
+ self.tracks[track_idx].update(
+ detections[detection_idx], classes[detection_idx], confidences[detection_idx])
+ for track_idx in unmatched_tracks:
+ self.tracks[track_idx].mark_missed()
+ for detection_idx in unmatched_detections:
+ self._initiate_track(detections[detection_idx], classes[detection_idx].item(), confidences[detection_idx].item())
+ self.tracks = [t for t in self.tracks if not t.is_deleted()]
+
+ # Update distance metric.
+ active_targets = [t.track_id for t in self.tracks if t.is_confirmed()]
+ features, targets = [], []
+ for track in self.tracks:
+ if not track.is_confirmed():
+ continue
+ features += track.features
+ targets += [track.track_id for _ in track.features]
+ self.metric.partial_fit(np.asarray(features), np.asarray(targets), active_targets)
+
+ def _full_cost_metric(self, tracks, dets, track_indices, detection_indices):
+ """
+ This implements the full lambda-based cost-metric. However, in doing so, it disregards
+ the possibility to gate the position only which is provided by
+ linear_assignment.gate_cost_matrix(). Instead, I gate by everything.
+ Note that the Mahalanobis distance is itself an unnormalised metric. Given the cosine
+ distance being normalised, we employ a quick and dirty normalisation based on the
+ threshold: that is, we divide the positional-cost by the gating threshold, thus ensuring
+ that the valid values range 0-1.
+ Note also that the authors work with the squared distance. I also sqrt this, so that it
+ is more intuitive in terms of values.
+ """
+ # Compute First the Position-based Cost Matrix
+ pos_cost = np.empty([len(track_indices), len(detection_indices)])
+ msrs = np.asarray([dets[i].to_xyah() for i in detection_indices])
+ for row, track_idx in enumerate(track_indices):
+ pos_cost[row, :] = np.sqrt(
+ self.kf.gating_distance(
+ tracks[track_idx].mean, tracks[track_idx].covariance, msrs, False
+ )
+ ) / self.GATING_THRESHOLD
+ pos_gate = pos_cost > 1.0
+ # Now Compute the Appearance-based Cost Matrix
+ app_cost = self.metric.distance(
+ np.array([dets[i].feature for i in detection_indices]),
+ np.array([tracks[i].track_id for i in track_indices]),
+ )
+ app_gate = app_cost > self.metric.matching_threshold
+ # Now combine and threshold
+ cost_matrix = self._lambda * pos_cost + (1 - self._lambda) * app_cost
+ cost_matrix[np.logical_or(pos_gate, app_gate)] = linear_assignment.INFTY_COST
+ # Return Matrix
+ return cost_matrix
+
+ def _match(self, detections):
+
+ def gated_metric(tracks, dets, track_indices, detection_indices):
+ features = np.array([dets[i].feature for i in detection_indices])
+ targets = np.array([tracks[i].track_id for i in track_indices])
+ cost_matrix = self.metric.distance(features, targets)
+ cost_matrix = linear_assignment.gate_cost_matrix(cost_matrix, tracks, dets, track_indices, detection_indices)
+
+ return cost_matrix
+
+ # Split track set into confirmed and unconfirmed tracks.
+ confirmed_tracks = [
+ i for i, t in enumerate(self.tracks) if t.is_confirmed()]
+ unconfirmed_tracks = [
+ i for i, t in enumerate(self.tracks) if not t.is_confirmed()]
+
+ # Associate confirmed tracks using appearance features.
+ matches_a, unmatched_tracks_a, unmatched_detections = \
+ linear_assignment.matching_cascade(
+ gated_metric, self.metric.matching_threshold, self.max_age,
+ self.tracks, detections, confirmed_tracks)
+
+ # Associate remaining tracks together with unconfirmed tracks using IOU.
+ iou_track_candidates = unconfirmed_tracks + [
+ k for k in unmatched_tracks_a if
+ self.tracks[k].time_since_update == 1]
+ unmatched_tracks_a = [
+ k for k in unmatched_tracks_a if
+ self.tracks[k].time_since_update != 1]
+ matches_b, unmatched_tracks_b, unmatched_detections = \
+ linear_assignment.min_cost_matching(
+ iou_matching.iou_cost, self.max_iou_distance, self.tracks,
+ detections, iou_track_candidates, unmatched_detections)
+
+ matches = matches_a + matches_b
+ unmatched_tracks = list(set(unmatched_tracks_a + unmatched_tracks_b))
+ return matches, unmatched_tracks, unmatched_detections
+
+ def _initiate_track(self, detection, class_id, conf):
+ self.tracks.append(Track(
+ detection.to_xyah(), self._next_id, class_id, conf, self.n_init, self.max_age, self.ema_alpha,
+ detection.feature))
+ self._next_id += 1
diff --git a/trackers/strong_sort/strong_sort.py b/trackers/strong_sort/strong_sort.py
new file mode 100644
index 0000000000000000000000000000000000000000..1f94873c20a51b8caec5ddee872449c1d5882dd8
--- /dev/null
+++ b/trackers/strong_sort/strong_sort.py
@@ -0,0 +1,139 @@
+import numpy as np
+import torch
+import sys
+import cv2
+import gdown
+from os.path import exists as file_exists, join
+import torchvision.transforms as transforms
+
+from sort.nn_matching import NearestNeighborDistanceMetric
+from sort.detection import Detection
+from sort.tracker import Tracker
+
+from reid_multibackend import ReIDDetectMultiBackend
+
+from yolov5.utils.general import xyxy2xywh
+
+
+class StrongSORT(object):
+ def __init__(self,
+ model_weights,
+ device,
+ fp16,
+ max_dist=0.2,
+ max_iou_distance=0.7,
+ max_age=70, n_init=3,
+ nn_budget=100,
+ mc_lambda=0.995,
+ ema_alpha=0.9
+ ):
+
+ self.model = ReIDDetectMultiBackend(weights=model_weights, device=device, fp16=fp16)
+
+ self.max_dist = max_dist
+ metric = NearestNeighborDistanceMetric(
+ "cosine", self.max_dist, nn_budget)
+ self.tracker = Tracker(
+ metric, max_iou_distance=max_iou_distance, max_age=max_age, n_init=n_init)
+
+ def update(self, dets, ori_img):
+
+ xyxys = dets[:, 0:4]
+ confs = dets[:, 4]
+ clss = dets[:, 5]
+
+ classes = clss.numpy()
+ xywhs = xyxy2xywh(xyxys.numpy())
+ confs = confs.numpy()
+ self.height, self.width = ori_img.shape[:2]
+
+ # generate detections
+ features = self._get_features(xywhs, ori_img)
+ bbox_tlwh = self._xywh_to_tlwh(xywhs)
+ detections = [Detection(bbox_tlwh[i], conf, features[i]) for i, conf in enumerate(
+ confs)]
+
+ # run on non-maximum supression
+ boxes = np.array([d.tlwh for d in detections])
+ scores = np.array([d.confidence for d in detections])
+
+ # update tracker
+ self.tracker.predict()
+ self.tracker.update(detections, clss, confs)
+
+ # output bbox identities
+ outputs = []
+ for track in self.tracker.tracks:
+ if not track.is_confirmed() or track.time_since_update > 1:
+ continue
+
+ box = track.to_tlwh()
+ x1, y1, x2, y2 = self._tlwh_to_xyxy(box)
+
+ track_id = track.track_id
+ class_id = track.class_id
+ conf = track.conf
+ outputs.append(np.array([x1, y1, x2, y2, track_id, class_id, conf]))
+ if len(outputs) > 0:
+ outputs = np.stack(outputs, axis=0)
+ return outputs
+
+ """
+ TODO:
+ Convert bbox from xc_yc_w_h to xtl_ytl_w_h
+ Thanks JieChen91@github.com for reporting this bug!
+ """
+ @staticmethod
+ def _xywh_to_tlwh(bbox_xywh):
+ if isinstance(bbox_xywh, np.ndarray):
+ bbox_tlwh = bbox_xywh.copy()
+ elif isinstance(bbox_xywh, torch.Tensor):
+ bbox_tlwh = bbox_xywh.clone()
+ bbox_tlwh[:, 0] = bbox_xywh[:, 0] - bbox_xywh[:, 2] / 2.
+ bbox_tlwh[:, 1] = bbox_xywh[:, 1] - bbox_xywh[:, 3] / 2.
+ return bbox_tlwh
+
+ def _xywh_to_xyxy(self, bbox_xywh):
+ x, y, w, h = bbox_xywh
+ x1 = max(int(x - w / 2), 0)
+ x2 = min(int(x + w / 2), self.width - 1)
+ y1 = max(int(y - h / 2), 0)
+ y2 = min(int(y + h / 2), self.height - 1)
+ return x1, y1, x2, y2
+
+ def _tlwh_to_xyxy(self, bbox_tlwh):
+ """
+ TODO:
+ Convert bbox from xtl_ytl_w_h to xc_yc_w_h
+ Thanks JieChen91@github.com for reporting this bug!
+ """
+ x, y, w, h = bbox_tlwh
+ x1 = max(int(x), 0)
+ x2 = min(int(x+w), self.width - 1)
+ y1 = max(int(y), 0)
+ y2 = min(int(y+h), self.height - 1)
+ return x1, y1, x2, y2
+
+ def increment_ages(self):
+ self.tracker.increment_ages()
+
+ def _xyxy_to_tlwh(self, bbox_xyxy):
+ x1, y1, x2, y2 = bbox_xyxy
+
+ t = x1
+ l = y1
+ w = int(x2 - x1)
+ h = int(y2 - y1)
+ return t, l, w, h
+
+ def _get_features(self, bbox_xywh, ori_img):
+ im_crops = []
+ for box in bbox_xywh:
+ x1, y1, x2, y2 = self._xywh_to_xyxy(box)
+ im = ori_img[y1:y2, x1:x2]
+ im_crops.append(im)
+ if im_crops:
+ features = self.model(im_crops)
+ else:
+ features = np.array([])
+ return features
diff --git a/trackers/strong_sort/utils/__init__.py b/trackers/strong_sort/utils/__init__.py
new file mode 100644
index 0000000000000000000000000000000000000000..e69de29bb2d1d6434b8b29ae775ad8c2e48c5391
diff --git a/trackers/strong_sort/utils/__pycache__/__init__.cpython-38.pyc b/trackers/strong_sort/utils/__pycache__/__init__.cpython-38.pyc
new file mode 100644
index 0000000000000000000000000000000000000000..26fbdda9f49d3d8fd16bbcc432e17f9ef90ee9af
Binary files /dev/null and b/trackers/strong_sort/utils/__pycache__/__init__.cpython-38.pyc differ
diff --git a/trackers/strong_sort/utils/__pycache__/__init__.cpython-39.pyc b/trackers/strong_sort/utils/__pycache__/__init__.cpython-39.pyc
new file mode 100644
index 0000000000000000000000000000000000000000..905fe9ec2b8e25e1f83929d7aed6f5fca92e3cea
Binary files /dev/null and b/trackers/strong_sort/utils/__pycache__/__init__.cpython-39.pyc differ
diff --git a/trackers/strong_sort/utils/__pycache__/parser.cpython-38.pyc b/trackers/strong_sort/utils/__pycache__/parser.cpython-38.pyc
new file mode 100644
index 0000000000000000000000000000000000000000..6b3ad2243b729bff927cd41c68fc07b087f70202
Binary files /dev/null and b/trackers/strong_sort/utils/__pycache__/parser.cpython-38.pyc differ
diff --git a/trackers/strong_sort/utils/__pycache__/parser.cpython-39.pyc b/trackers/strong_sort/utils/__pycache__/parser.cpython-39.pyc
new file mode 100644
index 0000000000000000000000000000000000000000..c2e85c8e54fd70daa234fca45ae369058d176662
Binary files /dev/null and b/trackers/strong_sort/utils/__pycache__/parser.cpython-39.pyc differ
diff --git a/trackers/strong_sort/utils/asserts.py b/trackers/strong_sort/utils/asserts.py
new file mode 100644
index 0000000000000000000000000000000000000000..59a73cc04025762d6490fcd2945a747d963def32
--- /dev/null
+++ b/trackers/strong_sort/utils/asserts.py
@@ -0,0 +1,13 @@
+from os import environ
+
+
+def assert_in(file, files_to_check):
+ if file not in files_to_check:
+ raise AssertionError("{} does not exist in the list".format(str(file)))
+ return True
+
+
+def assert_in_env(check_list: list):
+ for item in check_list:
+ assert_in(item, environ.keys())
+ return True
diff --git a/trackers/strong_sort/utils/draw.py b/trackers/strong_sort/utils/draw.py
new file mode 100644
index 0000000000000000000000000000000000000000..bc7cb537978e86805d5d9789785a8afe67df9030
--- /dev/null
+++ b/trackers/strong_sort/utils/draw.py
@@ -0,0 +1,36 @@
+import numpy as np
+import cv2
+
+palette = (2 ** 11 - 1, 2 ** 15 - 1, 2 ** 20 - 1)
+
+
+def compute_color_for_labels(label):
+ """
+ Simple function that adds fixed color depending on the class
+ """
+ color = [int((p * (label ** 2 - label + 1)) % 255) for p in palette]
+ return tuple(color)
+
+
+def draw_boxes(img, bbox, identities=None, offset=(0,0)):
+ for i,box in enumerate(bbox):
+ x1,y1,x2,y2 = [int(i) for i in box]
+ x1 += offset[0]
+ x2 += offset[0]
+ y1 += offset[1]
+ y2 += offset[1]
+ # box text and bar
+ id = int(identities[i]) if identities is not None else 0
+ color = compute_color_for_labels(id)
+ label = '{}{:d}'.format("", id)
+ t_size = cv2.getTextSize(label, cv2.FONT_HERSHEY_PLAIN, 2 , 2)[0]
+ cv2.rectangle(img,(x1, y1),(x2,y2),color,3)
+ cv2.rectangle(img,(x1, y1),(x1+t_size[0]+3,y1+t_size[1]+4), color,-1)
+ cv2.putText(img,label,(x1,y1+t_size[1]+4), cv2.FONT_HERSHEY_PLAIN, 2, [255,255,255], 2)
+ return img
+
+
+
+if __name__ == '__main__':
+ for i in range(82):
+ print(compute_color_for_labels(i))
diff --git a/trackers/strong_sort/utils/evaluation.py b/trackers/strong_sort/utils/evaluation.py
new file mode 100644
index 0000000000000000000000000000000000000000..100179407181933d59809b25400d115cfa789867
--- /dev/null
+++ b/trackers/strong_sort/utils/evaluation.py
@@ -0,0 +1,103 @@
+import os
+import numpy as np
+import copy
+import motmetrics as mm
+mm.lap.default_solver = 'lap'
+from utils.io import read_results, unzip_objs
+
+
+class Evaluator(object):
+
+ def __init__(self, data_root, seq_name, data_type):
+ self.data_root = data_root
+ self.seq_name = seq_name
+ self.data_type = data_type
+
+ self.load_annotations()
+ self.reset_accumulator()
+
+ def load_annotations(self):
+ assert self.data_type == 'mot'
+
+ gt_filename = os.path.join(self.data_root, self.seq_name, 'gt', 'gt.txt')
+ self.gt_frame_dict = read_results(gt_filename, self.data_type, is_gt=True)
+ self.gt_ignore_frame_dict = read_results(gt_filename, self.data_type, is_ignore=True)
+
+ def reset_accumulator(self):
+ self.acc = mm.MOTAccumulator(auto_id=True)
+
+ def eval_frame(self, frame_id, trk_tlwhs, trk_ids, rtn_events=False):
+ # results
+ trk_tlwhs = np.copy(trk_tlwhs)
+ trk_ids = np.copy(trk_ids)
+
+ # gts
+ gt_objs = self.gt_frame_dict.get(frame_id, [])
+ gt_tlwhs, gt_ids = unzip_objs(gt_objs)[:2]
+
+ # ignore boxes
+ ignore_objs = self.gt_ignore_frame_dict.get(frame_id, [])
+ ignore_tlwhs = unzip_objs(ignore_objs)[0]
+
+
+ # remove ignored results
+ keep = np.ones(len(trk_tlwhs), dtype=bool)
+ iou_distance = mm.distances.iou_matrix(ignore_tlwhs, trk_tlwhs, max_iou=0.5)
+ if len(iou_distance) > 0:
+ match_is, match_js = mm.lap.linear_sum_assignment(iou_distance)
+ match_is, match_js = map(lambda a: np.asarray(a, dtype=int), [match_is, match_js])
+ match_ious = iou_distance[match_is, match_js]
+
+ match_js = np.asarray(match_js, dtype=int)
+ match_js = match_js[np.logical_not(np.isnan(match_ious))]
+ keep[match_js] = False
+ trk_tlwhs = trk_tlwhs[keep]
+ trk_ids = trk_ids[keep]
+
+ # get distance matrix
+ iou_distance = mm.distances.iou_matrix(gt_tlwhs, trk_tlwhs, max_iou=0.5)
+
+ # acc
+ self.acc.update(gt_ids, trk_ids, iou_distance)
+
+ if rtn_events and iou_distance.size > 0 and hasattr(self.acc, 'last_mot_events'):
+ events = self.acc.last_mot_events # only supported by https://github.com/longcw/py-motmetrics
+ else:
+ events = None
+ return events
+
+ def eval_file(self, filename):
+ self.reset_accumulator()
+
+ result_frame_dict = read_results(filename, self.data_type, is_gt=False)
+ frames = sorted(list(set(self.gt_frame_dict.keys()) | set(result_frame_dict.keys())))
+ for frame_id in frames:
+ trk_objs = result_frame_dict.get(frame_id, [])
+ trk_tlwhs, trk_ids = unzip_objs(trk_objs)[:2]
+ self.eval_frame(frame_id, trk_tlwhs, trk_ids, rtn_events=False)
+
+ return self.acc
+
+ @staticmethod
+ def get_summary(accs, names, metrics=('mota', 'num_switches', 'idp', 'idr', 'idf1', 'precision', 'recall')):
+ names = copy.deepcopy(names)
+ if metrics is None:
+ metrics = mm.metrics.motchallenge_metrics
+ metrics = copy.deepcopy(metrics)
+
+ mh = mm.metrics.create()
+ summary = mh.compute_many(
+ accs,
+ metrics=metrics,
+ names=names,
+ generate_overall=True
+ )
+
+ return summary
+
+ @staticmethod
+ def save_summary(summary, filename):
+ import pandas as pd
+ writer = pd.ExcelWriter(filename)
+ summary.to_excel(writer)
+ writer.save()
diff --git a/trackers/strong_sort/utils/io.py b/trackers/strong_sort/utils/io.py
new file mode 100644
index 0000000000000000000000000000000000000000..2dc9afd24019cd930eef6c21ab9f579313dd3b3a
--- /dev/null
+++ b/trackers/strong_sort/utils/io.py
@@ -0,0 +1,133 @@
+import os
+from typing import Dict
+import numpy as np
+
+# from utils.log import get_logger
+
+
+def write_results(filename, results, data_type):
+ if data_type == 'mot':
+ save_format = '{frame},{id},{x1},{y1},{w},{h},-1,-1,-1,-1\n'
+ elif data_type == 'kitti':
+ save_format = '{frame} {id} pedestrian 0 0 -10 {x1} {y1} {x2} {y2} -10 -10 -10 -1000 -1000 -1000 -10\n'
+ else:
+ raise ValueError(data_type)
+
+ with open(filename, 'w') as f:
+ for frame_id, tlwhs, track_ids in results:
+ if data_type == 'kitti':
+ frame_id -= 1
+ for tlwh, track_id in zip(tlwhs, track_ids):
+ if track_id < 0:
+ continue
+ x1, y1, w, h = tlwh
+ x2, y2 = x1 + w, y1 + h
+ line = save_format.format(frame=frame_id, id=track_id, x1=x1, y1=y1, x2=x2, y2=y2, w=w, h=h)
+ f.write(line)
+
+
+# def write_results(filename, results_dict: Dict, data_type: str):
+# if not filename:
+# return
+# path = os.path.dirname(filename)
+# if not os.path.exists(path):
+# os.makedirs(path)
+
+# if data_type in ('mot', 'mcmot', 'lab'):
+# save_format = '{frame},{id},{x1},{y1},{w},{h},1,-1,-1,-1\n'
+# elif data_type == 'kitti':
+# save_format = '{frame} {id} pedestrian -1 -1 -10 {x1} {y1} {x2} {y2} -1 -1 -1 -1000 -1000 -1000 -10 {score}\n'
+# else:
+# raise ValueError(data_type)
+
+# with open(filename, 'w') as f:
+# for frame_id, frame_data in results_dict.items():
+# if data_type == 'kitti':
+# frame_id -= 1
+# for tlwh, track_id in frame_data:
+# if track_id < 0:
+# continue
+# x1, y1, w, h = tlwh
+# x2, y2 = x1 + w, y1 + h
+# line = save_format.format(frame=frame_id, id=track_id, x1=x1, y1=y1, x2=x2, y2=y2, w=w, h=h, score=1.0)
+# f.write(line)
+# logger.info('Save results to {}'.format(filename))
+
+
+def read_results(filename, data_type: str, is_gt=False, is_ignore=False):
+ if data_type in ('mot', 'lab'):
+ read_fun = read_mot_results
+ else:
+ raise ValueError('Unknown data type: {}'.format(data_type))
+
+ return read_fun(filename, is_gt, is_ignore)
+
+
+"""
+labels={'ped', ... % 1
+'person_on_vhcl', ... % 2
+'car', ... % 3
+'bicycle', ... % 4
+'mbike', ... % 5
+'non_mot_vhcl', ... % 6
+'static_person', ... % 7
+'distractor', ... % 8
+'occluder', ... % 9
+'occluder_on_grnd', ... %10
+'occluder_full', ... % 11
+'reflection', ... % 12
+'crowd' ... % 13
+};
+"""
+
+
+def read_mot_results(filename, is_gt, is_ignore):
+ valid_labels = {1}
+ ignore_labels = {2, 7, 8, 12}
+ results_dict = dict()
+ if os.path.isfile(filename):
+ with open(filename, 'r') as f:
+ for line in f.readlines():
+ linelist = line.split(',')
+ if len(linelist) < 7:
+ continue
+ fid = int(linelist[0])
+ if fid < 1:
+ continue
+ results_dict.setdefault(fid, list())
+
+ if is_gt:
+ if 'MOT16-' in filename or 'MOT17-' in filename:
+ label = int(float(linelist[7]))
+ mark = int(float(linelist[6]))
+ if mark == 0 or label not in valid_labels:
+ continue
+ score = 1
+ elif is_ignore:
+ if 'MOT16-' in filename or 'MOT17-' in filename:
+ label = int(float(linelist[7]))
+ vis_ratio = float(linelist[8])
+ if label not in ignore_labels and vis_ratio >= 0:
+ continue
+ else:
+ continue
+ score = 1
+ else:
+ score = float(linelist[6])
+
+ tlwh = tuple(map(float, linelist[2:6]))
+ target_id = int(linelist[1])
+
+ results_dict[fid].append((tlwh, target_id, score))
+
+ return results_dict
+
+
+def unzip_objs(objs):
+ if len(objs) > 0:
+ tlwhs, ids, scores = zip(*objs)
+ else:
+ tlwhs, ids, scores = [], [], []
+ tlwhs = np.asarray(tlwhs, dtype=float).reshape(-1, 4)
+
+ return tlwhs, ids, scores
\ No newline at end of file
diff --git a/trackers/strong_sort/utils/json_logger.py b/trackers/strong_sort/utils/json_logger.py
new file mode 100644
index 0000000000000000000000000000000000000000..0afd0b45df736866c49473db78286685d77660ac
--- /dev/null
+++ b/trackers/strong_sort/utils/json_logger.py
@@ -0,0 +1,383 @@
+"""
+References:
+ https://medium.com/analytics-vidhya/creating-a-custom-logging-mechanism-for-real-time-object-detection-using-tdd-4ca2cfcd0a2f
+"""
+import json
+from os import makedirs
+from os.path import exists, join
+from datetime import datetime
+
+
+class JsonMeta(object):
+ HOURS = 3
+ MINUTES = 59
+ SECONDS = 59
+ PATH_TO_SAVE = 'LOGS'
+ DEFAULT_FILE_NAME = 'remaining'
+
+
+class BaseJsonLogger(object):
+ """
+ This is the base class that returns __dict__ of its own
+ it also returns the dicts of objects in the attributes that are list instances
+
+ """
+
+ def dic(self):
+ # returns dicts of objects
+ out = {}
+ for k, v in self.__dict__.items():
+ if hasattr(v, 'dic'):
+ out[k] = v.dic()
+ elif isinstance(v, list):
+ out[k] = self.list(v)
+ else:
+ out[k] = v
+ return out
+
+ @staticmethod
+ def list(values):
+ # applies the dic method on items in the list
+ return [v.dic() if hasattr(v, 'dic') else v for v in values]
+
+
+class Label(BaseJsonLogger):
+ """
+ For each bounding box there are various categories with confidences. Label class keeps track of that information.
+ """
+
+ def __init__(self, category: str, confidence: float):
+ self.category = category
+ self.confidence = confidence
+
+
+class Bbox(BaseJsonLogger):
+ """
+ This module stores the information for each frame and use them in JsonParser
+ Attributes:
+ labels (list): List of label module.
+ top (int):
+ left (int):
+ width (int):
+ height (int):
+
+ Args:
+ bbox_id (float):
+ top (int):
+ left (int):
+ width (int):
+ height (int):
+
+ References:
+ Check Label module for better understanding.
+
+
+ """
+
+ def __init__(self, bbox_id, top, left, width, height):
+ self.labels = []
+ self.bbox_id = bbox_id
+ self.top = top
+ self.left = left
+ self.width = width
+ self.height = height
+
+ def add_label(self, category, confidence):
+ # adds category and confidence only if top_k is not exceeded.
+ self.labels.append(Label(category, confidence))
+
+ def labels_full(self, value):
+ return len(self.labels) == value
+
+
+class Frame(BaseJsonLogger):
+ """
+ This module stores the information for each frame and use them in JsonParser
+ Attributes:
+ timestamp (float): The elapsed time of captured frame
+ frame_id (int): The frame number of the captured video
+ bboxes (list of Bbox objects): Stores the list of bbox objects.
+
+ References:
+ Check Bbox class for better information
+
+ Args:
+ timestamp (float):
+ frame_id (int):
+
+ """
+
+ def __init__(self, frame_id: int, timestamp: float = None):
+ self.frame_id = frame_id
+ self.timestamp = timestamp
+ self.bboxes = []
+
+ def add_bbox(self, bbox_id: int, top: int, left: int, width: int, height: int):
+ bboxes_ids = [bbox.bbox_id for bbox in self.bboxes]
+ if bbox_id not in bboxes_ids:
+ self.bboxes.append(Bbox(bbox_id, top, left, width, height))
+ else:
+ raise ValueError("Frame with id: {} already has a Bbox with id: {}".format(self.frame_id, bbox_id))
+
+ def add_label_to_bbox(self, bbox_id: int, category: str, confidence: float):
+ bboxes = {bbox.id: bbox for bbox in self.bboxes}
+ if bbox_id in bboxes.keys():
+ res = bboxes.get(bbox_id)
+ res.add_label(category, confidence)
+ else:
+ raise ValueError('the bbox with id: {} does not exists!'.format(bbox_id))
+
+
+class BboxToJsonLogger(BaseJsonLogger):
+ """
+ ُ This module is designed to automate the task of logging jsons. An example json is used
+ to show the contents of json file shortly
+ Example:
+ {
+ "video_details": {
+ "frame_width": 1920,
+ "frame_height": 1080,
+ "frame_rate": 20,
+ "video_name": "/home/gpu/codes/MSD/pedestrian_2/project/public/camera1.avi"
+ },
+ "frames": [
+ {
+ "frame_id": 329,
+ "timestamp": 3365.1254
+ "bboxes": [
+ {
+ "labels": [
+ {
+ "category": "pedestrian",
+ "confidence": 0.9
+ }
+ ],
+ "bbox_id": 0,
+ "top": 1257,
+ "left": 138,
+ "width": 68,
+ "height": 109
+ }
+ ]
+ }],
+
+ Attributes:
+ frames (dict): It's a dictionary that maps each frame_id to json attributes.
+ video_details (dict): information about video file.
+ top_k_labels (int): shows the allowed number of labels
+ start_time (datetime object): we use it to automate the json output by time.
+
+ Args:
+ top_k_labels (int): shows the allowed number of labels
+
+ """
+
+ def __init__(self, top_k_labels: int = 1):
+ self.frames = {}
+ self.video_details = self.video_details = dict(frame_width=None, frame_height=None, frame_rate=None,
+ video_name=None)
+ self.top_k_labels = top_k_labels
+ self.start_time = datetime.now()
+
+ def set_top_k(self, value):
+ self.top_k_labels = value
+
+ def frame_exists(self, frame_id: int) -> bool:
+ """
+ Args:
+ frame_id (int):
+
+ Returns:
+ bool: true if frame_id is recognized
+ """
+ return frame_id in self.frames.keys()
+
+ def add_frame(self, frame_id: int, timestamp: float = None) -> None:
+ """
+ Args:
+ frame_id (int):
+ timestamp (float): opencv captured frame time property
+
+ Raises:
+ ValueError: if frame_id would not exist in class frames attribute
+
+ Returns:
+ None
+
+ """
+ if not self.frame_exists(frame_id):
+ self.frames[frame_id] = Frame(frame_id, timestamp)
+ else:
+ raise ValueError("Frame id: {} already exists".format(frame_id))
+
+ def bbox_exists(self, frame_id: int, bbox_id: int) -> bool:
+ """
+ Args:
+ frame_id:
+ bbox_id:
+
+ Returns:
+ bool: if bbox exists in frame bboxes list
+ """
+ bboxes = []
+ if self.frame_exists(frame_id=frame_id):
+ bboxes = [bbox.bbox_id for bbox in self.frames[frame_id].bboxes]
+ return bbox_id in bboxes
+
+ def find_bbox(self, frame_id: int, bbox_id: int):
+ """
+
+ Args:
+ frame_id:
+ bbox_id:
+
+ Returns:
+ bbox_id (int):
+
+ Raises:
+ ValueError: if bbox_id does not exist in the bbox list of specific frame.
+ """
+ if not self.bbox_exists(frame_id, bbox_id):
+ raise ValueError("frame with id: {} does not contain bbox with id: {}".format(frame_id, bbox_id))
+ bboxes = {bbox.bbox_id: bbox for bbox in self.frames[frame_id].bboxes}
+ return bboxes.get(bbox_id)
+
+ def add_bbox_to_frame(self, frame_id: int, bbox_id: int, top: int, left: int, width: int, height: int) -> None:
+ """
+
+ Args:
+ frame_id (int):
+ bbox_id (int):
+ top (int):
+ left (int):
+ width (int):
+ height (int):
+
+ Returns:
+ None
+
+ Raises:
+ ValueError: if bbox_id already exist in frame information with frame_id
+ ValueError: if frame_id does not exist in frames attribute
+ """
+ if self.frame_exists(frame_id):
+ frame = self.frames[frame_id]
+ if not self.bbox_exists(frame_id, bbox_id):
+ frame.add_bbox(bbox_id, top, left, width, height)
+ else:
+ raise ValueError(
+ "frame with frame_id: {} already contains the bbox with id: {} ".format(frame_id, bbox_id))
+ else:
+ raise ValueError("frame with frame_id: {} does not exist".format(frame_id))
+
+ def add_label_to_bbox(self, frame_id: int, bbox_id: int, category: str, confidence: float):
+ """
+ Args:
+ frame_id:
+ bbox_id:
+ category:
+ confidence: the confidence value returned from yolo detection
+
+ Returns:
+ None
+
+ Raises:
+ ValueError: if labels quota (top_k_labels) exceeds.
+ """
+ bbox = self.find_bbox(frame_id, bbox_id)
+ if not bbox.labels_full(self.top_k_labels):
+ bbox.add_label(category, confidence)
+ else:
+ raise ValueError("labels in frame_id: {}, bbox_id: {} is fulled".format(frame_id, bbox_id))
+
+ def add_video_details(self, frame_width: int = None, frame_height: int = None, frame_rate: int = None,
+ video_name: str = None):
+ self.video_details['frame_width'] = frame_width
+ self.video_details['frame_height'] = frame_height
+ self.video_details['frame_rate'] = frame_rate
+ self.video_details['video_name'] = video_name
+
+ def output(self):
+ output = {'video_details': self.video_details}
+ result = list(self.frames.values())
+ output['frames'] = [item.dic() for item in result]
+ return output
+
+ def json_output(self, output_name):
+ """
+ Args:
+ output_name:
+
+ Returns:
+ None
+
+ Notes:
+ It creates the json output with `output_name` name.
+ """
+ if not output_name.endswith('.json'):
+ output_name += '.json'
+ with open(output_name, 'w') as file:
+ json.dump(self.output(), file)
+ file.close()
+
+ def set_start(self):
+ self.start_time = datetime.now()
+
+ def schedule_output_by_time(self, output_dir=JsonMeta.PATH_TO_SAVE, hours: int = 0, minutes: int = 0,
+ seconds: int = 60) -> None:
+ """
+ Notes:
+ Creates folder and then periodically stores the jsons on that address.
+
+ Args:
+ output_dir (str): the directory where output files will be stored
+ hours (int):
+ minutes (int):
+ seconds (int):
+
+ Returns:
+ None
+
+ """
+ end = datetime.now()
+ interval = 0
+ interval += abs(min([hours, JsonMeta.HOURS]) * 3600)
+ interval += abs(min([minutes, JsonMeta.MINUTES]) * 60)
+ interval += abs(min([seconds, JsonMeta.SECONDS]))
+ diff = (end - self.start_time).seconds
+
+ if diff > interval:
+ output_name = self.start_time.strftime('%Y-%m-%d %H-%M-%S') + '.json'
+ if not exists(output_dir):
+ makedirs(output_dir)
+ output = join(output_dir, output_name)
+ self.json_output(output_name=output)
+ self.frames = {}
+ self.start_time = datetime.now()
+
+ def schedule_output_by_frames(self, frames_quota, frame_counter, output_dir=JsonMeta.PATH_TO_SAVE):
+ """
+ saves as the number of frames quota increases higher.
+ :param frames_quota:
+ :param frame_counter:
+ :param output_dir:
+ :return:
+ """
+ pass
+
+ def flush(self, output_dir):
+ """
+ Notes:
+ We use this function to output jsons whenever possible.
+ like the time that we exit the while loop of opencv.
+
+ Args:
+ output_dir:
+
+ Returns:
+ None
+
+ """
+ filename = self.start_time.strftime('%Y-%m-%d %H-%M-%S') + '-remaining.json'
+ output = join(output_dir, filename)
+ self.json_output(output_name=output)
diff --git a/trackers/strong_sort/utils/log.py b/trackers/strong_sort/utils/log.py
new file mode 100644
index 0000000000000000000000000000000000000000..0d48757dca88f35e9ea2cd1ca16e41bac9976a45
--- /dev/null
+++ b/trackers/strong_sort/utils/log.py
@@ -0,0 +1,17 @@
+import logging
+
+
+def get_logger(name='root'):
+ formatter = logging.Formatter(
+ # fmt='%(asctime)s [%(levelname)s]: %(filename)s(%(funcName)s:%(lineno)s) >> %(message)s')
+ fmt='%(asctime)s [%(levelname)s]: %(message)s', datefmt='%Y-%m-%d %H:%M:%S')
+
+ handler = logging.StreamHandler()
+ handler.setFormatter(formatter)
+
+ logger = logging.getLogger(name)
+ logger.setLevel(logging.INFO)
+ logger.addHandler(handler)
+ return logger
+
+
diff --git a/trackers/strong_sort/utils/parser.py b/trackers/strong_sort/utils/parser.py
new file mode 100644
index 0000000000000000000000000000000000000000..c29ed84479c6a7b8bc7148f3aac8941c7b261c3d
--- /dev/null
+++ b/trackers/strong_sort/utils/parser.py
@@ -0,0 +1,41 @@
+import os
+import yaml
+from easydict import EasyDict as edict
+
+
+class YamlParser(edict):
+ """
+ This is yaml parser based on EasyDict.
+ """
+
+ def __init__(self, cfg_dict=None, config_file=None):
+ if cfg_dict is None:
+ cfg_dict = {}
+
+ if config_file is not None:
+ assert(os.path.isfile(config_file))
+ with open(config_file, 'r') as fo:
+ yaml_ = yaml.load(fo.read(), Loader=yaml.FullLoader)
+ cfg_dict.update(yaml_)
+
+ super(YamlParser, self).__init__(cfg_dict)
+
+ def merge_from_file(self, config_file):
+ with open(config_file, 'r') as fo:
+ yaml_ = yaml.load(fo.read(), Loader=yaml.FullLoader)
+ self.update(yaml_)
+
+ def merge_from_dict(self, config_dict):
+ self.update(config_dict)
+
+
+def get_config(config_file=None):
+ return YamlParser(config_file=config_file)
+
+
+if __name__ == "__main__":
+ cfg = YamlParser(config_file="../configs/yolov3.yaml")
+ cfg.merge_from_file("../configs/strong_sort.yaml")
+
+ import ipdb
+ ipdb.set_trace()
diff --git a/trackers/strong_sort/utils/tools.py b/trackers/strong_sort/utils/tools.py
new file mode 100644
index 0000000000000000000000000000000000000000..965fb69c2df41510fd740a4ab57d8fc7b81012de
--- /dev/null
+++ b/trackers/strong_sort/utils/tools.py
@@ -0,0 +1,39 @@
+from functools import wraps
+from time import time
+
+
+def is_video(ext: str):
+ """
+ Returns true if ext exists in
+ allowed_exts for video files.
+
+ Args:
+ ext:
+
+ Returns:
+
+ """
+
+ allowed_exts = ('.mp4', '.webm', '.ogg', '.avi', '.wmv', '.mkv', '.3gp')
+ return any((ext.endswith(x) for x in allowed_exts))
+
+
+def tik_tok(func):
+ """
+ keep track of time for each process.
+ Args:
+ func:
+
+ Returns:
+
+ """
+ @wraps(func)
+ def _time_it(*args, **kwargs):
+ start = time()
+ try:
+ return func(*args, **kwargs)
+ finally:
+ end_ = time()
+ print("time: {:.03f}s, fps: {:.03f}".format(end_ - start, 1 / (end_ - start)))
+
+ return _time_it
diff --git a/val.py b/val.py
new file mode 100644
index 0000000000000000000000000000000000000000..a78230dfc219eaa68a71a81a7412bdcf2acad8b7
--- /dev/null
+++ b/val.py
@@ -0,0 +1,189 @@
+import os
+import sys
+import torch
+import logging
+import subprocess
+from subprocess import Popen
+import argparse
+import git
+from git import Repo
+import zipfile
+from pathlib import Path
+import shutil
+import threading
+
+FILE = Path(__file__).resolve()
+ROOT = FILE.parents[0] # yolov5 strongsort root directory
+WEIGHTS = ROOT / 'weights'
+
+if str(ROOT) not in sys.path:
+ sys.path.append(str(ROOT)) # add ROOT to PATH
+if str(ROOT / 'yolov5') not in sys.path:
+ sys.path.append(str(ROOT / 'yolov5')) # add yolov5 ROOT to PATH
+if str(ROOT / 'strong_sort') not in sys.path:
+ sys.path.append(str(ROOT / 'strong_sort')) # add strong_sort ROOT to PATH
+ROOT = Path(os.path.relpath(ROOT, Path.cwd())) # relative
+
+from yolov5.utils.general import LOGGER, check_requirements, print_args, increment_path
+from yolov5.utils.torch_utils import select_device
+from track import run
+
+
+def download_official_mot_eval_tool(dst_val_tools_folder):
+ # source: https://github.com/JonathonLuiten/TrackEval#official-evaluation-code
+ val_tools_url = "https://github.com/JonathonLuiten/TrackEval"
+ try:
+ Repo.clone_from(val_tools_url, dst_val_tools_folder)
+ LOGGER.info('Official MOT evaluation repo downloaded')
+ except git.exc.GitError as err:
+ LOGGER.info('Eval repo already downloaded')
+
+def download_mot_dataset(dst_val_tools_folder, benchmark):
+ gt_data_url = 'https://omnomnom.vision.rwth-aachen.de/data/TrackEval/data.zip'
+ subprocess.run(["wget", "-nc", gt_data_url, "-O", dst_val_tools_folder / 'data.zip']) # python module has no -nc nor -N flag
+ if not (dst_val_tools_folder / 'data').is_dir():
+ with zipfile.ZipFile(dst_val_tools_folder / 'data.zip', 'r') as zip_ref:
+ zip_ref.extractall(dst_val_tools_folder)
+ LOGGER.info('MOTs ground truth downloaded')
+ else:
+ LOGGER.info('gt already downloaded')
+
+ mot_gt_data_url = 'https://motchallenge.net/data/' + benchmark + '.zip'
+ subprocess.run(["wget", "-nc", mot_gt_data_url, "-O", dst_val_tools_folder / (benchmark + '.zip')]) # python module has no -nc nor -N flag
+ if not (dst_val_tools_folder / 'data' / benchmark).is_dir():
+ with zipfile.ZipFile(dst_val_tools_folder / (benchmark + '.zip'), 'r') as zip_ref:
+ if opt.benchmark == 'MOT16':
+ zip_ref.extractall(dst_val_tools_folder / 'data' / 'MOT16')
+ else:
+ zip_ref.extractall(dst_val_tools_folder / 'data')
+ LOGGER.info(f'{benchmark} images downloaded')
+ else:
+ LOGGER.info(f'{benchmark} data already downloaded')
+
+
+def parse_opt():
+ parser = argparse.ArgumentParser()
+ parser.add_argument('--yolo-weights', type=str, default= 'weights/best1.pt', help='model.pt path(s)')
+ parser.add_argument('--reid-weights', type=str, default=WEIGHTS / 'osnet_x1_0_dukemtmcreid.pt')
+ parser.add_argument('--tracking-method', type=str, default='strongsort', help='strongsort, ocsort')
+ parser.add_argument('--name', default='val', help='save results to project/name')
+ parser.add_argument('--project', default=ROOT / 'runs/track', help='save results to project/name')
+ parser.add_argument('--exist-ok', action='store_true', help='existing project/name ok, do not increment')
+ parser.add_argument('--benchmark', type=str, default='MOT17', help='MOT16, MOT17, MOT20')
+ parser.add_argument('--split', type=str, default='train', help='existing project/name ok, do not increment')
+ parser.add_argument('--eval-existing', type=str, default='', help='evaluate existing tracker results under mot_callenge/MOTXX-YY/...')
+ parser.add_argument('--conf-thres', type=float, default=0.45, help='confidence threshold')
+ parser.add_argument('--imgsz', '--img', '--img-size', nargs='+', type=int, default=[1280], help='inference size h,w')
+ parser.add_argument('--device', default='', help='cuda device, i.e. 0 or 0,1,2,3 or cpu')
+
+
+ opt = parser.parse_args()
+ device = []
+
+ for a in opt.device.split(','):
+ try:
+ a = int(a)
+ except ValueError:
+ pass
+ device.append(a)
+ opt.device = device
+
+ print_args(vars(opt))
+ return opt
+
+
+def main(opt):
+ check_requirements(requirements=ROOT / 'requirements.txt', exclude=('tensorboard', 'thop'))
+
+ # download eval files
+ dst_val_tools_folder = ROOT / 'val_utils'
+ download_official_mot_eval_tool(dst_val_tools_folder)
+
+ if any(opt.benchmark is s for s in ['MOT16', 'MOT17', 'MOT20']):
+ download_mot_dataset(dst_val_tools_folder, opt.benchmark)
+
+ # set paths
+ mot_seqs_path = dst_val_tools_folder / 'data' / opt.benchmark / opt.split
+
+ if opt.benchmark == 'MOT17':
+ # each sequences is present 3 times, one for each detector
+ # (DPM, FRCNN, SDP). Keep only sequences from one of them
+ seq_paths = sorted([str(p / 'img1') for p in Path(mot_seqs_path).iterdir() if Path(p).is_dir()])
+ seq_paths = [Path(p) for p in seq_paths if 'FRCNN' in p]
+ with open(dst_val_tools_folder / "data/gt/mot_challenge/seqmaps/MOT17-train.txt", "r") as f: #
+ lines = f.readlines()
+ # overwrite MOT17 evaluation sequences to evaluate so that they are not duplicated
+ with open(dst_val_tools_folder / "data/gt/mot_challenge/seqmaps/MOT17-train.txt", "w") as f:
+ for line in seq_paths:
+ f.write(str(line.parent.stem) + '\n')
+ else:
+ # this is not the case for MOT16, MOT20 or your custom dataset
+ seq_paths = [p / 'img1' for p in Path(mot_seqs_path).iterdir() if Path(p).is_dir()]
+
+ save_dir = increment_path(Path(opt.project) / opt.name, exist_ok=opt.exist_ok) # increment run
+ MOT_results_folder = dst_val_tools_folder / 'data' / 'trackers' / 'mot_challenge' / Path(str(opt.benchmark) + '-' + str(opt.split)) / save_dir.name / 'data'
+ (MOT_results_folder).mkdir(parents=True, exist_ok=True) # make
+
+ # extend devices to as many sequences are available
+ if any(isinstance(i,int) for i in opt.device) and len(opt.device) > 1:
+ devices = opt.device
+ for a in range(0, len(opt.device) % len(seq_paths)):
+ opt.device.extend(devices)
+ opt.device = opt.device[:len(seq_paths)]
+
+ if not opt.eval_existing:
+ processes = []
+ for i, seq_path in enumerate(seq_paths):
+ # spawn one subprocess per GPU in increasing order.
+ # When max devices are reached start at 0 again
+ tracking_subprocess_device = opt.device[i] if len(opt.device) > 1 else opt.device[0]
+
+ dst_seq_path = seq_path.parent / seq_path.parent.name
+ if not dst_seq_path.is_dir():
+ src_seq_path = seq_path
+ shutil.move(str(src_seq_path), str(dst_seq_path))
+
+ p = subprocess.Popen([
+ "python", "track.py", \
+ "--yolo-weights", opt.yolo_weights, \
+ "--reid-weights", opt.reid_weights, \
+ "--tracking-method", opt.tracking_method, \
+ "--conf-thres", str(opt.conf_thres), \
+ "--imgsz", str(opt.imgsz[0]), \
+ "--classes", str(0), \
+ "--name", save_dir.name, \
+ "--project", opt.project, \
+ "--device", str(tracking_subprocess_device), \
+ "--source", dst_seq_path, \
+ "--exist-ok", \
+ "--save-txt", \
+ ])
+ processes.append(p)
+
+ for p in processes:
+ p.wait()
+
+ results = (save_dir.parent / opt.eval_existing / 'tracks' if opt.eval_existing else save_dir / 'tracks').glob('*.txt')
+ for src in results:
+ if opt.eval_existing:
+ dst = MOT_results_folder.parent.parent / opt.eval_existing / 'data' / Path(src.stem + '.txt')
+ else:
+ dst = MOT_results_folder / Path(src.stem + '.txt')
+ dst.parent.mkdir(parents=True, exist_ok=True) # make
+ shutil.copyfile(src, dst)
+
+ # run the evaluation on the generated txts
+ subprocess.run([
+ "python", dst_val_tools_folder / "scripts/run_mot_challenge.py",\
+ "--BENCHMARK", opt.benchmark,\
+ "--TRACKERS_TO_EVAL", opt.eval_existing if opt.eval_existing else MOT_results_folder.parent.name,\
+ "--SPLIT_TO_EVAL", "train",\
+ "--METRICS", "HOTA", "CLEAR", "Identity",\
+ "--USE_PARALLEL", "True",\
+ "--NUM_PARALLEL_CORES", "4"\
+ ])
+
+
+if __name__ == "__main__":
+ opt = parse_opt()
+ main(opt)
diff --git a/val_utils/.gitignore b/val_utils/.gitignore
new file mode 100644
index 0000000000000000000000000000000000000000..9e6d0fe8e85eec8883fb07414879348d5a158853
--- /dev/null
+++ b/val_utils/.gitignore
@@ -0,0 +1,14 @@
+.idea
+.vscode
+**.pyc
+**__pycache__
+gt_data/*
+!gt_data/Readme.md
+tracker_output/*
+!tracker_output/Readme.md
+output/*
+data
+!goutput/Readme.md
+**/__pycache__
+.idea
+error_log.txt
diff --git a/val_utils/LICENSE b/val_utils/LICENSE
new file mode 100644
index 0000000000000000000000000000000000000000..70cbbf9ec0874865fcf827702be7f850251d4314
--- /dev/null
+++ b/val_utils/LICENSE
@@ -0,0 +1,21 @@
+MIT License
+
+Copyright (c) 2020 Jonathon Luiten
+
+Permission is hereby granted, free of charge, to any person obtaining a copy
+of this software and associated documentation files (the "Software"), to deal
+in the Software without restriction, including without limitation the rights
+to use, copy, modify, merge, publish, distribute, sublicense, and/or sell
+copies of the Software, and to permit persons to whom the Software is
+furnished to do so, subject to the following conditions:
+
+The above copyright notice and this permission notice shall be included in all
+copies or substantial portions of the Software.
+
+THE SOFTWARE IS PROVIDED "AS IS", WITHOUT WARRANTY OF ANY KIND, EXPRESS OR
+IMPLIED, INCLUDING BUT NOT LIMITED TO THE WARRANTIES OF MERCHANTABILITY,
+FITNESS FOR A PARTICULAR PURPOSE AND NONINFRINGEMENT. IN NO EVENT SHALL THE
+AUTHORS OR COPYRIGHT HOLDERS BE LIABLE FOR ANY CLAIM, DAMAGES OR OTHER
+LIABILITY, WHETHER IN AN ACTION OF CONTRACT, TORT OR OTHERWISE, ARISING FROM,
+OUT OF OR IN CONNECTION WITH THE SOFTWARE OR THE USE OR OTHER DEALINGS IN THE
+SOFTWARE.
diff --git a/val_utils/Readme.md b/val_utils/Readme.md
new file mode 100644
index 0000000000000000000000000000000000000000..9273a6f75ba115a1738b0b73952903a049c01ae3
--- /dev/null
+++ b/val_utils/Readme.md
@@ -0,0 +1,202 @@
+
+# TrackEval
+*Code for evaluating object tracking.*
+
+This codebase provides code for a number of different tracking evaluation metrics (including the [HOTA metrics](https://link.springer.com/article/10.1007/s11263-020-01375-2)), as well as supporting running all of these metrics on a number of different tracking benchmarks. Plus plotting of results and other things one may want to do for tracking evaluation.
+
+## **NEW**: RobMOTS Challenge 2021
+
+Call for submission to our [RobMOTS Challenge](https://eval.vision.rwth-aachen.de/rvsu-workshop21/?page_id=110) (Robust Multi-Object Tracking and Segmentation) held in conjunction with our [RVSU CVPR'21 Workshop](https://eval.vision.rwth-aachen.de/rvsu-workshop21/). Robust tracking evaluation against 8 tracking benchmarks. Challenge submission deadline June 15th. Also check out our workshop [call for papers](https://eval.vision.rwth-aachen.de/rvsu-workshop21/?page_id=74).
+
+## Official Evaluation Code
+
+The following benchmarks use TrackEval as their official evaluation code, check out the links to see TrackEval in action:
+
+ - **[RobMOTS](https://eval.vision.rwth-aachen.de/rvsu-workshop21/?page_id=110)** ([Official Readme](docs/RobMOTS-Official/Readme.md))
+ - **[KITTI Tracking](http://www.cvlibs.net/datasets/kitti/eval_tracking.php)**
+ - **[KITTI MOTS](http://www.cvlibs.net/datasets/kitti/eval_mots.php)**
+ - **[MOTChallenge](https://motchallenge.net/)** ([Official Readme](docs/MOTChallenge-Official/Readme.md))
+ - **[Open World Tracking](https://openworldtracking.github.io)** ([Official Readme](docs/OpenWorldTracking-Official))
+ - **[PersonPath22](https://amazon-research.github.io/tracking-dataset/personpath22.html)**
+
+
+If you run a tracking benchmark and want to use TrackEval as your official evaluation code, please contact Jonathon (contact details below).
+
+## Currently implemented metrics
+
+The following metrics are currently implemented:
+
+Metric Family | Sub metrics | Paper | Code | Notes |
+|----- | ----------- |----- | ----------- | ----- |
+| | | | | |
+|**HOTA metrics**|HOTA, DetA, AssA, LocA, DetPr, DetRe, AssPr, AssRe|[paper](https://link.springer.com/article/10.1007/s11263-020-01375-2)|[code](trackeval/metrics/hota.py)|**Recommended tracking metric**|
+|**CLEARMOT metrics**|MOTA, MOTP, MT, ML, Frag, etc.|[paper](https://link.springer.com/article/10.1155/2008/246309)|[code](trackeval/metrics/clear.py)| |
+|**Identity metrics**|IDF1, IDP, IDR|[paper](https://arxiv.org/abs/1609.01775)|[code](trackeval/metrics/identity.py)| |
+|**VACE metrics**|ATA, SFDA|[paper](https://link.springer.com/chapter/10.1007/11612704_16)|[code](trackeval/metrics/vace.py)| |
+|**Track mAP metrics**|Track mAP|[paper](https://arxiv.org/abs/1905.04804)|[code](trackeval/metrics/track_map.py)|Requires confidence scores|
+|**J & F metrics**|J&F, J, F|[paper](https://www.cv-foundation.org/openaccess/content_cvpr_2016/papers/Perazzi_A_Benchmark_Dataset_CVPR_2016_paper.pdf)|[code](trackeval/metrics/j_and_f.py)|Only for Seg Masks|
+|**ID Euclidean**|ID Euclidean|[paper](https://arxiv.org/pdf/2103.13516.pdf)|[code](trackeval/metrics/ideucl.py)| |
+
+
+## Currently implemented benchmarks
+
+The following benchmarks are currently implemented:
+
+Benchmark | Sub-benchmarks | Type | Website | Code | Data Format |
+|----- | ----------- |----- | ----------- | ----- | ----- |
+| | | | | | |
+|**RobMOTS**|Combination of 8 benchmarks|Seg Masks|[website](https://eval.vision.rwth-aachen.de/rvsu-workshop21/?page_id=110)|[code](trackeval/datasets/rob_mots.py)|[format](docs/RobMOTS-Official/Readme.md)|
+|**Open World Tracking**|TAO-OW|OpenWorld / Seg Masks|[website](https://openworldtracking.github.io)|[code](trackeval/datasets/tao_ow.py)|[format](docs/OpenWorldTracking-Official/Readme.md)|
+|**MOTChallenge**|MOT15/16/17/20|2D BBox|[website](https://motchallenge.net/)|[code](trackeval/datasets/mot_challenge_2d_box.py)|[format](docs/MOTChallenge-format.txt)|
+|**KITTI Tracking**| |2D BBox|[website](http://www.cvlibs.net/datasets/kitti/eval_tracking.php)|[code](trackeval/datasets/kitti_2d_box.py)|[format](docs/KITTI-format.txt)|
+|**BDD-100k**| |2D BBox|[website](https://bdd-data.berkeley.edu/)|[code](trackeval/datasets/bdd100k.py)|[format](docs/BDD100k-format.txt)|
+|**TAO**| |2D BBox|[website](https://taodataset.org/)|[code](trackeval/datasets/tao.py)|[format](docs/TAO-format.txt)|
+|**MOTS**|KITTI-MOTS, MOTS-Challenge|Seg Mask|[website](https://www.vision.rwth-aachen.de/page/mots)|[code](trackeval/datasets/mots_challenge.py) and [code](trackeval/datasets/kitti_mots.py)|[format](docs/MOTS-format.txt)|
+|**DAVIS**|Unsupervised|Seg Mask|[website](https://davischallenge.org/)|[code](trackeval/datasets/davis.py)|[format](docs/DAVIS-format.txt)|
+|**YouTube-VIS**| |Seg Mask|[website](https://youtube-vos.org/dataset/vis/)|[code](trackeval/datasets/youtube_vis.py)|[format](docs/YouTube-VIS-format.txt)|
+|**Head Tracking Challenge**| |2D BBox|[website](https://arxiv.org/pdf/2103.13516.pdf)|[code](trackeval/datasets/head_tracking_challenge.py)|[format](docs/MOTChallenge-format.txt)|
+|**PersonPath22**| |2D BBox|[website](https://github.com/amazon-research/tracking-dataset)|[code](trackeval/datasets/person_path_22.py)|[format](docs/MOTChallenge-format.txt)|
+|**BURST**| {Common, Long-tail, Open-world} Class-guided, {Point, Box, Mask} Exemplar-guided |Seg Mask|[website](https://github.com/Ali2500/BURST-benchmark)|[format](https://github.com/Ali2500/BURST-benchmark/blob/main/ANNOTATION_FORMAT.md)|
+
+## HOTA metrics
+
+This code is also the official reference implementation for the HOTA metrics:
+
+*[HOTA: A Higher Order Metric for Evaluating Multi-Object Tracking](https://link.springer.com/article/10.1007/s11263-020-01375-2). IJCV 2020. Jonathon Luiten, Aljosa Osep, Patrick Dendorfer, Philip Torr, Andreas Geiger, Laura Leal-Taixe and Bastian Leibe.*
+
+HOTA is a novel set of MOT evaluation metrics which enable better understanding of tracking behavior than previous metrics.
+
+For more information check out the following links:
+ - [Short blog post on HOTA](https://jonathonluiten.medium.com/how-to-evaluate-tracking-with-the-hota-metrics-754036d183e1) - **HIGHLY RECOMMENDED READING**
+ - [IJCV version of paper](https://link.springer.com/article/10.1007/s11263-020-01375-2) (Open Access)
+ - [ArXiv version of paper](https://arxiv.org/abs/2009.07736)
+ - [Code](trackeval/metrics/hota.py)
+
+## Properties of this codebase
+
+The code is written 100% in python with only numpy and scipy as minimum requirements.
+
+The code is designed to be easily understandable and easily extendable.
+
+The code is also extremely fast, running at more than 10x the speed of the both [MOTChallengeEvalKit](https://github.com/dendorferpatrick/MOTChallengeEvalKit), and [py-motmetrics](https://github.com/cheind/py-motmetrics) (see detailed speed comparison below).
+
+The implementation of CLEARMOT and ID metrics aligns perfectly with the [MOTChallengeEvalKit](https://github.com/dendorferpatrick/MOTChallengeEvalKit).
+
+By default the code prints results to the screen, saves results out as both a summary txt file and a detailed results csv file, and outputs plots of the results. All outputs are by default saved to the 'tracker' folder for each tracker.
+
+## Running the code
+
+The code can be run in one of two ways:
+
+ - From the terminal via one of the scripts [here](scripts/). See each script for instructions and arguments, hopefully this is self-explanatory.
+ - Directly by importing this package into your code, see the same scripts above for how.
+
+## Quickly evaluate on supported benchmarks
+
+To enable you to use TrackEval for evaluation as quickly and easily as possible, we provide ground-truth data, meta-data and example trackers for all currently supported benchmarks.
+You can download this here: [data.zip](https://omnomnom.vision.rwth-aachen.de/data/TrackEval/data.zip) (~150mb).
+
+The data for RobMOTS is separate and can be found here: [rob_mots_train_data.zip](https://omnomnom.vision.rwth-aachen.de/data/RobMOTS/train_data.zip) (~750mb).
+
+The data for PersonPath22 is separate and can be found here: [person_path_22_data.zip](https://tracking-dataset-eccv-2022.s3.us-east-2.amazonaws.com/person_path_22_data.zip) (~3mb).
+
+The easiest way to begin is to extract this zip into the repository root folder such that the file paths look like: TrackEval/data/gt/...
+
+This then corresponds to the default paths in the code. You can now run each of the scripts [here](scripts/) without providing any arguments and they will by default evaluate all trackers present in the supplied file structure. To evaluate your own tracking results, simply copy your files as a new tracker folder into the file structure at the same level as the example trackers (MPNTrack, CIWT, track_rcnn, qdtrack, ags, Tracktor++, STEm_Seg), ensuring the same file structure for your trackers as in the example.
+
+Of course, if your ground-truth and tracker files are located somewhere else you can simply use the script arguments to point the code toward your data.
+
+To ensure your tracker outputs data in the correct format, check out our format guides for each of the supported benchmarks [here](docs), or check out the example trackers provided.
+
+## Evaluate on your own custom benchmark
+
+To evaluate on your own data, you have two options:
+ - Write custom dataset code (more effort, rarely worth it).
+ - Convert your current dataset and trackers to the same format of an already implemented benchmark.
+
+To convert formats, check out the format specifications defined [here](docs).
+
+By default, we would recommend the MOTChallenge format, although any implemented format should work. Note that for many cases you will want to use the argument ```--DO_PREPROC False``` unless you want to run preprocessing to remove distractor objects.
+
+## Requirements
+ Code tested on Python 3.7.
+
+ - Minimum requirements: numpy, scipy
+ - For plotting: matplotlib
+ - For segmentation datasets (KITTI MOTS, MOTS-Challenge, DAVIS, YouTube-VIS): pycocotools
+ - For DAVIS dataset: Pillow
+ - For J & F metric: opencv_python, scikit_image
+ - For simples test-cases for metrics: pytest
+
+use ```pip3 -r install requirements.txt``` to install all possible requirements.
+
+use ```pip3 -r install minimum_requirments.txt``` to only install the minimum if you don't need the extra functionality as listed above.
+
+## Timing analysis
+
+Evaluating CLEAR + ID metrics on Lift_T tracker on MOT17-train (seconds) on a i7-9700K CPU with 8 physical cores (median of 3 runs):
+Num Cores|TrackEval|MOTChallenge|Speedup vs MOTChallenge|py-motmetrics|Speedup vs py-motmetrics
+:---|:---|:---|:---|:---|:---
+1|9.64|66.23|6.87x|99.65|10.34x
+4|3.01|29.42|9.77x| |33.11x*
+8|1.62|29.51|18.22x| |61.51x*
+
+*using a different number of cores as py-motmetrics doesn't allow multiprocessing.
+
+```
+python scripts/run_mot_challenge.py --BENCHMARK MOT17 --TRACKERS_TO_EVAL Lif_T --METRICS CLEAR Identity --USE_PARALLEL False --NUM_PARALLEL_CORES 1
+```
+
+Evaluating CLEAR + ID metrics on LPC_MOT tracker on MOT20-train (seconds) on a i7-9700K CPU with 8 physical cores (median of 3 runs):
+Num Cores|TrackEval|MOTChallenge|Speedup vs MOTChallenge|py-motmetrics|Speedup vs py-motmetrics
+:---|:---|:---|:---|:---|:---
+1|18.63|105.3|5.65x|175.17|9.40x
+
+```
+python scripts/run_mot_challenge.py --BENCHMARK MOT20 --TRACKERS_TO_EVAL LPC_MOT --METRICS CLEAR Identity --USE_PARALLEL False --NUM_PARALLEL_CORES 1
+```
+
+## License
+
+TrackEval is released under the [MIT License](LICENSE).
+
+## Contact
+
+If you encounter any problems with the code, please contact [Jonathon Luiten](https://www.vision.rwth-aachen.de/person/216/) ([luiten@vision.rwth-aachen.de](mailto:luiten@vision.rwth-aachen.de)).
+If anything is unclear, or hard to use, please leave a comment either via email or as an issue and I would love to help.
+
+## Dedication
+
+This codebase was built for you, in order to make your life easier! For anyone doing research on tracking or using trackers, please don't hesitate to reach out with any comments or suggestions on how things could be improved.
+
+## Contributing
+
+We welcome contributions of new metrics and new supported benchmarks. Also any other new features or code improvements. Send a PR, an email, or open an issue detailing what you'd like to add/change to begin a conversation.
+
+## Citing TrackEval
+
+If you use this code in your research, please use the following BibTeX entry:
+
+```BibTeX
+@misc{luiten2020trackeval,
+ author = {Jonathon Luiten, Arne Hoffhues},
+ title = {TrackEval},
+ howpublished = {\url{https://github.com/JonathonLuiten/TrackEval}},
+ year = {2020}
+}
+```
+
+Furthermore, if you use the HOTA metrics, please cite the following paper:
+
+```
+@article{luiten2020IJCV,
+ title={HOTA: A Higher Order Metric for Evaluating Multi-Object Tracking},
+ author={Luiten, Jonathon and Osep, Aljosa and Dendorfer, Patrick and Torr, Philip and Geiger, Andreas and Leal-Taix{\'e}, Laura and Leibe, Bastian},
+ journal={International Journal of Computer Vision},
+ pages={1--31},
+ year={2020},
+ publisher={Springer}
+}
+```
+
+If you use any other metrics please also cite the relevant papers, and don't forget to cite each of the benchmarks you evaluate on.
diff --git a/val_utils/docs/BDD100k-format.txt b/val_utils/docs/BDD100k-format.txt
new file mode 100644
index 0000000000000000000000000000000000000000..3939aa3b6e595996ffcaf37aada27590a679b05c
--- /dev/null
+++ b/val_utils/docs/BDD100k-format.txt
@@ -0,0 +1,95 @@
+Taken from: https://bdd-data.berkeley.edu/wad-2020.html
+
+BDD100K MOT Dataset
+
+To advance the study on multiple object tracking, we introduce BDD100K MOT Dataset. We provide 1,400 video sequences for training, 200 video sequences for validation and 400 video sequences for testing. Each video sequence is about 40 seconds long with 5 FPS resulting in approximately 200 frames per video.
+
+BDD100K MOT Dataset is not only diverse in visual scale among and within tracks, but in the temporal range of each track. Objects in the BDD100K MOT dataset also present complicated occlusion and reappearing patterns. An object may be fully occluded or move out of the frame, and then reappear later. BDD100K MOT Dataset shows real challenges of object re-identification for tracking in autonomous driving. Details about the MOT dataset can be found in the BDD100K paper (https://arxiv.org/abs/1805.04687). Access the BDD100K data website (https://bdd-data.berkeley.edu/) to download the data.
+
+Folder Structure
+bdd100k/
+├── images/
+| ├── track/
+| | ├── train/
+| | | ├── $VIDEO_NAME/
+| | | | ├── $VIDEO_NAME-$FRAME_INDEX.jpg
+| | ├── val/
+| | ├── test/
+├── labels-20/
+| ├── box-track/
+| | ├── train/
+| | | ├── $VIDEO_NAME.json
+| | | |
+| | ├── val/
+The frames for each video are stored in a folder in the images directory. The labels for each video are stored in a json file with the format detailed below.
+
+Label Format
+Each json file contains a list of frame objects, and each frame object has the format below. The format follows the schema of BDD100K data format (https://github.com/ucbdrive/bdd100k/blob/master/doc/format.md).
+
+- name: string
+- videoName: string
+- index: int
+- labels: [ ]
+ - id: string
+ - category: string
+ - attributes:
+ - Crowd: boolean
+ - Occluded: boolean
+ - Truncated: boolean
+ - box2d:
+ - x1: float
+ - y1: float
+ - x2: float
+ - y2: float
+There are 11 object categories in this release:
+
+pedestrian
+rider
+other person
+car
+bus
+truck
+train
+trailer
+other vehicle
+motorcycle
+bicycle
+
+Notes:
+The same instance shares "id" across frames.
+The "pedestrian", "bicycle", and "motorcycle" correspond to the "person", "bike", and "motor" classes in the BDD100K Detection dataset.
+We consider "other person", "trailer", and "other vehicle" as distractors, which are ignored during evaluation. We only evaluate the multi-object tracking of the other 8 categories.
+We set three super-categories: "person" (with classes "pedestrian" and"rider"), "vehicle" ("car", "bus", "truck", and "train"), and "bike" ("motorcycle" and "bicycle") for the purpose of evaluation.
+
+Submission Format
+The submission file for each of the two phases is a json file compressed by zip. Each json file is a list of frame objects with the format detailed below. The format also follows the schema of BDD100K data format (https://github.com/ucbdrive/bdd100k/blob/master/doc/format.md).
+
+- name: string
+- labels [ ]:
+ - id: string
+ - category: string
+ - box2d:
+ - x1: float
+ - y1: float
+ - x2: float
+ - y2: float
+
+Note that objects with the same identity share id across frames in a given video, and should be unique across different videos. Our evaluation will match the category string in evaluation, so you can assign your own integer ID for the categories in your model. But we recommend to encode the 8 relevant categories in the following order so that it is easier for the research community to share the models.
+
+pedestrian
+rider
+car
+truck
+bus
+train
+motorcycle
+bicycle
+
+The evaluation server will perform evaluation for each category and aggregate the results to compute the overall metrics. Then the server will merge both the ground-truth and predicted labels into super-categories and evaluate for each super- category.
+
+Evaluation
+Evaluation platform: We host our evaluation server on CodaLab (https://competitions.codalab.org/competitions/24492). There are two phases for the challenge: val phase and test phase. The final ranking will be based on the test phase.
+Pre-training: It is a fair game to pre-train your network with ImageNet or COCO, but if other datasets are used, please note in the submission description. We will rank the methods without using external datasets except ImageNet and COCO.
+Ignoring distractors: As a preprocessing step, all predicted boxes are matched and the ones matched to distractor ground-truth boxes ("other person", "trailer", and "other vehicle") are ignored.
+Crowd region: After bounding box matching, we ignore all detected false-positive boxes that has >50% overlap with the crowd region (ground-truth boxes with the "Crowd" attribute).
+Super-category: In addition to the evaluation of all 8 classes, we merge ground truth and prediction categories into 3 super-categories specified above, and evaluate the results for each super-category. The super-category evaluation results will be provided only for the purpose of reference.
\ No newline at end of file
diff --git a/val_utils/docs/DAVIS-format.txt b/val_utils/docs/DAVIS-format.txt
new file mode 100644
index 0000000000000000000000000000000000000000..7e29dbd6a30e2c51168fd07c10da16e9b02278eb
--- /dev/null
+++ b/val_utils/docs/DAVIS-format.txt
@@ -0,0 +1,28 @@
+Annotation Format:
+
+
+The annotations in each frame are stored in png format.
+This png is stored indexed i.e. it has a single channel and each pixel has a value from 0 to 254 that corresponds to a color palette attached to the png file.
+It is important to take this into account when decoding the png i.e. the output of decoding should be a single channel image and it should not be necessary to do any remap from RGB to indexes.
+The latter is crucial to preseve the index of each object so it can match to the correct object in evaluation.
+
+Each pixel that belongs to the same object has the same value in this png map through the whole video.
+Start at 1 for the first object, then 2, 3, 4 etc.
+The background (not an object) has value 0.
+Also note that invalid/void pixels are stored with a 254 value.
+
+
+These can be read like this:
+
+import PIL.Image as Image
+img = np.array(Image.open("000005.png"))
+
+
+or like this:
+
+ann_data = tf.read_file(ann_filename)
+ann = tf.image.decode_image(ann_data, dtype=tf.uint8, channels=1)
+
+
+See the code for loading the davis dataset for more details.
+
diff --git a/val_utils/docs/How_To/Add_a_new_metric.md b/val_utils/docs/How_To/Add_a_new_metric.md
new file mode 100644
index 0000000000000000000000000000000000000000..7a3d12c59657f3197808f65e7714cbb472786a5c
--- /dev/null
+++ b/val_utils/docs/How_To/Add_a_new_metric.md
@@ -0,0 +1,11 @@
+# How to add a new or custom family of evaluation metrics to TrackEval
+
+ - Create your metrics code in ```trackeval/metrics/.py```.
+ - It's probably easiest to start by copying an existing metrics code and editing it, e.g. ```trackeval/metrics/identity.py``` is probably the simplest.
+ - Your metric should be class, and it should inherit from the ```trackeval.metrics._base_metric._BaseMetric``` class.
+ - Define an ```__init__``` function that defines the different ```fields``` (values) that your metric will calculate. See ```trackeval/metrics/_base_metric.py``` for a list of currently used field types. Feel free to add new types.
+ - Define your code to actually calculate your metric for a single sequence and single class in a function called ```eval_sequence```, which takes a data dictionary as input, and returns a results dictionary as output.
+ - Define functions for how to combine your metric field values over a) sequences ```combine_sequences```, b) over classes ```combine_classes_class_averaged```, and c) over classes weighted by the number of detections ```combine_classes_det_averaged```.
+ - We find using a function such as the ```_compute_final_fields``` function that we use in the current metrics is convienient because it is likely used for metrics calculation and for the different metric combination, however this is not required.
+ - Register your new metric by adding it to ```trackeval/metrics/init.py```
+ - Your new metric can be used by passing the metrics class to a list of metrics which is passed to the evaluator (see files in ```scripts/*```).
diff --git a/val_utils/docs/KITTI-format.txt b/val_utils/docs/KITTI-format.txt
new file mode 100644
index 0000000000000000000000000000000000000000..bfe6d6368a57cdefd6d326a3b35b8bc4e982895a
--- /dev/null
+++ b/val_utils/docs/KITTI-format.txt
@@ -0,0 +1,160 @@
+Taken from download link found at: http://www.cvlibs.net/datasets/kitti/eval_tracking.php
+
+###########################################################################
+# THE KITTI VISION BENCHMARK SUITE: TRACKING BENCHMARK #
+# Andreas Geiger Philip Lenz Raquel Urtasun #
+# Karlsruhe Institute of Technology #
+# Toyota Technological Institute at Chicago #
+# www.cvlibs.net #
+###########################################################################
+
+For recent updates see http://www.cvlibs.net/datasets/kitti/eval_tracking.php.
+
+This file describes the KITTI tracking benchmarks, consisting of 21
+training sequences and 29 test sequences.
+
+Despite the fact that we have labeled 8 different classes, only the classes
+'Car' and 'Pedestrian' are evaluated in our benchmark, as only for those
+classes enough instances for a comprehensive evaluation have been labeled.
+
+The labeling process has been performed in two steps: First we hired a set
+of annotators, to label 3D bounding boxes for tracklets in 3D Velodyne
+point clouds. Since for a pedestrian tracklet, a single 3D bounding box
+tracklet (dimensions have been fixed) often fits badly, we additionally
+labeled the left/right boundaries of each object by making use of Mechanical
+Turk. We also collected labels of the object's occlusion state, and computed
+the object's truncation via backprojecting a car/pedestrian model into the
+image plane.
+
+NOTE: WHEN SUBMITTING RESULTS, PLEASE STORE THEM IN THE SAME DATA FORMAT IN
+WHICH THE GROUND TRUTH DATA IS PROVIDED (SEE BELOW), USING THE FILE NAMES
+0000.txt 0001.txt ... CREATE A ZIP ARCHIVE OF THEM AND STORE YOUR
+RESULTS (ONLY THE RESULTS OF THE TEST SET) IN ITS ROOT FOLDER. FOR A
+RE-SUBMISSION, _ONLY_ THE RE-SUBMITTED RESULTS WILL BE SHOWN IN THE TABLE.
+
+Data Format Description
+=======================
+
+The data for training and testing can be found in the corresponding folders.
+The sub-folders are structured as follows:
+
+ - image_02/%04d/ contains the left color camera sequence images (png)
+ - image_03/%04d/ contains the right color camera sequence images (png)
+ - label_02/ contains the left color camera label files (plain text files)
+ - calib/ contains the calibration for all four cameras (plain text files)
+
+The label files contain the following information.
+All values (numerical or strings) are separated via spaces, each row
+corresponds to one object. The 17 columns represent:
+
+#Values Name Description
+----------------------------------------------------------------------------
+ 1 frame Frame within the sequence where the object appearers
+ 1 track id Unique tracking id of this object within this sequence
+ 1 type Describes the type of object: 'Car', 'Van', 'Truck',
+ 'Pedestrian', 'Person_sitting', 'Cyclist', 'Tram',
+ 'Misc' or 'DontCare'
+ 1 truncated Integer (0,1,2) indicating the level of truncation.
+ Note that this is in contrast to the object detection
+ benchmark where truncation is a float in [0,1].
+ 1 occluded Integer (0,1,2,3) indicating occlusion state:
+ 0 = fully visible, 1 = partly occluded
+ 2 = largely occluded, 3 = unknown
+ 1 alpha Observation angle of object, ranging [-pi..pi]
+ 4 bbox 2D bounding box of object in the image (0-based index):
+ contains left, top, right, bottom pixel coordinates
+ 3 dimensions 3D object dimensions: height, width, length (in meters)
+ 3 location 3D object location x,y,z in camera coordinates (in meters)
+ 1 rotation_y Rotation ry around Y-axis in camera coordinates [-pi..pi]
+ 1 score Only for results: Float, indicating confidence in
+ detection, needed for p/r curves, higher is better.
+
+
+Here, 'DontCare' labels denote regions in which objects have not been labeled,
+for example because they have been too far away from the laser scanner. To
+prevent such objects from being counted as false positives our evaluation
+script will ignore objects tracked in don't care regions of the test set.
+You can use the don't care labels in the training set to avoid that your object
+detector/tracking algorithm is harvesting hard negatives from those areas,
+in case you consider non-object regions from the training images as negative
+examples.
+
+The reference point for the 3D bounding box for each object is centered on the
+bottom face of the box. The corners of bounding box are computed as follows with
+respect to the reference point and in the object coordinate system:
+x_corners = [l/2, l/2, -l/2, -l/2, l/2, l/2, -l/2, -l/2]^T
+y_corners = [0, 0, 0, 0, -h, -h, -h, -h ]^T
+z_corners = [w/2, -w/2, -w/2, w/2, w/2, -w/2, -w/2, w/2 ]^T
+with l=length, h=height, and w=width.
+
+The coordinates in the camera coordinate system can be projected in the image
+by using the 3x4 projection matrix in the calib folder, where for the left
+color camera for which the images are provided, P2 must be used. The
+difference between rotation_y and alpha is, that rotation_y is directly
+given in camera coordinates, while alpha also considers the vector from the
+camera center to the object center, to compute the relative orientation of
+the object with respect to the camera. For example, a car which is facing
+along the X-axis of the camera coordinate system corresponds to rotation_y=0,
+no matter where it is located in the X/Z plane (bird's eye view), while
+alpha is zero only, when this object is located along the Z-axis of the
+camera. When moving the car away from the Z-axis, the observation angle
+(\alpha) will change.
+
+An overview of the coordinate systems, reference point and geometrical
+definitions is given in cs_overview.pdf.
+
+To project a point from Velodyne coordinates into the left color image,
+you can use this formula: x = P2 * R0_rect * Tr_velo_to_cam * y
+For the right color image: x = P3 * R0_rect * Tr_velo_to_cam * y
+
+Note: All matrices are stored row-major, i.e., the first values correspond
+to the first row. R0_rect contains a 3x3 matrix which you need to extend to
+a 4x4 matrix by adding a 1 as the bottom-right element and 0's elsewhere.
+Tr_xxx is a 3x4 matrix (R|t), which you need to extend to a 4x4 matrix
+in the same way!
+
+The sensors were not moved between the different days while taking footage.
+However, the full camera calibration was performed for every day separately.
+Therefore, only 'Tr_imu_velo' is constant for all sequences.
+
+Note that while all this information is available for the training data,
+only the data which is actually needed for the particular benchmark must
+be provided to the evaluation server. However, all 17 values must be provided
+at all times, with the unused ones set to their default values (=invalid).
+Additionally a 18'th value must be provided
+with a floating value of the score for a particular tracked detection, where
+higher indicates higher confidence in the detection. The range of your scores
+will be automatically determined by our evaluation server, you don't have to
+normalize it, but it should be roughly linear.
+
+Tracking Benchmark
+==================
+
+The goal in the object tracking task is to estimate object tracklets for the
+classes 'Car', 'Pedestrian', and (optional) 'Cyclist'. The tracking
+algorithm must provide as output the 2D 0-based bounding boxes in each image in
+the sequence using the format specified above, as well as a score, indicating
+the confidence in the particular frame for this track. All other values must be
+set to their default values (=invalid), see above. One text file per sequence
+must be provided in a zip archive, where each file can contain many detections,
+depending on the number of objects per sequence. In our evaluation we only
+evaluate detections/objects larger than 25 pixel (height) in the image and do
+not count Vans as false positives for cars or Sitting Persons as wrong positives
+for Pedestrians due to their similarity in appearance. (All ignored objects
+are considered as DontCare areas.) As evaluation criterion we follow the
+HOTA, CLEARMOT and Mostly-Tracked/Partly-Tracked/Mostly-Lost metrics.
+
+Raw Data
+========
+
+Raw data is mapped to the tracking benchmark sequences and available for
+download.
+
+The velodyne and positioning data for training and testing can be found in the
+corresponding folders. The sub-folders are structured as follows:
+
+ - velodyne/%04d/ contains the raw velodyne point clouds (binary file)
+ - oxts/ contains the raw position (oxts) data (plain text files)
+
+
+
diff --git a/val_utils/docs/MOTChallenge-Official/Readme.md b/val_utils/docs/MOTChallenge-Official/Readme.md
new file mode 100644
index 0000000000000000000000000000000000000000..502b504ac16e7a31c56f721675ad206d4b0e53c2
--- /dev/null
+++ b/val_utils/docs/MOTChallenge-Official/Readme.md
@@ -0,0 +1,221 @@
+
+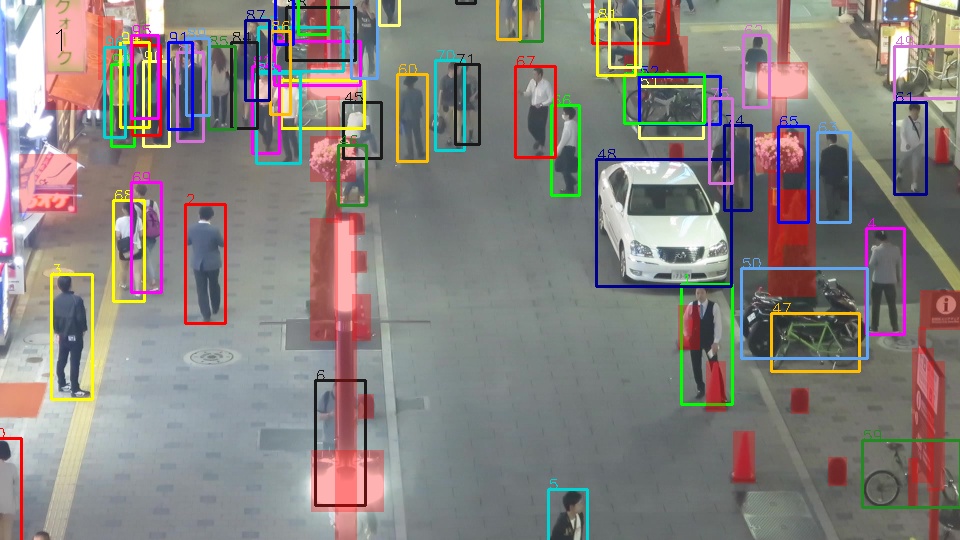
+# MOTChallenge Official Evaluation Kit - Multi-Object Tracking - MOT15, MOT16, MOT17, MOT20
+
+TrackEval is now the Official Evaluation Kit for MOTChallenge.
+
+This repository contains the evaluation scripts for the MOT challenges available at www.MOTChallenge.net.
+
+This codebase replaces the previous version that used to be accessible at https://github.com/dendorferpatrick/MOTChallengeEvalKit and is no longer maintained.
+
+Challenge Name | Data url |
+|----- | ----------- |
+|2D MOT 15| https://motchallenge.net/data/MOT15/ |
+|MOT 16| https://motchallenge.net/data/MOT16/ |
+|MOT 17| https://motchallenge.net/data/MOT17/ |
+|MOT 20| https://motchallenge.net/data/MOT20/ |
+
+## Requirements
+* Python (3.5 or newer)
+* Numpy and Scipy
+
+## Directories and Data
+The easiest way to get started is to simply download the TrackEval example data from here: [data.zip](https://omnomnom.vision.rwth-aachen.de/data/TrackEval/data.zip) (~150mb).
+
+This contains all the ground-truth, example trackers and meta-data that you will need.
+
+Extract this zip into the repository root folder such that the file paths look like: TrackEval/data/gt/...
+
+## Evaluation
+To run the evaluation for your method please run the script at ```TrackEval/scripts/run_mot_challenge.py```.
+
+Some of the basic arguments are described below. For more arguments, please see the script itself.
+
+```BENCHMARK```: Name of the benchmark, e.g. MOT15, MO16, MOT17 or MOT20 (default : MOT17)
+
+```SPLIT_TO_EVAL```: Data split on which to evalute e.g. train, test (default : train)
+
+```TRACKERS_TO_EVAL```: List of tracker names for which you wish to run evaluation. e.g. MPNTrack (default: all trackers in tracker folder)
+
+```METRICS```: List of metric families which you wish to compute. e.g. HOTA CLEAR Identity VACE (default: HOTA CLEAR Identity)
+
+```USE_PARALLEL```: Whether to run evaluation in parallel on multiple cores. (default: False)
+
+```NUM_PARALLEL_CORES```: Number of cores to use when running in parallel. (default: 8)
+
+An example is below (this will work on the supplied example data above):
+```
+python scripts/run_mot_challenge.py --BENCHMARK MOT17 --SPLIT_TO_EVAL train --TRACKERS_TO_EVAL MPNTrack --METRICS HOTA CLEAR Identity VACE --USE_PARALLEL False --NUM_PARALLEL_CORES 1
+```
+
+
+## Data Format
+
+The tracker file format should be the same as the ground truth file,
+which is a CSV text-file containing one object instance per line.
+Each line must contain 10 values:
+
+
+
+
+<frame>,
+<id>,
+<bb_left>,
+<bb_top>,
+<bb_width>,
+<bb_height>,
+<conf>,
+<x>,
+<y>,
+<z>
+
+
+
+The world coordinates x,y,z
+are ignored for the 2D challenge and can be filled with -1.
+Similarly, the bounding boxes are ignored for the 3D challenge.
+However, each line is still required to contain 10 values.
+
+All frame numbers, target IDs and bounding boxes are 1-based. Here is an example:
+
+
+
+
+## Evaluating on your own Data
+The repository also allows you to include your own datasets and evaluate your method on your own challenge ``````. To do so, follow these two steps:
+***1. Ground truth data preparation***
+Prepare your sequences in directory ```TrackEval/data/gt/mot_challenge/``` following this structure:
+
+```
+.
+|——
+ |—— gt
+ |—— gt.txt
+ |—— seqinfo.ini
+|——
+ |—— ……
+|——
+ |—— …...
+```
+
+***2. Sequence file***
+Create text files containing the sequence names; ```-train.txt```, ```-test.txt```, ```-test.txt``` inside the ```seqmaps``` folder, e.g.:
+```-all.txt```
+```
+name
+
+
+
+```
+
+```-train.txt```
+```
+name
+
+
+```
+
+```-test.txt```
+```
+name
+
+```
+
+
+To run the evaluation for your method adjust the file ```scripts/run_mot_challenge.py``` and set ```BENCHMARK = ```
+
+
+## Citation
+If you work with the code and the benchmark, please cite:
+
+***TrackEval***
+```
+@misc{luiten2020trackeval,
+ author = {Jonathon Luiten, Arne Hoffhues},
+ title = {TrackEval},
+ howpublished = {\url{https://github.com/JonathonLuiten/TrackEval}},
+ year = {2020}
+}
+```
+***MOTChallenge Journal***
+```
+@article{dendorfer2020motchallenge,
+ title={MOTChallenge: A Benchmark for Single-camera Multiple Target Tracking},
+ author={Dendorfer, Patrick and Osep, Aljosa and Milan, Anton and Schindler, Konrad and Cremers, Daniel and Reid, Ian and Roth, Stefan and Leal-Taix{\'e}, Laura},
+ journal={International Journal of Computer Vision},
+ pages={1--37},
+ year={2020},
+ publisher={Springer}
+}
+```
+***MOT 15***
+```
+@article{MOTChallenge2015,
+ title = {{MOTC}hallenge 2015: {T}owards a Benchmark for Multi-Target Tracking},
+ shorttitle = {MOTChallenge 2015},
+ url = {http://arxiv.org/abs/1504.01942},
+ journal = {arXiv:1504.01942 [cs]},
+ author = {Leal-Taix\'{e}, L. and Milan, A. and Reid, I. and Roth, S. and Schindler, K.},
+ month = apr,
+ year = {2015},
+ note = {arXiv: 1504.01942},
+ keywords = {Computer Science - Computer Vision and Pattern Recognition}
+}
+```
+***MOT 16, MOT 17***
+```
+@article{MOT16,
+ title = {{MOT}16: {A} Benchmark for Multi-Object Tracking},
+ shorttitle = {MOT16},
+ url = {http://arxiv.org/abs/1603.00831},
+ journal = {arXiv:1603.00831 [cs]},
+ author = {Milan, A. and Leal-Taix\'{e}, L. and Reid, I. and Roth, S. and Schindler, K.},
+ month = mar,
+ year = {2016},
+ note = {arXiv: 1603.00831},
+ keywords = {Computer Science - Computer Vision and Pattern Recognition}
+}
+```
+***MOT 20***
+```
+@article{MOTChallenge20,
+ title={MOT20: A benchmark for multi object tracking in crowded scenes},
+ shorttitle = {MOT20},
+ url = {http://arxiv.org/abs/1906.04567},
+ journal = {arXiv:2003.09003[cs]},
+ author = {Dendorfer, P. and Rezatofighi, H. and Milan, A. and Shi, J. and Cremers, D. and Reid, I. and Roth, S. and Schindler, K. and Leal-Taix\'{e}, L. },
+ month = mar,
+ year = {2020},
+ note = {arXiv: 2003.09003},
+ keywords = {Computer Science - Computer Vision and Pattern Recognition}
+}
+```
+***HOTA metrics***
+```
+@article{luiten2020IJCV,
+ title={HOTA: A Higher Order Metric for Evaluating Multi-Object Tracking},
+ author={Luiten, Jonathon and Osep, Aljosa and Dendorfer, Patrick and Torr, Philip and Geiger, Andreas and Leal-Taix{\'e}, Laura and Leibe, Bastian},
+ journal={International Journal of Computer Vision},
+ pages={1--31},
+ year={2020},
+ publisher={Springer}
+}
+```
+
+## Feedback and Contact
+We are constantly working on improving our benchmark to provide the best performance to the community.
+You can help us to make the benchmark better by open issues in the repo and reporting bugs.
+
+For general questions, please contact one of the following:
+
+```
+Patrick Dendorfer - patrick.dendorfer@tum.de
+Jonathon Luiten - luiten@vision.rwth-aachen.de
+Aljosa Osep - aljosa.osep@tum.de
+```
+
diff --git a/val_utils/docs/MOTChallenge-format.txt b/val_utils/docs/MOTChallenge-format.txt
new file mode 100644
index 0000000000000000000000000000000000000000..5a49c72aaafa242b4b900466b5ec994278671518
--- /dev/null
+++ b/val_utils/docs/MOTChallenge-format.txt
@@ -0,0 +1,37 @@
+Taken from: https://motchallenge.net/instructions/
+
+File Format
+
+Please submit your results as a single .zip file. The results for each sequence must be stored in a separate .txt file in the archive's root folder. The file name must be exactly like the sequence name (case sensitive).
+
+The file format should be the same as the ground truth file, which is a CSV text-file containing one object instance per line. Each line must contain 10 values:
+
+, , , , , , , , ,
+The conf value contains the detection confidence in the det.txt files. For the ground truth, it acts as a flag whether the entry is to be considered. A value of 0 means that this particular instance is ignored in the evaluation, while any other value can be used to mark it as active. For submitted results, all lines in the .txt file are considered. The world coordinates x,y,z are ignored for the 2D challenge and can be filled with -1. Similarly, the bounding boxes are ignored for the 3D challenge. However, each line is still required to contain 10 values.
+
+All frame numbers, target IDs and bounding boxes are 1-based. Here is an example:
+
+Tracking with bounding boxes
+(MOT15, MOT16, MOT17, MOT20)
+ 1, 3, 794.27, 247.59, 71.245, 174.88, -1, -1, -1, -1
+ 1, 6, 1648.1, 119.61, 66.504, 163.24, -1, -1, -1, -1
+ 1, 8, 875.49, 399.98, 95.303, 233.93, -1, -1, -1, -1
+ ...
+
+Multi Object Tracking & Segmentation
+(MOTS Challenge)
+Each line of an annotation txt file is structured like this (where rle means run-length encoding from COCO):
+
+time_frame id class_id img_height img_width rle
+An example line from a txt file:
+
+52 1005 1 375 1242 WSV:2d;1O10000O10000O1O100O100O1O100O1000000000000000O100O102N5K00O1O1N2O110OO2O001O1NTga3
+Meaning:
+time frame 52
+object id 1005 (meaning class id is 1, i.e. car and instance id is 5)
+class id 1
+image height 375
+image width 1242
+rle WSV:2d;1O10000O10000O1O100O100O1O100O1000000000000000O100O...1O1N
+
+image height, image width, and rle can be used together to decode a mask using cocotools(https://github.com/cocodataset/cocoapi) .
\ No newline at end of file
diff --git a/val_utils/docs/MOTS-format.txt b/val_utils/docs/MOTS-format.txt
new file mode 100644
index 0000000000000000000000000000000000000000..49244443beded2c4b62e34d8d13dd85d5e222bb3
--- /dev/null
+++ b/val_utils/docs/MOTS-format.txt
@@ -0,0 +1,45 @@
+Taken from: https://www.vision.rwth-aachen.de/page/mots
+
+
+Annotation Format
+We provide two alternative and equivalent formats, one encoded as png images, and one encoded as txt files. The txt files are smaller, and faster to be read in, but the cocotools are needed to decode the masks. For code to read the annotations also see mots_tools/blob/master/mots_common/io.py
+
+Note that in both formats an id value of 10,000 denotes an ignore region and 0 is background. The class id can be obtained by floor divison of the object id by 1000 (class_id = obj_id // 1000) and the instance id can be obtained by the object id modulo 1000 (instance_id = obj_id % 1000). The object ids are consistent over time.
+
+The class ids are the following
+
+car 1
+pedestrian 2
+png format
+The png format has a single color channel with 16 bits and can for example be read like this:
+
+import PIL.Image as Image
+img = np.array(Image.open("000005.png"))
+obj_ids = np.unique(img)
+# to correctly interpret the id of a single object
+obj_id = obj_ids[0]
+class_id = obj_id // 1000
+obj_instance_id = obj_id % 1000
+When using a TensorFlow input pipeline for reading the annotations, you can use
+
+ann_data = tf.read_file(ann_filename)
+ann = tf.image.decode_image(ann_data, dtype=tf.uint16, channels=1)
+
+
+txt format
+Each line of an annotation txt file is structured like this (where rle means run-length encoding from COCO):
+
+time_frame id class_id img_height img_width rle
+An example line from a txt file:
+
+52 1005 1 375 1242 WSV:2d;1O10000O10000O1O100O100O1O100O1000000000000000O100O102N5K00O1O1N2O110OO2O001O1NTga3
+Which means
+
+time frame 52
+object id 1005 (meaning class id is 1, i.e. car and instance id is 5)
+class id 1
+image height 375
+image width 1242
+rle WSV:2d;1O10000O10000O1O100O100O1O100O1000000000000000O100O...1O1N
+
+image height, image width, and rle can be used together to decode a mask using cocotools.
\ No newline at end of file
diff --git a/val_utils/docs/OpenWorldTracking-Official/Readme.md b/val_utils/docs/OpenWorldTracking-Official/Readme.md
new file mode 100644
index 0000000000000000000000000000000000000000..30588c28576ea02cc71b40863884ccf717243d8b
--- /dev/null
+++ b/val_utils/docs/OpenWorldTracking-Official/Readme.md
@@ -0,0 +1,49 @@
+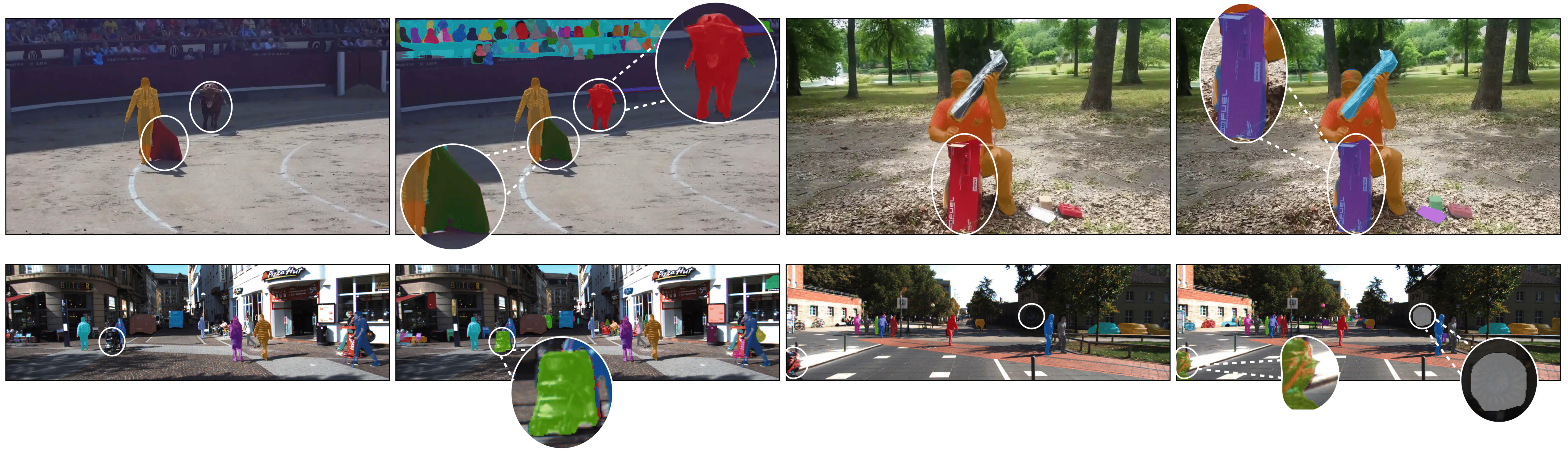
+# Opening Up Open-World Tracking - Official Evaluation Code
+
+TrackEval now contains the official evalution code for evaluating the task of **Open World Tracking**.
+
+This is the official code from the following paper:
+
+
Opening up Open-World Tracking
+Yang Liu*, Idil Esen Zulfikar*, Jonathon Luiten*, Achal Dave*, Deva Ramanan, Bastian Leibe, Aljoša Ošep, Laura Leal-Taixé
+*Equal contribution
+CVPR 2022
+
+[Paper](https://arxiv.org/abs/2104.11221)
+
+[Website](https://openworldtracking.github.io)
+
+## Running and understanding the code
+
+The code can be run by running the following script (see script for arguments and how to run):
+[TAO-OW run script](https://github.com/JonathonLuiten/TrackEval/blob/master/scripts/run_tao_ow.py)
+
+To understand the the data is being read and used, see the TAO-OW dataset class:
+[TAO-OW dataset class](https://github.com/JonathonLuiten/TrackEval/blob/master/trackeval/datasets/tao_ow.py)
+
+The implementation of the 'Open World Tracking Accuracy' (OWTA) metric proposed in the paper can be found here:
+[OWTA metric](https://github.com/JonathonLuiten/TrackEval/blob/master/trackeval/metrics/hota.py)
+
+## Citation
+If you work with the code and the benchmark, please cite:
+
+***Opening Up Open-World Tracking***
+```
+@inproceedings{liu2022opening,
+ title={Opening up Open-World Tracking},
+ author={Liu, Yang and Zulfikar, Idil Esen and Luiten, Jonathon and Dave, Achal and Ramanan, Deva and Leibe, Bastian and O{\v{s}}ep, Aljo{\v{s}}a and Leal-Taix{\'e}, Laura},
+ journal={Proceedings of the IEEE/CVF Conference on Computer Vision and Pattern Recognition},
+ year={2022}
+}
+```
+
+***TrackEval***
+```
+@misc{luiten2020trackeval,
+ author = {Jonathon Luiten, Arne Hoffhues},
+ title = {TrackEval},
+ howpublished = {\url{https://github.com/JonathonLuiten/TrackEval}},
+ year = {2020}
+}
+```
diff --git a/val_utils/docs/RobMOTS-Official/Readme.md b/val_utils/docs/RobMOTS-Official/Readme.md
new file mode 100644
index 0000000000000000000000000000000000000000..4cb225604d0fad600534d833f420757034f460ad
--- /dev/null
+++ b/val_utils/docs/RobMOTS-Official/Readme.md
@@ -0,0 +1,240 @@
+[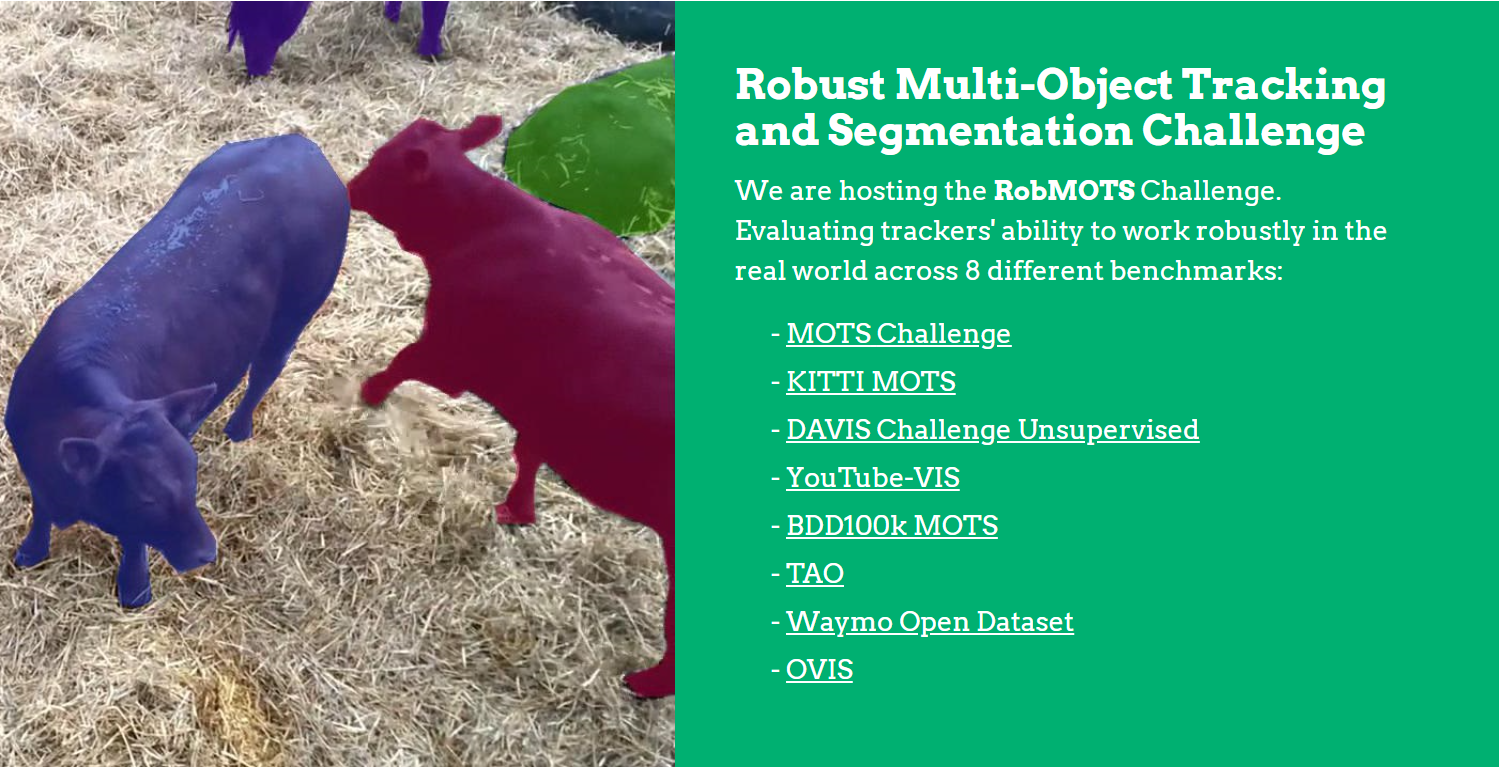](https://eval.vision.rwth-aachen.de/rvsu-workshop21/?page_id=110)
+
+# RobMOTS Official Evaluation Code
+
+### NEWS: [RobMOTS Challenge](https://eval.vision.rwth-aachen.de/rvsu-workshop21/?page_id=110) for the [RVSU CVPR'21 Workshop](https://eval.vision.rwth-aachen.de/rvsu-workshop21/) is now live!!!! Challenge deadline June 15.
+
+### NEWS: [Call for short papers](https://eval.vision.rwth-aachen.de/rvsu-workshop21/?page_id=74) (4 pages) on tracking and other video topics for [RVSU CVPR'21 Workshop](https://eval.vision.rwth-aachen.de/rvsu-workshop21/)!!!! Paper deadline June 4.
+
+TrackEval is now the Official Evaluation Kit for the RobMOTS Challenge.
+
+This repository contains the official evaluation code for the challenges available at the [RobMOTS Website](https://eval.vision.rwth-aachen.de/rvsu-workshop21/?page_id=110).
+
+The RobMOTS Challenge tests trackers' ability to work robustly across 8 different benchmarks, while tracking the [80 categories of objects from COCO](https://cocodataset.org/#explore).
+
+The following benchmarks are included:
+
+Benchmark | Website |
+|----- | ----------- |
+|MOTS Challenge| https://motchallenge.net/results/MOTS/ |
+|KITTI-MOTS| http://www.cvlibs.net/datasets/kitti/eval_mots.php |
+|DAVIS Challenge Unsupervised| https://davischallenge.org/challenge2020/unsupervised.html |
+|YouTube-VIS| https://youtube-vos.org/dataset/vis/ |
+|BDD100k MOTS| https://bdd-data.berkeley.edu/ |
+|TAO| https://taodataset.org/ |
+|Waymo Open Dataset| https://waymo.com/open/ |
+|OVIS| http://songbai.site/ovis/ |
+
+## Installing, obtaining the data, and running
+
+Simply follow the code snippet below to install the evaluation code, download the train groundtruth data and an example tracker, and run the evaluation code on the sample tracker.
+
+Note the code requires python 3.5 or higher.
+
+```
+# Download the TrackEval repo
+git clone https://github.com/JonathonLuiten/TrackEval.git
+
+# Move to repo folder
+cd TrackEval
+
+# Create a virtual env in the repo for evaluation
+python3 -m venv ./venv
+
+# Activate the virtual env
+source venv/bin/activate
+
+# Update pip to have the latest version of packages
+pip install --upgrade pip
+
+# Install the required packages
+pip install -r requirements.txt
+
+# Download the train gt data
+wget https://omnomnom.vision.rwth-aachen.de/data/RobMOTS/train_gt.zip
+
+# Unzip the train gt data you just downloaded.
+unzip train_gt.zip
+
+# Download the example tracker
+wget https://omnomnom.vision.rwth-aachen.de/data/RobMOTS/example_tracker.zip
+
+# Unzip the example tracker you just downloaded.
+unzip example_tracker.zip
+
+# Run the evaluation on the provided example tracker on the train split (using 4 cores in parallel)
+python scripts/run_rob_mots.py --ROBMOTS_SPLIT train --TRACKERS_TO_EVAL STP --USE_PARALLEL True --NUM_PARALLEL_CORES 4
+
+```
+
+You may further download the raw sequence images and supplied detections (as well as train GT data and example tracker) by following the ```Data Download``` link here:
+
+[RobMOTS Challenge Info](https://eval.vision.rwth-aachen.de/rvsu-workshop21/?page_id=110)
+
+## Accessing tracking evaluation results
+
+You will find the results of the evaluation (for the supplied tracker STP) in the folder ```TrackEval/data/trackers/rob_mots/train/STP/```.
+The overall summary of the results is in ```./final_results.csv```, and more detailed results per sequence and per class and results plots can be found under ```./results/*```.
+
+The ```final_results.csv``` can be most easily read by opening it in Excel or similar. The ```c```, ```d``` and ```f``` prepending the metric names refer respectively to ```class averaged```, ```detection averaged (class agnostic)``` and ```final``` (the geometric mean of class and detection averaged).
+
+## Supplied Detections
+
+To make creating your own tracker particularly easy, we supply a set of strong supplied detection.
+
+These detections are from the Detectron 2 Mask R-CNN X152 (very bottom model on this [page](https://github.com/facebookresearch/detectron2/blob/master/MODEL_ZOO.md) which achieves a COCO detection mAP score of 50.2).
+
+We then obtain segmentation masks for these detections using the Box2Seg Network (also called Refinement Net), which results in far more accurate masks than the default Mask R-CNN masks. The code for this can be found [here](https://github.com/JonathonLuiten/PReMVOS/tree/master/code/refinement_net).
+
+We supply two different supplied detections. The first is the ```raw_supplied``` detections, which is taking all 1000 detections output from the Mask R-CNN, and only removing those for which the maximum class score is less than 0.02 (here no non-maximum suppression, NMS, is run). These can be downloaded [here](https://eval.vision.rwth-aachen.de/rvsu-workshop21/?page_id=110).
+
+The second is ```non_overlap_supplied``` detections. These are the same detections as above, but with further processing steps applied to them. First we perform Non-Maximum Suppression (NMS) with a threshold of 0.5 to remove any masks which have an IoU of 0.5 or more with any other mask that has a higher score. Second we run a Non-Overlap algorithm which forces all of the masks for a single image to be non-overlapping. It does this by putting all the masks 'on top of' each other, ordered by score, such that masks with a lower score will be partially removed if a mask with a higher score partially overlaps them. Note that these detections are still only thresholded at a score of 0.02, in general we recommend further thresholding with a higher value to get a good balance of precision and recall.
+
+Code for this NMS and Non-Overlap algorithm can be found here:
+[Non-Overlap Code](https://github.com/JonathonLuiten/TrackEval/blob/master/trackeval/baselines/non_overlap.py).
+
+Note that for RobMOTS evaluation the final tracking results need to be 'non-overlapping' so we recommend using the ```non_overlap_supplied``` detections, however you may use the ```raw_supplied```, or your own or any other detections as you like.
+
+Supplied detections (both raw and non-overlapping) are available for the train, val and test sets.
+
+Example code for reading in these detections and using them can be found here:
+
+[Tracker Example](https://github.com/JonathonLuiten/TrackEval/blob/master/trackeval/baselines/stp.py).
+
+## Creating your own tracker
+
+We provide sample code ([Tracker Example](https://github.com/JonathonLuiten/TrackEval/blob/master/trackeval/baselines/stp.py)) for our STP tracker (Simplest Tracker Possible) which walks though how to create tracking results in the required RobMOTS format.
+
+This includes code for reading in the supplied detections and writing out the tracking results in the desired format, plus many other useful functions (IoU calculation etc).
+
+## Evaluating your own tracker
+
+To evaluate your tracker, put the results in the folder ```TrackEval/data/trackers/rob_mots/train/```, in a folder alongside the supplied tracker STP with the folder labelled as your tracker name, e.g. YOUR_TRACKER.
+
+You can then run the evaluation code on your tracker like this:
+
+```
+python scripts/run_rob_mots.py --ROBMOTS_SPLIT train --TRACKERS_TO_EVAL YOUR_TRACKER --USE_PARALLEL True --NUM_PARALLEL_CORES 4
+```
+
+## Data format
+
+For RobMOTS, trackers must submit their results in the following folder format:
+
+```
+|——
+ |—— .txt
+ |—— .txt
+ |—— .txt
+|——
+ |—— .txt
+ |—— .txt
+ |—— .txt
+```
+
+See the supplied STP tracker results (in the Train Data linked above) for an example.
+
+Thus there is one .txt file for each sequence. This file has one row per detection (object mask in one frame). Each row must have 7 values and has the following format:
+
+
+
+<Timestep>(int),
+<Track ID>(int),
+<Class Number>(int),
+<Detection Confidence>(float),
+<Image Height>(int),
+<Image Width>(int),
+<Compressed RLE Mask>(string),
+
+
+
+Timesteps are the same as the frame names for the supplied images. These start at 0.
+
+Track IDs must be unique across all classes within a frame. They can be non-unique across different sequences.
+
+The mapping of class numbers to class names can be found is [this file](https://github.com/JonathonLuiten/TrackEval/blob/master/trackeval/datasets/rob_mots_classmap.py). Note that this is the same as used in Detectron 2, and is the default COCO class ordering with the unused numbers removed.
+
+Detection Confidence score should be between 0 and 1. This is not used for HOTA evaluation, but is used for other eval metrics like Track mAP.
+
+Image height and width are needed to decode the compressed RLE mask representation.
+
+The Compressed RLE Mask is the same format used by coco, pycocotools and mots.
+
+An example of a tracker result file looks like this:
+
+```
+0 1 3 0.9917707443237305 1200 1920 VaTi0b0lT17F8K3M3N1O1N2O0O2M3N2N101O1O1O01O1O0100O100O01O1O100O10O1000O1000000000000000O1000001O0000000000000000O101O00000000000001O0000010O0110O0O100O1O2N1O2N0O2O2M3M2N2O1O2N5J;DgePZ1
+0 2 3 0.989478349685669 1200 1920 Ql^c05ZU12O2N001O0O10OTkNIaT17^kNKaT15^kNLbT14^kNMaT13^kNOaT11_kN0`T10_kN1`T11_kN0`T11_kN0`T1a0O00001O1O1O3M;E5K3M2N000000000O100000000000000000001O00001O2N1O1O1O000001O001O0O2O0O2M3M3M3N2O1O1O1N2O002N1O2N10O02N10000O1O101M3N2N2M7H^_g_1
+1 2 3 0.964085042476654 1200 1920 o_Uc03\U12O1O1N102N002N001O1O000O2O1O00002N6J1O001O2N1O3L3N2N4L5K2N1O000000000000001O1O2N01O01O010O01N2O0O2O1M4L3N2N101N2O001O1O100O0100000O1O1O1O2N6I4Mdm^`1
+```
+
+Note that for the evaluation to be valid, the masks must not overlap within one frame.
+
+The supplied detections have the same format (but with all the Track IDs being set to 0).
+
+The groundtruth data for most benchmarks is in the exact same format as above (usually Detection Confidence is set to 1.0). The exception is the few benchmarks for which the ground-truth is not segmentation masks but bounding boxes (Waymo and TAO). For these the last three columns are not there (height, width and mask) as these encode a mask, and instead there are 4 columns encoding the bounding box co-ordinates in the format ```x0 y0 x1 y1```, where x0 and y0 are the coordinates of the top left of the box and x1 and y0 are the coordinates for the bottom right.
+
+The groundtruth can also contain ignore regions. The are marked by being having a class number of 100 or larger. Class number 100 encodes and ignore region for all class, which class numbers higher than 100 encode ignore regions specific to each class. E.g. class number 105 are ignore regions for class 5.
+
+As well as the per sequence files described above, the groundtruth for each benchmark contains two more files ```clsmap.txt``` and ```seqmap.txt```.
+
+```clsmap.txt``` is a single row, space-separated, containing all of the valid classes that should be evaluated for each benchmark (not all benchmarks evaluate all of the coco classes).
+
+```seqmap.txt``` contains a list of the sequences to be evaluated for that benchmark. Each row has at least 4 values. These are:
+```
+
+```
+More than 4 values can be present, the remaining values are 'ignore classes for this sequence'. E.g. classes which are evaluated for the particular benchmark as a whole, but should be ignored for this sequence.
+
+## Visualizing GT and Tracker Masks
+
+We provide code for converting our .txt format with compressed RLE masks into .png format where it is easy to visualize the GT and Predicted masks.
+
+This code can be found here:
+
+[Vizualize Tracking Results](https://github.com/JonathonLuiten/TrackEval/blob/master/trackeval/baselines/vizualize.py).
+
+
+## Evaluate on the validation and test server
+
+The val and test GT will NOT be provided. However we provide a live evaluation server to upload your tracking results and evaluate it on the val and test set.
+
+The val server will allow infinite uploads, while the test will limit trackers to 4 uploads total.
+
+These evaluation servers can be found here: https://eval.vision.rwth-aachen.de/vision/
+
+Ensure that your files to upload are in the correct format. Examples of the correct way to upload files can be found here: [STP val upload](https://omnomnom.vision.rwth-aachen.de/data/RobMOTS/STP_val_upload.zip), [STP test upload](https://omnomnom.vision.rwth-aachen.de/data/RobMOTS/STP_test_upload.zip).
+
+## Citation
+If you work with the code and the benchmark, please cite:
+
+***TrackEval***
+```
+@misc{luiten2020trackeval,
+ author = {Jonathon Luiten, Arne Hoffhues},
+ title = {TrackEval},
+ howpublished = {\url{https://github.com/JonathonLuiten/TrackEval}},
+ year = {2020}
+}
+```
+***HOTA metrics***
+```
+@article{luiten2020IJCV,
+ title={HOTA: A Higher Order Metric for Evaluating Multi-Object Tracking},
+ author={Luiten, Jonathon and Osep, Aljosa and Dendorfer, Patrick and Torr, Philip and Geiger, Andreas and Leal-Taix{\'e}, Laura and Leibe, Bastian},
+ journal={International Journal of Computer Vision},
+ pages={1--31},
+ year={2020},
+ publisher={Springer}
+}
+```
+
+## Feedback and Contact
+We are constantly working on improving RobMOTS, and wish to provide the most useful support to the community.
+You can help us to make the benchmark better by open issues in the repo and reporting bugs.
+
+For general questions, please contact the following:
+
+```
+Jonathon Luiten - luiten@vision.rwth-aachen.de
+```
diff --git a/val_utils/docs/TAO-format.txt b/val_utils/docs/TAO-format.txt
new file mode 100644
index 0000000000000000000000000000000000000000..a1896742f53a286b1d48b6a2ce7b47825c372011
--- /dev/null
+++ b/val_utils/docs/TAO-format.txt
@@ -0,0 +1,56 @@
+Taken from: https://github.com/TAO-Dataset/tao/blob/master/tao/toolkit/tao/tao.py
+
+Annotation file format:
+{
+ "info" : info,
+ "images" : [image],
+ "videos": [video],
+ "tracks": [track],
+ "annotations" : [annotation],
+ "categories": [category],
+ "licenses" : [license],
+}
+info: As in MS COCO
+image: {
+ "id" : int,
+ "video_id": int,
+ "file_name" : str,
+ "license" : int,
+ # Redundant fields for COCO-compatibility
+ "width": int,
+ "height": int,
+ "frame_index": int
+}
+video: {
+ "id": int,
+ "name": str,
+ "width" : int,
+ "height" : int,
+ "neg_category_ids": [int],
+ "not_exhaustive_category_ids": [int],
+ "metadata": dict, # Metadata about the video
+}
+track: {
+ "id": int,
+ "category_id": int,
+ "video_id": int
+}
+category: {
+ "id": int,
+ "name": str,
+ "synset": str, # For non-LVIS objects, this is "unknown"
+ ... [other fields copied from LVIS v0.5 and unused]
+}
+annotation: {
+ "image_id": int,
+ "track_id": int,
+ "bbox": [x,y,width,height],
+ "area": float,
+ # Redundant field for compatibility with COCO scripts
+ "category_id": int
+}
+license: {
+ "id" : int,
+ "name" : str,
+ "url" : str,
+}
diff --git a/val_utils/docs/YouTube-VIS-format.txt b/val_utils/docs/YouTube-VIS-format.txt
new file mode 100644
index 0000000000000000000000000000000000000000..b5d542d5c8f9358dcd4d975cd7da8bbf99215256
--- /dev/null
+++ b/val_utils/docs/YouTube-VIS-format.txt
@@ -0,0 +1,44 @@
+Taken from: https://competitions.codalab.org/competitions/20128#participate-get-data
+
+The label file follows MSCOCO's style in json format. We adapt the entry name and label format for video. The definition of json file is:
+
+
+ {
+ "info" : info,
+ "videos" : [video],
+ "annotations" : [annotation],
+ "categories" : [category],
+ }
+ video{
+ "id" : int,
+ "width" : int,
+ "height" : int,
+ "length" : int,
+ "file_names" : [file_name],
+ }
+ annotation{
+ "id" : int,
+ "video_id" : int,
+ "category_id" : int,
+ "segmentations" : [RLE or [polygon] or None],
+ "areas" : [float or None],
+ "bboxes" : [[x,y,width,height] or None],
+ "iscrowd" : 0 or 1,
+ }
+ category{
+ "id" : int,
+ "name" : str,
+ "supercategory" : str,
+ }
+
+The submission file is also in json format. The file should contain a list of predictions:
+
+
+ prediction{
+ "video_id" : int,
+ "category_id" : int,
+ "segmentations" : [RLE or [polygon] or None],
+ "score" : float,
+ }
+
+The submission file should be named as "results.json", and compressed without any subfolder. There is an example "valid_submission_sample.zip" in download links above. The example is generated by our proposed MaskTrack R-CNN algorithm.
\ No newline at end of file
diff --git a/val_utils/minimum_requirements.txt b/val_utils/minimum_requirements.txt
new file mode 100644
index 0000000000000000000000000000000000000000..b63c322a857a3638f77c77a107f0cc4a814977e3
--- /dev/null
+++ b/val_utils/minimum_requirements.txt
@@ -0,0 +1,2 @@
+scipy==1.4.1
+numpy==1.18.1
diff --git a/val_utils/pyproject.toml b/val_utils/pyproject.toml
new file mode 100644
index 0000000000000000000000000000000000000000..374b58cbf4636f1e28bacf987ac2fe89ed27ccba
--- /dev/null
+++ b/val_utils/pyproject.toml
@@ -0,0 +1,6 @@
+[build-system]
+requires = [
+ "setuptools>=42",
+ "wheel"
+]
+build-backend = "setuptools.build_meta"
diff --git a/val_utils/requirements.txt b/val_utils/requirements.txt
new file mode 100644
index 0000000000000000000000000000000000000000..40d2a57c81d960e89adcb6ad7ea4d6509888a85e
--- /dev/null
+++ b/val_utils/requirements.txt
@@ -0,0 +1,10 @@
+numpy==1.18.1
+scipy==1.4.1
+pycocotools==2.0.2
+matplotlib==3.2.1
+opencv_python==4.4.0.46
+scikit_image==0.16.2
+pytest==6.0.1
+Pillow==8.1.2
+tqdm==4.64.0
+tabulate
diff --git a/val_utils/scripts/comparison_plots.py b/val_utils/scripts/comparison_plots.py
new file mode 100644
index 0000000000000000000000000000000000000000..a338f97c68a81d19fb1827085ddaffd18c825dea
--- /dev/null
+++ b/val_utils/scripts/comparison_plots.py
@@ -0,0 +1,20 @@
+import sys
+import os
+
+sys.path.insert(0, os.path.abspath(os.path.join(os.path.dirname(__file__), '..')))
+import trackeval # noqa: E402
+
+plots_folder = os.path.abspath(os.path.join(os.path.dirname(__file__), '..', 'data', 'plots'))
+tracker_folder = os.path.abspath(os.path.join(os.path.dirname(__file__), '..', 'data', 'trackers'))
+
+# dataset = os.path.join('kitti', 'kitti_2d_box_train')
+# classes = ['cars', 'pedestrian']
+
+dataset = os.path.join('mot_challenge', 'MOT17-train')
+classes = ['pedestrian']
+
+data_fol = os.path.join(tracker_folder, dataset)
+trackers = os.listdir(data_fol)
+out_loc = os.path.join(plots_folder, dataset)
+for cls in classes:
+ trackeval.plotting.plot_compare_trackers(data_fol, trackers, cls, out_loc)
diff --git a/val_utils/scripts/run_bdd.py b/val_utils/scripts/run_bdd.py
new file mode 100644
index 0000000000000000000000000000000000000000..2043cdf085803e3954cceaabb19f3b5b2ecd8876
--- /dev/null
+++ b/val_utils/scripts/run_bdd.py
@@ -0,0 +1,89 @@
+
+""" run_bdd.py
+
+Run example:
+run_bdd.py --USE_PARALLEL False --METRICS Hota --TRACKERS_TO_EVAL qdtrack
+
+Command Line Arguments: Defaults, # Comments
+ Eval arguments:
+ 'USE_PARALLEL': False,
+ 'NUM_PARALLEL_CORES': 8,
+ 'BREAK_ON_ERROR': True,
+ 'PRINT_RESULTS': True,
+ 'PRINT_ONLY_COMBINED': False,
+ 'PRINT_CONFIG': True,
+ 'TIME_PROGRESS': True,
+ 'OUTPUT_SUMMARY': True,
+ 'OUTPUT_DETAILED': True,
+ 'PLOT_CURVES': True,
+ Dataset arguments:
+ 'GT_FOLDER': os.path.join(code_path, 'data/gt/bdd100k/bdd100k_val'), # Location of GT data
+ 'TRACKERS_FOLDER': os.path.join(code_path, 'data/trackers/bdd100k/bdd100k_val'), # Trackers location
+ 'OUTPUT_FOLDER': None, # Where to save eval results (if None, same as TRACKERS_FOLDER)
+ 'TRACKERS_TO_EVAL': None, # Filenames of trackers to eval (if None, all in folder)
+ 'CLASSES_TO_EVAL': ['pedestrian', 'rider', 'car', 'bus', 'truck', 'train', 'motorcycle', 'bicycle'],
+ # Valid: ['pedestrian', 'rider', 'car', 'bus', 'truck', 'train', 'motorcycle', 'bicycle']
+ 'SPLIT_TO_EVAL': 'val', # Valid: 'training', 'val',
+ 'INPUT_AS_ZIP': False, # Whether tracker input files are zipped
+ 'PRINT_CONFIG': True, # Whether to print current config
+ 'TRACKER_SUB_FOLDER': 'data', # Tracker files are in TRACKER_FOLDER/tracker_name/TRACKER_SUB_FOLDER
+ 'OUTPUT_SUB_FOLDER': '', # Output files are saved in OUTPUT_FOLDER/tracker_name/OUTPUT_SUB_FOLDER
+ 'TRACKER_DISPLAY_NAMES': None, # Names of trackers to display, if None: TRACKERS_TO_EVAL
+ Metric arguments:
+ 'METRICS': ['Hota','Clear', 'ID', 'Count']
+"""
+
+import sys
+import os
+import argparse
+from multiprocessing import freeze_support
+
+sys.path.insert(0, os.path.abspath(os.path.join(os.path.dirname(__file__), '..')))
+import trackeval # noqa: E402
+
+if __name__ == '__main__':
+ freeze_support()
+
+ # Command line interface:
+ default_eval_config = trackeval.Evaluator.get_default_eval_config()
+ default_eval_config['PRINT_ONLY_COMBINED'] = True
+ default_dataset_config = trackeval.datasets.BDD100K.get_default_dataset_config()
+ default_metrics_config = {'METRICS': ['HOTA', 'CLEAR', 'Identity']}
+ config = {**default_eval_config, **default_dataset_config, **default_metrics_config} # Merge default configs
+ parser = argparse.ArgumentParser()
+ for setting in config.keys():
+ if type(config[setting]) == list or type(config[setting]) == type(None):
+ parser.add_argument("--" + setting, nargs='+')
+ else:
+ parser.add_argument("--" + setting)
+ args = parser.parse_args().__dict__
+ for setting in args.keys():
+ if args[setting] is not None:
+ if type(config[setting]) == type(True):
+ if args[setting] == 'True':
+ x = True
+ elif args[setting] == 'False':
+ x = False
+ else:
+ raise Exception('Command line parameter ' + setting + 'must be True or False')
+ elif type(config[setting]) == type(1):
+ x = int(args[setting])
+ elif type(args[setting]) == type(None):
+ x = None
+ else:
+ x = args[setting]
+ config[setting] = x
+ eval_config = {k: v for k, v in config.items() if k in default_eval_config.keys()}
+ dataset_config = {k: v for k, v in config.items() if k in default_dataset_config.keys()}
+ metrics_config = {k: v for k, v in config.items() if k in default_metrics_config.keys()}
+
+ # Run code
+ evaluator = trackeval.Evaluator(eval_config)
+ dataset_list = [trackeval.datasets.BDD100K(dataset_config)]
+ metrics_list = []
+ for metric in [trackeval.metrics.HOTA, trackeval.metrics.CLEAR, trackeval.metrics.Identity]:
+ if metric.get_name() in metrics_config['METRICS']:
+ metrics_list.append(metric())
+ if len(metrics_list) == 0:
+ raise Exception('No metrics selected for evaluation')
+ evaluator.evaluate(dataset_list, metrics_list)
\ No newline at end of file
diff --git a/val_utils/scripts/run_burst.py b/val_utils/scripts/run_burst.py
new file mode 100644
index 0000000000000000000000000000000000000000..595526436eb12c382123cf5ce4972e4aeb44a71f
--- /dev/null
+++ b/val_utils/scripts/run_burst.py
@@ -0,0 +1,173 @@
+""" run_burst.py
+
+The example commands given below expect the following folder structure:
+
+- data
+ - gt
+ - burst
+ - {val,test}
+ - all_classes
+ - all_classes.json (filename is irrelevant)
+ - trackers
+ - burst
+ - exemplar_guided
+ - {val,test}
+ - my_tracking_method
+ - data
+ - results.json (filename is irrelevant)
+ - class_guided
+ - {val,test}
+ - my_other_tracking_method
+ - data
+ - results.json (filename is irrelevant)
+
+Run example:
+
+1) Exemplar-guided tasks (all three tasks share the same eval logic):
+run_burst.py --USE_PARALLEL True --EXEMPLAR_GUIDED True --GT_FOLDER ../data/gt/burst/{val,test}/all_classes --TRACKERS_FOLDER ../data/trackers/burst/exemplar_guided/{val,test}
+
+2) Class-guided tasks (common class and long-tail):
+run_burst.py --USE_PARALLEL FTrue --EXEMPLAR_GUIDED False --GT_FOLDER ../data/gt/burst/{val,test}/all_classes --TRACKERS_FOLDER ../data/trackers/burst/class_guided/{val,test}
+
+3) Refer to run_burst_ow.py for open world evaluation
+
+Command Line Arguments: Defaults, # Comments
+ Eval arguments:
+ 'USE_PARALLEL': False,
+ 'NUM_PARALLEL_CORES': 8,
+ 'BREAK_ON_ERROR': True,
+ 'PRINT_RESULTS': True,
+ 'PRINT_ONLY_COMBINED': False,
+ 'PRINT_CONFIG': True,
+ 'TIME_PROGRESS': True,
+ 'OUTPUT_SUMMARY': True,
+ 'OUTPUT_DETAILED': True,
+ 'PLOT_CURVES': True,
+ Dataset arguments:
+ 'GT_FOLDER': os.path.join(code_path, 'data/gt/burst/val'), # Location of GT data
+ 'TRACKERS_FOLDER': os.path.join(code_path, 'data/trackers/burst/class-guided/'), # Trackers location
+ 'OUTPUT_FOLDER': None, # Where to save eval results (if None, same as TRACKERS_FOLDER)
+ 'TRACKERS_TO_EVAL': None, # Filenames of trackers to eval (if None, all in folder)
+ 'CLASSES_TO_EVAL': None, # Classes to eval (if None, all classes)
+ 'SPLIT_TO_EVAL': 'training', # Valid: 'training', 'val'
+ 'PRINT_CONFIG': True, # Whether to print current config
+ 'TRACKER_SUB_FOLDER': 'data', # Tracker files are in TRACKER_FOLDER/tracker_name/TRACKER_SUB_FOLDER
+ 'OUTPUT_SUB_FOLDER': '', # Output files are saved in OUTPUT_FOLDER/tracker_name/OUTPUT_SUB_FOLDER
+ 'TRACKER_DISPLAY_NAMES': None, # Names of trackers to display, if None: TRACKERS_TO_EVAL
+ 'MAX_DETECTIONS': 300, # Number of maximal allowed detections per image (0 for unlimited)
+ Metric arguments:
+ 'METRICS': ['HOTA', 'CLEAR', 'Identity', 'TrackMAP']
+"""
+
+import sys
+import os
+import argparse
+from tabulate import tabulate
+from multiprocessing import freeze_support
+
+sys.path.insert(0, os.path.abspath(os.path.join(os.path.dirname(__file__), '..')))
+import trackeval # noqa: E402
+
+
+def main():
+ freeze_support()
+
+ # Command line interface:
+ default_eval_config = trackeval.Evaluator.get_default_eval_config()
+ default_eval_config['PRINT_ONLY_COMBINED'] = True
+ default_eval_config['DISPLAY_LESS_PROGRESS'] = True
+ default_eval_config['PLOT_CURVES'] = False
+ default_eval_config["OUTPUT_DETAILED"] = False
+ default_eval_config["PRINT_RESULTS"] = False
+ default_eval_config["OUTPUT_SUMMARY"] = False
+
+ default_dataset_config = trackeval.datasets.BURST.get_default_dataset_config()
+
+ # default_metrics_config = {'METRICS': ['HOTA', 'CLEAR', 'Identity', 'TrackMAP']}
+ # default_metrics_config = {'METRICS': ['HOTA']}
+ default_metrics_config = {'METRICS': ['HOTA', 'TrackMAP']}
+ config = {**default_eval_config, **default_dataset_config, **default_metrics_config} # Merge default configs
+ parser = argparse.ArgumentParser()
+ for setting in config.keys():
+ if type(config[setting]) == list or type(config[setting]) == type(None):
+ parser.add_argument("--" + setting, nargs='+')
+ else:
+ parser.add_argument("--" + setting)
+ args = parser.parse_args().__dict__
+ for setting in args.keys():
+ if args[setting] is not None:
+ if type(config[setting]) == type(True):
+ if args[setting] == 'True':
+ x = True
+ elif args[setting] == 'False':
+ x = False
+ else:
+ raise Exception('Command line parameter ' + setting + 'must be True or False')
+ elif type(config[setting]) == type(1):
+ x = int(args[setting])
+ elif type(args[setting]) == type(None):
+ x = None
+ else:
+ x = args[setting]
+ config[setting] = x
+ eval_config = {k: v for k, v in config.items() if k in default_eval_config.keys()}
+ dataset_config = {k: v for k, v in config.items() if k in default_dataset_config.keys()}
+ metrics_config = {k: v for k, v in config.items() if k in default_metrics_config.keys()}
+
+ # Run code
+ evaluator = trackeval.Evaluator(eval_config)
+ dataset_list = [trackeval.datasets.BURST(dataset_config)]
+ metrics_list = []
+ for metric in [trackeval.metrics.TrackMAP, trackeval.metrics.CLEAR, trackeval.metrics.Identity,
+ trackeval.metrics.HOTA]:
+ if metric.get_name() in metrics_config['METRICS']:
+ metrics_list.append(metric())
+ if len(metrics_list) == 0:
+ raise Exception('No metrics selected for evaluation')
+ output_res, output_msg = evaluator.evaluate(dataset_list, metrics_list, show_progressbar=True)
+
+ class_name_to_id = {x['name']: x['id'] for x in dataset_list[0].gt_data['categories']}
+ known_list = [4, 13, 1038, 544, 1057, 34, 35, 36, 41, 45, 58, 60, 579, 1091, 1097, 1099, 78, 79, 81, 91, 1115,
+ 1117, 95, 1122, 99, 1132, 621, 1135, 625, 118, 1144, 126, 642, 1155, 133, 1162, 139, 154, 174, 185,
+ 699, 1215, 714, 717, 1229, 211, 729, 221, 229, 747, 235, 237, 779, 276, 805, 299, 829, 852, 347,
+ 371, 382, 896, 392, 926, 937, 428, 429, 961, 452, 979, 980, 982, 475, 480, 993, 1001, 502, 1018]
+
+ row_labels = ("HOTA", "DetA", "AssA", "AP")
+ trackers = list(output_res['BURST'].keys())
+ print("\n")
+
+ def average_metric(m):
+ return round(100*sum(m) / len(m), 2)
+
+ for tracker in trackers:
+ res = output_res['BURST'][tracker]['COMBINED_SEQ']
+ all_names = [x for x in res.keys() if (x != 'cls_comb_cls_av') and (x != 'cls_comb_det_av')]
+
+ class_split_names = {
+ "All": [x for x in res.keys() if (x != 'cls_comb_cls_av') and (x != 'cls_comb_det_av')],
+ "Common": [x for x in all_names if class_name_to_id[x] in known_list],
+ "Uncommon": [x for x in all_names if class_name_to_id[x] not in known_list]
+ }
+
+ # table columns: 'all', 'common', 'uncommon'
+ # table rows: HOTA, AssA, DetA, mAP
+ table_data = []
+
+ for row_label in row_labels:
+ row = [row_label]
+ for split_name in ["All", "Common", "Uncommon"]:
+ split_classes = class_split_names[split_name]
+
+ if row_label == "AP":
+ row.append(average_metric([res[c]['TrackMAP']["AP_all"].mean() for c in split_classes]))
+ else:
+ row.append(average_metric([res[c]['HOTA'][row_label].mean() for c in split_classes]))
+
+ table_data.append(row)
+
+ print(f"Results for Tracker: {tracker}\n")
+ print(tabulate(table_data, ["Metric", "All", "Common", "Uncommon"]))
+
+
+if __name__ == '__main__':
+ main()
diff --git a/val_utils/scripts/run_burst_ow.py b/val_utils/scripts/run_burst_ow.py
new file mode 100644
index 0000000000000000000000000000000000000000..3a941661f562046a9c7229417c3585edf6d19930
--- /dev/null
+++ b/val_utils/scripts/run_burst_ow.py
@@ -0,0 +1,117 @@
+""" run_burst_ow.py
+
+The example commands given below expect the following folder structure:
+
+- data
+ - gt
+ - burst
+ - {val,test}
+ - all_classes
+ - all_classes.json (filename is irrelevant)
+ - common_classes
+ - common_classes.json (filename is irrelevant)
+ - uncommon_classes.json
+ - uncommon_classes.json (filename is irrelevant)
+ - trackers
+ - burst
+ - open-world
+ - {val,test}
+ - my_tracking_method
+ - data
+ - results.json (filename is irrelevant)
+
+Run example:
+
+You'll need to run the eval script separately to get the OWTA metric for the three class splits. In the command below,
+replace with "common", "uncommon" and "all" to get the corresponding results.
+
+run_burst_ow.py --USE_PARALLEL True --GT_FOLDER data/gt/burst/{val,test}/_classes --TRACKERS_FOLDER data/trackers/burst/open-world/{val,test}
+
+Command Line Arguments: Defaults, # Comments
+ Eval arguments:
+ 'USE_PARALLEL': False,
+ 'NUM_PARALLEL_CORES': 8,
+ 'BREAK_ON_ERROR': True,
+ 'PRINT_RESULTS': True,
+ 'PRINT_ONLY_COMBINED': False,
+ 'PRINT_CONFIG': True,
+ 'TIME_PROGRESS': True,
+ 'OUTPUT_SUMMARY': True,
+ 'OUTPUT_DETAILED': True,
+ 'PLOT_CURVES': True,
+ Dataset arguments:
+ 'GT_FOLDER': os.path.join(code_path, '../data/gt/burst/{val,test}/_classes'), # Location of GT data
+ 'TRACKERS_FOLDER': os.path.join(code_path, '../data/trackers/burst/open-world/{val,test}), # Trackers location
+ 'OUTPUT_FOLDER': None, # Where to save eval results (if None, same as TRACKERS_FOLDER)
+ 'TRACKERS_TO_EVAL': None, # Filenames of trackers to eval (if None, all in folder)
+ 'CLASSES_TO_EVAL': None, # Classes to eval (if None, all classes)
+ 'SPLIT_TO_EVAL': 'training', # Valid: 'training', 'val'
+ 'PRINT_CONFIG': True, # Whether to print current config
+ 'TRACKER_SUB_FOLDER': 'data', # Tracker files are in TRACKER_FOLDER/tracker_name/TRACKER_SUB_FOLDER
+ 'OUTPUT_SUB_FOLDER': '', # Output files are saved in OUTPUT_FOLDER/tracker_name/OUTPUT_SUB_FOLDER
+ 'TRACKER_DISPLAY_NAMES': None, # Names of trackers to display, if None: TRACKERS_TO_EVAL
+ 'MAX_DETECTIONS': 300, # Number of maximal allowed detections per image (0 for unlimited)
+ 'SUBSET': 'unknown', # Evaluate on the following subsets ['all', 'known', 'unknown', 'distractor']
+ Metric arguments:
+ 'METRICS': ['HOTA', 'CLEAR', 'Identity', 'TrackMAP']
+"""
+
+import sys
+import os
+import argparse
+from multiprocessing import freeze_support
+
+sys.path.insert(0, os.path.abspath(os.path.join(os.path.dirname(__file__), '..')))
+import trackeval # noqa: E402
+
+if __name__ == '__main__':
+ freeze_support()
+
+ # Command line interface:
+ default_eval_config = trackeval.Evaluator.get_default_eval_config()
+ # print only combined since TrackMAP is undefined for per sequence breakdowns
+ default_eval_config['PRINT_ONLY_COMBINED'] = True
+ default_eval_config['DISPLAY_LESS_PROGRESS'] = True
+ default_dataset_config = trackeval.datasets.BURST_OW.get_default_dataset_config()
+ # default_metrics_config = {'METRICS': ['HOTA', 'CLEAR', 'Identity', 'TrackMAP']}
+ default_metrics_config = {'METRICS': ['HOTA']}
+ config = {**default_eval_config, **default_dataset_config, **default_metrics_config} # Merge default configs
+ parser = argparse.ArgumentParser()
+ for setting in config.keys():
+ if type(config[setting]) == list or type(config[setting]) == type(None):
+ parser.add_argument("--" + setting, nargs='+')
+ else:
+ parser.add_argument("--" + setting)
+ args = parser.parse_args().__dict__
+ for setting in args.keys():
+ if args[setting] is not None:
+ if type(config[setting]) == type(True):
+ if args[setting] == 'True':
+ x = True
+ elif args[setting] == 'False':
+ x = False
+ else:
+ raise Exception('Command line parameter ' + setting + 'must be True or False')
+ elif type(config[setting]) == type(1):
+ x = int(args[setting])
+ elif type(args[setting]) == type(None):
+ x = None
+ else:
+ x = args[setting]
+ config[setting] = x
+ eval_config = {k: v for k, v in config.items() if k in default_eval_config.keys()}
+ dataset_config = {k: v for k, v in config.items() if k in default_dataset_config.keys()}
+ metrics_config = {k: v for k, v in config.items() if k in default_metrics_config.keys()}
+
+ # Run code
+ evaluator = trackeval.Evaluator(eval_config)
+ dataset_list = [trackeval.datasets.BURST_OW(dataset_config)]
+ metrics_list = []
+ # for metric in [trackeval.metrics.TrackMAP, trackeval.metrics.CLEAR, trackeval.metrics.Identity,
+ # trackeval.metrics.HOTA]:
+ for metric in [trackeval.metrics.HOTA]:
+ if metric.get_name() in metrics_config['METRICS']:
+ metrics_list.append(metric())
+ if len(metrics_list) == 0:
+ raise Exception('No metrics selected for evaluation')
+ output_res, output_msg = evaluator.evaluate(dataset_list, metrics_list, show_progressbar=True)
diff --git a/val_utils/scripts/run_davis.py b/val_utils/scripts/run_davis.py
new file mode 100644
index 0000000000000000000000000000000000000000..2e26be2463526745b378842991524e6fb086af7f
--- /dev/null
+++ b/val_utils/scripts/run_davis.py
@@ -0,0 +1,90 @@
+""" run_davis.py
+
+Run example:
+run_davis.py --USE_PARALLEL False --METRICS HOTA --TRACKERS_TO_EVAL ags
+
+Command Line Arguments: Defaults, # Comments
+ Eval arguments:
+ 'USE_PARALLEL': False,
+ 'NUM_PARALLEL_CORES': 8,
+ 'BREAK_ON_ERROR': True,
+ 'PRINT_RESULTS': True,
+ 'PRINT_ONLY_COMBINED': False,
+ 'PRINT_CONFIG': True,
+ 'TIME_PROGRESS': True,
+ 'OUTPUT_SUMMARY': True,
+ 'OUTPUT_DETAILED': True,
+ 'PLOT_CURVES': True,
+ Dataset arguments:
+ ' 'GT_FOLDER': os.path.join(code_path, 'data/gt/davis/'), # Location of GT data
+ 'TRACKERS_FOLDER': os.path.join(code_path, 'data/trackers/davis/davis_val'), # Trackers location
+ 'OUTPUT_FOLDER': None, # Where to save eval results (if None, same as TRACKERS_FOLDER)
+ 'TRACKERS_TO_EVAL': None, # Filenames of trackers to eval (if None, all in folder)
+ 'SPLIT_TO_EVAL': 'val', # Valid: 'val', 'train'
+ 'PRINT_CONFIG': True, # Whether to print current config
+ 'TRACKER_SUB_FOLDER': 'data', # Tracker files are in TRACKER_FOLDER/tracker_name/TRACKER_SUB_FOLDER
+ 'OUTPUT_SUB_FOLDER': '', # Output files are saved in OUTPUT_FOLDER/tracker_name/OUTPUT_SUB_FOLDER
+ 'TRACKER_DISPLAY_NAMES': None, # Names of trackers to display, if None: TRACKERS_TO_EVAL
+ 'SEQMAP_FOLDER': None, # Where seqmaps are found (if None, GT_FOLDER/ImageSets/2017)
+ 'SEQMAP_FILE': None, # Directly specify seqmap file (if none use seqmap_folder/split-to-eval.txt)
+ 'SEQ_INFO': None, # If not None, directly specify sequences to eval and their number of timesteps
+ 'GT_LOC_FORMAT': '{gt_folder}/Annotations_unsupervised/480p/{seq}',
+ # '{gt_folder}/Annotations_unsupervised/480p/{seq}'
+ 'MAX_DETECTIONS': 0 # Maximum number of allowed detections per sequence (0 for no threshold)
+ Metric arguments:
+ 'METRICS': ['HOTA', 'CLEAR', 'Identity', 'JAndF']
+"""
+
+import sys
+import os
+import argparse
+from multiprocessing import freeze_support
+
+sys.path.insert(0, os.path.abspath(os.path.join(os.path.dirname(__file__), '..')))
+import trackeval # noqa: E402
+
+if __name__ == '__main__':
+ freeze_support()
+
+ # Command line interface:
+ default_eval_config = trackeval.Evaluator.get_default_eval_config()
+ default_dataset_config = trackeval.datasets.DAVIS.get_default_dataset_config()
+ default_metrics_config = {'METRICS': ['HOTA', 'CLEAR', 'Identity', 'JAndF']}
+ config = {**default_eval_config, **default_dataset_config, **default_metrics_config} # Merge default configs
+ parser = argparse.ArgumentParser()
+ for setting in config.keys():
+ if type(config[setting]) == list or type(config[setting]) == type(None):
+ parser.add_argument("--" + setting, nargs='+')
+ else:
+ parser.add_argument("--" + setting)
+ args = parser.parse_args().__dict__
+ for setting in args.keys():
+ if args[setting] is not None:
+ if type(config[setting]) == type(True):
+ if args[setting] == 'True':
+ x = True
+ elif args[setting] == 'False':
+ x = False
+ else:
+ raise Exception('Command line parameter ' + setting + 'must be True or False')
+ elif type(config[setting]) == type(1):
+ x = int(args[setting])
+ elif type(args[setting]) == type(None):
+ x = None
+ else:
+ x = args[setting]
+ config[setting] = x
+ eval_config = {k: v for k, v in config.items() if k in default_eval_config.keys()}
+ dataset_config = {k: v for k, v in config.items() if k in default_dataset_config.keys()}
+ metrics_config = {k: v for k, v in config.items() if k in default_metrics_config.keys()}
+
+ # Run code
+ evaluator = trackeval.Evaluator(eval_config)
+ dataset_list = [trackeval.datasets.DAVIS(dataset_config)]
+ metrics_list = []
+ for metric in [trackeval.metrics.HOTA, trackeval.metrics.CLEAR, trackeval.metrics.Identity, trackeval.metrics.JAndF]:
+ if metric.get_name() in metrics_config['METRICS']:
+ metrics_list.append(metric())
+ if len(metrics_list) == 0:
+ raise Exception('No metrics selected for evaluation')
+ evaluator.evaluate(dataset_list, metrics_list)
\ No newline at end of file
diff --git a/val_utils/scripts/run_headtracking_challenge.py b/val_utils/scripts/run_headtracking_challenge.py
new file mode 100644
index 0000000000000000000000000000000000000000..82dd597a2568ca60e956b6113f18068eea8d01c5
--- /dev/null
+++ b/val_utils/scripts/run_headtracking_challenge.py
@@ -0,0 +1,91 @@
+
+""" run_mot_challenge.py
+
+Run example:
+run_mot_challenge.py --USE_PARALLEL False --METRICS Hota --TRACKERS_TO_EVAL Lif_T
+
+Command Line Arguments: Defaults, # Comments
+ Eval arguments:
+ 'USE_PARALLEL': False,
+ 'NUM_PARALLEL_CORES': 8,
+ 'BREAK_ON_ERROR': True,
+ 'PRINT_RESULTS': True,
+ 'PRINT_ONLY_COMBINED': False,
+ 'PRINT_CONFIG': True,
+ 'TIME_PROGRESS': True,
+ 'OUTPUT_SUMMARY': True,
+ 'OUTPUT_DETAILED': True,
+ 'PLOT_CURVES': True,
+ Dataset arguments:
+ 'GT_FOLDER': os.path.join(code_path, 'data/gt/mot_challenge/'), # Location of GT data
+ 'TRACKERS_FOLDER': os.path.join(code_path, 'data/trackers/mot_challenge/'), # Trackers location
+ 'OUTPUT_FOLDER': None, # Where to save eval results (if None, same as TRACKERS_FOLDER)
+ 'TRACKERS_TO_EVAL': None, # Filenames of trackers to eval (if None, all in folder)
+ 'CLASSES_TO_EVAL': ['pedestrian'], # Valid: ['pedestrian']
+ 'BENCHMARK': 'MOT17', # Valid: 'MOT17', 'MOT16', 'MOT20', 'MOT15'
+ 'SPLIT_TO_EVAL': 'train', # Valid: 'train', 'test', 'all'
+ 'INPUT_AS_ZIP': False, # Whether tracker input files are zipped
+ 'PRINT_CONFIG': True, # Whether to print current config
+ 'DO_PREPROC': True, # Whether to perform preprocessing (never done for 2D_MOT_2015)
+ 'TRACKER_SUB_FOLDER': 'data', # Tracker files are in TRACKER_FOLDER/tracker_name/TRACKER_SUB_FOLDER
+ 'OUTPUT_SUB_FOLDER': '', # Output files are saved in OUTPUT_FOLDER/tracker_name/OUTPUT_SUB_FOLDER
+ Metric arguments:
+ 'METRICS': ['HOTA', 'CLEAR', 'Identity', 'IDEucl']
+"""
+
+import sys
+import os
+import argparse
+from multiprocessing import freeze_support
+
+sys.path.insert(0, os.path.abspath(os.path.join(os.path.dirname(__file__), '..')))
+import trackeval # noqa: E402
+
+if __name__ == '__main__':
+ freeze_support()
+
+ # Command line interface:
+ default_eval_config = trackeval.Evaluator.get_default_eval_config()
+ default_eval_config['DISPLAY_LESS_PROGRESS'] = False
+ default_dataset_config = trackeval.datasets.HeadTrackingChallenge.get_default_dataset_config()
+ default_metrics_config = {'METRICS': ['HOTA', 'CLEAR', 'Identity', 'IDEucl'], 'THRESHOLD': 0.4}
+ config = {**default_eval_config, **default_dataset_config, **default_metrics_config} # Merge default configs
+ parser = argparse.ArgumentParser()
+ for setting in config.keys():
+ if type(config[setting]) == list or type(config[setting]) == type(None):
+ parser.add_argument("--" + setting, nargs='+')
+ else:
+ parser.add_argument("--" + setting)
+ args = parser.parse_args().__dict__
+ for setting in args.keys():
+ if args[setting] is not None:
+ if type(config[setting]) == type(True):
+ if args[setting] == 'True':
+ x = True
+ elif args[setting] == 'False':
+ x = False
+ else:
+ raise Exception('Command line parameter ' + setting + 'must be True or False')
+ elif type(config[setting]) == type(1):
+ x = int(args[setting])
+ elif type(args[setting]) == type(None):
+ x = None
+ elif setting == 'SEQ_INFO':
+ x = dict(zip(args[setting], [None]*len(args[setting])))
+ else:
+ x = args[setting]
+ config[setting] = x
+ eval_config = {k: v for k, v in config.items() if k in default_eval_config.keys()}
+ dataset_config = {k: v for k, v in config.items() if k in default_dataset_config.keys()}
+ metrics_config = {k: v for k, v in config.items() if k in default_metrics_config.keys()}
+
+ # Run code
+ evaluator = trackeval.Evaluator(eval_config)
+ dataset_list = [trackeval.datasets.HeadTrackingChallenge(dataset_config)]
+ metrics_list = []
+ for metric in [trackeval.metrics.HOTA, trackeval.metrics.CLEAR, trackeval.metrics.Identity, trackeval.metrics.IDEucl]:
+ if metric.get_name() in metrics_config['METRICS']:
+ metrics_list.append(metric(metrics_config))
+ if len(metrics_list) == 0:
+ raise Exception('No metrics selected for evaluation')
+ evaluator.evaluate(dataset_list, metrics_list)
diff --git a/val_utils/scripts/run_kitti.py b/val_utils/scripts/run_kitti.py
new file mode 100644
index 0000000000000000000000000000000000000000..dbb63bde0a47ef51e4fe8c659c8de66ad4c8f29f
--- /dev/null
+++ b/val_utils/scripts/run_kitti.py
@@ -0,0 +1,87 @@
+
+""" run_kitti.py
+
+Run example:
+run_kitti.py --USE_PARALLEL False --METRICS Hota --TRACKERS_TO_EVAL CIWT
+
+Command Line Arguments: Defaults, # Comments
+ Eval arguments:
+ 'USE_PARALLEL': False,
+ 'NUM_PARALLEL_CORES': 8,
+ 'BREAK_ON_ERROR': True,
+ 'PRINT_RESULTS': True,
+ 'PRINT_ONLY_COMBINED': False,
+ 'PRINT_CONFIG': True,
+ 'TIME_PROGRESS': True,
+ 'OUTPUT_SUMMARY': True,
+ 'OUTPUT_DETAILED': True,
+ 'PLOT_CURVES': True,
+ Dataset arguments:
+ 'GT_FOLDER': os.path.join(code_path, 'data/gt/kitti/kitti_2d_box_train'), # Location of GT data
+ 'TRACKERS_FOLDER': os.path.join(code_path, 'data/trackers/kitti/kitti_2d_box_train/'), # Trackers location
+ 'OUTPUT_FOLDER': None, # Where to save eval results (if None, same as TRACKERS_FOLDER)
+ 'TRACKERS_TO_EVAL': None, # Filenames of trackers to eval (if None, all in folder)
+ 'CLASSES_TO_EVAL': ['car', 'pedestrian'], # Valid: ['car', 'pedestrian']
+ 'SPLIT_TO_EVAL': 'training', # Valid: 'training', 'val', 'training_minus_val', 'test'
+ 'INPUT_AS_ZIP': False, # Whether tracker input files are zipped
+ 'PRINT_CONFIG': True, # Whether to print current config
+ 'TRACKER_SUB_FOLDER': 'data', # Tracker files are in TRACKER_FOLDER/tracker_name/TRACKER_SUB_FOLDER
+ 'OUTPUT_SUB_FOLDER': '' # Output files are saved in OUTPUT_FOLDER/tracker_name/OUTPUT_SUB_FOLDER
+ Metric arguments:
+ 'METRICS': ['Hota','Clear', 'ID', 'Count']
+"""
+
+import sys
+import os
+import argparse
+from multiprocessing import freeze_support
+
+sys.path.insert(0, os.path.abspath(os.path.join(os.path.dirname(__file__), '..')))
+import trackeval # noqa: E402
+
+if __name__ == '__main__':
+ freeze_support()
+
+ # Command line interface:
+ default_eval_config = trackeval.Evaluator.get_default_eval_config()
+ default_eval_config['DISPLAY_LESS_PROGRESS'] = False
+ default_dataset_config = trackeval.datasets.Kitti2DBox.get_default_dataset_config()
+ default_metrics_config = {'METRICS': ['HOTA', 'CLEAR', 'Identity']}
+ config = {**default_eval_config, **default_dataset_config, **default_metrics_config} # Merge default configs
+ parser = argparse.ArgumentParser()
+ for setting in config.keys():
+ if type(config[setting]) == list or type(config[setting]) == type(None):
+ parser.add_argument("--" + setting, nargs='+')
+ else:
+ parser.add_argument("--" + setting)
+ args = parser.parse_args().__dict__
+ for setting in args.keys():
+ if args[setting] is not None:
+ if type(config[setting]) == type(True):
+ if args[setting] == 'True':
+ x = True
+ elif args[setting] == 'False':
+ x = False
+ else:
+ raise Exception('Command line parameter ' + setting + 'must be True or False')
+ elif type(config[setting]) == type(1):
+ x = int(args[setting])
+ elif type(args[setting]) == type(None):
+ x = None
+ else:
+ x = args[setting]
+ config[setting] = x
+ eval_config = {k: v for k, v in config.items() if k in default_eval_config.keys()}
+ dataset_config = {k: v for k, v in config.items() if k in default_dataset_config.keys()}
+ metrics_config = {k: v for k, v in config.items() if k in default_metrics_config.keys()}
+
+ # Run code
+ evaluator = trackeval.Evaluator(eval_config)
+ dataset_list = [trackeval.datasets.Kitti2DBox(dataset_config)]
+ metrics_list = []
+ for metric in [trackeval.metrics.HOTA, trackeval.metrics.CLEAR, trackeval.metrics.Identity]:
+ if metric.get_name() in metrics_config['METRICS']:
+ metrics_list.append(metric())
+ if len(metrics_list) == 0:
+ raise Exception('No metrics selected for evaluation')
+ evaluator.evaluate(dataset_list, metrics_list)
diff --git a/val_utils/scripts/run_kitti_mots.py b/val_utils/scripts/run_kitti_mots.py
new file mode 100644
index 0000000000000000000000000000000000000000..89d6b36a123ba3dc5d6230dceff57b3793e7368c
--- /dev/null
+++ b/val_utils/scripts/run_kitti_mots.py
@@ -0,0 +1,92 @@
+
+""" run_kitti_mots.py
+
+Run example:
+run_kitti_mots.py --USE_PARALLEL False --METRICS HOTA --TRACKERS_TO_EVAL trackrcnn
+
+Command Line Arguments: Defaults, # Comments
+ Eval arguments:
+ 'USE_PARALLEL': False,
+ 'NUM_PARALLEL_CORES': 8,
+ 'BREAK_ON_ERROR': True,
+ 'PRINT_RESULTS': True,
+ 'PRINT_ONLY_COMBINED': False,
+ 'PRINT_CONFIG': True,
+ 'TIME_PROGRESS': True,
+ 'OUTPUT_SUMMARY': True,
+ 'OUTPUT_DETAILED': True,
+ 'PLOT_CURVES': True,
+ Dataset arguments:
+ 'GT_FOLDER': os.path.join(code_path, 'data/gt/kitti/kitti_mots'), # Location of GT data
+ 'TRACKERS_FOLDER': os.path.join(code_path, 'data/trackers/kitti/kitti_mots_val'), # Location of all
+ # trackers
+ 'OUTPUT_FOLDER': None, # Where to save eval results (if None, same as TRACKERS_FOLDER)
+ 'TRACKERS_TO_EVAL': None, # Filenames of trackers to eval (if None, all in folder)
+ 'CLASSES_TO_EVAL': ['car', 'pedestrian'], # Valid: ['car', 'pedestrian']
+ 'SPLIT_TO_EVAL': 'val', # Valid: 'training', 'val'
+ 'INPUT_AS_ZIP': False, # Whether tracker input files are zipped
+ 'PRINT_CONFIG': True, # Whether to print current config
+ 'TRACKER_SUB_FOLDER': 'data', # Tracker files are in TRACKER_FOLDER/tracker_name/TRACKER_SUB_FOLDER
+ 'OUTPUT_SUB_FOLDER': '', # Output files are saved in OUTPUT_FOLDER/tracker_name/OUTPUT_SUB_FOLDER
+ 'SEQMAP_FOLDER': None, # Where seqmaps are found (if None, GT_FOLDER)
+ 'SEQMAP_FILE': None, # Directly specify seqmap file (if none use seqmap_folder/split_to_eval.seqmap)
+ 'SEQ_INFO': None, # If not None, directly specify sequences to eval and their number of timesteps
+ 'GT_LOC_FORMAT': '{gt_folder}/instances_txt/{seq}.txt', # format of gt localization
+ Metric arguments:
+ 'METRICS': ['HOTA', 'CLEAR', 'Identity']
+"""
+
+import sys
+import os
+import argparse
+from multiprocessing import freeze_support
+
+sys.path.insert(0, os.path.abspath(os.path.join(os.path.dirname(__file__), '..')))
+import trackeval # noqa: E402
+
+if __name__ == '__main__':
+ freeze_support()
+
+ # Command line interface:
+ default_eval_config = trackeval.Evaluator.get_default_eval_config()
+ default_eval_config['DISPLAY_LESS_PROGRESS'] = False
+ default_dataset_config = trackeval.datasets.KittiMOTS.get_default_dataset_config()
+ default_metrics_config = {'METRICS': ['HOTA', 'CLEAR', 'Identity']}
+ config = {**default_eval_config, **default_dataset_config, **default_metrics_config} # Merge default configs
+ parser = argparse.ArgumentParser()
+ for setting in config.keys():
+ if type(config[setting]) == list or type(config[setting]) == type(None):
+ parser.add_argument("--" + setting, nargs='+')
+ else:
+ parser.add_argument("--" + setting)
+ args = parser.parse_args().__dict__
+ for setting in args.keys():
+ if args[setting] is not None:
+ if type(config[setting]) == type(True):
+ if args[setting] == 'True':
+ x = True
+ elif args[setting] == 'False':
+ x = False
+ else:
+ raise Exception('Command line parameter ' + setting + 'must be True or False')
+ elif type(config[setting]) == type(1):
+ x = int(args[setting])
+ elif type(args[setting]) == type(None):
+ x = None
+ else:
+ x = args[setting]
+ config[setting] = x
+ eval_config = {k: v for k, v in config.items() if k in default_eval_config.keys()}
+ dataset_config = {k: v for k, v in config.items() if k in default_dataset_config.keys()}
+ metrics_config = {k: v for k, v in config.items() if k in default_metrics_config.keys()}
+
+ # Run code
+ evaluator = trackeval.Evaluator(eval_config)
+ dataset_list = [trackeval.datasets.KittiMOTS(dataset_config)]
+ metrics_list = []
+ for metric in [trackeval.metrics.HOTA, trackeval.metrics.CLEAR, trackeval.metrics.Identity, trackeval.metrics.JAndF]:
+ if metric.get_name() in metrics_config['METRICS']:
+ metrics_list.append(metric())
+ if len(metrics_list) == 0:
+ raise Exception('No metrics selected for evaluation')
+ evaluator.evaluate(dataset_list, metrics_list)
diff --git a/val_utils/scripts/run_mot_challenge.py b/val_utils/scripts/run_mot_challenge.py
new file mode 100644
index 0000000000000000000000000000000000000000..7b5a578a786e944e70fe1eafa6fb53d6d26f49c5
--- /dev/null
+++ b/val_utils/scripts/run_mot_challenge.py
@@ -0,0 +1,91 @@
+
+""" run_mot_challenge.py
+
+Run example:
+run_mot_challenge.py --USE_PARALLEL False --METRICS Hota --TRACKERS_TO_EVAL Lif_T
+
+Command Line Arguments: Defaults, # Comments
+ Eval arguments:
+ 'USE_PARALLEL': False,
+ 'NUM_PARALLEL_CORES': 8,
+ 'BREAK_ON_ERROR': True,
+ 'PRINT_RESULTS': True,
+ 'PRINT_ONLY_COMBINED': False,
+ 'PRINT_CONFIG': True,
+ 'TIME_PROGRESS': True,
+ 'OUTPUT_SUMMARY': True,
+ 'OUTPUT_DETAILED': True,
+ 'PLOT_CURVES': True,
+ Dataset arguments:
+ 'GT_FOLDER': os.path.join(code_path, 'data/gt/mot_challenge/'), # Location of GT data
+ 'TRACKERS_FOLDER': os.path.join(code_path, 'data/trackers/mot_challenge/'), # Trackers location
+ 'OUTPUT_FOLDER': None, # Where to save eval results (if None, same as TRACKERS_FOLDER)
+ 'TRACKERS_TO_EVAL': None, # Filenames of trackers to eval (if None, all in folder)
+ 'CLASSES_TO_EVAL': ['pedestrian'], # Valid: ['pedestrian']
+ 'BENCHMARK': 'MOT17', # Valid: 'MOT17', 'MOT16', 'MOT20', 'MOT15'
+ 'SPLIT_TO_EVAL': 'train', # Valid: 'train', 'test', 'all'
+ 'INPUT_AS_ZIP': False, # Whether tracker input files are zipped
+ 'PRINT_CONFIG': True, # Whether to print current config
+ 'DO_PREPROC': True, # Whether to perform preprocessing (never done for 2D_MOT_2015)
+ 'TRACKER_SUB_FOLDER': 'data', # Tracker files are in TRACKER_FOLDER/tracker_name/TRACKER_SUB_FOLDER
+ 'OUTPUT_SUB_FOLDER': '', # Output files are saved in OUTPUT_FOLDER/tracker_name/OUTPUT_SUB_FOLDER
+ Metric arguments:
+ 'METRICS': ['HOTA', 'CLEAR', 'Identity', 'VACE']
+"""
+
+import sys
+import os
+import argparse
+from multiprocessing import freeze_support
+
+sys.path.insert(0, os.path.abspath(os.path.join(os.path.dirname(__file__), '..')))
+import trackeval # noqa: E402
+
+if __name__ == '__main__':
+ freeze_support()
+
+ # Command line interface:
+ default_eval_config = trackeval.Evaluator.get_default_eval_config()
+ default_eval_config['DISPLAY_LESS_PROGRESS'] = False
+ default_dataset_config = trackeval.datasets.MotChallenge2DBox.get_default_dataset_config()
+ default_metrics_config = {'METRICS': ['HOTA', 'CLEAR', 'Identity'], 'THRESHOLD': 0.5}
+ config = {**default_eval_config, **default_dataset_config, **default_metrics_config} # Merge default configs
+ parser = argparse.ArgumentParser()
+ for setting in config.keys():
+ if type(config[setting]) == list or type(config[setting]) == type(None):
+ parser.add_argument("--" + setting, nargs='+')
+ else:
+ parser.add_argument("--" + setting)
+ args = parser.parse_args().__dict__
+ for setting in args.keys():
+ if args[setting] is not None:
+ if type(config[setting]) == type(True):
+ if args[setting] == 'True':
+ x = True
+ elif args[setting] == 'False':
+ x = False
+ else:
+ raise Exception('Command line parameter ' + setting + 'must be True or False')
+ elif type(config[setting]) == type(1):
+ x = int(args[setting])
+ elif type(args[setting]) == type(None):
+ x = None
+ elif setting == 'SEQ_INFO':
+ x = dict(zip(args[setting], [None]*len(args[setting])))
+ else:
+ x = args[setting]
+ config[setting] = x
+ eval_config = {k: v for k, v in config.items() if k in default_eval_config.keys()}
+ dataset_config = {k: v for k, v in config.items() if k in default_dataset_config.keys()}
+ metrics_config = {k: v for k, v in config.items() if k in default_metrics_config.keys()}
+
+ # Run code
+ evaluator = trackeval.Evaluator(eval_config)
+ dataset_list = [trackeval.datasets.MotChallenge2DBox(dataset_config)]
+ metrics_list = []
+ for metric in [trackeval.metrics.HOTA, trackeval.metrics.CLEAR, trackeval.metrics.Identity, trackeval.metrics.VACE]:
+ if metric.get_name() in metrics_config['METRICS']:
+ metrics_list.append(metric(metrics_config))
+ if len(metrics_list) == 0:
+ raise Exception('No metrics selected for evaluation')
+ evaluator.evaluate(dataset_list, metrics_list)
diff --git a/val_utils/scripts/run_mots_challenge.py b/val_utils/scripts/run_mots_challenge.py
new file mode 100644
index 0000000000000000000000000000000000000000..b5170660e2113d0d23dc1beb72c82830c4111b35
--- /dev/null
+++ b/val_utils/scripts/run_mots_challenge.py
@@ -0,0 +1,96 @@
+""" run_mots.py
+
+Run example:
+run_mots.py --USE_PARALLEL False --METRICS Hota --TRACKERS_TO_EVAL TrackRCNN
+
+Command Line Arguments: Defaults, # Comments
+ Eval arguments:
+ 'USE_PARALLEL': False,
+ 'NUM_PARALLEL_CORES': 8,
+ 'BREAK_ON_ERROR': True,
+ 'PRINT_RESULTS': True,
+ 'PRINT_ONLY_COMBINED': False,
+ 'PRINT_CONFIG': True,
+ 'TIME_PROGRESS': True,
+ 'OUTPUT_SUMMARY': True,
+ 'OUTPUT_DETAILED': True,
+ 'PLOT_CURVES': True,
+ Dataset arguments:
+ 'GT_FOLDER': os.path.join(code_path, 'data/gt/mot_challenge/'), # Location of GT data
+ 'TRACKERS_FOLDER': os.path.join(code_path, 'data/trackers/mot_challenge/'), # Trackers location
+ 'OUTPUT_FOLDER': None, # Where to save eval results (if None, same as TRACKERS_FOLDER)
+ 'TRACKERS_TO_EVAL': None, # Filenames of trackers to eval (if None, all in folder)
+ 'CLASSES_TO_EVAL': ['pedestrian'], # Valid: ['pedestrian']
+ 'SPLIT_TO_EVAL': 'train', # Valid: 'train', 'test'
+ 'INPUT_AS_ZIP': False, # Whether tracker input files are zipped
+ 'PRINT_CONFIG': True, # Whether to print current config
+ 'TRACKER_SUB_FOLDER': 'data', # Tracker files are in TRACKER_FOLDER/tracker_name/TRACKER_SUB_FOLDER
+ 'OUTPUT_SUB_FOLDER': '', # Output files are saved in OUTPUT_FOLDER/tracker_name/OUTPUT_SUB_FOLDER
+ 'SEQMAP_FOLDER': None, # Where seqmaps are found (if None, GT_FOLDER/seqmaps)
+ 'SEQMAP_FILE': None, # Directly specify seqmap file (if none use seqmap_folder/MOTS-split_to_eval)
+ 'SEQ_INFO': None, # If not None, directly specify sequences to eval and their number of timesteps
+ 'GT_LOC_FORMAT': '{gt_folder}/{seq}/gt/gt.txt', # '{gt_folder}/{seq}/gt/gt.txt'
+ 'SKIP_SPLIT_FOL': False, # If False, data is in GT_FOLDER/MOTS-SPLIT_TO_EVAL/ and in
+ # TRACKERS_FOLDER/MOTS-SPLIT_TO_EVAL/tracker/
+ # If True, then the middle 'MOTS-split' folder is skipped for both.
+ Metric arguments:
+ 'METRICS': ['HOTA','CLEAR', 'Identity', 'VACE', 'JAndF']
+"""
+
+import sys
+import os
+import argparse
+from multiprocessing import freeze_support
+
+sys.path.insert(0, os.path.abspath(os.path.join(os.path.dirname(__file__), '..')))
+import trackeval # noqa: E402
+
+if __name__ == '__main__':
+ freeze_support()
+
+ # Command line interface:
+ default_eval_config = trackeval.Evaluator.get_default_eval_config()
+ default_eval_config['DISPLAY_LESS_PROGRESS'] = False
+ default_dataset_config = trackeval.datasets.MOTSChallenge.get_default_dataset_config()
+ default_metrics_config = {'METRICS': ['HOTA', 'CLEAR', 'Identity']}
+ config = {**default_eval_config, **default_dataset_config, **default_metrics_config} # Merge default configs
+ parser = argparse.ArgumentParser()
+ for setting in config.keys():
+ if type(config[setting]) == list or type(config[setting]) == type(None):
+ parser.add_argument("--" + setting, nargs='+')
+ else:
+ parser.add_argument("--" + setting)
+ args = parser.parse_args().__dict__
+ for setting in args.keys():
+ if args[setting] is not None:
+ if type(config[setting]) == type(True):
+ if args[setting] == 'True':
+ x = True
+ elif args[setting] == 'False':
+ x = False
+ else:
+ raise Exception('Command line parameter ' + setting + 'must be True or False')
+ elif type(config[setting]) == type(1):
+ x = int(args[setting])
+ elif type(args[setting]) == type(None):
+ x = None
+ elif setting == 'SEQ_INFO':
+ x = dict(zip(args[setting], [None]*len(args[setting])))
+ else:
+ x = args[setting]
+ config[setting] = x
+ eval_config = {k: v for k, v in config.items() if k in default_eval_config.keys()}
+ dataset_config = {k: v for k, v in config.items() if k in default_dataset_config.keys()}
+ metrics_config = {k: v for k, v in config.items() if k in default_metrics_config.keys()}
+
+ # Run code
+ evaluator = trackeval.Evaluator(eval_config)
+ dataset_list = [trackeval.datasets.MOTSChallenge(dataset_config)]
+ metrics_list = []
+ for metric in [trackeval.metrics.HOTA, trackeval.metrics.CLEAR, trackeval.metrics.Identity, trackeval.metrics.VACE,
+ trackeval.metrics.JAndF]:
+ if metric.get_name() in metrics_config['METRICS']:
+ metrics_list.append(metric())
+ if len(metrics_list) == 0:
+ raise Exception('No metrics selected for evaluation')
+ evaluator.evaluate(dataset_list, metrics_list)
diff --git a/val_utils/scripts/run_person_path_22.py b/val_utils/scripts/run_person_path_22.py
new file mode 100644
index 0000000000000000000000000000000000000000..232b99c2b0d4077be7b5258c1c6b3593df93157c
--- /dev/null
+++ b/val_utils/scripts/run_person_path_22.py
@@ -0,0 +1,112 @@
+
+""" run_person_path_22.py
+
+Run example:
+python3 run_person_path_22.py \
+ --BENCHMARK person_path_22 \
+ --SPLIT_TO_EVAL test \
+ --TRACKERS_TO_EVAL custom_tracker \
+ --METRICS HOTA CLEAR Identity VACE \
+ --USE_PARALLEL True \
+ --NUM_PARALLEL_CORES 12 \
+ --TRACKERS_FOLDER $TRACKER_FOLDER
+
+where $TRACKER_FOLDER is the path of the folder containing the tracker predictions in MOTChallenge format.
+In particular, $TRACKER_FOLDER is expected to have the following structure:
+$TRACKER_FOLDER/
+ person_path_22-test/
+ custom_tracker/
+ data/
+ uid_vid_00008.mp4.txt
+ uid_vid_00009.mp4.txt
+ [...]
+ uid_vid_00235.mp4.txt
+
+Each text file contains the tracker predictions in MOTChallenge format for a given video.
+
+
+Command Line Arguments: Defaults, # Comments
+ Eval arguments:
+ 'USE_PARALLEL': False,
+ 'NUM_PARALLEL_CORES': 8,
+ 'BREAK_ON_ERROR': True,
+ 'PRINT_RESULTS': True,
+ 'PRINT_ONLY_COMBINED': False,
+ 'PRINT_CONFIG': True,
+ 'TIME_PROGRESS': True,
+ 'OUTPUT_SUMMARY': True,
+ 'OUTPUT_DETAILED': True,
+ 'PLOT_CURVES': True,
+ Dataset arguments:
+ 'GT_FOLDER': os.path.join(code_path, 'data/gt/person_path_22/'), # Location of GT data
+ 'TRACKERS_FOLDER': os.path.join(code_path, 'data/trackers/person_path_22/'), # Trackers location
+ 'OUTPUT_FOLDER': None, # Where to save eval results (if None, same as TRACKERS_FOLDER)
+ 'TRACKERS_TO_EVAL': None, # Filenames of trackers to eval (if None, all in folder)
+ 'CLASSES_TO_EVAL': ['pedestrian'], # Valid: ['pedestrian']
+ 'BENCHMARK': 'person_path_22', # Valid: 'person_path_22'
+ 'SPLIT_TO_EVAL': 'train', # Valid: 'train', 'test', 'all'
+ 'INPUT_AS_ZIP': False, # Whether tracker input files are zipped
+ 'PRINT_CONFIG': True, # Whether to print current config
+ 'DO_PREPROC': True, # Whether to perform preprocessing
+ 'TRACKER_SUB_FOLDER': 'data', # Tracker files are in TRACKER_FOLDER/tracker_name/TRACKER_SUB_FOLDER
+ 'OUTPUT_SUB_FOLDER': '', # Output files are saved in OUTPUT_FOLDER/tracker_name/OUTPUT_SUB_FOLDER
+ Metric arguments:
+ 'METRICS': ['HOTA', 'CLEAR', 'Identity', 'VACE']
+"""
+
+import sys
+import os
+import argparse
+from multiprocessing import freeze_support
+
+sys.path.insert(0, os.path.abspath(os.path.join(os.path.dirname(__file__), '..')))
+import trackeval # noqa: E402
+
+if __name__ == '__main__':
+ freeze_support()
+
+ # Command line interface:
+ default_eval_config = trackeval.Evaluator.get_default_eval_config()
+ default_eval_config['DISPLAY_LESS_PROGRESS'] = False
+ default_dataset_config = trackeval.datasets.PersonPath22.get_default_dataset_config()
+ default_metrics_config = {'METRICS': ['HOTA', 'CLEAR', 'Identity'], 'THRESHOLD': 0.5}
+ config = {**default_eval_config, **default_dataset_config, **default_metrics_config} # Merge default configs
+ parser = argparse.ArgumentParser()
+ for setting in config.keys():
+ if type(config[setting]) == list or type(config[setting]) == type(None):
+ parser.add_argument("--" + setting, nargs='+')
+ else:
+ parser.add_argument("--" + setting)
+ args = parser.parse_args().__dict__
+ for setting in args.keys():
+ if args[setting] is not None:
+ if type(config[setting]) == type(True):
+ if args[setting] == 'True':
+ x = True
+ elif args[setting] == 'False':
+ x = False
+ else:
+ raise Exception('Command line parameter ' + setting + 'must be True or False')
+ elif type(config[setting]) == type(1):
+ x = int(args[setting])
+ elif type(args[setting]) == type(None):
+ x = None
+ elif setting == 'SEQ_INFO':
+ x = dict(zip(args[setting], [None]*len(args[setting])))
+ else:
+ x = args[setting]
+ config[setting] = x
+ eval_config = {k: v for k, v in config.items() if k in default_eval_config.keys()}
+ dataset_config = {k: v for k, v in config.items() if k in default_dataset_config.keys()}
+ metrics_config = {k: v for k, v in config.items() if k in default_metrics_config.keys()}
+
+ # Run code
+ evaluator = trackeval.Evaluator(eval_config)
+ dataset_list = [trackeval.datasets.PersonPath22(dataset_config)]
+ metrics_list = []
+ for metric in [trackeval.metrics.HOTA, trackeval.metrics.CLEAR, trackeval.metrics.Identity, trackeval.metrics.VACE]:
+ if metric.get_name() in metrics_config['METRICS']:
+ metrics_list.append(metric(metrics_config))
+ if len(metrics_list) == 0:
+ raise Exception('No metrics selected for evaluation')
+ evaluator.evaluate(dataset_list, metrics_list)
diff --git a/val_utils/scripts/run_rob_mots.py b/val_utils/scripts/run_rob_mots.py
new file mode 100644
index 0000000000000000000000000000000000000000..0fa07fedf7b9c95a12081a6ebfd8c098f277d1be
--- /dev/null
+++ b/val_utils/scripts/run_rob_mots.py
@@ -0,0 +1,142 @@
+# python3 scripts/run_rob_mots.py --ROBMOTS_SPLIT train --TRACKERS_TO_EVAL STP --USE_PARALLEL True --NUM_PARALLEL_CORES 8
+
+import sys
+import os
+import csv
+import numpy as np
+from multiprocessing import freeze_support
+
+sys.path.insert(0, os.path.abspath(os.path.join(os.path.dirname(__file__), '..')))
+import trackeval # noqa: E402
+from trackeval import utils
+
+code_path = utils.get_code_path()
+
+if __name__ == '__main__':
+ freeze_support()
+
+ script_config = {
+ 'ROBMOTS_SPLIT': 'train', # 'train', # valid: 'train', 'val', 'test', 'test_live', 'test_post', 'test_all'
+ 'BENCHMARKS': None, # If None, use all for each split.
+ 'GT_FOLDER': os.path.join(code_path, 'data/gt/rob_mots'),
+ 'TRACKERS_FOLDER': os.path.join(code_path, 'data/trackers/rob_mots'),
+ }
+
+ default_eval_config = trackeval.Evaluator.get_default_eval_config()
+ default_eval_config['PRINT_ONLY_COMBINED'] = True
+ default_eval_config['DISPLAY_LESS_PROGRESS'] = True
+ default_dataset_config = trackeval.datasets.RobMOTS.get_default_dataset_config()
+ config = {**default_eval_config, **default_dataset_config, **script_config}
+
+ # Command line interface:
+ config = utils.update_config(config)
+
+ if not config['BENCHMARKS']:
+ if config['ROBMOTS_SPLIT'] == 'val':
+ config['BENCHMARKS'] = ['kitti_mots', 'bdd_mots', 'davis_unsupervised', 'youtube_vis', 'ovis',
+ 'tao', 'mots_challenge', 'waymo']
+ config['SPLIT_TO_EVAL'] = 'val'
+ elif config['ROBMOTS_SPLIT'] == 'test' or config['SPLIT_TO_EVAL'] == 'test_live':
+ config['BENCHMARKS'] = ['kitti_mots', 'bdd_mots', 'davis_unsupervised', 'youtube_vis', 'tao']
+ config['SPLIT_TO_EVAL'] = 'test'
+ elif config['ROBMOTS_SPLIT'] == 'test_post':
+ config['BENCHMARKS'] = ['mots_challenge', 'waymo', 'ovis']
+ config['SPLIT_TO_EVAL'] = 'test'
+ elif config['ROBMOTS_SPLIT'] == 'test_all':
+ config['BENCHMARKS'] = ['kitti_mots', 'bdd_mots', 'davis_unsupervised', 'youtube_vis', 'ovis',
+ 'tao', 'mots_challenge', 'waymo']
+ config['SPLIT_TO_EVAL'] = 'test'
+ elif config['ROBMOTS_SPLIT'] == 'train':
+ config['BENCHMARKS'] = ['kitti_mots', 'davis_unsupervised', 'youtube_vis', 'ovis', 'tao', 'bdd_mots']
+ config['SPLIT_TO_EVAL'] = 'train'
+ else:
+ config['SPLIT_TO_EVAL'] = config['ROBMOTS_SPLIT']
+
+ metrics_config = {'METRICS': ['HOTA']}
+ eval_config = {k: v for k, v in config.items() if k in config.keys()}
+ dataset_config = {k: v for k, v in config.items() if k in config.keys()}
+
+ # Run code
+ try:
+ dataset_list = []
+ for bench in config['BENCHMARKS']:
+ dataset_config['SUB_BENCHMARK'] = bench
+ dataset_list.append(trackeval.datasets.RobMOTS(dataset_config))
+ evaluator = trackeval.Evaluator(eval_config)
+ metrics_list = []
+ for metric in [trackeval.metrics.HOTA, trackeval.metrics.CLEAR, trackeval.metrics.Identity,
+ trackeval.metrics.VACE, trackeval.metrics.JAndF]:
+ if metric.get_name() in metrics_config['METRICS']:
+ metrics_list.append(metric())
+ if len(metrics_list) == 0:
+ raise Exception('No metrics selected for evaluation')
+ output_res, output_msg = evaluator.evaluate(dataset_list, metrics_list)
+ output = list(list(output_msg.values())[0].values())[0]
+
+ except Exception as err:
+ if type(err) == trackeval.utils.TrackEvalException:
+ output = str(err)
+ else:
+ output = 'Unknown error occurred.'
+
+ success = output == 'Success'
+ if not success:
+ output = 'ERROR, evaluation failed. \n\nError message: ' + output
+ print(output)
+
+ if config['TRACKERS_TO_EVAL']:
+ msg = "Thanks you for participating in the RobMOTS benchmark.\n\n"
+ msg += "The status of your evaluation is: \n" + output + '\n\n'
+ msg += "If your tracking results evaluated successfully on the evaluation server you can see your results here: \n"
+ msg += "https://eval.vision.rwth-aachen.de/vision/"
+ status_file = os.path.join(config['TRACKERS_FOLDER'], config['ROBMOTS_SPLIT'], config['TRACKERS_TO_EVAL'][0],
+ 'status.txt')
+ with open(status_file, 'w', newline='') as f:
+ f.write(msg)
+
+ if success:
+ # For each benchmark, combine the 'all' score with the 'cls_averaged' using geometric mean.
+ metrics_to_calc = ['HOTA', 'DetA', 'AssA', 'DetRe', 'DetPr', 'AssRe', 'AssPr', 'LocA']
+ trackers = list(output_res['RobMOTS.' + config['BENCHMARKS'][0]].keys())
+ for tracker in trackers:
+ # final_results[benchmark][result_type][metric]
+ final_results = {}
+ res = {bench: output_res['RobMOTS.' + bench][tracker]['COMBINED_SEQ'] for bench in config['BENCHMARKS']}
+ for bench in config['BENCHMARKS']:
+ final_results[bench] = {'cls_av': {}, 'det_av': {}, 'final': {}}
+ for metric in metrics_to_calc:
+ final_results[bench]['cls_av'][metric] = np.mean(res[bench]['cls_comb_cls_av']['HOTA'][metric])
+ final_results[bench]['det_av'][metric] = np.mean(res[bench]['all']['HOTA'][metric])
+ final_results[bench]['final'][metric] = \
+ np.sqrt(final_results[bench]['cls_av'][metric] * final_results[bench]['det_av'][metric])
+
+ # Take the arithmetic mean over all the benchmarks
+ final_results['overall'] = {'cls_av': {}, 'det_av': {}, 'final': {}}
+ for metric in metrics_to_calc:
+ final_results['overall']['cls_av'][metric] = \
+ np.mean([final_results[bench]['cls_av'][metric] for bench in config['BENCHMARKS']])
+ final_results['overall']['det_av'][metric] = \
+ np.mean([final_results[bench]['det_av'][metric] for bench in config['BENCHMARKS']])
+ final_results['overall']['final'][metric] = \
+ np.mean([final_results[bench]['final'][metric] for bench in config['BENCHMARKS']])
+
+ # Save out result
+ headers = [config['SPLIT_TO_EVAL']] + [x + '___' + metric for x in ['f', 'c', 'd'] for metric in
+ metrics_to_calc]
+
+
+ def rowify(d):
+ return [d[x][metric] for x in ['final', 'cls_av', 'det_av'] for metric in metrics_to_calc]
+
+
+ out_file = os.path.join(config['TRACKERS_FOLDER'], config['ROBMOTS_SPLIT'], tracker,
+ 'final_results.csv')
+
+ with open(out_file, 'w', newline='') as f:
+ writer = csv.writer(f, delimiter=',')
+ writer.writerow(headers)
+ writer.writerow(['overall'] + rowify(final_results['overall']))
+ for bench in config['BENCHMARKS']:
+ if bench == 'overall':
+ continue
+ writer.writerow([bench] + rowify(final_results[bench]))
diff --git a/val_utils/scripts/run_tao.py b/val_utils/scripts/run_tao.py
new file mode 100644
index 0000000000000000000000000000000000000000..c70f08d714024ac79be8b90ee19fef9ae952cca9
--- /dev/null
+++ b/val_utils/scripts/run_tao.py
@@ -0,0 +1,90 @@
+""" run_tao.py
+
+Run example:
+run_tao.py --USE_PARALLEL False --METRICS HOTA --TRACKERS_TO_EVAL Tracktor++
+
+Command Line Arguments: Defaults, # Comments
+ Eval arguments:
+ 'USE_PARALLEL': False,
+ 'NUM_PARALLEL_CORES': 8,
+ 'BREAK_ON_ERROR': True,
+ 'PRINT_RESULTS': True,
+ 'PRINT_ONLY_COMBINED': False,
+ 'PRINT_CONFIG': True,
+ 'TIME_PROGRESS': True,
+ 'OUTPUT_SUMMARY': True,
+ 'OUTPUT_DETAILED': True,
+ 'PLOT_CURVES': True,
+ Dataset arguments:
+ 'GT_FOLDER': os.path.join(code_path, 'data/gt/tao/tao_training'), # Location of GT data
+ 'TRACKERS_FOLDER': os.path.join(code_path, 'data/trackers/tao/tao_training'), # Trackers location
+ 'OUTPUT_FOLDER': None, # Where to save eval results (if None, same as TRACKERS_FOLDER)
+ 'TRACKERS_TO_EVAL': None, # Filenames of trackers to eval (if None, all in folder)
+ 'CLASSES_TO_EVAL': None, # Classes to eval (if None, all classes)
+ 'SPLIT_TO_EVAL': 'training', # Valid: 'training', 'val'
+ 'PRINT_CONFIG': True, # Whether to print current config
+ 'TRACKER_SUB_FOLDER': 'data', # Tracker files are in TRACKER_FOLDER/tracker_name/TRACKER_SUB_FOLDER
+ 'OUTPUT_SUB_FOLDER': '', # Output files are saved in OUTPUT_FOLDER/tracker_name/OUTPUT_SUB_FOLDER
+ 'TRACKER_DISPLAY_NAMES': None, # Names of trackers to display, if None: TRACKERS_TO_EVAL
+ 'MAX_DETECTIONS': 300, # Number of maximal allowed detections per image (0 for unlimited)
+ Metric arguments:
+ 'METRICS': ['HOTA', 'CLEAR', 'Identity', 'TrackMAP']
+"""
+
+import sys
+import os
+import argparse
+from multiprocessing import freeze_support
+
+sys.path.insert(0, os.path.abspath(os.path.join(os.path.dirname(__file__), '..')))
+import trackeval # noqa: E402
+
+if __name__ == '__main__':
+ freeze_support()
+
+ # Command line interface:
+ default_eval_config = trackeval.Evaluator.get_default_eval_config()
+ # print only combined since TrackMAP is undefined for per sequence breakdowns
+ default_eval_config['PRINT_ONLY_COMBINED'] = True
+ default_eval_config['DISPLAY_LESS_PROGRESS'] = True
+ default_dataset_config = trackeval.datasets.TAO.get_default_dataset_config()
+ default_metrics_config = {'METRICS': ['HOTA', 'CLEAR', 'Identity', 'TrackMAP']}
+ config = {**default_eval_config, **default_dataset_config, **default_metrics_config} # Merge default configs
+ parser = argparse.ArgumentParser()
+ for setting in config.keys():
+ if type(config[setting]) == list or type(config[setting]) == type(None):
+ parser.add_argument("--" + setting, nargs='+')
+ else:
+ parser.add_argument("--" + setting)
+ args = parser.parse_args().__dict__
+ for setting in args.keys():
+ if args[setting] is not None:
+ if type(config[setting]) == type(True):
+ if args[setting] == 'True':
+ x = True
+ elif args[setting] == 'False':
+ x = False
+ else:
+ raise Exception('Command line parameter ' + setting + 'must be True or False')
+ elif type(config[setting]) == type(1):
+ x = int(args[setting])
+ elif type(args[setting]) == type(None):
+ x = None
+ else:
+ x = args[setting]
+ config[setting] = x
+ eval_config = {k: v for k, v in config.items() if k in default_eval_config.keys()}
+ dataset_config = {k: v for k, v in config.items() if k in default_dataset_config.keys()}
+ metrics_config = {k: v for k, v in config.items() if k in default_metrics_config.keys()}
+
+ # Run code
+ evaluator = trackeval.Evaluator(eval_config)
+ dataset_list = [trackeval.datasets.TAO(dataset_config)]
+ metrics_list = []
+ for metric in [trackeval.metrics.TrackMAP, trackeval.metrics.CLEAR, trackeval.metrics.Identity,
+ trackeval.metrics.HOTA]:
+ if metric.get_name() in metrics_config['METRICS']:
+ metrics_list.append(metric())
+ if len(metrics_list) == 0:
+ raise Exception('No metrics selected for evaluation')
+ evaluator.evaluate(dataset_list, metrics_list)
\ No newline at end of file
diff --git a/val_utils/scripts/run_tao_ow.py b/val_utils/scripts/run_tao_ow.py
new file mode 100644
index 0000000000000000000000000000000000000000..2735dd40ab8dba2caed89666cad0e5810f1854e9
--- /dev/null
+++ b/val_utils/scripts/run_tao_ow.py
@@ -0,0 +1,92 @@
+""" run_tao.py
+
+Run example:
+run_tao_ow.py --USE_PARALLEL False --METRICS HOTA --TRACKERS_TO_EVAL Tracktor++
+
+Command Line Arguments: Defaults, # Comments
+ Eval arguments:
+ 'USE_PARALLEL': False,
+ 'NUM_PARALLEL_CORES': 8,
+ 'BREAK_ON_ERROR': True,
+ 'PRINT_RESULTS': True,
+ 'PRINT_ONLY_COMBINED': False,
+ 'PRINT_CONFIG': True,
+ 'TIME_PROGRESS': True,
+ 'OUTPUT_SUMMARY': True,
+ 'OUTPUT_DETAILED': True,
+ 'PLOT_CURVES': True,
+ Dataset arguments:
+ 'GT_FOLDER': os.path.join(code_path, 'data/gt/tao/tao_training'), # Location of GT data
+ 'TRACKERS_FOLDER': os.path.join(code_path, 'data/trackers/tao/tao_training'), # Trackers location
+ 'OUTPUT_FOLDER': None, # Where to save eval results (if None, same as TRACKERS_FOLDER)
+ 'TRACKERS_TO_EVAL': None, # Filenames of trackers to eval (if None, all in folder)
+ 'CLASSES_TO_EVAL': None, # Classes to eval (if None, all classes)
+ 'SPLIT_TO_EVAL': 'training', # Valid: 'training', 'val'
+ 'PRINT_CONFIG': True, # Whether to print current config
+ 'TRACKER_SUB_FOLDER': 'data', # Tracker files are in TRACKER_FOLDER/tracker_name/TRACKER_SUB_FOLDER
+ 'OUTPUT_SUB_FOLDER': '', # Output files are saved in OUTPUT_FOLDER/tracker_name/OUTPUT_SUB_FOLDER
+ 'TRACKER_DISPLAY_NAMES': None, # Names of trackers to display, if None: TRACKERS_TO_EVAL
+ 'MAX_DETECTIONS': 300, # Number of maximal allowed detections per image (0 for unlimited)
+ 'SUBSET': 'unknown', # Evaluate on the following subsets ['all', 'known', 'unknown', 'distractor']
+ Metric arguments:
+ 'METRICS': ['HOTA', 'CLEAR', 'Identity', 'TrackMAP']
+"""
+
+import sys
+import os
+import argparse
+from multiprocessing import freeze_support
+
+sys.path.insert(0, os.path.abspath(os.path.join(os.path.dirname(__file__), '..')))
+import trackeval # noqa: E402
+
+if __name__ == '__main__':
+ freeze_support()
+
+ # Command line interface:
+ default_eval_config = trackeval.Evaluator.get_default_eval_config()
+ # print only combined since TrackMAP is undefined for per sequence breakdowns
+ default_eval_config['PRINT_ONLY_COMBINED'] = True
+ default_eval_config['DISPLAY_LESS_PROGRESS'] = True
+ default_dataset_config = trackeval.datasets.TAO_OW.get_default_dataset_config()
+ default_metrics_config = {'METRICS': ['HOTA', 'CLEAR', 'Identity', 'TrackMAP']}
+ config = {**default_eval_config, **default_dataset_config, **default_metrics_config} # Merge default configs
+ parser = argparse.ArgumentParser()
+ for setting in config.keys():
+ if type(config[setting]) == list or type(config[setting]) == type(None):
+ parser.add_argument("--" + setting, nargs='+')
+ else:
+ parser.add_argument("--" + setting)
+ args = parser.parse_args().__dict__
+ for setting in args.keys():
+ if args[setting] is not None:
+ if type(config[setting]) == type(True):
+ if args[setting] == 'True':
+ x = True
+ elif args[setting] == 'False':
+ x = False
+ else:
+ raise Exception('Command line parameter ' + setting + 'must be True or False')
+ elif type(config[setting]) == type(1):
+ x = int(args[setting])
+ elif type(args[setting]) == type(None):
+ x = None
+ else:
+ x = args[setting]
+ config[setting] = x
+ eval_config = {k: v for k, v in config.items() if k in default_eval_config.keys()}
+ dataset_config = {k: v for k, v in config.items() if k in default_dataset_config.keys()}
+ metrics_config = {k: v for k, v in config.items() if k in default_metrics_config.keys()}
+
+ # Run code
+ evaluator = trackeval.Evaluator(eval_config)
+ dataset_list = [trackeval.datasets.TAO_OW(dataset_config)]
+ metrics_list = []
+ # for metric in [trackeval.metrics.TrackMAP, trackeval.metrics.CLEAR, trackeval.metrics.Identity,
+ # trackeval.metrics.HOTA]:
+ for metric in [trackeval.metrics.HOTA]:
+ if metric.get_name() in metrics_config['METRICS']:
+ metrics_list.append(metric())
+ if len(metrics_list) == 0:
+ raise Exception('No metrics selected for evaluation')
+ evaluator.evaluate(dataset_list, metrics_list)
\ No newline at end of file
diff --git a/val_utils/scripts/run_youtube_vis.py b/val_utils/scripts/run_youtube_vis.py
new file mode 100644
index 0000000000000000000000000000000000000000..d28569bc92ad9dc49e57e657bc81a2d3dccc7c51
--- /dev/null
+++ b/val_utils/scripts/run_youtube_vis.py
@@ -0,0 +1,101 @@
+
+""" run_youtube_vis.py
+Run example:
+run_youtube_vis.py --USE_PARALLEL False --METRICS HOTA --TRACKERS_TO_EVAL STEm_Seg
+Command Line Arguments: Defaults, # Comments
+ Eval arguments:
+ 'USE_PARALLEL': False,
+ 'NUM_PARALLEL_CORES': 8,
+ 'BREAK_ON_ERROR': True, # Raises exception and exits with error
+ 'RETURN_ON_ERROR': False, # if not BREAK_ON_ERROR, then returns from function on error
+ 'LOG_ON_ERROR': os.path.join(code_path, 'error_log.txt'), # if not None, save any errors into a log file.
+ 'PRINT_RESULTS': True,
+ 'PRINT_ONLY_COMBINED': False,
+ 'PRINT_CONFIG': True,
+ 'TIME_PROGRESS': True,
+ 'DISPLAY_LESS_PROGRESS': True,
+ 'OUTPUT_SUMMARY': True,
+ 'OUTPUT_EMPTY_CLASSES': True, # If False, summary files are not output for classes with no detections
+ 'OUTPUT_DETAILED': True,
+ 'PLOT_CURVES': True,
+ Dataset arguments:
+ 'GT_FOLDER': os.path.join(code_path, 'data/gt/youtube_vis/youtube_vis_training'), # Location of GT data
+ 'TRACKERS_FOLDER': os.path.join(code_path, 'data/trackers/youtube_vis/youtube_vis_training'),
+ # Trackers location
+ 'OUTPUT_FOLDER': None, # Where to save eval results (if None, same as TRACKERS_FOLDER)
+ 'TRACKERS_TO_EVAL': None, # Filenames of trackers to eval (if None, all in folder)
+ 'CLASSES_TO_EVAL': None, # Classes to eval (if None, all classes)
+ 'SPLIT_TO_EVAL': 'training', # Valid: 'training', 'val'
+ 'PRINT_CONFIG': True, # Whether to print current config
+ 'OUTPUT_SUB_FOLDER': '', # Output files are saved in OUTPUT_FOLDER/tracker_name/OUTPUT_SUB_FOLDER
+ 'TRACKER_SUB_FOLDER': 'data', # Tracker files are in TRACKER_FOLDER/tracker_name/TRACKER_SUB_FOLDER
+ 'TRACKER_DISPLAY_NAMES': None, # Names of trackers to display, if None: TRACKERS_TO_EVAL
+ Metric arguments:
+ 'METRICS': ['TrackMAP', 'HOTA', 'CLEAR', 'Identity']
+"""
+
+import sys
+import os
+import argparse
+from multiprocessing import freeze_support
+
+sys.path.insert(0, os.path.abspath(os.path.join(os.path.dirname(__file__), '..')))
+import trackeval # noqa: E402
+
+if __name__ == '__main__':
+ freeze_support()
+
+ # Command line interface:
+ default_eval_config = trackeval.Evaluator.get_default_eval_config()
+ # print only combined since TrackMAP is undefined for per sequence breakdowns
+ default_eval_config['PRINT_ONLY_COMBINED'] = True
+ default_dataset_config = trackeval.datasets.YouTubeVIS.get_default_dataset_config()
+ default_metrics_config = {'METRICS': ['TrackMAP', 'HOTA', 'CLEAR', 'Identity']}
+ config = {**default_eval_config, **default_dataset_config, **default_metrics_config} # Merge default configs
+ parser = argparse.ArgumentParser()
+ for setting in config.keys():
+ if type(config[setting]) == list or type(config[setting]) == type(None):
+ parser.add_argument("--" + setting, nargs='+')
+ else:
+ parser.add_argument("--" + setting)
+ args = parser.parse_args().__dict__
+ for setting in args.keys():
+ if args[setting] is not None:
+ if type(config[setting]) == type(True):
+ if args[setting] == 'True':
+ x = True
+ elif args[setting] == 'False':
+ x = False
+ else:
+ raise Exception('Command line parameter ' + setting + 'must be True or False')
+ elif type(config[setting]) == type(1):
+ x = int(args[setting])
+ elif type(args[setting]) == type(None):
+ x = None
+ else:
+ x = args[setting]
+ config[setting] = x
+ eval_config = {k: v for k, v in config.items() if k in default_eval_config.keys()}
+ dataset_config = {k: v for k, v in config.items() if k in default_dataset_config.keys()}
+ metrics_config = {k: v for k, v in config.items() if k in default_metrics_config.keys()}
+
+ # Run code
+ evaluator = trackeval.Evaluator(eval_config)
+ dataset_list = [trackeval.datasets.YouTubeVIS(dataset_config)]
+ metrics_list = []
+ for metric in [trackeval.metrics.TrackMAP, trackeval.metrics.HOTA, trackeval.metrics.CLEAR,
+ trackeval.metrics.Identity]:
+ if metric.get_name() in metrics_config['METRICS']:
+ # specify TrackMAP config for YouTubeVIS
+ if metric == trackeval.metrics.TrackMAP:
+ default_track_map_config = metric.get_default_metric_config()
+ default_track_map_config['USE_TIME_RANGES'] = False
+ default_track_map_config['AREA_RANGES'] = [[0 ** 2, 128 ** 2],
+ [ 128 ** 2, 256 ** 2],
+ [256 ** 2, 1e5 ** 2]]
+ metrics_list.append(metric(default_track_map_config))
+ else:
+ metrics_list.append(metric())
+ if len(metrics_list) == 0:
+ raise Exception('No metrics selected for evaluation')
+ evaluator.evaluate(dataset_list, metrics_list)
\ No newline at end of file
diff --git a/val_utils/setup.cfg b/val_utils/setup.cfg
new file mode 100644
index 0000000000000000000000000000000000000000..914f3de2e145992a4d55fdf294a81b7823e1f619
--- /dev/null
+++ b/val_utils/setup.cfg
@@ -0,0 +1,27 @@
+[metadata]
+name = trackeval
+version = 1.0.dev1
+author = Jonathon Luiten, Arne Hoffhues
+author_email = jonoluiten@gmail.com
+description = Code for evaluating object tracking
+long_description = file: Readme.md
+long_description_content_type = text/markdown
+url = https://github.com/JonathonLuiten/TrackEval
+project_urls =
+ Bug Tracker = https://github.com/JonathonLuiten/TrackEval/issues
+classifiers =
+ Programming Language :: Python :: 3
+ Programming Language :: Python :: 3 :: Only
+ License :: OSI Approved :: MIT License
+ Operating System :: OS Independent
+ Topic :: Scientific/Engineering
+license_files = LICENSE
+
+[options]
+install_requires =
+ numpy
+ scipy
+packages = find:
+
+[options.packages.find]
+include = trackeval*
diff --git a/val_utils/setup.py b/val_utils/setup.py
new file mode 100644
index 0000000000000000000000000000000000000000..606849326a4002007fd42060b51e69a19c18675c
--- /dev/null
+++ b/val_utils/setup.py
@@ -0,0 +1,3 @@
+from setuptools import setup
+
+setup()
diff --git a/val_utils/tests/test_all_quick.py b/val_utils/tests/test_all_quick.py
new file mode 100644
index 0000000000000000000000000000000000000000..89cd3e8c1b2061473ef148f10e60ffd07074d3f9
--- /dev/null
+++ b/val_utils/tests/test_all_quick.py
@@ -0,0 +1,75 @@
+""" Test to ensure that the code is working correctly.
+Should test ALL metrics across all datasets and splits currently supported.
+Only tests one tracker per dataset/split to give a quick test result.
+"""
+
+import sys
+import os
+import numpy as np
+from multiprocessing import freeze_support
+
+sys.path.insert(0, os.path.abspath(os.path.join(os.path.dirname(__file__), '..')))
+import trackeval # noqa: E402
+
+# Fixes multiprocessing on windows, does nothing otherwise
+if __name__ == '__main__':
+ freeze_support()
+
+eval_config = {'USE_PARALLEL': False,
+ 'NUM_PARALLEL_CORES': 8,
+ }
+evaluator = trackeval.Evaluator(eval_config)
+metrics_list = [trackeval.metrics.HOTA(), trackeval.metrics.CLEAR(), trackeval.metrics.Identity()]
+
+tests = [
+ {'DATASET': 'Kitti2DBox', 'SPLIT_TO_EVAL': 'training', 'TRACKERS_TO_EVAL': ['CIWT']},
+ {'DATASET': 'MotChallenge2DBox', 'BENCHMARK': 'MOT15', 'SPLIT_TO_EVAL': 'train', 'TRACKERS_TO_EVAL': ['MPNTrack']},
+ {'DATASET': 'MotChallenge2DBox', 'BENCHMARK': 'MOT16', 'SPLIT_TO_EVAL': 'train', 'TRACKERS_TO_EVAL': ['MPNTrack']},
+ {'DATASET': 'MotChallenge2DBox', 'BENCHMARK': 'MOT17', 'SPLIT_TO_EVAL': 'train', 'TRACKERS_TO_EVAL': ['MPNTrack']},
+ {'DATASET': 'MotChallenge2DBox', 'BENCHMARK': 'MOT20', 'SPLIT_TO_EVAL': 'train', 'TRACKERS_TO_EVAL': ['MPNTrack']},
+]
+
+for dataset_config in tests:
+
+ dataset_name = dataset_config.pop('DATASET')
+ if dataset_name == 'MotChallenge2DBox':
+ dataset_list = [trackeval.datasets.MotChallenge2DBox(dataset_config)]
+ file_loc = os.path.join('mot_challenge', dataset_config['BENCHMARK'] + '-' + dataset_config['SPLIT_TO_EVAL'])
+ elif dataset_name == 'Kitti2DBox':
+ dataset_list = [trackeval.datasets.Kitti2DBox(dataset_config)]
+ file_loc = os.path.join('kitti', 'kitti_2d_box_train')
+ else:
+ raise Exception('Dataset %s does not exist.' % dataset_name)
+
+ raw_results, messages = evaluator.evaluate(dataset_list, metrics_list)
+
+ classes = dataset_list[0].config['CLASSES_TO_EVAL']
+ tracker = dataset_config['TRACKERS_TO_EVAL'][0]
+ test_data_loc = os.path.join(os.path.dirname(__file__), '..', 'data', 'tests', file_loc)
+
+ for cls in classes:
+ results = {seq: raw_results[dataset_name][tracker][seq][cls] for seq in raw_results[dataset_name][tracker].keys()}
+ current_metrics_list = metrics_list + [trackeval.metrics.Count()]
+ metric_names = trackeval.utils.validate_metrics_list(current_metrics_list)
+
+ # Load expected results:
+ test_data = trackeval.utils.load_detail(os.path.join(test_data_loc, tracker, cls + '_detailed.csv'))
+
+ # Do checks
+ for seq in test_data.keys():
+ assert len(test_data[seq].keys()) > 250, len(test_data[seq].keys())
+
+ details = []
+ for metric, metric_name in zip(current_metrics_list, metric_names):
+ table_res = {seq_key: seq_value[metric_name] for seq_key, seq_value in results.items()}
+ details.append(metric.detailed_results(table_res))
+ res_fields = sum([list(s['COMBINED_SEQ'].keys()) for s in details], [])
+ res_values = sum([list(s[seq].values()) for s in details], [])
+ res_dict = dict(zip(res_fields, res_values))
+
+ for field in test_data[seq].keys():
+ assert np.isclose(res_dict[field], test_data[seq][field]), seq + ': ' + cls + ': ' + field
+
+ print('Tracker %s tests passed' % tracker)
+print('All tests passed')
+
diff --git a/val_utils/tests/test_davis.py b/val_utils/tests/test_davis.py
new file mode 100644
index 0000000000000000000000000000000000000000..dac0a3c957939edaae2a8b910ad2f1ce30f040bd
--- /dev/null
+++ b/val_utils/tests/test_davis.py
@@ -0,0 +1,68 @@
+import sys
+import os
+import numpy as np
+from multiprocessing import freeze_support
+
+sys.path.insert(0, os.path.abspath(os.path.join(os.path.dirname(__file__), '..')))
+import trackeval # noqa: E402
+
+# Fixes multiprocessing on windows, does nothing otherwise
+if __name__ == '__main__':
+ freeze_support()
+
+
+eval_config = {'USE_PARALLEL': False,
+ 'NUM_PARALLEL_CORES': 8,
+ 'PRINT_RESULTS': False,
+ 'PRINT_CONFIG': True,
+ 'TIME_PROGRESS': True,
+ 'DISPLAY_LESS_PROGRESS': True,
+ 'OUTPUT_SUMMARY': False,
+ 'OUTPUT_EMPTY_CLASSES': False,
+ 'OUTPUT_DETAILED': False,
+ 'PLOT_CURVES': False,
+ }
+evaluator = trackeval.Evaluator(eval_config)
+metrics_list = [trackeval.metrics.HOTA(), trackeval.metrics.CLEAR(), trackeval.metrics.Identity(),
+ trackeval.metrics.JAndF()]
+
+tests = [
+ {'SPLIT_TO_EVAL': 'val', 'TRACKERS_TO_EVAL': ['ags']},
+]
+
+for dataset_config in tests:
+
+ dataset_list = [trackeval.datasets.DAVIS(dataset_config)]
+ file_loc = os.path.join('davis', 'davis_unsupervised_' + dataset_config['SPLIT_TO_EVAL'])
+
+ raw_results, messages = evaluator.evaluate(dataset_list, metrics_list)
+
+ classes = dataset_list[0].config['CLASSES_TO_EVAL']
+ tracker = dataset_config['TRACKERS_TO_EVAL'][0]
+ test_data_loc = os.path.join(os.path.dirname(__file__), '..', 'data', 'tests', file_loc)
+
+ for cls in classes:
+ results = {seq: raw_results['DAVIS'][tracker][seq][cls] for seq in raw_results['DAVIS'][tracker].keys()}
+ current_metrics_list = metrics_list + [trackeval.metrics.Count()]
+ metric_names = trackeval.utils.validate_metrics_list(current_metrics_list)
+
+ # Load expected results:
+ test_data = trackeval.utils.load_detail(os.path.join(test_data_loc, tracker, cls + '_detailed.csv'))
+
+ # Do checks
+ for seq in test_data.keys():
+ assert len(test_data[seq].keys()) > 250, len(test_data[seq].keys())
+
+ details = []
+ for metric, metric_name in zip(current_metrics_list, metric_names):
+ table_res = {seq_key: seq_value[metric_name] for seq_key, seq_value in results.items()}
+ details.append(metric.detailed_results(table_res))
+ res_fields = sum([list(s['COMBINED_SEQ'].keys()) for s in details], [])
+ res_values = sum([list(s[seq].values()) for s in details], [])
+ res_dict = dict(zip(res_fields, res_values))
+
+ for field in test_data[seq].keys():
+ assert np.isclose(res_dict[field], test_data[seq][field]), seq + ': ' + cls + ': ' + field
+
+ print('Tracker %s tests passed' % tracker)
+print('All tests passed')
\ No newline at end of file
diff --git a/val_utils/tests/test_metrics.py b/val_utils/tests/test_metrics.py
new file mode 100644
index 0000000000000000000000000000000000000000..f4738245fb69a0071486a806a6063aaf8e63773b
--- /dev/null
+++ b/val_utils/tests/test_metrics.py
@@ -0,0 +1,212 @@
+import numpy as np
+import pytest
+
+import trackeval
+
+
+def no_confusion():
+ num_timesteps = 5
+ num_gt_ids = 2
+ num_tracker_ids = 2
+
+ # No overlap between pairs (0, 0) and (1, 1).
+ similarity = np.zeros([num_timesteps, num_gt_ids, num_tracker_ids])
+ similarity[:, 0, 1] = [0, 0, 0, 1, 1]
+ similarity[:, 1, 0] = [1, 1, 0, 0, 0]
+ gt_present = np.zeros([num_timesteps, num_gt_ids])
+ gt_present[:, 0] = [1, 1, 1, 1, 1]
+ gt_present[:, 1] = [1, 1, 1, 0, 0]
+ tracker_present = np.zeros([num_timesteps, num_tracker_ids])
+ tracker_present[:, 0] = [1, 1, 1, 1, 0]
+ tracker_present[:, 1] = [1, 1, 1, 1, 1]
+
+ expected = {
+ 'clear': {
+ 'CLR_TP': 4,
+ 'CLR_FN': 4,
+ 'CLR_FP': 5,
+ 'IDSW': 0,
+ 'MOTA': 1 - 9 / 8,
+ },
+ 'identity': {
+ 'IDTP': 4,
+ 'IDFN': 4,
+ 'IDFP': 5,
+ 'IDR': 4 / 8,
+ 'IDP': 4 / 9,
+ 'IDF1': 2 * 4 / 17,
+ },
+ 'vace': {
+ 'STDA': 2 / 5 + 2 / 4,
+ 'ATA': (2 / 5 + 2 / 4) / 2,
+ },
+ }
+
+ data = _from_dense(
+ num_timesteps=num_timesteps,
+ num_gt_ids=num_gt_ids,
+ num_tracker_ids=num_tracker_ids,
+ gt_present=gt_present,
+ tracker_present=tracker_present,
+ similarity=similarity,
+ )
+ return data, expected
+
+
+def with_confusion():
+ num_timesteps = 5
+ num_gt_ids = 2
+ num_tracker_ids = 2
+
+ similarity = np.zeros([num_timesteps, num_gt_ids, num_tracker_ids])
+ similarity[:, 0, 1] = [0, 0, 0, 1, 1]
+ similarity[:, 1, 0] = [1, 1, 0, 0, 0]
+ # Add some overlap between (0, 0) and (1, 1).
+ similarity[:, 0, 0] = [0, 0, 1, 0, 0]
+ similarity[:, 1, 1] = [0, 1, 0, 0, 0]
+ gt_present = np.zeros([num_timesteps, num_gt_ids])
+ gt_present[:, 0] = [1, 1, 1, 1, 1]
+ gt_present[:, 1] = [1, 1, 1, 0, 0]
+ tracker_present = np.zeros([num_timesteps, num_tracker_ids])
+ tracker_present[:, 0] = [1, 1, 1, 1, 0]
+ tracker_present[:, 1] = [1, 1, 1, 1, 1]
+
+ expected = {
+ 'clear': {
+ 'CLR_TP': 5,
+ 'CLR_FN': 3, # 8 - 5
+ 'CLR_FP': 4, # 9 - 5
+ 'IDSW': 1,
+ 'MOTA': 1 - 8 / 8,
+ },
+ 'identity': {
+ 'IDTP': 4,
+ 'IDFN': 4,
+ 'IDFP': 5,
+ 'IDR': 4 / 8,
+ 'IDP': 4 / 9,
+ 'IDF1': 2 * 4 / 17,
+ },
+ 'vace': {
+ 'STDA': 2 / 5 + 2 / 4,
+ 'ATA': (2 / 5 + 2 / 4) / 2,
+ },
+ }
+
+ data = _from_dense(
+ num_timesteps=num_timesteps,
+ num_gt_ids=num_gt_ids,
+ num_tracker_ids=num_tracker_ids,
+ gt_present=gt_present,
+ tracker_present=tracker_present,
+ similarity=similarity,
+ )
+ return data, expected
+
+
+def split_tracks():
+ num_timesteps = 5
+ num_gt_ids = 2
+ num_tracker_ids = 5
+
+ similarity = np.zeros([num_timesteps, num_gt_ids, num_tracker_ids])
+ # Split ground-truth 0 between tracks 0, 3.
+ similarity[:, 0, 0] = [1, 1, 0, 0, 0]
+ similarity[:, 0, 3] = [0, 0, 0, 1, 1]
+ # Split ground-truth 1 between tracks 1, 2, 4.
+ similarity[:, 1, 1] = [0, 0, 1, 1, 0]
+ similarity[:, 1, 2] = [0, 0, 0, 0, 1]
+ similarity[:, 1, 4] = [1, 1, 0, 0, 0]
+ gt_present = np.zeros([num_timesteps, num_gt_ids])
+ gt_present[:, 0] = [1, 1, 0, 1, 1]
+ gt_present[:, 1] = [1, 1, 1, 1, 1]
+ tracker_present = np.zeros([num_timesteps, num_tracker_ids])
+ tracker_present[:, 0] = [1, 1, 0, 0, 0]
+ tracker_present[:, 1] = [0, 0, 1, 1, 1]
+ tracker_present[:, 2] = [0, 0, 0, 0, 1]
+ tracker_present[:, 3] = [0, 0, 1, 1, 1]
+ tracker_present[:, 4] = [1, 1, 0, 0, 0]
+
+ expected = {
+ 'clear': {
+ 'CLR_TP': 9,
+ 'CLR_FN': 0, # 9 - 9
+ 'CLR_FP': 2, # 11 - 9
+ 'IDSW': 3,
+ 'MOTA': 1 - 5 / 9,
+ },
+ 'identity': {
+ 'IDTP': 4,
+ 'IDFN': 5, # 9 - 4
+ 'IDFP': 7, # 11 - 4
+ 'IDR': 4 / 9,
+ 'IDP': 4 / 11,
+ 'IDF1': 2 * 4 / 20,
+ },
+ 'vace': {
+ 'STDA': 2 / 4 + 2 / 5,
+ 'ATA': (2 / 4 + 2 / 5) / (0.5 * (2 + 5)),
+ },
+ }
+
+ data = _from_dense(
+ num_timesteps=num_timesteps,
+ num_gt_ids=num_gt_ids,
+ num_tracker_ids=num_tracker_ids,
+ gt_present=gt_present,
+ tracker_present=tracker_present,
+ similarity=similarity,
+ )
+ return data, expected
+
+
+def _from_dense(num_timesteps, num_gt_ids, num_tracker_ids, gt_present, tracker_present, similarity):
+ gt_subset = [np.flatnonzero(gt_present[t, :]) for t in range(num_timesteps)]
+ tracker_subset = [np.flatnonzero(tracker_present[t, :]) for t in range(num_timesteps)]
+ similarity_subset = [
+ similarity[t][gt_subset[t], :][:, tracker_subset[t]]
+ for t in range(num_timesteps)
+ ]
+ data = {
+ 'num_timesteps': num_timesteps,
+ 'num_gt_ids': num_gt_ids,
+ 'num_tracker_ids': num_tracker_ids,
+ 'num_gt_dets': np.sum(gt_present),
+ 'num_tracker_dets': np.sum(tracker_present),
+ 'gt_ids': gt_subset,
+ 'tracker_ids': tracker_subset,
+ 'similarity_scores': similarity_subset,
+ }
+ return data
+
+
+METRICS_BY_NAME = {
+ 'clear': trackeval.metrics.CLEAR(),
+ 'identity': trackeval.metrics.Identity(),
+ 'vace': trackeval.metrics.VACE(),
+}
+
+SEQUENCE_BY_NAME = {
+ 'no_confusion': no_confusion(),
+ 'with_confusion': with_confusion(),
+ 'split_tracks': split_tracks(),
+}
+
+
+@pytest.mark.parametrize('sequence_name,metric_name', [
+ ('no_confusion', 'clear'),
+ ('no_confusion', 'identity'),
+ ('no_confusion', 'vace'),
+ ('with_confusion', 'clear'),
+ ('with_confusion', 'identity'),
+ ('with_confusion', 'vace'),
+ ('split_tracks', 'clear'),
+ ('split_tracks', 'identity'),
+ ('split_tracks', 'vace'),
+])
+def test_metric(sequence_name, metric_name):
+ data, expected = SEQUENCE_BY_NAME[sequence_name]
+ metric = METRICS_BY_NAME[metric_name]
+ result = metric.eval_sequence(data)
+ for key, value in expected[metric_name].items():
+ assert result[key] == pytest.approx(value), key
diff --git a/val_utils/tests/test_mot17.py b/val_utils/tests/test_mot17.py
new file mode 100644
index 0000000000000000000000000000000000000000..92b9375428a63ab9e8eb8985de3baa16f01ae1f0
--- /dev/null
+++ b/val_utils/tests/test_mot17.py
@@ -0,0 +1,76 @@
+""" Test to ensure that the code is working correctly.
+Runs all metrics on 14 trackers for the MOT Challenge MOT17 benchmark.
+"""
+
+
+import sys
+import os
+import numpy as np
+from multiprocessing import freeze_support
+
+sys.path.insert(0, os.path.abspath(os.path.join(os.path.dirname(__file__), '..')))
+import trackeval # noqa: E402
+
+# Fixes multiprocessing on windows, does nothing otherwise
+if __name__ == '__main__':
+ freeze_support()
+
+eval_config = {'USE_PARALLEL': False,
+ 'NUM_PARALLEL_CORES': 8,
+ }
+evaluator = trackeval.Evaluator(eval_config)
+metrics_list = [trackeval.metrics.HOTA(), trackeval.metrics.CLEAR(), trackeval.metrics.Identity()]
+test_data_loc = os.path.join(os.path.dirname(__file__), '..', 'data', 'tests', 'mot_challenge', 'MOT17-train')
+trackers = [
+ 'DPMOT',
+ 'GNNMatch',
+ 'IA',
+ 'ISE_MOT17R',
+ 'Lif_T',
+ 'Lif_TsimInt',
+ 'LPC_MOT',
+ 'MAT',
+ 'MIFTv2',
+ 'MPNTrack',
+ 'SSAT',
+ 'TracktorCorr',
+ 'Tracktorv2',
+ 'UnsupTrack',
+]
+
+for tracker in trackers:
+ # Run code on tracker
+ dataset_config = {'TRACKERS_TO_EVAL': [tracker],
+ 'BENCHMARK': 'MOT17'}
+ dataset_list = [trackeval.datasets.MotChallenge2DBox(dataset_config)]
+ raw_results, messages = evaluator.evaluate(dataset_list, metrics_list)
+
+ results = {seq: raw_results['MotChallenge2DBox'][tracker][seq]['pedestrian'] for seq in
+ raw_results['MotChallenge2DBox'][tracker].keys()}
+ current_metrics_list = metrics_list + [trackeval.metrics.Count()]
+ metric_names = trackeval.utils.validate_metrics_list(current_metrics_list)
+
+ # Load expected results:
+ test_data = trackeval.utils.load_detail(os.path.join(test_data_loc, tracker, 'pedestrian_detailed.csv'))
+ assert len(test_data.keys()) == 22, len(test_data.keys())
+
+ # Do checks
+ for seq in test_data.keys():
+ assert len(test_data[seq].keys()) > 250, len(test_data[seq].keys())
+
+ details = []
+ for metric, metric_name in zip(current_metrics_list, metric_names):
+ table_res = {seq_key: seq_value[metric_name] for seq_key, seq_value in results.items()}
+ details.append(metric.detailed_results(table_res))
+ res_fields = sum([list(s['COMBINED_SEQ'].keys()) for s in details], [])
+ res_values = sum([list(s[seq].values()) for s in details], [])
+ res_dict = dict(zip(res_fields, res_values))
+
+ for field in test_data[seq].keys():
+ if not np.isclose(res_dict[field], test_data[seq][field]):
+ print(tracker, seq, res_dict[field], test_data[seq][field], field)
+ raise AssertionError
+
+ print('Tracker %s tests passed' % tracker)
+print('All tests passed')
+
diff --git a/val_utils/tests/test_mots.py b/val_utils/tests/test_mots.py
new file mode 100644
index 0000000000000000000000000000000000000000..8b80c83dfcbb10a20823737aeaddca9b36d4df5d
--- /dev/null
+++ b/val_utils/tests/test_mots.py
@@ -0,0 +1,66 @@
+import sys
+import os
+import numpy as np
+from multiprocessing import freeze_support
+
+sys.path.insert(0, os.path.abspath(os.path.join(os.path.dirname(__file__), '..')))
+import trackeval # noqa: E402
+
+# Fixes multiprocessing on windows, does nothing otherwise
+if __name__ == '__main__':
+ freeze_support()
+
+eval_config = {'USE_PARALLEL': False,
+ 'NUM_PARALLEL_CORES': 8,
+ }
+evaluator = trackeval.Evaluator(eval_config)
+metrics_list = [trackeval.metrics.HOTA(), trackeval.metrics.CLEAR(), trackeval.metrics.Identity()]
+
+tests = [
+ {'DATASET': 'KittiMOTS', 'SPLIT_TO_EVAL': 'val', 'TRACKERS_TO_EVAL': ['trackrcnn']},
+ {'DATASET': 'MOTSChallenge', 'SPLIT_TO_EVAL': 'train', 'TRACKERS_TO_EVAL': ['TrackRCNN']}
+]
+
+for dataset_config in tests:
+
+ dataset_name = dataset_config.pop('DATASET')
+ if dataset_name == 'MOTSChallenge':
+ dataset_list = [trackeval.datasets.MOTSChallenge(dataset_config)]
+ file_loc = os.path.join('mot_challenge', 'MOTS-' + dataset_config['SPLIT_TO_EVAL'])
+ elif dataset_name == 'KittiMOTS':
+ dataset_list = [trackeval.datasets.KittiMOTS(dataset_config)]
+ file_loc = os.path.join('kitti', 'kitti_mots_val')
+ else:
+ raise Exception('Dataset %s does not exist.' % dataset_name)
+
+ raw_results, messages = evaluator.evaluate(dataset_list, metrics_list)
+
+ classes = dataset_list[0].config['CLASSES_TO_EVAL']
+ tracker = dataset_config['TRACKERS_TO_EVAL'][0]
+ test_data_loc = os.path.join(os.path.dirname(__file__), '..', 'data', 'tests', file_loc)
+
+ for cls in classes:
+ results = {seq: raw_results[dataset_name][tracker][seq][cls] for seq in raw_results[dataset_name][tracker].keys()}
+ current_metrics_list = metrics_list + [trackeval.metrics.Count()]
+ metric_names = trackeval.utils.validate_metrics_list(current_metrics_list)
+
+ # Load expected results:
+ test_data = trackeval.utils.load_detail(os.path.join(test_data_loc, tracker, cls + '_detailed.csv'))
+
+ # Do checks
+ for seq in test_data.keys():
+ assert len(test_data[seq].keys()) > 250, len(test_data[seq].keys())
+
+ details = []
+ for metric, metric_name in zip(current_metrics_list, metric_names):
+ table_res = {seq_key: seq_value[metric_name] for seq_key, seq_value in results.items()}
+ details.append(metric.detailed_results(table_res))
+ res_fields = sum([list(s['COMBINED_SEQ'].keys()) for s in details], [])
+ res_values = sum([list(s[seq].values()) for s in details], [])
+ res_dict = dict(zip(res_fields, res_values))
+
+ for field in test_data[seq].keys():
+ assert np.isclose(res_dict[field], test_data[seq][field]), seq + ': ' + cls + ': ' + field
+
+ print('Tracker %s tests passed' % tracker)
+print('All tests passed')
\ No newline at end of file
diff --git a/val_utils/trackeval/__init__.py b/val_utils/trackeval/__init__.py
new file mode 100644
index 0000000000000000000000000000000000000000..dce62dab74cda480450101fdad794a9a87485ba7
--- /dev/null
+++ b/val_utils/trackeval/__init__.py
@@ -0,0 +1,5 @@
+from .eval import Evaluator
+from . import datasets
+from . import metrics
+from . import plotting
+from . import utils
diff --git a/val_utils/trackeval/_timing.py b/val_utils/trackeval/_timing.py
new file mode 100644
index 0000000000000000000000000000000000000000..4614ba3162ad8143b4e9b3c8d9bc286e7a684f4c
--- /dev/null
+++ b/val_utils/trackeval/_timing.py
@@ -0,0 +1,65 @@
+from functools import wraps
+from time import perf_counter
+import inspect
+
+DO_TIMING = False
+DISPLAY_LESS_PROGRESS = False
+timer_dict = {}
+counter = 0
+
+
+def time(f):
+ @wraps(f)
+ def wrap(*args, **kw):
+ if DO_TIMING:
+ # Run function with timing
+ ts = perf_counter()
+ result = f(*args, **kw)
+ te = perf_counter()
+ tt = te-ts
+
+ # Get function name
+ arg_names = inspect.getfullargspec(f)[0]
+ if arg_names[0] == 'self' and DISPLAY_LESS_PROGRESS:
+ return result
+ elif arg_names[0] == 'self':
+ method_name = type(args[0]).__name__ + '.' + f.__name__
+ else:
+ method_name = f.__name__
+
+ # Record accumulative time in each function for analysis
+ if method_name in timer_dict.keys():
+ timer_dict[method_name] += tt
+ else:
+ timer_dict[method_name] = tt
+
+ # If code is finished, display timing summary
+ if method_name == "Evaluator.evaluate":
+ print("")
+ print("Timing analysis:")
+ for key, value in timer_dict.items():
+ print('%-70s %2.4f sec' % (key, value))
+ else:
+ # Get function argument values for printing special arguments of interest
+ arg_titles = ['tracker', 'seq', 'cls']
+ arg_vals = []
+ for i, a in enumerate(arg_names):
+ if a in arg_titles:
+ arg_vals.append(args[i])
+ arg_text = '(' + ', '.join(arg_vals) + ')'
+
+ # Display methods and functions with different indentation.
+ if arg_names[0] == 'self':
+ print('%-74s %2.4f sec' % (' '*4 + method_name + arg_text, tt))
+ elif arg_names[0] == 'test':
+ pass
+ else:
+ global counter
+ counter += 1
+ print('%i %-70s %2.4f sec' % (counter, method_name + arg_text, tt))
+
+ return result
+ else:
+ # If config["TIME_PROGRESS"] is false, or config["USE_PARALLEL"] is true, run functions normally without timing.
+ return f(*args, **kw)
+ return wrap
diff --git a/val_utils/trackeval/baselines/__init__.py b/val_utils/trackeval/baselines/__init__.py
new file mode 100644
index 0000000000000000000000000000000000000000..ddc9864ddcbcc44fcac3edd60b8a34a9879996aa
--- /dev/null
+++ b/val_utils/trackeval/baselines/__init__.py
@@ -0,0 +1,6 @@
+import baseline_utils
+import stp
+import non_overlap
+import pascal_colormap
+import thresholder
+import vizualize
\ No newline at end of file
diff --git a/val_utils/trackeval/baselines/baseline_utils.py b/val_utils/trackeval/baselines/baseline_utils.py
new file mode 100644
index 0000000000000000000000000000000000000000..b6c88fd9c9d6556d6ae1d01f421c4b15bdb0cda3
--- /dev/null
+++ b/val_utils/trackeval/baselines/baseline_utils.py
@@ -0,0 +1,321 @@
+
+import os
+import csv
+import numpy as np
+from copy import deepcopy
+from PIL import Image
+from pycocotools import mask as mask_utils
+from scipy.optimize import linear_sum_assignment
+from trackeval.baselines.pascal_colormap import pascal_colormap
+
+
+def load_seq(file_to_load):
+ """ Load input data from file in RobMOTS format (e.g. provided detections).
+ Returns: Data object with the following structure (see STP :
+ data['cls'][t] = {'ids', 'scores', 'im_hs', 'im_ws', 'mask_rles'}
+ """
+ fp = open(file_to_load)
+ dialect = csv.Sniffer().sniff(fp.readline(), delimiters=' ')
+ dialect.skipinitialspace = True
+ fp.seek(0)
+ reader = csv.reader(fp, dialect)
+ read_data = {}
+ num_timesteps = 0
+ for i, row in enumerate(reader):
+ if row[-1] in '':
+ row = row[:-1]
+ t = int(row[0])
+ cid = row[1]
+ c = int(row[2])
+ s = row[3]
+ h = row[4]
+ w = row[5]
+ rle = row[6]
+
+ if t >= num_timesteps:
+ num_timesteps = t + 1
+
+ if c in read_data.keys():
+ if t in read_data[c].keys():
+ read_data[c][t]['ids'].append(cid)
+ read_data[c][t]['scores'].append(s)
+ read_data[c][t]['im_hs'].append(h)
+ read_data[c][t]['im_ws'].append(w)
+ read_data[c][t]['mask_rles'].append(rle)
+ else:
+ read_data[c][t] = {}
+ read_data[c][t]['ids'] = [cid]
+ read_data[c][t]['scores'] = [s]
+ read_data[c][t]['im_hs'] = [h]
+ read_data[c][t]['im_ws'] = [w]
+ read_data[c][t]['mask_rles'] = [rle]
+ else:
+ read_data[c] = {t: {}}
+ read_data[c][t]['ids'] = [cid]
+ read_data[c][t]['scores'] = [s]
+ read_data[c][t]['im_hs'] = [h]
+ read_data[c][t]['im_ws'] = [w]
+ read_data[c][t]['mask_rles'] = [rle]
+ fp.close()
+
+ data = {}
+ for c in read_data.keys():
+ data[c] = [{} for _ in range(num_timesteps)]
+ for t in range(num_timesteps):
+ if t in read_data[c].keys():
+ data[c][t]['ids'] = np.atleast_1d(read_data[c][t]['ids']).astype(int)
+ data[c][t]['scores'] = np.atleast_1d(read_data[c][t]['scores']).astype(float)
+ data[c][t]['im_hs'] = np.atleast_1d(read_data[c][t]['im_hs']).astype(int)
+ data[c][t]['im_ws'] = np.atleast_1d(read_data[c][t]['im_ws']).astype(int)
+ data[c][t]['mask_rles'] = np.atleast_1d(read_data[c][t]['mask_rles']).astype(str)
+ else:
+ data[c][t]['ids'] = np.empty(0).astype(int)
+ data[c][t]['scores'] = np.empty(0).astype(float)
+ data[c][t]['im_hs'] = np.empty(0).astype(int)
+ data[c][t]['im_ws'] = np.empty(0).astype(int)
+ data[c][t]['mask_rles'] = np.empty(0).astype(str)
+ return data
+
+
+def threshold(tdata, thresh):
+ """ Removes detections below a certian threshold ('thresh') score. """
+ new_data = {}
+ to_keep = tdata['scores'] > thresh
+ for field in ['ids', 'scores', 'im_hs', 'im_ws', 'mask_rles']:
+ new_data[field] = tdata[field][to_keep]
+ return new_data
+
+
+def create_coco_mask(mask_rles, im_hs, im_ws):
+ """ Converts mask as rle text (+ height and width) to encoded version used by pycocotools. """
+ coco_masks = [{'size': [h, w], 'counts': m.encode(encoding='UTF-8')}
+ for h, w, m in zip(im_hs, im_ws, mask_rles)]
+ return coco_masks
+
+
+def mask_iou(mask_rles1, mask_rles2, im_hs, im_ws, do_ioa=0):
+ """ Calculate mask IoU between two masks.
+ Further allows 'intersection over area' instead of IoU (over the area of mask_rle1).
+ Allows either to pass in 1 boolean for do_ioa for all mask_rles2 or also one for each mask_rles2.
+ It is recommended that mask_rles1 is a detection and mask_rles2 is a groundtruth.
+ """
+ coco_masks1 = create_coco_mask(mask_rles1, im_hs, im_ws)
+ coco_masks2 = create_coco_mask(mask_rles2, im_hs, im_ws)
+
+ if not hasattr(do_ioa, "__len__"):
+ do_ioa = [do_ioa]*len(coco_masks2)
+ assert(len(coco_masks2) == len(do_ioa))
+ if len(coco_masks1) == 0 or len(coco_masks2) == 0:
+ iou = np.zeros(len(coco_masks1), len(coco_masks2))
+ else:
+ iou = mask_utils.iou(coco_masks1, coco_masks2, do_ioa)
+ return iou
+
+
+def sort_by_score(t_data):
+ """ Sorts data by score """
+ sort_index = np.argsort(t_data['scores'])[::-1]
+ for k in t_data.keys():
+ t_data[k] = t_data[k][sort_index]
+ return t_data
+
+
+def mask_NMS(t_data, nms_threshold=0.5, already_sorted=False):
+ """ Remove redundant masks by performing non-maximum suppression (NMS) """
+
+ # Sort by score
+ if not already_sorted:
+ t_data = sort_by_score(t_data)
+
+ # Calculate the mask IoU between all detections in the timestep.
+ mask_ious_all = mask_iou(t_data['mask_rles'], t_data['mask_rles'], t_data['im_hs'], t_data['im_ws'])
+
+ # Determine which masks NMS should remove
+ # (those overlapping greater than nms_threshold with another mask that has a higher score)
+ num_dets = len(t_data['mask_rles'])
+ to_remove = [False for _ in range(num_dets)]
+ for i in range(num_dets):
+ if not to_remove[i]:
+ for j in range(i + 1, num_dets):
+ if mask_ious_all[i, j] > nms_threshold:
+ to_remove[j] = True
+
+ # Remove detections which should be removed
+ to_keep = np.logical_not(to_remove)
+ for k in t_data.keys():
+ t_data[k] = t_data[k][to_keep]
+
+ return t_data
+
+
+def non_overlap(t_data, already_sorted=False):
+ """ Enforces masks to be non-overlapping in an image, does this by putting masks 'on top of one another',
+ such that higher score masks 'occlude' and thus remove parts of lower scoring masks.
+
+ Help wanted: if anyone knows a way to do this WITHOUT converting the RLE to the np.array let me know, because that
+ would be MUCH more efficient. (I have tried, but haven't yet had success).
+ """
+
+ # Sort by score
+ if not already_sorted:
+ t_data = sort_by_score(t_data)
+
+ # Get coco masks
+ coco_masks = create_coco_mask(t_data['mask_rles'], t_data['im_hs'], t_data['im_ws'])
+
+ # Create a single np.array to hold all of the non-overlapping mask
+ masks_array = np.zeros((t_data['im_hs'][0], t_data['im_ws'][0]), 'uint8')
+
+ # Decode each mask into a np.array, and place it into the overall array for the whole frame.
+ # Since masks with the lowest score are placed first, they are 'partially overridden' by masks with a higher score
+ # if they overlap.
+ for i, mask in enumerate(coco_masks[::-1]):
+ masks_array[mask_utils.decode(mask).astype('bool')] = i + 1
+
+ # Encode the resulting np.array back into a set of coco_masks which are now non-overlapping.
+ num_dets = len(coco_masks)
+ for i, j in enumerate(range(1, num_dets + 1)[::-1]):
+ coco_masks[i] = mask_utils.encode(np.asfortranarray(masks_array == j, dtype=np.uint8))
+
+ # Convert from coco_mask back into our mask_rle format.
+ t_data['mask_rles'] = [m['counts'].decode("utf-8") for m in coco_masks]
+
+ return t_data
+
+
+def masks2boxes(mask_rles, im_hs, im_ws):
+ """ Extracts bounding boxes which surround a set of masks. """
+ coco_masks = create_coco_mask(mask_rles, im_hs, im_ws)
+ boxes = np.array([mask_utils.toBbox(x) for x in coco_masks])
+ if len(boxes) == 0:
+ boxes = np.empty((0, 4))
+ return boxes
+
+
+def box_iou(bboxes1, bboxes2, box_format='xywh', do_ioa=False, do_giou=False):
+ """ Calculates the IOU (intersection over union) between two arrays of boxes.
+ Allows variable box formats ('xywh' and 'x0y0x1y1').
+ If do_ioa (intersection over area), then calculates the intersection over the area of boxes1 - this is commonly
+ used to determine if detections are within crowd ignore region.
+ If do_giou (generalized intersection over union, then calculates giou.
+ """
+ if len(bboxes1) == 0 or len(bboxes2) == 0:
+ ious = np.zeros((len(bboxes1), len(bboxes2)))
+ return ious
+ if box_format in 'xywh':
+ # layout: (x0, y0, w, h)
+ bboxes1 = deepcopy(bboxes1)
+ bboxes2 = deepcopy(bboxes2)
+
+ bboxes1[:, 2] = bboxes1[:, 0] + bboxes1[:, 2]
+ bboxes1[:, 3] = bboxes1[:, 1] + bboxes1[:, 3]
+ bboxes2[:, 2] = bboxes2[:, 0] + bboxes2[:, 2]
+ bboxes2[:, 3] = bboxes2[:, 1] + bboxes2[:, 3]
+ elif box_format not in 'x0y0x1y1':
+ raise (Exception('box_format %s is not implemented' % box_format))
+
+ # layout: (x0, y0, x1, y1)
+ min_ = np.minimum(bboxes1[:, np.newaxis, :], bboxes2[np.newaxis, :, :])
+ max_ = np.maximum(bboxes1[:, np.newaxis, :], bboxes2[np.newaxis, :, :])
+ intersection = np.maximum(min_[..., 2] - max_[..., 0], 0) * np.maximum(min_[..., 3] - max_[..., 1], 0)
+ area1 = (bboxes1[..., 2] - bboxes1[..., 0]) * (bboxes1[..., 3] - bboxes1[..., 1])
+
+ if do_ioa:
+ ioas = np.zeros_like(intersection)
+ valid_mask = area1 > 0 + np.finfo('float').eps
+ ioas[valid_mask, :] = intersection[valid_mask, :] / area1[valid_mask][:, np.newaxis]
+
+ return ioas
+ else:
+ area2 = (bboxes2[..., 2] - bboxes2[..., 0]) * (bboxes2[..., 3] - bboxes2[..., 1])
+ union = area1[:, np.newaxis] + area2[np.newaxis, :] - intersection
+ intersection[area1 <= 0 + np.finfo('float').eps, :] = 0
+ intersection[:, area2 <= 0 + np.finfo('float').eps] = 0
+ intersection[union <= 0 + np.finfo('float').eps] = 0
+ union[union <= 0 + np.finfo('float').eps] = 1
+ ious = intersection / union
+
+ if do_giou:
+ enclosing_area = np.maximum(max_[..., 2] - min_[..., 0], 0) * np.maximum(max_[..., 3] - min_[..., 1], 0)
+ eps = 1e-7
+ # giou
+ ious = ious - ((enclosing_area - union) / (enclosing_area + eps))
+
+ return ious
+
+
+def match(match_scores):
+ match_rows, match_cols = linear_sum_assignment(-match_scores)
+ return match_rows, match_cols
+
+
+def write_seq(output_data, out_file):
+ out_loc = os.path.dirname(out_file)
+ if not os.path.exists(out_loc):
+ os.makedirs(out_loc, exist_ok=True)
+ fp = open(out_file, 'w', newline='')
+ writer = csv.writer(fp, delimiter=' ')
+ for row in output_data:
+ writer.writerow(row)
+ fp.close()
+
+
+def combine_classes(data):
+ """ Converts data from a class-separated to a class-combined format.
+ Input format: data['cls'][t] = {'ids', 'scores', 'im_hs', 'im_ws', 'mask_rles'}
+ Output format: data[t] = {'ids', 'scores', 'im_hs', 'im_ws', 'mask_rles', 'cls'}
+ """
+ output_data = [{} for _ in list(data.values())[0]]
+ for cls, cls_data in data.items():
+ for timestep, t_data in enumerate(cls_data):
+ for k in t_data.keys():
+ if k in output_data[timestep].keys():
+ output_data[timestep][k] += list(t_data[k])
+ else:
+ output_data[timestep][k] = list(t_data[k])
+ if 'cls' in output_data[timestep].keys():
+ output_data[timestep]['cls'] += [cls]*len(output_data[timestep]['ids'])
+ else:
+ output_data[timestep]['cls'] = [cls]*len(output_data[timestep]['ids'])
+
+ for timestep, t_data in enumerate(output_data):
+ for k in t_data.keys():
+ output_data[timestep][k] = np.array(output_data[timestep][k])
+
+ return output_data
+
+
+def save_as_png(t_data, out_file, im_h, im_w):
+ """ Save a set of segmentation masks into a PNG format, the same as used for the DAVIS dataset."""
+
+ if len(t_data['mask_rles']) > 0:
+ coco_masks = create_coco_mask(t_data['mask_rles'], t_data['im_hs'], t_data['im_ws'])
+
+ list_of_np_masks = [mask_utils.decode(mask) for mask in coco_masks]
+
+ png = np.zeros((t_data['im_hs'][0], t_data['im_ws'][0]))
+ for mask, c_id in zip(list_of_np_masks, t_data['ids']):
+ png[mask.astype("bool")] = c_id + 1
+ else:
+ png = np.zeros((im_h, im_w))
+
+ if not os.path.exists(os.path.dirname(out_file)):
+ os.makedirs(os.path.dirname(out_file))
+
+ colmap = (np.array(pascal_colormap) * 255).round().astype("uint8")
+ palimage = Image.new('P', (16, 16))
+ palimage.putpalette(colmap)
+ im = Image.fromarray(np.squeeze(png.astype("uint8")))
+ im2 = im.quantize(palette=palimage)
+ im2.save(out_file)
+
+
+def get_frame_size(data):
+ """ Gets frame height and width from data. """
+ for cls, cls_data in data.items():
+ for timestep, t_data in enumerate(cls_data):
+ if len(t_data['im_hs'] > 0):
+ im_h = t_data['im_hs'][0]
+ im_w = t_data['im_ws'][0]
+ return im_h, im_w
+ return None
diff --git a/val_utils/trackeval/baselines/non_overlap.py b/val_utils/trackeval/baselines/non_overlap.py
new file mode 100644
index 0000000000000000000000000000000000000000..43b131d01664501d9db45fbe9acf3b2d856a229f
--- /dev/null
+++ b/val_utils/trackeval/baselines/non_overlap.py
@@ -0,0 +1,92 @@
+"""
+Non-Overlap: Code to take in a set of raw detections and produce a set of non-overlapping detections from it.
+
+Author: Jonathon Luiten
+"""
+
+import os
+import sys
+from multiprocessing.pool import Pool
+from multiprocessing import freeze_support
+
+sys.path.insert(0, os.path.abspath(os.path.join(os.path.dirname(__file__), '..', '..')))
+from trackeval.baselines import baseline_utils as butils
+from trackeval.utils import get_code_path
+
+code_path = get_code_path()
+config = {
+ 'INPUT_FOL': os.path.join(code_path, 'data/detections/rob_mots/{split}/raw_supplied/data/'),
+ 'OUTPUT_FOL': os.path.join(code_path, 'data/detections/rob_mots/{split}/non_overlap_supplied/data/'),
+ 'SPLIT': 'train', # valid: 'train', 'val', 'test'.
+ 'Benchmarks': None, # If None, all benchmarks in SPLIT.
+
+ 'Num_Parallel_Cores': None, # If None, run without parallel.
+
+ 'THRESHOLD_NMS_MASK_IOU': 0.5,
+}
+
+
+def do_sequence(seq_file):
+
+ # Load input data from file (e.g. provided detections)
+ # data format: data['cls'][t] = {'ids', 'scores', 'im_hs', 'im_ws', 'mask_rles'}
+ data = butils.load_seq(seq_file)
+
+ # Converts data from a class-separated to a class-combined format.
+ # data[t] = {'ids', 'scores', 'im_hs', 'im_ws', 'mask_rles', 'cls'}
+ data = butils.combine_classes(data)
+
+ # Where to accumulate output data for writing out
+ output_data = []
+
+ # Run for each timestep.
+ for timestep, t_data in enumerate(data):
+
+ # Remove redundant masks by performing non-maximum suppression (NMS)
+ t_data = butils.mask_NMS(t_data, nms_threshold=config['THRESHOLD_NMS_MASK_IOU'])
+
+ # Perform non-overlap, to get non_overlapping masks.
+ t_data = butils.non_overlap(t_data, already_sorted=True)
+
+ # Save result in output format to write to file later.
+ # Output Format = [timestep ID class score im_h im_w mask_RLE]
+ for i in range(len(t_data['ids'])):
+ row = [timestep, int(t_data['ids'][i]), t_data['cls'][i], t_data['scores'][i], t_data['im_hs'][i],
+ t_data['im_ws'][i], t_data['mask_rles'][i]]
+ output_data.append(row)
+
+ # Write results to file
+ out_file = seq_file.replace(config['INPUT_FOL'].format(split=config['SPLIT']),
+ config['OUTPUT_FOL'].format(split=config['SPLIT']))
+ butils.write_seq(output_data, out_file)
+
+ print('DONE:', seq_file)
+
+
+if __name__ == '__main__':
+
+ # Required to fix bug in multiprocessing on windows.
+ freeze_support()
+
+ # Obtain list of sequences to run tracker for.
+ if config['Benchmarks']:
+ benchmarks = config['Benchmarks']
+ else:
+ benchmarks = ['davis_unsupervised', 'kitti_mots', 'youtube_vis', 'ovis', 'bdd_mots', 'tao']
+ if config['SPLIT'] != 'train':
+ benchmarks += ['waymo', 'mots_challenge']
+ seqs_todo = []
+ for bench in benchmarks:
+ bench_fol = os.path.join(config['INPUT_FOL'].format(split=config['SPLIT']), bench)
+ seqs_todo += [os.path.join(bench_fol, seq) for seq in os.listdir(bench_fol)]
+
+ # Run in parallel
+ if config['Num_Parallel_Cores']:
+ with Pool(config['Num_Parallel_Cores']) as pool:
+ results = pool.map(do_sequence, seqs_todo)
+
+ # Run in series
+ else:
+ for seq_todo in seqs_todo:
+ do_sequence(seq_todo)
+
diff --git a/val_utils/trackeval/baselines/pascal_colormap.py b/val_utils/trackeval/baselines/pascal_colormap.py
new file mode 100644
index 0000000000000000000000000000000000000000..b31f348931552bf0dde374a25cc878db5614511c
--- /dev/null
+++ b/val_utils/trackeval/baselines/pascal_colormap.py
@@ -0,0 +1,257 @@
+pascal_colormap = [
+ 0 , 0, 0,
+ 0.5020, 0, 0,
+ 0, 0.5020, 0,
+ 0.5020, 0.5020, 0,
+ 0, 0, 0.5020,
+ 0.5020, 0, 0.5020,
+ 0, 0.5020, 0.5020,
+ 0.5020, 0.5020, 0.5020,
+ 0.2510, 0, 0,
+ 0.7529, 0, 0,
+ 0.2510, 0.5020, 0,
+ 0.7529, 0.5020, 0,
+ 0.2510, 0, 0.5020,
+ 0.7529, 0, 0.5020,
+ 0.2510, 0.5020, 0.5020,
+ 0.7529, 0.5020, 0.5020,
+ 0, 0.2510, 0,
+ 0.5020, 0.2510, 0,
+ 0, 0.7529, 0,
+ 0.5020, 0.7529, 0,
+ 0, 0.2510, 0.5020,
+ 0.5020, 0.2510, 0.5020,
+ 0, 0.7529, 0.5020,
+ 0.5020, 0.7529, 0.5020,
+ 0.2510, 0.2510, 0,
+ 0.7529, 0.2510, 0,
+ 0.2510, 0.7529, 0,
+ 0.7529, 0.7529, 0,
+ 0.2510, 0.2510, 0.5020,
+ 0.7529, 0.2510, 0.5020,
+ 0.2510, 0.7529, 0.5020,
+ 0.7529, 0.7529, 0.5020,
+ 0, 0, 0.2510,
+ 0.5020, 0, 0.2510,
+ 0, 0.5020, 0.2510,
+ 0.5020, 0.5020, 0.2510,
+ 0, 0, 0.7529,
+ 0.5020, 0, 0.7529,
+ 0, 0.5020, 0.7529,
+ 0.5020, 0.5020, 0.7529,
+ 0.2510, 0, 0.2510,
+ 0.7529, 0, 0.2510,
+ 0.2510, 0.5020, 0.2510,
+ 0.7529, 0.5020, 0.2510,
+ 0.2510, 0, 0.7529,
+ 0.7529, 0, 0.7529,
+ 0.2510, 0.5020, 0.7529,
+ 0.7529, 0.5020, 0.7529,
+ 0, 0.2510, 0.2510,
+ 0.5020, 0.2510, 0.2510,
+ 0, 0.7529, 0.2510,
+ 0.5020, 0.7529, 0.2510,
+ 0, 0.2510, 0.7529,
+ 0.5020, 0.2510, 0.7529,
+ 0, 0.7529, 0.7529,
+ 0.5020, 0.7529, 0.7529,
+ 0.2510, 0.2510, 0.2510,
+ 0.7529, 0.2510, 0.2510,
+ 0.2510, 0.7529, 0.2510,
+ 0.7529, 0.7529, 0.2510,
+ 0.2510, 0.2510, 0.7529,
+ 0.7529, 0.2510, 0.7529,
+ 0.2510, 0.7529, 0.7529,
+ 0.7529, 0.7529, 0.7529,
+ 0.1255, 0, 0,
+ 0.6275, 0, 0,
+ 0.1255, 0.5020, 0,
+ 0.6275, 0.5020, 0,
+ 0.1255, 0, 0.5020,
+ 0.6275, 0, 0.5020,
+ 0.1255, 0.5020, 0.5020,
+ 0.6275, 0.5020, 0.5020,
+ 0.3765, 0, 0,
+ 0.8784, 0, 0,
+ 0.3765, 0.5020, 0,
+ 0.8784, 0.5020, 0,
+ 0.3765, 0, 0.5020,
+ 0.8784, 0, 0.5020,
+ 0.3765, 0.5020, 0.5020,
+ 0.8784, 0.5020, 0.5020,
+ 0.1255, 0.2510, 0,
+ 0.6275, 0.2510, 0,
+ 0.1255, 0.7529, 0,
+ 0.6275, 0.7529, 0,
+ 0.1255, 0.2510, 0.5020,
+ 0.6275, 0.2510, 0.5020,
+ 0.1255, 0.7529, 0.5020,
+ 0.6275, 0.7529, 0.5020,
+ 0.3765, 0.2510, 0,
+ 0.8784, 0.2510, 0,
+ 0.3765, 0.7529, 0,
+ 0.8784, 0.7529, 0,
+ 0.3765, 0.2510, 0.5020,
+ 0.8784, 0.2510, 0.5020,
+ 0.3765, 0.7529, 0.5020,
+ 0.8784, 0.7529, 0.5020,
+ 0.1255, 0, 0.2510,
+ 0.6275, 0, 0.2510,
+ 0.1255, 0.5020, 0.2510,
+ 0.6275, 0.5020, 0.2510,
+ 0.1255, 0, 0.7529,
+ 0.6275, 0, 0.7529,
+ 0.1255, 0.5020, 0.7529,
+ 0.6275, 0.5020, 0.7529,
+ 0.3765, 0, 0.2510,
+ 0.8784, 0, 0.2510,
+ 0.3765, 0.5020, 0.2510,
+ 0.8784, 0.5020, 0.2510,
+ 0.3765, 0, 0.7529,
+ 0.8784, 0, 0.7529,
+ 0.3765, 0.5020, 0.7529,
+ 0.8784, 0.5020, 0.7529,
+ 0.1255, 0.2510, 0.2510,
+ 0.6275, 0.2510, 0.2510,
+ 0.1255, 0.7529, 0.2510,
+ 0.6275, 0.7529, 0.2510,
+ 0.1255, 0.2510, 0.7529,
+ 0.6275, 0.2510, 0.7529,
+ 0.1255, 0.7529, 0.7529,
+ 0.6275, 0.7529, 0.7529,
+ 0.3765, 0.2510, 0.2510,
+ 0.8784, 0.2510, 0.2510,
+ 0.3765, 0.7529, 0.2510,
+ 0.8784, 0.7529, 0.2510,
+ 0.3765, 0.2510, 0.7529,
+ 0.8784, 0.2510, 0.7529,
+ 0.3765, 0.7529, 0.7529,
+ 0.8784, 0.7529, 0.7529,
+ 0, 0.1255, 0,
+ 0.5020, 0.1255, 0,
+ 0, 0.6275, 0,
+ 0.5020, 0.6275, 0,
+ 0, 0.1255, 0.5020,
+ 0.5020, 0.1255, 0.5020,
+ 0, 0.6275, 0.5020,
+ 0.5020, 0.6275, 0.5020,
+ 0.2510, 0.1255, 0,
+ 0.7529, 0.1255, 0,
+ 0.2510, 0.6275, 0,
+ 0.7529, 0.6275, 0,
+ 0.2510, 0.1255, 0.5020,
+ 0.7529, 0.1255, 0.5020,
+ 0.2510, 0.6275, 0.5020,
+ 0.7529, 0.6275, 0.5020,
+ 0, 0.3765, 0,
+ 0.5020, 0.3765, 0,
+ 0, 0.8784, 0,
+ 0.5020, 0.8784, 0,
+ 0, 0.3765, 0.5020,
+ 0.5020, 0.3765, 0.5020,
+ 0, 0.8784, 0.5020,
+ 0.5020, 0.8784, 0.5020,
+ 0.2510, 0.3765, 0,
+ 0.7529, 0.3765, 0,
+ 0.2510, 0.8784, 0,
+ 0.7529, 0.8784, 0,
+ 0.2510, 0.3765, 0.5020,
+ 0.7529, 0.3765, 0.5020,
+ 0.2510, 0.8784, 0.5020,
+ 0.7529, 0.8784, 0.5020,
+ 0, 0.1255, 0.2510,
+ 0.5020, 0.1255, 0.2510,
+ 0, 0.6275, 0.2510,
+ 0.5020, 0.6275, 0.2510,
+ 0, 0.1255, 0.7529,
+ 0.5020, 0.1255, 0.7529,
+ 0, 0.6275, 0.7529,
+ 0.5020, 0.6275, 0.7529,
+ 0.2510, 0.1255, 0.2510,
+ 0.7529, 0.1255, 0.2510,
+ 0.2510, 0.6275, 0.2510,
+ 0.7529, 0.6275, 0.2510,
+ 0.2510, 0.1255, 0.7529,
+ 0.7529, 0.1255, 0.7529,
+ 0.2510, 0.6275, 0.7529,
+ 0.7529, 0.6275, 0.7529,
+ 0, 0.3765, 0.2510,
+ 0.5020, 0.3765, 0.2510,
+ 0, 0.8784, 0.2510,
+ 0.5020, 0.8784, 0.2510,
+ 0, 0.3765, 0.7529,
+ 0.5020, 0.3765, 0.7529,
+ 0, 0.8784, 0.7529,
+ 0.5020, 0.8784, 0.7529,
+ 0.2510, 0.3765, 0.2510,
+ 0.7529, 0.3765, 0.2510,
+ 0.2510, 0.8784, 0.2510,
+ 0.7529, 0.8784, 0.2510,
+ 0.2510, 0.3765, 0.7529,
+ 0.7529, 0.3765, 0.7529,
+ 0.2510, 0.8784, 0.7529,
+ 0.7529, 0.8784, 0.7529,
+ 0.1255, 0.1255, 0,
+ 0.6275, 0.1255, 0,
+ 0.1255, 0.6275, 0,
+ 0.6275, 0.6275, 0,
+ 0.1255, 0.1255, 0.5020,
+ 0.6275, 0.1255, 0.5020,
+ 0.1255, 0.6275, 0.5020,
+ 0.6275, 0.6275, 0.5020,
+ 0.3765, 0.1255, 0,
+ 0.8784, 0.1255, 0,
+ 0.3765, 0.6275, 0,
+ 0.8784, 0.6275, 0,
+ 0.3765, 0.1255, 0.5020,
+ 0.8784, 0.1255, 0.5020,
+ 0.3765, 0.6275, 0.5020,
+ 0.8784, 0.6275, 0.5020,
+ 0.1255, 0.3765, 0,
+ 0.6275, 0.3765, 0,
+ 0.1255, 0.8784, 0,
+ 0.6275, 0.8784, 0,
+ 0.1255, 0.3765, 0.5020,
+ 0.6275, 0.3765, 0.5020,
+ 0.1255, 0.8784, 0.5020,
+ 0.6275, 0.8784, 0.5020,
+ 0.3765, 0.3765, 0,
+ 0.8784, 0.3765, 0,
+ 0.3765, 0.8784, 0,
+ 0.8784, 0.8784, 0,
+ 0.3765, 0.3765, 0.5020,
+ 0.8784, 0.3765, 0.5020,
+ 0.3765, 0.8784, 0.5020,
+ 0.8784, 0.8784, 0.5020,
+ 0.1255, 0.1255, 0.2510,
+ 0.6275, 0.1255, 0.2510,
+ 0.1255, 0.6275, 0.2510,
+ 0.6275, 0.6275, 0.2510,
+ 0.1255, 0.1255, 0.7529,
+ 0.6275, 0.1255, 0.7529,
+ 0.1255, 0.6275, 0.7529,
+ 0.6275, 0.6275, 0.7529,
+ 0.3765, 0.1255, 0.2510,
+ 0.8784, 0.1255, 0.2510,
+ 0.3765, 0.6275, 0.2510,
+ 0.8784, 0.6275, 0.2510,
+ 0.3765, 0.1255, 0.7529,
+ 0.8784, 0.1255, 0.7529,
+ 0.3765, 0.6275, 0.7529,
+ 0.8784, 0.6275, 0.7529,
+ 0.1255, 0.3765, 0.2510,
+ 0.6275, 0.3765, 0.2510,
+ 0.1255, 0.8784, 0.2510,
+ 0.6275, 0.8784, 0.2510,
+ 0.1255, 0.3765, 0.7529,
+ 0.6275, 0.3765, 0.7529,
+ 0.1255, 0.8784, 0.7529,
+ 0.6275, 0.8784, 0.7529,
+ 0.3765, 0.3765, 0.2510,
+ 0.8784, 0.3765, 0.2510,
+ 0.3765, 0.8784, 0.2510,
+ 0.8784, 0.8784, 0.2510,
+ 0.3765, 0.3765, 0.7529,
+ 0.8784, 0.3765, 0.7529,
+ 0.3765, 0.8784, 0.7529,
+ 0.8784, 0.8784, 0.7529]
\ No newline at end of file
diff --git a/val_utils/trackeval/baselines/stp.py b/val_utils/trackeval/baselines/stp.py
new file mode 100644
index 0000000000000000000000000000000000000000..c1c9d1e05a5958f35be9c034a623ecec047a2e6b
--- /dev/null
+++ b/val_utils/trackeval/baselines/stp.py
@@ -0,0 +1,144 @@
+"""
+STP: Simplest Tracker Possible
+
+Author: Jonathon Luiten
+
+This simple tracker, simply assigns track IDs which maximise the 'bounding box IoU' between previous tracks and current
+detections. It is also able to match detections to tracks at more than one timestep previously.
+"""
+
+import os
+import sys
+import numpy as np
+from multiprocessing.pool import Pool
+from multiprocessing import freeze_support
+
+sys.path.insert(0, os.path.abspath(os.path.join(os.path.dirname(__file__), '..', '..')))
+from trackeval.baselines import baseline_utils as butils
+from trackeval.utils import get_code_path
+
+code_path = get_code_path()
+config = {
+ 'INPUT_FOL': os.path.join(code_path, 'data/detections/rob_mots/{split}/non_overlap_supplied/data/'),
+ 'OUTPUT_FOL': os.path.join(code_path, 'data/trackers/rob_mots/{split}/STP/data/'),
+ 'SPLIT': 'train', # valid: 'train', 'val', 'test'.
+ 'Benchmarks': None, # If None, all benchmarks in SPLIT.
+
+ 'Num_Parallel_Cores': None, # If None, run without parallel.
+
+ 'DETECTION_THRESHOLD': 0.5,
+ 'ASSOCIATION_THRESHOLD': 1e-10,
+ 'MAX_FRAMES_SKIP': 7
+}
+
+
+def track_sequence(seq_file):
+
+ # Load input data from file (e.g. provided detections)
+ # data format: data['cls'][t] = {'ids', 'scores', 'im_hs', 'im_ws', 'mask_rles'}
+ data = butils.load_seq(seq_file)
+
+ # Where to accumulate output data for writing out
+ output_data = []
+
+ # To ensure IDs are unique per object across all classes.
+ curr_max_id = 0
+
+ # Run tracker for each class.
+ for cls, cls_data in data.items():
+
+ # Initialize container for holding previously tracked objects.
+ prev = {'boxes': np.empty((0, 4)),
+ 'ids': np.array([], np.int),
+ 'timesteps': np.array([])}
+
+ # Run tracker for each timestep.
+ for timestep, t_data in enumerate(cls_data):
+
+ # Threshold detections.
+ t_data = butils.threshold(t_data, config['DETECTION_THRESHOLD'])
+
+ # Convert mask dets to bounding boxes.
+ boxes = butils.masks2boxes(t_data['mask_rles'], t_data['im_hs'], t_data['im_ws'])
+
+ # Calculate IoU between previous and current frame dets.
+ ious = butils.box_iou(prev['boxes'], boxes)
+
+ # Score which decreases quickly for previous dets depending on how many timesteps before they come from.
+ prev_timestep_scores = np.power(10, -1 * prev['timesteps'])
+
+ # Matching score is such that it first tries to match 'most recent timesteps',
+ # and within each timestep maximised IoU.
+ match_scores = prev_timestep_scores[:, np.newaxis] * ious
+
+ # Find best matching between current dets and previous tracks.
+ match_rows, match_cols = butils.match(match_scores)
+
+ # Remove matches that have an IoU below a certain threshold.
+ actually_matched_mask = ious[match_rows, match_cols] > config['ASSOCIATION_THRESHOLD']
+ match_rows = match_rows[actually_matched_mask]
+ match_cols = match_cols[actually_matched_mask]
+
+ # Assign the prev track ID to the current dets if they were matched.
+ ids = np.nan * np.ones((len(boxes),), np.int)
+ ids[match_cols] = prev['ids'][match_rows]
+
+ # Create new track IDs for dets that were not matched to previous tracks.
+ num_not_matched = len(ids) - len(match_cols)
+ new_ids = np.arange(curr_max_id + 1, curr_max_id + num_not_matched + 1)
+ ids[np.isnan(ids)] = new_ids
+
+ # Update maximum ID to ensure future added tracks have a unique ID value.
+ curr_max_id += num_not_matched
+
+ # Drop tracks from 'previous tracks' if they have not been matched in the last MAX_FRAMES_SKIP frames.
+ unmatched_rows = [i for i in range(len(prev['ids'])) if
+ i not in match_rows and (prev['timesteps'][i] + 1 <= config['MAX_FRAMES_SKIP'])]
+
+ # Update the set of previous tracking results to include the newly tracked detections.
+ prev['ids'] = np.concatenate((ids, prev['ids'][unmatched_rows]), axis=0)
+ prev['boxes'] = np.concatenate((np.atleast_2d(boxes), np.atleast_2d(prev['boxes'][unmatched_rows])), axis=0)
+ prev['timesteps'] = np.concatenate((np.zeros((len(ids),)), prev['timesteps'][unmatched_rows] + 1), axis=0)
+
+ # Save result in output format to write to file later.
+ # Output Format = [timestep ID class score im_h im_w mask_RLE]
+ for i in range(len(t_data['ids'])):
+ row = [timestep, int(ids[i]), cls, t_data['scores'][i], t_data['im_hs'][i], t_data['im_ws'][i],
+ t_data['mask_rles'][i]]
+ output_data.append(row)
+
+ # Write results to file
+ out_file = seq_file.replace(config['INPUT_FOL'].format(split=config['SPLIT']),
+ config['OUTPUT_FOL'].format(split=config['SPLIT']))
+ butils.write_seq(output_data, out_file)
+
+ print('DONE:', seq_file)
+
+
+if __name__ == '__main__':
+
+ # Required to fix bug in multiprocessing on windows.
+ freeze_support()
+
+ # Obtain list of sequences to run tracker for.
+ if config['Benchmarks']:
+ benchmarks = config['Benchmarks']
+ else:
+ benchmarks = ['davis_unsupervised', 'kitti_mots', 'youtube_vis', 'ovis', 'bdd_mots', 'tao']
+ if config['SPLIT'] != 'train':
+ benchmarks += ['waymo', 'mots_challenge']
+ seqs_todo = []
+ for bench in benchmarks:
+ bench_fol = os.path.join(config['INPUT_FOL'].format(split=config['SPLIT']), bench)
+ seqs_todo += [os.path.join(bench_fol, seq) for seq in os.listdir(bench_fol)]
+
+ # Run in parallel
+ if config['Num_Parallel_Cores']:
+ with Pool(config['Num_Parallel_Cores']) as pool:
+ results = pool.map(track_sequence, seqs_todo)
+
+ # Run in series
+ else:
+ for seq_todo in seqs_todo:
+ track_sequence(seq_todo)
+
diff --git a/val_utils/trackeval/baselines/thresholder.py b/val_utils/trackeval/baselines/thresholder.py
new file mode 100644
index 0000000000000000000000000000000000000000..c589e10b95da311c03ed1045bc1d6af8f1a8c90e
--- /dev/null
+++ b/val_utils/trackeval/baselines/thresholder.py
@@ -0,0 +1,92 @@
+"""
+Thresholder
+
+Author: Jonathon Luiten
+
+Simply reads in a set of detection, thresholds them at a certain score threshold, and writes them out again.
+"""
+
+import os
+import sys
+from multiprocessing.pool import Pool
+from multiprocessing import freeze_support
+
+sys.path.insert(0, os.path.abspath(os.path.join(os.path.dirname(__file__), '..', '..')))
+from trackeval.baselines import baseline_utils as butils
+from trackeval.utils import get_code_path
+
+THRESHOLD = 0.2
+
+code_path = get_code_path()
+config = {
+ 'INPUT_FOL': os.path.join(code_path, 'data/detections/rob_mots/{split}/non_overlap_supplied/data/'),
+ 'OUTPUT_FOL': os.path.join(code_path, 'data/detections/rob_mots/{split}/threshold_' + str(100*THRESHOLD) + '/data/'),
+ 'SPLIT': 'train', # valid: 'train', 'val', 'test'.
+ 'Benchmarks': None, # If None, all benchmarks in SPLIT.
+
+ 'Num_Parallel_Cores': None, # If None, run without parallel.
+
+ 'DETECTION_THRESHOLD': THRESHOLD,
+}
+
+
+def do_sequence(seq_file):
+
+ # Load input data from file (e.g. provided detections)
+ # data format: data['cls'][t] = {'ids', 'scores', 'im_hs', 'im_ws', 'mask_rles'}
+ data = butils.load_seq(seq_file)
+
+ # Where to accumulate output data for writing out
+ output_data = []
+
+ # Run for each class.
+ for cls, cls_data in data.items():
+
+ # Run for each timestep.
+ for timestep, t_data in enumerate(cls_data):
+
+ # Threshold detections.
+ t_data = butils.threshold(t_data, config['DETECTION_THRESHOLD'])
+
+ # Save result in output format to write to file later.
+ # Output Format = [timestep ID class score im_h im_w mask_RLE]
+ for i in range(len(t_data['ids'])):
+ row = [timestep, int(t_data['ids'][i]), cls, t_data['scores'][i], t_data['im_hs'][i],
+ t_data['im_ws'][i], t_data['mask_rles'][i]]
+ output_data.append(row)
+
+ # Write results to file
+ out_file = seq_file.replace(config['INPUT_FOL'].format(split=config['SPLIT']),
+ config['OUTPUT_FOL'].format(split=config['SPLIT']))
+ butils.write_seq(output_data, out_file)
+
+ print('DONE:', seq_todo)
+
+
+if __name__ == '__main__':
+
+ # Required to fix bug in multiprocessing on windows.
+ freeze_support()
+
+ # Obtain list of sequences to run tracker for.
+ if config['Benchmarks']:
+ benchmarks = config['Benchmarks']
+ else:
+ benchmarks = ['davis_unsupervised', 'kitti_mots', 'youtube_vis', 'ovis', 'bdd_mots', 'tao']
+ if config['SPLIT'] != 'train':
+ benchmarks += ['waymo', 'mots_challenge']
+ seqs_todo = []
+ for bench in benchmarks:
+ bench_fol = os.path.join(config['INPUT_FOL'].format(split=config['SPLIT']), bench)
+ seqs_todo += [os.path.join(bench_fol, seq) for seq in os.listdir(bench_fol)]
+
+ # Run in parallel
+ if config['Num_Parallel_Cores']:
+ with Pool(config['Num_Parallel_Cores']) as pool:
+ results = pool.map(do_sequence, seqs_todo)
+
+ # Run in series
+ else:
+ for seq_todo in seqs_todo:
+ do_sequence(seq_todo)
+
diff --git a/val_utils/trackeval/baselines/vizualize.py b/val_utils/trackeval/baselines/vizualize.py
new file mode 100644
index 0000000000000000000000000000000000000000..568a30373cdb34cf122c9fd05b8496666d75f726
--- /dev/null
+++ b/val_utils/trackeval/baselines/vizualize.py
@@ -0,0 +1,94 @@
+"""
+Vizualize: Code which converts .txt rle tracking results into a visual .png format.
+
+Author: Jonathon Luiten
+"""
+
+import os
+import sys
+from multiprocessing.pool import Pool
+from multiprocessing import freeze_support
+
+sys.path.insert(0, os.path.abspath(os.path.join(os.path.dirname(__file__), '..', '..')))
+from trackeval.baselines import baseline_utils as butils
+from trackeval.utils import get_code_path
+from trackeval.datasets.rob_mots_classmap import cls_id_to_name
+
+code_path = get_code_path()
+config = {
+ # Tracker format:
+ 'INPUT_FOL': os.path.join(code_path, 'data/trackers/rob_mots/{split}/STP/data/{bench}'),
+ 'OUTPUT_FOL': os.path.join(code_path, 'data/viz/rob_mots/{split}/STP/data/{bench}'),
+ # GT format:
+ # 'INPUT_FOL': os.path.join(code_path, 'data/gt/rob_mots/{split}/{bench}/data/'),
+ # 'OUTPUT_FOL': os.path.join(code_path, 'data/gt_viz/rob_mots/{split}/{bench}/'),
+ 'SPLIT': 'train', # valid: 'train', 'val', 'test'.
+ 'Benchmarks': None, # If None, all benchmarks in SPLIT.
+ 'Num_Parallel_Cores': None, # If None, run without parallel.
+}
+
+
+def do_sequence(seq_file):
+ # Folder to save resulting visualization in
+ out_fol = seq_file.replace(config['INPUT_FOL'].format(split=config['SPLIT'], bench=bench),
+ config['OUTPUT_FOL'].format(split=config['SPLIT'], bench=bench)).replace('.txt', '')
+
+ # Load input data from file (e.g. provided detections)
+ # data format: data['cls'][t] = {'ids', 'scores', 'im_hs', 'im_ws', 'mask_rles'}
+ data = butils.load_seq(seq_file)
+
+ # Get frame size for visualizing empty frames
+ im_h, im_w = butils.get_frame_size(data)
+
+ # First run for each class.
+ for cls, cls_data in data.items():
+
+ if cls >= 100:
+ continue
+
+ # Run for each timestep.
+ for timestep, t_data in enumerate(cls_data):
+ # Save out visualization
+ out_file = os.path.join(out_fol, cls_id_to_name[cls], str(timestep).zfill(5) + '.png')
+ butils.save_as_png(t_data, out_file, im_h, im_w)
+
+
+ # Then run for all classes combined
+ # Converts data from a class-separated to a class-combined format.
+ data = butils.combine_classes(data)
+
+ # Run for each timestep.
+ for timestep, t_data in enumerate(data):
+ # Save out visualization
+ out_file = os.path.join(out_fol, 'all_classes', str(timestep).zfill(5) + '.png')
+ butils.save_as_png(t_data, out_file, im_h, im_w)
+
+ print('DONE:', seq_file)
+
+
+if __name__ == '__main__':
+
+ # Required to fix bug in multiprocessing on windows.
+ freeze_support()
+
+ # Obtain list of sequences to run tracker for.
+ if config['Benchmarks']:
+ benchmarks = config['Benchmarks']
+ else:
+ benchmarks = ['davis_unsupervised', 'kitti_mots', 'youtube_vis', 'ovis', 'bdd_mots', 'tao']
+ if config['SPLIT'] != 'train':
+ benchmarks += ['waymo', 'mots_challenge']
+ seqs_todo = []
+ for bench in benchmarks:
+ bench_fol = config['INPUT_FOL'].format(split=config['SPLIT'], bench=bench)
+ seqs_todo += [os.path.join(bench_fol, seq) for seq in os.listdir(bench_fol)]
+
+ # Run in parallel
+ if config['Num_Parallel_Cores']:
+ with Pool(config['Num_Parallel_Cores']) as pool:
+ results = pool.map(do_sequence, seqs_todo)
+
+ # Run in series
+ else:
+ for seq_todo in seqs_todo:
+ do_sequence(seq_todo)
diff --git a/val_utils/trackeval/datasets/__init__.py b/val_utils/trackeval/datasets/__init__.py
new file mode 100644
index 0000000000000000000000000000000000000000..4fdfa9dd590c6283d56e86419b863782fa619029
--- /dev/null
+++ b/val_utils/trackeval/datasets/__init__.py
@@ -0,0 +1,17 @@
+from .kitti_2d_box import Kitti2DBox
+from .kitti_mots import KittiMOTS
+from .mot_challenge_2d_box import MotChallenge2DBox
+from .mots_challenge import MOTSChallenge
+from .bdd100k import BDD100K
+from .davis import DAVIS
+from .tao import TAO
+from .tao_ow import TAO_OW
+try:
+ from .burst import BURST
+ from .burst_ow import BURST_OW
+except ImportError as err:
+ print(f"Error importing BURST due to missing underlying dependency: {err}")
+from .youtube_vis import YouTubeVIS
+from .head_tracking_challenge import HeadTrackingChallenge
+from .rob_mots import RobMOTS
+from .person_path_22 import PersonPath22
diff --git a/val_utils/trackeval/datasets/_base_dataset.py b/val_utils/trackeval/datasets/_base_dataset.py
new file mode 100644
index 0000000000000000000000000000000000000000..64bf9fc01dd3b7c45f10d1c874228aa32a062908
--- /dev/null
+++ b/val_utils/trackeval/datasets/_base_dataset.py
@@ -0,0 +1,326 @@
+import csv
+import io
+import zipfile
+import os
+import traceback
+import numpy as np
+from copy import deepcopy
+from abc import ABC, abstractmethod
+from .. import _timing
+from ..utils import TrackEvalException
+
+
+class _BaseDataset(ABC):
+ @abstractmethod
+ def __init__(self):
+ self.tracker_list = None
+ self.seq_list = None
+ self.class_list = None
+ self.output_fol = None
+ self.output_sub_fol = None
+ self.should_classes_combine = True
+ self.use_super_categories = False
+
+ # Functions to implement:
+
+ @staticmethod
+ @abstractmethod
+ def get_default_dataset_config():
+ ...
+
+ @abstractmethod
+ def _load_raw_file(self, tracker, seq, is_gt):
+ ...
+
+ @_timing.time
+ @abstractmethod
+ def get_preprocessed_seq_data(self, raw_data, cls):
+ ...
+
+ @abstractmethod
+ def _calculate_similarities(self, gt_dets_t, tracker_dets_t):
+ ...
+
+ # Helper functions for all datasets:
+
+ @classmethod
+ def get_class_name(cls):
+ return cls.__name__
+
+ def get_name(self):
+ return self.get_class_name()
+
+ def get_output_fol(self, tracker):
+ return os.path.join(self.output_fol, tracker, self.output_sub_fol)
+
+ def get_display_name(self, tracker):
+ """ Can be overwritten if the trackers name (in files) is different to how it should be displayed.
+ By default this method just returns the trackers name as is.
+ """
+ return tracker
+
+ def get_eval_info(self):
+ """Return info about the dataset needed for the Evaluator"""
+ return self.tracker_list, self.seq_list, self.class_list
+
+ @_timing.time
+ def get_raw_seq_data(self, tracker, seq):
+ """ Loads raw data (tracker and ground-truth) for a single tracker on a single sequence.
+ Raw data includes all of the information needed for both preprocessing and evaluation, for all classes.
+ A later function (get_processed_seq_data) will perform such preprocessing and extract relevant information for
+ the evaluation of each class.
+
+ This returns a dict which contains the fields:
+ [num_timesteps]: integer
+ [gt_ids, tracker_ids, gt_classes, tracker_classes, tracker_confidences]:
+ list (for each timestep) of 1D NDArrays (for each det).
+ [gt_dets, tracker_dets, gt_crowd_ignore_regions]: list (for each timestep) of lists of detections.
+ [similarity_scores]: list (for each timestep) of 2D NDArrays.
+ [gt_extras]: dict (for each extra) of lists (for each timestep) of 1D NDArrays (for each det).
+
+ gt_extras contains dataset specific information used for preprocessing such as occlusion and truncation levels.
+
+ Note that similarities are extracted as part of the dataset and not the metric, because almost all metrics are
+ independent of the exact method of calculating the similarity. However datasets are not (e.g. segmentation
+ masks vs 2D boxes vs 3D boxes).
+ We calculate the similarity before preprocessing because often both preprocessing and evaluation require it and
+ we don't wish to calculate this twice.
+ We calculate similarity between all gt and tracker classes (not just each class individually) to allow for
+ calculation of metrics such as class confusion matrices. Typically the impact of this on performance is low.
+ """
+ # Load raw data.
+ raw_gt_data = self._load_raw_file(tracker, seq, is_gt=True)
+ raw_tracker_data = self._load_raw_file(tracker, seq, is_gt=False)
+ raw_data = {**raw_tracker_data, **raw_gt_data} # Merges dictionaries
+
+ # Calculate similarities for each timestep.
+ similarity_scores = []
+ for t, (gt_dets_t, tracker_dets_t) in enumerate(zip(raw_data['gt_dets'], raw_data['tracker_dets'])):
+ ious = self._calculate_similarities(gt_dets_t, tracker_dets_t)
+ similarity_scores.append(ious)
+ raw_data['similarity_scores'] = similarity_scores
+ return raw_data
+
+ @staticmethod
+ def _load_simple_text_file(file, time_col=0, id_col=None, remove_negative_ids=False, valid_filter=None,
+ crowd_ignore_filter=None, convert_filter=None, is_zipped=False, zip_file=None,
+ force_delimiters=None):
+ """ Function that loads data which is in a commonly used text file format.
+ Assumes each det is given by one row of a text file.
+ There is no limit to the number or meaning of each column,
+ however one column needs to give the timestep of each det (time_col) which is default col 0.
+
+ The file dialect (deliminator, num cols, etc) is determined automatically.
+ This function automatically separates dets by timestep,
+ and is much faster than alternatives such as np.loadtext or pandas.
+
+ If remove_negative_ids is True and id_col is not None, dets with negative values in id_col are excluded.
+ These are not excluded from ignore data.
+
+ valid_filter can be used to only include certain classes.
+ It is a dict with ints as keys, and lists as values,
+ such that a row is included if "row[key].lower() is in value" for all key/value pairs in the dict.
+ If None, all classes are included.
+
+ crowd_ignore_filter can be used to read crowd_ignore regions separately. It has the same format as valid filter.
+
+ convert_filter can be used to convert value read to another format.
+ This is used most commonly to convert classes given as string to a class id.
+ This is a dict such that the key is the column to convert, and the value is another dict giving the mapping.
+
+ Optionally, input files could be a zip of multiple text files for storage efficiency.
+
+ Returns read_data and ignore_data.
+ Each is a dict (with keys as timesteps as strings) of lists (over dets) of lists (over column values).
+ Note that all data is returned as strings, and must be converted to float/int later if needed.
+ Note that timesteps will not be present in the returned dict keys if there are no dets for them
+ """
+
+ if remove_negative_ids and id_col is None:
+ raise TrackEvalException('remove_negative_ids is True, but id_col is not given.')
+ if crowd_ignore_filter is None:
+ crowd_ignore_filter = {}
+ if convert_filter is None:
+ convert_filter = {}
+ try:
+ if is_zipped: # Either open file directly or within a zip.
+ if zip_file is None:
+ raise TrackEvalException('is_zipped set to True, but no zip_file is given.')
+ archive = zipfile.ZipFile(os.path.join(zip_file), 'r')
+ fp = io.TextIOWrapper(archive.open(file, 'r'))
+ else:
+ fp = open(file)
+ read_data = {}
+ crowd_ignore_data = {}
+ fp.seek(0, os.SEEK_END)
+ # check if file is empty
+ if fp.tell():
+ fp.seek(0)
+ dialect = csv.Sniffer().sniff(fp.readline(), delimiters=force_delimiters) # Auto determine structure.
+ dialect.skipinitialspace = True # Deal with extra spaces between columns
+ fp.seek(0)
+ reader = csv.reader(fp, dialect)
+ for row in reader:
+ try:
+ # Deal with extra trailing spaces at the end of rows
+ if row[-1] in '':
+ row = row[:-1]
+ timestep = str(int(float(row[time_col])))
+ # Read ignore regions separately.
+ is_ignored = False
+ for ignore_key, ignore_value in crowd_ignore_filter.items():
+ if row[ignore_key].lower() in ignore_value:
+ # Convert values in one column (e.g. string to id)
+ for convert_key, convert_value in convert_filter.items():
+ row[convert_key] = convert_value[row[convert_key].lower()]
+ # Save data separated by timestep.
+ if timestep in crowd_ignore_data.keys():
+ crowd_ignore_data[timestep].append(row)
+ else:
+ crowd_ignore_data[timestep] = [row]
+ is_ignored = True
+ if is_ignored: # if det is an ignore region, it cannot be a normal det.
+ continue
+ # Exclude some dets if not valid.
+ if valid_filter is not None:
+ for key, value in valid_filter.items():
+ if row[key].lower() not in value:
+ continue
+ if remove_negative_ids:
+ if int(float(row[id_col])) < 0:
+ continue
+ # Convert values in one column (e.g. string to id)
+ for convert_key, convert_value in convert_filter.items():
+ row[convert_key] = convert_value[row[convert_key].lower()]
+ # Save data separated by timestep.
+ if timestep in read_data.keys():
+ read_data[timestep].append(row)
+ else:
+ read_data[timestep] = [row]
+ except Exception:
+ exc_str_init = 'In file %s the following line cannot be read correctly: \n' % os.path.basename(
+ file)
+ exc_str = ' '.join([exc_str_init]+row)
+ raise TrackEvalException(exc_str)
+ fp.close()
+ except Exception:
+ print('Error loading file: %s, printing traceback.' % file)
+ traceback.print_exc()
+ raise TrackEvalException(
+ 'File %s cannot be read because it is either not present or invalidly formatted' % os.path.basename(
+ file))
+ return read_data, crowd_ignore_data
+
+ @staticmethod
+ def _calculate_mask_ious(masks1, masks2, is_encoded=False, do_ioa=False):
+ """ Calculates the IOU (intersection over union) between two arrays of segmentation masks.
+ If is_encoded a run length encoding with pycocotools is assumed as input format, otherwise an input of numpy
+ arrays of the shape (num_masks, height, width) is assumed and the encoding is performed.
+ If do_ioa (intersection over area) , then calculates the intersection over the area of masks1 - this is commonly
+ used to determine if detections are within crowd ignore region.
+ :param masks1: first set of masks (numpy array of shape (num_masks, height, width) if not encoded,
+ else pycocotools rle encoded format)
+ :param masks2: second set of masks (numpy array of shape (num_masks, height, width) if not encoded,
+ else pycocotools rle encoded format)
+ :param is_encoded: whether the input is in pycocotools rle encoded format
+ :param do_ioa: whether to perform IoA computation
+ :return: the IoU/IoA scores
+ """
+
+ # Only loaded when run to reduce minimum requirements
+ from pycocotools import mask as mask_utils
+
+ # use pycocotools for run length encoding of masks
+ if not is_encoded:
+ masks1 = mask_utils.encode(np.array(np.transpose(masks1, (1, 2, 0)), order='F'))
+ masks2 = mask_utils.encode(np.array(np.transpose(masks2, (1, 2, 0)), order='F'))
+
+ # use pycocotools for iou computation of rle encoded masks
+ ious = mask_utils.iou(masks1, masks2, [do_ioa]*len(masks2))
+ if len(masks1) == 0 or len(masks2) == 0:
+ ious = np.asarray(ious).reshape(len(masks1), len(masks2))
+ assert (ious >= 0 - np.finfo('float').eps).all()
+ assert (ious <= 1 + np.finfo('float').eps).all()
+
+ return ious
+
+ @staticmethod
+ def _calculate_box_ious(bboxes1, bboxes2, box_format='xywh', do_ioa=False):
+ """ Calculates the IOU (intersection over union) between two arrays of boxes.
+ Allows variable box formats ('xywh' and 'x0y0x1y1').
+ If do_ioa (intersection over area) , then calculates the intersection over the area of boxes1 - this is commonly
+ used to determine if detections are within crowd ignore region.
+ """
+ if box_format in 'xywh':
+ # layout: (x0, y0, w, h)
+ bboxes1 = deepcopy(bboxes1)
+ bboxes2 = deepcopy(bboxes2)
+
+ bboxes1[:, 2] = bboxes1[:, 0] + bboxes1[:, 2]
+ bboxes1[:, 3] = bboxes1[:, 1] + bboxes1[:, 3]
+ bboxes2[:, 2] = bboxes2[:, 0] + bboxes2[:, 2]
+ bboxes2[:, 3] = bboxes2[:, 1] + bboxes2[:, 3]
+ elif box_format not in 'x0y0x1y1':
+ raise (TrackEvalException('box_format %s is not implemented' % box_format))
+
+ # layout: (x0, y0, x1, y1)
+ min_ = np.minimum(bboxes1[:, np.newaxis, :], bboxes2[np.newaxis, :, :])
+ max_ = np.maximum(bboxes1[:, np.newaxis, :], bboxes2[np.newaxis, :, :])
+ intersection = np.maximum(min_[..., 2] - max_[..., 0], 0) * np.maximum(min_[..., 3] - max_[..., 1], 0)
+ area1 = (bboxes1[..., 2] - bboxes1[..., 0]) * (bboxes1[..., 3] - bboxes1[..., 1])
+
+ if do_ioa:
+ ioas = np.zeros_like(intersection)
+ valid_mask = area1 > 0 + np.finfo('float').eps
+ ioas[valid_mask, :] = intersection[valid_mask, :] / area1[valid_mask][:, np.newaxis]
+
+ return ioas
+ else:
+ area2 = (bboxes2[..., 2] - bboxes2[..., 0]) * (bboxes2[..., 3] - bboxes2[..., 1])
+ union = area1[:, np.newaxis] + area2[np.newaxis, :] - intersection
+ intersection[area1 <= 0 + np.finfo('float').eps, :] = 0
+ intersection[:, area2 <= 0 + np.finfo('float').eps] = 0
+ intersection[union <= 0 + np.finfo('float').eps] = 0
+ union[union <= 0 + np.finfo('float').eps] = 1
+ ious = intersection / union
+ return ious
+
+ @staticmethod
+ def _calculate_euclidean_similarity(dets1, dets2, zero_distance=2.0):
+ """ Calculates the euclidean distance between two sets of detections, and then converts this into a similarity
+ measure with values between 0 and 1 using the following formula: sim = max(0, 1 - dist/zero_distance).
+ The default zero_distance of 2.0, corresponds to the default used in MOT15_3D, such that a 0.5 similarity
+ threshold corresponds to a 1m distance threshold for TPs.
+ """
+ dist = np.linalg.norm(dets1[:, np.newaxis]-dets2[np.newaxis, :], axis=2)
+ sim = np.maximum(0, 1 - dist/zero_distance)
+ return sim
+
+ @staticmethod
+ def _check_unique_ids(data, after_preproc=False):
+ """Check the requirement that the tracker_ids and gt_ids are unique per timestep"""
+ gt_ids = data['gt_ids']
+ tracker_ids = data['tracker_ids']
+ for t, (gt_ids_t, tracker_ids_t) in enumerate(zip(gt_ids, tracker_ids)):
+ if len(tracker_ids_t) > 0:
+ unique_ids, counts = np.unique(tracker_ids_t, return_counts=True)
+ if np.max(counts) != 1:
+ duplicate_ids = unique_ids[counts > 1]
+ exc_str_init = 'Tracker predicts the same ID more than once in a single timestep ' \
+ '(seq: %s, frame: %i, ids:' % (data['seq'], t+1)
+ exc_str = ' '.join([exc_str_init] + [str(d) for d in duplicate_ids]) + ')'
+ if after_preproc:
+ exc_str_init += '\n Note that this error occurred after preprocessing (but not before), ' \
+ 'so ids may not be as in file, and something seems wrong with preproc.'
+ raise TrackEvalException(exc_str)
+ if len(gt_ids_t) > 0:
+ unique_ids, counts = np.unique(gt_ids_t, return_counts=True)
+ if np.max(counts) != 1:
+ duplicate_ids = unique_ids[counts > 1]
+ exc_str_init = 'Ground-truth has the same ID more than once in a single timestep ' \
+ '(seq: %s, frame: %i, ids:' % (data['seq'], t+1)
+ exc_str = ' '.join([exc_str_init] + [str(d) for d in duplicate_ids]) + ')'
+ if after_preproc:
+ exc_str_init += '\n Note that this error occurred after preprocessing (but not before), ' \
+ 'so ids may not be as in file, and something seems wrong with preproc.'
+ raise TrackEvalException(exc_str)
diff --git a/val_utils/trackeval/datasets/bdd100k.py b/val_utils/trackeval/datasets/bdd100k.py
new file mode 100644
index 0000000000000000000000000000000000000000..cc4fd06ee49e520e9a8887239532816074aea41f
--- /dev/null
+++ b/val_utils/trackeval/datasets/bdd100k.py
@@ -0,0 +1,302 @@
+
+import os
+import json
+import numpy as np
+from scipy.optimize import linear_sum_assignment
+from ..utils import TrackEvalException
+from ._base_dataset import _BaseDataset
+from .. import utils
+from .. import _timing
+
+
+class BDD100K(_BaseDataset):
+ """Dataset class for BDD100K tracking"""
+
+ @staticmethod
+ def get_default_dataset_config():
+ """Default class config values"""
+ code_path = utils.get_code_path()
+ default_config = {
+ 'GT_FOLDER': os.path.join(code_path, 'data/gt/bdd100k/bdd100k_val'), # Location of GT data
+ 'TRACKERS_FOLDER': os.path.join(code_path, 'data/trackers/bdd100k/bdd100k_val'), # Trackers location
+ 'OUTPUT_FOLDER': None, # Where to save eval results (if None, same as TRACKERS_FOLDER)
+ 'TRACKERS_TO_EVAL': None, # Filenames of trackers to eval (if None, all in folder)
+ 'CLASSES_TO_EVAL': ['pedestrian', 'rider', 'car', 'bus', 'truck', 'train', 'motorcycle', 'bicycle'],
+ # Valid: ['pedestrian', 'rider', 'car', 'bus', 'truck', 'train', 'motorcycle', 'bicycle']
+ 'SPLIT_TO_EVAL': 'val', # Valid: 'training', 'val',
+ 'INPUT_AS_ZIP': False, # Whether tracker input files are zipped
+ 'PRINT_CONFIG': True, # Whether to print current config
+ 'TRACKER_SUB_FOLDER': 'data', # Tracker files are in TRACKER_FOLDER/tracker_name/TRACKER_SUB_FOLDER
+ 'OUTPUT_SUB_FOLDER': '', # Output files are saved in OUTPUT_FOLDER/tracker_name/OUTPUT_SUB_FOLDER
+ 'TRACKER_DISPLAY_NAMES': None, # Names of trackers to display, if None: TRACKERS_TO_EVAL
+ }
+ return default_config
+
+ def __init__(self, config=None):
+ """Initialise dataset, checking that all required files are present"""
+ super().__init__()
+ # Fill non-given config values with defaults
+ self.config = utils.init_config(config, self.get_default_dataset_config(), self.get_name())
+ self.gt_fol = self.config['GT_FOLDER']
+ self.tracker_fol = self.config['TRACKERS_FOLDER']
+ self.should_classes_combine = True
+ self.use_super_categories = True
+
+ self.output_fol = self.config['OUTPUT_FOLDER']
+ if self.output_fol is None:
+ self.output_fol = self.tracker_fol
+
+ self.tracker_sub_fol = self.config['TRACKER_SUB_FOLDER']
+ self.output_sub_fol = self.config['OUTPUT_SUB_FOLDER']
+
+ # Get classes to eval
+ self.valid_classes = ['pedestrian', 'rider', 'car', 'bus', 'truck', 'train', 'motorcycle', 'bicycle']
+ self.class_list = [cls.lower() if cls.lower() in self.valid_classes else None
+ for cls in self.config['CLASSES_TO_EVAL']]
+ if not all(self.class_list):
+ raise TrackEvalException('Attempted to evaluate an invalid class. Only classes [pedestrian, rider, car, '
+ 'bus, truck, train, motorcycle, bicycle] are valid.')
+ self.super_categories = {"HUMAN": [cls for cls in ["pedestrian", "rider"] if cls in self.class_list],
+ "VEHICLE": [cls for cls in ["car", "truck", "bus", "train"] if cls in self.class_list],
+ "BIKE": [cls for cls in ["motorcycle", "bicycle"] if cls in self.class_list]}
+ self.distractor_classes = ['other person', 'trailer', 'other vehicle']
+ self.class_name_to_class_id = {'pedestrian': 1, 'rider': 2, 'other person': 3, 'car': 4, 'bus': 5, 'truck': 6,
+ 'train': 7, 'trailer': 8, 'other vehicle': 9, 'motorcycle': 10, 'bicycle': 11}
+
+ # Get sequences to eval
+ self.seq_list = []
+ self.seq_lengths = {}
+
+ self.seq_list = [seq_file.replace('.json', '') for seq_file in os.listdir(self.gt_fol)]
+
+ # Get trackers to eval
+ if self.config['TRACKERS_TO_EVAL'] is None:
+ self.tracker_list = os.listdir(self.tracker_fol)
+ else:
+ self.tracker_list = self.config['TRACKERS_TO_EVAL']
+
+ if self.config['TRACKER_DISPLAY_NAMES'] is None:
+ self.tracker_to_disp = dict(zip(self.tracker_list, self.tracker_list))
+ elif (self.config['TRACKERS_TO_EVAL'] is not None) and (
+ len(self.config['TRACKER_DISPLAY_NAMES']) == len(self.tracker_list)):
+ self.tracker_to_disp = dict(zip(self.tracker_list, self.config['TRACKER_DISPLAY_NAMES']))
+ else:
+ raise TrackEvalException('List of tracker files and tracker display names do not match.')
+
+ for tracker in self.tracker_list:
+ for seq in self.seq_list:
+ curr_file = os.path.join(self.tracker_fol, tracker, self.tracker_sub_fol, seq + '.json')
+ if not os.path.isfile(curr_file):
+ print('Tracker file not found: ' + curr_file)
+ raise TrackEvalException(
+ 'Tracker file not found: ' + tracker + '/' + self.tracker_sub_fol + '/' + os.path.basename(
+ curr_file))
+
+ def get_display_name(self, tracker):
+ return self.tracker_to_disp[tracker]
+
+ def _load_raw_file(self, tracker, seq, is_gt):
+ """Load a file (gt or tracker) in the BDD100K format
+
+ If is_gt, this returns a dict which contains the fields:
+ [gt_ids, gt_classes] : list (for each timestep) of 1D NDArrays (for each det).
+ [gt_dets, gt_crowd_ignore_regions]: list (for each timestep) of lists of detections.
+
+ if not is_gt, this returns a dict which contains the fields:
+ [tracker_ids, tracker_classes, tracker_confidences] : list (for each timestep) of 1D NDArrays (for each det).
+ [tracker_dets]: list (for each timestep) of lists of detections.
+ """
+ # File location
+ if is_gt:
+ file = os.path.join(self.gt_fol, seq + '.json')
+ else:
+ file = os.path.join(self.tracker_fol, tracker, self.tracker_sub_fol, seq + '.json')
+
+ with open(file) as f:
+ data = json.load(f)
+
+ # sort data by frame index
+ data = sorted(data, key=lambda x: x['index'])
+
+ # check sequence length
+ if is_gt:
+ self.seq_lengths[seq] = len(data)
+ num_timesteps = len(data)
+ else:
+ num_timesteps = self.seq_lengths[seq]
+ if num_timesteps != len(data):
+ raise TrackEvalException('Number of ground truth and tracker timesteps do not match for sequence %s'
+ % seq)
+
+ # Convert data to required format
+ data_keys = ['ids', 'classes', 'dets']
+ if is_gt:
+ data_keys += ['gt_crowd_ignore_regions']
+ raw_data = {key: [None] * num_timesteps for key in data_keys}
+ for t in range(num_timesteps):
+ ig_ids = []
+ keep_ids = []
+ for i in range(len(data[t]['labels'])):
+ ann = data[t]['labels'][i]
+ if is_gt and (ann['category'] in self.distractor_classes or 'attributes' in ann.keys()
+ and ann['attributes']['Crowd']):
+ ig_ids.append(i)
+ else:
+ keep_ids.append(i)
+
+ if keep_ids:
+ raw_data['dets'][t] = np.atleast_2d([[data[t]['labels'][i]['box2d']['x1'],
+ data[t]['labels'][i]['box2d']['y1'],
+ data[t]['labels'][i]['box2d']['x2'],
+ data[t]['labels'][i]['box2d']['y2']
+ ] for i in keep_ids]).astype(float)
+ raw_data['ids'][t] = np.atleast_1d([data[t]['labels'][i]['id'] for i in keep_ids]).astype(int)
+ raw_data['classes'][t] = np.atleast_1d([self.class_name_to_class_id[data[t]['labels'][i]['category']]
+ for i in keep_ids]).astype(int)
+ else:
+ raw_data['dets'][t] = np.empty((0, 4)).astype(float)
+ raw_data['ids'][t] = np.empty(0).astype(int)
+ raw_data['classes'][t] = np.empty(0).astype(int)
+
+ if is_gt:
+ if ig_ids:
+ raw_data['gt_crowd_ignore_regions'][t] = np.atleast_2d([[data[t]['labels'][i]['box2d']['x1'],
+ data[t]['labels'][i]['box2d']['y1'],
+ data[t]['labels'][i]['box2d']['x2'],
+ data[t]['labels'][i]['box2d']['y2']
+ ] for i in ig_ids]).astype(float)
+ else:
+ raw_data['gt_crowd_ignore_regions'][t] = np.empty((0, 4)).astype(float)
+
+ if is_gt:
+ key_map = {'ids': 'gt_ids',
+ 'classes': 'gt_classes',
+ 'dets': 'gt_dets'}
+ else:
+ key_map = {'ids': 'tracker_ids',
+ 'classes': 'tracker_classes',
+ 'dets': 'tracker_dets'}
+ for k, v in key_map.items():
+ raw_data[v] = raw_data.pop(k)
+ raw_data['num_timesteps'] = num_timesteps
+ return raw_data
+
+ @_timing.time
+ def get_preprocessed_seq_data(self, raw_data, cls):
+ """ Preprocess data for a single sequence for a single class ready for evaluation.
+ Inputs:
+ - raw_data is a dict containing the data for the sequence already read in by get_raw_seq_data().
+ - cls is the class to be evaluated.
+ Outputs:
+ - data is a dict containing all of the information that metrics need to perform evaluation.
+ It contains the following fields:
+ [num_timesteps, num_gt_ids, num_tracker_ids, num_gt_dets, num_tracker_dets] : integers.
+ [gt_ids, tracker_ids, tracker_confidences]: list (for each timestep) of 1D NDArrays (for each det).
+ [gt_dets, tracker_dets]: list (for each timestep) of lists of detections.
+ [similarity_scores]: list (for each timestep) of 2D NDArrays.
+ Notes:
+ General preprocessing (preproc) occurs in 4 steps. Some datasets may not use all of these steps.
+ 1) Extract only detections relevant for the class to be evaluated (including distractor detections).
+ 2) Match gt dets and tracker dets. Remove tracker dets that are matched to a gt det that is of a
+ distractor class, or otherwise marked as to be removed.
+ 3) Remove unmatched tracker dets if they fall within a crowd ignore region or don't meet a certain
+ other criteria (e.g. are too small).
+ 4) Remove gt dets that were only useful for preprocessing and not for actual evaluation.
+ After the above preprocessing steps, this function also calculates the number of gt and tracker detections
+ and unique track ids. It also relabels gt and tracker ids to be contiguous and checks that ids are
+ unique within each timestep.
+
+ BDD100K:
+ In BDD100K, the 4 preproc steps are as follow:
+ 1) There are eight classes (pedestrian, rider, car, bus, truck, train, motorcycle, bicycle)
+ which are evaluated separately.
+ 2) For BDD100K there is no removal of matched tracker dets.
+ 3) Crowd ignore regions are used to remove unmatched detections.
+ 4) No removal of gt dets.
+ """
+ cls_id = self.class_name_to_class_id[cls]
+
+ data_keys = ['gt_ids', 'tracker_ids', 'gt_dets', 'tracker_dets', 'similarity_scores']
+ data = {key: [None] * raw_data['num_timesteps'] for key in data_keys}
+ unique_gt_ids = []
+ unique_tracker_ids = []
+ num_gt_dets = 0
+ num_tracker_dets = 0
+ for t in range(raw_data['num_timesteps']):
+
+ # Only extract relevant dets for this class for preproc and eval (cls)
+ gt_class_mask = np.atleast_1d(raw_data['gt_classes'][t] == cls_id)
+ gt_class_mask = gt_class_mask.astype(np.bool)
+ gt_ids = raw_data['gt_ids'][t][gt_class_mask]
+ gt_dets = raw_data['gt_dets'][t][gt_class_mask]
+
+ tracker_class_mask = np.atleast_1d(raw_data['tracker_classes'][t] == cls_id)
+ tracker_class_mask = tracker_class_mask.astype(np.bool)
+ tracker_ids = raw_data['tracker_ids'][t][tracker_class_mask]
+ tracker_dets = raw_data['tracker_dets'][t][tracker_class_mask]
+ similarity_scores = raw_data['similarity_scores'][t][gt_class_mask, :][:, tracker_class_mask]
+
+ # Match tracker and gt dets (with hungarian algorithm)
+ unmatched_indices = np.arange(tracker_ids.shape[0])
+ if gt_ids.shape[0] > 0 and tracker_ids.shape[0] > 0:
+ matching_scores = similarity_scores.copy()
+ matching_scores[matching_scores < 0.5 - np.finfo('float').eps] = 0
+ match_rows, match_cols = linear_sum_assignment(-matching_scores)
+ actually_matched_mask = matching_scores[match_rows, match_cols] > 0 + np.finfo('float').eps
+ match_cols = match_cols[actually_matched_mask]
+ unmatched_indices = np.delete(unmatched_indices, match_cols, axis=0)
+
+ # For unmatched tracker dets, remove those that are greater than 50% within a crowd ignore region.
+ unmatched_tracker_dets = tracker_dets[unmatched_indices, :]
+ crowd_ignore_regions = raw_data['gt_crowd_ignore_regions'][t]
+ intersection_with_ignore_region = self._calculate_box_ious(unmatched_tracker_dets, crowd_ignore_regions,
+ box_format='x0y0x1y1', do_ioa=True)
+ is_within_crowd_ignore_region = np.any(intersection_with_ignore_region > 0.5 + np.finfo('float').eps,
+ axis=1)
+
+ # Apply preprocessing to remove unwanted tracker dets.
+ to_remove_tracker = unmatched_indices[is_within_crowd_ignore_region]
+ data['tracker_ids'][t] = np.delete(tracker_ids, to_remove_tracker, axis=0)
+ data['tracker_dets'][t] = np.delete(tracker_dets, to_remove_tracker, axis=0)
+ similarity_scores = np.delete(similarity_scores, to_remove_tracker, axis=1)
+
+ data['gt_ids'][t] = gt_ids
+ data['gt_dets'][t] = gt_dets
+ data['similarity_scores'][t] = similarity_scores
+
+ unique_gt_ids += list(np.unique(data['gt_ids'][t]))
+ unique_tracker_ids += list(np.unique(data['tracker_ids'][t]))
+ num_tracker_dets += len(data['tracker_ids'][t])
+ num_gt_dets += len(data['gt_ids'][t])
+
+ # Re-label IDs such that there are no empty IDs
+ if len(unique_gt_ids) > 0:
+ unique_gt_ids = np.unique(unique_gt_ids)
+ gt_id_map = np.nan * np.ones((np.max(unique_gt_ids) + 1))
+ gt_id_map[unique_gt_ids] = np.arange(len(unique_gt_ids))
+ for t in range(raw_data['num_timesteps']):
+ if len(data['gt_ids'][t]) > 0:
+ data['gt_ids'][t] = gt_id_map[data['gt_ids'][t]].astype(np.int)
+ if len(unique_tracker_ids) > 0:
+ unique_tracker_ids = np.unique(unique_tracker_ids)
+ tracker_id_map = np.nan * np.ones((np.max(unique_tracker_ids) + 1))
+ tracker_id_map[unique_tracker_ids] = np.arange(len(unique_tracker_ids))
+ for t in range(raw_data['num_timesteps']):
+ if len(data['tracker_ids'][t]) > 0:
+ data['tracker_ids'][t] = tracker_id_map[data['tracker_ids'][t]].astype(np.int)
+
+ # Record overview statistics.
+ data['num_tracker_dets'] = num_tracker_dets
+ data['num_gt_dets'] = num_gt_dets
+ data['num_tracker_ids'] = len(unique_tracker_ids)
+ data['num_gt_ids'] = len(unique_gt_ids)
+ data['num_timesteps'] = raw_data['num_timesteps']
+
+ # Ensure that ids are unique per timestep.
+ self._check_unique_ids(data)
+
+ return data
+
+ def _calculate_similarities(self, gt_dets_t, tracker_dets_t):
+ similarity_scores = self._calculate_box_ious(gt_dets_t, tracker_dets_t, box_format='x0y0x1y1')
+ return similarity_scores
diff --git a/val_utils/trackeval/datasets/burst.py b/val_utils/trackeval/datasets/burst.py
new file mode 100644
index 0000000000000000000000000000000000000000..475c09e874f973a3cadbf6e5df0c5053cf3c48a2
--- /dev/null
+++ b/val_utils/trackeval/datasets/burst.py
@@ -0,0 +1,49 @@
+import os
+from .burst_helpers.burst_base import BURSTBase
+from .burst_helpers.format_converter import GroundTruthBURSTFormatToTAOFormatConverter, PredictionBURSTFormatToTAOFormatConverter
+from .. import utils
+
+
+class BURST(BURSTBase):
+ """Dataset class for TAO tracking"""
+
+ @staticmethod
+ def get_default_dataset_config():
+ tao_config = BURSTBase.get_default_dataset_config()
+ code_path = utils.get_code_path()
+
+ # e.g. 'data/gt/tsunami/exemplar_guided/'
+ tao_config['GT_FOLDER'] = os.path.join(
+ code_path, 'data/gt/burst/val/') # Location of GT data
+ # e.g. 'data/trackers/tsunami/exemplar_guided/mask_guided/validation/'
+ tao_config['TRACKERS_FOLDER'] = os.path.join(
+ code_path, 'data/trackers/burst/class-guided/') # Trackers location
+ # set to True or False
+ tao_config['EXEMPLAR_GUIDED'] = False
+ return tao_config
+
+ def _iou_type(self):
+ return 'mask'
+
+ def _box_or_mask_from_det(self, det):
+ return det['segmentation']
+
+ def _calculate_area_for_ann(self, ann):
+ import pycocotools.mask as cocomask
+ return cocomask.area(ann["segmentation"])
+
+ def _calculate_similarities(self, gt_dets_t, tracker_dets_t):
+ similarity_scores = self._calculate_mask_ious(gt_dets_t, tracker_dets_t, is_encoded=True, do_ioa=False)
+ return similarity_scores
+
+ def _is_exemplar_guided(self):
+ exemplar_guided = self.config['EXEMPLAR_GUIDED']
+ return exemplar_guided
+
+ def _postproc_ground_truth_data(self, data):
+ return GroundTruthBURSTFormatToTAOFormatConverter(data).convert()
+
+ def _postproc_prediction_data(self, data):
+ return PredictionBURSTFormatToTAOFormatConverter(
+ self.gt_data, data,
+ exemplar_guided=self._is_exemplar_guided()).convert()
diff --git a/val_utils/trackeval/datasets/burst_helpers/BURST_SPECIFIC_ISSUES.md b/val_utils/trackeval/datasets/burst_helpers/BURST_SPECIFIC_ISSUES.md
new file mode 100644
index 0000000000000000000000000000000000000000..184d53c26588dca01309227b90dd07a2af3da8b3
--- /dev/null
+++ b/val_utils/trackeval/datasets/burst_helpers/BURST_SPECIFIC_ISSUES.md
@@ -0,0 +1,7 @@
+The track ids in both ground truth and predictions are not globally unique, but
+start from 1 for each video. At the moment when converting from Ali format to
+TAO format, we remap the ids to be globally unique. It would be better to
+directly have this in the data though.
+
+
+Improve setting of EXEMPLAR_GUIDED flag, maybe this can be done automatically.
diff --git a/val_utils/trackeval/datasets/burst_helpers/__init__.py b/val_utils/trackeval/datasets/burst_helpers/__init__.py
new file mode 100644
index 0000000000000000000000000000000000000000..e69de29bb2d1d6434b8b29ae775ad8c2e48c5391
diff --git a/val_utils/trackeval/datasets/burst_helpers/burst_base.py b/val_utils/trackeval/datasets/burst_helpers/burst_base.py
new file mode 100644
index 0000000000000000000000000000000000000000..394eda4d5c4770c18e2ad66c4816fa1860be5eb5
--- /dev/null
+++ b/val_utils/trackeval/datasets/burst_helpers/burst_base.py
@@ -0,0 +1,591 @@
+import os
+import numpy as np
+import json
+import itertools
+from collections import defaultdict
+from scipy.optimize import linear_sum_assignment
+from trackeval.utils import TrackEvalException
+from trackeval.datasets._base_dataset import _BaseDataset
+from trackeval import utils
+from trackeval import _timing
+
+
+class BURSTBase(_BaseDataset):
+ """Dataset class for TAO tracking"""
+
+ def _postproc_ground_truth_data(self, data):
+ return data
+
+ def _postproc_prediction_data(self, data):
+ return data
+
+ def _iou_type(self):
+ return 'bbox'
+
+ def _box_or_mask_from_det(self, det):
+ return np.atleast_1d(det['bbox'])
+
+ def _calculate_area_for_ann(self, ann):
+ return ann["bbox"][2] * ann["bbox"][3]
+
+ @staticmethod
+ def get_default_dataset_config():
+ """Default class config values"""
+ code_path = utils.get_code_path()
+ default_config = {
+ 'GT_FOLDER': os.path.join(code_path, 'data/gt/tao/tao_training'), # Location of GT data
+ 'TRACKERS_FOLDER': os.path.join(code_path, 'data/trackers/tao/tao_training'), # Trackers location
+ 'OUTPUT_FOLDER': None, # Where to save eval results (if None, same as TRACKERS_FOLDER)
+ 'TRACKERS_TO_EVAL': None, # Filenames of trackers to eval (if None, all in folder)
+ 'CLASSES_TO_EVAL': None, # Classes to eval (if None, all classes)
+ 'SPLIT_TO_EVAL': 'training', # Valid: 'training', 'val'
+ 'PRINT_CONFIG': True, # Whether to print current config
+ 'TRACKER_SUB_FOLDER': 'data', # Tracker files are in TRACKER_FOLDER/tracker_name/TRACKER_SUB_FOLDER
+ 'OUTPUT_SUB_FOLDER': '', # Output files are saved in OUTPUT_FOLDER/tracker_name/OUTPUT_SUB_FOLDER
+ 'TRACKER_DISPLAY_NAMES': None, # Names of trackers to display, if None: TRACKERS_TO_EVAL
+ 'MAX_DETECTIONS': 300, # Number of maximal allowed detections per image (0 for unlimited)
+ 'EXEMPLAR_GUIDED': False,
+ }
+ return default_config
+
+ def __init__(self, config=None):
+ """Initialise dataset, checking that all required files are present"""
+ super().__init__()
+ # Fill non-given config values with defaults
+ self.config = utils.init_config(config, self.get_default_dataset_config(), self.get_name())
+ self.gt_fol = self.config['GT_FOLDER']
+ self.tracker_fol = self.config['TRACKERS_FOLDER']
+ self.should_classes_combine = True
+ self.use_super_categories = False
+
+ self.tracker_sub_fol = self.config['TRACKER_SUB_FOLDER']
+ self.output_fol = self.config['OUTPUT_FOLDER']
+ if self.output_fol is None:
+ self.output_fol = self.tracker_fol
+ self.output_sub_fol = self.config['OUTPUT_SUB_FOLDER']
+
+ gt_dir_files = [file for file in os.listdir(self.gt_fol) if file.endswith('.json')]
+ if len(gt_dir_files) != 1:
+ raise TrackEvalException(self.gt_fol + ' does not contain exactly one json file.')
+
+ with open(os.path.join(self.gt_fol, gt_dir_files[0])) as f:
+ self.gt_data = self._postproc_ground_truth_data(json.load(f))
+
+ # merge categories marked with a merged tag in TAO dataset
+ self._merge_categories(self.gt_data['annotations'] + self.gt_data['tracks'])
+
+ # Get sequences to eval and sequence information
+ self.seq_list = [vid['name'].replace('/', '-') for vid in self.gt_data['videos']]
+ self.seq_name_to_seq_id = {vid['name'].replace('/', '-'): vid['id'] for vid in self.gt_data['videos']}
+ # compute mappings from videos to annotation data
+ self.videos_to_gt_tracks, self.videos_to_gt_images = self._compute_vid_mappings(self.gt_data['annotations'])
+ # compute sequence lengths
+ self.seq_lengths = {vid['id']: 0 for vid in self.gt_data['videos']}
+ for img in self.gt_data['images']:
+ self.seq_lengths[img['video_id']] += 1
+ self.seq_to_images_to_timestep = self._compute_image_to_timestep_mappings()
+ self.seq_to_classes = {vid['id']: {'pos_cat_ids': list({track['category_id'] for track
+ in self.videos_to_gt_tracks[vid['id']]}),
+ 'neg_cat_ids': vid['neg_category_ids'],
+ 'not_exhaustively_labeled_cat_ids': vid['not_exhaustive_category_ids']}
+ for vid in self.gt_data['videos']}
+
+ # Get classes to eval
+ considered_vid_ids = [self.seq_name_to_seq_id[vid] for vid in self.seq_list]
+ seen_cats = set([cat_id for vid_id in considered_vid_ids for cat_id
+ in self.seq_to_classes[vid_id]['pos_cat_ids']])
+ # only classes with ground truth are evaluated in TAO, also we don't evaluate distactors.
+ distractors = {20, 63, 108, 180, 188, 204, 212, 247, 303, 403, 407, 415, 490, 504, 507, 513, 529, 567,
+ 569, 588, 672, 691, 702, 708, 711, 720, 736, 737, 798, 813, 815, 827, 831, 851, 877, 883,
+ 912, 971, 976, 1130, 1133, 1134, 1169, 1184, 1220}
+ self.valid_classes = [cls['name'] for cls in self.gt_data['categories'] if (cls['id'] in seen_cats) and (cls['id'] not in distractors)]
+ cls_name_to_cls_id_map = {cls['name']: cls['id'] for cls in self.gt_data['categories']}
+
+ if self.config['CLASSES_TO_EVAL']:
+ self.class_list = [cls.lower() if cls.lower() in self.valid_classes else None
+ for cls in self.config['CLASSES_TO_EVAL']]
+ if not all(self.class_list):
+ raise TrackEvalException('Attempted to evaluate an invalid class. Only classes ' +
+ ', '.join(self.valid_classes) +
+ ' are valid (classes present in ground truth data).')
+ else:
+ self.class_list = [cls for cls in self.valid_classes]
+ self.class_name_to_class_id = {k: v for k, v in cls_name_to_cls_id_map.items() if k in self.class_list}
+
+ # Get trackers to eval
+ if self.config['TRACKERS_TO_EVAL'] is None:
+ self.tracker_list = os.listdir(self.tracker_fol)
+ else:
+ self.tracker_list = self.config['TRACKERS_TO_EVAL']
+
+ if self.config['TRACKER_DISPLAY_NAMES'] is None:
+ self.tracker_to_disp = dict(zip(self.tracker_list, self.tracker_list))
+ elif (self.config['TRACKERS_TO_EVAL'] is not None) and (
+ len(self.config['TRACKER_DISPLAY_NAMES']) == len(self.tracker_list)):
+ self.tracker_to_disp = dict(zip(self.tracker_list, self.config['TRACKER_DISPLAY_NAMES']))
+ else:
+ raise TrackEvalException('List of tracker files and tracker display names do not match.')
+
+ self.tracker_data = {tracker: dict() for tracker in self.tracker_list}
+
+ for tracker in self.tracker_list:
+ tr_dir_files = [file for file in os.listdir(os.path.join(self.tracker_fol, tracker, self.tracker_sub_fol))
+ if file.endswith('.json')]
+ if len(tr_dir_files) != 1:
+ raise TrackEvalException(os.path.join(self.tracker_fol, tracker, self.tracker_sub_fol)
+ + ' does not contain exactly one json file.')
+ with open(os.path.join(self.tracker_fol, tracker, self.tracker_sub_fol, tr_dir_files[0])) as f:
+ curr_data = self._postproc_prediction_data(json.load(f))
+
+ # limit detections if MAX_DETECTIONS > 0
+ if self.config['MAX_DETECTIONS']:
+ curr_data = self._limit_dets_per_image(curr_data)
+
+ # fill missing video ids
+ self._fill_video_ids_inplace(curr_data)
+
+ # make track ids unique over whole evaluation set
+ self._make_track_ids_unique(curr_data)
+
+ # merge categories marked with a merged tag in TAO dataset
+ self._merge_categories(curr_data)
+
+ # get tracker sequence information
+ curr_videos_to_tracker_tracks, curr_videos_to_tracker_images = self._compute_vid_mappings(curr_data)
+ self.tracker_data[tracker]['vids_to_tracks'] = curr_videos_to_tracker_tracks
+ self.tracker_data[tracker]['vids_to_images'] = curr_videos_to_tracker_images
+
+ def get_display_name(self, tracker):
+ return self.tracker_to_disp[tracker]
+
+ def _load_raw_file(self, tracker, seq, is_gt):
+ """Load a file (gt or tracker) in the TAO format
+
+ If is_gt, this returns a dict which contains the fields:
+ [gt_ids, gt_classes] : list (for each timestep) of 1D NDArrays (for each det).
+ [gt_dets]: list (for each timestep) of lists of detections.
+ [classes_to_gt_tracks]: dictionary with class values as keys and list of dictionaries (with frame indices as
+ keys and corresponding segmentations as values) for each track
+ [classes_to_gt_track_ids, classes_to_gt_track_areas, classes_to_gt_track_lengths]: dictionary with class values
+ as keys and lists (for each track) as values
+
+ if not is_gt, this returns a dict which contains the fields:
+ [tracker_ids, tracker_classes, tracker_confidences] : list (for each timestep) of 1D NDArrays (for each det).
+ [tracker_dets]: list (for each timestep) of lists of detections.
+ [classes_to_dt_tracks]: dictionary with class values as keys and list of dictionaries (with frame indices as
+ keys and corresponding segmentations as values) for each track
+ [classes_to_dt_track_ids, classes_to_dt_track_areas, classes_to_dt_track_lengths]: dictionary with class values
+ as keys and lists as values
+ [classes_to_dt_track_scores]: dictionary with class values as keys and 1D numpy arrays as values
+ """
+ seq_id = self.seq_name_to_seq_id[seq]
+ # File location
+ if is_gt:
+ imgs = self.videos_to_gt_images[seq_id]
+ else:
+ imgs = self.tracker_data[tracker]['vids_to_images'][seq_id]
+
+ # Convert data to required format
+ num_timesteps = self.seq_lengths[seq_id]
+ img_to_timestep = self.seq_to_images_to_timestep[seq_id]
+ data_keys = ['ids', 'classes', 'dets']
+ if not is_gt:
+ data_keys += ['tracker_confidences']
+ raw_data = {key: [None] * num_timesteps for key in data_keys}
+ for img in imgs:
+ # some tracker data contains images without any ground truth information, these are ignored
+ try:
+ t = img_to_timestep[img['id']]
+ except KeyError:
+ continue
+ annotations = img['annotations']
+ raw_data['dets'][t] = np.atleast_2d([ann['bbox'] for ann in annotations]).astype(float)
+ raw_data['ids'][t] = np.atleast_1d([ann['track_id'] for ann in annotations]).astype(int)
+ raw_data['classes'][t] = np.atleast_1d([ann['category_id'] for ann in annotations]).astype(int)
+ if not is_gt:
+ raw_data['tracker_confidences'][t] = np.atleast_1d([ann['score'] for ann in annotations]).astype(float)
+
+ for t, d in enumerate(raw_data['dets']):
+ if d is None:
+ raw_data['dets'][t] = np.empty((0, 4)).astype(float)
+ raw_data['ids'][t] = np.empty(0).astype(int)
+ raw_data['classes'][t] = np.empty(0).astype(int)
+ if not is_gt:
+ raw_data['tracker_confidences'][t] = np.empty(0)
+
+ if is_gt:
+ key_map = {'ids': 'gt_ids',
+ 'classes': 'gt_classes',
+ 'dets': 'gt_dets'}
+ else:
+ key_map = {'ids': 'tracker_ids',
+ 'classes': 'tracker_classes',
+ 'dets': 'tracker_dets'}
+ for k, v in key_map.items():
+ raw_data[v] = raw_data.pop(k)
+
+ all_classes = [self.class_name_to_class_id[cls] for cls in self.class_list]
+ if is_gt:
+ classes_to_consider = all_classes
+ all_tracks = self.videos_to_gt_tracks[seq_id]
+ else:
+ classes_to_consider = self.seq_to_classes[seq_id]['pos_cat_ids'] \
+ + self.seq_to_classes[seq_id]['neg_cat_ids']
+ all_tracks = self.tracker_data[tracker]['vids_to_tracks'][seq_id]
+
+ classes_to_tracks = {cls: [track for track in all_tracks if track['category_id'] == cls]
+ if cls in classes_to_consider else [] for cls in all_classes}
+
+ # mapping from classes to track information
+ raw_data['classes_to_tracks'] = {cls: [{det['image_id']: self._box_or_mask_from_det(det)
+ for det in track['annotations']} for track in tracks]
+ for cls, tracks in classes_to_tracks.items()}
+ raw_data['classes_to_track_ids'] = {cls: [track['id'] for track in tracks]
+ for cls, tracks in classes_to_tracks.items()}
+ raw_data['classes_to_track_areas'] = {cls: [track['area'] for track in tracks]
+ for cls, tracks in classes_to_tracks.items()}
+ raw_data['classes_to_track_lengths'] = {cls: [len(track['annotations']) for track in tracks]
+ for cls, tracks in classes_to_tracks.items()}
+
+ if not is_gt:
+ raw_data['classes_to_dt_track_scores'] = {cls: np.array([np.mean([float(x['score'])
+ for x in track['annotations']])
+ for track in tracks])
+ for cls, tracks in classes_to_tracks.items()}
+
+ if is_gt:
+ key_map = {'classes_to_tracks': 'classes_to_gt_tracks',
+ 'classes_to_track_ids': 'classes_to_gt_track_ids',
+ 'classes_to_track_lengths': 'classes_to_gt_track_lengths',
+ 'classes_to_track_areas': 'classes_to_gt_track_areas'}
+ else:
+ key_map = {'classes_to_tracks': 'classes_to_dt_tracks',
+ 'classes_to_track_ids': 'classes_to_dt_track_ids',
+ 'classes_to_track_lengths': 'classes_to_dt_track_lengths',
+ 'classes_to_track_areas': 'classes_to_dt_track_areas'}
+ for k, v in key_map.items():
+ raw_data[v] = raw_data.pop(k)
+
+ raw_data['num_timesteps'] = num_timesteps
+ raw_data['neg_cat_ids'] = self.seq_to_classes[seq_id]['neg_cat_ids']
+ raw_data['not_exhaustively_labeled_cls'] = self.seq_to_classes[seq_id]['not_exhaustively_labeled_cat_ids']
+ raw_data['seq'] = seq
+ return raw_data
+
+ @_timing.time
+ def get_preprocessed_seq_data(self, raw_data, cls):
+ """ Preprocess data for a single sequence for a single class ready for evaluation.
+ Inputs:
+ - raw_data is a dict containing the data for the sequence already read in by get_raw_seq_data().
+ - cls is the class to be evaluated.
+ Outputs:
+ - data is a dict containing all of the information that metrics need to perform evaluation.
+ It contains the following fields:
+ [num_timesteps, num_gt_ids, num_tracker_ids, num_gt_dets, num_tracker_dets] : integers.
+ [gt_ids, tracker_ids, tracker_confidences]: list (for each timestep) of 1D NDArrays (for each det).
+ [gt_dets, tracker_dets]: list (for each timestep) of lists of detections.
+ [similarity_scores]: list (for each timestep) of 2D NDArrays.
+ Notes:
+ General preprocessing (preproc) occurs in 4 steps. Some datasets may not use all of these steps.
+ 1) Extract only detections relevant for the class to be evaluated (including distractor detections).
+ 2) Match gt dets and tracker dets. Remove tracker dets that are matched to a gt det that is of a
+ distractor class, or otherwise marked as to be removed.
+ 3) Remove unmatched tracker dets if they fall within a crowd ignore region or don't meet a certain
+ other criteria (e.g. are too small).
+ 4) Remove gt dets that were only useful for preprocessing and not for actual evaluation.
+ After the above preprocessing steps, this function also calculates the number of gt and tracker detections
+ and unique track ids. It also relabels gt and tracker ids to be contiguous and checks that ids are
+ unique within each timestep.
+ TAO:
+ In TAO, the 4 preproc steps are as follow:
+ 1) All classes present in the ground truth data are evaluated separately.
+ 2) No matched tracker detections are removed.
+ 3) Unmatched tracker detections are removed if there is not ground truth data and the class does not
+ belong to the categories marked as negative for this sequence. Additionally, unmatched tracker
+ detections for classes which are marked as not exhaustively labeled are removed.
+ 4) No gt detections are removed.
+ Further, for TrackMAP computation track representations for the given class are accessed from a dictionary
+ and the tracks from the tracker data are sorted according to the tracker confidence.
+ """
+ cls_id = self.class_name_to_class_id[cls]
+ is_not_exhaustively_labeled = cls_id in raw_data['not_exhaustively_labeled_cls']
+ is_neg_category = cls_id in raw_data['neg_cat_ids']
+
+ data_keys = ['gt_ids', 'tracker_ids', 'gt_dets', 'tracker_dets', 'tracker_confidences', 'similarity_scores']
+ data = {key: [None] * raw_data['num_timesteps'] for key in data_keys}
+ unique_gt_ids = []
+ unique_tracker_ids = []
+ num_gt_dets = 0
+ num_tracker_dets = 0
+
+ for t in range(raw_data['num_timesteps']):
+
+ # Only extract relevant dets for this class for preproc and eval (cls)
+ gt_class_mask = np.atleast_1d(raw_data['gt_classes'][t] == cls_id)
+ gt_class_mask = gt_class_mask.astype(np.bool)
+ gt_ids = raw_data['gt_ids'][t][gt_class_mask]
+ gt_dets = raw_data['gt_dets'][t][gt_class_mask]
+
+ tracker_class_mask = np.atleast_1d(raw_data['tracker_classes'][t] == cls_id)
+ tracker_class_mask = tracker_class_mask.astype(np.bool)
+ tracker_ids = raw_data['tracker_ids'][t][tracker_class_mask]
+ tracker_dets = raw_data['tracker_dets'][t][tracker_class_mask]
+ tracker_confidences = raw_data['tracker_confidences'][t][tracker_class_mask]
+ similarity_scores = raw_data['similarity_scores'][t][gt_class_mask, :][:, tracker_class_mask]
+
+ if not self.config['EXEMPLAR_GUIDED']:
+ # Match tracker and gt dets (with hungarian algorithm).
+ unmatched_indices = np.arange(tracker_ids.shape[0])
+ if gt_ids.shape[0] > 0 and tracker_ids.shape[0] > 0:
+ matching_scores = similarity_scores.copy()
+ matching_scores[matching_scores < 0.5 - np.finfo('float').eps] = 0
+ match_rows, match_cols = linear_sum_assignment(-matching_scores)
+ actually_matched_mask = matching_scores[match_rows, match_cols] > 0 + np.finfo('float').eps
+ match_cols = match_cols[actually_matched_mask]
+ unmatched_indices = np.delete(unmatched_indices, match_cols, axis=0)
+
+ if gt_ids.shape[0] == 0 and not is_neg_category:
+ to_remove_tracker = unmatched_indices
+ elif is_not_exhaustively_labeled:
+ to_remove_tracker = unmatched_indices
+ else:
+ to_remove_tracker = np.array([], dtype=np.int)
+
+ # remove all unwanted unmatched tracker detections
+ data['tracker_ids'][t] = np.delete(tracker_ids, to_remove_tracker, axis=0)
+ data['tracker_dets'][t] = np.delete(tracker_dets, to_remove_tracker, axis=0)
+ data['tracker_confidences'][t] = np.delete(tracker_confidences, to_remove_tracker, axis=0)
+ similarity_scores = np.delete(similarity_scores, to_remove_tracker, axis=1)
+ else:
+ data['tracker_ids'][t] = tracker_ids
+ data['tracker_dets'][t] = tracker_dets
+ data['tracker_confidences'][t] = tracker_confidences
+
+ data['gt_ids'][t] = gt_ids
+ data['gt_dets'][t] = gt_dets
+ data['similarity_scores'][t] = similarity_scores
+
+ unique_gt_ids += list(np.unique(data['gt_ids'][t]))
+ unique_tracker_ids += list(np.unique(data['tracker_ids'][t]))
+ num_tracker_dets += len(data['tracker_ids'][t])
+ num_gt_dets += len(data['gt_ids'][t])
+
+ # Re-label IDs such that there are no empty IDs
+ if len(unique_gt_ids) > 0:
+ unique_gt_ids = np.unique(unique_gt_ids)
+ gt_id_map = np.nan * np.ones((np.max(unique_gt_ids) + 1))
+ gt_id_map[unique_gt_ids] = np.arange(len(unique_gt_ids))
+ for t in range(raw_data['num_timesteps']):
+ if len(data['gt_ids'][t]) > 0:
+ data['gt_ids'][t] = gt_id_map[data['gt_ids'][t]].astype(np.int)
+ if len(unique_tracker_ids) > 0:
+ unique_tracker_ids = np.unique(unique_tracker_ids)
+ tracker_id_map = np.nan * np.ones((np.max(unique_tracker_ids) + 1))
+ tracker_id_map[unique_tracker_ids] = np.arange(len(unique_tracker_ids))
+ for t in range(raw_data['num_timesteps']):
+ if len(data['tracker_ids'][t]) > 0:
+ data['tracker_ids'][t] = tracker_id_map[data['tracker_ids'][t]].astype(np.int)
+
+ # Record overview statistics.
+ data['num_tracker_dets'] = num_tracker_dets
+ data['num_gt_dets'] = num_gt_dets
+ data['num_tracker_ids'] = len(unique_tracker_ids)
+ data['num_gt_ids'] = len(unique_gt_ids)
+ data['num_timesteps'] = raw_data['num_timesteps']
+ data['seq'] = raw_data['seq']
+
+ # get track representations
+ data['gt_tracks'] = raw_data['classes_to_gt_tracks'][cls_id]
+ data['gt_track_ids'] = raw_data['classes_to_gt_track_ids'][cls_id]
+ data['gt_track_lengths'] = raw_data['classes_to_gt_track_lengths'][cls_id]
+ data['gt_track_areas'] = raw_data['classes_to_gt_track_areas'][cls_id]
+ data['dt_tracks'] = raw_data['classes_to_dt_tracks'][cls_id]
+ data['dt_track_ids'] = raw_data['classes_to_dt_track_ids'][cls_id]
+ data['dt_track_lengths'] = raw_data['classes_to_dt_track_lengths'][cls_id]
+ data['dt_track_areas'] = raw_data['classes_to_dt_track_areas'][cls_id]
+ data['dt_track_scores'] = raw_data['classes_to_dt_track_scores'][cls_id]
+ data['not_exhaustively_labeled'] = is_not_exhaustively_labeled
+ data['iou_type'] = self._iou_type()
+
+ # sort tracker data tracks by tracker confidence scores
+ if data['dt_tracks']:
+ idx = np.argsort([-score for score in data['dt_track_scores']], kind="mergesort")
+ data['dt_track_scores'] = [data['dt_track_scores'][i] for i in idx]
+ data['dt_tracks'] = [data['dt_tracks'][i] for i in idx]
+ data['dt_track_ids'] = [data['dt_track_ids'][i] for i in idx]
+ data['dt_track_lengths'] = [data['dt_track_lengths'][i] for i in idx]
+ data['dt_track_areas'] = [data['dt_track_areas'][i] for i in idx]
+ # Ensure that ids are unique per timestep.
+ self._check_unique_ids(data)
+
+ return data
+
+ def _calculate_similarities(self, gt_dets_t, tracker_dets_t):
+ similarity_scores = self._calculate_box_ious(gt_dets_t, tracker_dets_t)
+ return similarity_scores
+
+ def _merge_categories(self, annotations):
+ """
+ Merges categories with a merged tag. Adapted from https://github.com/TAO-Dataset
+ :param annotations: the annotations in which the classes should be merged
+ :return: None
+ """
+ merge_map = {}
+ for category in self.gt_data['categories']:
+ if 'merged' in category:
+ for to_merge in category['merged']:
+ merge_map[to_merge['id']] = category['id']
+
+ for ann in annotations:
+ ann['category_id'] = merge_map.get(ann['category_id'], ann['category_id'])
+
+ def _compute_vid_mappings(self, annotations):
+ """
+ Computes mappings from Videos to corresponding tracks and images.
+ :param annotations: the annotations for which the mapping should be generated
+ :return: the video-to-track-mapping, the video-to-image-mapping
+ """
+ vids_to_tracks = {}
+ vids_to_imgs = {}
+ vid_ids = [vid['id'] for vid in self.gt_data['videos']]
+
+ # compute an mapping from image IDs to images
+ images = {}
+ for image in self.gt_data['images']:
+ images[image['id']] = image
+
+ for ann in annotations:
+ ann["area"] = self._calculate_area_for_ann(ann)
+
+ vid = ann["video_id"]
+ if ann["video_id"] not in vids_to_tracks.keys():
+ vids_to_tracks[ann["video_id"]] = list()
+ if ann["video_id"] not in vids_to_imgs.keys():
+ vids_to_imgs[ann["video_id"]] = list()
+
+ # Fill in vids_to_tracks
+ tid = ann["track_id"]
+ exist_tids = [track["id"] for track in vids_to_tracks[vid]]
+ try:
+ index1 = exist_tids.index(tid)
+ except ValueError:
+ index1 = -1
+ if tid not in exist_tids:
+ curr_track = {"id": tid, "category_id": ann["category_id"],
+ "video_id": vid, "annotations": [ann]}
+ vids_to_tracks[vid].append(curr_track)
+ else:
+ vids_to_tracks[vid][index1]["annotations"].append(ann)
+
+ # Fill in vids_to_imgs
+ img_id = ann['image_id']
+ exist_img_ids = [img["id"] for img in vids_to_imgs[vid]]
+ try:
+ index2 = exist_img_ids.index(img_id)
+ except ValueError:
+ index2 = -1
+ if index2 == -1:
+ curr_img = {"id": img_id, "annotations": [ann]}
+ vids_to_imgs[vid].append(curr_img)
+ else:
+ vids_to_imgs[vid][index2]["annotations"].append(ann)
+
+ # sort annotations by frame index and compute track area
+ for vid, tracks in vids_to_tracks.items():
+ for track in tracks:
+ track["annotations"] = sorted(
+ track['annotations'],
+ key=lambda x: images[x['image_id']]['frame_index'])
+ # Computer average area
+ track["area"] = (sum(x['area'] for x in track['annotations']) / len(track['annotations']))
+
+ # Ensure all videos are present
+ for vid_id in vid_ids:
+ if vid_id not in vids_to_tracks.keys():
+ vids_to_tracks[vid_id] = []
+ if vid_id not in vids_to_imgs.keys():
+ vids_to_imgs[vid_id] = []
+
+ return vids_to_tracks, vids_to_imgs
+
+ def _compute_image_to_timestep_mappings(self):
+ """
+ Computes a mapping from images to the corresponding timestep in the sequence.
+ :return: the image-to-timestep-mapping
+ """
+ images = {}
+ for image in self.gt_data['images']:
+ images[image['id']] = image
+
+ seq_to_imgs_to_timestep = {vid['id']: dict() for vid in self.gt_data['videos']}
+ for vid in seq_to_imgs_to_timestep:
+ curr_imgs = [img['id'] for img in self.videos_to_gt_images[vid]]
+ curr_imgs = sorted(curr_imgs, key=lambda x: images[x]['frame_index'])
+ seq_to_imgs_to_timestep[vid] = {curr_imgs[i]: i for i in range(len(curr_imgs))}
+
+ return seq_to_imgs_to_timestep
+
+ def _limit_dets_per_image(self, annotations):
+ """
+ Limits the number of detections for each image to config['MAX_DETECTIONS']. Adapted from
+ https://github.com/TAO-Dataset/
+ :param annotations: the annotations in which the detections should be limited
+ :return: the annotations with limited detections
+ """
+ max_dets = self.config['MAX_DETECTIONS']
+ img_ann = defaultdict(list)
+ for ann in annotations:
+ img_ann[ann["image_id"]].append(ann)
+
+ for img_id, _anns in img_ann.items():
+ if len(_anns) <= max_dets:
+ continue
+ _anns = sorted(_anns, key=lambda x: x["score"], reverse=True)
+ img_ann[img_id] = _anns[:max_dets]
+
+ return [ann for anns in img_ann.values() for ann in anns]
+
+ def _fill_video_ids_inplace(self, annotations):
+ """
+ Fills in missing video IDs inplace. Adapted from https://github.com/TAO-Dataset/
+ :param annotations: the annotations for which the videos IDs should be filled inplace
+ :return: None
+ """
+ missing_video_id = [x for x in annotations if 'video_id' not in x]
+ if missing_video_id:
+ image_id_to_video_id = {
+ x['id']: x['video_id'] for x in self.gt_data['images']
+ }
+ for x in missing_video_id:
+ x['video_id'] = image_id_to_video_id[x['image_id']]
+
+ @staticmethod
+ def _make_track_ids_unique(annotations):
+ """
+ Makes the track IDs unqiue over the whole annotation set. Adapted from https://github.com/TAO-Dataset/
+ :param annotations: the annotation set
+ :return: the number of updated IDs
+ """
+ track_id_videos = {}
+ track_ids_to_update = set()
+ max_track_id = 0
+ for ann in annotations:
+ t = ann['track_id']
+ if t not in track_id_videos:
+ track_id_videos[t] = ann['video_id']
+
+ if ann['video_id'] != track_id_videos[t]:
+ # Track id is assigned to multiple videos
+ track_ids_to_update.add(t)
+ max_track_id = max(max_track_id, t)
+
+ if track_ids_to_update:
+ #print('true')
+ next_id = itertools.count(max_track_id + 1)
+ new_track_ids = defaultdict(lambda: next(next_id))
+ for ann in annotations:
+ t = ann['track_id']
+ v = ann['video_id']
+ if t in track_ids_to_update:
+ ann['track_id'] = new_track_ids[t, v]
+ return len(track_ids_to_update)
diff --git a/val_utils/trackeval/datasets/burst_helpers/burst_ow_base.py b/val_utils/trackeval/datasets/burst_helpers/burst_ow_base.py
new file mode 100644
index 0000000000000000000000000000000000000000..bef14d2bf6362209d01075ad6976838ef121be2e
--- /dev/null
+++ b/val_utils/trackeval/datasets/burst_helpers/burst_ow_base.py
@@ -0,0 +1,675 @@
+import os
+import numpy as np
+import json
+import itertools
+from collections import defaultdict
+from scipy.optimize import linear_sum_assignment
+from trackeval.utils import TrackEvalException
+from trackeval.datasets._base_dataset import _BaseDataset
+from trackeval import utils
+from trackeval import _timing
+
+
+class BURST_OW_Base(_BaseDataset):
+ """Dataset class for TAO tracking"""
+
+ def _postproc_ground_truth_data(self, data):
+ return data
+
+ def _postproc_prediction_data(self, data):
+ return data
+
+ def _iou_type(self):
+ return 'bbox'
+
+ def _box_or_mask_from_det(self, det):
+ return np.atleast_1d(det['bbox'])
+
+ def _calculate_area_for_ann(self, ann):
+ return ann["bbox"][2] * ann["bbox"][3]
+
+ @staticmethod
+ def get_default_dataset_config():
+ """Default class config values"""
+ code_path = utils.get_code_path()
+ default_config = {
+ 'GT_FOLDER': os.path.join(code_path, 'data/gt/tao/tao_training'), # Location of GT data
+ 'TRACKERS_FOLDER': os.path.join(code_path, 'data/trackers/tao/tao_training'), # Trackers location
+ 'OUTPUT_FOLDER': None, # Where to save eval results (if None, same as TRACKERS_FOLDER)
+ 'TRACKERS_TO_EVAL': None, # Filenames of trackers to eval (if None, all in folder)
+ 'CLASSES_TO_EVAL': None, # Classes to eval (if None, all classes)
+ 'SPLIT_TO_EVAL': 'training', # Valid: 'training', 'val'
+ 'PRINT_CONFIG': True, # Whether to print current config
+ 'TRACKER_SUB_FOLDER': 'data', # Tracker files are in TRACKER_FOLDER/tracker_name/TRACKER_SUB_FOLDER
+ 'OUTPUT_SUB_FOLDER': '', # Output files are saved in OUTPUT_FOLDER/tracker_name/OUTPUT_SUB_FOLDER
+ 'TRACKER_DISPLAY_NAMES': None, # Names of trackers to display, if None: TRACKERS_TO_EVAL
+ 'MAX_DETECTIONS': 300, # Number of maximal allowed detections per image (0 for unlimited)
+ 'SUBSET': 'all'
+ }
+ return default_config
+
+ def __init__(self, config=None):
+ """Initialise dataset, checking that all required files are present"""
+ super().__init__()
+ # Fill non-given config values with defaults
+ self.config = utils.init_config(config, self.get_default_dataset_config(), self.get_name())
+ self.gt_fol = self.config['GT_FOLDER']
+ self.tracker_fol = self.config['TRACKERS_FOLDER']
+ self.should_classes_combine = True
+ self.use_super_categories = False
+
+ self.tracker_sub_fol = self.config['TRACKER_SUB_FOLDER']
+ self.output_fol = self.config['OUTPUT_FOLDER']
+ if self.output_fol is None:
+ self.output_fol = self.tracker_fol
+ self.output_sub_fol = self.config['OUTPUT_SUB_FOLDER']
+
+ gt_dir_files = [file for file in os.listdir(self.gt_fol) if file.endswith('.json')]
+ if len(gt_dir_files) != 1:
+ raise TrackEvalException(self.gt_fol + ' does not contain exactly one json file.')
+
+ with open(os.path.join(self.gt_fol, gt_dir_files[0])) as f:
+ self.gt_data = self._postproc_ground_truth_data(json.load(f))
+
+ self.subset = self.config['SUBSET']
+ if self.subset != 'all':
+ # Split GT data into `known`, `unknown` or `distractor`
+ self._split_known_unknown_distractor()
+ self.gt_data = self._filter_gt_data(self.gt_data)
+
+ # merge categories marked with a merged tag in TAO dataset
+ self._merge_categories(self.gt_data['annotations'] + self.gt_data['tracks'])
+
+ # Get sequences to eval and sequence information
+ self.seq_list = [vid['name'].replace('/', '-') for vid in self.gt_data['videos']]
+ self.seq_name_to_seq_id = {vid['name'].replace('/', '-'): vid['id'] for vid in self.gt_data['videos']}
+ # compute mappings from videos to annotation data
+ self.videos_to_gt_tracks, self.videos_to_gt_images = self._compute_vid_mappings(self.gt_data['annotations'])
+ # compute sequence lengths
+ self.seq_lengths = {vid['id']: 0 for vid in self.gt_data['videos']}
+ for img in self.gt_data['images']:
+ self.seq_lengths[img['video_id']] += 1
+ self.seq_to_images_to_timestep = self._compute_image_to_timestep_mappings()
+ self.seq_to_classes = {vid['id']: {'pos_cat_ids': list({track['category_id'] for track
+ in self.videos_to_gt_tracks[vid['id']]}),
+ 'neg_cat_ids': vid['neg_category_ids'],
+ 'not_exhaustively_labeled_cat_ids': vid['not_exhaustive_category_ids']}
+ for vid in self.gt_data['videos']}
+
+ # Get classes to eval
+ considered_vid_ids = [self.seq_name_to_seq_id[vid] for vid in self.seq_list]
+ seen_cats = set([cat_id for vid_id in considered_vid_ids for cat_id
+ in self.seq_to_classes[vid_id]['pos_cat_ids']])
+ # only classes with ground truth are evaluated in TAO
+ self.valid_classes = [cls['name'] for cls in self.gt_data['categories'] if cls['id'] in seen_cats]
+ # cls_name_to_cls_id_map = {cls['name']: cls['id'] for cls in self.gt_data['categories']}
+
+ if self.config['CLASSES_TO_EVAL']:
+ # self.class_list = [cls.lower() if cls.lower() in self.valid_classes else None
+ # for cls in self.config['CLASSES_TO_EVAL']]
+ self.class_list = ["object"] # class-agnostic
+ if not all(self.class_list):
+ raise TrackEvalException('Attempted to evaluate an invalid class. Only classes ' +
+ ', '.join(self.valid_classes) +
+ ' are valid (classes present in ground truth data).')
+ else:
+ # self.class_list = [cls for cls in self.valid_classes]
+ self.class_list = ["object"] # class-agnostic
+ # self.class_name_to_class_id = {k: v for k, v in cls_name_to_cls_id_map.items() if k in self.class_list}
+ self.class_name_to_class_id = {"object": 1} # class-agnostic
+
+ # Get trackers to eval
+ if self.config['TRACKERS_TO_EVAL'] is None:
+ self.tracker_list = os.listdir(self.tracker_fol)
+ else:
+ self.tracker_list = self.config['TRACKERS_TO_EVAL']
+
+ if self.config['TRACKER_DISPLAY_NAMES'] is None:
+ self.tracker_to_disp = dict(zip(self.tracker_list, self.tracker_list))
+ elif (self.config['TRACKERS_TO_EVAL'] is not None) and (
+ len(self.config['TRACKER_DISPLAY_NAMES']) == len(self.tracker_list)):
+ self.tracker_to_disp = dict(zip(self.tracker_list, self.config['TRACKER_DISPLAY_NAMES']))
+ else:
+ raise TrackEvalException('List of tracker files and tracker display names do not match.')
+
+ self.tracker_data = {tracker: dict() for tracker in self.tracker_list}
+
+ for tracker in self.tracker_list:
+ tr_dir_files = [file for file in os.listdir(os.path.join(self.tracker_fol, tracker, self.tracker_sub_fol))
+ if file.endswith('.json')]
+ if len(tr_dir_files) != 1:
+ raise TrackEvalException(os.path.join(self.tracker_fol, tracker, self.tracker_sub_fol)
+ + ' does not contain exactly one json file.')
+ with open(os.path.join(self.tracker_fol, tracker, self.tracker_sub_fol, tr_dir_files[0])) as f:
+ curr_data = self._postproc_prediction_data(json.load(f))
+
+ # limit detections if MAX_DETECTIONS > 0
+ if self.config['MAX_DETECTIONS']:
+ curr_data = self._limit_dets_per_image(curr_data)
+
+ # fill missing video ids
+ self._fill_video_ids_inplace(curr_data)
+
+ # make track ids unique over whole evaluation set
+ self._make_track_ids_unique(curr_data)
+
+ # merge categories marked with a merged tag in TAO dataset
+ self._merge_categories(curr_data)
+
+ # get tracker sequence information
+ curr_videos_to_tracker_tracks, curr_videos_to_tracker_images = self._compute_vid_mappings(curr_data)
+ self.tracker_data[tracker]['vids_to_tracks'] = curr_videos_to_tracker_tracks
+ self.tracker_data[tracker]['vids_to_images'] = curr_videos_to_tracker_images
+
+ def get_display_name(self, tracker):
+ return self.tracker_to_disp[tracker]
+
+ def _load_raw_file(self, tracker, seq, is_gt):
+ """Load a file (gt or tracker) in the TAO format
+
+ If is_gt, this returns a dict which contains the fields:
+ [gt_ids, gt_classes] : list (for each timestep) of 1D NDArrays (for each det).
+ [gt_dets]: list (for each timestep) of lists of detections.
+ [classes_to_gt_tracks]: dictionary with class values as keys and list of dictionaries (with frame indices as
+ keys and corresponding segmentations as values) for each track
+ [classes_to_gt_track_ids, classes_to_gt_track_areas, classes_to_gt_track_lengths]: dictionary with class values
+ as keys and lists (for each track) as values
+
+ if not is_gt, this returns a dict which contains the fields:
+ [tracker_ids, tracker_classes, tracker_confidences] : list (for each timestep) of 1D NDArrays (for each det).
+ [tracker_dets]: list (for each timestep) of lists of detections.
+ [classes_to_dt_tracks]: dictionary with class values as keys and list of dictionaries (with frame indices as
+ keys and corresponding segmentations as values) for each track
+ [classes_to_dt_track_ids, classes_to_dt_track_areas, classes_to_dt_track_lengths]: dictionary with class values
+ as keys and lists as values
+ [classes_to_dt_track_scores]: dictionary with class values as keys and 1D numpy arrays as values
+ """
+ seq_id = self.seq_name_to_seq_id[seq]
+ # File location
+ if is_gt:
+ imgs = self.videos_to_gt_images[seq_id]
+ else:
+ imgs = self.tracker_data[tracker]['vids_to_images'][seq_id]
+
+ # Convert data to required format
+ num_timesteps = self.seq_lengths[seq_id]
+ img_to_timestep = self.seq_to_images_to_timestep[seq_id]
+ data_keys = ['ids', 'classes', 'dets']
+ if not is_gt:
+ data_keys += ['tracker_confidences']
+ raw_data = {key: [None] * num_timesteps for key in data_keys}
+ for img in imgs:
+ # some tracker data contains images without any ground truth information, these are ignored
+ try:
+ t = img_to_timestep[img['id']]
+ except KeyError:
+ continue
+ annotations = img['annotations']
+ raw_data['dets'][t] = np.atleast_2d([ann['bbox'] for ann in annotations]).astype(float)
+ raw_data['ids'][t] = np.atleast_1d([ann['track_id'] for ann in annotations]).astype(int)
+ raw_data['classes'][t] = np.atleast_1d([1 for _ in annotations]).astype(int) # class-agnostic
+ if not is_gt:
+ raw_data['tracker_confidences'][t] = np.atleast_1d([ann['score'] for ann in annotations]).astype(float)
+
+ for t, d in enumerate(raw_data['dets']):
+ if d is None:
+ raw_data['dets'][t] = np.empty((0, 4)).astype(float)
+ raw_data['ids'][t] = np.empty(0).astype(int)
+ raw_data['classes'][t] = np.empty(0).astype(int)
+ if not is_gt:
+ raw_data['tracker_confidences'][t] = np.empty(0)
+
+ if is_gt:
+ key_map = {'ids': 'gt_ids',
+ 'classes': 'gt_classes',
+ 'dets': 'gt_dets'}
+ else:
+ key_map = {'ids': 'tracker_ids',
+ 'classes': 'tracker_classes',
+ 'dets': 'tracker_dets'}
+ for k, v in key_map.items():
+ raw_data[v] = raw_data.pop(k)
+
+ # all_classes = [self.class_name_to_class_id[cls] for cls in self.class_list]
+ all_classes = [1] # class-agnostic
+
+ if is_gt:
+ classes_to_consider = all_classes
+ all_tracks = self.videos_to_gt_tracks[seq_id]
+ else:
+ # classes_to_consider = self.seq_to_classes[seq_id]['pos_cat_ids'] \
+ # + self.seq_to_classes[seq_id]['neg_cat_ids']
+ classes_to_consider = all_classes # class-agnostic
+ all_tracks = self.tracker_data[tracker]['vids_to_tracks'][seq_id]
+
+ # classes_to_tracks = {cls: [track for track in all_tracks if track['category_id'] == cls]
+ # if cls in classes_to_consider else [] for cls in all_classes}
+ classes_to_tracks = {cls: [track for track in all_tracks]
+ if cls in classes_to_consider else [] for cls in all_classes} # class-agnostic
+
+ # mapping from classes to track information
+ raw_data['classes_to_tracks'] = {cls: [{det['image_id']: self._box_or_mask_from_det(det)
+ for det in track['annotations']} for track in tracks]
+ for cls, tracks in classes_to_tracks.items()}
+ raw_data['classes_to_track_ids'] = {cls: [track['id'] for track in tracks]
+ for cls, tracks in classes_to_tracks.items()}
+ raw_data['classes_to_track_areas'] = {cls: [track['area'] for track in tracks]
+ for cls, tracks in classes_to_tracks.items()}
+ raw_data['classes_to_track_lengths'] = {cls: [len(track['annotations']) for track in tracks]
+ for cls, tracks in classes_to_tracks.items()}
+
+ if not is_gt:
+ raw_data['classes_to_dt_track_scores'] = {cls: np.array([np.mean([float(x['score'])
+ for x in track['annotations']])
+ for track in tracks])
+ for cls, tracks in classes_to_tracks.items()}
+
+ if is_gt:
+ key_map = {'classes_to_tracks': 'classes_to_gt_tracks',
+ 'classes_to_track_ids': 'classes_to_gt_track_ids',
+ 'classes_to_track_lengths': 'classes_to_gt_track_lengths',
+ 'classes_to_track_areas': 'classes_to_gt_track_areas'}
+ else:
+ key_map = {'classes_to_tracks': 'classes_to_dt_tracks',
+ 'classes_to_track_ids': 'classes_to_dt_track_ids',
+ 'classes_to_track_lengths': 'classes_to_dt_track_lengths',
+ 'classes_to_track_areas': 'classes_to_dt_track_areas'}
+ for k, v in key_map.items():
+ raw_data[v] = raw_data.pop(k)
+
+ raw_data['num_timesteps'] = num_timesteps
+ raw_data['neg_cat_ids'] = self.seq_to_classes[seq_id]['neg_cat_ids']
+ raw_data['not_exhaustively_labeled_cls'] = self.seq_to_classes[seq_id]['not_exhaustively_labeled_cat_ids']
+ raw_data['seq'] = seq
+ return raw_data
+
+ @_timing.time
+ def get_preprocessed_seq_data(self, raw_data, cls):
+ """ Preprocess data for a single sequence for a single class ready for evaluation.
+ Inputs:
+ - raw_data is a dict containing the data for the sequence already read in by get_raw_seq_data().
+ - cls is the class to be evaluated.
+ Outputs:
+ - data is a dict containing all of the information that metrics need to perform evaluation.
+ It contains the following fields:
+ [num_timesteps, num_gt_ids, num_tracker_ids, num_gt_dets, num_tracker_dets] : integers.
+ [gt_ids, tracker_ids, tracker_confidences]: list (for each timestep) of 1D NDArrays (for each det).
+ [gt_dets, tracker_dets]: list (for each timestep) of lists of detections.
+ [similarity_scores]: list (for each timestep) of 2D NDArrays.
+ Notes:
+ General preprocessing (preproc) occurs in 4 steps. Some datasets may not use all of these steps.
+ 1) Extract only detections relevant for the class to be evaluated (including distractor detections).
+ 2) Match gt dets and tracker dets. Remove tracker dets that are matched to a gt det that is of a
+ distractor class, or otherwise marked as to be removed.
+ 3) Remove unmatched tracker dets if they fall within a crowd ignore region or don't meet a certain
+ other criteria (e.g. are too small).
+ 4) Remove gt dets that were only useful for preprocessing and not for actual evaluation.
+ After the above preprocessing steps, this function also calculates the number of gt and tracker detections
+ and unique track ids. It also relabels gt and tracker ids to be contiguous and checks that ids are
+ unique within each timestep.
+ TAO:
+ In TAO, the 4 preproc steps are as follow:
+ 1) All classes present in the ground truth data are evaluated separately.
+ 2) No matched tracker detections are removed.
+ 3) Unmatched tracker detections are removed if there is not ground truth data and the class does not
+ belong to the categories marked as negative for this sequence. Additionally, unmatched tracker
+ detections for classes which are marked as not exhaustively labeled are removed.
+ 4) No gt detections are removed.
+ Further, for TrackMAP computation track representations for the given class are accessed from a dictionary
+ and the tracks from the tracker data are sorted according to the tracker confidence.
+ """
+ cls_id = self.class_name_to_class_id[cls]
+ is_not_exhaustively_labeled = cls_id in raw_data['not_exhaustively_labeled_cls']
+ is_neg_category = cls_id in raw_data['neg_cat_ids']
+
+ data_keys = ['gt_ids', 'tracker_ids', 'gt_dets', 'tracker_dets', 'tracker_confidences', 'similarity_scores']
+ data = {key: [None] * raw_data['num_timesteps'] for key in data_keys}
+ unique_gt_ids = []
+ unique_tracker_ids = []
+ num_gt_dets = 0
+ num_tracker_dets = 0
+ for t in range(raw_data['num_timesteps']):
+
+ # Only extract relevant dets for this class for preproc and eval (cls)
+ gt_class_mask = np.atleast_1d(raw_data['gt_classes'][t] == cls_id)
+ gt_class_mask = gt_class_mask.astype(np.bool)
+ gt_ids = raw_data['gt_ids'][t][gt_class_mask]
+ gt_dets = raw_data['gt_dets'][t][gt_class_mask]
+
+ tracker_class_mask = np.atleast_1d(raw_data['tracker_classes'][t] == cls_id)
+ tracker_class_mask = tracker_class_mask.astype(np.bool)
+ tracker_ids = raw_data['tracker_ids'][t][tracker_class_mask]
+ tracker_dets = raw_data['tracker_dets'][t][tracker_class_mask]
+ tracker_confidences = raw_data['tracker_confidences'][t][tracker_class_mask]
+ similarity_scores = raw_data['similarity_scores'][t][gt_class_mask, :][:, tracker_class_mask]
+
+ # Match tracker and gt dets (with hungarian algorithm).
+ unmatched_indices = np.arange(tracker_ids.shape[0])
+ if gt_ids.shape[0] > 0 and tracker_ids.shape[0] > 0:
+ matching_scores = similarity_scores.copy()
+ matching_scores[matching_scores < 0.5 - np.finfo('float').eps] = 0
+ match_rows, match_cols = linear_sum_assignment(-matching_scores)
+ actually_matched_mask = matching_scores[match_rows, match_cols] > 0 + np.finfo('float').eps
+ match_cols = match_cols[actually_matched_mask]
+ unmatched_indices = np.delete(unmatched_indices, match_cols, axis=0)
+
+ if gt_ids.shape[0] == 0 and not is_neg_category:
+ to_remove_tracker = unmatched_indices
+ elif is_not_exhaustively_labeled:
+ to_remove_tracker = unmatched_indices
+ else:
+ to_remove_tracker = np.array([], dtype=np.int)
+
+ # remove all unwanted unmatched tracker detections
+ data['tracker_ids'][t] = np.delete(tracker_ids, to_remove_tracker, axis=0)
+ data['tracker_dets'][t] = np.delete(tracker_dets, to_remove_tracker, axis=0)
+ data['tracker_confidences'][t] = np.delete(tracker_confidences, to_remove_tracker, axis=0)
+ similarity_scores = np.delete(similarity_scores, to_remove_tracker, axis=1)
+
+ data['gt_ids'][t] = gt_ids
+ data['gt_dets'][t] = gt_dets
+ data['similarity_scores'][t] = similarity_scores
+
+ unique_gt_ids += list(np.unique(data['gt_ids'][t]))
+ unique_tracker_ids += list(np.unique(data['tracker_ids'][t]))
+ num_tracker_dets += len(data['tracker_ids'][t])
+ num_gt_dets += len(data['gt_ids'][t])
+
+ # Re-label IDs such that there are no empty IDs
+ if len(unique_gt_ids) > 0:
+ unique_gt_ids = np.unique(unique_gt_ids)
+ gt_id_map = np.nan * np.ones((np.max(unique_gt_ids) + 1))
+ gt_id_map[unique_gt_ids] = np.arange(len(unique_gt_ids))
+ for t in range(raw_data['num_timesteps']):
+ if len(data['gt_ids'][t]) > 0:
+ data['gt_ids'][t] = gt_id_map[data['gt_ids'][t]].astype(np.int)
+ if len(unique_tracker_ids) > 0:
+ unique_tracker_ids = np.unique(unique_tracker_ids)
+ tracker_id_map = np.nan * np.ones((np.max(unique_tracker_ids) + 1))
+ tracker_id_map[unique_tracker_ids] = np.arange(len(unique_tracker_ids))
+ for t in range(raw_data['num_timesteps']):
+ if len(data['tracker_ids'][t]) > 0:
+ data['tracker_ids'][t] = tracker_id_map[data['tracker_ids'][t]].astype(np.int)
+
+ # Record overview statistics.
+ data['num_tracker_dets'] = num_tracker_dets
+ data['num_gt_dets'] = num_gt_dets
+ data['num_tracker_ids'] = len(unique_tracker_ids)
+ data['num_gt_ids'] = len(unique_gt_ids)
+ data['num_timesteps'] = raw_data['num_timesteps']
+ data['seq'] = raw_data['seq']
+
+ # get track representations
+ data['gt_tracks'] = raw_data['classes_to_gt_tracks'][cls_id]
+ data['gt_track_ids'] = raw_data['classes_to_gt_track_ids'][cls_id]
+ data['gt_track_lengths'] = raw_data['classes_to_gt_track_lengths'][cls_id]
+ data['gt_track_areas'] = raw_data['classes_to_gt_track_areas'][cls_id]
+ data['dt_tracks'] = raw_data['classes_to_dt_tracks'][cls_id]
+ data['dt_track_ids'] = raw_data['classes_to_dt_track_ids'][cls_id]
+ data['dt_track_lengths'] = raw_data['classes_to_dt_track_lengths'][cls_id]
+ data['dt_track_areas'] = raw_data['classes_to_dt_track_areas'][cls_id]
+ data['dt_track_scores'] = raw_data['classes_to_dt_track_scores'][cls_id]
+ data['not_exhaustively_labeled'] = is_not_exhaustively_labeled
+ data['iou_type'] = self._iou_type()
+
+ # sort tracker data tracks by tracker confidence scores
+ if data['dt_tracks']:
+ idx = np.argsort([-score for score in data['dt_track_scores']], kind="mergesort")
+ data['dt_track_scores'] = [data['dt_track_scores'][i] for i in idx]
+ data['dt_tracks'] = [data['dt_tracks'][i] for i in idx]
+ data['dt_track_ids'] = [data['dt_track_ids'][i] for i in idx]
+ data['dt_track_lengths'] = [data['dt_track_lengths'][i] for i in idx]
+ data['dt_track_areas'] = [data['dt_track_areas'][i] for i in idx]
+ # Ensure that ids are unique per timestep.
+ self._check_unique_ids(data)
+
+ return data
+
+ def _calculate_similarities(self, gt_dets_t, tracker_dets_t):
+ similarity_scores = self._calculate_box_ious(gt_dets_t, tracker_dets_t)
+ return similarity_scores
+
+ def _merge_categories(self, annotations):
+ """
+ Merges categories with a merged tag. Adapted from https://github.com/TAO-Dataset
+ :param annotations: the annotations in which the classes should be merged
+ :return: None
+ """
+ merge_map = {}
+ for category in self.gt_data['categories']:
+ if 'merged' in category:
+ for to_merge in category['merged']:
+ merge_map[to_merge['id']] = category['id']
+
+ for ann in annotations:
+ ann['category_id'] = merge_map.get(ann['category_id'], ann['category_id'])
+
+ def _compute_vid_mappings(self, annotations):
+ """
+ Computes mappings from Videos to corresponding tracks and images.
+ :param annotations: the annotations for which the mapping should be generated
+ :return: the video-to-track-mapping, the video-to-image-mapping
+ """
+ vids_to_tracks = {}
+ vids_to_imgs = {}
+ vid_ids = [vid['id'] for vid in self.gt_data['videos']]
+
+ # compute an mapping from image IDs to images
+ images = {}
+ for image in self.gt_data['images']:
+ images[image['id']] = image
+
+ for ann in annotations:
+ ann["area"] = self._calculate_area_for_ann(ann)
+
+ vid = ann["video_id"]
+ if ann["video_id"] not in vids_to_tracks.keys():
+ vids_to_tracks[ann["video_id"]] = list()
+ if ann["video_id"] not in vids_to_imgs.keys():
+ vids_to_imgs[ann["video_id"]] = list()
+
+ # Fill in vids_to_tracks
+ tid = ann["track_id"]
+ exist_tids = [track["id"] for track in vids_to_tracks[vid]]
+ try:
+ index1 = exist_tids.index(tid)
+ except ValueError:
+ index1 = -1
+ if tid not in exist_tids:
+ curr_track = {"id": tid, "category_id": ann["category_id"],
+ "video_id": vid, "annotations": [ann]}
+ vids_to_tracks[vid].append(curr_track)
+ else:
+ vids_to_tracks[vid][index1]["annotations"].append(ann)
+
+ # Fill in vids_to_imgs
+ img_id = ann['image_id']
+ exist_img_ids = [img["id"] for img in vids_to_imgs[vid]]
+ try:
+ index2 = exist_img_ids.index(img_id)
+ except ValueError:
+ index2 = -1
+ if index2 == -1:
+ curr_img = {"id": img_id, "annotations": [ann]}
+ vids_to_imgs[vid].append(curr_img)
+ else:
+ vids_to_imgs[vid][index2]["annotations"].append(ann)
+
+ # sort annotations by frame index and compute track area
+ for vid, tracks in vids_to_tracks.items():
+ for track in tracks:
+ track["annotations"] = sorted(
+ track['annotations'],
+ key=lambda x: images[x['image_id']]['frame_index'])
+ # Computer average area
+ track["area"] = (sum(x['area'] for x in track['annotations']) / len(track['annotations']))
+
+ # Ensure all videos are present
+ for vid_id in vid_ids:
+ if vid_id not in vids_to_tracks.keys():
+ vids_to_tracks[vid_id] = []
+ if vid_id not in vids_to_imgs.keys():
+ vids_to_imgs[vid_id] = []
+
+ return vids_to_tracks, vids_to_imgs
+
+ def _compute_image_to_timestep_mappings(self):
+ """
+ Computes a mapping from images to the corresponding timestep in the sequence.
+ :return: the image-to-timestep-mapping
+ """
+ images = {}
+ for image in self.gt_data['images']:
+ images[image['id']] = image
+
+ seq_to_imgs_to_timestep = {vid['id']: dict() for vid in self.gt_data['videos']}
+ for vid in seq_to_imgs_to_timestep:
+ curr_imgs = [img['id'] for img in self.videos_to_gt_images[vid]]
+ curr_imgs = sorted(curr_imgs, key=lambda x: images[x]['frame_index'])
+ seq_to_imgs_to_timestep[vid] = {curr_imgs[i]: i for i in range(len(curr_imgs))}
+
+ return seq_to_imgs_to_timestep
+
+ def _limit_dets_per_image(self, annotations):
+ """
+ Limits the number of detections for each image to config['MAX_DETECTIONS']. Adapted from
+ https://github.com/TAO-Dataset/
+ :param annotations: the annotations in which the detections should be limited
+ :return: the annotations with limited detections
+ """
+ max_dets = self.config['MAX_DETECTIONS']
+ img_ann = defaultdict(list)
+ for ann in annotations:
+ img_ann[ann["image_id"]].append(ann)
+
+ for img_id, _anns in img_ann.items():
+ if len(_anns) <= max_dets:
+ continue
+ _anns = sorted(_anns, key=lambda x: x["score"], reverse=True)
+ img_ann[img_id] = _anns[:max_dets]
+
+ return [ann for anns in img_ann.values() for ann in anns]
+
+ def _fill_video_ids_inplace(self, annotations):
+ """
+ Fills in missing video IDs inplace. Adapted from https://github.com/TAO-Dataset/
+ :param annotations: the annotations for which the videos IDs should be filled inplace
+ :return: None
+ """
+ missing_video_id = [x for x in annotations if 'video_id' not in x]
+ if missing_video_id:
+ image_id_to_video_id = {
+ x['id']: x['video_id'] for x in self.gt_data['images']
+ }
+ for x in missing_video_id:
+ x['video_id'] = image_id_to_video_id[x['image_id']]
+
+ @staticmethod
+ def _make_track_ids_unique(annotations):
+ """
+ Makes the track IDs unqiue over the whole annotation set. Adapted from https://github.com/TAO-Dataset/
+ :param annotations: the annotation set
+ :return: the number of updated IDs
+ """
+ track_id_videos = {}
+ track_ids_to_update = set()
+ max_track_id = 0
+ for ann in annotations:
+ t = ann['track_id']
+ if t not in track_id_videos:
+ track_id_videos[t] = ann['video_id']
+
+ if ann['video_id'] != track_id_videos[t]:
+ # Track id is assigned to multiple videos
+ track_ids_to_update.add(t)
+ max_track_id = max(max_track_id, t)
+
+ if track_ids_to_update:
+ #print('true')
+ next_id = itertools.count(max_track_id + 1)
+ new_track_ids = defaultdict(lambda: next(next_id))
+ for ann in annotations:
+ t = ann['track_id']
+ v = ann['video_id']
+ if t in track_ids_to_update:
+ ann['track_id'] = new_track_ids[t, v]
+ return len(track_ids_to_update)
+
+ def _split_known_unknown_distractor(self):
+ all_ids = set([i for i in range(1, 2000)]) # 2000 is larger than the max category id in TAO-OW.
+ # `knowns` includes 78 TAO_category_ids that corresponds to 78 COCO classes.
+ # (The other 2 COCO classes do not have corresponding classes in TAO).
+ self.knowns = {4, 13, 1038, 544, 1057, 34, 35, 36, 41, 45, 58, 60, 579, 1091, 1097, 1099, 78, 79, 81, 91, 1115,
+ 1117, 95, 1122, 99, 1132, 621, 1135, 625, 118, 1144, 126, 642, 1155, 133, 1162, 139, 154, 174, 185,
+ 699, 1215, 714, 717, 1229, 211, 729, 221, 229, 747, 235, 237, 779, 276, 805, 299, 829, 852, 347,
+ 371, 382, 896, 392, 926, 937, 428, 429, 961, 452, 979, 980, 982, 475, 480, 993, 1001, 502, 1018}
+ # `distractors` is defined as in the paper "Opening up Open-World Tracking"
+ self.distractors = {20, 63, 108, 180, 188, 204, 212, 247, 303, 403, 407, 415, 490, 504, 507, 513, 529, 567,
+ 569, 588, 672, 691, 702, 708, 711, 720, 736, 737, 798, 813, 815, 827, 831, 851, 877, 883,
+ 912, 971, 976, 1130, 1133, 1134, 1169, 1184, 1220}
+ self.unknowns = all_ids.difference(self.knowns.union(self.distractors))
+
+ def _filter_gt_data(self, raw_gt_data):
+ """
+ Filter out irrelevant data in the raw_gt_data
+ Args:
+ raw_gt_data: directly loaded from json.
+
+ Returns:
+ filtered gt_data
+ """
+ valid_cat_ids = list()
+ if self.subset == "known":
+ valid_cat_ids = self.knowns
+ elif self.subset == "distractor":
+ valid_cat_ids = self.distractors
+ elif self.subset == "unknown":
+ valid_cat_ids = self.unknowns
+ # elif self.subset == "test_only_unknowns":
+ # valid_cat_ids = test_only_unknowns
+ else:
+ raise Exception("The parameter `SUBSET` is incorrect")
+
+ filtered = dict()
+ filtered["videos"] = raw_gt_data["videos"]
+ # filtered["videos"] = list()
+ unwanted_vid = set()
+ # for video in raw_gt_data["videos"]:
+ # datasrc = video["name"].split('/')[1]
+ # if datasrc in data_srcs:
+ # filtered["videos"].append(video)
+ # else:
+ # unwanted_vid.add(video["id"])
+
+ filtered["annotations"] = list()
+ for ann in raw_gt_data["annotations"]:
+ if (ann["video_id"] not in unwanted_vid) and (ann["category_id"] in valid_cat_ids):
+ filtered["annotations"].append(ann)
+
+ filtered["tracks"] = list()
+ for track in raw_gt_data["tracks"]:
+ if (track["video_id"] not in unwanted_vid) and (track["category_id"] in valid_cat_ids):
+ filtered["tracks"].append(track)
+
+ filtered["images"] = list()
+ for image in raw_gt_data["images"]:
+ if image["video_id"] not in unwanted_vid:
+ filtered["images"].append(image)
+
+ filtered["categories"] = list()
+ for cat in raw_gt_data["categories"]:
+ if cat["id"] in valid_cat_ids:
+ filtered["categories"].append(cat)
+
+ if "info" in raw_gt_data:
+ filtered["info"] = raw_gt_data["info"]
+ if "licenses" in raw_gt_data:
+ filtered["licenses"] = raw_gt_data["licenses"]
+
+ if "track_id_offsets" in raw_gt_data:
+ filtered["track_id_offsets"] = raw_gt_data["track_id_offsets"]
+
+ if "split" in raw_gt_data:
+ filtered["split"] = raw_gt_data["split"]
+
+ return filtered
diff --git a/val_utils/trackeval/datasets/burst_helpers/convert_burst_format_to_tao_format.py b/val_utils/trackeval/datasets/burst_helpers/convert_burst_format_to_tao_format.py
new file mode 100644
index 0000000000000000000000000000000000000000..129e42c96c835b8a67519245823b4e3f245eac60
--- /dev/null
+++ b/val_utils/trackeval/datasets/burst_helpers/convert_burst_format_to_tao_format.py
@@ -0,0 +1,39 @@
+import json
+import argparse
+from .format_converter import GroundTruthBURSTFormatToTAOFormatConverter, PredictionBURSTFormatToTAOFormatConverter
+
+
+def main(args):
+ with open(args.gt_input_file) as f:
+ ali_format_gt = json.load(f)
+ tao_format_gt = GroundTruthBURSTFormatToTAOFormatConverter(
+ ali_format_gt, args.split).convert()
+ with open(args.gt_output_file, 'w') as f:
+ json.dump(tao_format_gt, f)
+
+ if args.pred_input_file is None:
+ return
+ with open(args.pred_input_file) as f:
+ ali_format_pred = json.load(f)
+ tao_format_pred = PredictionBURSTFormatToTAOFormatConverter(
+ tao_format_gt, ali_format_pred, args.split,
+ args.exemplar_guided).convert()
+ with open(args.pred_output_file, 'w') as f:
+ json.dump(tao_format_pred, f)
+
+
+if __name__ == '__main__':
+ parser = argparse.ArgumentParser()
+ parser.add_argument(
+ '--gt_input_file', type=str,
+ default='../data/gt/tsunami/exemplar_guided/validation_all_annotations.json')
+ parser.add_argument('--gt_output_file', type=str,
+ default='/tmp/val_gt.json')
+ parser.add_argument('--pred_input_file', type=str,
+ default='../data/trackers/tsunami/exemplar_guided/STCN_off_the_shelf/data/results.json')
+ parser.add_argument('--pred_output_file', type=str,
+ default='/tmp/pred.json')
+ parser.add_argument('--split', type=str, default='validation')
+ parser.add_argument('--exemplar_guided', type=bool, default=True)
+ args_ = parser.parse_args()
+ main(args_)
diff --git a/val_utils/trackeval/datasets/burst_helpers/format_converter.py b/val_utils/trackeval/datasets/burst_helpers/format_converter.py
new file mode 100644
index 0000000000000000000000000000000000000000..da43a9a3c467eef6bb4f8b1668d65ea13f9c544f
--- /dev/null
+++ b/val_utils/trackeval/datasets/burst_helpers/format_converter.py
@@ -0,0 +1,259 @@
+import os
+import json
+import pycocotools.mask as cocomask
+from tabulate import tabulate
+from typing import Union
+
+
+def _global_track_id(*, local_track_id: Union[str, int],
+ video_id: Union[str, int],
+ track_id_mapping) -> int:
+ # remap local track ids into globally unique ids
+ return track_id_mapping[str(video_id)][str(local_track_id)]
+
+
+class GroundTruthBURSTFormatToTAOFormatConverter:
+ def __init__(self, ali_format):
+ self._ali_format = ali_format
+ self._split = ali_format['split']
+ self._categories = self._make_categories()
+ self._videos = []
+ self._annotations = []
+ self._tracks = {}
+ self._images = []
+ self._next_img_id = 0
+ self._next_ann_id = 0
+
+ self._track_id_mapping = self._load_track_id_mapping()
+
+ for seq in ali_format['sequences']:
+ self._visit_seq(seq)
+
+ def _load_track_id_mapping(self):
+ id_map = {}
+ next_global_track_id = 1
+ for seq in self._ali_format['sequences']:
+ seq_id = seq['id']
+ seq_id_map = {}
+ id_map[str(seq_id)] = seq_id_map
+ for local_track_id in seq['track_category_ids']:
+ seq_id_map[str(local_track_id)] = next_global_track_id
+ next_global_track_id += 1
+ return id_map
+
+ def global_track_id(self, *, local_track_id: Union[str, int],
+ video_id: Union[str, int]) -> int:
+ return _global_track_id(local_track_id=local_track_id,
+ video_id=video_id,
+ track_id_mapping=self._track_id_mapping)
+
+ def _visit_seq(self, seq):
+ self._make_video(seq)
+ imgs = self._make_images(seq)
+ self._make_annotations_and_tracks(seq, imgs)
+
+ def _make_images(self, seq):
+ imgs = []
+ for img_path in seq['annotated_image_paths']:
+ video = self._split + '/' + seq['dataset'] + '/' + seq['seq_name']
+ file_name = video + '/' + img_path
+
+ # TODO: once python 3.9 is more common, we can use this nicer and safer code
+ #stripped = img_path.removesuffix('.jpg').removesuffix('.png').removeprefix('frame')
+ stripped = img_path.replace('.jpg', '').replace('.png', '').replace('frame', '')
+
+ last = stripped.split('_')[-1]
+ frame_idx = int(last)
+
+ img = {'id': self._next_img_id, 'video': video,
+ 'width': seq['width'], 'height': seq['height'],
+ 'file_name': file_name,
+ 'frame_index': frame_idx,
+ 'video_id': seq['id']}
+ self._next_img_id += 1
+ self._images.append(img)
+ imgs.append(img)
+ return imgs
+
+ def _make_video(self, seq):
+ video_id = seq['id']
+ dataset = seq['dataset']
+ seq_name = seq['seq_name']
+ name = f'{self._split}/' + dataset + '/' + seq_name
+ video = {
+ 'id': video_id, 'width': seq['width'], 'height': seq['height'],
+ 'neg_category_ids': seq['neg_category_ids'],
+ 'not_exhaustive_category_ids': seq['not_exhaustive_category_ids'],
+ 'name': name, 'metadata': {'dataset': dataset}}
+ self._videos.append(video)
+
+ def _make_annotations_and_tracks(self, seq, imgs):
+ video_id = seq['id']
+ segs = seq['segmentations']
+ assert len(segs) == len(imgs), (len(segs), len(imgs))
+ for frame_segs, img in zip(segs, imgs):
+ for local_track_id, seg in frame_segs.items():
+ distractors = {20, 63, 108, 180, 188, 204, 212, 247, 303, 403, 407, 415, 490, 504, 507, 513, 529, 567,
+ 569, 588, 672, 691, 702, 708, 711, 720, 736, 737, 798, 813, 815, 827, 831, 851, 877, 883,
+ 912, 971, 976, 1130, 1133, 1134, 1169, 1184, 1220}
+ global_track_id = self.global_track_id(
+ local_track_id=local_track_id, video_id=seq['id'])
+ rle = seg['rle']
+ segmentation = {'counts': rle,
+ 'size': [img['height'], img['width']]}
+ image_id = img['id']
+ category_id = int(seq['track_category_ids'][local_track_id])
+ if category_id in distractors:
+ continue
+ coco_bbox = cocomask.toBbox(segmentation)
+ bbox = [int(x) for x in coco_bbox]
+ ann = {'segmentation': segmentation, 'id': self._next_ann_id,
+ 'image_id': image_id, 'category_id': category_id,
+ 'track_id': global_track_id, 'video_id': video_id,
+ 'bbox': bbox}
+ self._next_ann_id += 1
+ self._annotations.append(ann)
+
+ if global_track_id not in self._tracks:
+ track = {'id': global_track_id, 'category_id': category_id,
+ 'video_id': video_id}
+ self._tracks[global_track_id] = track
+
+ def convert(self):
+ tracks = sorted(self._tracks.values(), key=lambda t: t['id'])
+ return {'videos': self._videos, 'annotations': self._annotations,
+ 'tracks': tracks, 'images': self._images,
+ 'categories': self._categories,
+ 'track_id_mapping': self._track_id_mapping,
+ 'split': self._split}
+
+ def _make_categories(self):
+ tao_categories_path = os.path.join(os.path.dirname(__file__), 'tao_categories.json')
+ with open(tao_categories_path) as f:
+ return json.load(f)
+
+
+class PredictionBURSTFormatToTAOFormatConverter:
+ def __init__(self, gt, ali_format, exemplar_guided):
+ self._gt = gt
+ self._ali_format = ali_format
+ if 'split' in ali_format:
+ self._split = ali_format['split']
+ gt_split = self._gt['split']
+ assert self._split == gt_split, (self._split, gt_split)
+ else:
+ self._split = self._gt['split']
+ self._exemplar_guided = exemplar_guided
+ self._result = []
+ self._next_det_id = 0
+
+ self._img_by_filename = {}
+ for img in self._gt['images']:
+ file_name = img['file_name']
+ assert file_name not in self._img_by_filename
+ self._img_by_filename[file_name] = img
+
+ self._gt_track_by_track_id = {}
+ for track in self._gt['tracks']:
+ self._gt_track_by_track_id[int(track['id'])] = track
+
+ self._filtered_out_track_ids = set()
+
+ for seq in ali_format['sequences']:
+ self._visit_seq(seq)
+
+ if exemplar_guided and len(self._filtered_out_track_ids) > 0:
+ self.print_filter_out_debug_info(ali_format)
+
+ def print_filter_out_debug_info(self, ali_format):
+ track_ids_in_pred = set()
+ a_dict_for_debugging = {}
+ for seq in ali_format['sequences']:
+ for local_track_id in seq['track_category_ids']:
+ global_track_id = _global_track_id(
+ local_track_id=local_track_id, video_id=seq['id'],
+ track_id_mapping=self._gt['track_id_mapping'])
+ track_ids_in_pred.add(global_track_id)
+ a_dict_for_debugging[global_track_id] = {'seq': seq,
+ 'local_track_id': local_track_id}
+ print('Number of Track ids in pred:', len(track_ids_in_pred))
+ print('Exemplar Guided: Filtered out',
+ len(self._filtered_out_track_ids),
+ 'tracks which were not found in the ground truth.')
+ track_ids_after_filtering = set(d['track_id'] for d in self._result)
+ print('Number of tracks after filtering:',
+ len(track_ids_after_filtering))
+ problem_tracks = list(
+ track_ids_in_pred - track_ids_after_filtering - self._filtered_out_track_ids)
+ if len(problem_tracks) > 0:
+ print("\nWARNING:", len(problem_tracks),
+ "object tracks are not present. There could be a number of reasons for this:\n"
+ "(1) If you are running evaluation for the box/point exemplar-guided task then this is to be expected"
+ " because your tracker probably didn't predict masks for every ground-truth object instance.\n"
+ "(2) If you are running evaluation for the mask exemplar-guided task, then this could indicate a "
+ "problem. Assume that you copied the given first-frame object mask to your predicted result, this "
+ "should not happen. It could be that your predictions are at the wrong frame-rate i.e. you have no "
+ "predicted masks for video frames which will be evaluated.\n")
+
+ rows = []
+ for xx in problem_tracks:
+ rows.append([a_dict_for_debugging[xx]['seq']['dataset'],
+ a_dict_for_debugging[xx]['seq']['seq_name'],
+ a_dict_for_debugging[xx]['local_track_id']])
+
+ print("For your reference, the sequence name and track IDs for these missing tracks are:")
+ print(tabulate(rows, ["Dataset", "Sequence Name", "Track ID"]))
+
+ def _visit_seq(self, seq):
+ dataset = seq['dataset']
+ seq_name = seq['seq_name']
+ assert len(seq['segmentations']) == len(seq['annotated_image_paths'])
+ for frame_segs, img_path in zip(seq['segmentations'],
+ seq['annotated_image_paths']):
+ for local_track_id_str, track_det in frame_segs.items():
+ rle = track_det['rle']
+
+ file_name = self._split + '/' + dataset + '/' + seq_name + '/' + img_path
+ # the result might have a higher frame rate than the ground truth
+ if file_name not in self._img_by_filename:
+ continue
+
+ img = self._img_by_filename[file_name]
+ img_id = img['id']
+ height = img['height']
+ width = img['width']
+ segmentation = {'counts': rle, 'size': [height, width]}
+
+ local_track_id = int(local_track_id_str)
+ if self._exemplar_guided:
+ global_track_id = _global_track_id(
+ local_track_id=local_track_id, video_id=seq['id'],
+ track_id_mapping=self._gt['track_id_mapping'])
+ else:
+ global_track_id = local_track_id
+ coco_bbox = cocomask.toBbox(segmentation)
+ bbox = [int(x) for x in coco_bbox]
+ det = {'id': self._next_det_id, 'image_id': img_id,
+ 'track_id': global_track_id, 'bbox': bbox,
+ 'segmentation': segmentation}
+ if self._exemplar_guided:
+ if global_track_id not in self._gt_track_by_track_id:
+ self._filtered_out_track_ids.add(global_track_id)
+ continue
+ gt_track = self._gt_track_by_track_id[global_track_id]
+ category_id = gt_track['category_id']
+ det['category_id'] = category_id
+ elif 'category_id' in track_det:
+ det['category_id'] = track_det['category_id']
+ else:
+ category_id = seq['track_category_ids'][local_track_id_str]
+ det['category_id'] = category_id
+ self._next_det_id += 1
+ if 'score' in track_det:
+ det['score'] = track_det['score']
+ else:
+ det['score'] = 1.0
+ self._result.append(det)
+
+ def convert(self):
+ return self._result
diff --git a/val_utils/trackeval/datasets/burst_helpers/tao_categories.json b/val_utils/trackeval/datasets/burst_helpers/tao_categories.json
new file mode 100644
index 0000000000000000000000000000000000000000..0368949f21d28c54c1b6d60afd3b4762821da5d2
--- /dev/null
+++ b/val_utils/trackeval/datasets/burst_helpers/tao_categories.json
@@ -0,0 +1 @@
+[{"id": 1, "synset": "acorn.n.01", "synonyms": ["acorn"], "def": "nut from an oak tree", "name": "acorn"}, {"id": 2, "synset": "aerosol.n.02", "synonyms": ["aerosol_can", "spray_can"], "def": "a dispenser that holds a substance under pressure", "name": "aerosol_can"}, {"id": 3, "synset": "air_conditioner.n.01", "synonyms": ["air_conditioner"], "def": "a machine that keeps air cool and dry", "name": "air_conditioner"}, {"id": 4, "synset": "airplane.n.01", "synonyms": ["airplane", "aeroplane"], "def": "an aircraft that has a fixed wing and is powered by propellers or jets", "name": "airplane"}, {"id": 5, "synset": "alarm_clock.n.01", "synonyms": ["alarm_clock"], "def": "a clock that wakes a sleeper at some preset time", "name": "alarm_clock"}, {"id": 6, "synset": "alcohol.n.01", "synonyms": ["alcohol", "alcoholic_beverage"], "def": "a liquor or brew containing alcohol as the active agent", "name": "alcohol"}, {"id": 7, "synset": "alligator.n.02", "synonyms": ["alligator", "gator"], "def": "amphibious reptiles related to crocodiles but with shorter broader snouts", "name": "alligator"}, {"id": 8, "synset": "almond.n.02", "synonyms": ["almond"], "def": "oval-shaped edible seed of the almond tree", "name": "almond"}, {"id": 9, "synset": "ambulance.n.01", "synonyms": ["ambulance"], "def": "a vehicle that takes people to and from hospitals", "name": "ambulance"}, {"id": 10, "synset": "amplifier.n.01", "synonyms": ["amplifier"], "def": "electronic equipment that increases strength of signals", "name": "amplifier"}, {"id": 11, "synset": "anklet.n.03", "synonyms": ["anklet", "ankle_bracelet"], "def": "an ornament worn around the ankle", "name": "anklet"}, {"id": 12, "synset": "antenna.n.01", "synonyms": ["antenna", "aerial", "transmitting_aerial"], "def": "an electrical device that sends or receives radio or television signals", "name": "antenna"}, {"id": 13, "synset": "apple.n.01", "synonyms": ["apple"], "def": "fruit with red or yellow or green skin and sweet to tart crisp whitish flesh", "name": "apple"}, {"id": 14, "synset": "apple_juice.n.01", "synonyms": ["apple_juice"], "def": "the juice of apples", "name": "apple_juice"}, {"id": 15, "synset": "applesauce.n.01", "synonyms": ["applesauce"], "def": "puree of stewed apples usually sweetened and spiced", "name": "applesauce"}, {"id": 16, "synset": "apricot.n.02", "synonyms": ["apricot"], "def": "downy yellow to rosy-colored fruit resembling a small peach", "name": "apricot"}, {"id": 17, "synset": "apron.n.01", "synonyms": ["apron"], "def": "a garment of cloth that is tied about the waist and worn to protect clothing", "name": "apron"}, {"id": 18, "synset": "aquarium.n.01", "synonyms": ["aquarium", "fish_tank"], "def": "a tank/pool/bowl filled with water for keeping live fish and underwater animals", "name": "aquarium"}, {"id": 19, "synset": "armband.n.02", "synonyms": ["armband"], "def": "a band worn around the upper arm", "name": "armband"}, {"id": 20, "synset": "armchair.n.01", "synonyms": ["armchair"], "def": "chair with a support on each side for arms", "name": "armchair"}, {"id": 21, "synset": "armoire.n.01", "synonyms": ["armoire"], "def": "a large wardrobe or cabinet", "name": "armoire"}, {"id": 22, "synset": "armor.n.01", "synonyms": ["armor", "armour"], "def": "protective covering made of metal and used in combat", "name": "armor"}, {"id": 23, "synset": "artichoke.n.02", "synonyms": ["artichoke"], "def": "a thistlelike flower head with edible fleshy leaves and heart", "name": "artichoke"}, {"id": 24, "synset": "ashcan.n.01", "synonyms": ["trash_can", "garbage_can", "wastebin", "dustbin", "trash_barrel", "trash_bin"], "def": "a bin that holds rubbish until it is collected", "name": "trash_can"}, {"id": 25, "synset": "ashtray.n.01", "synonyms": ["ashtray"], "def": "a receptacle for the ash from smokers' cigars or cigarettes", "name": "ashtray"}, {"id": 26, "synset": "asparagus.n.02", "synonyms": ["asparagus"], "def": "edible young shoots of the asparagus plant", "name": "asparagus"}, {"id": 27, "synset": "atomizer.n.01", "synonyms": ["atomizer", "atomiser", "spray", "sprayer", "nebulizer", "nebuliser"], "def": "a dispenser that turns a liquid (such as perfume) into a fine mist", "name": "atomizer"}, {"id": 28, "synset": "avocado.n.01", "synonyms": ["avocado"], "def": "a pear-shaped fruit with green or blackish skin and rich yellowish pulp enclosing a single large seed", "name": "avocado"}, {"id": 29, "synset": "award.n.02", "synonyms": ["award", "accolade"], "def": "a tangible symbol signifying approval or distinction", "name": "award"}, {"id": 30, "synset": "awning.n.01", "synonyms": ["awning"], "def": "a canopy made of canvas to shelter people or things from rain or sun", "name": "awning"}, {"id": 31, "synset": "ax.n.01", "synonyms": ["ax", "axe"], "def": "an edge tool with a heavy bladed head mounted across a handle", "name": "ax"}, {"id": 32, "synset": "baby_buggy.n.01", "synonyms": ["baby_buggy", "baby_carriage", "perambulator", "pram", "stroller"], "def": "a small vehicle with four wheels in which a baby or child is pushed around", "name": "baby_buggy"}, {"id": 33, "synset": "backboard.n.01", "synonyms": ["basketball_backboard"], "def": "a raised vertical board with basket attached; used to play basketball", "name": "basketball_backboard"}, {"id": 34, "synset": "backpack.n.01", "synonyms": ["backpack", "knapsack", "packsack", "rucksack", "haversack"], "def": "a bag carried by a strap on your back or shoulder", "name": "backpack"}, {"id": 35, "synset": "bag.n.04", "synonyms": ["handbag", "purse", "pocketbook"], "def": "a container used for carrying money and small personal items or accessories", "name": "handbag"}, {"id": 36, "synset": "bag.n.06", "synonyms": ["suitcase", "baggage", "luggage"], "def": "cases used to carry belongings when traveling", "name": "suitcase"}, {"id": 37, "synset": "bagel.n.01", "synonyms": ["bagel", "beigel"], "def": "glazed yeast-raised doughnut-shaped roll with hard crust", "name": "bagel"}, {"id": 38, "synset": "bagpipe.n.01", "synonyms": ["bagpipe"], "def": "a tubular wind instrument; the player blows air into a bag and squeezes it out", "name": "bagpipe"}, {"id": 39, "synset": "baguet.n.01", "synonyms": ["baguet", "baguette"], "def": "narrow French stick loaf", "name": "baguet"}, {"id": 40, "synset": "bait.n.02", "synonyms": ["bait", "lure"], "def": "something used to lure fish or other animals into danger so they can be trapped or killed", "name": "bait"}, {"id": 41, "synset": "ball.n.06", "synonyms": ["ball"], "def": "a spherical object used as a plaything", "name": "ball"}, {"id": 42, "synset": "ballet_skirt.n.01", "synonyms": ["ballet_skirt", "tutu"], "def": "very short skirt worn by ballerinas", "name": "ballet_skirt"}, {"id": 43, "synset": "balloon.n.01", "synonyms": ["balloon"], "def": "large tough nonrigid bag filled with gas or heated air", "name": "balloon"}, {"id": 44, "synset": "bamboo.n.02", "synonyms": ["bamboo"], "def": "woody tropical grass having hollow woody stems", "name": "bamboo"}, {"id": 45, "synset": "banana.n.02", "synonyms": ["banana"], "def": "elongated crescent-shaped yellow fruit with soft sweet flesh", "name": "banana"}, {"id": 46, "synset": "band_aid.n.01", "synonyms": ["Band_Aid"], "def": "trade name for an adhesive bandage to cover small cuts or blisters", "name": "Band_Aid"}, {"id": 47, "synset": "bandage.n.01", "synonyms": ["bandage"], "def": "a piece of soft material that covers and protects an injured part of the body", "name": "bandage"}, {"id": 48, "synset": "bandanna.n.01", "synonyms": ["bandanna", "bandana"], "def": "large and brightly colored handkerchief; often used as a neckerchief", "name": "bandanna"}, {"id": 49, "synset": "banjo.n.01", "synonyms": ["banjo"], "def": "a stringed instrument of the guitar family with a long neck and circular body", "name": "banjo"}, {"id": 50, "synset": "banner.n.01", "synonyms": ["banner", "streamer"], "def": "long strip of cloth or paper used for decoration or advertising", "name": "banner"}, {"id": 51, "synset": "barbell.n.01", "synonyms": ["barbell"], "def": "a bar to which heavy discs are attached at each end; used in weightlifting", "name": "barbell"}, {"id": 52, "synset": "barge.n.01", "synonyms": ["barge"], "def": "a flatbottom boat for carrying heavy loads (especially on canals)", "name": "barge"}, {"id": 53, "synset": "barrel.n.02", "synonyms": ["barrel", "cask"], "def": "a cylindrical container that holds liquids", "name": "barrel"}, {"id": 54, "synset": "barrette.n.01", "synonyms": ["barrette"], "def": "a pin for holding women's hair in place", "name": "barrette"}, {"id": 55, "synset": "barrow.n.03", "synonyms": ["barrow", "garden_cart", "lawn_cart", "wheelbarrow"], "def": "a cart for carrying small loads; has handles and one or more wheels", "name": "barrow"}, {"id": 56, "synset": "base.n.03", "synonyms": ["baseball_base"], "def": "a place that the runner must touch before scoring", "name": "baseball_base"}, {"id": 57, "synset": "baseball.n.02", "synonyms": ["baseball"], "def": "a ball used in playing baseball", "name": "baseball"}, {"id": 58, "synset": "baseball_bat.n.01", "synonyms": ["baseball_bat"], "def": "an implement used in baseball by the batter", "name": "baseball_bat"}, {"id": 59, "synset": "baseball_cap.n.01", "synonyms": ["baseball_cap", "jockey_cap", "golf_cap"], "def": "a cap with a bill", "name": "baseball_cap"}, {"id": 60, "synset": "baseball_glove.n.01", "synonyms": ["baseball_glove", "baseball_mitt"], "def": "the handwear used by fielders in playing baseball", "name": "baseball_glove"}, {"id": 61, "synset": "basket.n.01", "synonyms": ["basket", "handbasket"], "def": "a container that is usually woven and has handles", "name": "basket"}, {"id": 62, "synset": "basket.n.03", "synonyms": ["basketball_hoop"], "def": "metal hoop supporting a net through which players try to throw the basketball", "name": "basketball_hoop"}, {"id": 63, "synset": "basketball.n.02", "synonyms": ["basketball"], "def": "an inflated ball used in playing basketball", "name": "basketball"}, {"id": 64, "synset": "bass_horn.n.01", "synonyms": ["bass_horn", "sousaphone", "tuba"], "def": "the lowest brass wind instrument", "name": "bass_horn"}, {"id": 65, "synset": "bat.n.01", "synonyms": ["bat_(animal)"], "def": "nocturnal mouselike mammal with forelimbs modified to form membranous wings", "name": "bat_(animal)"}, {"id": 66, "synset": "bath_mat.n.01", "synonyms": ["bath_mat"], "def": "a heavy towel or mat to stand on while drying yourself after a bath", "name": "bath_mat"}, {"id": 67, "synset": "bath_towel.n.01", "synonyms": ["bath_towel"], "def": "a large towel; to dry yourself after a bath", "name": "bath_towel"}, {"id": 68, "synset": "bathrobe.n.01", "synonyms": ["bathrobe"], "def": "a loose-fitting robe of towelling; worn after a bath or swim", "name": "bathrobe"}, {"id": 69, "synset": "bathtub.n.01", "synonyms": ["bathtub", "bathing_tub"], "def": "a large open container that you fill with water and use to wash the body", "name": "bathtub"}, {"id": 70, "synset": "batter.n.02", "synonyms": ["batter_(food)"], "def": "a liquid or semiliquid mixture, as of flour, eggs, and milk, used in cooking", "name": "batter_(food)"}, {"id": 71, "synset": "battery.n.02", "synonyms": ["battery"], "def": "a portable device that produces electricity", "name": "battery"}, {"id": 72, "synset": "beach_ball.n.01", "synonyms": ["beachball"], "def": "large and light ball; for play at the seaside", "name": "beachball"}, {"id": 73, "synset": "bead.n.01", "synonyms": ["bead"], "def": "a small ball with a hole through the middle used for ornamentation, jewellery, etc.", "name": "bead"}, {"id": 74, "synset": "beaker.n.01", "synonyms": ["beaker"], "def": "a flatbottomed jar made of glass or plastic; used for chemistry", "name": "beaker"}, {"id": 75, "synset": "bean_curd.n.01", "synonyms": ["bean_curd", "tofu"], "def": "cheeselike food made of curdled soybean milk", "name": "bean_curd"}, {"id": 76, "synset": "beanbag.n.01", "synonyms": ["beanbag"], "def": "a bag filled with dried beans or similar items; used in games or to sit on", "name": "beanbag"}, {"id": 77, "synset": "beanie.n.01", "synonyms": ["beanie", "beany"], "def": "a small skullcap; formerly worn by schoolboys and college freshmen", "name": "beanie"}, {"id": 78, "synset": "bear.n.01", "synonyms": ["bear"], "def": "large carnivorous or omnivorous mammals with shaggy coats and claws", "name": "bear"}, {"id": 79, "synset": "bed.n.01", "synonyms": ["bed"], "def": "a piece of furniture that provides a place to sleep", "name": "bed"}, {"id": 80, "synset": "bedspread.n.01", "synonyms": ["bedspread", "bedcover", "bed_covering", "counterpane", "spread"], "def": "decorative cover for a bed", "name": "bedspread"}, {"id": 81, "synset": "beef.n.01", "synonyms": ["cow"], "def": "cattle that are reared for their meat", "name": "cow"}, {"id": 82, "synset": "beef.n.02", "synonyms": ["beef_(food)", "boeuf_(food)"], "def": "meat from an adult domestic bovine", "name": "beef_(food)"}, {"id": 83, "synset": "beeper.n.01", "synonyms": ["beeper", "pager"], "def": "an device that beeps when the person carrying it is being paged", "name": "beeper"}, {"id": 84, "synset": "beer_bottle.n.01", "synonyms": ["beer_bottle"], "def": "a bottle that holds beer", "name": "beer_bottle"}, {"id": 85, "synset": "beer_can.n.01", "synonyms": ["beer_can"], "def": "a can that holds beer", "name": "beer_can"}, {"id": 86, "synset": "beetle.n.01", "synonyms": ["beetle"], "def": "insect with hard wing covers", "name": "beetle"}, {"id": 87, "synset": "bell.n.01", "synonyms": ["bell"], "def": "a hollow device made of metal that makes a ringing sound when struck", "name": "bell"}, {"id": 88, "synset": "bell_pepper.n.02", "synonyms": ["bell_pepper", "capsicum"], "def": "large bell-shaped sweet pepper in green or red or yellow or orange or black varieties", "name": "bell_pepper"}, {"id": 89, "synset": "belt.n.02", "synonyms": ["belt"], "def": "a band to tie or buckle around the body (usually at the waist)", "name": "belt"}, {"id": 90, "synset": "belt_buckle.n.01", "synonyms": ["belt_buckle"], "def": "the buckle used to fasten a belt", "name": "belt_buckle"}, {"id": 91, "synset": "bench.n.01", "synonyms": ["bench"], "def": "a long seat for more than one person", "name": "bench"}, {"id": 92, "synset": "beret.n.01", "synonyms": ["beret"], "def": "a cap with no brim or bill; made of soft cloth", "name": "beret"}, {"id": 93, "synset": "bib.n.02", "synonyms": ["bib"], "def": "a napkin tied under the chin of a child while eating", "name": "bib"}, {"id": 94, "synset": "bible.n.01", "synonyms": ["Bible"], "def": "the sacred writings of the Christian religions", "name": "Bible"}, {"id": 95, "synset": "bicycle.n.01", "synonyms": ["bicycle", "bike_(bicycle)"], "def": "a wheeled vehicle that has two wheels and is moved by foot pedals", "name": "bicycle"}, {"id": 96, "synset": "bill.n.09", "synonyms": ["visor", "vizor"], "def": "a brim that projects to the front to shade the eyes", "name": "visor"}, {"id": 97, "synset": "binder.n.03", "synonyms": ["binder", "ring-binder"], "def": "holds loose papers or magazines", "name": "binder"}, {"id": 98, "synset": "binoculars.n.01", "synonyms": ["binoculars", "field_glasses", "opera_glasses"], "def": "an optical instrument designed for simultaneous use by both eyes", "name": "binoculars"}, {"id": 99, "synset": "bird.n.01", "synonyms": ["bird"], "def": "animal characterized by feathers and wings", "name": "bird"}, {"id": 100, "synset": "bird_feeder.n.01", "synonyms": ["birdfeeder"], "def": "an outdoor device that supplies food for wild birds", "name": "birdfeeder"}, {"id": 101, "synset": "birdbath.n.01", "synonyms": ["birdbath"], "def": "an ornamental basin (usually in a garden) for birds to bathe in", "name": "birdbath"}, {"id": 102, "synset": "birdcage.n.01", "synonyms": ["birdcage"], "def": "a cage in which a bird can be kept", "name": "birdcage"}, {"id": 103, "synset": "birdhouse.n.01", "synonyms": ["birdhouse"], "def": "a shelter for birds", "name": "birdhouse"}, {"id": 104, "synset": "birthday_cake.n.01", "synonyms": ["birthday_cake"], "def": "decorated cake served at a birthday party", "name": "birthday_cake"}, {"id": 105, "synset": "birthday_card.n.01", "synonyms": ["birthday_card"], "def": "a card expressing a birthday greeting", "name": "birthday_card"}, {"id": 106, "synset": "biscuit.n.01", "synonyms": ["biscuit_(bread)"], "def": "small round bread leavened with baking-powder or soda", "name": "biscuit_(bread)"}, {"id": 107, "synset": "black_flag.n.01", "synonyms": ["pirate_flag"], "def": "a flag usually bearing a white skull and crossbones on a black background", "name": "pirate_flag"}, {"id": 108, "synset": "black_sheep.n.02", "synonyms": ["black_sheep"], "def": "sheep with a black coat", "name": "black_sheep"}, {"id": 109, "synset": "blackboard.n.01", "synonyms": ["blackboard", "chalkboard"], "def": "sheet of slate; for writing with chalk", "name": "blackboard"}, {"id": 110, "synset": "blanket.n.01", "synonyms": ["blanket"], "def": "bedding that keeps a person warm in bed", "name": "blanket"}, {"id": 111, "synset": "blazer.n.01", "synonyms": ["blazer", "sport_jacket", "sport_coat", "sports_jacket", "sports_coat"], "def": "lightweight jacket; often striped in the colors of a club or school", "name": "blazer"}, {"id": 112, "synset": "blender.n.01", "synonyms": ["blender", "liquidizer", "liquidiser"], "def": "an electrically powered mixer that mix or chop or liquefy foods", "name": "blender"}, {"id": 113, "synset": "blimp.n.02", "synonyms": ["blimp"], "def": "a small nonrigid airship used for observation or as a barrage balloon", "name": "blimp"}, {"id": 114, "synset": "blinker.n.01", "synonyms": ["blinker", "flasher"], "def": "a light that flashes on and off; used as a signal or to send messages", "name": "blinker"}, {"id": 115, "synset": "blueberry.n.02", "synonyms": ["blueberry"], "def": "sweet edible dark-blue berries of blueberry plants", "name": "blueberry"}, {"id": 116, "synset": "boar.n.02", "synonyms": ["boar"], "def": "an uncastrated male hog", "name": "boar"}, {"id": 117, "synset": "board.n.09", "synonyms": ["gameboard"], "def": "a flat portable surface (usually rectangular) designed for board games", "name": "gameboard"}, {"id": 118, "synset": "boat.n.01", "synonyms": ["boat", "ship_(boat)"], "def": "a vessel for travel on water", "name": "boat"}, {"id": 119, "synset": "bobbin.n.01", "synonyms": ["bobbin", "spool", "reel"], "def": "a thing around which thread/tape/film or other flexible materials can be wound", "name": "bobbin"}, {"id": 120, "synset": "bobby_pin.n.01", "synonyms": ["bobby_pin", "hairgrip"], "def": "a flat wire hairpin used to hold bobbed hair in place", "name": "bobby_pin"}, {"id": 121, "synset": "boiled_egg.n.01", "synonyms": ["boiled_egg", "coddled_egg"], "def": "egg cooked briefly in the shell in gently boiling water", "name": "boiled_egg"}, {"id": 122, "synset": "bolo_tie.n.01", "synonyms": ["bolo_tie", "bolo", "bola_tie", "bola"], "def": "a cord fastened around the neck with an ornamental clasp and worn as a necktie", "name": "bolo_tie"}, {"id": 123, "synset": "bolt.n.03", "synonyms": ["deadbolt"], "def": "the part of a lock that is engaged or withdrawn with a key", "name": "deadbolt"}, {"id": 124, "synset": "bolt.n.06", "synonyms": ["bolt"], "def": "a screw that screws into a nut to form a fastener", "name": "bolt"}, {"id": 125, "synset": "bonnet.n.01", "synonyms": ["bonnet"], "def": "a hat tied under the chin", "name": "bonnet"}, {"id": 126, "synset": "book.n.01", "synonyms": ["book"], "def": "a written work or composition that has been published", "name": "book"}, {"id": 127, "synset": "book_bag.n.01", "synonyms": ["book_bag"], "def": "a bag in which students carry their books", "name": "book_bag"}, {"id": 128, "synset": "bookcase.n.01", "synonyms": ["bookcase"], "def": "a piece of furniture with shelves for storing books", "name": "bookcase"}, {"id": 129, "synset": "booklet.n.01", "synonyms": ["booklet", "brochure", "leaflet", "pamphlet"], "def": "a small book usually having a paper cover", "name": "booklet"}, {"id": 130, "synset": "bookmark.n.01", "synonyms": ["bookmark", "bookmarker"], "def": "a marker (a piece of paper or ribbon) placed between the pages of a book", "name": "bookmark"}, {"id": 131, "synset": "boom.n.04", "synonyms": ["boom_microphone", "microphone_boom"], "def": "a pole carrying an overhead microphone projected over a film or tv set", "name": "boom_microphone"}, {"id": 132, "synset": "boot.n.01", "synonyms": ["boot"], "def": "footwear that covers the whole foot and lower leg", "name": "boot"}, {"id": 133, "synset": "bottle.n.01", "synonyms": ["bottle"], "def": "a glass or plastic vessel used for storing drinks or other liquids", "name": "bottle"}, {"id": 134, "synset": "bottle_opener.n.01", "synonyms": ["bottle_opener"], "def": "an opener for removing caps or corks from bottles", "name": "bottle_opener"}, {"id": 135, "synset": "bouquet.n.01", "synonyms": ["bouquet"], "def": "an arrangement of flowers that is usually given as a present", "name": "bouquet"}, {"id": 136, "synset": "bow.n.04", "synonyms": ["bow_(weapon)"], "def": "a weapon for shooting arrows", "name": "bow_(weapon)"}, {"id": 137, "synset": "bow.n.08", "synonyms": ["bow_(decorative_ribbons)"], "def": "a decorative interlacing of ribbons", "name": "bow_(decorative_ribbons)"}, {"id": 138, "synset": "bow_tie.n.01", "synonyms": ["bow-tie", "bowtie"], "def": "a man's tie that ties in a bow", "name": "bow-tie"}, {"id": 139, "synset": "bowl.n.03", "synonyms": ["bowl"], "def": "a dish that is round and open at the top for serving foods", "name": "bowl"}, {"id": 140, "synset": "bowl.n.08", "synonyms": ["pipe_bowl"], "def": "a small round container that is open at the top for holding tobacco", "name": "pipe_bowl"}, {"id": 141, "synset": "bowler_hat.n.01", "synonyms": ["bowler_hat", "bowler", "derby_hat", "derby", "plug_hat"], "def": "a felt hat that is round and hard with a narrow brim", "name": "bowler_hat"}, {"id": 142, "synset": "bowling_ball.n.01", "synonyms": ["bowling_ball"], "def": "a large ball with finger holes used in the sport of bowling", "name": "bowling_ball"}, {"id": 143, "synset": "bowling_pin.n.01", "synonyms": ["bowling_pin"], "def": "a club-shaped wooden object used in bowling", "name": "bowling_pin"}, {"id": 144, "synset": "boxing_glove.n.01", "synonyms": ["boxing_glove"], "def": "large glove coverings the fists of a fighter worn for the sport of boxing", "name": "boxing_glove"}, {"id": 145, "synset": "brace.n.06", "synonyms": ["suspenders"], "def": "elastic straps that hold trousers up (usually used in the plural)", "name": "suspenders"}, {"id": 146, "synset": "bracelet.n.02", "synonyms": ["bracelet", "bangle"], "def": "jewelry worn around the wrist for decoration", "name": "bracelet"}, {"id": 147, "synset": "brass.n.07", "synonyms": ["brass_plaque"], "def": "a memorial made of brass", "name": "brass_plaque"}, {"id": 148, "synset": "brassiere.n.01", "synonyms": ["brassiere", "bra", "bandeau"], "def": "an undergarment worn by women to support their breasts", "name": "brassiere"}, {"id": 149, "synset": "bread-bin.n.01", "synonyms": ["bread-bin", "breadbox"], "def": "a container used to keep bread or cake in", "name": "bread-bin"}, {"id": 150, "synset": "breechcloth.n.01", "synonyms": ["breechcloth", "breechclout", "loincloth"], "def": "a garment that provides covering for the loins", "name": "breechcloth"}, {"id": 151, "synset": "bridal_gown.n.01", "synonyms": ["bridal_gown", "wedding_gown", "wedding_dress"], "def": "a gown worn by the bride at a wedding", "name": "bridal_gown"}, {"id": 152, "synset": "briefcase.n.01", "synonyms": ["briefcase"], "def": "a case with a handle; for carrying papers or files or books", "name": "briefcase"}, {"id": 153, "synset": "bristle_brush.n.01", "synonyms": ["bristle_brush"], "def": "a brush that is made with the short stiff hairs of an animal or plant", "name": "bristle_brush"}, {"id": 154, "synset": "broccoli.n.01", "synonyms": ["broccoli"], "def": "plant with dense clusters of tight green flower buds", "name": "broccoli"}, {"id": 155, "synset": "brooch.n.01", "synonyms": ["broach"], "def": "a decorative pin worn by women", "name": "broach"}, {"id": 156, "synset": "broom.n.01", "synonyms": ["broom"], "def": "bundle of straws or twigs attached to a long handle; used for cleaning", "name": "broom"}, {"id": 157, "synset": "brownie.n.03", "synonyms": ["brownie"], "def": "square or bar of very rich chocolate cake usually with nuts", "name": "brownie"}, {"id": 158, "synset": "brussels_sprouts.n.01", "synonyms": ["brussels_sprouts"], "def": "the small edible cabbage-like buds growing along a stalk", "name": "brussels_sprouts"}, {"id": 159, "synset": "bubble_gum.n.01", "synonyms": ["bubble_gum"], "def": "a kind of chewing gum that can be blown into bubbles", "name": "bubble_gum"}, {"id": 160, "synset": "bucket.n.01", "synonyms": ["bucket", "pail"], "def": "a roughly cylindrical vessel that is open at the top", "name": "bucket"}, {"id": 161, "synset": "buggy.n.01", "synonyms": ["horse_buggy"], "def": "a small lightweight carriage; drawn by a single horse", "name": "horse_buggy"}, {"id": 162, "synset": "bull.n.11", "synonyms": ["bull"], "def": "mature male cow", "name": "bull"}, {"id": 163, "synset": "bulldog.n.01", "synonyms": ["bulldog"], "def": "a thickset short-haired dog with a large head and strong undershot lower jaw", "name": "bulldog"}, {"id": 164, "synset": "bulldozer.n.01", "synonyms": ["bulldozer", "dozer"], "def": "large powerful tractor; a large blade in front flattens areas of ground", "name": "bulldozer"}, {"id": 165, "synset": "bullet_train.n.01", "synonyms": ["bullet_train"], "def": "a high-speed passenger train", "name": "bullet_train"}, {"id": 166, "synset": "bulletin_board.n.02", "synonyms": ["bulletin_board", "notice_board"], "def": "a board that hangs on a wall; displays announcements", "name": "bulletin_board"}, {"id": 167, "synset": "bulletproof_vest.n.01", "synonyms": ["bulletproof_vest"], "def": "a vest capable of resisting the impact of a bullet", "name": "bulletproof_vest"}, {"id": 168, "synset": "bullhorn.n.01", "synonyms": ["bullhorn", "megaphone"], "def": "a portable loudspeaker with built-in microphone and amplifier", "name": "bullhorn"}, {"id": 169, "synset": "bully_beef.n.01", "synonyms": ["corned_beef", "corn_beef"], "def": "beef cured or pickled in brine", "name": "corned_beef"}, {"id": 170, "synset": "bun.n.01", "synonyms": ["bun", "roll"], "def": "small rounded bread either plain or sweet", "name": "bun"}, {"id": 171, "synset": "bunk_bed.n.01", "synonyms": ["bunk_bed"], "def": "beds built one above the other", "name": "bunk_bed"}, {"id": 172, "synset": "buoy.n.01", "synonyms": ["buoy"], "def": "a float attached by rope to the seabed to mark channels in a harbor or underwater hazards", "name": "buoy"}, {"id": 173, "synset": "burrito.n.01", "synonyms": ["burrito"], "def": "a flour tortilla folded around a filling", "name": "burrito"}, {"id": 174, "synset": "bus.n.01", "synonyms": ["bus_(vehicle)", "autobus", "charabanc", "double-decker", "motorbus", "motorcoach"], "def": "a vehicle carrying many passengers; used for public transport", "name": "bus_(vehicle)"}, {"id": 175, "synset": "business_card.n.01", "synonyms": ["business_card"], "def": "a card on which are printed the person's name and business affiliation", "name": "business_card"}, {"id": 176, "synset": "butcher_knife.n.01", "synonyms": ["butcher_knife"], "def": "a large sharp knife for cutting or trimming meat", "name": "butcher_knife"}, {"id": 177, "synset": "butter.n.01", "synonyms": ["butter"], "def": "an edible emulsion of fat globules made by churning milk or cream; for cooking and table use", "name": "butter"}, {"id": 178, "synset": "butterfly.n.01", "synonyms": ["butterfly"], "def": "insect typically having a slender body with knobbed antennae and broad colorful wings", "name": "butterfly"}, {"id": 179, "synset": "button.n.01", "synonyms": ["button"], "def": "a round fastener sewn to shirts and coats etc to fit through buttonholes", "name": "button"}, {"id": 180, "synset": "cab.n.03", "synonyms": ["cab_(taxi)", "taxi", "taxicab"], "def": "a car that takes passengers where they want to go in exchange for money", "name": "cab_(taxi)"}, {"id": 181, "synset": "cabana.n.01", "synonyms": ["cabana"], "def": "a small tent used as a dressing room beside the sea or a swimming pool", "name": "cabana"}, {"id": 182, "synset": "cabin_car.n.01", "synonyms": ["cabin_car", "caboose"], "def": "a car on a freight train for use of the train crew; usually the last car on the train", "name": "cabin_car"}, {"id": 183, "synset": "cabinet.n.01", "synonyms": ["cabinet"], "def": "a piece of furniture resembling a cupboard with doors and shelves and drawers", "name": "cabinet"}, {"id": 184, "synset": "cabinet.n.03", "synonyms": ["locker", "storage_locker"], "def": "a storage compartment for clothes and valuables; usually it has a lock", "name": "locker"}, {"id": 185, "synset": "cake.n.03", "synonyms": ["cake"], "def": "baked goods made from or based on a mixture of flour, sugar, eggs, and fat", "name": "cake"}, {"id": 186, "synset": "calculator.n.02", "synonyms": ["calculator"], "def": "a small machine that is used for mathematical calculations", "name": "calculator"}, {"id": 187, "synset": "calendar.n.02", "synonyms": ["calendar"], "def": "a list or register of events (appointments/social events/court cases, etc)", "name": "calendar"}, {"id": 188, "synset": "calf.n.01", "synonyms": ["calf"], "def": "young of domestic cattle", "name": "calf"}, {"id": 189, "synset": "camcorder.n.01", "synonyms": ["camcorder"], "def": "a portable television camera and videocassette recorder", "name": "camcorder"}, {"id": 190, "synset": "camel.n.01", "synonyms": ["camel"], "def": "cud-chewing mammal used as a draft or saddle animal in desert regions", "name": "camel"}, {"id": 191, "synset": "camera.n.01", "synonyms": ["camera"], "def": "equipment for taking photographs", "name": "camera"}, {"id": 192, "synset": "camera_lens.n.01", "synonyms": ["camera_lens"], "def": "a lens that focuses the image in a camera", "name": "camera_lens"}, {"id": 193, "synset": "camper.n.02", "synonyms": ["camper_(vehicle)", "camping_bus", "motor_home"], "def": "a recreational vehicle equipped for camping out while traveling", "name": "camper_(vehicle)"}, {"id": 194, "synset": "can.n.01", "synonyms": ["can", "tin_can"], "def": "airtight sealed metal container for food or drink or paint etc.", "name": "can"}, {"id": 195, "synset": "can_opener.n.01", "synonyms": ["can_opener", "tin_opener"], "def": "a device for cutting cans open", "name": "can_opener"}, {"id": 196, "synset": "candelabrum.n.01", "synonyms": ["candelabrum", "candelabra"], "def": "branched candlestick; ornamental; has several lights", "name": "candelabrum"}, {"id": 197, "synset": "candle.n.01", "synonyms": ["candle", "candlestick"], "def": "stick of wax with a wick in the middle", "name": "candle"}, {"id": 198, "synset": "candlestick.n.01", "synonyms": ["candle_holder"], "def": "a holder with sockets for candles", "name": "candle_holder"}, {"id": 199, "synset": "candy_bar.n.01", "synonyms": ["candy_bar"], "def": "a candy shaped as a bar", "name": "candy_bar"}, {"id": 200, "synset": "candy_cane.n.01", "synonyms": ["candy_cane"], "def": "a hard candy in the shape of a rod (usually with stripes)", "name": "candy_cane"}, {"id": 201, "synset": "cane.n.01", "synonyms": ["walking_cane"], "def": "a stick that people can lean on to help them walk", "name": "walking_cane"}, {"id": 202, "synset": "canister.n.02", "synonyms": ["canister", "cannister"], "def": "metal container for storing dry foods such as tea or flour", "name": "canister"}, {"id": 203, "synset": "cannon.n.02", "synonyms": ["cannon"], "def": "heavy gun fired from a tank", "name": "cannon"}, {"id": 204, "synset": "canoe.n.01", "synonyms": ["canoe"], "def": "small and light boat; pointed at both ends; propelled with a paddle", "name": "canoe"}, {"id": 205, "synset": "cantaloup.n.02", "synonyms": ["cantaloup", "cantaloupe"], "def": "the fruit of a cantaloup vine; small to medium-sized melon with yellowish flesh", "name": "cantaloup"}, {"id": 206, "synset": "canteen.n.01", "synonyms": ["canteen"], "def": "a flask for carrying water; used by soldiers or travelers", "name": "canteen"}, {"id": 207, "synset": "cap.n.01", "synonyms": ["cap_(headwear)"], "def": "a tight-fitting headwear", "name": "cap_(headwear)"}, {"id": 208, "synset": "cap.n.02", "synonyms": ["bottle_cap", "cap_(container_lid)"], "def": "a top (as for a bottle)", "name": "bottle_cap"}, {"id": 209, "synset": "cape.n.02", "synonyms": ["cape"], "def": "a sleeveless garment like a cloak but shorter", "name": "cape"}, {"id": 210, "synset": "cappuccino.n.01", "synonyms": ["cappuccino", "coffee_cappuccino"], "def": "equal parts of espresso and steamed milk", "name": "cappuccino"}, {"id": 211, "synset": "car.n.01", "synonyms": ["car_(automobile)", "auto_(automobile)", "automobile"], "def": "a motor vehicle with four wheels", "name": "car_(automobile)"}, {"id": 212, "synset": "car.n.02", "synonyms": ["railcar_(part_of_a_train)", "railway_car_(part_of_a_train)", "railroad_car_(part_of_a_train)"], "def": "a wheeled vehicle adapted to the rails of railroad", "name": "railcar_(part_of_a_train)"}, {"id": 213, "synset": "car.n.04", "synonyms": ["elevator_car"], "def": "where passengers ride up and down", "name": "elevator_car"}, {"id": 214, "synset": "car_battery.n.01", "synonyms": ["car_battery", "automobile_battery"], "def": "a battery in a motor vehicle", "name": "car_battery"}, {"id": 215, "synset": "card.n.02", "synonyms": ["identity_card"], "def": "a card certifying the identity of the bearer", "name": "identity_card"}, {"id": 216, "synset": "card.n.03", "synonyms": ["card"], "def": "a rectangular piece of paper used to send messages (e.g. greetings or pictures)", "name": "card"}, {"id": 217, "synset": "cardigan.n.01", "synonyms": ["cardigan"], "def": "knitted jacket that is fastened up the front with buttons or a zipper", "name": "cardigan"}, {"id": 218, "synset": "cargo_ship.n.01", "synonyms": ["cargo_ship", "cargo_vessel"], "def": "a ship designed to carry cargo", "name": "cargo_ship"}, {"id": 219, "synset": "carnation.n.01", "synonyms": ["carnation"], "def": "plant with pink to purple-red spice-scented usually double flowers", "name": "carnation"}, {"id": 220, "synset": "carriage.n.02", "synonyms": ["horse_carriage"], "def": "a vehicle with wheels drawn by one or more horses", "name": "horse_carriage"}, {"id": 221, "synset": "carrot.n.01", "synonyms": ["carrot"], "def": "deep orange edible root of the cultivated carrot plant", "name": "carrot"}, {"id": 222, "synset": "carryall.n.01", "synonyms": ["tote_bag"], "def": "a capacious bag or basket", "name": "tote_bag"}, {"id": 223, "synset": "cart.n.01", "synonyms": ["cart"], "def": "a heavy open wagon usually having two wheels and drawn by an animal", "name": "cart"}, {"id": 224, "synset": "carton.n.02", "synonyms": ["carton"], "def": "a box made of cardboard; opens by flaps on top", "name": "carton"}, {"id": 225, "synset": "cash_register.n.01", "synonyms": ["cash_register", "register_(for_cash_transactions)"], "def": "a cashbox with an adding machine to register transactions", "name": "cash_register"}, {"id": 226, "synset": "casserole.n.01", "synonyms": ["casserole"], "def": "food cooked and served in a casserole", "name": "casserole"}, {"id": 227, "synset": "cassette.n.01", "synonyms": ["cassette"], "def": "a container that holds a magnetic tape used for recording or playing sound or video", "name": "cassette"}, {"id": 228, "synset": "cast.n.05", "synonyms": ["cast", "plaster_cast", "plaster_bandage"], "def": "bandage consisting of a firm covering that immobilizes broken bones while they heal", "name": "cast"}, {"id": 229, "synset": "cat.n.01", "synonyms": ["cat"], "def": "a domestic house cat", "name": "cat"}, {"id": 230, "synset": "cauliflower.n.02", "synonyms": ["cauliflower"], "def": "edible compact head of white undeveloped flowers", "name": "cauliflower"}, {"id": 231, "synset": "caviar.n.01", "synonyms": ["caviar", "caviare"], "def": "salted roe of sturgeon or other large fish; usually served as an hors d'oeuvre", "name": "caviar"}, {"id": 232, "synset": "cayenne.n.02", "synonyms": ["cayenne_(spice)", "cayenne_pepper_(spice)", "red_pepper_(spice)"], "def": "ground pods and seeds of pungent red peppers of the genus Capsicum", "name": "cayenne_(spice)"}, {"id": 233, "synset": "cd_player.n.01", "synonyms": ["CD_player"], "def": "electronic equipment for playing compact discs (CDs)", "name": "CD_player"}, {"id": 234, "synset": "celery.n.01", "synonyms": ["celery"], "def": "widely cultivated herb with aromatic leaf stalks that are eaten raw or cooked", "name": "celery"}, {"id": 235, "synset": "cellular_telephone.n.01", "synonyms": ["cellular_telephone", "cellular_phone", "cellphone", "mobile_phone", "smart_phone"], "def": "a hand-held mobile telephone", "name": "cellular_telephone"}, {"id": 236, "synset": "chain_mail.n.01", "synonyms": ["chain_mail", "ring_mail", "chain_armor", "chain_armour", "ring_armor", "ring_armour"], "def": "(Middle Ages) flexible armor made of interlinked metal rings", "name": "chain_mail"}, {"id": 237, "synset": "chair.n.01", "synonyms": ["chair"], "def": "a seat for one person, with a support for the back", "name": "chair"}, {"id": 238, "synset": "chaise_longue.n.01", "synonyms": ["chaise_longue", "chaise", "daybed"], "def": "a long chair; for reclining", "name": "chaise_longue"}, {"id": 239, "synset": "champagne.n.01", "synonyms": ["champagne"], "def": "a white sparkling wine produced in Champagne or resembling that produced there", "name": "champagne"}, {"id": 240, "synset": "chandelier.n.01", "synonyms": ["chandelier"], "def": "branched lighting fixture; often ornate; hangs from the ceiling", "name": "chandelier"}, {"id": 241, "synset": "chap.n.04", "synonyms": ["chap"], "def": "leather leggings without a seat; worn over trousers by cowboys to protect their legs", "name": "chap"}, {"id": 242, "synset": "checkbook.n.01", "synonyms": ["checkbook", "chequebook"], "def": "a book issued to holders of checking accounts", "name": "checkbook"}, {"id": 243, "synset": "checkerboard.n.01", "synonyms": ["checkerboard"], "def": "a board having 64 squares of two alternating colors", "name": "checkerboard"}, {"id": 244, "synset": "cherry.n.03", "synonyms": ["cherry"], "def": "a red fruit with a single hard stone", "name": "cherry"}, {"id": 245, "synset": "chessboard.n.01", "synonyms": ["chessboard"], "def": "a checkerboard used to play chess", "name": "chessboard"}, {"id": 246, "synset": "chest_of_drawers.n.01", "synonyms": ["chest_of_drawers_(furniture)", "bureau_(furniture)", "chest_(furniture)"], "def": "furniture with drawers for keeping clothes", "name": "chest_of_drawers_(furniture)"}, {"id": 247, "synset": "chicken.n.02", "synonyms": ["chicken_(animal)"], "def": "a domestic fowl bred for flesh or eggs", "name": "chicken_(animal)"}, {"id": 248, "synset": "chicken_wire.n.01", "synonyms": ["chicken_wire"], "def": "a galvanized wire network with a hexagonal mesh; used to build fences", "name": "chicken_wire"}, {"id": 249, "synset": "chickpea.n.01", "synonyms": ["chickpea", "garbanzo"], "def": "the seed of the chickpea plant; usually dried", "name": "chickpea"}, {"id": 250, "synset": "chihuahua.n.03", "synonyms": ["Chihuahua"], "def": "an old breed of tiny short-haired dog with protruding eyes from Mexico", "name": "Chihuahua"}, {"id": 251, "synset": "chili.n.02", "synonyms": ["chili_(vegetable)", "chili_pepper_(vegetable)", "chilli_(vegetable)", "chilly_(vegetable)", "chile_(vegetable)"], "def": "very hot and finely tapering pepper of special pungency", "name": "chili_(vegetable)"}, {"id": 252, "synset": "chime.n.01", "synonyms": ["chime", "gong"], "def": "an instrument consisting of a set of bells that are struck with a hammer", "name": "chime"}, {"id": 253, "synset": "chinaware.n.01", "synonyms": ["chinaware"], "def": "dishware made of high quality porcelain", "name": "chinaware"}, {"id": 254, "synset": "chip.n.04", "synonyms": ["crisp_(potato_chip)", "potato_chip"], "def": "a thin crisp slice of potato fried in deep fat", "name": "crisp_(potato_chip)"}, {"id": 255, "synset": "chip.n.06", "synonyms": ["poker_chip"], "def": "a small disk-shaped counter used to represent money when gambling", "name": "poker_chip"}, {"id": 256, "synset": "chocolate_bar.n.01", "synonyms": ["chocolate_bar"], "def": "a bar of chocolate candy", "name": "chocolate_bar"}, {"id": 257, "synset": "chocolate_cake.n.01", "synonyms": ["chocolate_cake"], "def": "cake containing chocolate", "name": "chocolate_cake"}, {"id": 258, "synset": "chocolate_milk.n.01", "synonyms": ["chocolate_milk"], "def": "milk flavored with chocolate syrup", "name": "chocolate_milk"}, {"id": 259, "synset": "chocolate_mousse.n.01", "synonyms": ["chocolate_mousse"], "def": "dessert mousse made with chocolate", "name": "chocolate_mousse"}, {"id": 260, "synset": "choker.n.03", "synonyms": ["choker", "collar", "neckband"], "def": "necklace that fits tightly around the neck", "name": "choker"}, {"id": 261, "synset": "chopping_board.n.01", "synonyms": ["chopping_board", "cutting_board", "chopping_block"], "def": "a wooden board where meats or vegetables can be cut", "name": "chopping_board"}, {"id": 262, "synset": "chopstick.n.01", "synonyms": ["chopstick"], "def": "one of a pair of slender sticks used as oriental tableware to eat food with", "name": "chopstick"}, {"id": 263, "synset": "christmas_tree.n.05", "synonyms": ["Christmas_tree"], "def": "an ornamented evergreen used as a Christmas decoration", "name": "Christmas_tree"}, {"id": 264, "synset": "chute.n.02", "synonyms": ["slide"], "def": "sloping channel through which things can descend", "name": "slide"}, {"id": 265, "synset": "cider.n.01", "synonyms": ["cider", "cyder"], "def": "a beverage made from juice pressed from apples", "name": "cider"}, {"id": 266, "synset": "cigar_box.n.01", "synonyms": ["cigar_box"], "def": "a box for holding cigars", "name": "cigar_box"}, {"id": 267, "synset": "cigarette.n.01", "synonyms": ["cigarette"], "def": "finely ground tobacco wrapped in paper; for smoking", "name": "cigarette"}, {"id": 268, "synset": "cigarette_case.n.01", "synonyms": ["cigarette_case", "cigarette_pack"], "def": "a small flat case for holding cigarettes", "name": "cigarette_case"}, {"id": 269, "synset": "cistern.n.02", "synonyms": ["cistern", "water_tank"], "def": "a tank that holds the water used to flush a toilet", "name": "cistern"}, {"id": 270, "synset": "clarinet.n.01", "synonyms": ["clarinet"], "def": "a single-reed instrument with a straight tube", "name": "clarinet"}, {"id": 271, "synset": "clasp.n.01", "synonyms": ["clasp"], "def": "a fastener (as a buckle or hook) that is used to hold two things together", "name": "clasp"}, {"id": 272, "synset": "cleansing_agent.n.01", "synonyms": ["cleansing_agent", "cleanser", "cleaner"], "def": "a preparation used in cleaning something", "name": "cleansing_agent"}, {"id": 273, "synset": "clementine.n.01", "synonyms": ["clementine"], "def": "a variety of mandarin orange", "name": "clementine"}, {"id": 274, "synset": "clip.n.03", "synonyms": ["clip"], "def": "any of various small fasteners used to hold loose articles together", "name": "clip"}, {"id": 275, "synset": "clipboard.n.01", "synonyms": ["clipboard"], "def": "a small writing board with a clip at the top for holding papers", "name": "clipboard"}, {"id": 276, "synset": "clock.n.01", "synonyms": ["clock", "timepiece", "timekeeper"], "def": "a timepiece that shows the time of day", "name": "clock"}, {"id": 277, "synset": "clock_tower.n.01", "synonyms": ["clock_tower"], "def": "a tower with a large clock visible high up on an outside face", "name": "clock_tower"}, {"id": 278, "synset": "clothes_hamper.n.01", "synonyms": ["clothes_hamper", "laundry_basket", "clothes_basket"], "def": "a hamper that holds dirty clothes to be washed or wet clothes to be dried", "name": "clothes_hamper"}, {"id": 279, "synset": "clothespin.n.01", "synonyms": ["clothespin", "clothes_peg"], "def": "wood or plastic fastener; for holding clothes on a clothesline", "name": "clothespin"}, {"id": 280, "synset": "clutch_bag.n.01", "synonyms": ["clutch_bag"], "def": "a woman's strapless purse that is carried in the hand", "name": "clutch_bag"}, {"id": 281, "synset": "coaster.n.03", "synonyms": ["coaster"], "def": "a covering (plate or mat) that protects the surface of a table", "name": "coaster"}, {"id": 282, "synset": "coat.n.01", "synonyms": ["coat"], "def": "an outer garment that has sleeves and covers the body from shoulder down", "name": "coat"}, {"id": 283, "synset": "coat_hanger.n.01", "synonyms": ["coat_hanger", "clothes_hanger", "dress_hanger"], "def": "a hanger that is shaped like a person's shoulders", "name": "coat_hanger"}, {"id": 284, "synset": "coatrack.n.01", "synonyms": ["coatrack", "hatrack"], "def": "a rack with hooks for temporarily holding coats and hats", "name": "coatrack"}, {"id": 285, "synset": "cock.n.04", "synonyms": ["cock", "rooster"], "def": "adult male chicken", "name": "cock"}, {"id": 286, "synset": "coconut.n.02", "synonyms": ["coconut", "cocoanut"], "def": "large hard-shelled brown oval nut with a fibrous husk", "name": "coconut"}, {"id": 287, "synset": "coffee_filter.n.01", "synonyms": ["coffee_filter"], "def": "filter (usually of paper) that passes the coffee and retains the coffee grounds", "name": "coffee_filter"}, {"id": 288, "synset": "coffee_maker.n.01", "synonyms": ["coffee_maker", "coffee_machine"], "def": "a kitchen appliance for brewing coffee automatically", "name": "coffee_maker"}, {"id": 289, "synset": "coffee_table.n.01", "synonyms": ["coffee_table", "cocktail_table"], "def": "low table where magazines can be placed and coffee or cocktails are served", "name": "coffee_table"}, {"id": 290, "synset": "coffeepot.n.01", "synonyms": ["coffeepot"], "def": "tall pot in which coffee is brewed", "name": "coffeepot"}, {"id": 291, "synset": "coil.n.05", "synonyms": ["coil"], "def": "tubing that is wound in a spiral", "name": "coil"}, {"id": 292, "synset": "coin.n.01", "synonyms": ["coin"], "def": "a flat metal piece (usually a disc) used as money", "name": "coin"}, {"id": 293, "synset": "colander.n.01", "synonyms": ["colander", "cullender"], "def": "bowl-shaped strainer; used to wash or drain foods", "name": "colander"}, {"id": 294, "synset": "coleslaw.n.01", "synonyms": ["coleslaw", "slaw"], "def": "basically shredded cabbage", "name": "coleslaw"}, {"id": 295, "synset": "coloring_material.n.01", "synonyms": ["coloring_material", "colouring_material"], "def": "any material used for its color", "name": "coloring_material"}, {"id": 296, "synset": "combination_lock.n.01", "synonyms": ["combination_lock"], "def": "lock that can be opened only by turning dials in a special sequence", "name": "combination_lock"}, {"id": 297, "synset": "comforter.n.04", "synonyms": ["pacifier", "teething_ring"], "def": "device used for an infant to suck or bite on", "name": "pacifier"}, {"id": 298, "synset": "comic_book.n.01", "synonyms": ["comic_book"], "def": "a magazine devoted to comic strips", "name": "comic_book"}, {"id": 299, "synset": "computer_keyboard.n.01", "synonyms": ["computer_keyboard", "keyboard_(computer)"], "def": "a keyboard that is a data input device for computers", "name": "computer_keyboard"}, {"id": 300, "synset": "concrete_mixer.n.01", "synonyms": ["concrete_mixer", "cement_mixer"], "def": "a machine with a large revolving drum in which cement/concrete is mixed", "name": "concrete_mixer"}, {"id": 301, "synset": "cone.n.01", "synonyms": ["cone", "traffic_cone"], "def": "a cone-shaped object used to direct traffic", "name": "cone"}, {"id": 302, "synset": "control.n.09", "synonyms": ["control", "controller"], "def": "a mechanism that controls the operation of a machine", "name": "control"}, {"id": 303, "synset": "convertible.n.01", "synonyms": ["convertible_(automobile)"], "def": "a car that has top that can be folded or removed", "name": "convertible_(automobile)"}, {"id": 304, "synset": "convertible.n.03", "synonyms": ["sofa_bed"], "def": "a sofa that can be converted into a bed", "name": "sofa_bed"}, {"id": 305, "synset": "cookie.n.01", "synonyms": ["cookie", "cooky", "biscuit_(cookie)"], "def": "any of various small flat sweet cakes (`biscuit' is the British term)", "name": "cookie"}, {"id": 306, "synset": "cookie_jar.n.01", "synonyms": ["cookie_jar", "cooky_jar"], "def": "a jar in which cookies are kept (and sometimes money is hidden)", "name": "cookie_jar"}, {"id": 307, "synset": "cooking_utensil.n.01", "synonyms": ["cooking_utensil"], "def": "a kitchen utensil made of material that does not melt easily; used for cooking", "name": "cooking_utensil"}, {"id": 308, "synset": "cooler.n.01", "synonyms": ["cooler_(for_food)", "ice_chest"], "def": "an insulated box for storing food often with ice", "name": "cooler_(for_food)"}, {"id": 309, "synset": "cork.n.04", "synonyms": ["cork_(bottle_plug)", "bottle_cork"], "def": "the plug in the mouth of a bottle (especially a wine bottle)", "name": "cork_(bottle_plug)"}, {"id": 310, "synset": "corkboard.n.01", "synonyms": ["corkboard"], "def": "a sheet consisting of cork granules", "name": "corkboard"}, {"id": 311, "synset": "corkscrew.n.01", "synonyms": ["corkscrew", "bottle_screw"], "def": "a bottle opener that pulls corks", "name": "corkscrew"}, {"id": 312, "synset": "corn.n.03", "synonyms": ["edible_corn", "corn", "maize"], "def": "ears of corn that can be prepared and served for human food", "name": "edible_corn"}, {"id": 313, "synset": "cornbread.n.01", "synonyms": ["cornbread"], "def": "bread made primarily of cornmeal", "name": "cornbread"}, {"id": 314, "synset": "cornet.n.01", "synonyms": ["cornet", "horn", "trumpet"], "def": "a brass musical instrument with a narrow tube and a flared bell and many valves", "name": "cornet"}, {"id": 315, "synset": "cornice.n.01", "synonyms": ["cornice", "valance", "valance_board", "pelmet"], "def": "a decorative framework to conceal curtain fixtures at the top of a window casing", "name": "cornice"}, {"id": 316, "synset": "cornmeal.n.01", "synonyms": ["cornmeal"], "def": "coarsely ground corn", "name": "cornmeal"}, {"id": 317, "synset": "corset.n.01", "synonyms": ["corset", "girdle"], "def": "a woman's close-fitting foundation garment", "name": "corset"}, {"id": 318, "synset": "cos.n.02", "synonyms": ["romaine_lettuce"], "def": "lettuce with long dark-green leaves in a loosely packed elongated head", "name": "romaine_lettuce"}, {"id": 319, "synset": "costume.n.04", "synonyms": ["costume"], "def": "the attire characteristic of a country or a time or a social class", "name": "costume"}, {"id": 320, "synset": "cougar.n.01", "synonyms": ["cougar", "puma", "catamount", "mountain_lion", "panther"], "def": "large American feline resembling a lion", "name": "cougar"}, {"id": 321, "synset": "coverall.n.01", "synonyms": ["coverall"], "def": "a loose-fitting protective garment that is worn over other clothing", "name": "coverall"}, {"id": 322, "synset": "cowbell.n.01", "synonyms": ["cowbell"], "def": "a bell hung around the neck of cow so that the cow can be easily located", "name": "cowbell"}, {"id": 323, "synset": "cowboy_hat.n.01", "synonyms": ["cowboy_hat", "ten-gallon_hat"], "def": "a hat with a wide brim and a soft crown; worn by American ranch hands", "name": "cowboy_hat"}, {"id": 324, "synset": "crab.n.01", "synonyms": ["crab_(animal)"], "def": "decapod having eyes on short stalks and a broad flattened shell and pincers", "name": "crab_(animal)"}, {"id": 325, "synset": "cracker.n.01", "synonyms": ["cracker"], "def": "a thin crisp wafer", "name": "cracker"}, {"id": 326, "synset": "crape.n.01", "synonyms": ["crape", "crepe", "French_pancake"], "def": "small very thin pancake", "name": "crape"}, {"id": 327, "synset": "crate.n.01", "synonyms": ["crate"], "def": "a rugged box (usually made of wood); used for shipping", "name": "crate"}, {"id": 328, "synset": "crayon.n.01", "synonyms": ["crayon", "wax_crayon"], "def": "writing or drawing implement made of a colored stick of composition wax", "name": "crayon"}, {"id": 329, "synset": "cream_pitcher.n.01", "synonyms": ["cream_pitcher"], "def": "a small pitcher for serving cream", "name": "cream_pitcher"}, {"id": 330, "synset": "credit_card.n.01", "synonyms": ["credit_card", "charge_card", "debit_card"], "def": "a card, usually plastic, used to pay for goods and services", "name": "credit_card"}, {"id": 331, "synset": "crescent_roll.n.01", "synonyms": ["crescent_roll", "croissant"], "def": "very rich flaky crescent-shaped roll", "name": "crescent_roll"}, {"id": 332, "synset": "crib.n.01", "synonyms": ["crib", "cot"], "def": "baby bed with high sides made of slats", "name": "crib"}, {"id": 333, "synset": "crock.n.03", "synonyms": ["crock_pot", "earthenware_jar"], "def": "an earthen jar (made of baked clay)", "name": "crock_pot"}, {"id": 334, "synset": "crossbar.n.01", "synonyms": ["crossbar"], "def": "a horizontal bar that goes across something", "name": "crossbar"}, {"id": 335, "synset": "crouton.n.01", "synonyms": ["crouton"], "def": "a small piece of toasted or fried bread; served in soup or salads", "name": "crouton"}, {"id": 336, "synset": "crow.n.01", "synonyms": ["crow"], "def": "black birds having a raucous call", "name": "crow"}, {"id": 337, "synset": "crown.n.04", "synonyms": ["crown"], "def": "an ornamental jeweled headdress signifying sovereignty", "name": "crown"}, {"id": 338, "synset": "crucifix.n.01", "synonyms": ["crucifix"], "def": "representation of the cross on which Jesus died", "name": "crucifix"}, {"id": 339, "synset": "cruise_ship.n.01", "synonyms": ["cruise_ship", "cruise_liner"], "def": "a passenger ship used commercially for pleasure cruises", "name": "cruise_ship"}, {"id": 340, "synset": "cruiser.n.01", "synonyms": ["police_cruiser", "patrol_car", "police_car", "squad_car"], "def": "a car in which policemen cruise the streets", "name": "police_cruiser"}, {"id": 341, "synset": "crumb.n.03", "synonyms": ["crumb"], "def": "small piece of e.g. bread or cake", "name": "crumb"}, {"id": 342, "synset": "crutch.n.01", "synonyms": ["crutch"], "def": "a wooden or metal staff that fits under the armpit and reaches to the ground", "name": "crutch"}, {"id": 343, "synset": "cub.n.03", "synonyms": ["cub_(animal)"], "def": "the young of certain carnivorous mammals such as the bear or wolf or lion", "name": "cub_(animal)"}, {"id": 344, "synset": "cube.n.05", "synonyms": ["cube", "square_block"], "def": "a block in the (approximate) shape of a cube", "name": "cube"}, {"id": 345, "synset": "cucumber.n.02", "synonyms": ["cucumber", "cuke"], "def": "cylindrical green fruit with thin green rind and white flesh eaten as a vegetable", "name": "cucumber"}, {"id": 346, "synset": "cufflink.n.01", "synonyms": ["cufflink"], "def": "jewelry consisting of linked buttons used to fasten the cuffs of a shirt", "name": "cufflink"}, {"id": 347, "synset": "cup.n.01", "synonyms": ["cup"], "def": "a small open container usually used for drinking; usually has a handle", "name": "cup", "merged": [{"frequency": "f", "id": 504, "synset": "glass.n.02", "image_count": 92, "instance_count": 313, "synonyms": ["glass_(drink_container)", "drinking_glass"], "def": "a container for holding liquids while drinking", "name": "glass_(drink_container)"}, {"frequency": "f", "id": 720, "synset": "mug.n.04", "image_count": 39, "instance_count": 93, "synonyms": ["mug"], "def": "with handle and usually cylindrical", "name": "mug"}]}, {"id": 348, "synset": "cup.n.08", "synonyms": ["trophy_cup"], "def": "a metal vessel with handles that is awarded as a trophy to a competition winner", "name": "trophy_cup"}, {"id": 349, "synset": "cupcake.n.01", "synonyms": ["cupcake"], "def": "small cake baked in a muffin tin", "name": "cupcake"}, {"id": 350, "synset": "curler.n.01", "synonyms": ["hair_curler", "hair_roller", "hair_crimper"], "def": "a cylindrical tube around which the hair is wound to curl it", "name": "hair_curler"}, {"id": 351, "synset": "curling_iron.n.01", "synonyms": ["curling_iron"], "def": "a cylindrical home appliance that heats hair that has been curled around it", "name": "curling_iron"}, {"id": 352, "synset": "curtain.n.01", "synonyms": ["curtain", "drapery"], "def": "hanging cloth used as a blind (especially for a window)", "name": "curtain"}, {"id": 353, "synset": "cushion.n.03", "synonyms": ["cushion"], "def": "a soft bag filled with air or padding such as feathers or foam rubber", "name": "cushion"}, {"id": 354, "synset": "custard.n.01", "synonyms": ["custard"], "def": "sweetened mixture of milk and eggs baked or boiled or frozen", "name": "custard"}, {"id": 355, "synset": "cutter.n.06", "synonyms": ["cutting_tool"], "def": "a cutting implement; a tool for cutting", "name": "cutting_tool"}, {"id": 356, "synset": "cylinder.n.04", "synonyms": ["cylinder"], "def": "a cylindrical container", "name": "cylinder"}, {"id": 357, "synset": "cymbal.n.01", "synonyms": ["cymbal"], "def": "a percussion instrument consisting of a concave brass disk", "name": "cymbal"}, {"id": 358, "synset": "dachshund.n.01", "synonyms": ["dachshund", "dachsie", "badger_dog"], "def": "small long-bodied short-legged breed of dog having a short sleek coat and long drooping ears", "name": "dachshund"}, {"id": 359, "synset": "dagger.n.01", "synonyms": ["dagger"], "def": "a short knife with a pointed blade used for piercing or stabbing", "name": "dagger"}, {"id": 360, "synset": "dartboard.n.01", "synonyms": ["dartboard"], "def": "a circular board of wood or cork used as the target in the game of darts", "name": "dartboard"}, {"id": 361, "synset": "date.n.08", "synonyms": ["date_(fruit)"], "def": "sweet edible fruit of the date palm with a single long woody seed", "name": "date_(fruit)"}, {"id": 362, "synset": "deck_chair.n.01", "synonyms": ["deck_chair", "beach_chair"], "def": "a folding chair for use outdoors; a wooden frame supports a length of canvas", "name": "deck_chair"}, {"id": 363, "synset": "deer.n.01", "synonyms": ["deer", "cervid"], "def": "distinguished from Bovidae by the male's having solid deciduous antlers", "name": "deer"}, {"id": 364, "synset": "dental_floss.n.01", "synonyms": ["dental_floss", "floss"], "def": "a soft thread for cleaning the spaces between the teeth", "name": "dental_floss"}, {"id": 365, "synset": "desk.n.01", "synonyms": ["desk"], "def": "a piece of furniture with a writing surface and usually drawers or other compartments", "name": "desk"}, {"id": 366, "synset": "detergent.n.01", "synonyms": ["detergent"], "def": "a surface-active chemical widely used in industry and laundering", "name": "detergent"}, {"id": 367, "synset": "diaper.n.01", "synonyms": ["diaper"], "def": "garment consisting of a folded cloth drawn up between the legs and fastened at the waist", "name": "diaper"}, {"id": 368, "synset": "diary.n.01", "synonyms": ["diary", "journal"], "def": "a daily written record of (usually personal) experiences and observations", "name": "diary"}, {"id": 369, "synset": "die.n.01", "synonyms": ["die", "dice"], "def": "a small cube with 1 to 6 spots on the six faces; used in gambling", "name": "die"}, {"id": 370, "synset": "dinghy.n.01", "synonyms": ["dinghy", "dory", "rowboat"], "def": "a small boat of shallow draft with seats and oars with which it is propelled", "name": "dinghy"}, {"id": 371, "synset": "dining_table.n.01", "synonyms": ["dining_table"], "def": "a table at which meals are served", "name": "dining_table"}, {"id": 372, "synset": "dinner_jacket.n.01", "synonyms": ["tux", "tuxedo"], "def": "semiformal evening dress for men", "name": "tux"}, {"id": 373, "synset": "dish.n.01", "synonyms": ["dish"], "def": "a piece of dishware normally used as a container for holding or serving food", "name": "dish"}, {"id": 374, "synset": "dish.n.05", "synonyms": ["dish_antenna"], "def": "directional antenna consisting of a parabolic reflector", "name": "dish_antenna"}, {"id": 375, "synset": "dishrag.n.01", "synonyms": ["dishrag", "dishcloth"], "def": "a cloth for washing dishes", "name": "dishrag"}, {"id": 376, "synset": "dishtowel.n.01", "synonyms": ["dishtowel", "tea_towel"], "def": "a towel for drying dishes", "name": "dishtowel"}, {"id": 377, "synset": "dishwasher.n.01", "synonyms": ["dishwasher", "dishwashing_machine"], "def": "a machine for washing dishes", "name": "dishwasher"}, {"id": 378, "synset": "dishwasher_detergent.n.01", "synonyms": ["dishwasher_detergent", "dishwashing_detergent", "dishwashing_liquid"], "def": "a low-sudsing detergent designed for use in dishwashers", "name": "dishwasher_detergent"}, {"id": 379, "synset": "diskette.n.01", "synonyms": ["diskette", "floppy", "floppy_disk"], "def": "a small plastic magnetic disk enclosed in a stiff envelope used to store data", "name": "diskette"}, {"id": 380, "synset": "dispenser.n.01", "synonyms": ["dispenser"], "def": "a container so designed that the contents can be used in prescribed amounts", "name": "dispenser"}, {"id": 381, "synset": "dixie_cup.n.01", "synonyms": ["Dixie_cup", "paper_cup"], "def": "a disposable cup made of paper; for holding drinks", "name": "Dixie_cup"}, {"id": 382, "synset": "dog.n.01", "synonyms": ["dog"], "def": "a common domesticated dog", "name": "dog"}, {"id": 383, "synset": "dog_collar.n.01", "synonyms": ["dog_collar"], "def": "a collar for a dog", "name": "dog_collar"}, {"id": 384, "synset": "doll.n.01", "synonyms": ["doll"], "def": "a toy replica of a HUMAN (NOT AN ANIMAL)", "name": "doll"}, {"id": 385, "synset": "dollar.n.02", "synonyms": ["dollar", "dollar_bill", "one_dollar_bill"], "def": "a piece of paper money worth one dollar", "name": "dollar"}, {"id": 386, "synset": "dolphin.n.02", "synonyms": ["dolphin"], "def": "any of various small toothed whales with a beaklike snout; larger than porpoises", "name": "dolphin"}, {"id": 387, "synset": "domestic_ass.n.01", "synonyms": ["domestic_ass", "donkey"], "def": "domestic beast of burden descended from the African wild ass; patient but stubborn", "name": "domestic_ass"}, {"id": 388, "synset": "domino.n.03", "synonyms": ["eye_mask"], "def": "a mask covering the upper part of the face but with holes for the eyes", "name": "eye_mask"}, {"id": 389, "synset": "doorbell.n.01", "synonyms": ["doorbell", "buzzer"], "def": "a button at an outer door that gives a ringing or buzzing signal when pushed", "name": "doorbell"}, {"id": 390, "synset": "doorknob.n.01", "synonyms": ["doorknob", "doorhandle"], "def": "a knob used to open a door (often called `doorhandle' in Great Britain)", "name": "doorknob"}, {"id": 391, "synset": "doormat.n.02", "synonyms": ["doormat", "welcome_mat"], "def": "a mat placed outside an exterior door for wiping the shoes before entering", "name": "doormat"}, {"id": 392, "synset": "doughnut.n.02", "synonyms": ["doughnut", "donut"], "def": "a small ring-shaped friedcake", "name": "doughnut"}, {"id": 393, "synset": "dove.n.01", "synonyms": ["dove"], "def": "any of numerous small pigeons", "name": "dove"}, {"id": 394, "synset": "dragonfly.n.01", "synonyms": ["dragonfly"], "def": "slender-bodied non-stinging insect having iridescent wings that are outspread at rest", "name": "dragonfly"}, {"id": 395, "synset": "drawer.n.01", "synonyms": ["drawer"], "def": "a boxlike container in a piece of furniture; made so as to slide in and out", "name": "drawer"}, {"id": 396, "synset": "drawers.n.01", "synonyms": ["underdrawers", "boxers", "boxershorts"], "def": "underpants worn by men", "name": "underdrawers"}, {"id": 397, "synset": "dress.n.01", "synonyms": ["dress", "frock"], "def": "a one-piece garment for a woman; has skirt and bodice", "name": "dress"}, {"id": 398, "synset": "dress_hat.n.01", "synonyms": ["dress_hat", "high_hat", "opera_hat", "silk_hat", "top_hat"], "def": "a man's hat with a tall crown; usually covered with silk or with beaver fur", "name": "dress_hat"}, {"id": 399, "synset": "dress_suit.n.01", "synonyms": ["dress_suit"], "def": "formalwear consisting of full evening dress for men", "name": "dress_suit"}, {"id": 400, "synset": "dresser.n.05", "synonyms": ["dresser"], "def": "a cabinet with shelves", "name": "dresser"}, {"id": 401, "synset": "drill.n.01", "synonyms": ["drill"], "def": "a tool with a sharp rotating point for making holes in hard materials", "name": "drill"}, {"id": 402, "synset": "drinking_fountain.n.01", "synonyms": ["drinking_fountain"], "def": "a public fountain to provide a jet of drinking water", "name": "drinking_fountain"}, {"id": 403, "synset": "drone.n.04", "synonyms": ["drone"], "def": "an aircraft without a pilot that is operated by remote control", "name": "drone"}, {"id": 404, "synset": "dropper.n.01", "synonyms": ["dropper", "eye_dropper"], "def": "pipet consisting of a small tube with a vacuum bulb at one end for drawing liquid in and releasing it a drop at a time", "name": "dropper"}, {"id": 405, "synset": "drum.n.01", "synonyms": ["drum_(musical_instrument)"], "def": "a musical percussion instrument; usually consists of a hollow cylinder with a membrane stretched across each end", "name": "drum_(musical_instrument)"}, {"id": 406, "synset": "drumstick.n.02", "synonyms": ["drumstick"], "def": "a stick used for playing a drum", "name": "drumstick"}, {"id": 407, "synset": "duck.n.01", "synonyms": ["duck"], "def": "small web-footed broad-billed swimming bird", "name": "duck"}, {"id": 408, "synset": "duckling.n.02", "synonyms": ["duckling"], "def": "young duck", "name": "duckling"}, {"id": 409, "synset": "duct_tape.n.01", "synonyms": ["duct_tape"], "def": "a wide silvery adhesive tape", "name": "duct_tape"}, {"id": 410, "synset": "duffel_bag.n.01", "synonyms": ["duffel_bag", "duffle_bag", "duffel", "duffle"], "def": "a large cylindrical bag of heavy cloth", "name": "duffel_bag"}, {"id": 411, "synset": "dumbbell.n.01", "synonyms": ["dumbbell"], "def": "an exercising weight with two ball-like ends connected by a short handle", "name": "dumbbell"}, {"id": 412, "synset": "dumpster.n.01", "synonyms": ["dumpster"], "def": "a container designed to receive and transport and dump waste", "name": "dumpster"}, {"id": 413, "synset": "dustpan.n.02", "synonyms": ["dustpan"], "def": "a short-handled receptacle into which dust can be swept", "name": "dustpan"}, {"id": 414, "synset": "dutch_oven.n.02", "synonyms": ["Dutch_oven"], "def": "iron or earthenware cooking pot; used for stews", "name": "Dutch_oven"}, {"id": 415, "synset": "eagle.n.01", "synonyms": ["eagle"], "def": "large birds of prey noted for their broad wings and strong soaring flight", "name": "eagle"}, {"id": 416, "synset": "earphone.n.01", "synonyms": ["earphone", "earpiece", "headphone"], "def": "device for listening to audio that is held over or inserted into the ear", "name": "earphone"}, {"id": 417, "synset": "earplug.n.01", "synonyms": ["earplug"], "def": "a soft plug that is inserted into the ear canal to block sound", "name": "earplug"}, {"id": 418, "synset": "earring.n.01", "synonyms": ["earring"], "def": "jewelry to ornament the ear", "name": "earring"}, {"id": 419, "synset": "easel.n.01", "synonyms": ["easel"], "def": "an upright tripod for displaying something (usually an artist's canvas)", "name": "easel"}, {"id": 420, "synset": "eclair.n.01", "synonyms": ["eclair"], "def": "oblong cream puff", "name": "eclair"}, {"id": 421, "synset": "eel.n.01", "synonyms": ["eel"], "def": "an elongate fish with fatty flesh", "name": "eel"}, {"id": 422, "synset": "egg.n.02", "synonyms": ["egg", "eggs"], "def": "oval reproductive body of a fowl (especially a hen) used as food", "name": "egg"}, {"id": 423, "synset": "egg_roll.n.01", "synonyms": ["egg_roll", "spring_roll"], "def": "minced vegetables and meat wrapped in a pancake and fried", "name": "egg_roll"}, {"id": 424, "synset": "egg_yolk.n.01", "synonyms": ["egg_yolk", "yolk_(egg)"], "def": "the yellow spherical part of an egg", "name": "egg_yolk"}, {"id": 425, "synset": "eggbeater.n.02", "synonyms": ["eggbeater", "eggwhisk"], "def": "a mixer for beating eggs or whipping cream", "name": "eggbeater"}, {"id": 426, "synset": "eggplant.n.01", "synonyms": ["eggplant", "aubergine"], "def": "egg-shaped vegetable having a shiny skin typically dark purple", "name": "eggplant"}, {"id": 427, "synset": "electric_chair.n.01", "synonyms": ["electric_chair"], "def": "a chair-shaped instrument of execution by electrocution", "name": "electric_chair"}, {"id": 428, "synset": "electric_refrigerator.n.01", "synonyms": ["refrigerator"], "def": "a refrigerator in which the coolant is pumped around by an electric motor", "name": "refrigerator"}, {"id": 429, "synset": "elephant.n.01", "synonyms": ["elephant"], "def": "a common elephant", "name": "elephant"}, {"id": 430, "synset": "elk.n.01", "synonyms": ["elk", "moose"], "def": "large northern deer with enormous flattened antlers in the male", "name": "elk"}, {"id": 431, "synset": "envelope.n.01", "synonyms": ["envelope"], "def": "a flat (usually rectangular) container for a letter, thin package, etc.", "name": "envelope"}, {"id": 432, "synset": "eraser.n.01", "synonyms": ["eraser"], "def": "an implement used to erase something", "name": "eraser"}, {"id": 433, "synset": "escargot.n.01", "synonyms": ["escargot"], "def": "edible snail usually served in the shell with a sauce of melted butter and garlic", "name": "escargot"}, {"id": 434, "synset": "eyepatch.n.01", "synonyms": ["eyepatch"], "def": "a protective cloth covering for an injured eye", "name": "eyepatch"}, {"id": 435, "synset": "falcon.n.01", "synonyms": ["falcon"], "def": "birds of prey having long pointed powerful wings adapted for swift flight", "name": "falcon"}, {"id": 436, "synset": "fan.n.01", "synonyms": ["fan"], "def": "a device for creating a current of air by movement of a surface or surfaces", "name": "fan"}, {"id": 437, "synset": "faucet.n.01", "synonyms": ["faucet", "spigot", "tap"], "def": "a regulator for controlling the flow of a liquid from a reservoir", "name": "faucet"}, {"id": 438, "synset": "fedora.n.01", "synonyms": ["fedora"], "def": "a hat made of felt with a creased crown", "name": "fedora"}, {"id": 439, "synset": "ferret.n.02", "synonyms": ["ferret"], "def": "domesticated albino variety of the European polecat bred for hunting rats and rabbits", "name": "ferret"}, {"id": 440, "synset": "ferris_wheel.n.01", "synonyms": ["Ferris_wheel"], "def": "a large wheel with suspended seats that remain upright as the wheel rotates", "name": "Ferris_wheel"}, {"id": 441, "synset": "ferry.n.01", "synonyms": ["ferry", "ferryboat"], "def": "a boat that transports people or vehicles across a body of water and operates on a regular schedule", "name": "ferry"}, {"id": 442, "synset": "fig.n.04", "synonyms": ["fig_(fruit)"], "def": "fleshy sweet pear-shaped yellowish or purple fruit eaten fresh or preserved or dried", "name": "fig_(fruit)"}, {"id": 443, "synset": "fighter.n.02", "synonyms": ["fighter_jet", "fighter_aircraft", "attack_aircraft"], "def": "a high-speed military or naval airplane designed to destroy enemy targets", "name": "fighter_jet"}, {"id": 444, "synset": "figurine.n.01", "synonyms": ["figurine"], "def": "a small carved or molded figure", "name": "figurine"}, {"id": 445, "synset": "file.n.03", "synonyms": ["file_cabinet", "filing_cabinet"], "def": "office furniture consisting of a container for keeping papers in order", "name": "file_cabinet"}, {"id": 446, "synset": "file.n.04", "synonyms": ["file_(tool)"], "def": "a steel hand tool with small sharp teeth on some or all of its surfaces; used for smoothing wood or metal", "name": "file_(tool)"}, {"id": 447, "synset": "fire_alarm.n.02", "synonyms": ["fire_alarm", "smoke_alarm"], "def": "an alarm that is tripped off by fire or smoke", "name": "fire_alarm"}, {"id": 448, "synset": "fire_engine.n.01", "synonyms": ["fire_engine", "fire_truck"], "def": "large trucks that carry firefighters and equipment to the site of a fire", "name": "fire_engine"}, {"id": 449, "synset": "fire_extinguisher.n.01", "synonyms": ["fire_extinguisher", "extinguisher"], "def": "a manually operated device for extinguishing small fires", "name": "fire_extinguisher"}, {"id": 450, "synset": "fire_hose.n.01", "synonyms": ["fire_hose"], "def": "a large hose that carries water from a fire hydrant to the site of the fire", "name": "fire_hose"}, {"id": 451, "synset": "fireplace.n.01", "synonyms": ["fireplace"], "def": "an open recess in a wall at the base of a chimney where a fire can be built", "name": "fireplace"}, {"id": 452, "synset": "fireplug.n.01", "synonyms": ["fireplug", "fire_hydrant", "hydrant"], "def": "an upright hydrant for drawing water to use in fighting a fire", "name": "fireplug"}, {"id": 453, "synset": "fish.n.01", "synonyms": ["fish"], "def": "any of various mostly cold-blooded aquatic vertebrates usually having scales and breathing through gills", "name": "fish"}, {"id": 454, "synset": "fish.n.02", "synonyms": ["fish_(food)"], "def": "the flesh of fish used as food", "name": "fish_(food)"}, {"id": 455, "synset": "fishbowl.n.02", "synonyms": ["fishbowl", "goldfish_bowl"], "def": "a transparent bowl in which small fish are kept", "name": "fishbowl"}, {"id": 456, "synset": "fishing_boat.n.01", "synonyms": ["fishing_boat", "fishing_vessel"], "def": "a vessel for fishing", "name": "fishing_boat"}, {"id": 457, "synset": "fishing_rod.n.01", "synonyms": ["fishing_rod", "fishing_pole"], "def": "a rod that is used in fishing to extend the fishing line", "name": "fishing_rod"}, {"id": 458, "synset": "flag.n.01", "synonyms": ["flag"], "def": "emblem usually consisting of a rectangular piece of cloth of distinctive design (do not include pole)", "name": "flag"}, {"id": 459, "synset": "flagpole.n.02", "synonyms": ["flagpole", "flagstaff"], "def": "a tall staff or pole on which a flag is raised", "name": "flagpole"}, {"id": 460, "synset": "flamingo.n.01", "synonyms": ["flamingo"], "def": "large pink web-footed bird with down-bent bill", "name": "flamingo"}, {"id": 461, "synset": "flannel.n.01", "synonyms": ["flannel"], "def": "a soft light woolen fabric; used for clothing", "name": "flannel"}, {"id": 462, "synset": "flash.n.10", "synonyms": ["flash", "flashbulb"], "def": "a lamp for providing momentary light to take a photograph", "name": "flash"}, {"id": 463, "synset": "flashlight.n.01", "synonyms": ["flashlight", "torch"], "def": "a small portable battery-powered electric lamp", "name": "flashlight"}, {"id": 464, "synset": "fleece.n.03", "synonyms": ["fleece"], "def": "a soft bulky fabric with deep pile; used chiefly for clothing", "name": "fleece"}, {"id": 465, "synset": "flip-flop.n.02", "synonyms": ["flip-flop_(sandal)"], "def": "a backless sandal held to the foot by a thong between two toes", "name": "flip-flop_(sandal)"}, {"id": 466, "synset": "flipper.n.01", "synonyms": ["flipper_(footwear)", "fin_(footwear)"], "def": "a shoe to aid a person in swimming", "name": "flipper_(footwear)"}, {"id": 467, "synset": "flower_arrangement.n.01", "synonyms": ["flower_arrangement", "floral_arrangement"], "def": "a decorative arrangement of flowers", "name": "flower_arrangement"}, {"id": 468, "synset": "flute.n.02", "synonyms": ["flute_glass", "champagne_flute"], "def": "a tall narrow wineglass", "name": "flute_glass"}, {"id": 469, "synset": "foal.n.01", "synonyms": ["foal"], "def": "a young horse", "name": "foal"}, {"id": 470, "synset": "folding_chair.n.01", "synonyms": ["folding_chair"], "def": "a chair that can be folded flat for storage", "name": "folding_chair"}, {"id": 471, "synset": "food_processor.n.01", "synonyms": ["food_processor"], "def": "a kitchen appliance for shredding, blending, chopping, or slicing food", "name": "food_processor"}, {"id": 472, "synset": "football.n.02", "synonyms": ["football_(American)"], "def": "the inflated oblong ball used in playing American football", "name": "football_(American)"}, {"id": 473, "synset": "football_helmet.n.01", "synonyms": ["football_helmet"], "def": "a padded helmet with a face mask to protect the head of football players", "name": "football_helmet"}, {"id": 474, "synset": "footstool.n.01", "synonyms": ["footstool", "footrest"], "def": "a low seat or a stool to rest the feet of a seated person", "name": "footstool"}, {"id": 475, "synset": "fork.n.01", "synonyms": ["fork"], "def": "cutlery used for serving and eating food", "name": "fork"}, {"id": 476, "synset": "forklift.n.01", "synonyms": ["forklift"], "def": "an industrial vehicle with a power operated fork in front that can be inserted under loads to lift and move them", "name": "forklift"}, {"id": 477, "synset": "freight_car.n.01", "synonyms": ["freight_car"], "def": "a railway car that carries freight", "name": "freight_car"}, {"id": 478, "synset": "french_toast.n.01", "synonyms": ["French_toast"], "def": "bread slice dipped in egg and milk and fried", "name": "French_toast"}, {"id": 479, "synset": "freshener.n.01", "synonyms": ["freshener", "air_freshener"], "def": "anything that freshens", "name": "freshener"}, {"id": 480, "synset": "frisbee.n.01", "synonyms": ["frisbee"], "def": "a light, plastic disk propelled with a flip of the wrist for recreation or competition", "name": "frisbee"}, {"id": 481, "synset": "frog.n.01", "synonyms": ["frog", "toad", "toad_frog"], "def": "a tailless stout-bodied amphibians with long hind limbs for leaping", "name": "frog"}, {"id": 482, "synset": "fruit_juice.n.01", "synonyms": ["fruit_juice"], "def": "drink produced by squeezing or crushing fruit", "name": "fruit_juice"}, {"id": 483, "synset": "fruit_salad.n.01", "synonyms": ["fruit_salad"], "def": "salad composed of fruits", "name": "fruit_salad"}, {"id": 484, "synset": "frying_pan.n.01", "synonyms": ["frying_pan", "frypan", "skillet"], "def": "a pan used for frying foods", "name": "frying_pan"}, {"id": 485, "synset": "fudge.n.01", "synonyms": ["fudge"], "def": "soft creamy candy", "name": "fudge"}, {"id": 486, "synset": "funnel.n.02", "synonyms": ["funnel"], "def": "a cone-shaped utensil used to channel a substance into a container with a small mouth", "name": "funnel"}, {"id": 487, "synset": "futon.n.01", "synonyms": ["futon"], "def": "a pad that is used for sleeping on the floor or on a raised frame", "name": "futon"}, {"id": 488, "synset": "gag.n.02", "synonyms": ["gag", "muzzle"], "def": "restraint put into a person's mouth to prevent speaking or shouting", "name": "gag"}, {"id": 489, "synset": "garbage.n.03", "synonyms": ["garbage"], "def": "a receptacle where waste can be discarded", "name": "garbage"}, {"id": 490, "synset": "garbage_truck.n.01", "synonyms": ["garbage_truck"], "def": "a truck for collecting domestic refuse", "name": "garbage_truck"}, {"id": 491, "synset": "garden_hose.n.01", "synonyms": ["garden_hose"], "def": "a hose used for watering a lawn or garden", "name": "garden_hose"}, {"id": 492, "synset": "gargle.n.01", "synonyms": ["gargle", "mouthwash"], "def": "a medicated solution used for gargling and rinsing the mouth", "name": "gargle"}, {"id": 493, "synset": "gargoyle.n.02", "synonyms": ["gargoyle"], "def": "an ornament consisting of a grotesquely carved figure of a person or animal", "name": "gargoyle"}, {"id": 494, "synset": "garlic.n.02", "synonyms": ["garlic", "ail"], "def": "aromatic bulb used as seasoning", "name": "garlic"}, {"id": 495, "synset": "gasmask.n.01", "synonyms": ["gasmask", "respirator", "gas_helmet"], "def": "a protective face mask with a filter", "name": "gasmask"}, {"id": 496, "synset": "gazelle.n.01", "synonyms": ["gazelle"], "def": "small swift graceful antelope of Africa and Asia having lustrous eyes", "name": "gazelle"}, {"id": 497, "synset": "gelatin.n.02", "synonyms": ["gelatin", "jelly"], "def": "an edible jelly made with gelatin and used as a dessert or salad base or a coating for foods", "name": "gelatin"}, {"id": 498, "synset": "gem.n.02", "synonyms": ["gemstone"], "def": "a crystalline rock that can be cut and polished for jewelry", "name": "gemstone"}, {"id": 499, "synset": "giant_panda.n.01", "synonyms": ["giant_panda", "panda", "panda_bear"], "def": "large black-and-white herbivorous mammal of bamboo forests of China and Tibet", "name": "giant_panda"}, {"id": 500, "synset": "gift_wrap.n.01", "synonyms": ["gift_wrap"], "def": "attractive wrapping paper suitable for wrapping gifts", "name": "gift_wrap"}, {"id": 501, "synset": "ginger.n.03", "synonyms": ["ginger", "gingerroot"], "def": "the root of the common ginger plant; used fresh as a seasoning", "name": "ginger"}, {"id": 502, "synset": "giraffe.n.01", "synonyms": ["giraffe"], "def": "tall animal having a spotted coat and small horns and very long neck and legs", "name": "giraffe"}, {"id": 503, "synset": "girdle.n.02", "synonyms": ["cincture", "sash", "waistband", "waistcloth"], "def": "a band of material around the waist that strengthens a skirt or trousers", "name": "cincture"}, {"id": 504, "synset": "glass.n.02", "synonyms": ["glass_(drink_container)", "drinking_glass"], "def": "a container for holding liquids while drinking", "name": "glass_(drink_container)"}, {"id": 505, "synset": "globe.n.03", "synonyms": ["globe"], "def": "a sphere on which a map (especially of the earth) is represented", "name": "globe"}, {"id": 506, "synset": "glove.n.02", "synonyms": ["glove"], "def": "handwear covering the hand", "name": "glove"}, {"id": 507, "synset": "goat.n.01", "synonyms": ["goat"], "def": "a common goat", "name": "goat"}, {"id": 508, "synset": "goggles.n.01", "synonyms": ["goggles"], "def": "tight-fitting spectacles worn to protect the eyes", "name": "goggles"}, {"id": 509, "synset": "goldfish.n.01", "synonyms": ["goldfish"], "def": "small golden or orange-red freshwater fishes used as pond or aquarium pets", "name": "goldfish"}, {"id": 510, "synset": "golf_club.n.02", "synonyms": ["golf_club", "golf-club"], "def": "golf equipment used by a golfer to hit a golf ball", "name": "golf_club"}, {"id": 511, "synset": "golfcart.n.01", "synonyms": ["golfcart"], "def": "a small motor vehicle in which golfers can ride between shots", "name": "golfcart"}, {"id": 512, "synset": "gondola.n.02", "synonyms": ["gondola_(boat)"], "def": "long narrow flat-bottomed boat propelled by sculling; traditionally used on canals of Venice", "name": "gondola_(boat)"}, {"id": 513, "synset": "goose.n.01", "synonyms": ["goose"], "def": "loud, web-footed long-necked aquatic birds usually larger than ducks", "name": "goose"}, {"id": 514, "synset": "gorilla.n.01", "synonyms": ["gorilla"], "def": "largest ape", "name": "gorilla"}, {"id": 515, "synset": "gourd.n.02", "synonyms": ["gourd"], "def": "any of numerous inedible fruits with hard rinds", "name": "gourd"}, {"id": 516, "synset": "gown.n.04", "synonyms": ["surgical_gown", "scrubs_(surgical_clothing)"], "def": "protective garment worn by surgeons during operations", "name": "surgical_gown"}, {"id": 517, "synset": "grape.n.01", "synonyms": ["grape"], "def": "any of various juicy fruit with green or purple skins; grow in clusters", "name": "grape"}, {"id": 518, "synset": "grasshopper.n.01", "synonyms": ["grasshopper"], "def": "plant-eating insect with hind legs adapted for leaping", "name": "grasshopper"}, {"id": 519, "synset": "grater.n.01", "synonyms": ["grater"], "def": "utensil with sharp perforations for shredding foods (as vegetables or cheese)", "name": "grater"}, {"id": 520, "synset": "gravestone.n.01", "synonyms": ["gravestone", "headstone", "tombstone"], "def": "a stone that is used to mark a grave", "name": "gravestone"}, {"id": 521, "synset": "gravy_boat.n.01", "synonyms": ["gravy_boat", "gravy_holder"], "def": "a dish (often boat-shaped) for serving gravy or sauce", "name": "gravy_boat"}, {"id": 522, "synset": "green_bean.n.02", "synonyms": ["green_bean"], "def": "a common bean plant cultivated for its slender green edible pods", "name": "green_bean"}, {"id": 523, "synset": "green_onion.n.01", "synonyms": ["green_onion", "spring_onion", "scallion"], "def": "a young onion before the bulb has enlarged", "name": "green_onion"}, {"id": 524, "synset": "griddle.n.01", "synonyms": ["griddle"], "def": "cooking utensil consisting of a flat heated surface on which food is cooked", "name": "griddle"}, {"id": 525, "synset": "grillroom.n.01", "synonyms": ["grillroom", "grill_(restaurant)"], "def": "a restaurant where food is cooked on a grill", "name": "grillroom"}, {"id": 526, "synset": "grinder.n.04", "synonyms": ["grinder_(tool)"], "def": "a machine tool that polishes metal", "name": "grinder_(tool)"}, {"id": 527, "synset": "grits.n.01", "synonyms": ["grits", "hominy_grits"], "def": "coarsely ground corn boiled as a breakfast dish", "name": "grits"}, {"id": 528, "synset": "grizzly.n.01", "synonyms": ["grizzly", "grizzly_bear"], "def": "powerful brownish-yellow bear of the uplands of western North America", "name": "grizzly"}, {"id": 529, "synset": "grocery_bag.n.01", "synonyms": ["grocery_bag"], "def": "a sack for holding customer's groceries", "name": "grocery_bag", "merged": [{"frequency": "f", "id": 912, "synset": "sack.n.01", "image_count": 37, "instance_count": 76, "synonyms": ["plastic_bag", "paper_bag"], "def": "a bag made of paper or plastic for holding customer's purchases", "name": "plastic_bag"}, {"frequency": "c", "id": 967, "synset": "shopping_bag.n.01", "image_count": 9, "instance_count": 18, "synonyms": ["shopping_bag"], "def": "a bag made of plastic or strong paper (often with handles); used to transport goods after shopping", "name": "shopping_bag"}]}, {"id": 530, "synset": "guacamole.n.01", "synonyms": ["guacamole"], "def": "a dip made of mashed avocado mixed with chopped onions and other seasonings", "name": "guacamole"}, {"id": 531, "synset": "guitar.n.01", "synonyms": ["guitar"], "def": "a stringed instrument usually having six strings; played by strumming or plucking", "name": "guitar"}, {"id": 532, "synset": "gull.n.02", "synonyms": ["gull", "seagull"], "def": "mostly white aquatic bird having long pointed wings and short legs", "name": "gull"}, {"id": 533, "synset": "gun.n.01", "synonyms": ["gun"], "def": "a weapon that discharges a bullet at high velocity from a metal tube", "name": "gun"}, {"id": 534, "synset": "hair_spray.n.01", "synonyms": ["hair_spray"], "def": "substance sprayed on the hair to hold it in place", "name": "hair_spray"}, {"id": 535, "synset": "hairbrush.n.01", "synonyms": ["hairbrush"], "def": "a brush used to groom a person's hair", "name": "hairbrush"}, {"id": 536, "synset": "hairnet.n.01", "synonyms": ["hairnet"], "def": "a small net that someone wears over their hair to keep it in place", "name": "hairnet"}, {"id": 537, "synset": "hairpin.n.01", "synonyms": ["hairpin"], "def": "a double pronged pin used to hold women's hair in place", "name": "hairpin"}, {"id": 538, "synset": "ham.n.01", "synonyms": ["ham", "jambon", "gammon"], "def": "meat cut from the thigh of a hog (usually smoked)", "name": "ham"}, {"id": 539, "synset": "hamburger.n.01", "synonyms": ["hamburger", "beefburger", "burger"], "def": "a sandwich consisting of a patty of minced beef served on a bun", "name": "hamburger"}, {"id": 540, "synset": "hammer.n.02", "synonyms": ["hammer"], "def": "a hand tool with a heavy head and a handle; used to deliver an impulsive force by striking", "name": "hammer"}, {"id": 541, "synset": "hammock.n.02", "synonyms": ["hammock"], "def": "a hanging bed of canvas or rope netting (usually suspended between two trees)", "name": "hammock"}, {"id": 542, "synset": "hamper.n.02", "synonyms": ["hamper"], "def": "a basket usually with a cover", "name": "hamper"}, {"id": 543, "synset": "hamster.n.01", "synonyms": ["hamster"], "def": "short-tailed burrowing rodent with large cheek pouches", "name": "hamster"}, {"id": 544, "synset": "hand_blower.n.01", "synonyms": ["hair_dryer"], "def": "a hand-held electric blower that can blow warm air onto the hair", "name": "hair_dryer"}, {"id": 545, "synset": "hand_glass.n.01", "synonyms": ["hand_glass", "hand_mirror"], "def": "a mirror intended to be held in the hand", "name": "hand_glass"}, {"id": 546, "synset": "hand_towel.n.01", "synonyms": ["hand_towel", "face_towel"], "def": "a small towel used to dry the hands or face", "name": "hand_towel"}, {"id": 547, "synset": "handcart.n.01", "synonyms": ["handcart", "pushcart", "hand_truck"], "def": "wheeled vehicle that can be pushed by a person", "name": "handcart"}, {"id": 548, "synset": "handcuff.n.01", "synonyms": ["handcuff"], "def": "shackle that consists of a metal loop that can be locked around the wrist", "name": "handcuff"}, {"id": 549, "synset": "handkerchief.n.01", "synonyms": ["handkerchief"], "def": "a square piece of cloth used for wiping the eyes or nose or as a costume accessory", "name": "handkerchief"}, {"id": 550, "synset": "handle.n.01", "synonyms": ["handle", "grip", "handgrip"], "def": "the appendage to an object that is designed to be held in order to use or move it", "name": "handle"}, {"id": 551, "synset": "handsaw.n.01", "synonyms": ["handsaw", "carpenter's_saw"], "def": "a saw used with one hand for cutting wood", "name": "handsaw"}, {"id": 552, "synset": "hardback.n.01", "synonyms": ["hardback_book", "hardcover_book"], "def": "a book with cardboard or cloth or leather covers", "name": "hardback_book"}, {"id": 553, "synset": "harmonium.n.01", "synonyms": ["harmonium", "organ_(musical_instrument)", "reed_organ_(musical_instrument)"], "def": "a free-reed instrument in which air is forced through the reeds by bellows", "name": "harmonium"}, {"id": 554, "synset": "hat.n.01", "synonyms": ["hat"], "def": "headwear that protects the head from bad weather, sun, or worn for fashion", "name": "hat", "merged": [{"frequency": "c", "id": 207, "synset": "cap.n.01", "image_count": 2, "instance_count": 5, "synonyms": ["cap_(headwear)"], "def": "a tight-fitting headwear", "name": "cap_(headwear)"}]}, {"id": 555, "synset": "hatbox.n.01", "synonyms": ["hatbox"], "def": "a round piece of luggage for carrying hats", "name": "hatbox"}, {"id": 556, "synset": "hatch.n.03", "synonyms": ["hatch"], "def": "a movable barrier covering a hatchway", "name": "hatch"}, {"id": 557, "synset": "head_covering.n.01", "synonyms": ["veil"], "def": "a garment that covers the head and face", "name": "veil"}, {"id": 558, "synset": "headband.n.01", "synonyms": ["headband"], "def": "a band worn around or over the head", "name": "headband"}, {"id": 559, "synset": "headboard.n.01", "synonyms": ["headboard"], "def": "a vertical board or panel forming the head of a bedstead", "name": "headboard"}, {"id": 560, "synset": "headlight.n.01", "synonyms": ["headlight", "headlamp"], "def": "a powerful light with reflector; attached to the front of an automobile or locomotive", "name": "headlight"}, {"id": 561, "synset": "headscarf.n.01", "synonyms": ["headscarf"], "def": "a kerchief worn over the head and tied under the chin", "name": "headscarf"}, {"id": 562, "synset": "headset.n.01", "synonyms": ["headset"], "def": "receiver consisting of a pair of headphones", "name": "headset"}, {"id": 563, "synset": "headstall.n.01", "synonyms": ["headstall_(for_horses)", "headpiece_(for_horses)"], "def": "the band that is the part of a bridle that fits around a horse's head", "name": "headstall_(for_horses)"}, {"id": 564, "synset": "hearing_aid.n.02", "synonyms": ["hearing_aid"], "def": "an acoustic device used to direct sound to the ear of a hearing-impaired person", "name": "hearing_aid"}, {"id": 565, "synset": "heart.n.02", "synonyms": ["heart"], "def": "a muscular organ; its contractions move the blood through the body", "name": "heart"}, {"id": 566, "synset": "heater.n.01", "synonyms": ["heater", "warmer"], "def": "device that heats water or supplies warmth to a room", "name": "heater"}, {"id": 567, "synset": "helicopter.n.01", "synonyms": ["helicopter"], "def": "an aircraft without wings that obtains its lift from the rotation of overhead blades", "name": "helicopter"}, {"id": 568, "synset": "helmet.n.02", "synonyms": ["helmet"], "def": "a protective headgear made of hard material to resist blows", "name": "helmet"}, {"id": 569, "synset": "heron.n.02", "synonyms": ["heron"], "def": "grey or white wading bird with long neck and long legs and (usually) long bill", "name": "heron"}, {"id": 570, "synset": "highchair.n.01", "synonyms": ["highchair", "feeding_chair"], "def": "a chair for feeding a very young child", "name": "highchair"}, {"id": 571, "synset": "hinge.n.01", "synonyms": ["hinge"], "def": "a joint that holds two parts together so that one can swing relative to the other", "name": "hinge"}, {"id": 572, "synset": "hippopotamus.n.01", "synonyms": ["hippopotamus"], "def": "massive thick-skinned animal living in or around rivers of tropical Africa", "name": "hippopotamus"}, {"id": 573, "synset": "hockey_stick.n.01", "synonyms": ["hockey_stick"], "def": "sports implement consisting of a stick used by hockey players to move the puck", "name": "hockey_stick"}, {"id": 574, "synset": "hog.n.03", "synonyms": ["hog", "pig"], "def": "domestic swine", "name": "hog"}, {"id": 575, "synset": "home_plate.n.01", "synonyms": ["home_plate_(baseball)", "home_base_(baseball)"], "def": "(baseball) a rubber slab where the batter stands; it must be touched by a base runner in order to score", "name": "home_plate_(baseball)"}, {"id": 576, "synset": "honey.n.01", "synonyms": ["honey"], "def": "a sweet yellow liquid produced by bees", "name": "honey"}, {"id": 577, "synset": "hood.n.06", "synonyms": ["fume_hood", "exhaust_hood"], "def": "metal covering leading to a vent that exhausts smoke or fumes", "name": "fume_hood"}, {"id": 578, "synset": "hook.n.05", "synonyms": ["hook"], "def": "a curved or bent implement for suspending or pulling something", "name": "hook"}, {"id": 579, "synset": "horse.n.01", "synonyms": ["horse"], "def": "a common horse", "name": "horse"}, {"id": 580, "synset": "hose.n.03", "synonyms": ["hose", "hosepipe"], "def": "a flexible pipe for conveying a liquid or gas", "name": "hose"}, {"id": 581, "synset": "hot-air_balloon.n.01", "synonyms": ["hot-air_balloon"], "def": "balloon for travel through the air in a basket suspended below a large bag of heated air", "name": "hot-air_balloon"}, {"id": 582, "synset": "hot_plate.n.01", "synonyms": ["hotplate"], "def": "a portable electric appliance for heating or cooking or keeping food warm", "name": "hotplate"}, {"id": 583, "synset": "hot_sauce.n.01", "synonyms": ["hot_sauce"], "def": "a pungent peppery sauce", "name": "hot_sauce"}, {"id": 584, "synset": "hourglass.n.01", "synonyms": ["hourglass"], "def": "a sandglass timer that runs for sixty minutes", "name": "hourglass"}, {"id": 585, "synset": "houseboat.n.01", "synonyms": ["houseboat"], "def": "a barge that is designed and equipped for use as a dwelling", "name": "houseboat"}, {"id": 586, "synset": "hummingbird.n.01", "synonyms": ["hummingbird"], "def": "tiny American bird having brilliant iridescent plumage and long slender bills", "name": "hummingbird"}, {"id": 587, "synset": "hummus.n.01", "synonyms": ["hummus", "humus", "hommos", "hoummos", "humous"], "def": "a thick spread made from mashed chickpeas", "name": "hummus"}, {"id": 588, "synset": "ice_bear.n.01", "synonyms": ["polar_bear"], "def": "white bear of Arctic regions", "name": "polar_bear"}, {"id": 589, "synset": "ice_cream.n.01", "synonyms": ["icecream"], "def": "frozen dessert containing cream and sugar and flavoring", "name": "icecream"}, {"id": 590, "synset": "ice_lolly.n.01", "synonyms": ["popsicle"], "def": "ice cream or water ice on a small wooden stick", "name": "popsicle"}, {"id": 591, "synset": "ice_maker.n.01", "synonyms": ["ice_maker"], "def": "an appliance included in some electric refrigerators for making ice cubes", "name": "ice_maker"}, {"id": 592, "synset": "ice_pack.n.01", "synonyms": ["ice_pack", "ice_bag"], "def": "a waterproof bag filled with ice: applied to the body (especially the head) to cool or reduce swelling", "name": "ice_pack"}, {"id": 593, "synset": "ice_skate.n.01", "synonyms": ["ice_skate"], "def": "skate consisting of a boot with a steel blade fitted to the sole", "name": "ice_skate"}, {"id": 594, "synset": "ice_tea.n.01", "synonyms": ["ice_tea", "iced_tea"], "def": "strong tea served over ice", "name": "ice_tea"}, {"id": 595, "synset": "igniter.n.01", "synonyms": ["igniter", "ignitor", "lighter"], "def": "a substance or device used to start a fire", "name": "igniter"}, {"id": 596, "synset": "incense.n.01", "synonyms": ["incense"], "def": "a substance that produces a fragrant odor when burned", "name": "incense"}, {"id": 597, "synset": "inhaler.n.01", "synonyms": ["inhaler", "inhalator"], "def": "a dispenser that produces a chemical vapor to be inhaled through mouth or nose", "name": "inhaler"}, {"id": 598, "synset": "ipod.n.01", "synonyms": ["iPod"], "def": "a pocket-sized device used to play music files", "name": "iPod"}, {"id": 599, "synset": "iron.n.04", "synonyms": ["iron_(for_clothing)", "smoothing_iron_(for_clothing)"], "def": "home appliance consisting of a flat metal base that is heated and used to smooth cloth", "name": "iron_(for_clothing)"}, {"id": 600, "synset": "ironing_board.n.01", "synonyms": ["ironing_board"], "def": "narrow padded board on collapsible supports; used for ironing clothes", "name": "ironing_board"}, {"id": 601, "synset": "jacket.n.01", "synonyms": ["jacket"], "def": "a waist-length coat", "name": "jacket"}, {"id": 602, "synset": "jam.n.01", "synonyms": ["jam"], "def": "preserve of crushed fruit", "name": "jam"}, {"id": 603, "synset": "jean.n.01", "synonyms": ["jean", "blue_jean", "denim"], "def": "(usually plural) close-fitting trousers of heavy denim for manual work or casual wear", "name": "jean"}, {"id": 604, "synset": "jeep.n.01", "synonyms": ["jeep", "landrover"], "def": "a car suitable for traveling over rough terrain", "name": "jeep"}, {"id": 605, "synset": "jelly_bean.n.01", "synonyms": ["jelly_bean", "jelly_egg"], "def": "sugar-glazed jellied candy", "name": "jelly_bean"}, {"id": 606, "synset": "jersey.n.03", "synonyms": ["jersey", "T-shirt", "tee_shirt"], "def": "a close-fitting pullover shirt", "name": "jersey"}, {"id": 607, "synset": "jet.n.01", "synonyms": ["jet_plane", "jet-propelled_plane"], "def": "an airplane powered by one or more jet engines", "name": "jet_plane"}, {"id": 608, "synset": "jewelry.n.01", "synonyms": ["jewelry", "jewellery"], "def": "an adornment (as a bracelet or ring or necklace) made of precious metals and set with gems (or imitation gems)", "name": "jewelry"}, {"id": 609, "synset": "joystick.n.02", "synonyms": ["joystick"], "def": "a control device for computers consisting of a vertical handle that can move freely in two directions", "name": "joystick"}, {"id": 610, "synset": "jump_suit.n.01", "synonyms": ["jumpsuit"], "def": "one-piece garment fashioned after a parachutist's uniform", "name": "jumpsuit"}, {"id": 611, "synset": "kayak.n.01", "synonyms": ["kayak"], "def": "a small canoe consisting of a light frame made watertight with animal skins", "name": "kayak"}, {"id": 612, "synset": "keg.n.02", "synonyms": ["keg"], "def": "small cask or barrel", "name": "keg"}, {"id": 613, "synset": "kennel.n.01", "synonyms": ["kennel", "doghouse"], "def": "outbuilding that serves as a shelter for a dog", "name": "kennel"}, {"id": 614, "synset": "kettle.n.01", "synonyms": ["kettle", "boiler"], "def": "a metal pot for stewing or boiling; usually has a lid", "name": "kettle"}, {"id": 615, "synset": "key.n.01", "synonyms": ["key"], "def": "metal instrument used to unlock a lock", "name": "key"}, {"id": 616, "synset": "keycard.n.01", "synonyms": ["keycard"], "def": "a plastic card used to gain access typically to a door", "name": "keycard"}, {"id": 617, "synset": "kilt.n.01", "synonyms": ["kilt"], "def": "a knee-length pleated tartan skirt worn by men as part of the traditional dress in the Highlands of northern Scotland", "name": "kilt"}, {"id": 618, "synset": "kimono.n.01", "synonyms": ["kimono"], "def": "a loose robe; imitated from robes originally worn by Japanese", "name": "kimono"}, {"id": 619, "synset": "kitchen_sink.n.01", "synonyms": ["kitchen_sink"], "def": "a sink in a kitchen", "name": "kitchen_sink"}, {"id": 620, "synset": "kitchen_table.n.01", "synonyms": ["kitchen_table"], "def": "a table in the kitchen", "name": "kitchen_table"}, {"id": 621, "synset": "kite.n.03", "synonyms": ["kite"], "def": "plaything consisting of a light frame covered with tissue paper; flown in wind at end of a string", "name": "kite"}, {"id": 622, "synset": "kitten.n.01", "synonyms": ["kitten", "kitty"], "def": "young domestic cat", "name": "kitten"}, {"id": 623, "synset": "kiwi.n.03", "synonyms": ["kiwi_fruit"], "def": "fuzzy brown egg-shaped fruit with slightly tart green flesh", "name": "kiwi_fruit"}, {"id": 624, "synset": "knee_pad.n.01", "synonyms": ["knee_pad"], "def": "protective garment consisting of a pad worn by football or baseball or hockey players", "name": "knee_pad"}, {"id": 625, "synset": "knife.n.01", "synonyms": ["knife"], "def": "tool with a blade and point used as a cutting instrument", "name": "knife"}, {"id": 626, "synset": "knight.n.02", "synonyms": ["knight_(chess_piece)", "horse_(chess_piece)"], "def": "a chess game piece shaped to resemble the head of a horse", "name": "knight_(chess_piece)"}, {"id": 627, "synset": "knitting_needle.n.01", "synonyms": ["knitting_needle"], "def": "needle consisting of a slender rod with pointed ends; usually used in pairs", "name": "knitting_needle"}, {"id": 628, "synset": "knob.n.02", "synonyms": ["knob"], "def": "a round handle often found on a door", "name": "knob"}, {"id": 629, "synset": "knocker.n.05", "synonyms": ["knocker_(on_a_door)", "doorknocker"], "def": "a device (usually metal and ornamental) attached by a hinge to a door", "name": "knocker_(on_a_door)"}, {"id": 630, "synset": "koala.n.01", "synonyms": ["koala", "koala_bear"], "def": "sluggish tailless Australian marsupial with grey furry ears and coat", "name": "koala"}, {"id": 631, "synset": "lab_coat.n.01", "synonyms": ["lab_coat", "laboratory_coat"], "def": "a light coat worn to protect clothing from substances used while working in a laboratory", "name": "lab_coat"}, {"id": 632, "synset": "ladder.n.01", "synonyms": ["ladder"], "def": "steps consisting of two parallel members connected by rungs", "name": "ladder"}, {"id": 633, "synset": "ladle.n.01", "synonyms": ["ladle"], "def": "a spoon-shaped vessel with a long handle frequently used to transfer liquids", "name": "ladle"}, {"id": 634, "synset": "ladybug.n.01", "synonyms": ["ladybug", "ladybeetle", "ladybird_beetle"], "def": "small round bright-colored and spotted beetle, typically red and black", "name": "ladybug"}, {"id": 635, "synset": "lamb.n.01", "synonyms": ["lamb_(animal)"], "def": "young sheep", "name": "lamb_(animal)"}, {"id": 636, "synset": "lamb_chop.n.01", "synonyms": ["lamb-chop", "lambchop"], "def": "chop cut from a lamb", "name": "lamb-chop"}, {"id": 637, "synset": "lamp.n.02", "synonyms": ["lamp"], "def": "a piece of furniture holding one or more electric light bulbs", "name": "lamp"}, {"id": 638, "synset": "lamppost.n.01", "synonyms": ["lamppost"], "def": "a metal post supporting an outdoor lamp (such as a streetlight)", "name": "lamppost"}, {"id": 639, "synset": "lampshade.n.01", "synonyms": ["lampshade"], "def": "a protective ornamental shade used to screen a light bulb from direct view", "name": "lampshade"}, {"id": 640, "synset": "lantern.n.01", "synonyms": ["lantern"], "def": "light in a transparent protective case", "name": "lantern"}, {"id": 641, "synset": "lanyard.n.02", "synonyms": ["lanyard", "laniard"], "def": "a cord worn around the neck to hold a knife or whistle, etc.", "name": "lanyard"}, {"id": 642, "synset": "laptop.n.01", "synonyms": ["laptop_computer", "notebook_computer"], "def": "a portable computer small enough to use in your lap", "name": "laptop_computer"}, {"id": 643, "synset": "lasagna.n.01", "synonyms": ["lasagna", "lasagne"], "def": "baked dish of layers of lasagna pasta with sauce and cheese and meat or vegetables", "name": "lasagna"}, {"id": 644, "synset": "latch.n.02", "synonyms": ["latch"], "def": "a bar that can be lowered or slid into a groove to fasten a door or gate", "name": "latch"}, {"id": 645, "synset": "lawn_mower.n.01", "synonyms": ["lawn_mower"], "def": "garden tool for mowing grass on lawns", "name": "lawn_mower"}, {"id": 646, "synset": "leather.n.01", "synonyms": ["leather"], "def": "an animal skin made smooth and flexible by removing the hair and then tanning", "name": "leather"}, {"id": 647, "synset": "legging.n.01", "synonyms": ["legging_(clothing)", "leging_(clothing)", "leg_covering"], "def": "a garment covering the leg (usually extending from the knee to the ankle)", "name": "legging_(clothing)"}, {"id": 648, "synset": "lego.n.01", "synonyms": ["Lego", "Lego_set"], "def": "a child's plastic construction set for making models from blocks", "name": "Lego"}, {"id": 649, "synset": "lemon.n.01", "synonyms": ["lemon"], "def": "yellow oval fruit with juicy acidic flesh", "name": "lemon"}, {"id": 650, "synset": "lemonade.n.01", "synonyms": ["lemonade"], "def": "sweetened beverage of diluted lemon juice", "name": "lemonade"}, {"id": 651, "synset": "lettuce.n.02", "synonyms": ["lettuce"], "def": "leafy plant commonly eaten in salad or on sandwiches", "name": "lettuce"}, {"id": 652, "synset": "license_plate.n.01", "synonyms": ["license_plate", "numberplate"], "def": "a plate mounted on the front and back of car and bearing the car's registration number", "name": "license_plate"}, {"id": 653, "synset": "life_buoy.n.01", "synonyms": ["life_buoy", "lifesaver", "life_belt", "life_ring"], "def": "a ring-shaped life preserver used to prevent drowning (NOT a life-jacket or vest)", "name": "life_buoy"}, {"id": 654, "synset": "life_jacket.n.01", "synonyms": ["life_jacket", "life_vest"], "def": "life preserver consisting of a sleeveless jacket of buoyant or inflatable design", "name": "life_jacket"}, {"id": 655, "synset": "light_bulb.n.01", "synonyms": ["lightbulb"], "def": "glass bulb or tube shaped electric device that emits light (DO NOT MARK LAMPS AS A WHOLE)", "name": "lightbulb"}, {"id": 656, "synset": "lightning_rod.n.02", "synonyms": ["lightning_rod", "lightning_conductor"], "def": "a metallic conductor that is attached to a high point and leads to the ground", "name": "lightning_rod"}, {"id": 657, "synset": "lime.n.06", "synonyms": ["lime"], "def": "the green acidic fruit of any of various lime trees", "name": "lime"}, {"id": 658, "synset": "limousine.n.01", "synonyms": ["limousine"], "def": "long luxurious car; usually driven by a chauffeur", "name": "limousine"}, {"id": 659, "synset": "linen.n.02", "synonyms": ["linen_paper"], "def": "a high-quality paper made of linen fibers or with a linen finish", "name": "linen_paper"}, {"id": 660, "synset": "lion.n.01", "synonyms": ["lion"], "def": "large gregarious predatory cat of Africa and India", "name": "lion"}, {"id": 661, "synset": "lip_balm.n.01", "synonyms": ["lip_balm"], "def": "a balm applied to the lips", "name": "lip_balm"}, {"id": 662, "synset": "lipstick.n.01", "synonyms": ["lipstick", "lip_rouge"], "def": "makeup that is used to color the lips", "name": "lipstick"}, {"id": 663, "synset": "liquor.n.01", "synonyms": ["liquor", "spirits", "hard_liquor", "liqueur", "cordial"], "def": "an alcoholic beverage that is distilled rather than fermented", "name": "liquor"}, {"id": 664, "synset": "lizard.n.01", "synonyms": ["lizard"], "def": "a reptile with usually two pairs of legs and a tapering tail", "name": "lizard"}, {"id": 665, "synset": "loafer.n.02", "synonyms": ["Loafer_(type_of_shoe)"], "def": "a low leather step-in shoe", "name": "Loafer_(type_of_shoe)"}, {"id": 666, "synset": "log.n.01", "synonyms": ["log"], "def": "a segment of the trunk of a tree when stripped of branches", "name": "log"}, {"id": 667, "synset": "lollipop.n.02", "synonyms": ["lollipop"], "def": "hard candy on a stick", "name": "lollipop"}, {"id": 668, "synset": "lotion.n.01", "synonyms": ["lotion"], "def": "any of various cosmetic preparations that are applied to the skin", "name": "lotion"}, {"id": 669, "synset": "loudspeaker.n.01", "synonyms": ["speaker_(stero_equipment)"], "def": "electronic device that produces sound often as part of a stereo system", "name": "speaker_(stero_equipment)"}, {"id": 670, "synset": "love_seat.n.01", "synonyms": ["loveseat"], "def": "small sofa that seats two people", "name": "loveseat"}, {"id": 671, "synset": "machine_gun.n.01", "synonyms": ["machine_gun"], "def": "a rapidly firing automatic gun", "name": "machine_gun"}, {"id": 672, "synset": "magazine.n.02", "synonyms": ["magazine"], "def": "a paperback periodic publication", "name": "magazine"}, {"id": 673, "synset": "magnet.n.01", "synonyms": ["magnet"], "def": "a device that attracts iron and produces a magnetic field", "name": "magnet"}, {"id": 674, "synset": "mail_slot.n.01", "synonyms": ["mail_slot"], "def": "a slot (usually in a door) through which mail can be delivered", "name": "mail_slot"}, {"id": 675, "synset": "mailbox.n.01", "synonyms": ["mailbox_(at_home)", "letter_box_(at_home)"], "def": "a private box for delivery of mail", "name": "mailbox_(at_home)"}, {"id": 676, "synset": "mallet.n.01", "synonyms": ["mallet"], "def": "a sports implement with a long handle and a hammer-like head used to hit a ball", "name": "mallet"}, {"id": 677, "synset": "mammoth.n.01", "synonyms": ["mammoth"], "def": "any of numerous extinct elephants widely distributed in the Pleistocene", "name": "mammoth"}, {"id": 678, "synset": "mandarin.n.05", "synonyms": ["mandarin_orange"], "def": "a somewhat flat reddish-orange loose skinned citrus of China", "name": "mandarin_orange"}, {"id": 679, "synset": "manger.n.01", "synonyms": ["manger", "trough"], "def": "a container (usually in a barn or stable) from which cattle or horses feed", "name": "manger"}, {"id": 680, "synset": "manhole.n.01", "synonyms": ["manhole"], "def": "a hole (usually with a flush cover) through which a person can gain access to an underground structure", "name": "manhole"}, {"id": 681, "synset": "map.n.01", "synonyms": ["map"], "def": "a diagrammatic representation of the earth's surface (or part of it)", "name": "map"}, {"id": 682, "synset": "marker.n.03", "synonyms": ["marker"], "def": "a writing implement for making a mark", "name": "marker"}, {"id": 683, "synset": "martini.n.01", "synonyms": ["martini"], "def": "a cocktail made of gin (or vodka) with dry vermouth", "name": "martini"}, {"id": 684, "synset": "mascot.n.01", "synonyms": ["mascot"], "def": "a person or animal that is adopted by a team or other group as a symbolic figure", "name": "mascot"}, {"id": 685, "synset": "mashed_potato.n.01", "synonyms": ["mashed_potato"], "def": "potato that has been peeled and boiled and then mashed", "name": "mashed_potato"}, {"id": 686, "synset": "masher.n.02", "synonyms": ["masher"], "def": "a kitchen utensil used for mashing (e.g. potatoes)", "name": "masher"}, {"id": 687, "synset": "mask.n.04", "synonyms": ["mask", "facemask"], "def": "a protective covering worn over the face", "name": "mask"}, {"id": 688, "synset": "mast.n.01", "synonyms": ["mast"], "def": "a vertical spar for supporting sails", "name": "mast"}, {"id": 689, "synset": "mat.n.03", "synonyms": ["mat_(gym_equipment)", "gym_mat"], "def": "sports equipment consisting of a piece of thick padding on the floor for gymnastics", "name": "mat_(gym_equipment)"}, {"id": 690, "synset": "matchbox.n.01", "synonyms": ["matchbox"], "def": "a box for holding matches", "name": "matchbox"}, {"id": 691, "synset": "mattress.n.01", "synonyms": ["mattress"], "def": "a thick pad filled with resilient material used as a bed or part of a bed", "name": "mattress"}, {"id": 692, "synset": "measuring_cup.n.01", "synonyms": ["measuring_cup"], "def": "graduated cup used to measure liquid or granular ingredients", "name": "measuring_cup"}, {"id": 693, "synset": "measuring_stick.n.01", "synonyms": ["measuring_stick", "ruler_(measuring_stick)", "measuring_rod"], "def": "measuring instrument having a sequence of marks at regular intervals", "name": "measuring_stick"}, {"id": 694, "synset": "meatball.n.01", "synonyms": ["meatball"], "def": "ground meat formed into a ball and fried or simmered in broth", "name": "meatball"}, {"id": 695, "synset": "medicine.n.02", "synonyms": ["medicine"], "def": "something that treats or prevents or alleviates the symptoms of disease", "name": "medicine"}, {"id": 696, "synset": "melon.n.01", "synonyms": ["melon"], "def": "fruit of the gourd family having a hard rind and sweet juicy flesh", "name": "melon"}, {"id": 697, "synset": "microphone.n.01", "synonyms": ["microphone"], "def": "device for converting sound waves into electrical energy", "name": "microphone"}, {"id": 698, "synset": "microscope.n.01", "synonyms": ["microscope"], "def": "magnifier of the image of small objects", "name": "microscope"}, {"id": 699, "synset": "microwave.n.02", "synonyms": ["microwave_oven"], "def": "kitchen appliance that cooks food by passing an electromagnetic wave through it", "name": "microwave_oven"}, {"id": 700, "synset": "milestone.n.01", "synonyms": ["milestone", "milepost"], "def": "stone post at side of a road to show distances", "name": "milestone"}, {"id": 701, "synset": "milk.n.01", "synonyms": ["milk"], "def": "a white nutritious liquid secreted by mammals and used as food by human beings", "name": "milk"}, {"id": 702, "synset": "minivan.n.01", "synonyms": ["minivan"], "def": "a small box-shaped passenger van", "name": "minivan"}, {"id": 703, "synset": "mint.n.05", "synonyms": ["mint_candy"], "def": "a candy that is flavored with a mint oil", "name": "mint_candy"}, {"id": 704, "synset": "mirror.n.01", "synonyms": ["mirror"], "def": "polished surface that forms images by reflecting light", "name": "mirror"}, {"id": 705, "synset": "mitten.n.01", "synonyms": ["mitten"], "def": "glove that encases the thumb separately and the other four fingers together", "name": "mitten"}, {"id": 706, "synset": "mixer.n.04", "synonyms": ["mixer_(kitchen_tool)", "stand_mixer"], "def": "a kitchen utensil that is used for mixing foods", "name": "mixer_(kitchen_tool)"}, {"id": 707, "synset": "money.n.03", "synonyms": ["money"], "def": "the official currency issued by a government or national bank", "name": "money"}, {"id": 708, "synset": "monitor.n.04", "synonyms": ["monitor_(computer_equipment) computer_monitor"], "def": "a computer monitor", "name": "monitor_(computer_equipment) computer_monitor"}, {"id": 709, "synset": "monkey.n.01", "synonyms": ["monkey"], "def": "any of various long-tailed primates", "name": "monkey"}, {"id": 710, "synset": "motor.n.01", "synonyms": ["motor"], "def": "machine that converts other forms of energy into mechanical energy and so imparts motion", "name": "motor"}, {"id": 711, "synset": "motor_scooter.n.01", "synonyms": ["motor_scooter", "scooter"], "def": "a wheeled vehicle with small wheels and a low-powered engine", "name": "motor_scooter"}, {"id": 712, "synset": "motor_vehicle.n.01", "synonyms": ["motor_vehicle", "automotive_vehicle"], "def": "a self-propelled wheeled vehicle that does not run on rails", "name": "motor_vehicle"}, {"id": 713, "synset": "motorboat.n.01", "synonyms": ["motorboat", "powerboat"], "def": "a boat propelled by an internal-combustion engine", "name": "motorboat"}, {"id": 714, "synset": "motorcycle.n.01", "synonyms": ["motorcycle"], "def": "a motor vehicle with two wheels and a strong frame", "name": "motorcycle"}, {"id": 715, "synset": "mound.n.01", "synonyms": ["mound_(baseball)", "pitcher's_mound"], "def": "(baseball) the slight elevation on which the pitcher stands", "name": "mound_(baseball)"}, {"id": 716, "synset": "mouse.n.01", "synonyms": ["mouse_(animal_rodent)"], "def": "a small rodent with pointed snouts and small ears on elongated bodies with slender usually hairless tails", "name": "mouse_(animal_rodent)"}, {"id": 717, "synset": "mouse.n.04", "synonyms": ["mouse_(computer_equipment)", "computer_mouse"], "def": "a computer input device that controls an on-screen pointer", "name": "mouse_(computer_equipment)"}, {"id": 718, "synset": "mousepad.n.01", "synonyms": ["mousepad"], "def": "a small portable pad that provides an operating surface for a computer mouse", "name": "mousepad"}, {"id": 719, "synset": "muffin.n.01", "synonyms": ["muffin"], "def": "a sweet quick bread baked in a cup-shaped pan", "name": "muffin"}, {"id": 720, "synset": "mug.n.04", "synonyms": ["mug"], "def": "with handle and usually cylindrical", "name": "mug"}, {"id": 721, "synset": "mushroom.n.02", "synonyms": ["mushroom"], "def": "a common mushroom", "name": "mushroom"}, {"id": 722, "synset": "music_stool.n.01", "synonyms": ["music_stool", "piano_stool"], "def": "a stool for piano players; usually adjustable in height", "name": "music_stool"}, {"id": 723, "synset": "musical_instrument.n.01", "synonyms": ["musical_instrument", "instrument_(musical)"], "def": "any of various devices or contrivances that can be used to produce musical tones or sounds", "name": "musical_instrument"}, {"id": 724, "synset": "nailfile.n.01", "synonyms": ["nailfile"], "def": "a small flat file for shaping the nails", "name": "nailfile"}, {"id": 725, "synset": "nameplate.n.01", "synonyms": ["nameplate"], "def": "a plate bearing a name", "name": "nameplate"}, {"id": 726, "synset": "napkin.n.01", "synonyms": ["napkin", "table_napkin", "serviette"], "def": "a small piece of table linen or paper that is used to wipe the mouth and to cover the lap in order to protect clothing", "name": "napkin"}, {"id": 727, "synset": "neckerchief.n.01", "synonyms": ["neckerchief"], "def": "a kerchief worn around the neck", "name": "neckerchief"}, {"id": 728, "synset": "necklace.n.01", "synonyms": ["necklace"], "def": "jewelry consisting of a cord or chain (often bearing gems) worn about the neck as an ornament", "name": "necklace"}, {"id": 729, "synset": "necktie.n.01", "synonyms": ["necktie", "tie_(necktie)"], "def": "neckwear consisting of a long narrow piece of material worn under a collar and tied in knot at the front", "name": "necktie"}, {"id": 730, "synset": "needle.n.03", "synonyms": ["needle"], "def": "a sharp pointed implement (usually metal)", "name": "needle"}, {"id": 731, "synset": "nest.n.01", "synonyms": ["nest"], "def": "a structure in which animals lay eggs or give birth to their young", "name": "nest"}, {"id": 732, "synset": "newsstand.n.01", "synonyms": ["newsstand"], "def": "a stall where newspapers and other periodicals are sold", "name": "newsstand"}, {"id": 733, "synset": "nightwear.n.01", "synonyms": ["nightshirt", "nightwear", "sleepwear", "nightclothes"], "def": "garments designed to be worn in bed", "name": "nightshirt"}, {"id": 734, "synset": "nosebag.n.01", "synonyms": ["nosebag_(for_animals)", "feedbag"], "def": "a canvas bag that is used to feed an animal (such as a horse); covers the muzzle and fastens at the top of the head", "name": "nosebag_(for_animals)"}, {"id": 735, "synset": "noseband.n.01", "synonyms": ["noseband_(for_animals)", "nosepiece_(for_animals)"], "def": "a strap that is the part of a bridle that goes over the animal's nose", "name": "noseband_(for_animals)"}, {"id": 736, "synset": "notebook.n.01", "synonyms": ["notebook"], "def": "a book with blank pages for recording notes or memoranda", "name": "notebook"}, {"id": 737, "synset": "notepad.n.01", "synonyms": ["notepad"], "def": "a pad of paper for keeping notes", "name": "notepad"}, {"id": 738, "synset": "nut.n.03", "synonyms": ["nut"], "def": "a small metal block (usually square or hexagonal) with internal screw thread to be fitted onto a bolt", "name": "nut"}, {"id": 739, "synset": "nutcracker.n.01", "synonyms": ["nutcracker"], "def": "a hand tool used to crack nuts open", "name": "nutcracker"}, {"id": 740, "synset": "oar.n.01", "synonyms": ["oar"], "def": "an implement used to propel or steer a boat", "name": "oar"}, {"id": 741, "synset": "octopus.n.01", "synonyms": ["octopus_(food)"], "def": "tentacles of octopus prepared as food", "name": "octopus_(food)"}, {"id": 742, "synset": "octopus.n.02", "synonyms": ["octopus_(animal)"], "def": "bottom-living cephalopod having a soft oval body with eight long tentacles", "name": "octopus_(animal)"}, {"id": 743, "synset": "oil_lamp.n.01", "synonyms": ["oil_lamp", "kerosene_lamp", "kerosine_lamp"], "def": "a lamp that burns oil (as kerosine) for light", "name": "oil_lamp"}, {"id": 744, "synset": "olive_oil.n.01", "synonyms": ["olive_oil"], "def": "oil from olives", "name": "olive_oil"}, {"id": 745, "synset": "omelet.n.01", "synonyms": ["omelet", "omelette"], "def": "beaten eggs cooked until just set; may be folded around e.g. ham or cheese or jelly", "name": "omelet"}, {"id": 746, "synset": "onion.n.01", "synonyms": ["onion"], "def": "the bulb of an onion plant", "name": "onion"}, {"id": 747, "synset": "orange.n.01", "synonyms": ["orange_(fruit)"], "def": "orange (FRUIT of an orange tree)", "name": "orange_(fruit)"}, {"id": 748, "synset": "orange_juice.n.01", "synonyms": ["orange_juice"], "def": "bottled or freshly squeezed juice of oranges", "name": "orange_juice"}, {"id": 749, "synset": "oregano.n.01", "synonyms": ["oregano", "marjoram"], "def": "aromatic Eurasian perennial herb used in cooking and baking", "name": "oregano"}, {"id": 750, "synset": "ostrich.n.02", "synonyms": ["ostrich"], "def": "fast-running African flightless bird with two-toed feet; largest living bird", "name": "ostrich"}, {"id": 751, "synset": "ottoman.n.03", "synonyms": ["ottoman", "pouf", "pouffe", "hassock"], "def": "thick cushion used as a seat", "name": "ottoman"}, {"id": 752, "synset": "overall.n.01", "synonyms": ["overalls_(clothing)"], "def": "work clothing consisting of denim trousers usually with a bib and shoulder straps", "name": "overalls_(clothing)"}, {"id": 753, "synset": "owl.n.01", "synonyms": ["owl"], "def": "nocturnal bird of prey with hawk-like beak and claws and large head with front-facing eyes", "name": "owl"}, {"id": 754, "synset": "packet.n.03", "synonyms": ["packet"], "def": "a small package or bundle", "name": "packet"}, {"id": 755, "synset": "pad.n.03", "synonyms": ["inkpad", "inking_pad", "stamp_pad"], "def": "absorbent material saturated with ink used to transfer ink evenly to a rubber stamp", "name": "inkpad"}, {"id": 756, "synset": "pad.n.04", "synonyms": ["pad"], "def": "a flat mass of soft material used for protection, stuffing, or comfort", "name": "pad"}, {"id": 757, "synset": "paddle.n.04", "synonyms": ["paddle", "boat_paddle"], "def": "a short light oar used without an oarlock to propel a canoe or small boat", "name": "paddle"}, {"id": 758, "synset": "padlock.n.01", "synonyms": ["padlock"], "def": "a detachable, portable lock", "name": "padlock"}, {"id": 759, "synset": "paintbox.n.01", "synonyms": ["paintbox"], "def": "a box containing a collection of cubes or tubes of artists' paint", "name": "paintbox"}, {"id": 760, "synset": "paintbrush.n.01", "synonyms": ["paintbrush"], "def": "a brush used as an applicator to apply paint", "name": "paintbrush"}, {"id": 761, "synset": "painting.n.01", "synonyms": ["painting"], "def": "graphic art consisting of an artistic composition made by applying paints to a surface", "name": "painting"}, {"id": 762, "synset": "pajama.n.02", "synonyms": ["pajamas", "pyjamas"], "def": "loose-fitting nightclothes worn for sleeping or lounging", "name": "pajamas"}, {"id": 763, "synset": "palette.n.02", "synonyms": ["palette", "pallet"], "def": "board that provides a flat surface on which artists mix paints and the range of colors used", "name": "palette"}, {"id": 764, "synset": "pan.n.01", "synonyms": ["pan_(for_cooking)", "cooking_pan"], "def": "cooking utensil consisting of a wide metal vessel", "name": "pan_(for_cooking)"}, {"id": 765, "synset": "pan.n.03", "synonyms": ["pan_(metal_container)"], "def": "shallow container made of metal", "name": "pan_(metal_container)"}, {"id": 766, "synset": "pancake.n.01", "synonyms": ["pancake"], "def": "a flat cake of thin batter fried on both sides on a griddle", "name": "pancake"}, {"id": 767, "synset": "pantyhose.n.01", "synonyms": ["pantyhose"], "def": "a woman's tights consisting of underpants and stockings", "name": "pantyhose"}, {"id": 768, "synset": "papaya.n.02", "synonyms": ["papaya"], "def": "large oval melon-like tropical fruit with yellowish flesh", "name": "papaya"}, {"id": 769, "synset": "paper_clip.n.01", "synonyms": ["paperclip"], "def": "a wire or plastic clip for holding sheets of paper together", "name": "paperclip"}, {"id": 770, "synset": "paper_plate.n.01", "synonyms": ["paper_plate"], "def": "a disposable plate made of cardboard", "name": "paper_plate"}, {"id": 771, "synset": "paper_towel.n.01", "synonyms": ["paper_towel"], "def": "a disposable towel made of absorbent paper", "name": "paper_towel"}, {"id": 772, "synset": "paperback_book.n.01", "synonyms": ["paperback_book", "paper-back_book", "softback_book", "soft-cover_book"], "def": "a book with paper covers", "name": "paperback_book"}, {"id": 773, "synset": "paperweight.n.01", "synonyms": ["paperweight"], "def": "a weight used to hold down a stack of papers", "name": "paperweight"}, {"id": 774, "synset": "parachute.n.01", "synonyms": ["parachute"], "def": "rescue equipment consisting of a device that fills with air and retards your fall", "name": "parachute"}, {"id": 775, "synset": "parakeet.n.01", "synonyms": ["parakeet", "parrakeet", "parroket", "paraquet", "paroquet", "parroquet"], "def": "any of numerous small slender long-tailed parrots", "name": "parakeet"}, {"id": 776, "synset": "parasail.n.01", "synonyms": ["parasail_(sports)"], "def": "parachute that will lift a person up into the air when it is towed by a motorboat or a car", "name": "parasail_(sports)"}, {"id": 777, "synset": "parchment.n.01", "synonyms": ["parchment"], "def": "a superior paper resembling sheepskin", "name": "parchment"}, {"id": 778, "synset": "parka.n.01", "synonyms": ["parka", "anorak"], "def": "a kind of heavy jacket (`windcheater' is a British term)", "name": "parka"}, {"id": 779, "synset": "parking_meter.n.01", "synonyms": ["parking_meter"], "def": "a coin-operated timer located next to a parking space", "name": "parking_meter"}, {"id": 780, "synset": "parrot.n.01", "synonyms": ["parrot"], "def": "usually brightly colored tropical birds with short hooked beaks and the ability to mimic sounds", "name": "parrot"}, {"id": 781, "synset": "passenger_car.n.01", "synonyms": ["passenger_car_(part_of_a_train)", "coach_(part_of_a_train)"], "def": "a railcar where passengers ride", "name": "passenger_car_(part_of_a_train)"}, {"id": 782, "synset": "passenger_ship.n.01", "synonyms": ["passenger_ship"], "def": "a ship built to carry passengers", "name": "passenger_ship"}, {"id": 783, "synset": "passport.n.02", "synonyms": ["passport"], "def": "a document issued by a country to a citizen allowing that person to travel abroad and re-enter the home country", "name": "passport"}, {"id": 784, "synset": "pastry.n.02", "synonyms": ["pastry"], "def": "any of various baked foods made of dough or batter", "name": "pastry"}, {"id": 785, "synset": "patty.n.01", "synonyms": ["patty_(food)"], "def": "small flat mass of chopped food", "name": "patty_(food)"}, {"id": 786, "synset": "pea.n.01", "synonyms": ["pea_(food)"], "def": "seed of a pea plant used for food", "name": "pea_(food)"}, {"id": 787, "synset": "peach.n.03", "synonyms": ["peach"], "def": "downy juicy fruit with sweet yellowish or whitish flesh", "name": "peach"}, {"id": 788, "synset": "peanut_butter.n.01", "synonyms": ["peanut_butter"], "def": "a spread made from ground peanuts", "name": "peanut_butter"}, {"id": 789, "synset": "pear.n.01", "synonyms": ["pear"], "def": "sweet juicy gritty-textured fruit available in many varieties", "name": "pear"}, {"id": 790, "synset": "peeler.n.03", "synonyms": ["peeler_(tool_for_fruit_and_vegetables)"], "def": "a device for peeling vegetables or fruits", "name": "peeler_(tool_for_fruit_and_vegetables)"}, {"id": 791, "synset": "pegboard.n.01", "synonyms": ["pegboard"], "def": "a board perforated with regularly spaced holes into which pegs can be fitted", "name": "pegboard"}, {"id": 792, "synset": "pelican.n.01", "synonyms": ["pelican"], "def": "large long-winged warm-water seabird having a large bill with a distensible pouch for fish", "name": "pelican"}, {"id": 793, "synset": "pen.n.01", "synonyms": ["pen"], "def": "a writing implement with a point from which ink flows", "name": "pen"}, {"id": 794, "synset": "pencil.n.01", "synonyms": ["pencil"], "def": "a thin cylindrical pointed writing implement made of wood and graphite", "name": "pencil"}, {"id": 795, "synset": "pencil_box.n.01", "synonyms": ["pencil_box", "pencil_case"], "def": "a box for holding pencils", "name": "pencil_box"}, {"id": 796, "synset": "pencil_sharpener.n.01", "synonyms": ["pencil_sharpener"], "def": "a rotary implement for sharpening the point on pencils", "name": "pencil_sharpener"}, {"id": 797, "synset": "pendulum.n.01", "synonyms": ["pendulum"], "def": "an apparatus consisting of an object mounted so that it swings freely under the influence of gravity", "name": "pendulum"}, {"id": 798, "synset": "penguin.n.01", "synonyms": ["penguin"], "def": "short-legged flightless birds of cold southern regions having webbed feet and wings modified as flippers", "name": "penguin"}, {"id": 799, "synset": "pennant.n.02", "synonyms": ["pennant"], "def": "a flag longer than it is wide (and often tapering)", "name": "pennant"}, {"id": 800, "synset": "penny.n.02", "synonyms": ["penny_(coin)"], "def": "a coin worth one-hundredth of the value of the basic unit", "name": "penny_(coin)"}, {"id": 801, "synset": "pepper.n.03", "synonyms": ["pepper", "peppercorn"], "def": "pungent seasoning from the berry of the common pepper plant; whole or ground", "name": "pepper"}, {"id": 802, "synset": "pepper_mill.n.01", "synonyms": ["pepper_mill", "pepper_grinder"], "def": "a mill for grinding pepper", "name": "pepper_mill"}, {"id": 803, "synset": "perfume.n.02", "synonyms": ["perfume"], "def": "a toiletry that emits and diffuses a fragrant odor", "name": "perfume"}, {"id": 804, "synset": "persimmon.n.02", "synonyms": ["persimmon"], "def": "orange fruit resembling a plum; edible when fully ripe", "name": "persimmon"}, {"id": 805, "synset": "person.n.01", "synonyms": ["baby", "child", "boy", "girl", "man", "woman", "person", "human"], "def": "a human being", "name": "baby"}, {"id": 806, "synset": "pet.n.01", "synonyms": ["pet"], "def": "a domesticated animal kept for companionship or amusement", "name": "pet"}, {"id": 807, "synset": "petfood.n.01", "synonyms": ["petfood", "pet-food"], "def": "food prepared for animal pets", "name": "petfood"}, {"id": 808, "synset": "pew.n.01", "synonyms": ["pew_(church_bench)", "church_bench"], "def": "long bench with backs; used in church by the congregation", "name": "pew_(church_bench)"}, {"id": 809, "synset": "phonebook.n.01", "synonyms": ["phonebook", "telephone_book", "telephone_directory"], "def": "a directory containing an alphabetical list of telephone subscribers and their telephone numbers", "name": "phonebook"}, {"id": 810, "synset": "phonograph_record.n.01", "synonyms": ["phonograph_record", "phonograph_recording", "record_(phonograph_recording)"], "def": "sound recording consisting of a typically black disk with a continuous groove", "name": "phonograph_record"}, {"id": 811, "synset": "piano.n.01", "synonyms": ["piano"], "def": "a keyboard instrument that is played by depressing keys that cause hammers to strike tuned strings and produce sounds", "name": "piano"}, {"id": 812, "synset": "pickle.n.01", "synonyms": ["pickle"], "def": "vegetables (especially cucumbers) preserved in brine or vinegar", "name": "pickle"}, {"id": 813, "synset": "pickup.n.01", "synonyms": ["pickup_truck"], "def": "a light truck with an open body and low sides and a tailboard", "name": "pickup_truck"}, {"id": 814, "synset": "pie.n.01", "synonyms": ["pie"], "def": "dish baked in pastry-lined pan often with a pastry top", "name": "pie"}, {"id": 815, "synset": "pigeon.n.01", "synonyms": ["pigeon"], "def": "wild and domesticated birds having a heavy body and short legs", "name": "pigeon"}, {"id": 816, "synset": "piggy_bank.n.01", "synonyms": ["piggy_bank", "penny_bank"], "def": "a child's coin bank (often shaped like a pig)", "name": "piggy_bank"}, {"id": 817, "synset": "pillow.n.01", "synonyms": ["pillow"], "def": "a cushion to support the head of a sleeping person", "name": "pillow"}, {"id": 818, "synset": "pin.n.09", "synonyms": ["pin_(non_jewelry)"], "def": "a small slender (often pointed) piece of wood or metal used to support or fasten or attach things", "name": "pin_(non_jewelry)"}, {"id": 819, "synset": "pineapple.n.02", "synonyms": ["pineapple"], "def": "large sweet fleshy tropical fruit with a tuft of stiff leaves", "name": "pineapple"}, {"id": 820, "synset": "pinecone.n.01", "synonyms": ["pinecone"], "def": "the seed-producing cone of a pine tree", "name": "pinecone"}, {"id": 821, "synset": "ping-pong_ball.n.01", "synonyms": ["ping-pong_ball"], "def": "light hollow ball used in playing table tennis", "name": "ping-pong_ball"}, {"id": 822, "synset": "pinwheel.n.03", "synonyms": ["pinwheel"], "def": "a toy consisting of vanes of colored paper or plastic that is pinned to a stick and spins when it is pointed into the wind", "name": "pinwheel"}, {"id": 823, "synset": "pipe.n.01", "synonyms": ["tobacco_pipe"], "def": "a tube with a small bowl at one end; used for smoking tobacco", "name": "tobacco_pipe"}, {"id": 824, "synset": "pipe.n.02", "synonyms": ["pipe", "piping"], "def": "a long tube made of metal or plastic that is used to carry water or oil or gas etc.", "name": "pipe"}, {"id": 825, "synset": "pistol.n.01", "synonyms": ["pistol", "handgun"], "def": "a firearm that is held and fired with one hand", "name": "pistol"}, {"id": 826, "synset": "pita.n.01", "synonyms": ["pita_(bread)", "pocket_bread"], "def": "usually small round bread that can open into a pocket for filling", "name": "pita_(bread)"}, {"id": 827, "synset": "pitcher.n.02", "synonyms": ["pitcher_(vessel_for_liquid)", "ewer"], "def": "an open vessel with a handle and a spout for pouring", "name": "pitcher_(vessel_for_liquid)"}, {"id": 828, "synset": "pitchfork.n.01", "synonyms": ["pitchfork"], "def": "a long-handled hand tool with sharp widely spaced prongs for lifting and pitching hay", "name": "pitchfork"}, {"id": 829, "synset": "pizza.n.01", "synonyms": ["pizza"], "def": "Italian open pie made of thin bread dough spread with a spiced mixture of e.g. tomato sauce and cheese", "name": "pizza"}, {"id": 830, "synset": "place_mat.n.01", "synonyms": ["place_mat"], "def": "a mat placed on a table for an individual place setting", "name": "place_mat"}, {"id": 831, "synset": "plate.n.04", "synonyms": ["plate"], "def": "dish on which food is served or from which food is eaten", "name": "plate"}, {"id": 832, "synset": "platter.n.01", "synonyms": ["platter"], "def": "a large shallow dish used for serving food", "name": "platter"}, {"id": 833, "synset": "playing_card.n.01", "synonyms": ["playing_card"], "def": "one of a pack of cards that are used to play card games", "name": "playing_card"}, {"id": 834, "synset": "playpen.n.01", "synonyms": ["playpen"], "def": "a portable enclosure in which babies may be left to play", "name": "playpen"}, {"id": 835, "synset": "pliers.n.01", "synonyms": ["pliers", "plyers"], "def": "a gripping hand tool with two hinged arms and (usually) serrated jaws", "name": "pliers"}, {"id": 836, "synset": "plow.n.01", "synonyms": ["plow_(farm_equipment)", "plough_(farm_equipment)"], "def": "a farm tool having one or more heavy blades to break the soil and cut a furrow prior to sowing", "name": "plow_(farm_equipment)"}, {"id": 837, "synset": "pocket_watch.n.01", "synonyms": ["pocket_watch"], "def": "a watch that is carried in a small watch pocket", "name": "pocket_watch"}, {"id": 838, "synset": "pocketknife.n.01", "synonyms": ["pocketknife"], "def": "a knife with a blade that folds into the handle; suitable for carrying in the pocket", "name": "pocketknife"}, {"id": 839, "synset": "poker.n.01", "synonyms": ["poker_(fire_stirring_tool)", "stove_poker", "fire_hook"], "def": "fire iron consisting of a metal rod with a handle; used to stir a fire", "name": "poker_(fire_stirring_tool)"}, {"id": 840, "synset": "pole.n.01", "synonyms": ["pole", "post"], "def": "a long (usually round) rod of wood or metal or plastic", "name": "pole"}, {"id": 841, "synset": "police_van.n.01", "synonyms": ["police_van", "police_wagon", "paddy_wagon", "patrol_wagon"], "def": "van used by police to transport prisoners", "name": "police_van"}, {"id": 842, "synset": "polo_shirt.n.01", "synonyms": ["polo_shirt", "sport_shirt"], "def": "a shirt with short sleeves designed for comfort and casual wear", "name": "polo_shirt"}, {"id": 843, "synset": "poncho.n.01", "synonyms": ["poncho"], "def": "a blanket-like cloak with a hole in the center for the head", "name": "poncho"}, {"id": 844, "synset": "pony.n.05", "synonyms": ["pony"], "def": "any of various breeds of small gentle horses usually less than five feet high at the shoulder", "name": "pony"}, {"id": 845, "synset": "pool_table.n.01", "synonyms": ["pool_table", "billiard_table", "snooker_table"], "def": "game equipment consisting of a heavy table on which pool is played", "name": "pool_table"}, {"id": 846, "synset": "pop.n.02", "synonyms": ["pop_(soda)", "soda_(pop)", "tonic", "soft_drink"], "def": "a sweet drink containing carbonated water and flavoring", "name": "pop_(soda)"}, {"id": 847, "synset": "portrait.n.02", "synonyms": ["portrait", "portrayal"], "def": "any likeness of a person, in any medium", "name": "portrait"}, {"id": 848, "synset": "postbox.n.01", "synonyms": ["postbox_(public)", "mailbox_(public)"], "def": "public box for deposit of mail", "name": "postbox_(public)"}, {"id": 849, "synset": "postcard.n.01", "synonyms": ["postcard", "postal_card", "mailing-card"], "def": "a card for sending messages by post without an envelope", "name": "postcard"}, {"id": 850, "synset": "poster.n.01", "synonyms": ["poster", "placard"], "def": "a sign posted in a public place as an advertisement", "name": "poster"}, {"id": 851, "synset": "pot.n.01", "synonyms": ["pot"], "def": "metal or earthenware cooking vessel that is usually round and deep; often has a handle and lid", "name": "pot"}, {"id": 852, "synset": "pot.n.04", "synonyms": ["flowerpot"], "def": "a container in which plants are cultivated", "name": "flowerpot"}, {"id": 853, "synset": "potato.n.01", "synonyms": ["potato"], "def": "an edible tuber native to South America", "name": "potato"}, {"id": 854, "synset": "potholder.n.01", "synonyms": ["potholder"], "def": "an insulated pad for holding hot pots", "name": "potholder"}, {"id": 855, "synset": "pottery.n.01", "synonyms": ["pottery", "clayware"], "def": "ceramic ware made from clay and baked in a kiln", "name": "pottery"}, {"id": 856, "synset": "pouch.n.01", "synonyms": ["pouch"], "def": "a small or medium size container for holding or carrying things", "name": "pouch"}, {"id": 857, "synset": "power_shovel.n.01", "synonyms": ["power_shovel", "excavator", "digger"], "def": "a machine for excavating", "name": "power_shovel"}, {"id": 858, "synset": "prawn.n.01", "synonyms": ["prawn", "shrimp"], "def": "any of various edible decapod crustaceans", "name": "prawn"}, {"id": 859, "synset": "printer.n.03", "synonyms": ["printer", "printing_machine"], "def": "a machine that prints", "name": "printer"}, {"id": 860, "synset": "projectile.n.01", "synonyms": ["projectile_(weapon)", "missile"], "def": "a weapon that is forcibly thrown or projected at a targets", "name": "projectile_(weapon)"}, {"id": 861, "synset": "projector.n.02", "synonyms": ["projector"], "def": "an optical instrument that projects an enlarged image onto a screen", "name": "projector"}, {"id": 862, "synset": "propeller.n.01", "synonyms": ["propeller", "propellor"], "def": "a mechanical device that rotates to push against air or water", "name": "propeller"}, {"id": 863, "synset": "prune.n.01", "synonyms": ["prune"], "def": "dried plum", "name": "prune"}, {"id": 864, "synset": "pudding.n.01", "synonyms": ["pudding"], "def": "any of various soft thick unsweetened baked dishes", "name": "pudding"}, {"id": 865, "synset": "puffer.n.02", "synonyms": ["puffer_(fish)", "pufferfish", "blowfish", "globefish"], "def": "fishes whose elongated spiny body can inflate itself with water or air to form a globe", "name": "puffer_(fish)"}, {"id": 866, "synset": "puffin.n.01", "synonyms": ["puffin"], "def": "seabirds having short necks and brightly colored compressed bills", "name": "puffin"}, {"id": 867, "synset": "pug.n.01", "synonyms": ["pug-dog"], "def": "small compact smooth-coated breed of Asiatic origin having a tightly curled tail and broad flat wrinkled muzzle", "name": "pug-dog"}, {"id": 868, "synset": "pumpkin.n.02", "synonyms": ["pumpkin"], "def": "usually large pulpy deep-yellow round fruit of the squash family maturing in late summer or early autumn", "name": "pumpkin"}, {"id": 869, "synset": "punch.n.03", "synonyms": ["puncher"], "def": "a tool for making holes or indentations", "name": "puncher"}, {"id": 870, "synset": "puppet.n.01", "synonyms": ["puppet", "marionette"], "def": "a small figure of a person operated from above with strings by a puppeteer", "name": "puppet"}, {"id": 871, "synset": "puppy.n.01", "synonyms": ["puppy"], "def": "a young dog", "name": "puppy"}, {"id": 872, "synset": "quesadilla.n.01", "synonyms": ["quesadilla"], "def": "a tortilla that is filled with cheese and heated", "name": "quesadilla"}, {"id": 873, "synset": "quiche.n.02", "synonyms": ["quiche"], "def": "a tart filled with rich unsweetened custard; often contains other ingredients (as cheese or ham or seafood or vegetables)", "name": "quiche"}, {"id": 874, "synset": "quilt.n.01", "synonyms": ["quilt", "comforter"], "def": "bedding made of two layers of cloth filled with stuffing and stitched together", "name": "quilt"}, {"id": 875, "synset": "rabbit.n.01", "synonyms": ["rabbit"], "def": "any of various burrowing animals of the family Leporidae having long ears and short tails", "name": "rabbit"}, {"id": 876, "synset": "racer.n.02", "synonyms": ["race_car", "racing_car"], "def": "a fast car that competes in races", "name": "race_car"}, {"id": 877, "synset": "racket.n.04", "synonyms": ["racket", "racquet"], "def": "a sports implement used to strike a ball in various games", "name": "racket"}, {"id": 878, "synset": "radar.n.01", "synonyms": ["radar"], "def": "measuring instrument in which the echo of a pulse of microwave radiation is used to detect and locate distant objects", "name": "radar"}, {"id": 879, "synset": "radiator.n.03", "synonyms": ["radiator"], "def": "a mechanism consisting of a metal honeycomb through which hot fluids circulate", "name": "radiator"}, {"id": 880, "synset": "radio_receiver.n.01", "synonyms": ["radio_receiver", "radio_set", "radio", "tuner_(radio)"], "def": "an electronic receiver that detects and demodulates and amplifies transmitted radio signals", "name": "radio_receiver"}, {"id": 881, "synset": "radish.n.03", "synonyms": ["radish", "daikon"], "def": "pungent edible root of any of various cultivated radish plants", "name": "radish"}, {"id": 882, "synset": "raft.n.01", "synonyms": ["raft"], "def": "a flat float (usually made of logs or planks) that can be used for transport or as a platform for swimmers", "name": "raft"}, {"id": 883, "synset": "rag_doll.n.01", "synonyms": ["rag_doll"], "def": "a cloth doll that is stuffed and (usually) painted", "name": "rag_doll"}, {"id": 884, "synset": "raincoat.n.01", "synonyms": ["raincoat", "waterproof_jacket"], "def": "a water-resistant coat", "name": "raincoat"}, {"id": 885, "synset": "ram.n.05", "synonyms": ["ram_(animal)"], "def": "uncastrated adult male sheep", "name": "ram_(animal)"}, {"id": 886, "synset": "raspberry.n.02", "synonyms": ["raspberry"], "def": "red or black edible aggregate berries usually smaller than the related blackberries", "name": "raspberry"}, {"id": 887, "synset": "rat.n.01", "synonyms": ["rat"], "def": "any of various long-tailed rodents similar to but larger than a mouse", "name": "rat"}, {"id": 888, "synset": "razorblade.n.01", "synonyms": ["razorblade"], "def": "a blade that has very sharp edge", "name": "razorblade"}, {"id": 889, "synset": "reamer.n.01", "synonyms": ["reamer_(juicer)", "juicer", "juice_reamer"], "def": "a squeezer with a conical ridged center that is used for squeezing juice from citrus fruit", "name": "reamer_(juicer)"}, {"id": 890, "synset": "rearview_mirror.n.01", "synonyms": ["rearview_mirror"], "def": "car mirror that reflects the view out of the rear window", "name": "rearview_mirror"}, {"id": 891, "synset": "receipt.n.02", "synonyms": ["receipt"], "def": "an acknowledgment (usually tangible) that payment has been made", "name": "receipt"}, {"id": 892, "synset": "recliner.n.01", "synonyms": ["recliner", "reclining_chair", "lounger_(chair)"], "def": "an armchair whose back can be lowered and foot can be raised to allow the sitter to recline in it", "name": "recliner"}, {"id": 893, "synset": "record_player.n.01", "synonyms": ["record_player", "phonograph_(record_player)", "turntable"], "def": "machine in which rotating records cause a stylus to vibrate and the vibrations are amplified acoustically or electronically", "name": "record_player"}, {"id": 894, "synset": "red_cabbage.n.02", "synonyms": ["red_cabbage"], "def": "compact head of purplish-red leaves", "name": "red_cabbage"}, {"id": 895, "synset": "reflector.n.01", "synonyms": ["reflector"], "def": "device that reflects light, radiation, etc.", "name": "reflector"}, {"id": 896, "synset": "remote_control.n.01", "synonyms": ["remote_control"], "def": "a device that can be used to control a machine or apparatus from a distance", "name": "remote_control"}, {"id": 897, "synset": "rhinoceros.n.01", "synonyms": ["rhinoceros"], "def": "massive powerful herbivorous odd-toed ungulate of southeast Asia and Africa having very thick skin and one or two horns on the snout", "name": "rhinoceros"}, {"id": 898, "synset": "rib.n.03", "synonyms": ["rib_(food)"], "def": "cut of meat including one or more ribs", "name": "rib_(food)"}, {"id": 899, "synset": "rifle.n.01", "synonyms": ["rifle"], "def": "a shoulder firearm with a long barrel", "name": "rifle"}, {"id": 900, "synset": "ring.n.08", "synonyms": ["ring"], "def": "jewelry consisting of a circlet of precious metal (often set with jewels) worn on the finger", "name": "ring"}, {"id": 901, "synset": "river_boat.n.01", "synonyms": ["river_boat"], "def": "a boat used on rivers or to ply a river", "name": "river_boat"}, {"id": 902, "synset": "road_map.n.02", "synonyms": ["road_map"], "def": "(NOT A ROAD) a MAP showing roads (for automobile travel)", "name": "road_map"}, {"id": 903, "synset": "robe.n.01", "synonyms": ["robe"], "def": "any loose flowing garment", "name": "robe"}, {"id": 904, "synset": "rocking_chair.n.01", "synonyms": ["rocking_chair"], "def": "a chair mounted on rockers", "name": "rocking_chair"}, {"id": 905, "synset": "roller_skate.n.01", "synonyms": ["roller_skate"], "def": "a shoe with pairs of rollers (small hard wheels) fixed to the sole", "name": "roller_skate"}, {"id": 906, "synset": "rollerblade.n.01", "synonyms": ["Rollerblade"], "def": "an in-line variant of a roller skate", "name": "Rollerblade"}, {"id": 907, "synset": "rolling_pin.n.01", "synonyms": ["rolling_pin"], "def": "utensil consisting of a cylinder (usually of wood) with a handle at each end; used to roll out dough", "name": "rolling_pin"}, {"id": 908, "synset": "root_beer.n.01", "synonyms": ["root_beer"], "def": "carbonated drink containing extracts of roots and herbs", "name": "root_beer"}, {"id": 909, "synset": "router.n.02", "synonyms": ["router_(computer_equipment)"], "def": "a device that forwards data packets between computer networks", "name": "router_(computer_equipment)"}, {"id": 910, "synset": "rubber_band.n.01", "synonyms": ["rubber_band", "elastic_band"], "def": "a narrow band of elastic rubber used to hold things (such as papers) together", "name": "rubber_band"}, {"id": 911, "synset": "runner.n.08", "synonyms": ["runner_(carpet)"], "def": "a long narrow carpet", "name": "runner_(carpet)"}, {"id": 912, "synset": "sack.n.01", "synonyms": ["plastic_bag", "paper_bag"], "def": "a bag made of paper or plastic for holding customer's purchases", "name": "plastic_bag"}, {"id": 913, "synset": "saddle.n.01", "synonyms": ["saddle_(on_an_animal)"], "def": "a seat for the rider of a horse or camel", "name": "saddle_(on_an_animal)"}, {"id": 914, "synset": "saddle_blanket.n.01", "synonyms": ["saddle_blanket", "saddlecloth", "horse_blanket"], "def": "stable gear consisting of a blanket placed under the saddle", "name": "saddle_blanket"}, {"id": 915, "synset": "saddlebag.n.01", "synonyms": ["saddlebag"], "def": "a large bag (or pair of bags) hung over a saddle", "name": "saddlebag"}, {"id": 916, "synset": "safety_pin.n.01", "synonyms": ["safety_pin"], "def": "a pin in the form of a clasp; has a guard so the point of the pin will not stick the user", "name": "safety_pin"}, {"id": 917, "synset": "sail.n.01", "synonyms": ["sail"], "def": "a large piece of fabric by means of which wind is used to propel a sailing vessel", "name": "sail"}, {"id": 918, "synset": "salad.n.01", "synonyms": ["salad"], "def": "food mixtures either arranged on a plate or tossed and served with a moist dressing; usually consisting of or including greens", "name": "salad"}, {"id": 919, "synset": "salad_plate.n.01", "synonyms": ["salad_plate", "salad_bowl"], "def": "a plate or bowl for individual servings of salad", "name": "salad_plate"}, {"id": 920, "synset": "salami.n.01", "synonyms": ["salami"], "def": "highly seasoned fatty sausage of pork and beef usually dried", "name": "salami"}, {"id": 921, "synset": "salmon.n.01", "synonyms": ["salmon_(fish)"], "def": "any of various large food and game fishes of northern waters", "name": "salmon_(fish)"}, {"id": 922, "synset": "salmon.n.03", "synonyms": ["salmon_(food)"], "def": "flesh of any of various marine or freshwater fish of the family Salmonidae", "name": "salmon_(food)"}, {"id": 923, "synset": "salsa.n.01", "synonyms": ["salsa"], "def": "spicy sauce of tomatoes and onions and chili peppers to accompany Mexican foods", "name": "salsa"}, {"id": 924, "synset": "saltshaker.n.01", "synonyms": ["saltshaker"], "def": "a shaker with a perforated top for sprinkling salt", "name": "saltshaker"}, {"id": 925, "synset": "sandal.n.01", "synonyms": ["sandal_(type_of_shoe)"], "def": "a shoe consisting of a sole fastened by straps to the foot", "name": "sandal_(type_of_shoe)"}, {"id": 926, "synset": "sandwich.n.01", "synonyms": ["sandwich"], "def": "two (or more) slices of bread with a filling between them", "name": "sandwich"}, {"id": 927, "synset": "satchel.n.01", "synonyms": ["satchel"], "def": "luggage consisting of a small case with a flat bottom and (usually) a shoulder strap", "name": "satchel"}, {"id": 928, "synset": "saucepan.n.01", "synonyms": ["saucepan"], "def": "a deep pan with a handle; used for stewing or boiling", "name": "saucepan"}, {"id": 929, "synset": "saucer.n.02", "synonyms": ["saucer"], "def": "a small shallow dish for holding a cup at the table", "name": "saucer"}, {"id": 930, "synset": "sausage.n.01", "synonyms": ["sausage"], "def": "highly seasoned minced meat stuffed in casings", "name": "sausage"}, {"id": 931, "synset": "sawhorse.n.01", "synonyms": ["sawhorse", "sawbuck"], "def": "a framework for holding wood that is being sawed", "name": "sawhorse"}, {"id": 932, "synset": "sax.n.02", "synonyms": ["saxophone"], "def": "a wind instrument with a `J'-shaped form typically made of brass", "name": "saxophone"}, {"id": 933, "synset": "scale.n.07", "synonyms": ["scale_(measuring_instrument)"], "def": "a measuring instrument for weighing; shows amount of mass", "name": "scale_(measuring_instrument)"}, {"id": 934, "synset": "scarecrow.n.01", "synonyms": ["scarecrow", "strawman"], "def": "an effigy in the shape of a man to frighten birds away from seeds", "name": "scarecrow"}, {"id": 935, "synset": "scarf.n.01", "synonyms": ["scarf"], "def": "a garment worn around the head or neck or shoulders for warmth or decoration", "name": "scarf"}, {"id": 936, "synset": "school_bus.n.01", "synonyms": ["school_bus"], "def": "a bus used to transport children to or from school", "name": "school_bus"}, {"id": 937, "synset": "scissors.n.01", "synonyms": ["scissors"], "def": "a tool having two crossed pivoting blades with looped handles", "name": "scissors"}, {"id": 938, "synset": "scoreboard.n.01", "synonyms": ["scoreboard"], "def": "a large board for displaying the score of a contest (and some other information)", "name": "scoreboard"}, {"id": 939, "synset": "scrambled_eggs.n.01", "synonyms": ["scrambled_eggs"], "def": "eggs beaten and cooked to a soft firm consistency while stirring", "name": "scrambled_eggs"}, {"id": 940, "synset": "scraper.n.01", "synonyms": ["scraper"], "def": "any of various hand tools for scraping", "name": "scraper"}, {"id": 941, "synset": "scratcher.n.03", "synonyms": ["scratcher"], "def": "a device used for scratching", "name": "scratcher"}, {"id": 942, "synset": "screwdriver.n.01", "synonyms": ["screwdriver"], "def": "a hand tool for driving screws; has a tip that fits into the head of a screw", "name": "screwdriver"}, {"id": 943, "synset": "scrub_brush.n.01", "synonyms": ["scrubbing_brush"], "def": "a brush with short stiff bristles for heavy cleaning", "name": "scrubbing_brush", "merged": [{"frequency": "c", "id": 153, "synset": "bristle_brush.n.01", "image_count": 3, "instance_count": 3, "synonyms": ["bristle_brush"], "def": "a brush that is made with the short stiff hairs of an animal or plant", "name": "bristle_brush"}]}, {"id": 944, "synset": "sculpture.n.01", "synonyms": ["sculpture"], "def": "a three-dimensional work of art", "name": "sculpture"}, {"id": 945, "synset": "seabird.n.01", "synonyms": ["seabird", "seafowl"], "def": "a bird that frequents coastal waters and the open ocean: gulls; pelicans; gannets; cormorants; albatrosses; petrels; etc.", "name": "seabird"}, {"id": 946, "synset": "seahorse.n.02", "synonyms": ["seahorse"], "def": "small fish with horse-like heads bent sharply downward and curled tails", "name": "seahorse"}, {"id": 947, "synset": "seaplane.n.01", "synonyms": ["seaplane", "hydroplane"], "def": "an airplane that can land on or take off from water", "name": "seaplane"}, {"id": 948, "synset": "seashell.n.01", "synonyms": ["seashell"], "def": "the shell of a marine organism", "name": "seashell"}, {"id": 949, "synset": "seedling.n.01", "synonyms": ["seedling"], "def": "young plant or tree grown from a seed", "name": "seedling"}, {"id": 950, "synset": "serving_dish.n.01", "synonyms": ["serving_dish"], "def": "a dish used for serving food", "name": "serving_dish"}, {"id": 951, "synset": "sewing_machine.n.01", "synonyms": ["sewing_machine"], "def": "a textile machine used as a home appliance for sewing", "name": "sewing_machine"}, {"id": 952, "synset": "shaker.n.03", "synonyms": ["shaker"], "def": "a container in which something can be shaken", "name": "shaker"}, {"id": 953, "synset": "shampoo.n.01", "synonyms": ["shampoo"], "def": "cleansing agent consisting of soaps or detergents used for washing the hair", "name": "shampoo"}, {"id": 954, "synset": "shark.n.01", "synonyms": ["shark"], "def": "typically large carnivorous fishes with sharpe teeth", "name": "shark"}, {"id": 955, "synset": "sharpener.n.01", "synonyms": ["sharpener"], "def": "any implement that is used to make something (an edge or a point) sharper", "name": "sharpener"}, {"id": 956, "synset": "sharpie.n.03", "synonyms": ["Sharpie"], "def": "a pen with indelible ink that will write on any surface", "name": "Sharpie"}, {"id": 957, "synset": "shaver.n.03", "synonyms": ["shaver_(electric)", "electric_shaver", "electric_razor"], "def": "a razor powered by an electric motor", "name": "shaver_(electric)"}, {"id": 958, "synset": "shaving_cream.n.01", "synonyms": ["shaving_cream", "shaving_soap"], "def": "toiletry consisting that forms a rich lather for softening the beard before shaving", "name": "shaving_cream"}, {"id": 959, "synset": "shawl.n.01", "synonyms": ["shawl"], "def": "cloak consisting of an oblong piece of cloth used to cover the head and shoulders", "name": "shawl"}, {"id": 960, "synset": "shears.n.01", "synonyms": ["shears"], "def": "large scissors with strong blades", "name": "shears"}, {"id": 961, "synset": "sheep.n.01", "synonyms": ["sheep"], "def": "woolly usually horned ruminant mammal related to the goat", "name": "sheep"}, {"id": 962, "synset": "shepherd_dog.n.01", "synonyms": ["shepherd_dog", "sheepdog"], "def": "any of various usually long-haired breeds of dog reared to herd and guard sheep", "name": "shepherd_dog"}, {"id": 963, "synset": "sherbert.n.01", "synonyms": ["sherbert", "sherbet"], "def": "a frozen dessert made primarily of fruit juice and sugar", "name": "sherbert"}, {"id": 964, "synset": "shield.n.02", "synonyms": ["shield"], "def": "armor carried on the arm to intercept blows", "name": "shield"}, {"id": 965, "synset": "shirt.n.01", "synonyms": ["shirt"], "def": "a garment worn on the upper half of the body", "name": "shirt"}, {"id": 966, "synset": "shoe.n.01", "synonyms": ["shoe", "sneaker_(type_of_shoe)", "tennis_shoe"], "def": "common footwear covering the foot", "name": "shoe"}, {"id": 967, "synset": "shopping_bag.n.01", "synonyms": ["shopping_bag"], "def": "a bag made of plastic or strong paper (often with handles); used to transport goods after shopping", "name": "shopping_bag"}, {"id": 968, "synset": "shopping_cart.n.01", "synonyms": ["shopping_cart"], "def": "a handcart that holds groceries or other goods while shopping", "name": "shopping_cart"}, {"id": 969, "synset": "short_pants.n.01", "synonyms": ["short_pants", "shorts_(clothing)", "trunks_(clothing)"], "def": "trousers that end at or above the knee", "name": "short_pants"}, {"id": 970, "synset": "shot_glass.n.01", "synonyms": ["shot_glass"], "def": "a small glass adequate to hold a single swallow of whiskey", "name": "shot_glass"}, {"id": 971, "synset": "shoulder_bag.n.01", "synonyms": ["shoulder_bag"], "def": "a large handbag that can be carried by a strap looped over the shoulder", "name": "shoulder_bag"}, {"id": 972, "synset": "shovel.n.01", "synonyms": ["shovel"], "def": "a hand tool for lifting loose material such as snow, dirt, etc.", "name": "shovel"}, {"id": 973, "synset": "shower.n.01", "synonyms": ["shower_head"], "def": "a plumbing fixture that sprays water over you", "name": "shower_head"}, {"id": 974, "synset": "shower_curtain.n.01", "synonyms": ["shower_curtain"], "def": "a curtain that keeps water from splashing out of the shower area", "name": "shower_curtain"}, {"id": 975, "synset": "shredder.n.01", "synonyms": ["shredder_(for_paper)"], "def": "a device that shreds documents", "name": "shredder_(for_paper)"}, {"id": 976, "synset": "sieve.n.01", "synonyms": ["sieve", "screen_(sieve)"], "def": "a strainer for separating lumps from powdered material or grading particles", "name": "sieve"}, {"id": 977, "synset": "signboard.n.01", "synonyms": ["signboard"], "def": "structure displaying a board on which advertisements can be posted", "name": "signboard"}, {"id": 978, "synset": "silo.n.01", "synonyms": ["silo"], "def": "a cylindrical tower used for storing goods", "name": "silo"}, {"id": 979, "synset": "sink.n.01", "synonyms": ["sink"], "def": "plumbing fixture consisting of a water basin fixed to a wall or floor and having a drainpipe", "name": "sink"}, {"id": 980, "synset": "skateboard.n.01", "synonyms": ["skateboard"], "def": "a board with wheels that is ridden in a standing or crouching position and propelled by foot", "name": "skateboard"}, {"id": 981, "synset": "skewer.n.01", "synonyms": ["skewer"], "def": "a long pin for holding meat in position while it is being roasted", "name": "skewer"}, {"id": 982, "synset": "ski.n.01", "synonyms": ["ski"], "def": "sports equipment for skiing on snow", "name": "ski"}, {"id": 983, "synset": "ski_boot.n.01", "synonyms": ["ski_boot"], "def": "a stiff boot that is fastened to a ski with a ski binding", "name": "ski_boot"}, {"id": 984, "synset": "ski_parka.n.01", "synonyms": ["ski_parka", "ski_jacket"], "def": "a parka to be worn while skiing", "name": "ski_parka"}, {"id": 985, "synset": "ski_pole.n.01", "synonyms": ["ski_pole"], "def": "a pole with metal points used as an aid in skiing", "name": "ski_pole"}, {"id": 986, "synset": "skirt.n.02", "synonyms": ["skirt"], "def": "a garment hanging from the waist; worn mainly by girls and women", "name": "skirt"}, {"id": 987, "synset": "sled.n.01", "synonyms": ["sled", "sledge", "sleigh"], "def": "a vehicle or flat object for transportation over snow by sliding or pulled by dogs, etc.", "name": "sled"}, {"id": 988, "synset": "sleeping_bag.n.01", "synonyms": ["sleeping_bag"], "def": "large padded bag designed to be slept in outdoors", "name": "sleeping_bag"}, {"id": 989, "synset": "sling.n.05", "synonyms": ["sling_(bandage)", "triangular_bandage"], "def": "bandage to support an injured forearm; slung over the shoulder or neck", "name": "sling_(bandage)"}, {"id": 990, "synset": "slipper.n.01", "synonyms": ["slipper_(footwear)", "carpet_slipper_(footwear)"], "def": "low footwear that can be slipped on and off easily; usually worn indoors", "name": "slipper_(footwear)"}, {"id": 991, "synset": "smoothie.n.02", "synonyms": ["smoothie"], "def": "a thick smooth drink consisting of fresh fruit pureed with ice cream or yoghurt or milk", "name": "smoothie"}, {"id": 992, "synset": "snake.n.01", "synonyms": ["snake", "serpent"], "def": "limbless scaly elongate reptile; some are venomous", "name": "snake"}, {"id": 993, "synset": "snowboard.n.01", "synonyms": ["snowboard"], "def": "a board that resembles a broad ski or a small surfboard; used in a standing position to slide down snow-covered slopes", "name": "snowboard"}, {"id": 994, "synset": "snowman.n.01", "synonyms": ["snowman"], "def": "a figure of a person made of packed snow", "name": "snowman"}, {"id": 995, "synset": "snowmobile.n.01", "synonyms": ["snowmobile"], "def": "tracked vehicle for travel on snow having skis in front", "name": "snowmobile"}, {"id": 996, "synset": "soap.n.01", "synonyms": ["soap"], "def": "a cleansing agent made from the salts of vegetable or animal fats", "name": "soap"}, {"id": 997, "synset": "soccer_ball.n.01", "synonyms": ["soccer_ball"], "def": "an inflated ball used in playing soccer (called `football' outside of the United States)", "name": "soccer_ball"}, {"id": 998, "synset": "sock.n.01", "synonyms": ["sock"], "def": "cloth covering for the foot; worn inside the shoe; reaches to between the ankle and the knee", "name": "sock"}, {"id": 999, "synset": "soda_fountain.n.02", "synonyms": ["soda_fountain"], "def": "an apparatus for dispensing soda water", "name": "soda_fountain"}, {"id": 1000, "synset": "soda_water.n.01", "synonyms": ["carbonated_water", "club_soda", "seltzer", "sparkling_water"], "def": "effervescent beverage artificially charged with carbon dioxide", "name": "carbonated_water"}, {"id": 1001, "synset": "sofa.n.01", "synonyms": ["sofa", "couch", "lounge"], "def": "an upholstered seat for more than one person", "name": "sofa"}, {"id": 1002, "synset": "softball.n.01", "synonyms": ["softball"], "def": "ball used in playing softball", "name": "softball"}, {"id": 1003, "synset": "solar_array.n.01", "synonyms": ["solar_array", "solar_battery", "solar_panel"], "def": "electrical device consisting of a large array of connected solar cells", "name": "solar_array"}, {"id": 1004, "synset": "sombrero.n.02", "synonyms": ["sombrero"], "def": "a straw hat with a tall crown and broad brim; worn in American southwest and in Mexico", "name": "sombrero"}, {"id": 1005, "synset": "soup.n.01", "synonyms": ["soup"], "def": "liquid food especially of meat or fish or vegetable stock often containing pieces of solid food", "name": "soup"}, {"id": 1006, "synset": "soup_bowl.n.01", "synonyms": ["soup_bowl"], "def": "a bowl for serving soup", "name": "soup_bowl"}, {"id": 1007, "synset": "soupspoon.n.01", "synonyms": ["soupspoon"], "def": "a spoon with a rounded bowl for eating soup", "name": "soupspoon"}, {"id": 1008, "synset": "sour_cream.n.01", "synonyms": ["sour_cream", "soured_cream"], "def": "soured light cream", "name": "sour_cream"}, {"id": 1009, "synset": "soya_milk.n.01", "synonyms": ["soya_milk", "soybean_milk", "soymilk"], "def": "a milk substitute containing soybean flour and water; used in some infant formulas and in making tofu", "name": "soya_milk"}, {"id": 1010, "synset": "space_shuttle.n.01", "synonyms": ["space_shuttle"], "def": "a reusable spacecraft with wings for a controlled descent through the Earth's atmosphere", "name": "space_shuttle"}, {"id": 1011, "synset": "sparkler.n.02", "synonyms": ["sparkler_(fireworks)"], "def": "a firework that burns slowly and throws out a shower of sparks", "name": "sparkler_(fireworks)"}, {"id": 1012, "synset": "spatula.n.02", "synonyms": ["spatula"], "def": "a hand tool with a thin flexible blade used to mix or spread soft substances", "name": "spatula"}, {"id": 1013, "synset": "spear.n.01", "synonyms": ["spear", "lance"], "def": "a long pointed rod used as a tool or weapon", "name": "spear"}, {"id": 1014, "synset": "spectacles.n.01", "synonyms": ["spectacles", "specs", "eyeglasses", "glasses"], "def": "optical instrument consisting of a frame that holds a pair of lenses for correcting defective vision", "name": "spectacles"}, {"id": 1015, "synset": "spice_rack.n.01", "synonyms": ["spice_rack"], "def": "a rack for displaying containers filled with spices", "name": "spice_rack"}, {"id": 1016, "synset": "spider.n.01", "synonyms": ["spider"], "def": "predatory arachnid with eight legs, two poison fangs, two feelers, and usually two silk-spinning organs at the back end of the body", "name": "spider"}, {"id": 1017, "synset": "sponge.n.01", "synonyms": ["sponge"], "def": "a porous mass usable to absorb water typically used for cleaning", "name": "sponge"}, {"id": 1018, "synset": "spoon.n.01", "synonyms": ["spoon"], "def": "a piece of cutlery with a shallow bowl-shaped container and a handle", "name": "spoon"}, {"id": 1019, "synset": "sportswear.n.01", "synonyms": ["sportswear", "athletic_wear", "activewear"], "def": "attire worn for sport or for casual wear", "name": "sportswear"}, {"id": 1020, "synset": "spotlight.n.02", "synonyms": ["spotlight"], "def": "a lamp that produces a strong beam of light to illuminate a restricted area; used to focus attention of a stage performer", "name": "spotlight"}, {"id": 1021, "synset": "squirrel.n.01", "synonyms": ["squirrel"], "def": "a kind of arboreal rodent having a long bushy tail", "name": "squirrel"}, {"id": 1022, "synset": "stapler.n.01", "synonyms": ["stapler_(stapling_machine)"], "def": "a machine that inserts staples into sheets of paper in order to fasten them together", "name": "stapler_(stapling_machine)"}, {"id": 1023, "synset": "starfish.n.01", "synonyms": ["starfish", "sea_star"], "def": "echinoderms characterized by five arms extending from a central disk", "name": "starfish"}, {"id": 1024, "synset": "statue.n.01", "synonyms": ["statue_(sculpture)"], "def": "a sculpture representing a human or animal", "name": "statue_(sculpture)"}, {"id": 1025, "synset": "steak.n.01", "synonyms": ["steak_(food)"], "def": "a slice of meat cut from the fleshy part of an animal or large fish", "name": "steak_(food)"}, {"id": 1026, "synset": "steak_knife.n.01", "synonyms": ["steak_knife"], "def": "a sharp table knife used in eating steak", "name": "steak_knife"}, {"id": 1027, "synset": "steamer.n.02", "synonyms": ["steamer_(kitchen_appliance)"], "def": "a cooking utensil that can be used to cook food by steaming it", "name": "steamer_(kitchen_appliance)"}, {"id": 1028, "synset": "steering_wheel.n.01", "synonyms": ["steering_wheel"], "def": "a handwheel that is used for steering", "name": "steering_wheel"}, {"id": 1029, "synset": "stencil.n.01", "synonyms": ["stencil"], "def": "a sheet of material (metal, plastic, etc.) that has been perforated with a pattern; ink or paint can pass through the perforations to create the printed pattern on the surface below", "name": "stencil"}, {"id": 1030, "synset": "step_ladder.n.01", "synonyms": ["stepladder"], "def": "a folding portable ladder hinged at the top", "name": "stepladder"}, {"id": 1031, "synset": "step_stool.n.01", "synonyms": ["step_stool"], "def": "a stool that has one or two steps that fold under the seat", "name": "step_stool"}, {"id": 1032, "synset": "stereo.n.01", "synonyms": ["stereo_(sound_system)"], "def": "electronic device for playing audio", "name": "stereo_(sound_system)"}, {"id": 1033, "synset": "stew.n.02", "synonyms": ["stew"], "def": "food prepared by stewing especially meat or fish with vegetables", "name": "stew"}, {"id": 1034, "synset": "stirrer.n.02", "synonyms": ["stirrer"], "def": "an implement used for stirring", "name": "stirrer"}, {"id": 1035, "synset": "stirrup.n.01", "synonyms": ["stirrup"], "def": "support consisting of metal loops into which rider's feet go", "name": "stirrup"}, {"id": 1036, "synset": "stocking.n.01", "synonyms": ["stockings_(leg_wear)"], "def": "close-fitting hosiery to cover the foot and leg; come in matched pairs", "name": "stockings_(leg_wear)"}, {"id": 1037, "synset": "stool.n.01", "synonyms": ["stool"], "def": "a simple seat without a back or arms", "name": "stool"}, {"id": 1038, "synset": "stop_sign.n.01", "synonyms": ["stop_sign"], "def": "a traffic sign to notify drivers that they must come to a complete stop", "name": "stop_sign"}, {"id": 1039, "synset": "stoplight.n.01", "synonyms": ["brake_light"], "def": "a red light on the rear of a motor vehicle that signals when the brakes are applied", "name": "brake_light"}, {"id": 1040, "synset": "stove.n.01", "synonyms": ["stove", "kitchen_stove", "range_(kitchen_appliance)", "kitchen_range", "cooking_stove"], "def": "a kitchen appliance used for cooking food", "name": "stove"}, {"id": 1041, "synset": "strainer.n.01", "synonyms": ["strainer"], "def": "a filter to retain larger pieces while smaller pieces and liquids pass through", "name": "strainer"}, {"id": 1042, "synset": "strap.n.01", "synonyms": ["strap"], "def": "an elongated strip of material for binding things together or holding", "name": "strap"}, {"id": 1043, "synset": "straw.n.04", "synonyms": ["straw_(for_drinking)", "drinking_straw"], "def": "a thin paper or plastic tube used to suck liquids into the mouth", "name": "straw_(for_drinking)"}, {"id": 1044, "synset": "strawberry.n.01", "synonyms": ["strawberry"], "def": "sweet fleshy red fruit", "name": "strawberry"}, {"id": 1045, "synset": "street_sign.n.01", "synonyms": ["street_sign"], "def": "a sign visible from the street", "name": "street_sign"}, {"id": 1046, "synset": "streetlight.n.01", "synonyms": ["streetlight", "street_lamp"], "def": "a lamp supported on a lamppost; for illuminating a street", "name": "streetlight"}, {"id": 1047, "synset": "string_cheese.n.01", "synonyms": ["string_cheese"], "def": "cheese formed in long strings twisted together", "name": "string_cheese"}, {"id": 1048, "synset": "stylus.n.02", "synonyms": ["stylus"], "def": "a pointed tool for writing or drawing or engraving", "name": "stylus"}, {"id": 1049, "synset": "subwoofer.n.01", "synonyms": ["subwoofer"], "def": "a loudspeaker that is designed to reproduce very low bass frequencies", "name": "subwoofer"}, {"id": 1050, "synset": "sugar_bowl.n.01", "synonyms": ["sugar_bowl"], "def": "a dish in which sugar is served", "name": "sugar_bowl"}, {"id": 1051, "synset": "sugarcane.n.01", "synonyms": ["sugarcane_(plant)"], "def": "juicy canes whose sap is a source of molasses and commercial sugar; fresh canes are sometimes chewed for the juice", "name": "sugarcane_(plant)"}, {"id": 1052, "synset": "suit.n.01", "synonyms": ["suit_(clothing)"], "def": "a set of garments (usually including a jacket and trousers or skirt) for outerwear all of the same fabric and color", "name": "suit_(clothing)"}, {"id": 1053, "synset": "sunflower.n.01", "synonyms": ["sunflower"], "def": "any plant of the genus Helianthus having large flower heads with dark disk florets and showy yellow rays", "name": "sunflower"}, {"id": 1054, "synset": "sunglasses.n.01", "synonyms": ["sunglasses"], "def": "spectacles that are darkened or polarized to protect the eyes from the glare of the sun", "name": "sunglasses"}, {"id": 1055, "synset": "sunhat.n.01", "synonyms": ["sunhat"], "def": "a hat with a broad brim that protects the face from direct exposure to the sun", "name": "sunhat"}, {"id": 1056, "synset": "sunscreen.n.01", "synonyms": ["sunscreen", "sunblock"], "def": "a cream spread on the skin; contains a chemical to filter out ultraviolet light and so protect from sunburn", "name": "sunscreen"}, {"id": 1057, "synset": "surfboard.n.01", "synonyms": ["surfboard"], "def": "a narrow buoyant board for riding surf", "name": "surfboard"}, {"id": 1058, "synset": "sushi.n.01", "synonyms": ["sushi"], "def": "rice (with raw fish) wrapped in seaweed", "name": "sushi"}, {"id": 1059, "synset": "swab.n.02", "synonyms": ["mop"], "def": "cleaning implement consisting of absorbent material fastened to a handle; for cleaning floors", "name": "mop"}, {"id": 1060, "synset": "sweat_pants.n.01", "synonyms": ["sweat_pants"], "def": "loose-fitting trousers with elastic cuffs; worn by athletes", "name": "sweat_pants"}, {"id": 1061, "synset": "sweatband.n.02", "synonyms": ["sweatband"], "def": "a band of material tied around the forehead or wrist to absorb sweat", "name": "sweatband"}, {"id": 1062, "synset": "sweater.n.01", "synonyms": ["sweater"], "def": "a crocheted or knitted garment covering the upper part of the body", "name": "sweater"}, {"id": 1063, "synset": "sweatshirt.n.01", "synonyms": ["sweatshirt"], "def": "cotton knit pullover with long sleeves worn during athletic activity", "name": "sweatshirt"}, {"id": 1064, "synset": "sweet_potato.n.02", "synonyms": ["sweet_potato"], "def": "the edible tuberous root of the sweet potato vine", "name": "sweet_potato"}, {"id": 1065, "synset": "swimsuit.n.01", "synonyms": ["swimsuit", "swimwear", "bathing_suit", "swimming_costume", "bathing_costume", "swimming_trunks", "bathing_trunks"], "def": "garment worn for swimming", "name": "swimsuit"}, {"id": 1066, "synset": "sword.n.01", "synonyms": ["sword"], "def": "a cutting or thrusting weapon that has a long metal blade", "name": "sword"}, {"id": 1067, "synset": "syringe.n.01", "synonyms": ["syringe"], "def": "a medical instrument used to inject or withdraw fluids", "name": "syringe"}, {"id": 1068, "synset": "tabasco.n.02", "synonyms": ["Tabasco_sauce"], "def": "very spicy sauce (trade name Tabasco) made from fully-aged red peppers", "name": "Tabasco_sauce"}, {"id": 1069, "synset": "table-tennis_table.n.01", "synonyms": ["table-tennis_table", "ping-pong_table"], "def": "a table used for playing table tennis", "name": "table-tennis_table"}, {"id": 1070, "synset": "table.n.02", "synonyms": ["table"], "def": "a piece of furniture having a smooth flat top that is usually supported by one or more vertical legs", "name": "table"}, {"id": 1071, "synset": "table_lamp.n.01", "synonyms": ["table_lamp"], "def": "a lamp that sits on a table", "name": "table_lamp"}, {"id": 1072, "synset": "tablecloth.n.01", "synonyms": ["tablecloth"], "def": "a covering spread over a dining table", "name": "tablecloth"}, {"id": 1073, "synset": "tachometer.n.01", "synonyms": ["tachometer"], "def": "measuring instrument for indicating speed of rotation", "name": "tachometer"}, {"id": 1074, "synset": "taco.n.02", "synonyms": ["taco"], "def": "a small tortilla cupped around a filling", "name": "taco"}, {"id": 1075, "synset": "tag.n.02", "synonyms": ["tag"], "def": "a label associated with something for the purpose of identification or information", "name": "tag"}, {"id": 1076, "synset": "taillight.n.01", "synonyms": ["taillight", "rear_light"], "def": "lamp (usually red) mounted at the rear of a motor vehicle", "name": "taillight"}, {"id": 1077, "synset": "tambourine.n.01", "synonyms": ["tambourine"], "def": "a shallow drum with a single drumhead and with metallic disks in the sides", "name": "tambourine"}, {"id": 1078, "synset": "tank.n.01", "synonyms": ["army_tank", "armored_combat_vehicle", "armoured_combat_vehicle"], "def": "an enclosed armored military vehicle; has a cannon and moves on caterpillar treads", "name": "army_tank"}, {"id": 1079, "synset": "tank.n.02", "synonyms": ["tank_(storage_vessel)", "storage_tank"], "def": "a large (usually metallic) vessel for holding gases or liquids", "name": "tank_(storage_vessel)"}, {"id": 1080, "synset": "tank_top.n.01", "synonyms": ["tank_top_(clothing)"], "def": "a tight-fitting sleeveless shirt with wide shoulder straps and low neck and no front opening", "name": "tank_top_(clothing)"}, {"id": 1081, "synset": "tape.n.01", "synonyms": ["tape_(sticky_cloth_or_paper)"], "def": "a long thin piece of cloth or paper as used for binding or fastening", "name": "tape_(sticky_cloth_or_paper)"}, {"id": 1082, "synset": "tape.n.04", "synonyms": ["tape_measure", "measuring_tape"], "def": "measuring instrument consisting of a narrow strip (cloth or metal) marked in inches or centimeters and used for measuring lengths", "name": "tape_measure"}, {"id": 1083, "synset": "tapestry.n.02", "synonyms": ["tapestry"], "def": "a heavy textile with a woven design; used for curtains and upholstery", "name": "tapestry"}, {"id": 1084, "synset": "tarpaulin.n.01", "synonyms": ["tarp"], "def": "waterproofed canvas", "name": "tarp"}, {"id": 1085, "synset": "tartan.n.01", "synonyms": ["tartan", "plaid"], "def": "a cloth having a crisscross design", "name": "tartan"}, {"id": 1086, "synset": "tassel.n.01", "synonyms": ["tassel"], "def": "adornment consisting of a bunch of cords fastened at one end", "name": "tassel"}, {"id": 1087, "synset": "tea_bag.n.01", "synonyms": ["tea_bag"], "def": "a measured amount of tea in a bag for an individual serving of tea", "name": "tea_bag"}, {"id": 1088, "synset": "teacup.n.02", "synonyms": ["teacup"], "def": "a cup from which tea is drunk", "name": "teacup"}, {"id": 1089, "synset": "teakettle.n.01", "synonyms": ["teakettle"], "def": "kettle for boiling water to make tea", "name": "teakettle"}, {"id": 1090, "synset": "teapot.n.01", "synonyms": ["teapot"], "def": "pot for brewing tea; usually has a spout and handle", "name": "teapot"}, {"id": 1091, "synset": "teddy.n.01", "synonyms": ["teddy_bear"], "def": "plaything consisting of a child's toy bear (usually plush and stuffed with soft materials)", "name": "teddy_bear"}, {"id": 1092, "synset": "telephone.n.01", "synonyms": ["telephone", "phone", "telephone_set"], "def": "electronic device for communicating by voice over long distances", "name": "telephone"}, {"id": 1093, "synset": "telephone_booth.n.01", "synonyms": ["telephone_booth", "phone_booth", "call_box", "telephone_box", "telephone_kiosk"], "def": "booth for using a telephone", "name": "telephone_booth"}, {"id": 1094, "synset": "telephone_pole.n.01", "synonyms": ["telephone_pole", "telegraph_pole", "telegraph_post"], "def": "tall pole supporting telephone wires", "name": "telephone_pole"}, {"id": 1095, "synset": "telephoto_lens.n.01", "synonyms": ["telephoto_lens", "zoom_lens"], "def": "a camera lens that magnifies the image", "name": "telephoto_lens"}, {"id": 1096, "synset": "television_camera.n.01", "synonyms": ["television_camera", "tv_camera"], "def": "television equipment for capturing and recording video", "name": "television_camera"}, {"id": 1097, "synset": "television_receiver.n.01", "synonyms": ["television_set", "tv", "tv_set"], "def": "an electronic device that receives television signals and displays them on a screen", "name": "television_set"}, {"id": 1098, "synset": "tennis_ball.n.01", "synonyms": ["tennis_ball"], "def": "ball about the size of a fist used in playing tennis", "name": "tennis_ball"}, {"id": 1099, "synset": "tennis_racket.n.01", "synonyms": ["tennis_racket"], "def": "a racket used to play tennis", "name": "tennis_racket"}, {"id": 1100, "synset": "tequila.n.01", "synonyms": ["tequila"], "def": "Mexican liquor made from fermented juices of an agave plant", "name": "tequila"}, {"id": 1101, "synset": "thermometer.n.01", "synonyms": ["thermometer"], "def": "measuring instrument for measuring temperature", "name": "thermometer"}, {"id": 1102, "synset": "thermos.n.01", "synonyms": ["thermos_bottle"], "def": "vacuum flask that preserves temperature of hot or cold drinks", "name": "thermos_bottle"}, {"id": 1103, "synset": "thermostat.n.01", "synonyms": ["thermostat"], "def": "a regulator for automatically regulating temperature by starting or stopping the supply of heat", "name": "thermostat"}, {"id": 1104, "synset": "thimble.n.02", "synonyms": ["thimble"], "def": "a small metal cap to protect the finger while sewing; can be used as a small container", "name": "thimble"}, {"id": 1105, "synset": "thread.n.01", "synonyms": ["thread", "yarn"], "def": "a fine cord of twisted fibers (of cotton or silk or wool or nylon etc.) used in sewing and weaving", "name": "thread"}, {"id": 1106, "synset": "thumbtack.n.01", "synonyms": ["thumbtack", "drawing_pin", "pushpin"], "def": "a tack for attaching papers to a bulletin board or drawing board", "name": "thumbtack"}, {"id": 1107, "synset": "tiara.n.01", "synonyms": ["tiara"], "def": "a jeweled headdress worn by women on formal occasions", "name": "tiara"}, {"id": 1108, "synset": "tiger.n.02", "synonyms": ["tiger"], "def": "large feline of forests in most of Asia having a tawny coat with black stripes", "name": "tiger"}, {"id": 1109, "synset": "tights.n.01", "synonyms": ["tights_(clothing)", "leotards"], "def": "skintight knit hose covering the body from the waist to the feet worn by acrobats and dancers and as stockings by women and girls", "name": "tights_(clothing)"}, {"id": 1110, "synset": "timer.n.01", "synonyms": ["timer", "stopwatch"], "def": "a timepiece that measures a time interval and signals its end", "name": "timer"}, {"id": 1111, "synset": "tinfoil.n.01", "synonyms": ["tinfoil"], "def": "foil made of tin or an alloy of tin and lead", "name": "tinfoil"}, {"id": 1112, "synset": "tinsel.n.01", "synonyms": ["tinsel"], "def": "a showy decoration that is basically valueless", "name": "tinsel"}, {"id": 1113, "synset": "tissue.n.02", "synonyms": ["tissue_paper"], "def": "a soft thin (usually translucent) paper", "name": "tissue_paper"}, {"id": 1114, "synset": "toast.n.01", "synonyms": ["toast_(food)"], "def": "slice of bread that has been toasted", "name": "toast_(food)"}, {"id": 1115, "synset": "toaster.n.02", "synonyms": ["toaster"], "def": "a kitchen appliance (usually electric) for toasting bread", "name": "toaster"}, {"id": 1116, "synset": "toaster_oven.n.01", "synonyms": ["toaster_oven"], "def": "kitchen appliance consisting of a small electric oven for toasting or warming food", "name": "toaster_oven"}, {"id": 1117, "synset": "toilet.n.02", "synonyms": ["toilet"], "def": "a plumbing fixture for defecation and urination", "name": "toilet"}, {"id": 1118, "synset": "toilet_tissue.n.01", "synonyms": ["toilet_tissue", "toilet_paper", "bathroom_tissue"], "def": "a soft thin absorbent paper for use in toilets", "name": "toilet_tissue"}, {"id": 1119, "synset": "tomato.n.01", "synonyms": ["tomato"], "def": "mildly acid red or yellow pulpy fruit eaten as a vegetable", "name": "tomato"}, {"id": 1120, "synset": "tongs.n.01", "synonyms": ["tongs"], "def": "any of various devices for taking hold of objects; usually have two hinged legs with handles above and pointed hooks below", "name": "tongs"}, {"id": 1121, "synset": "toolbox.n.01", "synonyms": ["toolbox"], "def": "a box or chest or cabinet for holding hand tools", "name": "toolbox"}, {"id": 1122, "synset": "toothbrush.n.01", "synonyms": ["toothbrush"], "def": "small brush; has long handle; used to clean teeth", "name": "toothbrush"}, {"id": 1123, "synset": "toothpaste.n.01", "synonyms": ["toothpaste"], "def": "a dentifrice in the form of a paste", "name": "toothpaste"}, {"id": 1124, "synset": "toothpick.n.01", "synonyms": ["toothpick"], "def": "pick consisting of a small strip of wood or plastic; used to pick food from between the teeth", "name": "toothpick"}, {"id": 1125, "synset": "top.n.09", "synonyms": ["cover"], "def": "covering for a hole (especially a hole in the top of a container)", "name": "cover"}, {"id": 1126, "synset": "tortilla.n.01", "synonyms": ["tortilla"], "def": "thin unleavened pancake made from cornmeal or wheat flour", "name": "tortilla"}, {"id": 1127, "synset": "tow_truck.n.01", "synonyms": ["tow_truck"], "def": "a truck equipped to hoist and pull wrecked cars (or to remove cars from no-parking zones)", "name": "tow_truck"}, {"id": 1128, "synset": "towel.n.01", "synonyms": ["towel"], "def": "a rectangular piece of absorbent cloth (or paper) for drying or wiping", "name": "towel"}, {"id": 1129, "synset": "towel_rack.n.01", "synonyms": ["towel_rack", "towel_rail", "towel_bar"], "def": "a rack consisting of one or more bars on which towels can be hung", "name": "towel_rack"}, {"id": 1130, "synset": "toy.n.03", "synonyms": ["toy"], "def": "a device regarded as providing amusement", "name": "toy"}, {"id": 1131, "synset": "tractor.n.01", "synonyms": ["tractor_(farm_equipment)"], "def": "a wheeled vehicle with large wheels; used in farming and other applications", "name": "tractor_(farm_equipment)"}, {"id": 1132, "synset": "traffic_light.n.01", "synonyms": ["traffic_light"], "def": "a device to control vehicle traffic often consisting of three or more lights", "name": "traffic_light"}, {"id": 1133, "synset": "trail_bike.n.01", "synonyms": ["dirt_bike"], "def": "a lightweight motorcycle equipped with rugged tires and suspension for off-road use", "name": "dirt_bike"}, {"id": 1134, "synset": "trailer_truck.n.01", "synonyms": ["trailer_truck", "tractor_trailer", "trucking_rig", "articulated_lorry", "semi_truck"], "def": "a truck consisting of a tractor and trailer together", "name": "trailer_truck"}, {"id": 1135, "synset": "train.n.01", "synonyms": ["train_(railroad_vehicle)", "railroad_train"], "def": "public or private transport provided by a line of railway cars coupled together and drawn by a locomotive", "name": "train_(railroad_vehicle)"}, {"id": 1136, "synset": "trampoline.n.01", "synonyms": ["trampoline"], "def": "gymnastic apparatus consisting of a strong canvas sheet attached with springs to a metal frame", "name": "trampoline"}, {"id": 1137, "synset": "tray.n.01", "synonyms": ["tray"], "def": "an open receptacle for holding or displaying or serving articles or food", "name": "tray"}, {"id": 1138, "synset": "tree_house.n.01", "synonyms": ["tree_house"], "def": "(NOT A TREE) a PLAYHOUSE built in the branches of a tree", "name": "tree_house"}, {"id": 1139, "synset": "trench_coat.n.01", "synonyms": ["trench_coat"], "def": "a military style raincoat; belted with deep pockets", "name": "trench_coat"}, {"id": 1140, "synset": "triangle.n.05", "synonyms": ["triangle_(musical_instrument)"], "def": "a percussion instrument consisting of a metal bar bent in the shape of an open triangle", "name": "triangle_(musical_instrument)"}, {"id": 1141, "synset": "tricycle.n.01", "synonyms": ["tricycle"], "def": "a vehicle with three wheels that is moved by foot pedals", "name": "tricycle"}, {"id": 1142, "synset": "tripod.n.01", "synonyms": ["tripod"], "def": "a three-legged rack used for support", "name": "tripod"}, {"id": 1143, "synset": "trouser.n.01", "synonyms": ["trousers", "pants_(clothing)"], "def": "a garment extending from the waist to the knee or ankle, covering each leg separately", "name": "trousers"}, {"id": 1144, "synset": "truck.n.01", "synonyms": ["truck"], "def": "an automotive vehicle suitable for hauling", "name": "truck"}, {"id": 1145, "synset": "truffle.n.03", "synonyms": ["truffle_(chocolate)", "chocolate_truffle"], "def": "creamy chocolate candy", "name": "truffle_(chocolate)"}, {"id": 1146, "synset": "trunk.n.02", "synonyms": ["trunk"], "def": "luggage consisting of a large strong case used when traveling or for storage", "name": "trunk"}, {"id": 1147, "synset": "tub.n.02", "synonyms": ["vat"], "def": "a large open vessel for holding or storing liquids", "name": "vat"}, {"id": 1148, "synset": "turban.n.01", "synonyms": ["turban"], "def": "a traditional headdress consisting of a long scarf wrapped around the head", "name": "turban"}, {"id": 1149, "synset": "turkey.n.01", "synonyms": ["turkey_(bird)"], "def": "large gallinaceous bird with fan-shaped tail; widely domesticated for food", "name": "turkey_(bird)"}, {"id": 1150, "synset": "turkey.n.04", "synonyms": ["turkey_(food)"], "def": "flesh of large domesticated fowl usually roasted", "name": "turkey_(food)"}, {"id": 1151, "synset": "turnip.n.01", "synonyms": ["turnip"], "def": "widely cultivated plant having a large fleshy edible white or yellow root", "name": "turnip"}, {"id": 1152, "synset": "turtle.n.02", "synonyms": ["turtle"], "def": "any of various aquatic and land reptiles having a bony shell and flipper-like limbs for swimming", "name": "turtle"}, {"id": 1153, "synset": "turtleneck.n.01", "synonyms": ["turtleneck_(clothing)", "polo-neck"], "def": "a sweater or jersey with a high close-fitting collar", "name": "turtleneck_(clothing)"}, {"id": 1154, "synset": "typewriter.n.01", "synonyms": ["typewriter"], "def": "hand-operated character printer for printing written messages one character at a time", "name": "typewriter"}, {"id": 1155, "synset": "umbrella.n.01", "synonyms": ["umbrella"], "def": "a lightweight handheld collapsible canopy", "name": "umbrella"}, {"id": 1156, "synset": "underwear.n.01", "synonyms": ["underwear", "underclothes", "underclothing", "underpants"], "def": "undergarment worn next to the skin and under the outer garments", "name": "underwear"}, {"id": 1157, "synset": "unicycle.n.01", "synonyms": ["unicycle"], "def": "a vehicle with a single wheel that is driven by pedals", "name": "unicycle"}, {"id": 1158, "synset": "urinal.n.01", "synonyms": ["urinal"], "def": "a plumbing fixture (usually attached to the wall) used by men to urinate", "name": "urinal"}, {"id": 1159, "synset": "urn.n.01", "synonyms": ["urn"], "def": "a large vase that usually has a pedestal or feet", "name": "urn"}, {"id": 1160, "synset": "vacuum.n.04", "synonyms": ["vacuum_cleaner"], "def": "an electrical home appliance that cleans by suction", "name": "vacuum_cleaner"}, {"id": 1161, "synset": "valve.n.03", "synonyms": ["valve"], "def": "control consisting of a mechanical device for controlling the flow of a fluid", "name": "valve"}, {"id": 1162, "synset": "vase.n.01", "synonyms": ["vase"], "def": "an open jar of glass or porcelain used as an ornament or to hold flowers", "name": "vase"}, {"id": 1163, "synset": "vending_machine.n.01", "synonyms": ["vending_machine"], "def": "a slot machine for selling goods", "name": "vending_machine"}, {"id": 1164, "synset": "vent.n.01", "synonyms": ["vent", "blowhole", "air_vent"], "def": "a hole for the escape of gas or air", "name": "vent"}, {"id": 1165, "synset": "videotape.n.01", "synonyms": ["videotape"], "def": "a video recording made on magnetic tape", "name": "videotape"}, {"id": 1166, "synset": "vinegar.n.01", "synonyms": ["vinegar"], "def": "sour-tasting liquid produced usually by oxidation of the alcohol in wine or cider and used as a condiment or food preservative", "name": "vinegar"}, {"id": 1167, "synset": "violin.n.01", "synonyms": ["violin", "fiddle"], "def": "bowed stringed instrument that is the highest member of the violin family", "name": "violin"}, {"id": 1168, "synset": "vodka.n.01", "synonyms": ["vodka"], "def": "unaged colorless liquor originating in Russia", "name": "vodka"}, {"id": 1169, "synset": "volleyball.n.02", "synonyms": ["volleyball"], "def": "an inflated ball used in playing volleyball", "name": "volleyball"}, {"id": 1170, "synset": "vulture.n.01", "synonyms": ["vulture"], "def": "any of various large birds of prey having naked heads and weak claws and feeding chiefly on carrion", "name": "vulture"}, {"id": 1171, "synset": "waffle.n.01", "synonyms": ["waffle"], "def": "pancake batter baked in a waffle iron", "name": "waffle"}, {"id": 1172, "synset": "waffle_iron.n.01", "synonyms": ["waffle_iron"], "def": "a kitchen appliance for baking waffles", "name": "waffle_iron"}, {"id": 1173, "synset": "wagon.n.01", "synonyms": ["wagon"], "def": "any of various kinds of wheeled vehicles drawn by an animal or a tractor", "name": "wagon"}, {"id": 1174, "synset": "wagon_wheel.n.01", "synonyms": ["wagon_wheel"], "def": "a wheel of a wagon", "name": "wagon_wheel"}, {"id": 1175, "synset": "walking_stick.n.01", "synonyms": ["walking_stick"], "def": "a stick carried in the hand for support in walking", "name": "walking_stick", "merged": [{"frequency": "c", "id": 201, "synset": "cane.n.01", "image_count": 5, "instance_count": 5, "synonyms": ["walking_cane"], "def": "a stick that people can lean on to help them walk", "name": "walking_cane"}]}, {"id": 1176, "synset": "wall_clock.n.01", "synonyms": ["wall_clock"], "def": "a clock mounted on a wall", "name": "wall_clock"}, {"id": 1177, "synset": "wall_socket.n.01", "synonyms": ["wall_socket", "wall_plug", "electric_outlet", "electrical_outlet", "outlet", "electric_receptacle"], "def": "receptacle providing a place in a wiring system where current can be taken to run electrical devices", "name": "wall_socket"}, {"id": 1178, "synset": "wallet.n.01", "synonyms": ["wallet", "billfold"], "def": "a pocket-size case for holding papers and paper money", "name": "wallet"}, {"id": 1179, "synset": "walrus.n.01", "synonyms": ["walrus"], "def": "either of two large northern marine mammals having ivory tusks and tough hide over thick blubber", "name": "walrus"}, {"id": 1180, "synset": "wardrobe.n.01", "synonyms": ["wardrobe"], "def": "a tall piece of furniture that provides storage space for clothes; has a door and rails or hooks for hanging clothes", "name": "wardrobe"}, {"id": 1181, "synset": "wasabi.n.02", "synonyms": ["wasabi"], "def": "the thick green root of the wasabi plant that the Japanese use in cooking and that tastes like strong horseradish", "name": "wasabi"}, {"id": 1182, "synset": "washer.n.03", "synonyms": ["automatic_washer", "washing_machine"], "def": "a home appliance for washing clothes and linens automatically", "name": "automatic_washer"}, {"id": 1183, "synset": "watch.n.01", "synonyms": ["watch", "wristwatch"], "def": "a small, portable timepiece", "name": "watch"}, {"id": 1184, "synset": "water_bottle.n.01", "synonyms": ["water_bottle"], "def": "a bottle for holding water", "name": "water_bottle"}, {"id": 1185, "synset": "water_cooler.n.01", "synonyms": ["water_cooler"], "def": "a device for cooling and dispensing drinking water", "name": "water_cooler"}, {"id": 1186, "synset": "water_faucet.n.01", "synonyms": ["water_faucet", "water_tap", "tap_(water_faucet)"], "def": "a faucet for drawing water from a pipe or cask", "name": "water_faucet"}, {"id": 1187, "synset": "water_filter.n.01", "synonyms": ["water_filter"], "def": "a filter to remove impurities from the water supply", "name": "water_filter"}, {"id": 1188, "synset": "water_heater.n.01", "synonyms": ["water_heater", "hot-water_heater"], "def": "a heater and storage tank to supply heated water", "name": "water_heater"}, {"id": 1189, "synset": "water_jug.n.01", "synonyms": ["water_jug"], "def": "a jug that holds water", "name": "water_jug"}, {"id": 1190, "synset": "water_pistol.n.01", "synonyms": ["water_gun", "squirt_gun"], "def": "plaything consisting of a toy pistol that squirts water", "name": "water_gun"}, {"id": 1191, "synset": "water_scooter.n.01", "synonyms": ["water_scooter", "sea_scooter", "jet_ski"], "def": "a motorboat resembling a motor scooter (NOT A SURFBOARD OR WATER SKI)", "name": "water_scooter"}, {"id": 1192, "synset": "water_ski.n.01", "synonyms": ["water_ski"], "def": "broad ski for skimming over water towed by a speedboat (DO NOT MARK WATER)", "name": "water_ski"}, {"id": 1193, "synset": "water_tower.n.01", "synonyms": ["water_tower"], "def": "a large reservoir for water", "name": "water_tower"}, {"id": 1194, "synset": "watering_can.n.01", "synonyms": ["watering_can"], "def": "a container with a handle and a spout with a perforated nozzle; used to sprinkle water over plants", "name": "watering_can"}, {"id": 1195, "synset": "watermelon.n.02", "synonyms": ["watermelon"], "def": "large oblong or roundish melon with a hard green rind and sweet watery red or occasionally yellowish pulp", "name": "watermelon"}, {"id": 1196, "synset": "weathervane.n.01", "synonyms": ["weathervane", "vane_(weathervane)", "wind_vane"], "def": "mechanical device attached to an elevated structure; rotates freely to show the direction of the wind", "name": "weathervane"}, {"id": 1197, "synset": "webcam.n.01", "synonyms": ["webcam"], "def": "a digital camera designed to take digital photographs and transmit them over the internet", "name": "webcam"}, {"id": 1198, "synset": "wedding_cake.n.01", "synonyms": ["wedding_cake", "bridecake"], "def": "a rich cake with two or more tiers and covered with frosting and decorations; served at a wedding reception", "name": "wedding_cake"}, {"id": 1199, "synset": "wedding_ring.n.01", "synonyms": ["wedding_ring", "wedding_band"], "def": "a ring given to the bride and/or groom at the wedding", "name": "wedding_ring"}, {"id": 1200, "synset": "wet_suit.n.01", "synonyms": ["wet_suit"], "def": "a close-fitting garment made of a permeable material; worn in cold water to retain body heat", "name": "wet_suit"}, {"id": 1201, "synset": "wheel.n.01", "synonyms": ["wheel"], "def": "a circular frame with spokes (or a solid disc) that can rotate on a shaft or axle", "name": "wheel"}, {"id": 1202, "synset": "wheelchair.n.01", "synonyms": ["wheelchair"], "def": "a movable chair mounted on large wheels", "name": "wheelchair"}, {"id": 1203, "synset": "whipped_cream.n.01", "synonyms": ["whipped_cream"], "def": "cream that has been beaten until light and fluffy", "name": "whipped_cream"}, {"id": 1204, "synset": "whiskey.n.01", "synonyms": ["whiskey"], "def": "a liquor made from fermented mash of grain", "name": "whiskey"}, {"id": 1205, "synset": "whistle.n.03", "synonyms": ["whistle"], "def": "a small wind instrument that produces a whistling sound by blowing into it", "name": "whistle"}, {"id": 1206, "synset": "wick.n.02", "synonyms": ["wick"], "def": "a loosely woven cord in a candle or oil lamp that is lit on fire", "name": "wick"}, {"id": 1207, "synset": "wig.n.01", "synonyms": ["wig"], "def": "hairpiece covering the head and made of real or synthetic hair", "name": "wig"}, {"id": 1208, "synset": "wind_chime.n.01", "synonyms": ["wind_chime"], "def": "a decorative arrangement of pieces of metal or glass or pottery that hang together loosely so the wind can cause them to tinkle", "name": "wind_chime"}, {"id": 1209, "synset": "windmill.n.01", "synonyms": ["windmill"], "def": "a mill that is powered by the wind", "name": "windmill"}, {"id": 1210, "synset": "window_box.n.01", "synonyms": ["window_box_(for_plants)"], "def": "a container for growing plants on a windowsill", "name": "window_box_(for_plants)"}, {"id": 1211, "synset": "windshield_wiper.n.01", "synonyms": ["windshield_wiper", "windscreen_wiper", "wiper_(for_windshield/screen)"], "def": "a mechanical device that cleans the windshield", "name": "windshield_wiper"}, {"id": 1212, "synset": "windsock.n.01", "synonyms": ["windsock", "air_sock", "air-sleeve", "wind_sleeve", "wind_cone"], "def": "a truncated cloth cone mounted on a mast/pole; shows wind direction", "name": "windsock"}, {"id": 1213, "synset": "wine_bottle.n.01", "synonyms": ["wine_bottle"], "def": "a bottle for holding wine", "name": "wine_bottle"}, {"id": 1214, "synset": "wine_bucket.n.01", "synonyms": ["wine_bucket", "wine_cooler"], "def": "a bucket of ice used to chill a bottle of wine", "name": "wine_bucket"}, {"id": 1215, "synset": "wineglass.n.01", "synonyms": ["wineglass"], "def": "a glass that has a stem and in which wine is served", "name": "wineglass"}, {"id": 1216, "synset": "wing_chair.n.01", "synonyms": ["wing_chair"], "def": "easy chair having wings on each side of a high back", "name": "wing_chair"}, {"id": 1217, "synset": "winker.n.02", "synonyms": ["blinder_(for_horses)"], "def": "blinds that prevent a horse from seeing something on either side", "name": "blinder_(for_horses)"}, {"id": 1218, "synset": "wok.n.01", "synonyms": ["wok"], "def": "pan with a convex bottom; used for frying in Chinese cooking", "name": "wok"}, {"id": 1219, "synset": "wolf.n.01", "synonyms": ["wolf"], "def": "a wild carnivorous mammal of the dog family, living and hunting in packs", "name": "wolf"}, {"id": 1220, "synset": "wooden_spoon.n.02", "synonyms": ["wooden_spoon"], "def": "a spoon made of wood", "name": "wooden_spoon"}, {"id": 1221, "synset": "wreath.n.01", "synonyms": ["wreath"], "def": "an arrangement of flowers, leaves, or stems fastened in a ring", "name": "wreath"}, {"id": 1222, "synset": "wrench.n.03", "synonyms": ["wrench", "spanner"], "def": "a hand tool that is used to hold or twist a nut or bolt", "name": "wrench"}, {"id": 1223, "synset": "wristband.n.01", "synonyms": ["wristband"], "def": "band consisting of a part of a sleeve that covers the wrist", "name": "wristband"}, {"id": 1224, "synset": "wristlet.n.01", "synonyms": ["wristlet", "wrist_band"], "def": "a band or bracelet worn around the wrist", "name": "wristlet"}, {"id": 1225, "synset": "yacht.n.01", "synonyms": ["yacht"], "def": "an expensive vessel propelled by sail or power and used for cruising or racing", "name": "yacht"}, {"id": 1226, "synset": "yak.n.02", "synonyms": ["yak"], "def": "large long-haired wild ox of Tibet often domesticated", "name": "yak"}, {"id": 1227, "synset": "yogurt.n.01", "synonyms": ["yogurt", "yoghurt", "yoghourt"], "def": "a custard-like food made from curdled milk", "name": "yogurt"}, {"id": 1228, "synset": "yoke.n.07", "synonyms": ["yoke_(animal_equipment)"], "def": "gear joining two animals at the neck; NOT egg yolk", "name": "yoke_(animal_equipment)"}, {"id": 1229, "synset": "zebra.n.01", "synonyms": ["zebra"], "def": "any of several fleet black-and-white striped African equines", "name": "zebra"}, {"id": 1230, "synset": "zucchini.n.02", "synonyms": ["zucchini", "courgette"], "def": "small cucumber-shaped vegetable marrow; typically dark green", "name": "zucchini"}]
\ No newline at end of file
diff --git a/val_utils/trackeval/datasets/burst_ow.py b/val_utils/trackeval/datasets/burst_ow.py
new file mode 100644
index 0000000000000000000000000000000000000000..da775456e6c539c07c85db1fc9cad5998d8baaeb
--- /dev/null
+++ b/val_utils/trackeval/datasets/burst_ow.py
@@ -0,0 +1,91 @@
+import json
+import os
+from .burst_helpers.burst_ow_base import BURST_OW_Base
+from .burst_helpers.format_converter import GroundTruthBURSTFormatToTAOFormatConverter, PredictionBURSTFormatToTAOFormatConverter
+from .. import utils
+
+
+class BURST_OW(BURST_OW_Base):
+ """Dataset class for TAO tracking"""
+
+ @staticmethod
+ def get_default_dataset_config():
+ tao_config = BURST_OW_Base.get_default_dataset_config()
+ code_path = utils.get_code_path()
+ tao_config['GT_FOLDER'] = os.path.join(
+ code_path, 'data/gt/burst/all_classes/val/') # Location of GT data
+ tao_config['TRACKERS_FOLDER'] = os.path.join(
+ code_path, 'data/trackers/burst/open-world/val/') # Trackers location
+ return tao_config
+
+ def _iou_type(self):
+ return 'mask'
+
+ def _box_or_mask_from_det(self, det):
+ if "segmentation" in det:
+ return det["segmentation"]
+ else:
+ return det["mask"]
+
+ def _calculate_area_for_ann(self, ann):
+ import pycocotools.mask as cocomask
+ seg = self._box_or_mask_from_det(ann)
+ return cocomask.area(seg)
+
+ def _calculate_similarities(self, gt_dets_t, tracker_dets_t):
+ similarity_scores = self._calculate_mask_ious(gt_dets_t, tracker_dets_t, is_encoded=True, do_ioa=False)
+ return similarity_scores
+
+ def _postproc_ground_truth_data(self, data):
+ return GroundTruthBURSTFormatToTAOFormatConverter(data).convert()
+
+ def _postproc_prediction_data(self, data):
+ # if it's a list, it's already in TAO format and not in Ali format
+ # however the image ids do not match and need to be remapped
+ if isinstance(data, list):
+ _remap_image_ids(data, self.gt_data)
+ return data
+
+ return PredictionBURSTFormatToTAOFormatConverter(
+ self.gt_data, data,
+ exemplar_guided=False).convert()
+
+
+def _remap_image_ids(pred_data, ali_gt_data):
+ code_path = utils.get_code_path()
+ if 'split' in ali_gt_data:
+ split = ali_gt_data['split']
+ else:
+ split = 'val'
+
+ if split in ('val', 'validation'):
+ tao_gt_path = os.path.join(
+ code_path, 'data/gt/tao/tao_validation/gt.json')
+ else:
+ tao_gt_path = os.path.join(
+ code_path, 'data/gt/tao/tao_test/test_without_annotations.json')
+
+ with open(tao_gt_path) as f:
+ tao_gt = json.load(f)
+
+ tao_img_by_id = {}
+ for img in tao_gt['images']:
+ img_id = img['id']
+ tao_img_by_id[img_id] = img
+
+ ali_img_id_by_filename = {}
+ for ali_img in ali_gt_data['images']:
+ ali_img_id = ali_img['id']
+ file_name = ali_img['file_name'].replace("validation", "val")
+ ali_img_id_by_filename[file_name] = ali_img_id
+
+ ali_img_id_by_tao_img_id = {}
+ for tao_img_id, tao_img in tao_img_by_id.items():
+ file_name = tao_img['file_name']
+ ali_img_id = ali_img_id_by_filename[file_name]
+ ali_img_id_by_tao_img_id[tao_img_id] = ali_img_id
+
+ for det in pred_data:
+ tao_img_id = det['image_id']
+ ali_img_id = ali_img_id_by_tao_img_id[tao_img_id]
+ det['image_id'] = ali_img_id
diff --git a/val_utils/trackeval/datasets/davis.py b/val_utils/trackeval/datasets/davis.py
new file mode 100644
index 0000000000000000000000000000000000000000..9db25e933b0c4951f2ea70c5c4d806fb6b08ccd5
--- /dev/null
+++ b/val_utils/trackeval/datasets/davis.py
@@ -0,0 +1,276 @@
+import os
+import csv
+import numpy as np
+from ._base_dataset import _BaseDataset
+from ..utils import TrackEvalException
+from .. import utils
+from .. import _timing
+
+
+class DAVIS(_BaseDataset):
+ """Dataset class for DAVIS tracking"""
+
+ @staticmethod
+ def get_default_dataset_config():
+ """Default class config values"""
+ code_path = utils.get_code_path()
+ default_config = {
+ 'GT_FOLDER': os.path.join(code_path, 'data/gt/davis/davis_unsupervised_val/'), # Location of GT data
+ 'TRACKERS_FOLDER': os.path.join(code_path, 'data/trackers/davis/davis_unsupervised_val/'), # Trackers location
+ 'OUTPUT_FOLDER': None, # Where to save eval results (if None, same as TRACKERS_FOLDER)
+ 'TRACKERS_TO_EVAL': None, # Filenames of trackers to eval (if None, all in folder)
+ 'SPLIT_TO_EVAL': 'val', # Valid: 'val', 'train'
+ 'CLASSES_TO_EVAL': ['general'],
+ 'PRINT_CONFIG': True, # Whether to print current config
+ 'TRACKER_SUB_FOLDER': 'data', # Tracker files are in TRACKER_FOLDER/tracker_name/TRACKER_SUB_FOLDER
+ 'OUTPUT_SUB_FOLDER': '', # Output files are saved in OUTPUT_FOLDER/tracker_name/OUTPUT_SUB_FOLDER
+ 'TRACKER_DISPLAY_NAMES': None, # Names of trackers to display, if None: TRACKERS_TO_EVAL
+ 'SEQMAP_FILE': None, # Specify seqmap file
+ 'SEQ_INFO': None, # If not None, directly specify sequences to eval and their number of timesteps
+ # '{gt_folder}/Annotations_unsupervised/480p/{seq}'
+ 'MAX_DETECTIONS': 0 # Maximum number of allowed detections per sequence (0 for no threshold)
+ }
+ return default_config
+
+ def __init__(self, config=None):
+ """Initialise dataset, checking that all required files are present"""
+ super().__init__()
+ # Fill non-given config values with defaults
+ self.config = utils.init_config(config, self.get_default_dataset_config(), self.get_name())
+ # defining a default class since there are no classes in DAVIS
+ self.should_classes_combine = False
+ self.use_super_categories = False
+
+ self.gt_fol = self.config['GT_FOLDER']
+ self.tracker_fol = self.config['TRACKERS_FOLDER']
+
+ self.output_sub_fol = self.config['OUTPUT_SUB_FOLDER']
+ self.tracker_sub_fol = self.config['TRACKER_SUB_FOLDER']
+
+ self.output_fol = self.config['OUTPUT_FOLDER']
+ if self.output_fol is None:
+ self.output_fol = self.config['TRACKERS_FOLDER']
+
+ self.max_det = self.config['MAX_DETECTIONS']
+
+ # Get classes to eval
+ self.valid_classes = ['general']
+ self.class_list = [cls.lower() if cls.lower() in self.valid_classes else None
+ for cls in self.config['CLASSES_TO_EVAL']]
+ if not all(self.class_list):
+ raise TrackEvalException('Attempted to evaluate an invalid class. Only general class is valid.')
+
+ # Get sequences to eval
+ if self.config["SEQ_INFO"]:
+ self.seq_list = list(self.config["SEQ_INFO"].keys())
+ self.seq_lengths = self.config["SEQ_INFO"]
+ elif self.config["SEQMAP_FILE"]:
+ self.seq_list = []
+ seqmap_file = self.config["SEQMAP_FILE"]
+ if not os.path.isfile(seqmap_file):
+ raise TrackEvalException('no seqmap found: ' + os.path.basename(seqmap_file))
+ with open(seqmap_file) as fp:
+ reader = csv.reader(fp)
+ for i, row in enumerate(reader):
+ if row[0] == '':
+ continue
+ seq = row[0]
+ self.seq_list.append(seq)
+ else:
+ self.seq_list = os.listdir(self.gt_fol)
+
+ self.seq_lengths = {seq: len(os.listdir(os.path.join(self.gt_fol, seq))) for seq in self.seq_list}
+
+ # Get trackers to eval
+ if self.config['TRACKERS_TO_EVAL'] is None:
+ self.tracker_list = os.listdir(self.tracker_fol)
+ else:
+ self.tracker_list = self.config['TRACKERS_TO_EVAL']
+ for tracker in self.tracker_list:
+ for seq in self.seq_list:
+ curr_dir = os.path.join(self.tracker_fol, tracker, self.tracker_sub_fol, seq)
+ if not os.path.isdir(curr_dir):
+ print('Tracker directory not found: ' + curr_dir)
+ raise TrackEvalException('Tracker directory not found: ' +
+ os.path.join(tracker, self.tracker_sub_fol, seq))
+ tr_timesteps = len(os.listdir(curr_dir))
+ if self.seq_lengths[seq] != tr_timesteps:
+ raise TrackEvalException('GT folder and tracker folder have a different number'
+ 'timesteps for tracker %s and sequence %s' % (tracker, seq))
+
+ if self.config['TRACKER_DISPLAY_NAMES'] is None:
+ self.tracker_to_disp = dict(zip(self.tracker_list, self.tracker_list))
+ elif (self.config['TRACKERS_TO_EVAL'] is not None) and (
+ len(self.config['TRACKER_DISPLAY_NAMES']) == len(self.tracker_list)):
+ self.tracker_to_disp = dict(zip(self.tracker_list, self.config['TRACKER_DISPLAY_NAMES']))
+ else:
+ raise TrackEvalException('List of tracker files and tracker display names do not match.')
+
+ def _load_raw_file(self, tracker, seq, is_gt):
+ """Load a file (gt or tracker) in the DAVIS format
+
+ If is_gt, this returns a dict which contains the fields:
+ [gt_ids] : list (for each timestep) of 1D NDArrays (for each det).
+ [gt_dets]: list (for each timestep) of lists of detections.
+ [masks_void]: list of masks with void pixels (pixels to be ignored during evaluation)
+
+ if not is_gt, this returns a dict which contains the fields:
+ [tracker_ids] : list (for each timestep) of 1D NDArrays (for each det).
+ [tracker_dets]: list (for each timestep) of lists of detections.
+ """
+
+ # Only loaded when run to reduce minimum requirements
+ from pycocotools import mask as mask_utils
+ from PIL import Image
+
+ # File location
+ if is_gt:
+ seq_dir = os.path.join(self.gt_fol, seq)
+ else:
+ seq_dir = os.path.join(self.tracker_fol, tracker, self.tracker_sub_fol, seq)
+
+ num_timesteps = self.seq_lengths[seq]
+ data_keys = ['ids', 'dets', 'masks_void']
+ raw_data = {key: [None] * num_timesteps for key in data_keys}
+
+ # read frames
+ frames = [os.path.join(seq_dir, im_name) for im_name in sorted(os.listdir(seq_dir))]
+
+ id_list = []
+ for t in range(num_timesteps):
+ frame = np.array(Image.open(frames[t]))
+ if is_gt:
+ void = frame == 255
+ frame[void] = 0
+ raw_data['masks_void'][t] = mask_utils.encode(np.asfortranarray(void.astype(np.uint8)))
+ id_values = np.unique(frame)
+ id_values = id_values[id_values != 0]
+ id_list += list(id_values)
+ tmp = np.ones((len(id_values), *frame.shape))
+ tmp = tmp * id_values[:, None, None]
+ masks = np.array(tmp == frame[None, ...]).astype(np.uint8)
+ raw_data['dets'][t] = mask_utils.encode(np.array(np.transpose(masks, (1, 2, 0)), order='F'))
+ raw_data['ids'][t] = id_values.astype(int)
+ num_objects = len(np.unique(id_list))
+
+ if not is_gt and num_objects > self.max_det > 0:
+ raise Exception('Number of proposals (%i) for sequence %s exceeds number of maximum allowed proposals (%i).'
+ % (num_objects, seq, self.max_det))
+
+ if is_gt:
+ key_map = {'ids': 'gt_ids',
+ 'dets': 'gt_dets'}
+ else:
+ key_map = {'ids': 'tracker_ids',
+ 'dets': 'tracker_dets'}
+ for k, v in key_map.items():
+ raw_data[v] = raw_data.pop(k)
+ raw_data["num_timesteps"] = num_timesteps
+ raw_data['mask_shape'] = np.array(Image.open(frames[0])).shape
+ if is_gt:
+ raw_data['num_gt_ids'] = num_objects
+ else:
+ raw_data['num_tracker_ids'] = num_objects
+ return raw_data
+
+ @_timing.time
+ def get_preprocessed_seq_data(self, raw_data, cls):
+ """ Preprocess data for a single sequence for a single class ready for evaluation.
+ Inputs:
+ - raw_data is a dict containing the data for the sequence already read in by get_raw_seq_data().
+ - cls is the class to be evaluated.
+ Outputs:
+ - data is a dict containing all of the information that metrics need to perform evaluation.
+ It contains the following fields:
+ [num_timesteps, num_gt_ids, num_tracker_ids, num_gt_dets, num_tracker_dets] : integers.
+ [gt_ids, tracker_ids]: list (for each timestep) of 1D NDArrays (for each det).
+ [gt_dets, tracker_dets]: list (for each timestep) of lists of detection masks.
+ [similarity_scores]: list (for each timestep) of 2D NDArrays.
+ Notes:
+ General preprocessing (preproc) occurs in 4 steps. Some datasets may not use all of these steps.
+ 1) Extract only detections relevant for the class to be evaluated (including distractor detections).
+ 2) Match gt dets and tracker dets. Remove tracker dets that are matched to a gt det that is of a
+ distractor class, or otherwise marked as to be removed.
+ 3) Remove unmatched tracker dets if they fall within a crowd ignore region or don't meet a certain
+ other criteria (e.g. are too small).
+ 4) Remove gt dets that were only useful for preprocessing and not for actual evaluation.
+ After the above preprocessing steps, this function also calculates the number of gt and tracker detections
+ and unique track ids. It also relabels gt and tracker ids to be contiguous and checks that ids are
+ unique within each timestep.
+
+ DAVIS:
+ In DAVIS, the 4 preproc steps are as follow:
+ 1) There are no classes, all detections are evaluated jointly
+ 2) No matched tracker detections are removed.
+ 3) No unmatched tracker detections are removed.
+ 4) There are no ground truth detections (e.g. those of distractor classes) to be removed.
+ Preprocessing special to DAVIS: Pixels which are marked as void in the ground truth are set to zero in the
+ tracker detections since they are not considered during evaluation.
+ """
+
+ # Only loaded when run to reduce minimum requirements
+ from pycocotools import mask as mask_utils
+
+ data_keys = ['gt_ids', 'tracker_ids', 'gt_dets', 'tracker_dets', 'similarity_scores']
+ data = {key: [None] * raw_data['num_timesteps'] for key in data_keys}
+ num_gt_dets = 0
+ num_tracker_dets = 0
+ unique_gt_ids = []
+ unique_tracker_ids = []
+ num_timesteps = raw_data['num_timesteps']
+
+ # count detections
+ for t in range(num_timesteps):
+ num_gt_dets += len(raw_data['gt_dets'][t])
+ num_tracker_dets += len(raw_data['tracker_dets'][t])
+ unique_gt_ids += list(np.unique(raw_data['gt_ids'][t]))
+ unique_tracker_ids += list(np.unique(raw_data['tracker_ids'][t]))
+
+ data['gt_ids'] = raw_data['gt_ids']
+ data['gt_dets'] = raw_data['gt_dets']
+ data['similarity_scores'] = raw_data['similarity_scores']
+ data['tracker_ids'] = raw_data['tracker_ids']
+
+ # set void pixels in tracker detections to zero
+ for t in range(num_timesteps):
+ void_mask = raw_data['masks_void'][t]
+ if mask_utils.area(void_mask) > 0:
+ void_mask_ious = np.atleast_1d(mask_utils.iou(raw_data['tracker_dets'][t], [void_mask], [False]))
+ if void_mask_ious.any():
+ rows, columns = np.where(void_mask_ious > 0)
+ for r in rows:
+ det = mask_utils.decode(raw_data['tracker_dets'][t][r])
+ void = mask_utils.decode(void_mask).astype(np.bool)
+ det[void] = 0
+ det = mask_utils.encode(np.array(det, order='F').astype(np.uint8))
+ raw_data['tracker_dets'][t][r] = det
+ data['tracker_dets'] = raw_data['tracker_dets']
+
+ # Re-label IDs such that there are no empty IDs
+ if len(unique_gt_ids) > 0:
+ unique_gt_ids = np.unique(unique_gt_ids)
+ gt_id_map = np.nan * np.ones((np.max(unique_gt_ids) + 1))
+ gt_id_map[unique_gt_ids] = np.arange(len(unique_gt_ids))
+ for t in range(raw_data['num_timesteps']):
+ if len(data['gt_ids'][t]) > 0:
+ data['gt_ids'][t] = gt_id_map[data['gt_ids'][t]].astype(np.int)
+ if len(unique_tracker_ids) > 0:
+ unique_tracker_ids = np.unique(unique_tracker_ids)
+ tracker_id_map = np.nan * np.ones((np.max(unique_tracker_ids) + 1))
+ tracker_id_map[unique_tracker_ids] = np.arange(len(unique_tracker_ids))
+ for t in range(raw_data['num_timesteps']):
+ if len(data['tracker_ids'][t]) > 0:
+ data['tracker_ids'][t] = tracker_id_map[data['tracker_ids'][t]].astype(np.int)
+
+ # Record overview statistics.
+ data['num_tracker_dets'] = num_tracker_dets
+ data['num_gt_dets'] = num_gt_dets
+ data['num_tracker_ids'] = raw_data['num_tracker_ids']
+ data['num_gt_ids'] = raw_data['num_gt_ids']
+ data['mask_shape'] = raw_data['mask_shape']
+ data['num_timesteps'] = num_timesteps
+ return data
+
+ def _calculate_similarities(self, gt_dets_t, tracker_dets_t):
+ similarity_scores = self._calculate_mask_ious(gt_dets_t, tracker_dets_t, is_encoded=True, do_ioa=False)
+ return similarity_scores
diff --git a/val_utils/trackeval/datasets/head_tracking_challenge.py b/val_utils/trackeval/datasets/head_tracking_challenge.py
new file mode 100644
index 0000000000000000000000000000000000000000..469e9a3c7701c384ce9ad9149fcdff2cc896afac
--- /dev/null
+++ b/val_utils/trackeval/datasets/head_tracking_challenge.py
@@ -0,0 +1,459 @@
+import os
+import csv
+import configparser
+import numpy as np
+from scipy.optimize import linear_sum_assignment
+from ._base_dataset import _BaseDataset
+from .. import utils
+from .. import _timing
+from ..utils import TrackEvalException
+
+
+class HeadTrackingChallenge(_BaseDataset):
+ """Dataset class for Head Tracking Challenge - 2D bounding box tracking"""
+
+ @staticmethod
+ def get_default_dataset_config():
+ """Default class config values"""
+ code_path = utils.get_code_path()
+ default_config = {
+ 'GT_FOLDER': os.path.join(code_path, 'data/gt/mot_challenge/'), # Location of GT data
+ 'TRACKERS_FOLDER': os.path.join(code_path, 'data/trackers/mot_challenge/'), # Trackers location
+ 'OUTPUT_FOLDER': None, # Where to save eval results (if None, same as TRACKERS_FOLDER)
+ 'TRACKERS_TO_EVAL': None, # Filenames of trackers to eval (if None, all in folder)
+ 'CLASSES_TO_EVAL': ['pedestrian'], # Valid: ['pedestrian']
+ 'BENCHMARK': 'HT', # Valid: 'HT'. Refers to "Head Tracking or the dataset CroHD"
+ 'SPLIT_TO_EVAL': 'train', # Valid: 'train', 'test', 'all'
+ 'INPUT_AS_ZIP': False, # Whether tracker input files are zipped
+ 'PRINT_CONFIG': True, # Whether to print current config
+ 'DO_PREPROC': True, # Whether to perform preprocessing (never done for MOT15)
+ 'TRACKER_SUB_FOLDER': 'data', # Tracker files are in TRACKER_FOLDER/tracker_name/TRACKER_SUB_FOLDER
+ 'OUTPUT_SUB_FOLDER': '', # Output files are saved in OUTPUT_FOLDER/tracker_name/OUTPUT_SUB_FOLDER
+ 'TRACKER_DISPLAY_NAMES': None, # Names of trackers to display, if None: TRACKERS_TO_EVAL
+ 'SEQMAP_FOLDER': None, # Where seqmaps are found (if None, GT_FOLDER/seqmaps)
+ 'SEQMAP_FILE': None, # Directly specify seqmap file (if none use seqmap_folder/benchmark-split_to_eval)
+ 'SEQ_INFO': None, # If not None, directly specify sequences to eval and their number of timesteps
+ 'GT_LOC_FORMAT': '{gt_folder}/{seq}/gt/gt.txt', # '{gt_folder}/{seq}/gt/gt.txt'
+ 'SKIP_SPLIT_FOL': False, # If False, data is in GT_FOLDER/BENCHMARK-SPLIT_TO_EVAL/ and in
+ # TRACKERS_FOLDER/BENCHMARK-SPLIT_TO_EVAL/tracker/
+ # If True, then the middle 'benchmark-split' folder is skipped for both.
+ }
+ return default_config
+
+ def __init__(self, config=None):
+ """Initialise dataset, checking that all required files are present"""
+ super().__init__()
+ # Fill non-given config values with defaults
+ self.config = utils.init_config(config, self.get_default_dataset_config(), self.get_name())
+
+ self.benchmark = self.config['BENCHMARK']
+ gt_set = self.config['BENCHMARK'] + '-' + self.config['SPLIT_TO_EVAL']
+ self.gt_set = gt_set
+ if not self.config['SKIP_SPLIT_FOL']:
+ split_fol = gt_set
+ else:
+ split_fol = ''
+ self.gt_fol = os.path.join(self.config['GT_FOLDER'], split_fol)
+ self.tracker_fol = os.path.join(self.config['TRACKERS_FOLDER'], split_fol)
+ self.should_classes_combine = False
+ self.use_super_categories = False
+ self.data_is_zipped = self.config['INPUT_AS_ZIP']
+ self.do_preproc = self.config['DO_PREPROC']
+
+ self.output_fol = self.config['OUTPUT_FOLDER']
+ if self.output_fol is None:
+ self.output_fol = self.tracker_fol
+
+ self.tracker_sub_fol = self.config['TRACKER_SUB_FOLDER']
+ self.output_sub_fol = self.config['OUTPUT_SUB_FOLDER']
+
+ # Get classes to eval
+ self.valid_classes = ['pedestrian']
+ self.class_list = [cls.lower() if cls.lower() in self.valid_classes else None
+ for cls in self.config['CLASSES_TO_EVAL']]
+ if not all(self.class_list):
+ raise TrackEvalException('Attempted to evaluate an invalid class. Only pedestrian class is valid.')
+ self.class_name_to_class_id = {'pedestrian': 1, 'static': 2, 'ignore': 3, 'person_on_vehicle': 4}
+ self.valid_class_numbers = list(self.class_name_to_class_id.values())
+
+ # Get sequences to eval and check gt files exist
+ self.seq_list, self.seq_lengths = self._get_seq_info()
+ if len(self.seq_list) < 1:
+ raise TrackEvalException('No sequences are selected to be evaluated.')
+
+ # Check gt files exist
+ for seq in self.seq_list:
+ if not self.data_is_zipped:
+ curr_file = self.config["GT_LOC_FORMAT"].format(gt_folder=self.gt_fol, seq=seq)
+ if not os.path.isfile(curr_file):
+ print('GT file not found ' + curr_file)
+ raise TrackEvalException('GT file not found for sequence: ' + seq)
+ if self.data_is_zipped:
+ curr_file = os.path.join(self.gt_fol, 'data.zip')
+ if not os.path.isfile(curr_file):
+ print('GT file not found ' + curr_file)
+ raise TrackEvalException('GT file not found: ' + os.path.basename(curr_file))
+
+ # Get trackers to eval
+ if self.config['TRACKERS_TO_EVAL'] is None:
+ self.tracker_list = os.listdir(self.tracker_fol)
+ else:
+ self.tracker_list = self.config['TRACKERS_TO_EVAL']
+
+ if self.config['TRACKER_DISPLAY_NAMES'] is None:
+ self.tracker_to_disp = dict(zip(self.tracker_list, self.tracker_list))
+ elif (self.config['TRACKERS_TO_EVAL'] is not None) and (
+ len(self.config['TRACKER_DISPLAY_NAMES']) == len(self.tracker_list)):
+ self.tracker_to_disp = dict(zip(self.tracker_list, self.config['TRACKER_DISPLAY_NAMES']))
+ else:
+ raise TrackEvalException('List of tracker files and tracker display names do not match.')
+
+ for tracker in self.tracker_list:
+ if self.data_is_zipped:
+ curr_file = os.path.join(self.tracker_fol, tracker, self.tracker_sub_fol + '.zip')
+ if not os.path.isfile(curr_file):
+ print('Tracker file not found: ' + curr_file)
+ raise TrackEvalException('Tracker file not found: ' + tracker + '/' + os.path.basename(curr_file))
+ else:
+ for seq in self.seq_list:
+ curr_file = os.path.join(self.tracker_fol, tracker, self.tracker_sub_fol, seq + '.txt')
+ if not os.path.isfile(curr_file):
+ print('Tracker file not found: ' + curr_file)
+ raise TrackEvalException(
+ 'Tracker file not found: ' + tracker + '/' + self.tracker_sub_fol + '/' + os.path.basename(
+ curr_file))
+
+ def get_display_name(self, tracker):
+ return self.tracker_to_disp[tracker]
+
+ def _get_seq_info(self):
+ seq_list = []
+ seq_lengths = {}
+ if self.config["SEQ_INFO"]:
+ seq_list = list(self.config["SEQ_INFO"].keys())
+ seq_lengths = self.config["SEQ_INFO"]
+
+ # If sequence length is 'None' tries to read sequence length from .ini files.
+ for seq, seq_length in seq_lengths.items():
+ if seq_length is None:
+ ini_file = os.path.join(self.gt_fol, seq, 'seqinfo.ini')
+ if not os.path.isfile(ini_file):
+ raise TrackEvalException('ini file does not exist: ' + seq + '/' + os.path.basename(ini_file))
+ ini_data = configparser.ConfigParser()
+ ini_data.read(ini_file)
+ seq_lengths[seq] = int(ini_data['Sequence']['seqLength'])
+
+ else:
+ if self.config["SEQMAP_FILE"]:
+ seqmap_file = self.config["SEQMAP_FILE"]
+ else:
+ if self.config["SEQMAP_FOLDER"] is None:
+ seqmap_file = os.path.join(self.config['GT_FOLDER'], 'seqmaps', self.gt_set + '.txt')
+ else:
+ seqmap_file = os.path.join(self.config["SEQMAP_FOLDER"], self.gt_set + '.txt')
+ if not os.path.isfile(seqmap_file):
+ print('no seqmap found: ' + seqmap_file)
+ raise TrackEvalException('no seqmap found: ' + os.path.basename(seqmap_file))
+ with open(seqmap_file) as fp:
+ reader = csv.reader(fp)
+ for i, row in enumerate(reader):
+ if i == 0 or row[0] == '':
+ continue
+ seq = row[0]
+ seq_list.append(seq)
+ ini_file = os.path.join(self.gt_fol, seq, 'seqinfo.ini')
+ if not os.path.isfile(ini_file):
+ raise TrackEvalException('ini file does not exist: ' + seq + '/' + os.path.basename(ini_file))
+ ini_data = configparser.ConfigParser()
+ ini_data.read(ini_file)
+ seq_lengths[seq] = int(ini_data['Sequence']['seqLength'])
+ return seq_list, seq_lengths
+
+ def _load_raw_file(self, tracker, seq, is_gt):
+ """Load a file (gt or tracker) in the MOT Challenge 2D box format
+
+ If is_gt, this returns a dict which contains the fields:
+ [gt_ids, gt_classes] : list (for each timestep) of 1D NDArrays (for each det).
+ [gt_dets, gt_crowd_ignore_regions]: list (for each timestep) of lists of detections.
+ [gt_extras] : list (for each timestep) of dicts (for each extra) of 1D NDArrays (for each det).
+
+ if not is_gt, this returns a dict which contains the fields:
+ [tracker_ids, tracker_classes, tracker_confidences] : list (for each timestep) of 1D NDArrays (for each det).
+ [tracker_dets]: list (for each timestep) of lists of detections.
+ """
+ # File location
+ if self.data_is_zipped:
+ if is_gt:
+ zip_file = os.path.join(self.gt_fol, 'data.zip')
+ else:
+ zip_file = os.path.join(self.tracker_fol, tracker, self.tracker_sub_fol + '.zip')
+ file = seq + '.txt'
+ else:
+ zip_file = None
+ if is_gt:
+ file = self.config["GT_LOC_FORMAT"].format(gt_folder=self.gt_fol, seq=seq)
+ else:
+ file = os.path.join(self.tracker_fol, tracker, self.tracker_sub_fol, seq + '.txt')
+
+ # Load raw data from text file
+ read_data, ignore_data = self._load_simple_text_file(file, is_zipped=self.data_is_zipped, zip_file=zip_file)
+
+ # Convert data to required format
+ num_timesteps = self.seq_lengths[seq]
+ data_keys = ['ids', 'classes', 'dets']
+ if is_gt:
+ data_keys += ['gt_crowd_ignore_regions', 'gt_extras']
+ else:
+ data_keys += ['tracker_confidences']
+
+ if self.benchmark == 'HT':
+ data_keys += ['visibility']
+ data_keys += ['gt_conf']
+ raw_data = {key: [None] * num_timesteps for key in data_keys}
+
+ # Check for any extra time keys
+ current_time_keys = [str( t+ 1) for t in range(num_timesteps)]
+ extra_time_keys = [x for x in read_data.keys() if x not in current_time_keys]
+ if len(extra_time_keys) > 0:
+ if is_gt:
+ text = 'Ground-truth'
+ else:
+ text = 'Tracking'
+ raise TrackEvalException(
+ text + ' data contains the following invalid timesteps in seq %s: ' % seq + ', '.join(
+ [str(x) + ', ' for x in extra_time_keys]))
+
+ for t in range(num_timesteps):
+ time_key = str(t+1)
+ if time_key in read_data.keys():
+ try:
+ time_data = np.asarray(read_data[time_key], dtype=np.float)
+ except ValueError:
+ if is_gt:
+ raise TrackEvalException(
+ 'Cannot convert gt data for sequence %s to float. Is data corrupted?' % seq)
+ else:
+ raise TrackEvalException(
+ 'Cannot convert tracking data from tracker %s, sequence %s to float. Is data corrupted?' % (
+ tracker, seq))
+ try:
+ raw_data['dets'][t] = np.atleast_2d(time_data[:, 2:6])
+ raw_data['ids'][t] = np.atleast_1d(time_data[:, 1]).astype(int)
+ except IndexError:
+ if is_gt:
+ err = 'Cannot load gt data from sequence %s, because there is not enough ' \
+ 'columns in the data.' % seq
+ raise TrackEvalException(err)
+ else:
+ err = 'Cannot load tracker data from tracker %s, sequence %s, because there is not enough ' \
+ 'columns in the data.' % (tracker, seq)
+ raise TrackEvalException(err)
+ if time_data.shape[1] >= 8:
+ raw_data['gt_conf'][t] = np.atleast_1d(time_data[:, 6]).astype(float)
+ raw_data['visibility'][t] = np.atleast_1d(time_data[:, 8]).astype(float)
+ raw_data['classes'][t] = np.atleast_1d(time_data[:, 7]).astype(int)
+ else:
+ if not is_gt:
+ raw_data['classes'][t] = np.ones_like(raw_data['ids'][t])
+ else:
+ raise TrackEvalException(
+ 'GT data is not in a valid format, there is not enough rows in seq %s, timestep %i.' % (
+ seq, t))
+ if is_gt:
+ gt_extras_dict = {'zero_marked': np.atleast_1d(time_data[:, 6].astype(int))}
+ raw_data['gt_extras'][t] = gt_extras_dict
+ else:
+ raw_data['tracker_confidences'][t] = np.atleast_1d(time_data[:, 6])
+ else:
+ raw_data['dets'][t] = np.empty((0, 4))
+ raw_data['ids'][t] = np.empty(0).astype(int)
+ raw_data['classes'][t] = np.empty(0).astype(int)
+ if is_gt:
+ gt_extras_dict = {'zero_marked': np.empty(0)}
+ raw_data['gt_extras'][t] = gt_extras_dict
+ else:
+ raw_data['tracker_confidences'][t] = np.empty(0)
+ if is_gt:
+ raw_data['gt_crowd_ignore_regions'][t] = np.empty((0, 4))
+
+ if is_gt:
+ key_map = {'ids': 'gt_ids',
+ 'classes': 'gt_classes',
+ 'dets': 'gt_dets'}
+ else:
+ key_map = {'ids': 'tracker_ids',
+ 'classes': 'tracker_classes',
+ 'dets': 'tracker_dets'}
+ for k, v in key_map.items():
+ raw_data[v] = raw_data.pop(k)
+ raw_data['num_timesteps'] = num_timesteps
+ raw_data['seq'] = seq
+ return raw_data
+
+ @_timing.time
+ def get_preprocessed_seq_data(self, raw_data, cls):
+ """ Preprocess data for a single sequence for a single class ready for evaluation.
+ Inputs:
+ - raw_data is a dict containing the data for the sequence already read in by get_raw_seq_data().
+ - cls is the class to be evaluated.
+ Outputs:
+ - data is a dict containing all of the information that metrics need to perform evaluation.
+ It contains the following fields:
+ [num_timesteps, num_gt_ids, num_tracker_ids, num_gt_dets, num_tracker_dets] : integers.
+ [gt_ids, tracker_ids, tracker_confidences]: list (for each timestep) of 1D NDArrays (for each det).
+ [gt_dets, tracker_dets]: list (for each timestep) of lists of detections.
+ [similarity_scores]: list (for each timestep) of 2D NDArrays.
+ Notes:
+ General preprocessing (preproc) occurs in 4 steps. Some datasets may not use all of these steps.
+ 1) Extract only detections relevant for the class to be evaluated (including distractor detections).
+ 2) Match gt dets and tracker dets. Remove tracker dets that are matched to a gt det that is of a
+ distractor class, or otherwise marked as to be removed.
+ 3) Remove unmatched tracker dets if they fall within a crowd ignore region or don't meet a certain
+ other criteria (e.g. are too small).
+ 4) Remove gt dets that were only useful for preprocessing and not for actual evaluation.
+ After the above preprocessing steps, this function also calculates the number of gt and tracker detections
+ and unique track ids. It also relabels gt and tracker ids to be contiguous and checks that ids are
+ unique within each timestep.
+
+ MOT Challenge:
+ In MOT Challenge, the 4 preproc steps are as follow:
+ 1) There is only one class (pedestrian) to be evaluated, but all other classes are used for preproc.
+ 2) Predictions are matched against all gt boxes (regardless of class), those matching with distractor
+ objects are removed.
+ 3) There is no crowd ignore regions.
+ 4) All gt dets except pedestrian are removed, also removes pedestrian gt dets marked with zero_marked.
+ """
+ # Check that input data has unique ids
+ self._check_unique_ids(raw_data)
+
+ # 'static': 2, 'ignore': 3, 'person_on_vehicle':
+
+ distractor_class_names = ['static', 'ignore', 'person_on_vehicle']
+
+ distractor_classes = [self.class_name_to_class_id[x] for x in distractor_class_names]
+ cls_id = self.class_name_to_class_id[cls]
+
+ data_keys = ['gt_ids', 'tracker_ids', 'gt_dets', 'tracker_dets', 'tracker_confidences',
+ 'similarity_scores', 'gt_visibility']
+ data = {key: [None] * raw_data['num_timesteps'] for key in data_keys}
+ unique_gt_ids = []
+ unique_tracker_ids = []
+ num_gt_dets = 0
+ num_tracker_dets = 0
+ for t in range(raw_data['num_timesteps']):
+
+ # Get all data
+ gt_ids = raw_data['gt_ids'][t]
+ gt_dets = raw_data['gt_dets'][t]
+ gt_classes = raw_data['gt_classes'][t]
+ gt_visibility = raw_data['visibility'][t]
+ gt_conf = raw_data['gt_conf'][t]
+
+ gt_zero_marked = raw_data['gt_extras'][t]['zero_marked']
+
+ tracker_ids = raw_data['tracker_ids'][t]
+ tracker_dets = raw_data['tracker_dets'][t]
+ tracker_classes = raw_data['tracker_classes'][t]
+ tracker_confidences = raw_data['tracker_confidences'][t]
+ similarity_scores = raw_data['similarity_scores'][t]
+
+ # Evaluation is ONLY valid for pedestrian class
+ if len(tracker_classes) > 0 and np.max(tracker_classes) > 1:
+ raise TrackEvalException(
+ 'Evaluation is only valid for pedestrian class. Non pedestrian class (%i) found in sequence %s at '
+ 'timestep %i.' % (np.max(tracker_classes), raw_data['seq'], t))
+
+ # Match tracker and gt dets (with hungarian algorithm) and remove tracker dets which match with gt dets
+ # which are labeled as belonging to a distractor class.
+ to_remove_tracker = np.array([], np.int)
+ if self.do_preproc and self.benchmark != 'MOT15' and gt_ids.shape[0] > 0 and tracker_ids.shape[0] > 0:
+
+ # Check all classes are valid:
+ invalid_classes = np.setdiff1d(np.unique(gt_classes), self.valid_class_numbers)
+ if len(invalid_classes) > 0:
+ print(' '.join([str(x) for x in invalid_classes]))
+ raise(TrackEvalException('Attempting to evaluate using invalid gt classes. '
+ 'This warning only triggers if preprocessing is performed, '
+ 'e.g. not for MOT15 or where prepropressing is explicitly disabled. '
+ 'Please either check your gt data, or disable preprocessing. '
+ 'The following invalid classes were found in timestep ' + str(t) + ': ' +
+ ' '.join([str(x) for x in invalid_classes])))
+
+ matching_scores = similarity_scores.copy()
+
+ matching_scores[matching_scores < 0.4 - np.finfo('float').eps] = 0
+
+ match_rows, match_cols = linear_sum_assignment(-matching_scores)
+ actually_matched_mask = matching_scores[match_rows, match_cols] > 0 + np.finfo('float').eps
+ match_rows = match_rows[actually_matched_mask]
+ match_cols = match_cols[actually_matched_mask]
+
+ is_distractor_class = np.logical_not(np.isin(gt_classes[match_rows], cls_id))
+ if self.benchmark == 'HT':
+ is_invisible_class = gt_visibility[match_rows] < np.finfo('float').eps
+ low_conf_class = gt_conf[match_rows] < np.finfo('float').eps
+ are_distractors = np.logical_or(is_invisible_class, is_distractor_class, low_conf_class)
+ to_remove_tracker = match_cols[are_distractors]
+ else:
+ to_remove_tracker = match_cols[is_distractor_class]
+
+ # Apply preprocessing to remove all unwanted tracker dets.
+ data['tracker_ids'][t] = np.delete(tracker_ids, to_remove_tracker, axis=0)
+ data['tracker_dets'][t] = np.delete(tracker_dets, to_remove_tracker, axis=0)
+ data['tracker_confidences'][t] = np.delete(tracker_confidences, to_remove_tracker, axis=0)
+ similarity_scores = np.delete(similarity_scores, to_remove_tracker, axis=1)
+
+ # Remove gt detections marked as to remove (zero marked), and also remove gt detections not in pedestrian
+ if self.do_preproc and self.benchmark == 'HT':
+ gt_to_keep_mask = (np.not_equal(gt_zero_marked, 0)) & \
+ (np.equal(gt_classes, cls_id)) & \
+ (gt_visibility > 0.) & \
+ (gt_conf > 0.)
+
+ else:
+ # There are no classes for MOT15
+ gt_to_keep_mask = np.not_equal(gt_zero_marked, 0)
+ data['gt_ids'][t] = gt_ids[gt_to_keep_mask]
+ data['gt_dets'][t] = gt_dets[gt_to_keep_mask, :]
+ data['similarity_scores'][t] = similarity_scores[gt_to_keep_mask]
+ data['gt_visibility'][t] = gt_visibility # No mask!
+
+
+ unique_gt_ids += list(np.unique(data['gt_ids'][t]))
+ unique_tracker_ids += list(np.unique(data['tracker_ids'][t]))
+ num_tracker_dets += len(data['tracker_ids'][t])
+ num_gt_dets += len(data['gt_ids'][t])
+
+
+ # Re-label IDs such that there are no empty IDs
+ if len(unique_gt_ids) > 0:
+ unique_gt_ids = np.unique(unique_gt_ids)
+ gt_id_map = np.nan * np.ones((np.max(unique_gt_ids) + 1))
+ gt_id_map[unique_gt_ids] = np.arange(len(unique_gt_ids))
+ for t in range(raw_data['num_timesteps']):
+ if len(data['gt_ids'][t]) > 0:
+ data['gt_ids'][t] = gt_id_map[data['gt_ids'][t]].astype(np.int)
+ if len(unique_tracker_ids) > 0:
+ unique_tracker_ids = np.unique(unique_tracker_ids)
+ tracker_id_map = np.nan * np.ones((np.max(unique_tracker_ids) + 1))
+ tracker_id_map[unique_tracker_ids] = np.arange(len(unique_tracker_ids))
+ for t in range(raw_data['num_timesteps']):
+ if len(data['tracker_ids'][t]) > 0:
+ data['tracker_ids'][t] = tracker_id_map[data['tracker_ids'][t]].astype(np.int)
+
+ # Record overview statistics.
+ data['num_tracker_dets'] = num_tracker_dets
+ data['num_gt_dets'] = num_gt_dets
+ data['num_tracker_ids'] = len(unique_tracker_ids)
+ data['num_gt_ids'] = len(unique_gt_ids)
+ data['num_timesteps'] = raw_data['num_timesteps']
+ data['seq'] = raw_data['seq']
+
+ # Ensure again that ids are unique per timestep after preproc.
+ self._check_unique_ids(data, after_preproc=True)
+
+ return data
+
+ def _calculate_similarities(self, gt_dets_t, tracker_dets_t):
+ similarity_scores = self._calculate_box_ious(gt_dets_t, tracker_dets_t, box_format='xywh')
+ return similarity_scores
diff --git a/val_utils/trackeval/datasets/kitti_2d_box.py b/val_utils/trackeval/datasets/kitti_2d_box.py
new file mode 100644
index 0000000000000000000000000000000000000000..c582c4378fc347d4c3b9f264523784c2b79b76d8
--- /dev/null
+++ b/val_utils/trackeval/datasets/kitti_2d_box.py
@@ -0,0 +1,389 @@
+
+import os
+import csv
+import numpy as np
+from scipy.optimize import linear_sum_assignment
+from ._base_dataset import _BaseDataset
+from .. import utils
+from ..utils import TrackEvalException
+from .. import _timing
+
+
+class Kitti2DBox(_BaseDataset):
+ """Dataset class for KITTI 2D bounding box tracking"""
+
+ @staticmethod
+ def get_default_dataset_config():
+ """Default class config values"""
+ code_path = utils.get_code_path()
+ default_config = {
+ 'GT_FOLDER': os.path.join(code_path, 'data/gt/kitti/kitti_2d_box_train'), # Location of GT data
+ 'TRACKERS_FOLDER': os.path.join(code_path, 'data/trackers/kitti/kitti_2d_box_train/'), # Trackers location
+ 'OUTPUT_FOLDER': None, # Where to save eval results (if None, same as TRACKERS_FOLDER)
+ 'TRACKERS_TO_EVAL': None, # Filenames of trackers to eval (if None, all in folder)
+ 'CLASSES_TO_EVAL': ['car', 'pedestrian'], # Valid: ['car', 'pedestrian']
+ 'SPLIT_TO_EVAL': 'training', # Valid: 'training', 'val', 'training_minus_val', 'test'
+ 'INPUT_AS_ZIP': False, # Whether tracker input files are zipped
+ 'PRINT_CONFIG': True, # Whether to print current config
+ 'TRACKER_SUB_FOLDER': 'data', # Tracker files are in TRACKER_FOLDER/tracker_name/TRACKER_SUB_FOLDER
+ 'OUTPUT_SUB_FOLDER': '', # Output files are saved in OUTPUT_FOLDER/tracker_name/OUTPUT_SUB_FOLDER
+ 'TRACKER_DISPLAY_NAMES': None, # Names of trackers to display, if None: TRACKERS_TO_EVAL
+ }
+ return default_config
+
+ def __init__(self, config=None):
+ """Initialise dataset, checking that all required files are present"""
+ super().__init__()
+ # Fill non-given config values with defaults
+ self.config = utils.init_config(config, self.get_default_dataset_config(), self.get_name())
+ self.gt_fol = self.config['GT_FOLDER']
+ self.tracker_fol = self.config['TRACKERS_FOLDER']
+ self.should_classes_combine = False
+ self.use_super_categories = False
+ self.data_is_zipped = self.config['INPUT_AS_ZIP']
+
+ self.output_fol = self.config['OUTPUT_FOLDER']
+ if self.output_fol is None:
+ self.output_fol = self.tracker_fol
+
+ self.tracker_sub_fol = self.config['TRACKER_SUB_FOLDER']
+ self.output_sub_fol = self.config['OUTPUT_SUB_FOLDER']
+
+ self.max_occlusion = 2
+ self.max_truncation = 0
+ self.min_height = 25
+
+ # Get classes to eval
+ self.valid_classes = ['car', 'pedestrian']
+ self.class_list = [cls.lower() if cls.lower() in self.valid_classes else None
+ for cls in self.config['CLASSES_TO_EVAL']]
+ if not all(self.class_list):
+ raise TrackEvalException('Attempted to evaluate an invalid class. Only classes [car, pedestrian] are valid.')
+ self.class_name_to_class_id = {'car': 1, 'van': 2, 'truck': 3, 'pedestrian': 4, 'person': 5, # person sitting
+ 'cyclist': 6, 'tram': 7, 'misc': 8, 'dontcare': 9, 'car_2': 1}
+
+ # Get sequences to eval and check gt files exist
+ self.seq_list = []
+ self.seq_lengths = {}
+ seqmap_name = 'evaluate_tracking.seqmap.' + self.config['SPLIT_TO_EVAL']
+ seqmap_file = os.path.join(self.gt_fol, seqmap_name)
+ if not os.path.isfile(seqmap_file):
+ raise TrackEvalException('no seqmap found: ' + os.path.basename(seqmap_file))
+ with open(seqmap_file) as fp:
+ dialect = csv.Sniffer().sniff(fp.read(1024))
+ fp.seek(0)
+ reader = csv.reader(fp, dialect)
+ for row in reader:
+ if len(row) >= 4:
+ seq = row[0]
+ self.seq_list.append(seq)
+ self.seq_lengths[seq] = int(row[3])
+ if not self.data_is_zipped:
+ curr_file = os.path.join(self.gt_fol, 'label_02', seq + '.txt')
+ if not os.path.isfile(curr_file):
+ raise TrackEvalException('GT file not found: ' + os.path.basename(curr_file))
+ if self.data_is_zipped:
+ curr_file = os.path.join(self.gt_fol, 'data.zip')
+ if not os.path.isfile(curr_file):
+ raise TrackEvalException('GT file not found: ' + os.path.basename(curr_file))
+
+ # Get trackers to eval
+ if self.config['TRACKERS_TO_EVAL'] is None:
+ self.tracker_list = os.listdir(self.tracker_fol)
+ else:
+ self.tracker_list = self.config['TRACKERS_TO_EVAL']
+
+ if self.config['TRACKER_DISPLAY_NAMES'] is None:
+ self.tracker_to_disp = dict(zip(self.tracker_list, self.tracker_list))
+ elif (self.config['TRACKERS_TO_EVAL'] is not None) and (
+ len(self.config['TRACKER_DISPLAY_NAMES']) == len(self.tracker_list)):
+ self.tracker_to_disp = dict(zip(self.tracker_list, self.config['TRACKER_DISPLAY_NAMES']))
+ else:
+ raise TrackEvalException('List of tracker files and tracker display names do not match.')
+
+ for tracker in self.tracker_list:
+ if self.data_is_zipped:
+ curr_file = os.path.join(self.tracker_fol, tracker, self.tracker_sub_fol + '.zip')
+ if not os.path.isfile(curr_file):
+ raise TrackEvalException('Tracker file not found: ' + tracker + '/' + os.path.basename(curr_file))
+ else:
+ for seq in self.seq_list:
+ curr_file = os.path.join(self.tracker_fol, tracker, self.tracker_sub_fol, seq + '.txt')
+ if not os.path.isfile(curr_file):
+ raise TrackEvalException(
+ 'Tracker file not found: ' + tracker + '/' + self.tracker_sub_fol + '/' + os.path.basename(
+ curr_file))
+
+ def get_display_name(self, tracker):
+ return self.tracker_to_disp[tracker]
+
+ def _load_raw_file(self, tracker, seq, is_gt):
+ """Load a file (gt or tracker) in the kitti 2D box format
+
+ If is_gt, this returns a dict which contains the fields:
+ [gt_ids, gt_classes] : list (for each timestep) of 1D NDArrays (for each det).
+ [gt_dets, gt_crowd_ignore_regions]: list (for each timestep) of lists of detections.
+ [gt_extras] : list (for each timestep) of dicts (for each extra) of 1D NDArrays (for each det).
+
+ if not is_gt, this returns a dict which contains the fields:
+ [tracker_ids, tracker_classes, tracker_confidences] : list (for each timestep) of 1D NDArrays (for each det).
+ [tracker_dets]: list (for each timestep) of lists of detections.
+ """
+ # File location
+ if self.data_is_zipped:
+ if is_gt:
+ zip_file = os.path.join(self.gt_fol, 'data.zip')
+ else:
+ zip_file = os.path.join(self.tracker_fol, tracker, self.tracker_sub_fol + '.zip')
+ file = seq + '.txt'
+ else:
+ zip_file = None
+ if is_gt:
+ file = os.path.join(self.gt_fol, 'label_02', seq + '.txt')
+ else:
+ file = os.path.join(self.tracker_fol, tracker, self.tracker_sub_fol, seq + '.txt')
+
+ # Ignore regions
+ if is_gt:
+ crowd_ignore_filter = {2: ['dontcare']}
+ else:
+ crowd_ignore_filter = None
+
+ # Valid classes
+ valid_filter = {2: [x for x in self.class_list]}
+ if is_gt:
+ if 'car' in self.class_list:
+ valid_filter[2].append('van')
+ if 'pedestrian' in self.class_list:
+ valid_filter[2] += ['person']
+
+ # Convert kitti class strings to class ids
+ convert_filter = {2: self.class_name_to_class_id}
+
+ # Load raw data from text file
+ read_data, ignore_data = self._load_simple_text_file(file, time_col=0, id_col=1, remove_negative_ids=True,
+ valid_filter=valid_filter,
+ crowd_ignore_filter=crowd_ignore_filter,
+ convert_filter=convert_filter,
+ is_zipped=self.data_is_zipped, zip_file=zip_file)
+ # Convert data to required format
+ num_timesteps = self.seq_lengths[seq]
+ data_keys = ['ids', 'classes', 'dets']
+ if is_gt:
+ data_keys += ['gt_crowd_ignore_regions', 'gt_extras']
+ else:
+ data_keys += ['tracker_confidences']
+ raw_data = {key: [None] * num_timesteps for key in data_keys}
+
+ # Check for any extra time keys
+ current_time_keys = [str(t) for t in range(num_timesteps)]
+ extra_time_keys = [x for x in read_data.keys() if x not in current_time_keys]
+ if len(extra_time_keys) > 0:
+ if is_gt:
+ text = 'Ground-truth'
+ else:
+ text = 'Tracking'
+ raise TrackEvalException(
+ text + ' data contains the following invalid timesteps in seq %s: ' % seq + ', '.join(
+ [str(x) + ', ' for x in extra_time_keys]))
+
+ for t in range(num_timesteps):
+ time_key = str(t)
+ if time_key in read_data.keys():
+ time_data = np.asarray(read_data[time_key], dtype=np.float)
+ raw_data['dets'][t] = np.atleast_2d(time_data[:, 6:10])
+ raw_data['ids'][t] = np.atleast_1d(time_data[:, 1]).astype(int)
+ raw_data['classes'][t] = np.atleast_1d(time_data[:, 2]).astype(int)
+ if is_gt:
+ gt_extras_dict = {'truncation': np.atleast_1d(time_data[:, 3].astype(int)),
+ 'occlusion': np.atleast_1d(time_data[:, 4].astype(int))}
+ raw_data['gt_extras'][t] = gt_extras_dict
+ else:
+ if time_data.shape[1] > 17:
+ raw_data['tracker_confidences'][t] = np.atleast_1d(time_data[:, 17])
+ else:
+ raw_data['tracker_confidences'][t] = np.ones(time_data.shape[0])
+ else:
+ raw_data['dets'][t] = np.empty((0, 4))
+ raw_data['ids'][t] = np.empty(0).astype(int)
+ raw_data['classes'][t] = np.empty(0).astype(int)
+ if is_gt:
+ gt_extras_dict = {'truncation': np.empty(0),
+ 'occlusion': np.empty(0)}
+ raw_data['gt_extras'][t] = gt_extras_dict
+ else:
+ raw_data['tracker_confidences'][t] = np.empty(0)
+ if is_gt:
+ if time_key in ignore_data.keys():
+ time_ignore = np.asarray(ignore_data[time_key], dtype=np.float)
+ raw_data['gt_crowd_ignore_regions'][t] = np.atleast_2d(time_ignore[:, 6:10])
+ else:
+ raw_data['gt_crowd_ignore_regions'][t] = np.empty((0, 4))
+
+ if is_gt:
+ key_map = {'ids': 'gt_ids',
+ 'classes': 'gt_classes',
+ 'dets': 'gt_dets'}
+ else:
+ key_map = {'ids': 'tracker_ids',
+ 'classes': 'tracker_classes',
+ 'dets': 'tracker_dets'}
+ for k, v in key_map.items():
+ raw_data[v] = raw_data.pop(k)
+ raw_data['num_timesteps'] = num_timesteps
+ raw_data['seq'] = seq
+ return raw_data
+
+ @_timing.time
+ def get_preprocessed_seq_data(self, raw_data, cls):
+ """ Preprocess data for a single sequence for a single class ready for evaluation.
+ Inputs:
+ - raw_data is a dict containing the data for the sequence already read in by get_raw_seq_data().
+ - cls is the class to be evaluated.
+ Outputs:
+ - data is a dict containing all of the information that metrics need to perform evaluation.
+ It contains the following fields:
+ [num_timesteps, num_gt_ids, num_tracker_ids, num_gt_dets, num_tracker_dets] : integers.
+ [gt_ids, tracker_ids, tracker_confidences]: list (for each timestep) of 1D NDArrays (for each det).
+ [gt_dets, tracker_dets]: list (for each timestep) of lists of detections.
+ [similarity_scores]: list (for each timestep) of 2D NDArrays.
+ Notes:
+ General preprocessing (preproc) occurs in 4 steps. Some datasets may not use all of these steps.
+ 1) Extract only detections relevant for the class to be evaluated (including distractor detections).
+ 2) Match gt dets and tracker dets. Remove tracker dets that are matched to a gt det that is of a
+ distractor class, or otherwise marked as to be removed.
+ 3) Remove unmatched tracker dets if they fall within a crowd ignore region or don't meet a certain
+ other criteria (e.g. are too small).
+ 4) Remove gt dets that were only useful for preprocessing and not for actual evaluation.
+ After the above preprocessing steps, this function also calculates the number of gt and tracker detections
+ and unique track ids. It also relabels gt and tracker ids to be contiguous and checks that ids are
+ unique within each timestep.
+
+ KITTI:
+ In KITTI, the 4 preproc steps are as follow:
+ 1) There are two classes (pedestrian and car) which are evaluated separately.
+ 2) For the pedestrian class, the 'person' class is distractor objects (people sitting).
+ For the car class, the 'van' class are distractor objects.
+ GT boxes marked as having occlusion level > 2 or truncation level > 0 are also treated as
+ distractors.
+ 3) Crowd ignore regions are used to remove unmatched detections. Also unmatched detections with
+ height <= 25 pixels are removed.
+ 4) Distractor gt dets (including truncated and occluded) are removed.
+ """
+ if cls == 'pedestrian':
+ distractor_classes = [self.class_name_to_class_id['person']]
+ elif cls == 'car':
+ distractor_classes = [self.class_name_to_class_id['van']]
+ else:
+ raise (TrackEvalException('Class %s is not evaluatable' % cls))
+ cls_id = self.class_name_to_class_id[cls]
+
+ data_keys = ['gt_ids', 'tracker_ids', 'gt_dets', 'tracker_dets', 'tracker_confidences', 'similarity_scores']
+ data = {key: [None] * raw_data['num_timesteps'] for key in data_keys}
+ unique_gt_ids = []
+ unique_tracker_ids = []
+ num_gt_dets = 0
+ num_tracker_dets = 0
+ for t in range(raw_data['num_timesteps']):
+
+ # Only extract relevant dets for this class for preproc and eval (cls + distractor classes)
+ gt_class_mask = np.sum([raw_data['gt_classes'][t] == c for c in [cls_id] + distractor_classes], axis=0)
+ gt_class_mask = gt_class_mask.astype(np.bool)
+ gt_ids = raw_data['gt_ids'][t][gt_class_mask]
+ gt_dets = raw_data['gt_dets'][t][gt_class_mask]
+ gt_classes = raw_data['gt_classes'][t][gt_class_mask]
+ gt_occlusion = raw_data['gt_extras'][t]['occlusion'][gt_class_mask]
+ gt_truncation = raw_data['gt_extras'][t]['truncation'][gt_class_mask]
+
+ tracker_class_mask = np.atleast_1d(raw_data['tracker_classes'][t] == cls_id)
+ tracker_class_mask = tracker_class_mask.astype(np.bool)
+ tracker_ids = raw_data['tracker_ids'][t][tracker_class_mask]
+ tracker_dets = raw_data['tracker_dets'][t][tracker_class_mask]
+ tracker_confidences = raw_data['tracker_confidences'][t][tracker_class_mask]
+ similarity_scores = raw_data['similarity_scores'][t][gt_class_mask, :][:, tracker_class_mask]
+
+ # Match tracker and gt dets (with hungarian algorithm) and remove tracker dets which match with gt dets
+ # which are labeled as truncated, occluded, or belonging to a distractor class.
+ to_remove_matched = np.array([], np.int)
+ unmatched_indices = np.arange(tracker_ids.shape[0])
+ if gt_ids.shape[0] > 0 and tracker_ids.shape[0] > 0:
+ matching_scores = similarity_scores.copy()
+ matching_scores[matching_scores < 0.5 - np.finfo('float').eps] = 0
+ match_rows, match_cols = linear_sum_assignment(-matching_scores)
+ actually_matched_mask = matching_scores[match_rows, match_cols] > 0 + np.finfo('float').eps
+ match_rows = match_rows[actually_matched_mask]
+ match_cols = match_cols[actually_matched_mask]
+
+ is_distractor_class = np.isin(gt_classes[match_rows], distractor_classes)
+ is_occluded_or_truncated = np.logical_or(
+ gt_occlusion[match_rows] > self.max_occlusion + np.finfo('float').eps,
+ gt_truncation[match_rows] > self.max_truncation + np.finfo('float').eps)
+ to_remove_matched = np.logical_or(is_distractor_class, is_occluded_or_truncated)
+ to_remove_matched = match_cols[to_remove_matched]
+ unmatched_indices = np.delete(unmatched_indices, match_cols, axis=0)
+
+ # For unmatched tracker dets, also remove those smaller than a minimum height.
+ unmatched_tracker_dets = tracker_dets[unmatched_indices, :]
+ unmatched_heights = unmatched_tracker_dets[:, 3] - unmatched_tracker_dets[:, 1]
+ is_too_small = unmatched_heights <= self.min_height + np.finfo('float').eps
+
+ # For unmatched tracker dets, also remove those that are greater than 50% within a crowd ignore region.
+ crowd_ignore_regions = raw_data['gt_crowd_ignore_regions'][t]
+ intersection_with_ignore_region = self._calculate_box_ious(unmatched_tracker_dets, crowd_ignore_regions,
+ box_format='x0y0x1y1', do_ioa=True)
+ is_within_crowd_ignore_region = np.any(intersection_with_ignore_region > 0.5 + np.finfo('float').eps, axis=1)
+
+ # Apply preprocessing to remove all unwanted tracker dets.
+ to_remove_unmatched = unmatched_indices[np.logical_or(is_too_small, is_within_crowd_ignore_region)]
+ to_remove_tracker = np.concatenate((to_remove_matched, to_remove_unmatched), axis=0)
+ data['tracker_ids'][t] = np.delete(tracker_ids, to_remove_tracker, axis=0)
+ data['tracker_dets'][t] = np.delete(tracker_dets, to_remove_tracker, axis=0)
+ data['tracker_confidences'][t] = np.delete(tracker_confidences, to_remove_tracker, axis=0)
+ similarity_scores = np.delete(similarity_scores, to_remove_tracker, axis=1)
+
+ # Also remove gt dets that were only useful for preprocessing and are not needed for evaluation.
+ # These are those that are occluded, truncated and from distractor objects.
+ gt_to_keep_mask = (np.less_equal(gt_occlusion, self.max_occlusion)) & \
+ (np.less_equal(gt_truncation, self.max_truncation)) & \
+ (np.equal(gt_classes, cls_id))
+ data['gt_ids'][t] = gt_ids[gt_to_keep_mask]
+ data['gt_dets'][t] = gt_dets[gt_to_keep_mask, :]
+ data['similarity_scores'][t] = similarity_scores[gt_to_keep_mask]
+
+ unique_gt_ids += list(np.unique(data['gt_ids'][t]))
+ unique_tracker_ids += list(np.unique(data['tracker_ids'][t]))
+ num_tracker_dets += len(data['tracker_ids'][t])
+ num_gt_dets += len(data['gt_ids'][t])
+
+ # Re-label IDs such that there are no empty IDs
+ if len(unique_gt_ids) > 0:
+ unique_gt_ids = np.unique(unique_gt_ids)
+ gt_id_map = np.nan * np.ones((np.max(unique_gt_ids) + 1))
+ gt_id_map[unique_gt_ids] = np.arange(len(unique_gt_ids))
+ for t in range(raw_data['num_timesteps']):
+ if len(data['gt_ids'][t]) > 0:
+ data['gt_ids'][t] = gt_id_map[data['gt_ids'][t]].astype(np.int)
+ if len(unique_tracker_ids) > 0:
+ unique_tracker_ids = np.unique(unique_tracker_ids)
+ tracker_id_map = np.nan * np.ones((np.max(unique_tracker_ids) + 1))
+ tracker_id_map[unique_tracker_ids] = np.arange(len(unique_tracker_ids))
+ for t in range(raw_data['num_timesteps']):
+ if len(data['tracker_ids'][t]) > 0:
+ data['tracker_ids'][t] = tracker_id_map[data['tracker_ids'][t]].astype(np.int)
+
+ # Record overview statistics.
+ data['num_tracker_dets'] = num_tracker_dets
+ data['num_gt_dets'] = num_gt_dets
+ data['num_tracker_ids'] = len(unique_tracker_ids)
+ data['num_gt_ids'] = len(unique_gt_ids)
+ data['num_timesteps'] = raw_data['num_timesteps']
+ data['seq'] = raw_data['seq']
+
+ # Ensure that ids are unique per timestep.
+ self._check_unique_ids(data)
+
+ return data
+
+ def _calculate_similarities(self, gt_dets_t, tracker_dets_t):
+ similarity_scores = self._calculate_box_ious(gt_dets_t, tracker_dets_t, box_format='x0y0x1y1')
+ return similarity_scores
diff --git a/val_utils/trackeval/datasets/kitti_mots.py b/val_utils/trackeval/datasets/kitti_mots.py
new file mode 100644
index 0000000000000000000000000000000000000000..9e04d3c2b128ce0dc80c5180a40895ef1bc8dc25
--- /dev/null
+++ b/val_utils/trackeval/datasets/kitti_mots.py
@@ -0,0 +1,426 @@
+import os
+import csv
+import numpy as np
+from scipy.optimize import linear_sum_assignment
+from ._base_dataset import _BaseDataset
+from .. import utils
+from .. import _timing
+from ..utils import TrackEvalException
+
+
+class KittiMOTS(_BaseDataset):
+ """Dataset class for KITTI MOTS tracking"""
+
+ @staticmethod
+ def get_default_dataset_config():
+ """Default class config values"""
+ code_path = utils.get_code_path()
+ default_config = {
+ 'GT_FOLDER': os.path.join(code_path, 'data/gt/kitti/kitti_mots_val'), # Location of GT data
+ 'TRACKERS_FOLDER': os.path.join(code_path, 'data/trackers/kitti/kitti_mots_val'), # Trackers location
+ 'OUTPUT_FOLDER': None, # Where to save eval results (if None, same as TRACKERS_FOLDER)
+ 'TRACKERS_TO_EVAL': None, # Filenames of trackers to eval (if None, all in folder)
+ 'CLASSES_TO_EVAL': ['car', 'pedestrian'], # Valid: ['car', 'pedestrian']
+ 'SPLIT_TO_EVAL': 'val', # Valid: 'training', 'val'
+ 'INPUT_AS_ZIP': False, # Whether tracker input files are zipped
+ 'PRINT_CONFIG': True, # Whether to print current config
+ 'TRACKER_SUB_FOLDER': 'data', # Tracker files are in TRACKER_FOLDER/tracker_name/TRACKER_SUB_FOLDER
+ 'OUTPUT_SUB_FOLDER': '', # Output files are saved in OUTPUT_FOLDER/tracker_name/OUTPUT_SUB_FOLDER
+ 'TRACKER_DISPLAY_NAMES': None, # Names of trackers to display, if None: TRACKERS_TO_EVAL
+ 'SEQMAP_FOLDER': None, # Where seqmaps are found (if None, GT_FOLDER)
+ 'SEQMAP_FILE': None, # Directly specify seqmap file (if none use seqmap_folder/split_to_eval.seqmap)
+ 'SEQ_INFO': None, # If not None, directly specify sequences to eval and their number of timesteps
+ 'GT_LOC_FORMAT': '{gt_folder}/label_02/{seq}.txt', # format of gt localization
+ }
+ return default_config
+
+ def __init__(self, config=None):
+ """Initialise dataset, checking that all required files are present"""
+ super().__init__()
+ # Fill non-given config values with defaults
+ self.config = utils.init_config(config, self.get_default_dataset_config(), self.get_name())
+ self.gt_fol = self.config['GT_FOLDER']
+ self.tracker_fol = self.config['TRACKERS_FOLDER']
+ self.split_to_eval = self.config['SPLIT_TO_EVAL']
+ self.should_classes_combine = False
+ self.use_super_categories = False
+ self.data_is_zipped = self.config['INPUT_AS_ZIP']
+
+ self.output_fol = self.config['OUTPUT_FOLDER']
+ if self.output_fol is None:
+ self.output_fol = self.tracker_fol
+
+ self.tracker_sub_fol = self.config['TRACKER_SUB_FOLDER']
+ self.output_sub_fol = self.config['OUTPUT_SUB_FOLDER']
+
+ # Get classes to eval
+ self.valid_classes = ['car', 'pedestrian']
+ self.class_list = [cls.lower() if cls.lower() in self.valid_classes else None
+ for cls in self.config['CLASSES_TO_EVAL']]
+ if not all(self.class_list):
+ raise TrackEvalException('Attempted to evaluate an invalid class. '
+ 'Only classes [car, pedestrian] are valid.')
+ self.class_name_to_class_id = {'car': '1', 'pedestrian': '2', 'ignore': '10'}
+
+ # Get sequences to eval and check gt files exist
+ self.seq_list, self.seq_lengths = self._get_seq_info()
+ if len(self.seq_list) < 1:
+ raise TrackEvalException('No sequences are selected to be evaluated.')
+
+ # Check gt files exist
+ for seq in self.seq_list:
+ if not self.data_is_zipped:
+ curr_file = self.config["GT_LOC_FORMAT"].format(gt_folder=self.gt_fol, seq=seq)
+ if not os.path.isfile(curr_file):
+ print('GT file not found ' + curr_file)
+ raise TrackEvalException('GT file not found for sequence: ' + seq)
+ if self.data_is_zipped:
+ curr_file = os.path.join(self.gt_fol, 'data.zip')
+ if not os.path.isfile(curr_file):
+ raise TrackEvalException('GT file not found: ' + os.path.basename(curr_file))
+
+ # Get trackers to eval
+ if self.config['TRACKERS_TO_EVAL'] is None:
+ self.tracker_list = os.listdir(self.tracker_fol)
+ else:
+ self.tracker_list = self.config['TRACKERS_TO_EVAL']
+
+ if self.config['TRACKER_DISPLAY_NAMES'] is None:
+ self.tracker_to_disp = dict(zip(self.tracker_list, self.tracker_list))
+ elif (self.config['TRACKERS_TO_EVAL'] is not None) and (
+ len(self.config['TRACKER_DISPLAY_NAMES']) == len(self.tracker_list)):
+ self.tracker_to_disp = dict(zip(self.tracker_list, self.config['TRACKER_DISPLAY_NAMES']))
+ else:
+ raise TrackEvalException('List of tracker files and tracker display names do not match.')
+
+ for tracker in self.tracker_list:
+ if self.data_is_zipped:
+ curr_file = os.path.join(self.tracker_fol, tracker, self.tracker_sub_fol + '.zip')
+ if not os.path.isfile(curr_file):
+ print('Tracker file not found: ' + curr_file)
+ raise TrackEvalException('Tracker file not found: ' + tracker + '/' + os.path.basename(curr_file))
+ else:
+ for seq in self.seq_list:
+ curr_file = os.path.join(self.tracker_fol, tracker, self.tracker_sub_fol, seq + '.txt')
+ if not os.path.isfile(curr_file):
+ print('Tracker file not found: ' + curr_file)
+ raise TrackEvalException(
+ 'Tracker file not found: ' + tracker + '/' + self.tracker_sub_fol + '/' + os.path.basename(
+ curr_file))
+
+ def get_display_name(self, tracker):
+ return self.tracker_to_disp[tracker]
+
+ def _get_seq_info(self):
+ seq_list = []
+ seq_lengths = {}
+ seqmap_name = 'evaluate_mots.seqmap.' + self.config['SPLIT_TO_EVAL']
+
+ if self.config["SEQ_INFO"]:
+ seq_list = list(self.config["SEQ_INFO"].keys())
+ seq_lengths = self.config["SEQ_INFO"]
+ else:
+ if self.config["SEQMAP_FILE"]:
+ seqmap_file = self.config["SEQMAP_FILE"]
+ else:
+ if self.config["SEQMAP_FOLDER"] is None:
+ seqmap_file = os.path.join(self.config['GT_FOLDER'], seqmap_name)
+ else:
+ seqmap_file = os.path.join(self.config["SEQMAP_FOLDER"], seqmap_name)
+ if not os.path.isfile(seqmap_file):
+ print('no seqmap found: ' + seqmap_file)
+ raise TrackEvalException('no seqmap found: ' + os.path.basename(seqmap_file))
+ with open(seqmap_file) as fp:
+ reader = csv.reader(fp)
+ for i, _ in enumerate(reader):
+ dialect = csv.Sniffer().sniff(fp.read(1024))
+ fp.seek(0)
+ reader = csv.reader(fp, dialect)
+ for row in reader:
+ if len(row) >= 4:
+ seq = "%04d" % int(row[0])
+ seq_list.append(seq)
+ seq_lengths[seq] = int(row[3]) + 1
+ return seq_list, seq_lengths
+
+ def _load_raw_file(self, tracker, seq, is_gt):
+ """Load a file (gt or tracker) in the KITTI MOTS format
+
+ If is_gt, this returns a dict which contains the fields:
+ [gt_ids, gt_classes] : list (for each timestep) of 1D NDArrays (for each det).
+ [gt_dets]: list (for each timestep) of lists of detections.
+ [gt_ignore_region]: list (for each timestep) of masks for the ignore regions
+
+ if not is_gt, this returns a dict which contains the fields:
+ [tracker_ids, tracker_classes] : list (for each timestep) of 1D NDArrays (for each det).
+ [tracker_dets]: list (for each timestep) of lists of detections.
+ """
+
+ # Only loaded when run to reduce minimum requirements
+ from pycocotools import mask as mask_utils
+
+ # File location
+ if self.data_is_zipped:
+ if is_gt:
+ zip_file = os.path.join(self.gt_fol, 'data.zip')
+ else:
+ zip_file = os.path.join(self.tracker_fol, tracker, self.tracker_sub_fol + '.zip')
+ file = seq + '.txt'
+ else:
+ zip_file = None
+ if is_gt:
+ file = self.config["GT_LOC_FORMAT"].format(gt_folder=self.gt_fol, seq=seq)
+ else:
+ file = os.path.join(self.tracker_fol, tracker, self.tracker_sub_fol, seq + '.txt')
+
+ # Ignore regions
+ if is_gt:
+ crowd_ignore_filter = {2: ['10']}
+ else:
+ crowd_ignore_filter = None
+
+ # Load raw data from text file
+ read_data, ignore_data = self._load_simple_text_file(file, crowd_ignore_filter=crowd_ignore_filter,
+ is_zipped=self.data_is_zipped, zip_file=zip_file,
+ force_delimiters=' ')
+
+ # Convert data to required format
+ num_timesteps = self.seq_lengths[seq]
+ data_keys = ['ids', 'classes', 'dets']
+ if is_gt:
+ data_keys += ['gt_ignore_region']
+ raw_data = {key: [None] * num_timesteps for key in data_keys}
+
+ # Check for any extra time keys
+ current_time_keys = [str(t) for t in range(num_timesteps)]
+ extra_time_keys = [x for x in read_data.keys() if x not in current_time_keys]
+ if len(extra_time_keys) > 0:
+ if is_gt:
+ text = 'Ground-truth'
+ else:
+ text = 'Tracking'
+ raise TrackEvalException(
+ text + ' data contains the following invalid timesteps in seq %s: ' % seq + ', '.join(
+ [str(x) + ', ' for x in extra_time_keys]))
+
+ for t in range(num_timesteps):
+ time_key = str(t)
+ # list to collect all masks of a timestep to check for overlapping areas
+ all_masks = []
+ if time_key in read_data.keys():
+ try:
+ raw_data['dets'][t] = [{'size': [int(region[3]), int(region[4])],
+ 'counts': region[5].encode(encoding='UTF-8')}
+ for region in read_data[time_key]]
+ raw_data['ids'][t] = np.atleast_1d([region[1] for region in read_data[time_key]]).astype(int)
+ raw_data['classes'][t] = np.atleast_1d([region[2] for region in read_data[time_key]]).astype(int)
+ all_masks += raw_data['dets'][t]
+ except IndexError:
+ self._raise_index_error(is_gt, tracker, seq)
+ except ValueError:
+ self._raise_value_error(is_gt, tracker, seq)
+ else:
+ raw_data['dets'][t] = []
+ raw_data['ids'][t] = np.empty(0).astype(int)
+ raw_data['classes'][t] = np.empty(0).astype(int)
+ if is_gt:
+ if time_key in ignore_data.keys():
+ try:
+ time_ignore = [{'size': [int(region[3]), int(region[4])],
+ 'counts': region[5].encode(encoding='UTF-8')}
+ for region in ignore_data[time_key]]
+ raw_data['gt_ignore_region'][t] = mask_utils.merge([mask for mask in time_ignore],
+ intersect=False)
+ all_masks += [raw_data['gt_ignore_region'][t]]
+ except IndexError:
+ self._raise_index_error(is_gt, tracker, seq)
+ except ValueError:
+ self._raise_value_error(is_gt, tracker, seq)
+ else:
+ raw_data['gt_ignore_region'][t] = mask_utils.merge([], intersect=False)
+
+ # check for overlapping masks
+ if all_masks:
+ masks_merged = all_masks[0]
+ for mask in all_masks[1:]:
+ if mask_utils.area(mask_utils.merge([masks_merged, mask], intersect=True)) != 0.0:
+ raise TrackEvalException(
+ 'Tracker has overlapping masks. Tracker: ' + tracker + ' Seq: ' + seq + ' Timestep: ' + str(
+ t))
+ masks_merged = mask_utils.merge([masks_merged, mask], intersect=False)
+
+ if is_gt:
+ key_map = {'ids': 'gt_ids',
+ 'classes': 'gt_classes',
+ 'dets': 'gt_dets'}
+ else:
+ key_map = {'ids': 'tracker_ids',
+ 'classes': 'tracker_classes',
+ 'dets': 'tracker_dets'}
+ for k, v in key_map.items():
+ raw_data[v] = raw_data.pop(k)
+ raw_data["num_timesteps"] = num_timesteps
+ raw_data['seq'] = seq
+ return raw_data
+
+ @_timing.time
+ def get_preprocessed_seq_data(self, raw_data, cls):
+ """ Preprocess data for a single sequence for a single class ready for evaluation.
+ Inputs:
+ - raw_data is a dict containing the data for the sequence already read in by get_raw_seq_data().
+ - cls is the class to be evaluated.
+ Outputs:
+ - data is a dict containing all of the information that metrics need to perform evaluation.
+ It contains the following fields:
+ [num_timesteps, num_gt_ids, num_tracker_ids, num_gt_dets, num_tracker_dets] : integers.
+ [gt_ids, tracker_ids]: list (for each timestep) of 1D NDArrays (for each det).
+ [gt_dets, tracker_dets]: list (for each timestep) of lists of detection masks.
+ [similarity_scores]: list (for each timestep) of 2D NDArrays.
+ Notes:
+ General preprocessing (preproc) occurs in 4 steps. Some datasets may not use all of these steps.
+ 1) Extract only detections relevant for the class to be evaluated (including distractor detections).
+ 2) Match gt dets and tracker dets. Remove tracker dets that are matched to a gt det that is of a
+ distractor class, or otherwise marked as to be removed.
+ 3) Remove unmatched tracker dets if they fall within a crowd ignore region or don't meet a certain
+ other criteria (e.g. are too small).
+ 4) Remove gt dets that were only useful for preprocessing and not for actual evaluation.
+ After the above preprocessing steps, this function also calculates the number of gt and tracker detections
+ and unique track ids. It also relabels gt and tracker ids to be contiguous and checks that ids are
+ unique within each timestep.
+
+ KITTI MOTS:
+ In KITTI MOTS, the 4 preproc steps are as follow:
+ 1) There are two classes (car and pedestrian) which are evaluated separately.
+ 2) There are no ground truth detections marked as to be removed/distractor classes.
+ Therefore also no matched tracker detections are removed.
+ 3) Ignore regions are used to remove unmatched detections (at least 50% overlap with ignore region).
+ 4) There are no ground truth detections (e.g. those of distractor classes) to be removed.
+ """
+ # Check that input data has unique ids
+ self._check_unique_ids(raw_data)
+
+ cls_id = int(self.class_name_to_class_id[cls])
+
+ data_keys = ['gt_ids', 'tracker_ids', 'gt_dets', 'tracker_dets', 'similarity_scores']
+ data = {key: [None] * raw_data['num_timesteps'] for key in data_keys}
+ unique_gt_ids = []
+ unique_tracker_ids = []
+ num_gt_dets = 0
+ num_tracker_dets = 0
+ for t in range(raw_data['num_timesteps']):
+
+ # Only extract relevant dets for this class for preproc and eval (cls)
+ gt_class_mask = np.atleast_1d(raw_data['gt_classes'][t] == cls_id)
+ gt_class_mask = gt_class_mask.astype(np.bool)
+ gt_ids = raw_data['gt_ids'][t][gt_class_mask]
+ gt_dets = [raw_data['gt_dets'][t][ind] for ind in range(len(gt_class_mask)) if gt_class_mask[ind]]
+
+ tracker_class_mask = np.atleast_1d(raw_data['tracker_classes'][t] == cls_id)
+ tracker_class_mask = tracker_class_mask.astype(np.bool)
+ tracker_ids = raw_data['tracker_ids'][t][tracker_class_mask]
+ tracker_dets = [raw_data['tracker_dets'][t][ind] for ind in range(len(tracker_class_mask)) if
+ tracker_class_mask[ind]]
+ similarity_scores = raw_data['similarity_scores'][t][gt_class_mask, :][:, tracker_class_mask]
+
+ # Match tracker and gt dets (with hungarian algorithm)
+ unmatched_indices = np.arange(tracker_ids.shape[0])
+ if gt_ids.shape[0] > 0 and tracker_ids.shape[0] > 0:
+ matching_scores = similarity_scores.copy()
+ matching_scores[matching_scores < 0.5 - np.finfo('float').eps] = -10000
+ match_rows, match_cols = linear_sum_assignment(-matching_scores)
+ actually_matched_mask = matching_scores[match_rows, match_cols] > 0 + np.finfo('float').eps
+ match_cols = match_cols[actually_matched_mask]
+
+ unmatched_indices = np.delete(unmatched_indices, match_cols, axis=0)
+
+ # For unmatched tracker dets, remove those that are greater than 50% within a crowd ignore region.
+ unmatched_tracker_dets = [tracker_dets[i] for i in range(len(tracker_dets)) if i in unmatched_indices]
+ ignore_region = raw_data['gt_ignore_region'][t]
+ intersection_with_ignore_region = self._calculate_mask_ious(unmatched_tracker_dets, [ignore_region],
+ is_encoded=True, do_ioa=True)
+ is_within_ignore_region = np.any(intersection_with_ignore_region > 0.5 + np.finfo('float').eps, axis=1)
+
+ # Apply preprocessing to remove unwanted tracker dets.
+ to_remove_tracker = unmatched_indices[is_within_ignore_region]
+ data['tracker_ids'][t] = np.delete(tracker_ids, to_remove_tracker, axis=0)
+ data['tracker_dets'][t] = np.delete(tracker_dets, to_remove_tracker, axis=0)
+ similarity_scores = np.delete(similarity_scores, to_remove_tracker, axis=1)
+
+ # Keep all ground truth detections
+ data['gt_ids'][t] = gt_ids
+ data['gt_dets'][t] = gt_dets
+ data['similarity_scores'][t] = similarity_scores
+
+ unique_gt_ids += list(np.unique(data['gt_ids'][t]))
+ unique_tracker_ids += list(np.unique(data['tracker_ids'][t]))
+ num_tracker_dets += len(data['tracker_ids'][t])
+ num_gt_dets += len(data['gt_ids'][t])
+
+ # Re-label IDs such that there are no empty IDs
+ if len(unique_gt_ids) > 0:
+ unique_gt_ids = np.unique(unique_gt_ids)
+ gt_id_map = np.nan * np.ones((np.max(unique_gt_ids) + 1))
+ gt_id_map[unique_gt_ids] = np.arange(len(unique_gt_ids))
+ for t in range(raw_data['num_timesteps']):
+ if len(data['gt_ids'][t]) > 0:
+ data['gt_ids'][t] = gt_id_map[data['gt_ids'][t]].astype(np.int)
+ if len(unique_tracker_ids) > 0:
+ unique_tracker_ids = np.unique(unique_tracker_ids)
+ tracker_id_map = np.nan * np.ones((np.max(unique_tracker_ids) + 1))
+ tracker_id_map[unique_tracker_ids] = np.arange(len(unique_tracker_ids))
+ for t in range(raw_data['num_timesteps']):
+ if len(data['tracker_ids'][t]) > 0:
+ data['tracker_ids'][t] = tracker_id_map[data['tracker_ids'][t]].astype(np.int)
+
+ # Record overview statistics.
+ data['num_tracker_dets'] = num_tracker_dets
+ data['num_gt_dets'] = num_gt_dets
+ data['num_tracker_ids'] = len(unique_tracker_ids)
+ data['num_gt_ids'] = len(unique_gt_ids)
+ data['num_timesteps'] = raw_data['num_timesteps']
+ data['seq'] = raw_data['seq']
+ data['cls'] = cls
+
+ # Ensure again that ids are unique per timestep after preproc.
+ self._check_unique_ids(data, after_preproc=True)
+
+ return data
+
+ def _calculate_similarities(self, gt_dets_t, tracker_dets_t):
+ similarity_scores = self._calculate_mask_ious(gt_dets_t, tracker_dets_t, is_encoded=True, do_ioa=False)
+ return similarity_scores
+
+ @staticmethod
+ def _raise_index_error(is_gt, tracker, seq):
+ """
+ Auxiliary method to raise an evaluation error in case of an index error while reading files.
+ :param is_gt: whether gt or tracker data is read
+ :param tracker: the name of the tracker
+ :param seq: the name of the seq
+ :return: None
+ """
+ if is_gt:
+ err = 'Cannot load gt data from sequence %s, because there are not enough ' \
+ 'columns in the data.' % seq
+ raise TrackEvalException(err)
+ else:
+ err = 'Cannot load tracker data from tracker %s, sequence %s, because there are not enough ' \
+ 'columns in the data.' % (tracker, seq)
+ raise TrackEvalException(err)
+
+ @staticmethod
+ def _raise_value_error(is_gt, tracker, seq):
+ """
+ Auxiliary method to raise an evaluation error in case of an value error while reading files.
+ :param is_gt: whether gt or tracker data is read
+ :param tracker: the name of the tracker
+ :param seq: the name of the seq
+ :return: None
+ """
+ if is_gt:
+ raise TrackEvalException(
+ 'GT data for sequence %s cannot be converted to the right format. Is data corrupted?' % seq)
+ else:
+ raise TrackEvalException(
+ 'Tracking data from tracker %s, sequence %s cannot be converted to the right format. '
+ 'Is data corrupted?' % (tracker, seq))
diff --git a/val_utils/trackeval/datasets/mot_challenge_2d_box.py b/val_utils/trackeval/datasets/mot_challenge_2d_box.py
new file mode 100644
index 0000000000000000000000000000000000000000..68aac5127374cf8d0a6f62392e7c490e6c252b79
--- /dev/null
+++ b/val_utils/trackeval/datasets/mot_challenge_2d_box.py
@@ -0,0 +1,437 @@
+import os
+import csv
+import configparser
+import numpy as np
+from scipy.optimize import linear_sum_assignment
+from ._base_dataset import _BaseDataset
+from .. import utils
+from .. import _timing
+from ..utils import TrackEvalException
+
+
+class MotChallenge2DBox(_BaseDataset):
+ """Dataset class for MOT Challenge 2D bounding box tracking"""
+
+ @staticmethod
+ def get_default_dataset_config():
+ """Default class config values"""
+ code_path = utils.get_code_path()
+ default_config = {
+ 'GT_FOLDER': os.path.join(code_path, 'data/gt/mot_challenge/'), # Location of GT data
+ 'TRACKERS_FOLDER': os.path.join(code_path, 'data/trackers/mot_challenge/'), # Trackers location
+ 'OUTPUT_FOLDER': None, # Where to save eval results (if None, same as TRACKERS_FOLDER)
+ 'TRACKERS_TO_EVAL': None, # Filenames of trackers to eval (if None, all in folder)
+ 'CLASSES_TO_EVAL': ['pedestrian'], # Valid: ['pedestrian']
+ 'BENCHMARK': 'MOT17', # Valid: 'MOT17', 'MOT16', 'MOT20', 'MOT15'
+ 'SPLIT_TO_EVAL': 'train', # Valid: 'train', 'test', 'all'
+ 'INPUT_AS_ZIP': False, # Whether tracker input files are zipped
+ 'PRINT_CONFIG': True, # Whether to print current config
+ 'DO_PREPROC': True, # Whether to perform preprocessing (never done for MOT15)
+ 'TRACKER_SUB_FOLDER': 'data', # Tracker files are in TRACKER_FOLDER/tracker_name/TRACKER_SUB_FOLDER
+ 'OUTPUT_SUB_FOLDER': '', # Output files are saved in OUTPUT_FOLDER/tracker_name/OUTPUT_SUB_FOLDER
+ 'TRACKER_DISPLAY_NAMES': None, # Names of trackers to display, if None: TRACKERS_TO_EVAL
+ 'SEQMAP_FOLDER': None, # Where seqmaps are found (if None, GT_FOLDER/seqmaps)
+ 'SEQMAP_FILE': None, # Directly specify seqmap file (if none use seqmap_folder/benchmark-split_to_eval)
+ 'SEQ_INFO': None, # If not None, directly specify sequences to eval and their number of timesteps
+ 'GT_LOC_FORMAT': '{gt_folder}/{seq}/gt/gt.txt', # '{gt_folder}/{seq}/gt/gt.txt'
+ 'SKIP_SPLIT_FOL': False, # If False, data is in GT_FOLDER/BENCHMARK-SPLIT_TO_EVAL/ and in
+ # TRACKERS_FOLDER/BENCHMARK-SPLIT_TO_EVAL/tracker/
+ # If True, then the middle 'benchmark-split' folder is skipped for both.
+ }
+ return default_config
+
+ def __init__(self, config=None):
+ """Initialise dataset, checking that all required files are present"""
+ super().__init__()
+ # Fill non-given config values with defaults
+ self.config = utils.init_config(config, self.get_default_dataset_config(), self.get_name())
+
+ self.benchmark = self.config['BENCHMARK']
+ gt_set = self.config['BENCHMARK'] + '-' + self.config['SPLIT_TO_EVAL']
+ self.gt_set = gt_set
+ if not self.config['SKIP_SPLIT_FOL']:
+ split_fol = gt_set
+ else:
+ split_fol = ''
+ self.gt_fol = os.path.join(self.config['GT_FOLDER'], split_fol)
+ self.tracker_fol = os.path.join(self.config['TRACKERS_FOLDER'], split_fol)
+ self.should_classes_combine = False
+ self.use_super_categories = False
+ self.data_is_zipped = self.config['INPUT_AS_ZIP']
+ self.do_preproc = self.config['DO_PREPROC']
+
+ self.output_fol = self.config['OUTPUT_FOLDER']
+ if self.output_fol is None:
+ self.output_fol = self.tracker_fol
+
+ self.tracker_sub_fol = self.config['TRACKER_SUB_FOLDER']
+ self.output_sub_fol = self.config['OUTPUT_SUB_FOLDER']
+
+ # Get classes to eval
+ self.valid_classes = ['pedestrian']
+ self.class_list = [cls.lower() if cls.lower() in self.valid_classes else None
+ for cls in self.config['CLASSES_TO_EVAL']]
+ if not all(self.class_list):
+ raise TrackEvalException('Attempted to evaluate an invalid class. Only pedestrian class is valid.')
+ self.class_name_to_class_id = {'pedestrian': 1, 'person_on_vehicle': 2, 'car': 3, 'bicycle': 4, 'motorbike': 5,
+ 'non_mot_vehicle': 6, 'static_person': 7, 'distractor': 8, 'occluder': 9,
+ 'occluder_on_ground': 10, 'occluder_full': 11, 'reflection': 12, 'crowd': 13}
+ self.valid_class_numbers = list(self.class_name_to_class_id.values())
+
+ # Get sequences to eval and check gt files exist
+ self.seq_list, self.seq_lengths = self._get_seq_info()
+ if len(self.seq_list) < 1:
+ raise TrackEvalException('No sequences are selected to be evaluated.')
+
+ # Check gt files exist
+ for seq in self.seq_list:
+ if not self.data_is_zipped:
+ curr_file = self.config["GT_LOC_FORMAT"].format(gt_folder=self.gt_fol, seq=seq)
+ if not os.path.isfile(curr_file):
+ print('GT file not found ' + curr_file)
+ raise TrackEvalException('GT file not found for sequence: ' + seq)
+ if self.data_is_zipped:
+ curr_file = os.path.join(self.gt_fol, 'data.zip')
+ if not os.path.isfile(curr_file):
+ print('GT file not found ' + curr_file)
+ raise TrackEvalException('GT file not found: ' + os.path.basename(curr_file))
+
+ # Get trackers to eval
+ if self.config['TRACKERS_TO_EVAL'] is None:
+ self.tracker_list = os.listdir(self.tracker_fol)
+ else:
+ self.tracker_list = self.config['TRACKERS_TO_EVAL']
+
+ if self.config['TRACKER_DISPLAY_NAMES'] is None:
+ self.tracker_to_disp = dict(zip(self.tracker_list, self.tracker_list))
+ elif (self.config['TRACKERS_TO_EVAL'] is not None) and (
+ len(self.config['TRACKER_DISPLAY_NAMES']) == len(self.tracker_list)):
+ self.tracker_to_disp = dict(zip(self.tracker_list, self.config['TRACKER_DISPLAY_NAMES']))
+ else:
+ raise TrackEvalException('List of tracker files and tracker display names do not match.')
+
+ for tracker in self.tracker_list:
+ if self.data_is_zipped:
+ curr_file = os.path.join(self.tracker_fol, tracker, self.tracker_sub_fol + '.zip')
+ if not os.path.isfile(curr_file):
+ print('Tracker file not found: ' + curr_file)
+ raise TrackEvalException('Tracker file not found: ' + tracker + '/' + os.path.basename(curr_file))
+ else:
+ for seq in self.seq_list:
+ curr_file = os.path.join(self.tracker_fol, tracker, self.tracker_sub_fol, seq + '.txt')
+ if not os.path.isfile(curr_file):
+ print('Tracker file not found: ' + curr_file)
+ raise TrackEvalException(
+ 'Tracker file not found: ' + tracker + '/' + self.tracker_sub_fol + '/' + os.path.basename(
+ curr_file))
+
+ def get_display_name(self, tracker):
+ return self.tracker_to_disp[tracker]
+
+ def _get_seq_info(self):
+ seq_list = []
+ seq_lengths = {}
+ if self.config["SEQ_INFO"]:
+ seq_list = list(self.config["SEQ_INFO"].keys())
+ seq_lengths = self.config["SEQ_INFO"]
+
+ # If sequence length is 'None' tries to read sequence length from .ini files.
+ for seq, seq_length in seq_lengths.items():
+ if seq_length is None:
+ ini_file = os.path.join(self.gt_fol, seq, 'seqinfo.ini')
+ if not os.path.isfile(ini_file):
+ raise TrackEvalException('ini file does not exist: ' + seq + '/' + os.path.basename(ini_file))
+ ini_data = configparser.ConfigParser()
+ ini_data.read(ini_file)
+ seq_lengths[seq] = int(ini_data['Sequence']['seqLength'])
+
+ else:
+ if self.config["SEQMAP_FILE"]:
+ seqmap_file = self.config["SEQMAP_FILE"]
+ else:
+ if self.config["SEQMAP_FOLDER"] is None:
+ seqmap_file = os.path.join(self.config['GT_FOLDER'], 'seqmaps', self.gt_set + '.txt')
+ else:
+ seqmap_file = os.path.join(self.config["SEQMAP_FOLDER"], self.gt_set + '.txt')
+ if not os.path.isfile(seqmap_file):
+ print('no seqmap found: ' + seqmap_file)
+ raise TrackEvalException('no seqmap found: ' + os.path.basename(seqmap_file))
+ with open(seqmap_file) as fp:
+ reader = csv.reader(fp)
+ for i, row in enumerate(reader):
+ if i == 0 or row[0] == '':
+ continue
+ seq = row[0]
+ seq_list.append(seq)
+ ini_file = os.path.join(self.gt_fol, seq, 'seqinfo.ini')
+ if not os.path.isfile(ini_file):
+ raise TrackEvalException('ini file does not exist: ' + seq + '/' + os.path.basename(ini_file))
+ ini_data = configparser.ConfigParser()
+ ini_data.read(ini_file)
+ seq_lengths[seq] = int(ini_data['Sequence']['seqLength'])
+ return seq_list, seq_lengths
+
+ def _load_raw_file(self, tracker, seq, is_gt):
+ """Load a file (gt or tracker) in the MOT Challenge 2D box format
+
+ If is_gt, this returns a dict which contains the fields:
+ [gt_ids, gt_classes] : list (for each timestep) of 1D NDArrays (for each det).
+ [gt_dets, gt_crowd_ignore_regions]: list (for each timestep) of lists of detections.
+ [gt_extras] : list (for each timestep) of dicts (for each extra) of 1D NDArrays (for each det).
+
+ if not is_gt, this returns a dict which contains the fields:
+ [tracker_ids, tracker_classes, tracker_confidences] : list (for each timestep) of 1D NDArrays (for each det).
+ [tracker_dets]: list (for each timestep) of lists of detections.
+ """
+ # File location
+ if self.data_is_zipped:
+ if is_gt:
+ zip_file = os.path.join(self.gt_fol, 'data.zip')
+ else:
+ zip_file = os.path.join(self.tracker_fol, tracker, self.tracker_sub_fol + '.zip')
+ file = seq + '.txt'
+ else:
+ zip_file = None
+ if is_gt:
+ file = self.config["GT_LOC_FORMAT"].format(gt_folder=self.gt_fol, seq=seq)
+ else:
+ file = os.path.join(self.tracker_fol, tracker, self.tracker_sub_fol, seq + '.txt')
+
+ # Load raw data from text file
+ read_data, ignore_data = self._load_simple_text_file(file, is_zipped=self.data_is_zipped, zip_file=zip_file)
+
+ # Convert data to required format
+ num_timesteps = self.seq_lengths[seq]
+ data_keys = ['ids', 'classes', 'dets']
+ if is_gt:
+ data_keys += ['gt_crowd_ignore_regions', 'gt_extras']
+ else:
+ data_keys += ['tracker_confidences']
+ raw_data = {key: [None] * num_timesteps for key in data_keys}
+
+ # Check for any extra time keys
+ current_time_keys = [str( t+ 1) for t in range(num_timesteps)]
+ extra_time_keys = [x for x in read_data.keys() if x not in current_time_keys]
+ if len(extra_time_keys) > 0:
+ if is_gt:
+ text = 'Ground-truth'
+ else:
+ text = 'Tracking'
+ raise TrackEvalException(
+ text + ' data contains the following invalid timesteps in seq %s: ' % seq + ', '.join(
+ [str(x) + ', ' for x in extra_time_keys]))
+
+ for t in range(num_timesteps):
+ time_key = str(t+1)
+ if time_key in read_data.keys():
+ try:
+ time_data = np.asarray(read_data[time_key], dtype=np.float)
+ except ValueError:
+ if is_gt:
+ raise TrackEvalException(
+ 'Cannot convert gt data for sequence %s to float. Is data corrupted?' % seq)
+ else:
+ raise TrackEvalException(
+ 'Cannot convert tracking data from tracker %s, sequence %s to float. Is data corrupted?' % (
+ tracker, seq))
+ try:
+ raw_data['dets'][t] = np.atleast_2d(time_data[:, 2:6])
+ raw_data['ids'][t] = np.atleast_1d(time_data[:, 1]).astype(int)
+ except IndexError:
+ if is_gt:
+ err = 'Cannot load gt data from sequence %s, because there is not enough ' \
+ 'columns in the data.' % seq
+ raise TrackEvalException(err)
+ else:
+ err = 'Cannot load tracker data from tracker %s, sequence %s, because there is not enough ' \
+ 'columns in the data.' % (tracker, seq)
+ raise TrackEvalException(err)
+ if time_data.shape[1] >= 8:
+ raw_data['classes'][t] = np.atleast_1d(time_data[:, 7]).astype(int)
+ else:
+ if not is_gt:
+ raw_data['classes'][t] = np.ones_like(raw_data['ids'][t])
+ else:
+ raise TrackEvalException(
+ 'GT data is not in a valid format, there is not enough rows in seq %s, timestep %i.' % (
+ seq, t))
+ if is_gt:
+ gt_extras_dict = {'zero_marked': np.atleast_1d(time_data[:, 6].astype(int))}
+ raw_data['gt_extras'][t] = gt_extras_dict
+ else:
+ raw_data['tracker_confidences'][t] = np.atleast_1d(time_data[:, 6])
+ else:
+ raw_data['dets'][t] = np.empty((0, 4))
+ raw_data['ids'][t] = np.empty(0).astype(int)
+ raw_data['classes'][t] = np.empty(0).astype(int)
+ if is_gt:
+ gt_extras_dict = {'zero_marked': np.empty(0)}
+ raw_data['gt_extras'][t] = gt_extras_dict
+ else:
+ raw_data['tracker_confidences'][t] = np.empty(0)
+ if is_gt:
+ raw_data['gt_crowd_ignore_regions'][t] = np.empty((0, 4))
+
+ if is_gt:
+ key_map = {'ids': 'gt_ids',
+ 'classes': 'gt_classes',
+ 'dets': 'gt_dets'}
+ else:
+ key_map = {'ids': 'tracker_ids',
+ 'classes': 'tracker_classes',
+ 'dets': 'tracker_dets'}
+ for k, v in key_map.items():
+ raw_data[v] = raw_data.pop(k)
+ raw_data['num_timesteps'] = num_timesteps
+ raw_data['seq'] = seq
+ return raw_data
+
+ @_timing.time
+ def get_preprocessed_seq_data(self, raw_data, cls):
+ """ Preprocess data for a single sequence for a single class ready for evaluation.
+ Inputs:
+ - raw_data is a dict containing the data for the sequence already read in by get_raw_seq_data().
+ - cls is the class to be evaluated.
+ Outputs:
+ - data is a dict containing all of the information that metrics need to perform evaluation.
+ It contains the following fields:
+ [num_timesteps, num_gt_ids, num_tracker_ids, num_gt_dets, num_tracker_dets] : integers.
+ [gt_ids, tracker_ids, tracker_confidences]: list (for each timestep) of 1D NDArrays (for each det).
+ [gt_dets, tracker_dets]: list (for each timestep) of lists of detections.
+ [similarity_scores]: list (for each timestep) of 2D NDArrays.
+ Notes:
+ General preprocessing (preproc) occurs in 4 steps. Some datasets may not use all of these steps.
+ 1) Extract only detections relevant for the class to be evaluated (including distractor detections).
+ 2) Match gt dets and tracker dets. Remove tracker dets that are matched to a gt det that is of a
+ distractor class, or otherwise marked as to be removed.
+ 3) Remove unmatched tracker dets if they fall within a crowd ignore region or don't meet a certain
+ other criteria (e.g. are too small).
+ 4) Remove gt dets that were only useful for preprocessing and not for actual evaluation.
+ After the above preprocessing steps, this function also calculates the number of gt and tracker detections
+ and unique track ids. It also relabels gt and tracker ids to be contiguous and checks that ids are
+ unique within each timestep.
+
+ MOT Challenge:
+ In MOT Challenge, the 4 preproc steps are as follow:
+ 1) There is only one class (pedestrian) to be evaluated, but all other classes are used for preproc.
+ 2) Predictions are matched against all gt boxes (regardless of class), those matching with distractor
+ objects are removed.
+ 3) There is no crowd ignore regions.
+ 4) All gt dets except pedestrian are removed, also removes pedestrian gt dets marked with zero_marked.
+ """
+ # Check that input data has unique ids
+ self._check_unique_ids(raw_data)
+
+ distractor_class_names = ['person_on_vehicle', 'static_person', 'distractor', 'reflection']
+ if self.benchmark == 'MOT20':
+ distractor_class_names.append('non_mot_vehicle')
+ distractor_classes = [self.class_name_to_class_id[x] for x in distractor_class_names]
+ cls_id = self.class_name_to_class_id[cls]
+
+ data_keys = ['gt_ids', 'tracker_ids', 'gt_dets', 'tracker_dets', 'tracker_confidences', 'similarity_scores']
+ data = {key: [None] * raw_data['num_timesteps'] for key in data_keys}
+ unique_gt_ids = []
+ unique_tracker_ids = []
+ num_gt_dets = 0
+ num_tracker_dets = 0
+ for t in range(raw_data['num_timesteps']):
+
+ # Get all data
+ gt_ids = raw_data['gt_ids'][t]
+ gt_dets = raw_data['gt_dets'][t]
+ gt_classes = raw_data['gt_classes'][t]
+ gt_zero_marked = raw_data['gt_extras'][t]['zero_marked']
+
+ tracker_ids = raw_data['tracker_ids'][t]
+ tracker_dets = raw_data['tracker_dets'][t]
+ tracker_classes = raw_data['tracker_classes'][t]
+ tracker_confidences = raw_data['tracker_confidences'][t]
+ similarity_scores = raw_data['similarity_scores'][t]
+
+ # Evaluation is ONLY valid for pedestrian class
+ if len(tracker_classes) > 0 and np.max(tracker_classes) > 1:
+ raise TrackEvalException(
+ 'Evaluation is only valid for pedestrian class. Non pedestrian class (%i) found in sequence %s at '
+ 'timestep %i.' % (np.max(tracker_classes), raw_data['seq'], t))
+
+ # Match tracker and gt dets (with hungarian algorithm) and remove tracker dets which match with gt dets
+ # which are labeled as belonging to a distractor class.
+ to_remove_tracker = np.array([], np.int)
+ if self.do_preproc and self.benchmark != 'MOT15' and gt_ids.shape[0] > 0 and tracker_ids.shape[0] > 0:
+
+ # Check all classes are valid:
+ invalid_classes = np.setdiff1d(np.unique(gt_classes), self.valid_class_numbers)
+ if len(invalid_classes) > 0:
+ print(' '.join([str(x) for x in invalid_classes]))
+ raise(TrackEvalException('Attempting to evaluate using invalid gt classes. '
+ 'This warning only triggers if preprocessing is performed, '
+ 'e.g. not for MOT15 or where prepropressing is explicitly disabled. '
+ 'Please either check your gt data, or disable preprocessing. '
+ 'The following invalid classes were found in timestep ' + str(t) + ': ' +
+ ' '.join([str(x) for x in invalid_classes])))
+
+ matching_scores = similarity_scores.copy()
+ matching_scores[matching_scores < 0.5 - np.finfo('float').eps] = 0
+ match_rows, match_cols = linear_sum_assignment(-matching_scores)
+ actually_matched_mask = matching_scores[match_rows, match_cols] > 0 + np.finfo('float').eps
+ match_rows = match_rows[actually_matched_mask]
+ match_cols = match_cols[actually_matched_mask]
+
+ is_distractor_class = np.isin(gt_classes[match_rows], distractor_classes)
+ to_remove_tracker = match_cols[is_distractor_class]
+
+ # Apply preprocessing to remove all unwanted tracker dets.
+ data['tracker_ids'][t] = np.delete(tracker_ids, to_remove_tracker, axis=0)
+ data['tracker_dets'][t] = np.delete(tracker_dets, to_remove_tracker, axis=0)
+ data['tracker_confidences'][t] = np.delete(tracker_confidences, to_remove_tracker, axis=0)
+ similarity_scores = np.delete(similarity_scores, to_remove_tracker, axis=1)
+
+ # Remove gt detections marked as to remove (zero marked), and also remove gt detections not in pedestrian
+ # class (not applicable for MOT15)
+ if self.do_preproc and self.benchmark != 'MOT15':
+ gt_to_keep_mask = (np.not_equal(gt_zero_marked, 0)) & \
+ (np.equal(gt_classes, cls_id))
+ else:
+ # There are no classes for MOT15
+ gt_to_keep_mask = np.not_equal(gt_zero_marked, 0)
+ data['gt_ids'][t] = gt_ids[gt_to_keep_mask]
+ data['gt_dets'][t] = gt_dets[gt_to_keep_mask, :]
+ data['similarity_scores'][t] = similarity_scores[gt_to_keep_mask]
+
+ unique_gt_ids += list(np.unique(data['gt_ids'][t]))
+ unique_tracker_ids += list(np.unique(data['tracker_ids'][t]))
+ num_tracker_dets += len(data['tracker_ids'][t])
+ num_gt_dets += len(data['gt_ids'][t])
+
+ # Re-label IDs such that there are no empty IDs
+ if len(unique_gt_ids) > 0:
+ unique_gt_ids = np.unique(unique_gt_ids)
+ gt_id_map = np.nan * np.ones((np.max(unique_gt_ids) + 1))
+ gt_id_map[unique_gt_ids] = np.arange(len(unique_gt_ids))
+ for t in range(raw_data['num_timesteps']):
+ if len(data['gt_ids'][t]) > 0:
+ data['gt_ids'][t] = gt_id_map[data['gt_ids'][t]].astype(np.int)
+ if len(unique_tracker_ids) > 0:
+ unique_tracker_ids = np.unique(unique_tracker_ids)
+ tracker_id_map = np.nan * np.ones((np.max(unique_tracker_ids) + 1))
+ tracker_id_map[unique_tracker_ids] = np.arange(len(unique_tracker_ids))
+ for t in range(raw_data['num_timesteps']):
+ if len(data['tracker_ids'][t]) > 0:
+ data['tracker_ids'][t] = tracker_id_map[data['tracker_ids'][t]].astype(np.int)
+
+ # Record overview statistics.
+ data['num_tracker_dets'] = num_tracker_dets
+ data['num_gt_dets'] = num_gt_dets
+ data['num_tracker_ids'] = len(unique_tracker_ids)
+ data['num_gt_ids'] = len(unique_gt_ids)
+ data['num_timesteps'] = raw_data['num_timesteps']
+ data['seq'] = raw_data['seq']
+
+ # Ensure again that ids are unique per timestep after preproc.
+ self._check_unique_ids(data, after_preproc=True)
+
+ return data
+
+ def _calculate_similarities(self, gt_dets_t, tracker_dets_t):
+ similarity_scores = self._calculate_box_ious(gt_dets_t, tracker_dets_t, box_format='xywh')
+ return similarity_scores
diff --git a/val_utils/trackeval/datasets/mots_challenge.py b/val_utils/trackeval/datasets/mots_challenge.py
new file mode 100644
index 0000000000000000000000000000000000000000..191b43842d1e5e6b358ab72fb95594279fe69aae
--- /dev/null
+++ b/val_utils/trackeval/datasets/mots_challenge.py
@@ -0,0 +1,446 @@
+import os
+import csv
+import configparser
+import numpy as np
+from scipy.optimize import linear_sum_assignment
+from ._base_dataset import _BaseDataset
+from .. import utils
+from .. import _timing
+from ..utils import TrackEvalException
+
+
+class MOTSChallenge(_BaseDataset):
+ """Dataset class for MOTS Challenge tracking"""
+
+ @staticmethod
+ def get_default_dataset_config():
+ """Default class config values"""
+ code_path = utils.get_code_path()
+ default_config = {
+ 'GT_FOLDER': os.path.join(code_path, 'data/gt/mot_challenge/'), # Location of GT data
+ 'TRACKERS_FOLDER': os.path.join(code_path, 'data/trackers/mot_challenge/'), # Trackers location
+ 'OUTPUT_FOLDER': None, # Where to save eval results (if None, same as TRACKERS_FOLDER)
+ 'TRACKERS_TO_EVAL': None, # Filenames of trackers to eval (if None, all in folder)
+ 'CLASSES_TO_EVAL': ['pedestrian'], # Valid: ['pedestrian']
+ 'SPLIT_TO_EVAL': 'train', # Valid: 'train', 'test'
+ 'INPUT_AS_ZIP': False, # Whether tracker input files are zipped
+ 'PRINT_CONFIG': True, # Whether to print current config
+ 'TRACKER_SUB_FOLDER': 'data', # Tracker files are in TRACKER_FOLDER/tracker_name/TRACKER_SUB_FOLDER
+ 'OUTPUT_SUB_FOLDER': '', # Output files are saved in OUTPUT_FOLDER/tracker_name/OUTPUT_SUB_FOLDER
+ 'TRACKER_DISPLAY_NAMES': None, # Names of trackers to display, if None: TRACKERS_TO_EVAL
+ 'SEQMAP_FOLDER': None, # Where seqmaps are found (if None, GT_FOLDER/seqmaps)
+ 'SEQMAP_FILE': None, # Directly specify seqmap file (if none use seqmap_folder/MOTS-split_to_eval)
+ 'SEQ_INFO': None, # If not None, directly specify sequences to eval and their number of timesteps
+ 'GT_LOC_FORMAT': '{gt_folder}/{seq}/gt/gt.txt', # '{gt_folder}/{seq}/gt/gt.txt'
+ 'SKIP_SPLIT_FOL': False, # If False, data is in GT_FOLDER/MOTS-SPLIT_TO_EVAL/ and in
+ # TRACKERS_FOLDER/MOTS-SPLIT_TO_EVAL/tracker/
+ # If True, then the middle 'MOTS-split' folder is skipped for both.
+ }
+ return default_config
+
+ def __init__(self, config=None):
+ """Initialise dataset, checking that all required files are present"""
+ super().__init__()
+ # Fill non-given config values with defaults
+ self.config = utils.init_config(config, self.get_default_dataset_config(), self.get_name())
+
+ self.benchmark = 'MOTS'
+ self.gt_set = self.benchmark + '-' + self.config['SPLIT_TO_EVAL']
+ if not self.config['SKIP_SPLIT_FOL']:
+ split_fol = self.gt_set
+ else:
+ split_fol = ''
+ self.gt_fol = os.path.join(self.config['GT_FOLDER'], split_fol)
+ self.tracker_fol = os.path.join(self.config['TRACKERS_FOLDER'], split_fol)
+ self.should_classes_combine = False
+ self.use_super_categories = False
+ self.data_is_zipped = self.config['INPUT_AS_ZIP']
+
+ self.output_fol = self.config['OUTPUT_FOLDER']
+ if self.output_fol is None:
+ self.output_fol = self.tracker_fol
+
+ self.tracker_sub_fol = self.config['TRACKER_SUB_FOLDER']
+ self.output_sub_fol = self.config['OUTPUT_SUB_FOLDER']
+
+ # Get classes to eval
+ self.valid_classes = ['pedestrian']
+ self.class_list = [cls.lower() if cls.lower() in self.valid_classes else None
+ for cls in self.config['CLASSES_TO_EVAL']]
+ if not all(self.class_list):
+ raise TrackEvalException('Attempted to evaluate an invalid class. Only pedestrian class is valid.')
+ self.class_name_to_class_id = {'pedestrian': '2', 'ignore': '10'}
+
+ # Get sequences to eval and check gt files exist
+ self.seq_list, self.seq_lengths = self._get_seq_info()
+ if len(self.seq_list) < 1:
+ raise TrackEvalException('No sequences are selected to be evaluated.')
+
+ # Check gt files exist
+ for seq in self.seq_list:
+ if not self.data_is_zipped:
+ curr_file = self.config["GT_LOC_FORMAT"].format(gt_folder=self.gt_fol, seq=seq)
+ if not os.path.isfile(curr_file):
+ print('GT file not found ' + curr_file)
+ raise TrackEvalException('GT file not found for sequence: ' + seq)
+ if self.data_is_zipped:
+ curr_file = os.path.join(self.gt_fol, 'data.zip')
+ if not os.path.isfile(curr_file):
+ print('GT file not found ' + curr_file)
+ raise TrackEvalException('GT file not found: ' + os.path.basename(curr_file))
+
+ # Get trackers to eval
+ if self.config['TRACKERS_TO_EVAL'] is None:
+ self.tracker_list = os.listdir(self.tracker_fol)
+ else:
+ self.tracker_list = self.config['TRACKERS_TO_EVAL']
+
+ if self.config['TRACKER_DISPLAY_NAMES'] is None:
+ self.tracker_to_disp = dict(zip(self.tracker_list, self.tracker_list))
+ elif (self.config['TRACKERS_TO_EVAL'] is not None) and (
+ len(self.config['TRACKER_DISPLAY_NAMES']) == len(self.tracker_list)):
+ self.tracker_to_disp = dict(zip(self.tracker_list, self.config['TRACKER_DISPLAY_NAMES']))
+ else:
+ raise TrackEvalException('List of tracker files and tracker display names do not match.')
+
+ for tracker in self.tracker_list:
+ if self.data_is_zipped:
+ curr_file = os.path.join(self.tracker_fol, tracker, self.tracker_sub_fol + '.zip')
+ if not os.path.isfile(curr_file):
+ print('Tracker file not found: ' + curr_file)
+ raise TrackEvalException('Tracker file not found: ' + tracker + '/' + os.path.basename(curr_file))
+ else:
+ for seq in self.seq_list:
+ curr_file = os.path.join(self.tracker_fol, tracker, self.tracker_sub_fol, seq + '.txt')
+ if not os.path.isfile(curr_file):
+ print('Tracker file not found: ' + curr_file)
+ raise TrackEvalException(
+ 'Tracker file not found: ' + tracker + '/' + self.tracker_sub_fol + '/' + os.path.basename(
+ curr_file))
+
+ def get_display_name(self, tracker):
+ return self.tracker_to_disp[tracker]
+
+ def _get_seq_info(self):
+ seq_list = []
+ seq_lengths = {}
+ if self.config["SEQ_INFO"]:
+ seq_list = list(self.config["SEQ_INFO"].keys())
+ seq_lengths = self.config["SEQ_INFO"]
+
+ # If sequence length is 'None' tries to read sequence length from .ini files.
+ for seq, seq_length in seq_lengths.items():
+ if seq_length is None:
+ ini_file = os.path.join(self.gt_fol, seq, 'seqinfo.ini')
+ if not os.path.isfile(ini_file):
+ raise TrackEvalException('ini file does not exist: ' + seq + '/' + os.path.basename(ini_file))
+ ini_data = configparser.ConfigParser()
+ ini_data.read(ini_file)
+ seq_lengths[seq] = int(ini_data['Sequence']['seqLength'])
+
+ else:
+ if self.config["SEQMAP_FILE"]:
+ seqmap_file = self.config["SEQMAP_FILE"]
+ else:
+ if self.config["SEQMAP_FOLDER"] is None:
+ seqmap_file = os.path.join(self.config['GT_FOLDER'], 'seqmaps', self.gt_set + '.txt')
+ else:
+ seqmap_file = os.path.join(self.config["SEQMAP_FOLDER"], self.gt_set + '.txt')
+ if not os.path.isfile(seqmap_file):
+ print('no seqmap found: ' + seqmap_file)
+ raise TrackEvalException('no seqmap found: ' + os.path.basename(seqmap_file))
+ with open(seqmap_file) as fp:
+ reader = csv.reader(fp)
+ for i, row in enumerate(reader):
+ if i == 0 or row[0] == '':
+ continue
+ seq = row[0]
+ seq_list.append(seq)
+ ini_file = os.path.join(self.gt_fol, seq, 'seqinfo.ini')
+ if not os.path.isfile(ini_file):
+ raise TrackEvalException('ini file does not exist: ' + seq + '/' + os.path.basename(ini_file))
+ ini_data = configparser.ConfigParser()
+ ini_data.read(ini_file)
+ seq_lengths[seq] = int(ini_data['Sequence']['seqLength'])
+ return seq_list, seq_lengths
+
+ def _load_raw_file(self, tracker, seq, is_gt):
+ """Load a file (gt or tracker) in the MOTS Challenge format
+
+ If is_gt, this returns a dict which contains the fields:
+ [gt_ids, gt_classes] : list (for each timestep) of 1D NDArrays (for each det).
+ [gt_dets]: list (for each timestep) of lists of detections.
+ [gt_ignore_region]: list (for each timestep) of masks for the ignore regions
+
+ if not is_gt, this returns a dict which contains the fields:
+ [tracker_ids, tracker_classes] : list (for each timestep) of 1D NDArrays (for each det).
+ [tracker_dets]: list (for each timestep) of lists of detections.
+ """
+
+ # Only loaded when run to reduce minimum requirements
+ from pycocotools import mask as mask_utils
+
+ # File location
+ if self.data_is_zipped:
+ if is_gt:
+ zip_file = os.path.join(self.gt_fol, 'data.zip')
+ else:
+ zip_file = os.path.join(self.tracker_fol, tracker, self.tracker_sub_fol + '.zip')
+ file = seq + '.txt'
+ else:
+ zip_file = None
+ if is_gt:
+ file = self.config["GT_LOC_FORMAT"].format(gt_folder=self.gt_fol, seq=seq)
+ else:
+ file = os.path.join(self.tracker_fol, tracker, self.tracker_sub_fol, seq + '.txt')
+
+ # Ignore regions
+ if is_gt:
+ crowd_ignore_filter = {2: ['10']}
+ else:
+ crowd_ignore_filter = None
+
+ # Load raw data from text file
+ read_data, ignore_data = self._load_simple_text_file(file, crowd_ignore_filter=crowd_ignore_filter,
+ is_zipped=self.data_is_zipped, zip_file=zip_file,
+ force_delimiters=' ')
+
+ # Convert data to required format
+ num_timesteps = self.seq_lengths[seq]
+ data_keys = ['ids', 'classes', 'dets']
+ if is_gt:
+ data_keys += ['gt_ignore_region']
+ raw_data = {key: [None] * num_timesteps for key in data_keys}
+
+ # Check for any extra time keys
+ current_time_keys = [str(t + 1) for t in range(num_timesteps)]
+ extra_time_keys = [x for x in read_data.keys() if x not in current_time_keys]
+ if len(extra_time_keys) > 0:
+ if is_gt:
+ text = 'Ground-truth'
+ else:
+ text = 'Tracking'
+ raise TrackEvalException(
+ text + ' data contains the following invalid timesteps in seq %s: ' % seq + ', '.join(
+ [str(x) + ', ' for x in extra_time_keys]))
+
+ for t in range(num_timesteps):
+ time_key = str(t+1)
+ # list to collect all masks of a timestep to check for overlapping areas
+ all_masks = []
+ if time_key in read_data.keys():
+ try:
+ raw_data['dets'][t] = [{'size': [int(region[3]), int(region[4])],
+ 'counts': region[5].encode(encoding='UTF-8')}
+ for region in read_data[time_key]]
+ raw_data['ids'][t] = np.atleast_1d([region[1] for region in read_data[time_key]]).astype(int)
+ raw_data['classes'][t] = np.atleast_1d([region[2] for region in read_data[time_key]]).astype(int)
+ all_masks += raw_data['dets'][t]
+ except IndexError:
+ self._raise_index_error(is_gt, tracker, seq)
+ except ValueError:
+ self._raise_value_error(is_gt, tracker, seq)
+ else:
+ raw_data['dets'][t] = []
+ raw_data['ids'][t] = np.empty(0).astype(int)
+ raw_data['classes'][t] = np.empty(0).astype(int)
+ if is_gt:
+ if time_key in ignore_data.keys():
+ try:
+ time_ignore = [{'size': [int(region[3]), int(region[4])],
+ 'counts': region[5].encode(encoding='UTF-8')}
+ for region in ignore_data[time_key]]
+ raw_data['gt_ignore_region'][t] = mask_utils.merge([mask for mask in time_ignore],
+ intersect=False)
+ all_masks += [raw_data['gt_ignore_region'][t]]
+ except IndexError:
+ self._raise_index_error(is_gt, tracker, seq)
+ except ValueError:
+ self._raise_value_error(is_gt, tracker, seq)
+ else:
+ raw_data['gt_ignore_region'][t] = mask_utils.merge([], intersect=False)
+
+ # check for overlapping masks
+ if all_masks:
+ masks_merged = all_masks[0]
+ for mask in all_masks[1:]:
+ if mask_utils.area(mask_utils.merge([masks_merged, mask], intersect=True)) != 0.0:
+ raise TrackEvalException(
+ 'Tracker has overlapping masks. Tracker: ' + tracker + ' Seq: ' + seq + ' Timestep: ' + str(
+ t))
+ masks_merged = mask_utils.merge([masks_merged, mask], intersect=False)
+
+ if is_gt:
+ key_map = {'ids': 'gt_ids',
+ 'classes': 'gt_classes',
+ 'dets': 'gt_dets'}
+ else:
+ key_map = {'ids': 'tracker_ids',
+ 'classes': 'tracker_classes',
+ 'dets': 'tracker_dets'}
+ for k, v in key_map.items():
+ raw_data[v] = raw_data.pop(k)
+ raw_data['num_timesteps'] = num_timesteps
+ raw_data['seq'] = seq
+ return raw_data
+
+ @_timing.time
+ def get_preprocessed_seq_data(self, raw_data, cls):
+ """ Preprocess data for a single sequence for a single class ready for evaluation.
+ Inputs:
+ - raw_data is a dict containing the data for the sequence already read in by get_raw_seq_data().
+ - cls is the class to be evaluated.
+ Outputs:
+ - data is a dict containing all of the information that metrics need to perform evaluation.
+ It contains the following fields:
+ [num_timesteps, num_gt_ids, num_tracker_ids, num_gt_dets, num_tracker_dets] : integers.
+ [gt_ids, tracker_ids]: list (for each timestep) of 1D NDArrays (for each det).
+ [gt_dets, tracker_dets]: list (for each timestep) of lists of detection masks.
+ [similarity_scores]: list (for each timestep) of 2D NDArrays.
+ Notes:
+ General preprocessing (preproc) occurs in 4 steps. Some datasets may not use all of these steps.
+ 1) Extract only detections relevant for the class to be evaluated (including distractor detections).
+ 2) Match gt dets and tracker dets. Remove tracker dets that are matched to a gt det that is of a
+ distractor class, or otherwise marked as to be removed.
+ 3) Remove unmatched tracker dets if they fall within a crowd ignore region or don't meet a certain
+ other criteria (e.g. are too small).
+ 4) Remove gt dets that were only useful for preprocessing and not for actual evaluation.
+ After the above preprocessing steps, this function also calculates the number of gt and tracker detections
+ and unique track ids. It also relabels gt and tracker ids to be contiguous and checks that ids are
+ unique within each timestep.
+
+ MOTS Challenge:
+ In MOTS Challenge, the 4 preproc steps are as follow:
+ 1) There is only one class (pedestrians) to be evaluated.
+ 2) There are no ground truth detections marked as to be removed/distractor classes.
+ Therefore also no matched tracker detections are removed.
+ 3) Ignore regions are used to remove unmatched detections (at least 50% overlap with ignore region).
+ 4) There are no ground truth detections (e.g. those of distractor classes) to be removed.
+ """
+ # Check that input data has unique ids
+ self._check_unique_ids(raw_data)
+
+ cls_id = int(self.class_name_to_class_id[cls])
+
+ data_keys = ['gt_ids', 'tracker_ids', 'gt_dets', 'tracker_dets', 'similarity_scores']
+ data = {key: [None] * raw_data['num_timesteps'] for key in data_keys}
+ unique_gt_ids = []
+ unique_tracker_ids = []
+ num_gt_dets = 0
+ num_tracker_dets = 0
+ for t in range(raw_data['num_timesteps']):
+
+ # Only extract relevant dets for this class for preproc and eval (cls)
+ gt_class_mask = np.atleast_1d(raw_data['gt_classes'][t] == cls_id)
+ gt_class_mask = gt_class_mask.astype(np.bool)
+ gt_ids = raw_data['gt_ids'][t][gt_class_mask]
+ gt_dets = [raw_data['gt_dets'][t][ind] for ind in range(len(gt_class_mask)) if gt_class_mask[ind]]
+
+ tracker_class_mask = np.atleast_1d(raw_data['tracker_classes'][t] == cls_id)
+ tracker_class_mask = tracker_class_mask.astype(np.bool)
+ tracker_ids = raw_data['tracker_ids'][t][tracker_class_mask]
+ tracker_dets = [raw_data['tracker_dets'][t][ind] for ind in range(len(tracker_class_mask)) if
+ tracker_class_mask[ind]]
+ similarity_scores = raw_data['similarity_scores'][t][gt_class_mask, :][:, tracker_class_mask]
+
+ # Match tracker and gt dets (with hungarian algorithm)
+ unmatched_indices = np.arange(tracker_ids.shape[0])
+ if gt_ids.shape[0] > 0 and tracker_ids.shape[0] > 0:
+ matching_scores = similarity_scores.copy()
+ matching_scores[matching_scores < 0.5 - np.finfo('float').eps] = -10000
+ match_rows, match_cols = linear_sum_assignment(-matching_scores)
+ actually_matched_mask = matching_scores[match_rows, match_cols] > 0 + np.finfo('float').eps
+ match_cols = match_cols[actually_matched_mask]
+
+ unmatched_indices = np.delete(unmatched_indices, match_cols, axis=0)
+
+ # For unmatched tracker dets, remove those that are greater than 50% within a crowd ignore region.
+ unmatched_tracker_dets = [tracker_dets[i] for i in range(len(tracker_dets)) if i in unmatched_indices]
+ ignore_region = raw_data['gt_ignore_region'][t]
+ intersection_with_ignore_region = self._calculate_mask_ious(unmatched_tracker_dets, [ignore_region],
+ is_encoded=True, do_ioa=True)
+ is_within_ignore_region = np.any(intersection_with_ignore_region > 0.5 + np.finfo('float').eps, axis=1)
+
+ # Apply preprocessing to remove unwanted tracker dets.
+ to_remove_tracker = unmatched_indices[is_within_ignore_region]
+ data['tracker_ids'][t] = np.delete(tracker_ids, to_remove_tracker, axis=0)
+ data['tracker_dets'][t] = np.delete(tracker_dets, to_remove_tracker, axis=0)
+ similarity_scores = np.delete(similarity_scores, to_remove_tracker, axis=1)
+
+ # Keep all ground truth detections
+ data['gt_ids'][t] = gt_ids
+ data['gt_dets'][t] = gt_dets
+ data['similarity_scores'][t] = similarity_scores
+
+ unique_gt_ids += list(np.unique(data['gt_ids'][t]))
+ unique_tracker_ids += list(np.unique(data['tracker_ids'][t]))
+ num_tracker_dets += len(data['tracker_ids'][t])
+ num_gt_dets += len(data['gt_ids'][t])
+
+ # Re-label IDs such that there are no empty IDs
+ if len(unique_gt_ids) > 0:
+ unique_gt_ids = np.unique(unique_gt_ids)
+ gt_id_map = np.nan * np.ones((np.max(unique_gt_ids) + 1))
+ gt_id_map[unique_gt_ids] = np.arange(len(unique_gt_ids))
+ for t in range(raw_data['num_timesteps']):
+ if len(data['gt_ids'][t]) > 0:
+ data['gt_ids'][t] = gt_id_map[data['gt_ids'][t]].astype(np.int)
+ if len(unique_tracker_ids) > 0:
+ unique_tracker_ids = np.unique(unique_tracker_ids)
+ tracker_id_map = np.nan * np.ones((np.max(unique_tracker_ids) + 1))
+ tracker_id_map[unique_tracker_ids] = np.arange(len(unique_tracker_ids))
+ for t in range(raw_data['num_timesteps']):
+ if len(data['tracker_ids'][t]) > 0:
+ data['tracker_ids'][t] = tracker_id_map[data['tracker_ids'][t]].astype(np.int)
+
+ # Record overview statistics.
+ data['num_tracker_dets'] = num_tracker_dets
+ data['num_gt_dets'] = num_gt_dets
+ data['num_tracker_ids'] = len(unique_tracker_ids)
+ data['num_gt_ids'] = len(unique_gt_ids)
+ data['num_timesteps'] = raw_data['num_timesteps']
+ data['seq'] = raw_data['seq']
+
+ # Ensure again that ids are unique per timestep after preproc.
+ self._check_unique_ids(data, after_preproc=True)
+
+ return data
+
+ def _calculate_similarities(self, gt_dets_t, tracker_dets_t):
+ similarity_scores = self._calculate_mask_ious(gt_dets_t, tracker_dets_t, is_encoded=True, do_ioa=False)
+ return similarity_scores
+
+ @staticmethod
+ def _raise_index_error(is_gt, tracker, seq):
+ """
+ Auxiliary method to raise an evaluation error in case of an index error while reading files.
+ :param is_gt: whether gt or tracker data is read
+ :param tracker: the name of the tracker
+ :param seq: the name of the seq
+ :return: None
+ """
+ if is_gt:
+ err = 'Cannot load gt data from sequence %s, because there are not enough ' \
+ 'columns in the data.' % seq
+ raise TrackEvalException(err)
+ else:
+ err = 'Cannot load tracker data from tracker %s, sequence %s, because there are not enough ' \
+ 'columns in the data.' % (tracker, seq)
+ raise TrackEvalException(err)
+
+ @staticmethod
+ def _raise_value_error(is_gt, tracker, seq):
+ """
+ Auxiliary method to raise an evaluation error in case of an value error while reading files.
+ :param is_gt: whether gt or tracker data is read
+ :param tracker: the name of the tracker
+ :param seq: the name of the seq
+ :return: None
+ """
+ if is_gt:
+ raise TrackEvalException(
+ 'GT data for sequence %s cannot be converted to the right format. Is data corrupted?' % seq)
+ else:
+ raise TrackEvalException(
+ 'Tracking data from tracker %s, sequence %s cannot be converted to the right format. '
+ 'Is data corrupted?' % (tracker, seq))
diff --git a/val_utils/trackeval/datasets/person_path_22.py b/val_utils/trackeval/datasets/person_path_22.py
new file mode 100644
index 0000000000000000000000000000000000000000..177954a82009d68e7e4a68a2087255fc5dcac42e
--- /dev/null
+++ b/val_utils/trackeval/datasets/person_path_22.py
@@ -0,0 +1,452 @@
+import os
+import csv
+import configparser
+import numpy as np
+from scipy.optimize import linear_sum_assignment
+from ._base_dataset import _BaseDataset
+from .. import utils
+from .. import _timing
+from ..utils import TrackEvalException
+
+class PersonPath22(_BaseDataset):
+ """Dataset class for MOT Challenge 2D bounding box tracking"""
+
+ @staticmethod
+ def get_default_dataset_config():
+ """Default class config values"""
+ code_path = utils.get_code_path()
+ default_config = {
+ 'GT_FOLDER': os.path.join(code_path, 'data/gt/person_path_22/'), # Location of GT data
+ 'TRACKERS_FOLDER': os.path.join(code_path, 'data/trackers/person_path_22/'), # Trackers location
+ 'OUTPUT_FOLDER': None, # Where to save eval results (if None, same as TRACKERS_FOLDER)
+ 'TRACKERS_TO_EVAL': None, # Filenames of trackers to eval (if None, all in folder)
+ 'CLASSES_TO_EVAL': ['pedestrian'], # Valid: ['pedestrian']
+ 'BENCHMARK': 'person_path_22', # Valid: 'person_path_22'
+ 'SPLIT_TO_EVAL': 'test', # Valid: 'train', 'test', 'all'
+ 'INPUT_AS_ZIP': False, # Whether tracker input files are zipped
+ 'PRINT_CONFIG': True, # Whether to print current config
+ 'DO_PREPROC': True, # Whether to perform preprocessing
+ 'TRACKER_SUB_FOLDER': 'data', # Tracker files are in TRACKER_FOLDER/tracker_name/TRACKER_SUB_FOLDER
+ 'OUTPUT_SUB_FOLDER': '', # Output files are saved in OUTPUT_FOLDER/tracker_name/OUTPUT_SUB_FOLDER
+ 'TRACKER_DISPLAY_NAMES': None, # Names of trackers to display, if None: TRACKERS_TO_EVAL
+ 'SEQMAP_FOLDER': None, # Where seqmaps are found (if None, GT_FOLDER/seqmaps)
+ 'SEQMAP_FILE': None, # Directly specify seqmap file (if none use seqmap_folder/benchmark-split_to_eval)
+ 'SEQ_INFO': None, # If not None, directly specify sequences to eval and their number of timesteps
+ 'GT_LOC_FORMAT': '{gt_folder}/{seq}/gt/gt.txt', # '{gt_folder}/{seq}/gt/gt.txt'
+ 'SKIP_SPLIT_FOL': False, # If False, data is in GT_FOLDER/BENCHMARK-SPLIT_TO_EVAL/ and in
+ # TRACKERS_FOLDER/BENCHMARK-SPLIT_TO_EVAL/tracker/
+ # If True, then the middle 'benchmark-split' folder is skipped for both.
+ }
+ return default_config
+
+ def __init__(self, config=None):
+ """Initialise dataset, checking that all required files are present"""
+ super().__init__()
+ # Fill non-given config values with defaults
+ self.config = utils.init_config(config, self.get_default_dataset_config(), self.get_name())
+
+ self.benchmark = self.config['BENCHMARK']
+ gt_set = self.config['BENCHMARK'] + '-' + self.config['SPLIT_TO_EVAL']
+ self.gt_set = gt_set
+ if not self.config['SKIP_SPLIT_FOL']:
+ split_fol = gt_set
+ else:
+ split_fol = ''
+ self.gt_fol = os.path.join(self.config['GT_FOLDER'], split_fol)
+ self.tracker_fol = os.path.join(self.config['TRACKERS_FOLDER'], split_fol)
+ self.should_classes_combine = False
+ self.use_super_categories = False
+ self.data_is_zipped = self.config['INPUT_AS_ZIP']
+ self.do_preproc = self.config['DO_PREPROC']
+
+ self.output_fol = self.config['OUTPUT_FOLDER']
+ if self.output_fol is None:
+ self.output_fol = self.tracker_fol
+
+ self.tracker_sub_fol = self.config['TRACKER_SUB_FOLDER']
+ self.output_sub_fol = self.config['OUTPUT_SUB_FOLDER']
+
+ # Get classes to eval
+ self.valid_classes = ['pedestrian']
+ self.class_list = [cls.lower() if cls.lower() in self.valid_classes else None
+ for cls in self.config['CLASSES_TO_EVAL']]
+ if not all(self.class_list):
+ raise TrackEvalException('Attempted to evaluate an invalid class. Only pedestrian class is valid.')
+ self.class_name_to_class_id = {'pedestrian': 1, 'person_on_vehicle': 2, 'car': 3, 'bicycle': 4, 'motorbike': 5,
+ 'non_mot_vehicle': 6, 'static_person': 7, 'distractor': 8, 'occluder': 9,
+ 'occluder_on_ground': 10, 'occluder_full': 11, 'reflection': 12, 'crowd': 13}
+ self.valid_class_numbers = list(self.class_name_to_class_id.values())
+
+ # Get sequences to eval and check gt files exist
+ self.seq_list, self.seq_lengths = self._get_seq_info()
+ if len(self.seq_list) < 1:
+ raise TrackEvalException('No sequences are selected to be evaluated.')
+
+ # Check gt files exist
+ for seq in self.seq_list:
+ if not self.data_is_zipped:
+ curr_file = self.config["GT_LOC_FORMAT"].format(gt_folder=self.gt_fol, seq=seq)
+ if not os.path.isfile(curr_file):
+ print('GT file not found ' + curr_file)
+ raise TrackEvalException('GT file not found for sequence: ' + seq)
+ if self.data_is_zipped:
+ curr_file = os.path.join(self.gt_fol, 'data.zip')
+ if not os.path.isfile(curr_file):
+ print('GT file not found ' + curr_file)
+ raise TrackEvalException('GT file not found: ' + os.path.basename(curr_file))
+
+ # Get trackers to eval
+ if self.config['TRACKERS_TO_EVAL'] is None:
+ self.tracker_list = os.listdir(self.tracker_fol)
+ else:
+ self.tracker_list = self.config['TRACKERS_TO_EVAL']
+
+ if self.config['TRACKER_DISPLAY_NAMES'] is None:
+ self.tracker_to_disp = dict(zip(self.tracker_list, self.tracker_list))
+ elif (self.config['TRACKERS_TO_EVAL'] is not None) and (
+ len(self.config['TRACKER_DISPLAY_NAMES']) == len(self.tracker_list)):
+ self.tracker_to_disp = dict(zip(self.tracker_list, self.config['TRACKER_DISPLAY_NAMES']))
+ else:
+ raise TrackEvalException('List of tracker files and tracker display names do not match.')
+
+ for tracker in self.tracker_list:
+ if self.data_is_zipped:
+ curr_file = os.path.join(self.tracker_fol, tracker, self.tracker_sub_fol + '.zip')
+ if not os.path.isfile(curr_file):
+ print('Tracker file not found: ' + curr_file)
+ raise TrackEvalException('Tracker file not found: ' + tracker + '/' + os.path.basename(curr_file))
+ else:
+ for seq in self.seq_list:
+ curr_file = os.path.join(self.tracker_fol, tracker, self.tracker_sub_fol, seq + '.txt')
+ if not os.path.isfile(curr_file):
+ print('Tracker file not found: ' + curr_file)
+ raise TrackEvalException(
+ 'Tracker file not found: ' + tracker + '/' + self.tracker_sub_fol + '/' + os.path.basename(
+ curr_file))
+
+ def get_display_name(self, tracker):
+ return self.tracker_to_disp[tracker]
+
+ def _get_seq_info(self):
+ seq_list = []
+ seq_lengths = {}
+ if self.config["SEQ_INFO"]:
+ seq_list = list(self.config["SEQ_INFO"].keys())
+ seq_lengths = self.config["SEQ_INFO"]
+
+ # If sequence length is 'None' tries to read sequence length from .ini files.
+ for seq, seq_length in seq_lengths.items():
+ if seq_length is None:
+ ini_file = os.path.join(self.gt_fol, seq, 'seqinfo.ini')
+ if not os.path.isfile(ini_file):
+ raise TrackEvalException('ini file does not exist: ' + seq + '/' + os.path.basename(ini_file))
+ ini_data = configparser.ConfigParser()
+ ini_data.read(ini_file)
+ seq_lengths[seq] = int(ini_data['Sequence']['seqLength'])
+
+ else:
+ if self.config["SEQMAP_FILE"]:
+ seqmap_file = self.config["SEQMAP_FILE"]
+ else:
+ if self.config["SEQMAP_FOLDER"] is None:
+ seqmap_file = os.path.join(self.config['GT_FOLDER'], 'seqmaps', self.gt_set + '.txt')
+ else:
+ seqmap_file = os.path.join(self.config["SEQMAP_FOLDER"], self.gt_set + '.txt')
+ if not os.path.isfile(seqmap_file):
+ print('no seqmap found: ' + seqmap_file)
+ raise TrackEvalException('no seqmap found: ' + os.path.basename(seqmap_file))
+ with open(seqmap_file) as fp:
+ reader = csv.reader(fp)
+ for i, row in enumerate(reader):
+ if i == 0 or row[0] == '':
+ continue
+ seq = row[0]
+ seq_list.append(seq)
+ ini_file = os.path.join(self.gt_fol, seq, 'seqinfo.ini')
+ if not os.path.isfile(ini_file):
+ raise TrackEvalException('ini file does not exist: ' + seq + '/' + os.path.basename(ini_file))
+ ini_data = configparser.ConfigParser()
+ ini_data.read(ini_file)
+ seq_lengths[seq] = int(ini_data['Sequence']['seqLength'])
+ return seq_list, seq_lengths
+
+ def _load_raw_file(self, tracker, seq, is_gt):
+ """Load a file (gt or tracker) in the MOT Challenge 2D box format
+
+ If is_gt, this returns a dict which contains the fields:
+ [gt_ids, gt_classes] : list (for each timestep) of 1D NDArrays (for each det).
+ [gt_dets, gt_crowd_ignore_regions]: list (for each timestep) of lists of detections.
+ [gt_extras] : list (for each timestep) of dicts (for each extra) of 1D NDArrays (for each det).
+
+ if not is_gt, this returns a dict which contains the fields:
+ [tracker_ids, tracker_classes, tracker_confidences] : list (for each timestep) of 1D NDArrays (for each det).
+ [tracker_dets]: list (for each timestep) of lists of detections.
+ """
+ # File location
+ if self.data_is_zipped:
+ if is_gt:
+ zip_file = os.path.join(self.gt_fol, 'data.zip')
+ else:
+ zip_file = os.path.join(self.tracker_fol, tracker, self.tracker_sub_fol + '.zip')
+ file = seq + '.txt'
+ else:
+ zip_file = None
+ if is_gt:
+ file = self.config["GT_LOC_FORMAT"].format(gt_folder=self.gt_fol, seq=seq)
+ else:
+ file = os.path.join(self.tracker_fol, tracker, self.tracker_sub_fol, seq + '.txt')
+
+ # Ignore regions
+ if is_gt:
+ crowd_ignore_filter = {7: ['13']}
+ else:
+ crowd_ignore_filter = None
+
+ # Load raw data from text file
+ read_data, ignore_data = self._load_simple_text_file(file, is_zipped=self.data_is_zipped, zip_file=zip_file, crowd_ignore_filter=crowd_ignore_filter)
+
+ # Convert data to required format
+ num_timesteps = self.seq_lengths[seq]
+ data_keys = ['ids', 'classes', 'dets']
+ if is_gt:
+ data_keys += ['gt_crowd_ignore_regions', 'gt_extras']
+ else:
+ data_keys += ['tracker_confidences']
+ raw_data = {key: [None] * num_timesteps for key in data_keys}
+
+ # Check for any extra time keys
+ current_time_keys = [str( t+ 1) for t in range(num_timesteps)]
+ extra_time_keys = [x for x in read_data.keys() if x not in current_time_keys]
+ if len(extra_time_keys) > 0:
+ if is_gt:
+ text = 'Ground-truth'
+ else:
+ text = 'Tracking'
+ raise TrackEvalException(
+ text + ' data contains the following invalid timesteps in seq %s: ' % seq + ', '.join(
+ [str(x) + ', ' for x in extra_time_keys]))
+
+ for t in range(num_timesteps):
+ time_key = str(t+1)
+ if time_key in read_data.keys():
+ try:
+ time_data = np.asarray(read_data[time_key], dtype=np.float)
+ except ValueError:
+ if is_gt:
+ raise TrackEvalException(
+ 'Cannot convert gt data for sequence %s to float. Is data corrupted?' % seq)
+ else:
+ raise TrackEvalException(
+ 'Cannot convert tracking data from tracker %s, sequence %s to float. Is data corrupted?' % (
+ tracker, seq))
+ try:
+ raw_data['dets'][t] = np.atleast_2d(time_data[:, 2:6])
+ raw_data['ids'][t] = np.atleast_1d(time_data[:, 1]).astype(int)
+ except IndexError:
+ if is_gt:
+ err = 'Cannot load gt data from sequence %s, because there is not enough ' \
+ 'columns in the data.' % seq
+ raise TrackEvalException(err)
+ else:
+ err = 'Cannot load tracker data from tracker %s, sequence %s, because there is not enough ' \
+ 'columns in the data.' % (tracker, seq)
+ raise TrackEvalException(err)
+ if time_data.shape[1] >= 8:
+ raw_data['classes'][t] = np.atleast_1d(time_data[:, 7]).astype(int)
+ else:
+ if not is_gt:
+ raw_data['classes'][t] = np.ones_like(raw_data['ids'][t])
+ else:
+ raise TrackEvalException(
+ 'GT data is not in a valid format, there is not enough rows in seq %s, timestep %i.' % (
+ seq, t))
+ if is_gt:
+ gt_extras_dict = {'zero_marked': np.atleast_1d(time_data[:, 6].astype(int))}
+ raw_data['gt_extras'][t] = gt_extras_dict
+ else:
+ raw_data['tracker_confidences'][t] = np.atleast_1d(time_data[:, 6])
+ else:
+ raw_data['dets'][t] = np.empty((0, 4))
+ raw_data['ids'][t] = np.empty(0).astype(int)
+ raw_data['classes'][t] = np.empty(0).astype(int)
+ if is_gt:
+ gt_extras_dict = {'zero_marked': np.empty(0)}
+ raw_data['gt_extras'][t] = gt_extras_dict
+ else:
+ raw_data['tracker_confidences'][t] = np.empty(0)
+ if is_gt:
+ if time_key in ignore_data.keys():
+ time_ignore = np.asarray(ignore_data[time_key], dtype=np.float)
+ raw_data['gt_crowd_ignore_regions'][t] = np.atleast_2d(time_ignore[:, 2:6])
+ else:
+ raw_data['gt_crowd_ignore_regions'][t] = np.empty((0, 4))
+
+ if is_gt:
+ key_map = {'ids': 'gt_ids',
+ 'classes': 'gt_classes',
+ 'dets': 'gt_dets'}
+ else:
+ key_map = {'ids': 'tracker_ids',
+ 'classes': 'tracker_classes',
+ 'dets': 'tracker_dets'}
+ for k, v in key_map.items():
+ raw_data[v] = raw_data.pop(k)
+ raw_data['num_timesteps'] = num_timesteps
+ raw_data['seq'] = seq
+ return raw_data
+
+ @_timing.time
+ def get_preprocessed_seq_data(self, raw_data, cls):
+ """ Preprocess data for a single sequence for a single class ready for evaluation.
+ Inputs:
+ - raw_data is a dict containing the data for the sequence already read in by get_raw_seq_data().
+ - cls is the class to be evaluated.
+ Outputs:
+ - data is a dict containing all of the information that metrics need to perform evaluation.
+ It contains the following fields:
+ [num_timesteps, num_gt_ids, num_tracker_ids, num_gt_dets, num_tracker_dets] : integers.
+ [gt_ids, tracker_ids, tracker_confidences]: list (for each timestep) of 1D NDArrays (for each det).
+ [gt_dets, tracker_dets]: list (for each timestep) of lists of detections.
+ [similarity_scores]: list (for each timestep) of 2D NDArrays.
+ Notes:
+ General preprocessing (preproc) occurs in 4 steps. Some datasets may not use all of these steps.
+ 1) Extract only detections relevant for the class to be evaluated (including distractor detections).
+ 2) Match gt dets and tracker dets. Remove tracker dets that are matched to a gt det that is of a
+ distractor class, or otherwise marked as to be removed.
+ 3) Remove unmatched tracker dets if they fall within a crowd ignore region or don't meet a certain
+ other criteria (e.g. are too small).
+ 4) Remove gt dets that were only useful for preprocessing and not for actual evaluation.
+ After the above preprocessing steps, this function also calculates the number of gt and tracker detections
+ and unique track ids. It also relabels gt and tracker ids to be contiguous and checks that ids are
+ unique within each timestep.
+
+ MOT Challenge:
+ In MOT Challenge, the 4 preproc steps are as follow:
+ 1) There is only one class (pedestrian) to be evaluated, but all other classes are used for preproc.
+ 2) Predictions are matched against all gt boxes (regardless of class), those matching with distractor
+ objects are removed.
+ 3) There is no crowd ignore regions.
+ 4) All gt dets except pedestrian are removed, also removes pedestrian gt dets marked with zero_marked.
+ """
+ # Check that input data has unique ids
+ self._check_unique_ids(raw_data)
+
+ distractor_class_names = ['person_on_vehicle', 'static_person', 'distractor', 'reflection']
+ if self.benchmark == 'MOT20':
+ distractor_class_names.append('non_mot_vehicle')
+ distractor_classes = [self.class_name_to_class_id[x] for x in distractor_class_names]
+ cls_id = self.class_name_to_class_id[cls]
+
+ data_keys = ['gt_ids', 'tracker_ids', 'gt_dets', 'tracker_dets', 'tracker_confidences', 'similarity_scores']
+ data = {key: [None] * raw_data['num_timesteps'] for key in data_keys}
+ unique_gt_ids = []
+ unique_tracker_ids = []
+ num_gt_dets = 0
+ num_tracker_dets = 0
+ for t in range(raw_data['num_timesteps']):
+
+ # Get all data
+ gt_ids = raw_data['gt_ids'][t]
+ gt_dets = raw_data['gt_dets'][t]
+ gt_classes = raw_data['gt_classes'][t]
+ gt_zero_marked = raw_data['gt_extras'][t]['zero_marked']
+
+ tracker_ids = raw_data['tracker_ids'][t]
+ tracker_dets = raw_data['tracker_dets'][t]
+ tracker_classes = raw_data['tracker_classes'][t]
+ tracker_confidences = raw_data['tracker_confidences'][t]
+ similarity_scores = raw_data['similarity_scores'][t]
+ crowd_ignore_regions = raw_data['gt_crowd_ignore_regions'][t]
+
+ # Evaluation is ONLY valid for pedestrian class
+ if len(tracker_classes) > 0 and np.max(tracker_classes) > 1:
+ raise TrackEvalException(
+ 'Evaluation is only valid for pedestrian class. Non pedestrian class (%i) found in sequence %s at '
+ 'timestep %i.' % (np.max(tracker_classes), raw_data['seq'], t))
+
+ # Match tracker and gt dets (with hungarian algorithm) and remove tracker dets which match with gt dets
+ # which are labeled as belonging to a distractor class.
+ to_remove_tracker = np.array([], np.int)
+ if self.do_preproc and self.benchmark != 'MOT15' and (gt_ids.shape[0] > 0 or len(crowd_ignore_regions) > 0) and tracker_ids.shape[0] > 0:
+
+ # Check all classes are valid:
+ invalid_classes = np.setdiff1d(np.unique(gt_classes), self.valid_class_numbers)
+ if len(invalid_classes) > 0:
+ print(' '.join([str(x) for x in invalid_classes]))
+ raise(TrackEvalException('Attempting to evaluate using invalid gt classes. '
+ 'This warning only triggers if preprocessing is performed, '
+ 'e.g. not for MOT15 or where prepropressing is explicitly disabled. '
+ 'Please either check your gt data, or disable preprocessing. '
+ 'The following invalid classes were found in timestep ' + str(t) + ': ' +
+ ' '.join([str(x) for x in invalid_classes])))
+
+ matching_scores = similarity_scores.copy()
+ matching_scores[matching_scores < 0.5 - np.finfo('float').eps] = 0
+ match_rows, match_cols = linear_sum_assignment(-matching_scores)
+ actually_matched_mask = matching_scores[match_rows, match_cols] > 0 + np.finfo('float').eps
+ match_rows = match_rows[actually_matched_mask]
+ match_cols = match_cols[actually_matched_mask]
+
+ is_distractor_class = np.isin(gt_classes[match_rows], distractor_classes)
+ to_remove_tracker = match_cols[is_distractor_class]
+
+ # remove bounding boxes that overlap with crowd ignore region.
+ intersection_with_ignore_region = self._calculate_box_ious(tracker_dets, crowd_ignore_regions, box_format='xywh', do_ioa=True)
+ is_within_crowd_ignore_region = np.any(intersection_with_ignore_region > 0.95 + np.finfo('float').eps, axis=1)
+ to_remove_tracker = np.unique(np.concatenate([to_remove_tracker, np.where(is_within_crowd_ignore_region)[0]]))
+
+ # Apply preprocessing to remove all unwanted tracker dets.
+ data['tracker_ids'][t] = np.delete(tracker_ids, to_remove_tracker, axis=0)
+ data['tracker_dets'][t] = np.delete(tracker_dets, to_remove_tracker, axis=0)
+ data['tracker_confidences'][t] = np.delete(tracker_confidences, to_remove_tracker, axis=0)
+ similarity_scores = np.delete(similarity_scores, to_remove_tracker, axis=1)
+
+ # Remove gt detections marked as to remove (zero marked), and also remove gt detections not in pedestrian
+ # class (not applicable for MOT15)
+ if self.do_preproc and self.benchmark != 'MOT15':
+ gt_to_keep_mask = (np.not_equal(gt_zero_marked, 0)) & \
+ (np.equal(gt_classes, cls_id))
+ else:
+ # There are no classes for MOT15
+ gt_to_keep_mask = np.not_equal(gt_zero_marked, 0)
+ data['gt_ids'][t] = gt_ids[gt_to_keep_mask]
+ data['gt_dets'][t] = gt_dets[gt_to_keep_mask, :]
+ data['similarity_scores'][t] = similarity_scores[gt_to_keep_mask]
+
+ unique_gt_ids += list(np.unique(data['gt_ids'][t]))
+ unique_tracker_ids += list(np.unique(data['tracker_ids'][t]))
+ num_tracker_dets += len(data['tracker_ids'][t])
+ num_gt_dets += len(data['gt_ids'][t])
+
+ # Re-label IDs such that there are no empty IDs
+ if len(unique_gt_ids) > 0:
+ unique_gt_ids = np.unique(unique_gt_ids)
+ gt_id_map = np.nan * np.ones((np.max(unique_gt_ids) + 1))
+ gt_id_map[unique_gt_ids] = np.arange(len(unique_gt_ids))
+ for t in range(raw_data['num_timesteps']):
+ if len(data['gt_ids'][t]) > 0:
+ data['gt_ids'][t] = gt_id_map[data['gt_ids'][t]].astype(np.int)
+ if len(unique_tracker_ids) > 0:
+ unique_tracker_ids = np.unique(unique_tracker_ids)
+ tracker_id_map = np.nan * np.ones((np.max(unique_tracker_ids) + 1))
+ tracker_id_map[unique_tracker_ids] = np.arange(len(unique_tracker_ids))
+ for t in range(raw_data['num_timesteps']):
+ if len(data['tracker_ids'][t]) > 0:
+ data['tracker_ids'][t] = tracker_id_map[data['tracker_ids'][t]].astype(np.int)
+
+ # Record overview statistics.
+ data['num_tracker_dets'] = num_tracker_dets
+ data['num_gt_dets'] = num_gt_dets
+ data['num_tracker_ids'] = len(unique_tracker_ids)
+ data['num_gt_ids'] = len(unique_gt_ids)
+ data['num_timesteps'] = raw_data['num_timesteps']
+ data['seq'] = raw_data['seq']
+
+ # Ensure again that ids are unique per timestep after preproc.
+ self._check_unique_ids(data, after_preproc=True)
+
+ return data
+
+ def _calculate_similarities(self, gt_dets_t, tracker_dets_t):
+ similarity_scores = self._calculate_box_ious(gt_dets_t, tracker_dets_t, box_format='xywh')
+ return similarity_scores
diff --git a/val_utils/trackeval/datasets/rob_mots.py b/val_utils/trackeval/datasets/rob_mots.py
new file mode 100644
index 0000000000000000000000000000000000000000..d6a6d1e9f47f824191b674c4f5ef9b3719907742
--- /dev/null
+++ b/val_utils/trackeval/datasets/rob_mots.py
@@ -0,0 +1,508 @@
+
+import os
+import csv
+import numpy as np
+from scipy.optimize import linear_sum_assignment
+from ._base_dataset import _BaseDataset
+from .. import utils
+from ..utils import TrackEvalException
+from .. import _timing
+from ..datasets.rob_mots_classmap import cls_id_to_name
+
+
+class RobMOTS(_BaseDataset):
+
+ @staticmethod
+ def get_default_dataset_config():
+ """Default class config values"""
+ code_path = utils.get_code_path()
+ default_config = {
+ 'GT_FOLDER': os.path.join(code_path, 'data/gt/rob_mots'), # Location of GT data
+ 'TRACKERS_FOLDER': os.path.join(code_path, 'data/trackers/rob_mots'), # Trackers location
+ 'OUTPUT_FOLDER': None, # Where to save eval results (if None, same as TRACKERS_FOLDER)
+ 'TRACKERS_TO_EVAL': None, # Filenames of trackers to eval (if None, all in folder)
+ 'SUB_BENCHMARK': None, # REQUIRED. Sub-benchmark to eval. If None, then error.
+ # ['mots_challenge', 'kitti_mots', 'bdd_mots', 'davis_unsupervised', 'youtube_vis', 'ovis', 'waymo', 'tao']
+ 'CLASSES_TO_EVAL': None, # List of classes to eval. If None, then it does all COCO classes.
+ 'SPLIT_TO_EVAL': 'train', # valid: ['train', 'val', 'test']
+ 'INPUT_AS_ZIP': False, # Whether tracker input files are zipped
+ 'PRINT_CONFIG': True, # Whether to print current config
+ 'OUTPUT_SUB_FOLDER': 'results', # Output files are saved in OUTPUT_FOLDER/DATA_LOC_FORMAT/OUTPUT_SUB_FOLDER
+ 'TRACKER_SUB_FOLDER': 'data', # Tracker files are in TRACKER_FOLDER/DATA_LOC_FORMAT/TRACKER_SUB_FOLDER
+ 'TRACKER_DISPLAY_NAMES': None, # Names of trackers to display, if None: TRACKERS_TO_EVAL
+ 'SEQMAP_FOLDER': None, # Where seqmaps are found (if None, GT_FOLDER/dataset_subfolder/seqmaps)
+ 'SEQMAP_FILE': None, # Directly specify seqmap file (if none use SEQMAP_FOLDER/BENCHMARK_SPLIT_TO_EVAL)
+ 'CLSMAP_FOLDER': None, # Where seqmaps are found (if None, GT_FOLDER/dataset_subfolder/clsmaps)
+ 'CLSMAP_FILE': None, # Directly specify seqmap file (if none use CLSMAP_FOLDER/BENCHMARK_SPLIT_TO_EVAL)
+ }
+ return default_config
+
+ def __init__(self, config=None):
+ super().__init__()
+ # Fill non-given config values with defaults
+ self.config = utils.init_config(config, self.get_default_dataset_config())
+
+ self.split = self.config['SPLIT_TO_EVAL']
+ valid_benchmarks = ['mots_challenge', 'kitti_mots', 'bdd_mots', 'davis_unsupervised', 'youtube_vis', 'ovis', 'waymo', 'tao']
+ self.box_gt_benchmarks = ['waymo', 'tao']
+
+ self.sub_benchmark = self.config['SUB_BENCHMARK']
+ if not self.sub_benchmark:
+ raise TrackEvalException('SUB_BENCHMARK config input is required (there is no default value)' +
+ ', '.join(valid_benchmarks) + ' are valid.')
+ if self.sub_benchmark not in valid_benchmarks:
+ raise TrackEvalException('Attempted to evaluate an invalid benchmark: ' + self.sub_benchmark + '. Only benchmarks ' +
+ ', '.join(valid_benchmarks) + ' are valid.')
+
+ self.gt_fol = self.config['GT_FOLDER']
+ self.tracker_fol = os.path.join(self.config['TRACKERS_FOLDER'], self.config['SPLIT_TO_EVAL'])
+ self.data_is_zipped = self.config['INPUT_AS_ZIP']
+
+ self.output_fol = self.config['OUTPUT_FOLDER']
+ if self.output_fol is None:
+ self.output_fol = self.tracker_fol
+
+ self.tracker_sub_fol = self.config['TRACKER_SUB_FOLDER']
+ self.output_sub_fol = os.path.join(self.config['OUTPUT_SUB_FOLDER'], self.sub_benchmark)
+
+ # Loops through all sub-benchmarks, and reads in seqmaps to info on all sequences to eval.
+ self._get_seq_info()
+
+ if len(self.seq_list) < 1:
+ raise TrackEvalException('No sequences are selected to be evaluated.')
+
+ valid_class_ids = np.atleast_1d(np.genfromtxt(os.path.join(self.gt_fol, self.split, self.sub_benchmark,
+ 'clsmap.txt')))
+ valid_classes = [cls_id_to_name[int(x)] for x in valid_class_ids] + ['all']
+ self.valid_class_ids = valid_class_ids
+ self.class_name_to_class_id = {cls_name: cls_id for cls_id, cls_name in cls_id_to_name.items()}
+ self.class_name_to_class_id['all'] = -1
+ if not self.config['CLASSES_TO_EVAL']:
+ self.class_list = valid_classes
+ else:
+ self.class_list = [cls if cls in valid_classes else None
+ for cls in self.config['CLASSES_TO_EVAL']]
+ if not all(self.class_list):
+ raise TrackEvalException('Attempted to evaluate an invalid class. Only classes ' +
+ ', '.join(valid_classes) + ' are valid.')
+
+ # Check gt files exist
+ for seq in self.seq_list:
+ if not self.data_is_zipped:
+ curr_file = os.path.join(self.gt_fol, self.split, self.sub_benchmark, 'data', seq + '.txt')
+ if not os.path.isfile(curr_file):
+ print('GT file not found ' + curr_file)
+ raise TrackEvalException('GT file not found for sequence: ' + seq)
+ if self.data_is_zipped:
+ curr_file = os.path.join(self.gt_fol, self.split, self.sub_benchmark, 'data.zip')
+ if not os.path.isfile(curr_file):
+ raise TrackEvalException('GT file not found: ' + os.path.basename(curr_file))
+
+ # Get trackers to eval
+ if self.config['TRACKERS_TO_EVAL'] is None:
+ self.tracker_list = os.listdir(self.tracker_fol)
+ else:
+ self.tracker_list = self.config['TRACKERS_TO_EVAL']
+
+ if self.config['TRACKER_DISPLAY_NAMES'] is None:
+ self.tracker_to_disp = dict(zip(self.tracker_list, self.tracker_list))
+ elif (self.config['TRACKERS_TO_EVAL'] is not None) and (
+ len(self.config['TRACKER_DISPLAY_NAMES']) == len(self.tracker_list)):
+ self.tracker_to_disp = dict(zip(self.tracker_list, self.config['TRACKER_DISPLAY_NAMES']))
+ else:
+ raise TrackEvalException('List of tracker files and tracker display names do not match.')
+
+ for tracker in self.tracker_list:
+ if self.data_is_zipped:
+ curr_file = os.path.join(self.tracker_fol, tracker, 'data.zip')
+ if not os.path.isfile(curr_file):
+ raise TrackEvalException('Tracker file not found: ' + os.path.basename(curr_file))
+ else:
+ for seq in self.seq_list:
+ curr_file = os.path.join(self.tracker_fol, tracker, self.tracker_sub_fol, self.sub_benchmark, seq
+ + '.txt')
+ if not os.path.isfile(curr_file):
+ print('Tracker file not found: ' + curr_file)
+ raise TrackEvalException(
+ 'Tracker file not found: ' + self.sub_benchmark + '/' + os.path.basename(curr_file))
+
+ def get_name(self):
+ return self.get_class_name() + '.' + self.sub_benchmark
+
+ def _get_seq_info(self):
+ self.seq_list = []
+ self.seq_lengths = {}
+ self.seq_sizes = {}
+ self.seq_ignore_class_ids = {}
+ if self.config["SEQMAP_FILE"]:
+ seqmap_file = self.config["SEQMAP_FILE"]
+ else:
+ if self.config["SEQMAP_FOLDER"] is None:
+ seqmap_file = os.path.join(self.gt_fol, self.split, self.sub_benchmark, 'seqmap.txt')
+ else:
+ seqmap_file = os.path.join(self.config["SEQMAP_FOLDER"], self.split + '.seqmap')
+ if not os.path.isfile(seqmap_file):
+ print('no seqmap found: ' + seqmap_file)
+ raise TrackEvalException('no seqmap found: ' + os.path.basename(seqmap_file))
+ with open(seqmap_file) as fp:
+ dialect = csv.Sniffer().sniff(fp.readline(), delimiters=' ')
+ fp.seek(0)
+ reader = csv.reader(fp, dialect)
+ for i, row in enumerate(reader):
+ if len(row) >= 4:
+ # first col: sequence, second col: sequence length, third and fourth col: sequence height/width
+ # The rest of the columns list the 'sequence ignore class ids' which are classes not penalized as
+ # FPs for this sequence.
+ seq = row[0]
+ self.seq_list.append(seq)
+ self.seq_lengths[seq] = int(row[1])
+ self.seq_sizes[seq] = (int(row[2]), int(row[3]))
+ self.seq_ignore_class_ids[seq] = [int(row[x]) for x in range(4, len(row))]
+
+ def get_display_name(self, tracker):
+ return self.tracker_to_disp[tracker]
+
+ def _load_raw_file(self, tracker, seq, is_gt):
+ """Load a file (gt or tracker) in the unified RobMOTS format.
+
+ If is_gt, this returns a dict which contains the fields:
+ [gt_ids, gt_classes] : list (for each timestep) of 1D NDArrays (for each det).
+ [gt_dets, gt_crowd_ignore_regions]: list (for each timestep) of lists of detections.
+
+ if not is_gt, this returns a dict which contains the fields:
+ [tracker_ids, tracker_classes, tracker_confidences] : list (for each timestep) of 1D NDArrays (for each det).
+ [tracker_dets]: list (for each timestep) of lists of detections.
+ """
+ # import to reduce minimum requirements
+ from pycocotools import mask as mask_utils
+
+ # File location
+ if self.data_is_zipped:
+ if is_gt:
+ zip_file = os.path.join(self.gt_fol, self.split, self.sub_benchmark, 'data.zip')
+ else:
+ zip_file = os.path.join(self.tracker_fol, tracker, 'data.zip')
+ file = seq + '.txt'
+ else:
+ zip_file = None
+ if is_gt:
+ file = os.path.join(self.gt_fol, self.split, self.sub_benchmark, 'data', seq + '.txt')
+ else:
+ file = os.path.join(self.tracker_fol, tracker, self.tracker_sub_fol, self.sub_benchmark, seq + '.txt')
+
+ # Load raw data from text file
+ read_data, ignore_data = self._load_simple_text_file(file, is_zipped=self.data_is_zipped, zip_file=zip_file,
+ force_delimiters=' ')
+
+ # Convert data to required format
+ num_timesteps = self.seq_lengths[seq]
+ data_keys = ['ids', 'classes', 'dets']
+ if not is_gt:
+ data_keys += ['tracker_confidences']
+ raw_data = {key: [None] * num_timesteps for key in data_keys}
+ for t in range(num_timesteps):
+ time_key = str(t)
+ # list to collect all masks of a timestep to check for overlapping areas (for segmentation datasets)
+ all_valid_masks = []
+ if time_key in read_data.keys():
+ try:
+ raw_data['ids'][t] = np.atleast_1d([det[1] for det in read_data[time_key]]).astype(int)
+ raw_data['classes'][t] = np.atleast_1d([det[2] for det in read_data[time_key]]).astype(int)
+ if (not is_gt) or (self.sub_benchmark not in self.box_gt_benchmarks):
+ raw_data['dets'][t] = [{'size': [int(region[4]), int(region[5])],
+ 'counts': region[6].encode(encoding='UTF-8')}
+ for region in read_data[time_key]]
+ all_valid_masks += [mask for mask, cls in zip(raw_data['dets'][t], raw_data['classes'][t]) if
+ cls < 100]
+ else:
+ raw_data['dets'][t] = np.atleast_2d([det[4:8] for det in read_data[time_key]]).astype(float)
+
+ if not is_gt:
+ raw_data['tracker_confidences'][t] = np.atleast_1d([det[3] for det
+ in read_data[time_key]]).astype(float)
+ except IndexError:
+ self._raise_index_error(is_gt, self.sub_benchmark, seq)
+ except ValueError:
+ self._raise_value_error(is_gt, self.sub_benchmark, seq)
+ # no detection in this timestep
+ else:
+ if (not is_gt) or (self.sub_benchmark not in self.box_gt_benchmarks):
+ raw_data['dets'][t] = []
+ else:
+ raw_data['dets'][t] = np.empty((0, 4)).astype(float)
+ raw_data['ids'][t] = np.empty(0).astype(int)
+ raw_data['classes'][t] = np.empty(0).astype(int)
+ if not is_gt:
+ raw_data['tracker_confidences'][t] = np.empty(0).astype(float)
+
+ # check for overlapping masks
+ if all_valid_masks:
+ masks_merged = all_valid_masks[0]
+ for mask in all_valid_masks[1:]:
+ if mask_utils.area(mask_utils.merge([masks_merged, mask], intersect=True)) != 0.0:
+ err = 'Overlapping masks in frame %d' % t
+ raise TrackEvalException(err)
+ masks_merged = mask_utils.merge([masks_merged, mask], intersect=False)
+
+ if is_gt:
+ key_map = {'ids': 'gt_ids',
+ 'classes': 'gt_classes',
+ 'dets': 'gt_dets'}
+ else:
+ key_map = {'ids': 'tracker_ids',
+ 'classes': 'tracker_classes',
+ 'dets': 'tracker_dets'}
+
+ for k, v in key_map.items():
+ raw_data[v] = raw_data.pop(k)
+
+ raw_data['num_timesteps'] = num_timesteps
+ raw_data['frame_size'] = self.seq_sizes[seq]
+ raw_data['seq'] = seq
+ return raw_data
+
+ @staticmethod
+ def _raise_index_error(is_gt, sub_benchmark, seq):
+ """
+ Auxiliary method to raise an evaluation error in case of an index error while reading files.
+ :param is_gt: whether gt or tracker data is read
+ :param tracker: the name of the tracker
+ :param seq: the name of the seq
+ :return: None
+ """
+ if is_gt:
+ err = 'Cannot load gt data from sequence %s, because there are not enough ' \
+ 'columns in the data.' % seq
+ raise TrackEvalException(err)
+ else:
+ err = 'Cannot load tracker data from benchmark %s, sequence %s, because there are not enough ' \
+ 'columns in the data.' % (sub_benchmark, seq)
+ raise TrackEvalException(err)
+
+ @staticmethod
+ def _raise_value_error(is_gt, sub_benchmark, seq):
+ """
+ Auxiliary method to raise an evaluation error in case of an value error while reading files.
+ :param is_gt: whether gt or tracker data is read
+ :param tracker: the name of the tracker
+ :param seq: the name of the seq
+ :return: None
+ """
+ if is_gt:
+ raise TrackEvalException(
+ 'GT data for sequence %s cannot be converted to the right format. Is data corrupted?' % seq)
+ else:
+ raise TrackEvalException(
+ 'Tracking data from benchmark %s, sequence %s cannot be converted to the right format. '
+ 'Is data corrupted?' % (sub_benchmark, seq))
+
+ @_timing.time
+ def get_preprocessed_seq_data(self, raw_data, cls):
+ """ Preprocess data for a single sequence for a single class ready for evaluation.
+ Inputs:
+ - raw_data is a dict containing the data for the sequence already read in by get_raw_seq_data().
+ - cls is the class to be evaluated.
+ Outputs:
+ - data is a dict containing all of the information that metrics need to perform evaluation.
+ It contains the following fields:
+ [num_timesteps, num_gt_ids, num_tracker_ids, num_gt_dets, num_tracker_dets] : integers.
+ [gt_ids, tracker_ids, tracker_confidences]: list (for each timestep) of 1D NDArrays (for each det).
+ [gt_dets, tracker_dets]: list (for each timestep) of lists of detections.
+ [similarity_scores]: list (for each timestep) of 2D NDArrays.
+ Notes:
+ Preprocessing (preproc) occurs in 3 steps.
+ 1) Extract only detections relevant for the class to be evaluated.
+ 2) Match gt dets and tracker dets. Tracker dets that are to a gt det (TPs) are marked as not to be
+ removed.
+ 3) Remove unmatched tracker dets if they fall within an ignore region or are too small, or if that class
+ is marked as an ignore class for that sequence.
+ After the above preprocessing steps, this function also calculates the number of gt and tracker detections
+ and unique track ids. It also relabels gt and tracker ids to be contiguous and checks that ids are
+ unique within each timestep.
+ Note that there is a special 'all' class, which evaluates all of the COCO classes together in a
+ 'class agnostic' fashion.
+ """
+ # import to reduce minimum requirements
+ from pycocotools import mask as mask_utils
+
+ # Check that input data has unique ids
+ self._check_unique_ids(raw_data)
+
+ cls_id = self.class_name_to_class_id[cls]
+ ignore_class_id = cls_id+100
+ seq = raw_data['seq']
+
+ data_keys = ['gt_ids', 'tracker_ids', 'gt_dets', 'tracker_dets', 'tracker_confidences', 'similarity_scores']
+ data = {key: [None] * raw_data['num_timesteps'] for key in data_keys}
+ unique_gt_ids = []
+ unique_tracker_ids = []
+ num_gt_dets = 0
+ num_tracker_dets = 0
+
+ for t in range(raw_data['num_timesteps']):
+
+ # Only extract relevant dets for this class
+ if cls == 'all':
+ gt_class_mask = raw_data['gt_classes'][t] < 100
+ # For waymo, combine predictions for [car, truck, bus, motorcycle] into car, because they are all annotated
+ # together as one 'vehicle' class.
+ elif self.sub_benchmark == 'waymo' and cls == 'car':
+ waymo_vehicle_classes = np.array([3, 4, 6, 8])
+ gt_class_mask = np.isin(raw_data['gt_classes'][t], waymo_vehicle_classes)
+ else:
+ gt_class_mask = raw_data['gt_classes'][t] == cls_id
+ gt_class_mask = gt_class_mask.astype(np.bool)
+ gt_ids = raw_data['gt_ids'][t][gt_class_mask]
+ if cls == 'all':
+ ignore_regions_mask = raw_data['gt_classes'][t] >= 100
+ else:
+ ignore_regions_mask = raw_data['gt_classes'][t] == ignore_class_id
+ ignore_regions_mask = np.logical_or(ignore_regions_mask, raw_data['gt_classes'][t] == 100)
+ if self.sub_benchmark in self.box_gt_benchmarks:
+ gt_dets = raw_data['gt_dets'][t][gt_class_mask]
+ ignore_regions_box = raw_data['gt_dets'][t][ignore_regions_mask]
+ if len(ignore_regions_box) > 0:
+ ignore_regions_box[:, 2] = ignore_regions_box[:, 2] - ignore_regions_box[:, 0]
+ ignore_regions_box[:, 3] = ignore_regions_box[:, 3] - ignore_regions_box[:, 1]
+ ignore_regions = mask_utils.frPyObjects(ignore_regions_box, self.seq_sizes[seq][0], self.seq_sizes[seq][1])
+ else:
+ ignore_regions = []
+ else:
+ gt_dets = [raw_data['gt_dets'][t][ind] for ind in range(len(gt_class_mask)) if gt_class_mask[ind]]
+ ignore_regions = [raw_data['gt_dets'][t][ind] for ind in range(len(ignore_regions_mask)) if
+ ignore_regions_mask[ind]]
+
+ if cls == 'all':
+ tracker_class_mask = np.ones_like(raw_data['tracker_classes'][t])
+ else:
+ tracker_class_mask = np.atleast_1d(raw_data['tracker_classes'][t] == cls_id)
+ tracker_class_mask = tracker_class_mask.astype(np.bool)
+ tracker_ids = raw_data['tracker_ids'][t][tracker_class_mask]
+ tracker_dets = [raw_data['tracker_dets'][t][ind] for ind in range(len(tracker_class_mask)) if
+ tracker_class_mask[ind]]
+ tracker_confidences = raw_data['tracker_confidences'][t][tracker_class_mask]
+ similarity_scores = raw_data['similarity_scores'][t][gt_class_mask, :][:, tracker_class_mask]
+ tracker_classes = raw_data['tracker_classes'][t][tracker_class_mask]
+
+ # Only do preproc if there are ignore regions defined to remove
+ if tracker_ids.shape[0] > 0:
+
+ # Match tracker and gt dets (with hungarian algorithm)
+ unmatched_indices = np.arange(tracker_ids.shape[0])
+ if gt_ids.shape[0] > 0 and tracker_ids.shape[0] > 0:
+ matching_scores = similarity_scores.copy()
+ matching_scores[matching_scores < 0.5 - np.finfo('float').eps] = 0
+ match_rows, match_cols = linear_sum_assignment(-matching_scores)
+ actually_matched_mask = matching_scores[match_rows, match_cols] > 0 + np.finfo('float').eps
+ # match_rows = match_rows[actually_matched_mask]
+ match_cols = match_cols[actually_matched_mask]
+ unmatched_indices = np.delete(unmatched_indices, match_cols, axis=0)
+
+ # For unmatched tracker dets remove those that are greater than 50% within an ignore region.
+ # unmatched_tracker_dets = tracker_dets[unmatched_indices, :]
+ # crowd_ignore_regions = raw_data['gt_ignore_regions'][t]
+ # intersection_with_ignore_region = self. \
+ # _calculate_box_ious(unmatched_tracker_dets, crowd_ignore_regions, box_format='x0y0x1y1',
+ # do_ioa=True)
+
+
+ if cls_id in self.seq_ignore_class_ids[seq]:
+ # Remove unmatched detections for classes that are marked as 'ignore' for the whole sequence.
+ to_remove_tracker = unmatched_indices
+ else:
+ unmatched_tracker_dets = [tracker_dets[i] for i in range(len(tracker_dets)) if
+ i in unmatched_indices]
+
+ # For unmatched tracker dets remove those that are too small.
+ tracker_boxes_t = mask_utils.toBbox(unmatched_tracker_dets)
+ unmatched_widths = tracker_boxes_t[:, 2]
+ unmatched_heights = tracker_boxes_t[:, 3]
+ unmatched_size = np.maximum(unmatched_heights, unmatched_widths)
+ min_size = np.min(self.seq_sizes[seq])/8
+ is_too_small = unmatched_size <= min_size + np.finfo('float').eps
+
+ # For unmatched tracker dets remove those that are greater than 50% within an ignore region.
+ if ignore_regions:
+ ignore_region_merged = ignore_regions[0]
+ for mask in ignore_regions[1:]:
+ ignore_region_merged = mask_utils.merge([ignore_region_merged, mask], intersect=False)
+ intersection_with_ignore_region = self. \
+ _calculate_mask_ious(unmatched_tracker_dets, [ignore_region_merged], is_encoded=True, do_ioa=True)
+ is_within_ignore_region = np.any(intersection_with_ignore_region > 0.5 + np.finfo('float').eps, axis=1)
+ to_remove_tracker = unmatched_indices[np.logical_or(is_too_small, is_within_ignore_region)]
+ else:
+ to_remove_tracker = unmatched_indices[is_too_small]
+
+ # For the special 'all' class, you need to remove unmatched detections from all ignore classes and
+ # non-evaluated classes.
+ if cls == 'all':
+ unmatched_tracker_classes = [tracker_classes[i] for i in range(len(tracker_classes)) if
+ i in unmatched_indices]
+ is_ignore_class = np.isin(unmatched_tracker_classes, self.seq_ignore_class_ids[seq])
+ is_not_evaled_class = np.logical_not(np.isin(unmatched_tracker_classes, self.valid_class_ids))
+ to_remove_all = unmatched_indices[np.logical_or(is_ignore_class, is_not_evaled_class)]
+ to_remove_tracker = np.concatenate([to_remove_tracker, to_remove_all], axis=0)
+ else:
+ to_remove_tracker = np.array([], dtype=np.int)
+
+ # remove all unwanted tracker detections
+ data['tracker_ids'][t] = np.delete(tracker_ids, to_remove_tracker, axis=0)
+ data['tracker_dets'][t] = np.delete(tracker_dets, to_remove_tracker, axis=0)
+ data['tracker_confidences'][t] = np.delete(tracker_confidences, to_remove_tracker, axis=0)
+ similarity_scores = np.delete(similarity_scores, to_remove_tracker, axis=1)
+
+ # keep all ground truth detections
+ data['gt_ids'][t] = gt_ids
+ data['gt_dets'][t] = gt_dets
+ data['similarity_scores'][t] = similarity_scores
+
+ unique_gt_ids += list(np.unique(data['gt_ids'][t]))
+ unique_tracker_ids += list(np.unique(data['tracker_ids'][t]))
+ num_tracker_dets += len(data['tracker_ids'][t])
+ num_gt_dets += len(data['gt_ids'][t])
+
+ # Re-label IDs such that there are no empty IDs
+ if len(unique_gt_ids) > 0:
+ unique_gt_ids = np.unique(unique_gt_ids)
+ gt_id_map = np.nan * np.ones((np.max(unique_gt_ids) + 1))
+ gt_id_map[unique_gt_ids] = np.arange(len(unique_gt_ids))
+ for t in range(raw_data['num_timesteps']):
+ if len(data['gt_ids'][t]) > 0:
+ data['gt_ids'][t] = gt_id_map[data['gt_ids'][t]].astype(np.int)
+ if len(unique_tracker_ids) > 0:
+ unique_tracker_ids = np.unique(unique_tracker_ids)
+ tracker_id_map = np.nan * np.ones((np.max(unique_tracker_ids) + 1))
+ tracker_id_map[unique_tracker_ids] = np.arange(len(unique_tracker_ids))
+ for t in range(raw_data['num_timesteps']):
+ if len(data['tracker_ids'][t]) > 0:
+ data['tracker_ids'][t] = tracker_id_map[data['tracker_ids'][t]].astype(np.int)
+
+ # Record overview statistics.
+ data['num_tracker_dets'] = num_tracker_dets
+ data['num_gt_dets'] = num_gt_dets
+ data['num_tracker_ids'] = len(unique_tracker_ids)
+ data['num_gt_ids'] = len(unique_gt_ids)
+ data['num_timesteps'] = raw_data['num_timesteps']
+ data['seq'] = raw_data['seq']
+ data['frame_size'] = raw_data['frame_size']
+
+ # Ensure that ids are unique per timestep.
+ self._check_unique_ids(data, after_preproc=True)
+
+ return data
+
+ def _calculate_similarities(self, gt_dets_t, tracker_dets_t):
+
+ # Only loaded when run to reduce minimum requirements
+ from pycocotools import mask as mask_utils
+
+ if self.sub_benchmark in self.box_gt_benchmarks:
+ # Convert tracker masks to bboxes (for benchmarks with only bbox ground-truth),
+ # and then convert to x0y0x1y1 format.
+ tracker_boxes_t = mask_utils.toBbox(tracker_dets_t)
+ tracker_boxes_t[:, 2] = tracker_boxes_t[:, 0] + tracker_boxes_t[:, 2]
+ tracker_boxes_t[:, 3] = tracker_boxes_t[:, 1] + tracker_boxes_t[:, 3]
+ similarity_scores = self._calculate_box_ious(gt_dets_t, tracker_boxes_t, box_format='x0y0x1y1')
+ else:
+ similarity_scores = self._calculate_mask_ious(gt_dets_t, tracker_dets_t, is_encoded=True, do_ioa=False)
+ return similarity_scores
diff --git a/val_utils/trackeval/datasets/rob_mots_classmap.py b/val_utils/trackeval/datasets/rob_mots_classmap.py
new file mode 100644
index 0000000000000000000000000000000000000000..1b3644d0b6dc28d3a088f1cadfe71e1b1cb970f6
--- /dev/null
+++ b/val_utils/trackeval/datasets/rob_mots_classmap.py
@@ -0,0 +1,81 @@
+cls_id_to_name = {
+ 1: 'person',
+ 2: 'bicycle',
+ 3: 'car',
+ 4: 'motorcycle',
+ 5: 'airplane',
+ 6: 'bus',
+ 7: 'train',
+ 8: 'truck',
+ 9: 'boat',
+ 10: 'traffic light',
+ 11: 'fire hydrant',
+ 12: 'stop sign',
+ 13: 'parking meter',
+ 14: 'bench',
+ 15: 'bird',
+ 16: 'cat',
+ 17: 'dog',
+ 18: 'horse',
+ 19: 'sheep',
+ 20: 'cow',
+ 21: 'elephant',
+ 22: 'bear',
+ 23: 'zebra',
+ 24: 'giraffe',
+ 25: 'backpack',
+ 26: 'umbrella',
+ 27: 'handbag',
+ 28: 'tie',
+ 29: 'suitcase',
+ 30: 'frisbee',
+ 31: 'skis',
+ 32: 'snowboard',
+ 33: 'sports ball',
+ 34: 'kite',
+ 35: 'baseball bat',
+ 36: 'baseball glove',
+ 37: 'skateboard',
+ 38: 'surfboard',
+ 39: 'tennis racket',
+ 40: 'bottle',
+ 41: 'wine glass',
+ 42: 'cup',
+ 43: 'fork',
+ 44: 'knife',
+ 45: 'spoon',
+ 46: 'bowl',
+ 47: 'banana',
+ 48: 'apple',
+ 49: 'sandwich',
+ 50: 'orange',
+ 51: 'broccoli',
+ 52: 'carrot',
+ 53: 'hot dog',
+ 54: 'pizza',
+ 55: 'donut',
+ 56: 'cake',
+ 57: 'chair',
+ 58: 'couch',
+ 59: 'potted plant',
+ 60: 'bed',
+ 61: 'dining table',
+ 62: 'toilet',
+ 63: 'tv',
+ 64: 'laptop',
+ 65: 'mouse',
+ 66: 'remote',
+ 67: 'keyboard',
+ 68: 'cell phone',
+ 69: 'microwave',
+ 70: 'oven',
+ 71: 'toaster',
+ 72: 'sink',
+ 73: 'refrigerator',
+ 74: 'book',
+ 75: 'clock',
+ 76: 'vase',
+ 77: 'scissors',
+ 78: 'teddy bear',
+ 79: 'hair drier',
+ 80: 'toothbrush'}
\ No newline at end of file
diff --git a/val_utils/trackeval/datasets/run_rob_mots.py b/val_utils/trackeval/datasets/run_rob_mots.py
new file mode 100644
index 0000000000000000000000000000000000000000..87c141238d830003f40d7637819c2394bb874c38
--- /dev/null
+++ b/val_utils/trackeval/datasets/run_rob_mots.py
@@ -0,0 +1,113 @@
+
+# python3 scripts\run_rob_mots.py --ROBMOTS_SPLIT val --TRACKERS_TO_EVAL tracker_name (e.g. STP) --USE_PARALLEL True --NUM_PARALLEL_CORES 4
+
+import sys
+import os
+import csv
+import numpy as np
+from multiprocessing import freeze_support
+
+sys.path.insert(0, os.path.abspath(os.path.join(os.path.dirname(__file__), '..')))
+import trackeval # noqa: E402
+from trackeval import utils
+code_path = utils.get_code_path()
+
+if __name__ == '__main__':
+ freeze_support()
+
+ script_config = {
+ 'ROBMOTS_SPLIT': 'train', # 'train', # valid: 'train', 'val', 'test', 'test_live', 'test_post', 'test_all'
+ 'BENCHMARKS': ['kitti_mots', 'davis_unsupervised', 'youtube_vis', 'ovis', 'tao'], # 'bdd_mots' coming soon
+ 'GT_FOLDER': os.path.join(code_path, 'data/gt/rob_mots'),
+ 'TRACKERS_FOLDER': os.path.join(code_path, 'data/trackers/rob_mots'),
+ }
+
+ default_eval_config = trackeval.Evaluator.get_default_eval_config()
+ default_eval_config['PRINT_ONLY_COMBINED'] = True
+ default_eval_config['DISPLAY_LESS_PROGRESS'] = True
+ default_dataset_config = trackeval.datasets.RobMOTS.get_default_dataset_config()
+ config = {**default_eval_config, **default_dataset_config, **script_config}
+
+ # Command line interface:
+ config = utils.update_config(config)
+
+ if config['ROBMOTS_SPLIT'] == 'val':
+ config['BENCHMARKS'] = ['kitti_mots', 'bdd_mots', 'davis_unsupervised', 'youtube_vis', 'ovis',
+ 'tao', 'mots_challenge']
+ config['SPLIT_TO_EVAL'] = 'val'
+ elif config['ROBMOTS_SPLIT'] == 'test' or config['SPLIT_TO_EVAL'] == 'test_live':
+ config['BENCHMARKS'] = ['kitti_mots', 'bdd_mots', 'davis_unsupervised', 'youtube_vis', 'ovis', 'tao']
+ config['SPLIT_TO_EVAL'] = 'test'
+ elif config['ROBMOTS_SPLIT'] == 'test_post':
+ config['BENCHMARKS'] = ['mots_challenge', 'waymo']
+ config['SPLIT_TO_EVAL'] = 'test'
+ elif config['ROBMOTS_SPLIT'] == 'test_all':
+ config['BENCHMARKS'] = ['kitti_mots', 'bdd_mots', 'davis_unsupervised', 'youtube_vis', 'ovis',
+ 'tao', 'mots_challenge', 'waymo']
+ config['SPLIT_TO_EVAL'] = 'test'
+ elif config['ROBMOTS_SPLIT'] == 'train':
+ config['BENCHMARKS'] = ['kitti_mots', 'davis_unsupervised', 'youtube_vis', 'ovis', 'tao'] # 'bdd_mots' coming soon
+ config['SPLIT_TO_EVAL'] = 'train'
+
+ metrics_config = {'METRICS': ['HOTA']}
+ # metrics_config = {'METRICS': ['HOTA', 'CLEAR', 'Identity']}
+ eval_config = {k: v for k, v in config.items() if k in config.keys()}
+ dataset_config = {k: v for k, v in config.items() if k in config.keys()}
+
+ # Run code
+ dataset_list = []
+ for bench in config['BENCHMARKS']:
+ dataset_config['SUB_BENCHMARK'] = bench
+ dataset_list.append(trackeval.datasets.RobMOTS(dataset_config))
+ evaluator = trackeval.Evaluator(eval_config)
+ metrics_list = []
+ for metric in [trackeval.metrics.HOTA, trackeval.metrics.CLEAR, trackeval.metrics.Identity]:
+ if metric.get_name() in metrics_config['METRICS']:
+ metrics_list.append(metric())
+ if len(metrics_list) == 0:
+ raise Exception('No metrics selected for evaluation')
+ output_res, output_msg = evaluator.evaluate(dataset_list, metrics_list)
+
+
+ # For each benchmark, combine the 'all' score with the 'cls_averaged' using geometric mean.
+ metrics_to_calc = ['HOTA', 'DetA', 'AssA', 'DetRe', 'DetPr', 'AssRe', 'AssPr', 'LocA']
+ trackers = list(output_res['RobMOTS.' + config['BENCHMARKS'][0]].keys())
+ for tracker in trackers:
+ # final_results[benchmark][result_type][metric]
+ final_results = {}
+ res = {bench: output_res['RobMOTS.' + bench][tracker]['COMBINED_SEQ'] for bench in config['BENCHMARKS']}
+ for bench in config['BENCHMARKS']:
+ final_results[bench] = {'cls_av': {}, 'det_av': {}, 'final': {}}
+ for metric in metrics_to_calc:
+ final_results[bench]['cls_av'][metric] = np.mean(res[bench]['cls_comb_cls_av']['HOTA'][metric])
+ final_results[bench]['det_av'][metric] = np.mean(res[bench]['all']['HOTA'][metric])
+ final_results[bench]['final'][metric] = \
+ np.sqrt(final_results[bench]['cls_av'][metric] * final_results[bench]['det_av'][metric])
+
+ # Take the arithmetic mean over all the benchmarks
+ final_results['overall'] = {'cls_av': {}, 'det_av': {}, 'final': {}}
+ for metric in metrics_to_calc:
+ final_results['overall']['cls_av'][metric] = \
+ np.mean([final_results[bench]['cls_av'][metric] for bench in config['BENCHMARKS']])
+ final_results['overall']['det_av'][metric] = \
+ np.mean([final_results[bench]['det_av'][metric] for bench in config['BENCHMARKS']])
+ final_results['overall']['final'][metric] = \
+ np.mean([final_results[bench]['final'][metric] for bench in config['BENCHMARKS']])
+
+ # Save out result
+ headers = [config['SPLIT_TO_EVAL']] + [x + '___' + metric for x in ['f', 'c', 'd'] for metric in metrics_to_calc]
+
+ def rowify(d):
+ return [d[x][metric] for x in ['final', 'cls_av', 'det_av'] for metric in metrics_to_calc]
+
+ out_file = os.path.join(script_config['TRACKERS_FOLDER'], script_config['ROBMOTS_SPLIT'], tracker,
+ 'final_results.csv')
+
+ with open(out_file, 'w', newline='') as f:
+ writer = csv.writer(f, delimiter=',')
+ writer.writerow(headers)
+ writer.writerow(['overall'] + rowify(final_results['overall']))
+ for bench in config['BENCHMARKS']:
+ if bench == 'overall':
+ continue
+ writer.writerow([bench] + rowify(final_results[bench]))
diff --git a/val_utils/trackeval/datasets/tao.py b/val_utils/trackeval/datasets/tao.py
new file mode 100644
index 0000000000000000000000000000000000000000..e8461676a82d8c8aaac071ae5f9a8b5db2723049
--- /dev/null
+++ b/val_utils/trackeval/datasets/tao.py
@@ -0,0 +1,566 @@
+import os
+import numpy as np
+import json
+import itertools
+from collections import defaultdict
+from scipy.optimize import linear_sum_assignment
+from ..utils import TrackEvalException
+from ._base_dataset import _BaseDataset
+from .. import utils
+from .. import _timing
+
+
+class TAO(_BaseDataset):
+ """Dataset class for TAO tracking"""
+
+ @staticmethod
+ def get_default_dataset_config():
+ """Default class config values"""
+ code_path = utils.get_code_path()
+ default_config = {
+ 'GT_FOLDER': os.path.join(code_path, 'data/gt/tao/tao_training'), # Location of GT data
+ 'TRACKERS_FOLDER': os.path.join(code_path, 'data/trackers/tao/tao_training'), # Trackers location
+ 'OUTPUT_FOLDER': None, # Where to save eval results (if None, same as TRACKERS_FOLDER)
+ 'TRACKERS_TO_EVAL': None, # Filenames of trackers to eval (if None, all in folder)
+ 'CLASSES_TO_EVAL': None, # Classes to eval (if None, all classes)
+ 'SPLIT_TO_EVAL': 'training', # Valid: 'training', 'val'
+ 'PRINT_CONFIG': True, # Whether to print current config
+ 'TRACKER_SUB_FOLDER': 'data', # Tracker files are in TRACKER_FOLDER/tracker_name/TRACKER_SUB_FOLDER
+ 'OUTPUT_SUB_FOLDER': '', # Output files are saved in OUTPUT_FOLDER/tracker_name/OUTPUT_SUB_FOLDER
+ 'TRACKER_DISPLAY_NAMES': None, # Names of trackers to display, if None: TRACKERS_TO_EVAL
+ 'MAX_DETECTIONS': 300, # Number of maximal allowed detections per image (0 for unlimited)
+ }
+ return default_config
+
+ def __init__(self, config=None):
+ """Initialise dataset, checking that all required files are present"""
+ super().__init__()
+ # Fill non-given config values with defaults
+ self.config = utils.init_config(config, self.get_default_dataset_config(), self.get_name())
+ self.gt_fol = self.config['GT_FOLDER']
+ self.tracker_fol = self.config['TRACKERS_FOLDER']
+ self.should_classes_combine = True
+ self.use_super_categories = False
+
+ self.tracker_sub_fol = self.config['TRACKER_SUB_FOLDER']
+ self.output_fol = self.config['OUTPUT_FOLDER']
+ if self.output_fol is None:
+ self.output_fol = self.tracker_fol
+ self.output_sub_fol = self.config['OUTPUT_SUB_FOLDER']
+
+ gt_dir_files = [file for file in os.listdir(self.gt_fol) if file.endswith('.json')]
+ if len(gt_dir_files) != 1:
+ raise TrackEvalException(self.gt_fol + ' does not contain exactly one json file.')
+
+ with open(os.path.join(self.gt_fol, gt_dir_files[0])) as f:
+ self.gt_data = json.load(f)
+
+ # merge categories marked with a merged tag in TAO dataset
+ self._merge_categories(self.gt_data['annotations'] + self.gt_data['tracks'])
+
+ # Get sequences to eval and sequence information
+ self.seq_list = [vid['name'].replace('/', '-') for vid in self.gt_data['videos']]
+ self.seq_name_to_seq_id = {vid['name'].replace('/', '-'): vid['id'] for vid in self.gt_data['videos']}
+ # compute mappings from videos to annotation data
+ self.videos_to_gt_tracks, self.videos_to_gt_images = self._compute_vid_mappings(self.gt_data['annotations'])
+ # compute sequence lengths
+ self.seq_lengths = {vid['id']: 0 for vid in self.gt_data['videos']}
+ for img in self.gt_data['images']:
+ self.seq_lengths[img['video_id']] += 1
+ self.seq_to_images_to_timestep = self._compute_image_to_timestep_mappings()
+ self.seq_to_classes = {vid['id']: {'pos_cat_ids': list({track['category_id'] for track
+ in self.videos_to_gt_tracks[vid['id']]}),
+ 'neg_cat_ids': vid['neg_category_ids'],
+ 'not_exhaustively_labeled_cat_ids': vid['not_exhaustive_category_ids']}
+ for vid in self.gt_data['videos']}
+
+ # Get classes to eval
+ considered_vid_ids = [self.seq_name_to_seq_id[vid] for vid in self.seq_list]
+ seen_cats = set([cat_id for vid_id in considered_vid_ids for cat_id
+ in self.seq_to_classes[vid_id]['pos_cat_ids']])
+ # only classes with ground truth are evaluated in TAO
+ self.valid_classes = [cls['name'] for cls in self.gt_data['categories'] if cls['id'] in seen_cats]
+ cls_name_to_cls_id_map = {cls['name']: cls['id'] for cls in self.gt_data['categories']}
+
+ if self.config['CLASSES_TO_EVAL']:
+ self.class_list = [cls.lower() if cls.lower() in self.valid_classes else None
+ for cls in self.config['CLASSES_TO_EVAL']]
+ if not all(self.class_list):
+ raise TrackEvalException('Attempted to evaluate an invalid class. Only classes ' +
+ ', '.join(self.valid_classes) +
+ ' are valid (classes present in ground truth data).')
+ else:
+ self.class_list = [cls for cls in self.valid_classes]
+ self.class_name_to_class_id = {k: v for k, v in cls_name_to_cls_id_map.items() if k in self.class_list}
+
+ # Get trackers to eval
+ if self.config['TRACKERS_TO_EVAL'] is None:
+ self.tracker_list = os.listdir(self.tracker_fol)
+ else:
+ self.tracker_list = self.config['TRACKERS_TO_EVAL']
+
+ if self.config['TRACKER_DISPLAY_NAMES'] is None:
+ self.tracker_to_disp = dict(zip(self.tracker_list, self.tracker_list))
+ elif (self.config['TRACKERS_TO_EVAL'] is not None) and (
+ len(self.config['TRACKER_DISPLAY_NAMES']) == len(self.tracker_list)):
+ self.tracker_to_disp = dict(zip(self.tracker_list, self.config['TRACKER_DISPLAY_NAMES']))
+ else:
+ raise TrackEvalException('List of tracker files and tracker display names do not match.')
+
+ self.tracker_data = {tracker: dict() for tracker in self.tracker_list}
+
+ for tracker in self.tracker_list:
+ tr_dir_files = [file for file in os.listdir(os.path.join(self.tracker_fol, tracker, self.tracker_sub_fol))
+ if file.endswith('.json')]
+ if len(tr_dir_files) != 1:
+ raise TrackEvalException(os.path.join(self.tracker_fol, tracker, self.tracker_sub_fol)
+ + ' does not contain exactly one json file.')
+ with open(os.path.join(self.tracker_fol, tracker, self.tracker_sub_fol, tr_dir_files[0])) as f:
+ curr_data = json.load(f)
+
+ # limit detections if MAX_DETECTIONS > 0
+ if self.config['MAX_DETECTIONS']:
+ curr_data = self._limit_dets_per_image(curr_data)
+
+ # fill missing video ids
+ self._fill_video_ids_inplace(curr_data)
+
+ # make track ids unique over whole evaluation set
+ self._make_track_ids_unique(curr_data)
+
+ # merge categories marked with a merged tag in TAO dataset
+ self._merge_categories(curr_data)
+
+ # get tracker sequence information
+ curr_videos_to_tracker_tracks, curr_videos_to_tracker_images = self._compute_vid_mappings(curr_data)
+ self.tracker_data[tracker]['vids_to_tracks'] = curr_videos_to_tracker_tracks
+ self.tracker_data[tracker]['vids_to_images'] = curr_videos_to_tracker_images
+
+ def get_display_name(self, tracker):
+ return self.tracker_to_disp[tracker]
+
+ def _load_raw_file(self, tracker, seq, is_gt):
+ """Load a file (gt or tracker) in the TAO format
+
+ If is_gt, this returns a dict which contains the fields:
+ [gt_ids, gt_classes] : list (for each timestep) of 1D NDArrays (for each det).
+ [gt_dets]: list (for each timestep) of lists of detections.
+ [classes_to_gt_tracks]: dictionary with class values as keys and list of dictionaries (with frame indices as
+ keys and corresponding segmentations as values) for each track
+ [classes_to_gt_track_ids, classes_to_gt_track_areas, classes_to_gt_track_lengths]: dictionary with class values
+ as keys and lists (for each track) as values
+
+ if not is_gt, this returns a dict which contains the fields:
+ [tracker_ids, tracker_classes, tracker_confidences] : list (for each timestep) of 1D NDArrays (for each det).
+ [tracker_dets]: list (for each timestep) of lists of detections.
+ [classes_to_dt_tracks]: dictionary with class values as keys and list of dictionaries (with frame indices as
+ keys and corresponding segmentations as values) for each track
+ [classes_to_dt_track_ids, classes_to_dt_track_areas, classes_to_dt_track_lengths]: dictionary with class values
+ as keys and lists as values
+ [classes_to_dt_track_scores]: dictionary with class values as keys and 1D numpy arrays as values
+ """
+ seq_id = self.seq_name_to_seq_id[seq]
+ # File location
+ if is_gt:
+ imgs = self.videos_to_gt_images[seq_id]
+ else:
+ imgs = self.tracker_data[tracker]['vids_to_images'][seq_id]
+
+ # Convert data to required format
+ num_timesteps = self.seq_lengths[seq_id]
+ img_to_timestep = self.seq_to_images_to_timestep[seq_id]
+ data_keys = ['ids', 'classes', 'dets']
+ if not is_gt:
+ data_keys += ['tracker_confidences']
+ raw_data = {key: [None] * num_timesteps for key in data_keys}
+ for img in imgs:
+ # some tracker data contains images without any ground truth information, these are ignored
+ try:
+ t = img_to_timestep[img['id']]
+ except KeyError:
+ continue
+ annotations = img['annotations']
+ raw_data['dets'][t] = np.atleast_2d([ann['bbox'] for ann in annotations]).astype(float)
+ raw_data['ids'][t] = np.atleast_1d([ann['track_id'] for ann in annotations]).astype(int)
+ raw_data['classes'][t] = np.atleast_1d([ann['category_id'] for ann in annotations]).astype(int)
+ if not is_gt:
+ raw_data['tracker_confidences'][t] = np.atleast_1d([ann['score'] for ann in annotations]).astype(float)
+
+ for t, d in enumerate(raw_data['dets']):
+ if d is None:
+ raw_data['dets'][t] = np.empty((0, 4)).astype(float)
+ raw_data['ids'][t] = np.empty(0).astype(int)
+ raw_data['classes'][t] = np.empty(0).astype(int)
+ if not is_gt:
+ raw_data['tracker_confidences'][t] = np.empty(0)
+
+ if is_gt:
+ key_map = {'ids': 'gt_ids',
+ 'classes': 'gt_classes',
+ 'dets': 'gt_dets'}
+ else:
+ key_map = {'ids': 'tracker_ids',
+ 'classes': 'tracker_classes',
+ 'dets': 'tracker_dets'}
+ for k, v in key_map.items():
+ raw_data[v] = raw_data.pop(k)
+
+ all_classes = [self.class_name_to_class_id[cls] for cls in self.class_list]
+ if is_gt:
+ classes_to_consider = all_classes
+ all_tracks = self.videos_to_gt_tracks[seq_id]
+ else:
+ classes_to_consider = self.seq_to_classes[seq_id]['pos_cat_ids'] \
+ + self.seq_to_classes[seq_id]['neg_cat_ids']
+ all_tracks = self.tracker_data[tracker]['vids_to_tracks'][seq_id]
+
+ classes_to_tracks = {cls: [track for track in all_tracks if track['category_id'] == cls]
+ if cls in classes_to_consider else [] for cls in all_classes}
+
+ # mapping from classes to track information
+ raw_data['classes_to_tracks'] = {cls: [{det['image_id']: np.atleast_1d(det['bbox'])
+ for det in track['annotations']} for track in tracks]
+ for cls, tracks in classes_to_tracks.items()}
+ raw_data['classes_to_track_ids'] = {cls: [track['id'] for track in tracks]
+ for cls, tracks in classes_to_tracks.items()}
+ raw_data['classes_to_track_areas'] = {cls: [track['area'] for track in tracks]
+ for cls, tracks in classes_to_tracks.items()}
+ raw_data['classes_to_track_lengths'] = {cls: [len(track['annotations']) for track in tracks]
+ for cls, tracks in classes_to_tracks.items()}
+
+ if not is_gt:
+ raw_data['classes_to_dt_track_scores'] = {cls: np.array([np.mean([float(x['score'])
+ for x in track['annotations']])
+ for track in tracks])
+ for cls, tracks in classes_to_tracks.items()}
+
+ if is_gt:
+ key_map = {'classes_to_tracks': 'classes_to_gt_tracks',
+ 'classes_to_track_ids': 'classes_to_gt_track_ids',
+ 'classes_to_track_lengths': 'classes_to_gt_track_lengths',
+ 'classes_to_track_areas': 'classes_to_gt_track_areas'}
+ else:
+ key_map = {'classes_to_tracks': 'classes_to_dt_tracks',
+ 'classes_to_track_ids': 'classes_to_dt_track_ids',
+ 'classes_to_track_lengths': 'classes_to_dt_track_lengths',
+ 'classes_to_track_areas': 'classes_to_dt_track_areas'}
+ for k, v in key_map.items():
+ raw_data[v] = raw_data.pop(k)
+
+ raw_data['num_timesteps'] = num_timesteps
+ raw_data['neg_cat_ids'] = self.seq_to_classes[seq_id]['neg_cat_ids']
+ raw_data['not_exhaustively_labeled_cls'] = self.seq_to_classes[seq_id]['not_exhaustively_labeled_cat_ids']
+ raw_data['seq'] = seq
+ return raw_data
+
+ @_timing.time
+ def get_preprocessed_seq_data(self, raw_data, cls):
+ """ Preprocess data for a single sequence for a single class ready for evaluation.
+ Inputs:
+ - raw_data is a dict containing the data for the sequence already read in by get_raw_seq_data().
+ - cls is the class to be evaluated.
+ Outputs:
+ - data is a dict containing all of the information that metrics need to perform evaluation.
+ It contains the following fields:
+ [num_timesteps, num_gt_ids, num_tracker_ids, num_gt_dets, num_tracker_dets] : integers.
+ [gt_ids, tracker_ids, tracker_confidences]: list (for each timestep) of 1D NDArrays (for each det).
+ [gt_dets, tracker_dets]: list (for each timestep) of lists of detections.
+ [similarity_scores]: list (for each timestep) of 2D NDArrays.
+ Notes:
+ General preprocessing (preproc) occurs in 4 steps. Some datasets may not use all of these steps.
+ 1) Extract only detections relevant for the class to be evaluated (including distractor detections).
+ 2) Match gt dets and tracker dets. Remove tracker dets that are matched to a gt det that is of a
+ distractor class, or otherwise marked as to be removed.
+ 3) Remove unmatched tracker dets if they fall within a crowd ignore region or don't meet a certain
+ other criteria (e.g. are too small).
+ 4) Remove gt dets that were only useful for preprocessing and not for actual evaluation.
+ After the above preprocessing steps, this function also calculates the number of gt and tracker detections
+ and unique track ids. It also relabels gt and tracker ids to be contiguous and checks that ids are
+ unique within each timestep.
+ TAO:
+ In TAO, the 4 preproc steps are as follow:
+ 1) All classes present in the ground truth data are evaluated separately.
+ 2) No matched tracker detections are removed.
+ 3) Unmatched tracker detections are removed if there is not ground truth data and the class does not
+ belong to the categories marked as negative for this sequence. Additionally, unmatched tracker
+ detections for classes which are marked as not exhaustively labeled are removed.
+ 4) No gt detections are removed.
+ Further, for TrackMAP computation track representations for the given class are accessed from a dictionary
+ and the tracks from the tracker data are sorted according to the tracker confidence.
+ """
+ cls_id = self.class_name_to_class_id[cls]
+ is_not_exhaustively_labeled = cls_id in raw_data['not_exhaustively_labeled_cls']
+ is_neg_category = cls_id in raw_data['neg_cat_ids']
+
+ data_keys = ['gt_ids', 'tracker_ids', 'gt_dets', 'tracker_dets', 'tracker_confidences', 'similarity_scores']
+ data = {key: [None] * raw_data['num_timesteps'] for key in data_keys}
+ unique_gt_ids = []
+ unique_tracker_ids = []
+ num_gt_dets = 0
+ num_tracker_dets = 0
+ for t in range(raw_data['num_timesteps']):
+
+ # Only extract relevant dets for this class for preproc and eval (cls)
+ gt_class_mask = np.atleast_1d(raw_data['gt_classes'][t] == cls_id)
+ gt_class_mask = gt_class_mask.astype(np.bool)
+ gt_ids = raw_data['gt_ids'][t][gt_class_mask]
+ gt_dets = raw_data['gt_dets'][t][gt_class_mask]
+
+ tracker_class_mask = np.atleast_1d(raw_data['tracker_classes'][t] == cls_id)
+ tracker_class_mask = tracker_class_mask.astype(np.bool)
+ tracker_ids = raw_data['tracker_ids'][t][tracker_class_mask]
+ tracker_dets = raw_data['tracker_dets'][t][tracker_class_mask]
+ tracker_confidences = raw_data['tracker_confidences'][t][tracker_class_mask]
+ similarity_scores = raw_data['similarity_scores'][t][gt_class_mask, :][:, tracker_class_mask]
+
+ # Match tracker and gt dets (with hungarian algorithm).
+ unmatched_indices = np.arange(tracker_ids.shape[0])
+ if gt_ids.shape[0] > 0 and tracker_ids.shape[0] > 0:
+ matching_scores = similarity_scores.copy()
+ matching_scores[matching_scores < 0.5 - np.finfo('float').eps] = 0
+ match_rows, match_cols = linear_sum_assignment(-matching_scores)
+ actually_matched_mask = matching_scores[match_rows, match_cols] > 0 + np.finfo('float').eps
+ match_cols = match_cols[actually_matched_mask]
+ unmatched_indices = np.delete(unmatched_indices, match_cols, axis=0)
+
+ if gt_ids.shape[0] == 0 and not is_neg_category:
+ to_remove_tracker = unmatched_indices
+ elif is_not_exhaustively_labeled:
+ to_remove_tracker = unmatched_indices
+ else:
+ to_remove_tracker = np.array([], dtype=np.int)
+
+ # remove all unwanted unmatched tracker detections
+ data['tracker_ids'][t] = np.delete(tracker_ids, to_remove_tracker, axis=0)
+ data['tracker_dets'][t] = np.delete(tracker_dets, to_remove_tracker, axis=0)
+ data['tracker_confidences'][t] = np.delete(tracker_confidences, to_remove_tracker, axis=0)
+ similarity_scores = np.delete(similarity_scores, to_remove_tracker, axis=1)
+
+ data['gt_ids'][t] = gt_ids
+ data['gt_dets'][t] = gt_dets
+ data['similarity_scores'][t] = similarity_scores
+
+ unique_gt_ids += list(np.unique(data['gt_ids'][t]))
+ unique_tracker_ids += list(np.unique(data['tracker_ids'][t]))
+ num_tracker_dets += len(data['tracker_ids'][t])
+ num_gt_dets += len(data['gt_ids'][t])
+
+ # Re-label IDs such that there are no empty IDs
+ if len(unique_gt_ids) > 0:
+ unique_gt_ids = np.unique(unique_gt_ids)
+ gt_id_map = np.nan * np.ones((np.max(unique_gt_ids) + 1))
+ gt_id_map[unique_gt_ids] = np.arange(len(unique_gt_ids))
+ for t in range(raw_data['num_timesteps']):
+ if len(data['gt_ids'][t]) > 0:
+ data['gt_ids'][t] = gt_id_map[data['gt_ids'][t]].astype(np.int)
+ if len(unique_tracker_ids) > 0:
+ unique_tracker_ids = np.unique(unique_tracker_ids)
+ tracker_id_map = np.nan * np.ones((np.max(unique_tracker_ids) + 1))
+ tracker_id_map[unique_tracker_ids] = np.arange(len(unique_tracker_ids))
+ for t in range(raw_data['num_timesteps']):
+ if len(data['tracker_ids'][t]) > 0:
+ data['tracker_ids'][t] = tracker_id_map[data['tracker_ids'][t]].astype(np.int)
+
+ # Record overview statistics.
+ data['num_tracker_dets'] = num_tracker_dets
+ data['num_gt_dets'] = num_gt_dets
+ data['num_tracker_ids'] = len(unique_tracker_ids)
+ data['num_gt_ids'] = len(unique_gt_ids)
+ data['num_timesteps'] = raw_data['num_timesteps']
+ data['seq'] = raw_data['seq']
+
+ # get track representations
+ data['gt_tracks'] = raw_data['classes_to_gt_tracks'][cls_id]
+ data['gt_track_ids'] = raw_data['classes_to_gt_track_ids'][cls_id]
+ data['gt_track_lengths'] = raw_data['classes_to_gt_track_lengths'][cls_id]
+ data['gt_track_areas'] = raw_data['classes_to_gt_track_areas'][cls_id]
+ data['dt_tracks'] = raw_data['classes_to_dt_tracks'][cls_id]
+ data['dt_track_ids'] = raw_data['classes_to_dt_track_ids'][cls_id]
+ data['dt_track_lengths'] = raw_data['classes_to_dt_track_lengths'][cls_id]
+ data['dt_track_areas'] = raw_data['classes_to_dt_track_areas'][cls_id]
+ data['dt_track_scores'] = raw_data['classes_to_dt_track_scores'][cls_id]
+ data['not_exhaustively_labeled'] = is_not_exhaustively_labeled
+ data['iou_type'] = 'bbox'
+
+ # sort tracker data tracks by tracker confidence scores
+ if data['dt_tracks']:
+ idx = np.argsort([-score for score in data['dt_track_scores']], kind="mergesort")
+ data['dt_track_scores'] = [data['dt_track_scores'][i] for i in idx]
+ data['dt_tracks'] = [data['dt_tracks'][i] for i in idx]
+ data['dt_track_ids'] = [data['dt_track_ids'][i] for i in idx]
+ data['dt_track_lengths'] = [data['dt_track_lengths'][i] for i in idx]
+ data['dt_track_areas'] = [data['dt_track_areas'][i] for i in idx]
+ # Ensure that ids are unique per timestep.
+ self._check_unique_ids(data)
+
+ return data
+
+ def _calculate_similarities(self, gt_dets_t, tracker_dets_t):
+ similarity_scores = self._calculate_box_ious(gt_dets_t, tracker_dets_t)
+ return similarity_scores
+
+ def _merge_categories(self, annotations):
+ """
+ Merges categories with a merged tag. Adapted from https://github.com/TAO-Dataset
+ :param annotations: the annotations in which the classes should be merged
+ :return: None
+ """
+ merge_map = {}
+ for category in self.gt_data['categories']:
+ if 'merged' in category:
+ for to_merge in category['merged']:
+ merge_map[to_merge['id']] = category['id']
+
+ for ann in annotations:
+ ann['category_id'] = merge_map.get(ann['category_id'], ann['category_id'])
+
+ def _compute_vid_mappings(self, annotations):
+ """
+ Computes mappings from Videos to corresponding tracks and images.
+ :param annotations: the annotations for which the mapping should be generated
+ :return: the video-to-track-mapping, the video-to-image-mapping
+ """
+ vids_to_tracks = {}
+ vids_to_imgs = {}
+ vid_ids = [vid['id'] for vid in self.gt_data['videos']]
+
+ # compute an mapping from image IDs to images
+ images = {}
+ for image in self.gt_data['images']:
+ images[image['id']] = image
+
+ for ann in annotations:
+ ann["area"] = ann["bbox"][2] * ann["bbox"][3]
+
+ vid = ann["video_id"]
+ if ann["video_id"] not in vids_to_tracks.keys():
+ vids_to_tracks[ann["video_id"]] = list()
+ if ann["video_id"] not in vids_to_imgs.keys():
+ vids_to_imgs[ann["video_id"]] = list()
+
+ # Fill in vids_to_tracks
+ tid = ann["track_id"]
+ exist_tids = [track["id"] for track in vids_to_tracks[vid]]
+ try:
+ index1 = exist_tids.index(tid)
+ except ValueError:
+ index1 = -1
+ if tid not in exist_tids:
+ curr_track = {"id": tid, "category_id": ann['category_id'],
+ "video_id": vid, "annotations": [ann]}
+ vids_to_tracks[vid].append(curr_track)
+ else:
+ vids_to_tracks[vid][index1]["annotations"].append(ann)
+
+ # Fill in vids_to_imgs
+ img_id = ann['image_id']
+ exist_img_ids = [img["id"] for img in vids_to_imgs[vid]]
+ try:
+ index2 = exist_img_ids.index(img_id)
+ except ValueError:
+ index2 = -1
+ if index2 == -1:
+ curr_img = {"id": img_id, "annotations": [ann]}
+ vids_to_imgs[vid].append(curr_img)
+ else:
+ vids_to_imgs[vid][index2]["annotations"].append(ann)
+
+ # sort annotations by frame index and compute track area
+ for vid, tracks in vids_to_tracks.items():
+ for track in tracks:
+ track["annotations"] = sorted(
+ track['annotations'],
+ key=lambda x: images[x['image_id']]['frame_index'])
+ # Computer average area
+ track["area"] = (sum(x['area'] for x in track['annotations']) / len(track['annotations']))
+
+ # Ensure all videos are present
+ for vid_id in vid_ids:
+ if vid_id not in vids_to_tracks.keys():
+ vids_to_tracks[vid_id] = []
+ if vid_id not in vids_to_imgs.keys():
+ vids_to_imgs[vid_id] = []
+
+ return vids_to_tracks, vids_to_imgs
+
+ def _compute_image_to_timestep_mappings(self):
+ """
+ Computes a mapping from images to the corresponding timestep in the sequence.
+ :return: the image-to-timestep-mapping
+ """
+ images = {}
+ for image in self.gt_data['images']:
+ images[image['id']] = image
+
+ seq_to_imgs_to_timestep = {vid['id']: dict() for vid in self.gt_data['videos']}
+ for vid in seq_to_imgs_to_timestep:
+ curr_imgs = [img['id'] for img in self.videos_to_gt_images[vid]]
+ curr_imgs = sorted(curr_imgs, key=lambda x: images[x]['frame_index'])
+ seq_to_imgs_to_timestep[vid] = {curr_imgs[i]: i for i in range(len(curr_imgs))}
+
+ return seq_to_imgs_to_timestep
+
+ def _limit_dets_per_image(self, annotations):
+ """
+ Limits the number of detections for each image to config['MAX_DETECTIONS']. Adapted from
+ https://github.com/TAO-Dataset/
+ :param annotations: the annotations in which the detections should be limited
+ :return: the annotations with limited detections
+ """
+ max_dets = self.config['MAX_DETECTIONS']
+ img_ann = defaultdict(list)
+ for ann in annotations:
+ img_ann[ann["image_id"]].append(ann)
+
+ for img_id, _anns in img_ann.items():
+ if len(_anns) <= max_dets:
+ continue
+ _anns = sorted(_anns, key=lambda x: x["score"], reverse=True)
+ img_ann[img_id] = _anns[:max_dets]
+
+ return [ann for anns in img_ann.values() for ann in anns]
+
+ def _fill_video_ids_inplace(self, annotations):
+ """
+ Fills in missing video IDs inplace. Adapted from https://github.com/TAO-Dataset/
+ :param annotations: the annotations for which the videos IDs should be filled inplace
+ :return: None
+ """
+ missing_video_id = [x for x in annotations if 'video_id' not in x]
+ if missing_video_id:
+ image_id_to_video_id = {
+ x['id']: x['video_id'] for x in self.gt_data['images']
+ }
+ for x in missing_video_id:
+ x['video_id'] = image_id_to_video_id[x['image_id']]
+
+ @staticmethod
+ def _make_track_ids_unique(annotations):
+ """
+ Makes the track IDs unqiue over the whole annotation set. Adapted from https://github.com/TAO-Dataset/
+ :param annotations: the annotation set
+ :return: the number of updated IDs
+ """
+ track_id_videos = {}
+ track_ids_to_update = set()
+ max_track_id = 0
+ for ann in annotations:
+ t = ann['track_id']
+ if t not in track_id_videos:
+ track_id_videos[t] = ann['video_id']
+
+ if ann['video_id'] != track_id_videos[t]:
+ # Track id is assigned to multiple videos
+ track_ids_to_update.add(t)
+ max_track_id = max(max_track_id, t)
+
+ if track_ids_to_update:
+ print('true')
+ next_id = itertools.count(max_track_id + 1)
+ new_track_ids = defaultdict(lambda: next(next_id))
+ for ann in annotations:
+ t = ann['track_id']
+ v = ann['video_id']
+ if t in track_ids_to_update:
+ ann['track_id'] = new_track_ids[t, v]
+ return len(track_ids_to_update)
diff --git a/val_utils/trackeval/datasets/tao_ow.py b/val_utils/trackeval/datasets/tao_ow.py
new file mode 100644
index 0000000000000000000000000000000000000000..40f80d7876ed9f27fb108b732315c4f8c6fc0984
--- /dev/null
+++ b/val_utils/trackeval/datasets/tao_ow.py
@@ -0,0 +1,652 @@
+import os
+import numpy as np
+import json
+import itertools
+from collections import defaultdict
+from scipy.optimize import linear_sum_assignment
+from ..utils import TrackEvalException
+from ._base_dataset import _BaseDataset
+from .. import utils
+from .. import _timing
+
+
+class TAO_OW(_BaseDataset):
+ """Dataset class for TAO tracking"""
+
+ @staticmethod
+ def get_default_dataset_config():
+ """Default class config values"""
+ code_path = utils.get_code_path()
+ default_config = {
+ 'GT_FOLDER': os.path.join(code_path, 'data/gt/tao/tao_training'), # Location of GT data
+ 'TRACKERS_FOLDER': os.path.join(code_path, 'data/trackers/tao/tao_training'), # Trackers location
+ 'OUTPUT_FOLDER': None, # Where to save eval results (if None, same as TRACKERS_FOLDER)
+ 'TRACKERS_TO_EVAL': None, # Filenames of trackers to eval (if None, all in folder)
+ 'CLASSES_TO_EVAL': None, # Classes to eval (if None, all classes)
+ 'SPLIT_TO_EVAL': 'training', # Valid: 'training', 'val'
+ 'PRINT_CONFIG': True, # Whether to print current config
+ 'TRACKER_SUB_FOLDER': 'data', # Tracker files are in TRACKER_FOLDER/tracker_name/TRACKER_SUB_FOLDER
+ 'OUTPUT_SUB_FOLDER': '', # Output files are saved in OUTPUT_FOLDER/tracker_name/OUTPUT_SUB_FOLDER
+ 'TRACKER_DISPLAY_NAMES': None, # Names of trackers to display, if None: TRACKERS_TO_EVAL
+ 'MAX_DETECTIONS': 300, # Number of maximal allowed detections per image (0 for unlimited)
+ 'SUBSET': 'all'
+ }
+ return default_config
+
+ def __init__(self, config=None):
+ """Initialise dataset, checking that all required files are present"""
+ super().__init__()
+ # Fill non-given config values with defaults
+ self.config = utils.init_config(config, self.get_default_dataset_config(), self.get_name())
+ self.gt_fol = self.config['GT_FOLDER']
+ self.tracker_fol = self.config['TRACKERS_FOLDER']
+ self.should_classes_combine = True
+ self.use_super_categories = False
+
+ self.tracker_sub_fol = self.config['TRACKER_SUB_FOLDER']
+ self.output_fol = self.config['OUTPUT_FOLDER']
+ if self.output_fol is None:
+ self.output_fol = self.tracker_fol
+ self.output_sub_fol = self.config['OUTPUT_SUB_FOLDER']
+
+ gt_dir_files = [file for file in os.listdir(self.gt_fol) if file.endswith('.json')]
+ if len(gt_dir_files) != 1:
+ raise TrackEvalException(self.gt_fol + ' does not contain exactly one json file.')
+
+ with open(os.path.join(self.gt_fol, gt_dir_files[0])) as f:
+ self.gt_data = json.load(f)
+
+ self.subset = self.config['SUBSET']
+ if self.subset != 'all':
+ # Split GT data into `known`, `unknown` or `distractor`
+ self._split_known_unknown_distractor()
+ self.gt_data = self._filter_gt_data(self.gt_data)
+
+ # merge categories marked with a merged tag in TAO dataset
+ self._merge_categories(self.gt_data['annotations'] + self.gt_data['tracks'])
+
+ # Get sequences to eval and sequence information
+ self.seq_list = [vid['name'].replace('/', '-') for vid in self.gt_data['videos']]
+ self.seq_name_to_seq_id = {vid['name'].replace('/', '-'): vid['id'] for vid in self.gt_data['videos']}
+ # compute mappings from videos to annotation data
+ self.videos_to_gt_tracks, self.videos_to_gt_images = self._compute_vid_mappings(self.gt_data['annotations'])
+ # compute sequence lengths
+ self.seq_lengths = {vid['id']: 0 for vid in self.gt_data['videos']}
+ for img in self.gt_data['images']:
+ self.seq_lengths[img['video_id']] += 1
+ self.seq_to_images_to_timestep = self._compute_image_to_timestep_mappings()
+ self.seq_to_classes = {vid['id']: {'pos_cat_ids': list({track['category_id'] for track
+ in self.videos_to_gt_tracks[vid['id']]}),
+ 'neg_cat_ids': vid['neg_category_ids'],
+ 'not_exhaustively_labeled_cat_ids': vid['not_exhaustive_category_ids']}
+ for vid in self.gt_data['videos']}
+
+ # Get classes to eval
+ considered_vid_ids = [self.seq_name_to_seq_id[vid] for vid in self.seq_list]
+ seen_cats = set([cat_id for vid_id in considered_vid_ids for cat_id
+ in self.seq_to_classes[vid_id]['pos_cat_ids']])
+ # only classes with ground truth are evaluated in TAO
+ self.valid_classes = [cls['name'] for cls in self.gt_data['categories'] if cls['id'] in seen_cats]
+ # cls_name_to_cls_id_map = {cls['name']: cls['id'] for cls in self.gt_data['categories']}
+
+ if self.config['CLASSES_TO_EVAL']:
+ # self.class_list = [cls.lower() if cls.lower() in self.valid_classes else None
+ # for cls in self.config['CLASSES_TO_EVAL']]
+ self.class_list = ["object"] # class-agnostic
+ if not all(self.class_list):
+ raise TrackEvalException('Attempted to evaluate an invalid class. Only classes ' +
+ ', '.join(self.valid_classes) +
+ ' are valid (classes present in ground truth data).')
+ else:
+ # self.class_list = [cls for cls in self.valid_classes]
+ self.class_list = ["object"] # class-agnostic
+ # self.class_name_to_class_id = {k: v for k, v in cls_name_to_cls_id_map.items() if k in self.class_list}
+ self.class_name_to_class_id = {"object": 1} # class-agnostic
+
+ # Get trackers to eval
+ if self.config['TRACKERS_TO_EVAL'] is None:
+ self.tracker_list = os.listdir(self.tracker_fol)
+ else:
+ self.tracker_list = self.config['TRACKERS_TO_EVAL']
+
+ if self.config['TRACKER_DISPLAY_NAMES'] is None:
+ self.tracker_to_disp = dict(zip(self.tracker_list, self.tracker_list))
+ elif (self.config['TRACKERS_TO_EVAL'] is not None) and (
+ len(self.config['TRACKER_DISPLAY_NAMES']) == len(self.tracker_list)):
+ self.tracker_to_disp = dict(zip(self.tracker_list, self.config['TRACKER_DISPLAY_NAMES']))
+ else:
+ raise TrackEvalException('List of tracker files and tracker display names do not match.')
+
+ self.tracker_data = {tracker: dict() for tracker in self.tracker_list}
+
+ for tracker in self.tracker_list:
+ tr_dir_files = [file for file in os.listdir(os.path.join(self.tracker_fol, tracker, self.tracker_sub_fol))
+ if file.endswith('.json')]
+ if len(tr_dir_files) != 1:
+ raise TrackEvalException(os.path.join(self.tracker_fol, tracker, self.tracker_sub_fol)
+ + ' does not contain exactly one json file.')
+ with open(os.path.join(self.tracker_fol, tracker, self.tracker_sub_fol, tr_dir_files[0])) as f:
+ curr_data = json.load(f)
+
+ # limit detections if MAX_DETECTIONS > 0
+ if self.config['MAX_DETECTIONS']:
+ curr_data = self._limit_dets_per_image(curr_data)
+
+ # fill missing video ids
+ self._fill_video_ids_inplace(curr_data)
+
+ # make track ids unique over whole evaluation set
+ self._make_track_ids_unique(curr_data)
+
+ # merge categories marked with a merged tag in TAO dataset
+ self._merge_categories(curr_data)
+
+ # get tracker sequence information
+ curr_videos_to_tracker_tracks, curr_videos_to_tracker_images = self._compute_vid_mappings(curr_data)
+ self.tracker_data[tracker]['vids_to_tracks'] = curr_videos_to_tracker_tracks
+ self.tracker_data[tracker]['vids_to_images'] = curr_videos_to_tracker_images
+
+ def get_display_name(self, tracker):
+ return self.tracker_to_disp[tracker]
+
+ def _load_raw_file(self, tracker, seq, is_gt):
+ """Load a file (gt or tracker) in the TAO format
+
+ If is_gt, this returns a dict which contains the fields:
+ [gt_ids, gt_classes] : list (for each timestep) of 1D NDArrays (for each det).
+ [gt_dets]: list (for each timestep) of lists of detections.
+ [classes_to_gt_tracks]: dictionary with class values as keys and list of dictionaries (with frame indices as
+ keys and corresponding segmentations as values) for each track
+ [classes_to_gt_track_ids, classes_to_gt_track_areas, classes_to_gt_track_lengths]: dictionary with class values
+ as keys and lists (for each track) as values
+
+ if not is_gt, this returns a dict which contains the fields:
+ [tracker_ids, tracker_classes, tracker_confidences] : list (for each timestep) of 1D NDArrays (for each det).
+ [tracker_dets]: list (for each timestep) of lists of detections.
+ [classes_to_dt_tracks]: dictionary with class values as keys and list of dictionaries (with frame indices as
+ keys and corresponding segmentations as values) for each track
+ [classes_to_dt_track_ids, classes_to_dt_track_areas, classes_to_dt_track_lengths]: dictionary with class values
+ as keys and lists as values
+ [classes_to_dt_track_scores]: dictionary with class values as keys and 1D numpy arrays as values
+ """
+ seq_id = self.seq_name_to_seq_id[seq]
+ # File location
+ if is_gt:
+ imgs = self.videos_to_gt_images[seq_id]
+ else:
+ imgs = self.tracker_data[tracker]['vids_to_images'][seq_id]
+
+ # Convert data to required format
+ num_timesteps = self.seq_lengths[seq_id]
+ img_to_timestep = self.seq_to_images_to_timestep[seq_id]
+ data_keys = ['ids', 'classes', 'dets']
+ if not is_gt:
+ data_keys += ['tracker_confidences']
+ raw_data = {key: [None] * num_timesteps for key in data_keys}
+ for img in imgs:
+ # some tracker data contains images without any ground truth information, these are ignored
+ try:
+ t = img_to_timestep[img['id']]
+ except KeyError:
+ continue
+ annotations = img['annotations']
+ raw_data['dets'][t] = np.atleast_2d([ann['bbox'] for ann in annotations]).astype(float)
+ raw_data['ids'][t] = np.atleast_1d([ann['track_id'] for ann in annotations]).astype(int)
+ raw_data['classes'][t] = np.atleast_1d([1 for _ in annotations]).astype(int) # class-agnostic
+ if not is_gt:
+ raw_data['tracker_confidences'][t] = np.atleast_1d([ann['score'] for ann in annotations]).astype(float)
+
+ for t, d in enumerate(raw_data['dets']):
+ if d is None:
+ raw_data['dets'][t] = np.empty((0, 4)).astype(float)
+ raw_data['ids'][t] = np.empty(0).astype(int)
+ raw_data['classes'][t] = np.empty(0).astype(int)
+ if not is_gt:
+ raw_data['tracker_confidences'][t] = np.empty(0)
+
+ if is_gt:
+ key_map = {'ids': 'gt_ids',
+ 'classes': 'gt_classes',
+ 'dets': 'gt_dets'}
+ else:
+ key_map = {'ids': 'tracker_ids',
+ 'classes': 'tracker_classes',
+ 'dets': 'tracker_dets'}
+ for k, v in key_map.items():
+ raw_data[v] = raw_data.pop(k)
+
+ # all_classes = [self.class_name_to_class_id[cls] for cls in self.class_list]
+ all_classes = [1] # class-agnostic
+
+ if is_gt:
+ classes_to_consider = all_classes
+ all_tracks = self.videos_to_gt_tracks[seq_id]
+ else:
+ # classes_to_consider = self.seq_to_classes[seq_id]['pos_cat_ids'] \
+ # + self.seq_to_classes[seq_id]['neg_cat_ids']
+ classes_to_consider = all_classes # class-agnostic
+ all_tracks = self.tracker_data[tracker]['vids_to_tracks'][seq_id]
+
+ # classes_to_tracks = {cls: [track for track in all_tracks if track['category_id'] == cls]
+ # if cls in classes_to_consider else [] for cls in all_classes}
+ classes_to_tracks = {cls: [track for track in all_tracks]
+ if cls in classes_to_consider else [] for cls in all_classes} # class-agnostic
+
+ # mapping from classes to track information
+ raw_data['classes_to_tracks'] = {cls: [{det['image_id']: np.atleast_1d(det['bbox'])
+ for det in track['annotations']} for track in tracks]
+ for cls, tracks in classes_to_tracks.items()}
+ raw_data['classes_to_track_ids'] = {cls: [track['id'] for track in tracks]
+ for cls, tracks in classes_to_tracks.items()}
+ raw_data['classes_to_track_areas'] = {cls: [track['area'] for track in tracks]
+ for cls, tracks in classes_to_tracks.items()}
+ raw_data['classes_to_track_lengths'] = {cls: [len(track['annotations']) for track in tracks]
+ for cls, tracks in classes_to_tracks.items()}
+
+ if not is_gt:
+ raw_data['classes_to_dt_track_scores'] = {cls: np.array([np.mean([float(x['score'])
+ for x in track['annotations']])
+ for track in tracks])
+ for cls, tracks in classes_to_tracks.items()}
+
+ if is_gt:
+ key_map = {'classes_to_tracks': 'classes_to_gt_tracks',
+ 'classes_to_track_ids': 'classes_to_gt_track_ids',
+ 'classes_to_track_lengths': 'classes_to_gt_track_lengths',
+ 'classes_to_track_areas': 'classes_to_gt_track_areas'}
+ else:
+ key_map = {'classes_to_tracks': 'classes_to_dt_tracks',
+ 'classes_to_track_ids': 'classes_to_dt_track_ids',
+ 'classes_to_track_lengths': 'classes_to_dt_track_lengths',
+ 'classes_to_track_areas': 'classes_to_dt_track_areas'}
+ for k, v in key_map.items():
+ raw_data[v] = raw_data.pop(k)
+
+ raw_data['num_timesteps'] = num_timesteps
+ raw_data['neg_cat_ids'] = self.seq_to_classes[seq_id]['neg_cat_ids']
+ raw_data['not_exhaustively_labeled_cls'] = self.seq_to_classes[seq_id]['not_exhaustively_labeled_cat_ids']
+ raw_data['seq'] = seq
+ return raw_data
+
+ @_timing.time
+ def get_preprocessed_seq_data(self, raw_data, cls):
+ """ Preprocess data for a single sequence for a single class ready for evaluation.
+ Inputs:
+ - raw_data is a dict containing the data for the sequence already read in by get_raw_seq_data().
+ - cls is the class to be evaluated.
+ Outputs:
+ - data is a dict containing all of the information that metrics need to perform evaluation.
+ It contains the following fields:
+ [num_timesteps, num_gt_ids, num_tracker_ids, num_gt_dets, num_tracker_dets] : integers.
+ [gt_ids, tracker_ids, tracker_confidences]: list (for each timestep) of 1D NDArrays (for each det).
+ [gt_dets, tracker_dets]: list (for each timestep) of lists of detections.
+ [similarity_scores]: list (for each timestep) of 2D NDArrays.
+ Notes:
+ General preprocessing (preproc) occurs in 4 steps. Some datasets may not use all of these steps.
+ 1) Extract only detections relevant for the class to be evaluated (including distractor detections).
+ 2) Match gt dets and tracker dets. Remove tracker dets that are matched to a gt det that is of a
+ distractor class, or otherwise marked as to be removed.
+ 3) Remove unmatched tracker dets if they fall within a crowd ignore region or don't meet a certain
+ other criteria (e.g. are too small).
+ 4) Remove gt dets that were only useful for preprocessing and not for actual evaluation.
+ After the above preprocessing steps, this function also calculates the number of gt and tracker detections
+ and unique track ids. It also relabels gt and tracker ids to be contiguous and checks that ids are
+ unique within each timestep.
+ TAO:
+ In TAO, the 4 preproc steps are as follow:
+ 1) All classes present in the ground truth data are evaluated separately.
+ 2) No matched tracker detections are removed.
+ 3) Unmatched tracker detections are removed if there is not ground truth data and the class does not
+ belong to the categories marked as negative for this sequence. Additionally, unmatched tracker
+ detections for classes which are marked as not exhaustively labeled are removed.
+ 4) No gt detections are removed.
+ Further, for TrackMAP computation track representations for the given class are accessed from a dictionary
+ and the tracks from the tracker data are sorted according to the tracker confidence.
+ """
+ cls_id = self.class_name_to_class_id[cls]
+ is_not_exhaustively_labeled = cls_id in raw_data['not_exhaustively_labeled_cls']
+ is_neg_category = cls_id in raw_data['neg_cat_ids']
+
+ data_keys = ['gt_ids', 'tracker_ids', 'gt_dets', 'tracker_dets', 'tracker_confidences', 'similarity_scores']
+ data = {key: [None] * raw_data['num_timesteps'] for key in data_keys}
+ unique_gt_ids = []
+ unique_tracker_ids = []
+ num_gt_dets = 0
+ num_tracker_dets = 0
+ for t in range(raw_data['num_timesteps']):
+
+ # Only extract relevant dets for this class for preproc and eval (cls)
+ gt_class_mask = np.atleast_1d(raw_data['gt_classes'][t] == cls_id)
+ gt_class_mask = gt_class_mask.astype(np.bool)
+ gt_ids = raw_data['gt_ids'][t][gt_class_mask]
+ gt_dets = raw_data['gt_dets'][t][gt_class_mask]
+
+ tracker_class_mask = np.atleast_1d(raw_data['tracker_classes'][t] == cls_id)
+ tracker_class_mask = tracker_class_mask.astype(np.bool)
+ tracker_ids = raw_data['tracker_ids'][t][tracker_class_mask]
+ tracker_dets = raw_data['tracker_dets'][t][tracker_class_mask]
+ tracker_confidences = raw_data['tracker_confidences'][t][tracker_class_mask]
+ similarity_scores = raw_data['similarity_scores'][t][gt_class_mask, :][:, tracker_class_mask]
+
+ # Match tracker and gt dets (with hungarian algorithm).
+ unmatched_indices = np.arange(tracker_ids.shape[0])
+ if gt_ids.shape[0] > 0 and tracker_ids.shape[0] > 0:
+ matching_scores = similarity_scores.copy()
+ matching_scores[matching_scores < 0.5 - np.finfo('float').eps] = 0
+ match_rows, match_cols = linear_sum_assignment(-matching_scores)
+ actually_matched_mask = matching_scores[match_rows, match_cols] > 0 + np.finfo('float').eps
+ match_cols = match_cols[actually_matched_mask]
+ unmatched_indices = np.delete(unmatched_indices, match_cols, axis=0)
+
+ if gt_ids.shape[0] == 0 and not is_neg_category:
+ to_remove_tracker = unmatched_indices
+ elif is_not_exhaustively_labeled:
+ to_remove_tracker = unmatched_indices
+ else:
+ to_remove_tracker = np.array([], dtype=np.int)
+
+ # remove all unwanted unmatched tracker detections
+ data['tracker_ids'][t] = np.delete(tracker_ids, to_remove_tracker, axis=0)
+ data['tracker_dets'][t] = np.delete(tracker_dets, to_remove_tracker, axis=0)
+ data['tracker_confidences'][t] = np.delete(tracker_confidences, to_remove_tracker, axis=0)
+ similarity_scores = np.delete(similarity_scores, to_remove_tracker, axis=1)
+
+ data['gt_ids'][t] = gt_ids
+ data['gt_dets'][t] = gt_dets
+ data['similarity_scores'][t] = similarity_scores
+
+ unique_gt_ids += list(np.unique(data['gt_ids'][t]))
+ unique_tracker_ids += list(np.unique(data['tracker_ids'][t]))
+ num_tracker_dets += len(data['tracker_ids'][t])
+ num_gt_dets += len(data['gt_ids'][t])
+
+ # Re-label IDs such that there are no empty IDs
+ if len(unique_gt_ids) > 0:
+ unique_gt_ids = np.unique(unique_gt_ids)
+ gt_id_map = np.nan * np.ones((np.max(unique_gt_ids) + 1))
+ gt_id_map[unique_gt_ids] = np.arange(len(unique_gt_ids))
+ for t in range(raw_data['num_timesteps']):
+ if len(data['gt_ids'][t]) > 0:
+ data['gt_ids'][t] = gt_id_map[data['gt_ids'][t]].astype(np.int)
+ if len(unique_tracker_ids) > 0:
+ unique_tracker_ids = np.unique(unique_tracker_ids)
+ tracker_id_map = np.nan * np.ones((np.max(unique_tracker_ids) + 1))
+ tracker_id_map[unique_tracker_ids] = np.arange(len(unique_tracker_ids))
+ for t in range(raw_data['num_timesteps']):
+ if len(data['tracker_ids'][t]) > 0:
+ data['tracker_ids'][t] = tracker_id_map[data['tracker_ids'][t]].astype(np.int)
+
+ # Record overview statistics.
+ data['num_tracker_dets'] = num_tracker_dets
+ data['num_gt_dets'] = num_gt_dets
+ data['num_tracker_ids'] = len(unique_tracker_ids)
+ data['num_gt_ids'] = len(unique_gt_ids)
+ data['num_timesteps'] = raw_data['num_timesteps']
+ data['seq'] = raw_data['seq']
+
+ # get track representations
+ data['gt_tracks'] = raw_data['classes_to_gt_tracks'][cls_id]
+ data['gt_track_ids'] = raw_data['classes_to_gt_track_ids'][cls_id]
+ data['gt_track_lengths'] = raw_data['classes_to_gt_track_lengths'][cls_id]
+ data['gt_track_areas'] = raw_data['classes_to_gt_track_areas'][cls_id]
+ data['dt_tracks'] = raw_data['classes_to_dt_tracks'][cls_id]
+ data['dt_track_ids'] = raw_data['classes_to_dt_track_ids'][cls_id]
+ data['dt_track_lengths'] = raw_data['classes_to_dt_track_lengths'][cls_id]
+ data['dt_track_areas'] = raw_data['classes_to_dt_track_areas'][cls_id]
+ data['dt_track_scores'] = raw_data['classes_to_dt_track_scores'][cls_id]
+ data['not_exhaustively_labeled'] = is_not_exhaustively_labeled
+ data['iou_type'] = 'bbox'
+
+ # sort tracker data tracks by tracker confidence scores
+ if data['dt_tracks']:
+ idx = np.argsort([-score for score in data['dt_track_scores']], kind="mergesort")
+ data['dt_track_scores'] = [data['dt_track_scores'][i] for i in idx]
+ data['dt_tracks'] = [data['dt_tracks'][i] for i in idx]
+ data['dt_track_ids'] = [data['dt_track_ids'][i] for i in idx]
+ data['dt_track_lengths'] = [data['dt_track_lengths'][i] for i in idx]
+ data['dt_track_areas'] = [data['dt_track_areas'][i] for i in idx]
+ # Ensure that ids are unique per timestep.
+ self._check_unique_ids(data)
+
+ return data
+
+ def _calculate_similarities(self, gt_dets_t, tracker_dets_t):
+ similarity_scores = self._calculate_box_ious(gt_dets_t, tracker_dets_t)
+ return similarity_scores
+
+ def _merge_categories(self, annotations):
+ """
+ Merges categories with a merged tag. Adapted from https://github.com/TAO-Dataset
+ :param annotations: the annotations in which the classes should be merged
+ :return: None
+ """
+ merge_map = {}
+ for category in self.gt_data['categories']:
+ if 'merged' in category:
+ for to_merge in category['merged']:
+ merge_map[to_merge['id']] = category['id']
+
+ for ann in annotations:
+ ann['category_id'] = merge_map.get(ann['category_id'], ann['category_id'])
+
+ def _compute_vid_mappings(self, annotations):
+ """
+ Computes mappings from Videos to corresponding tracks and images.
+ :param annotations: the annotations for which the mapping should be generated
+ :return: the video-to-track-mapping, the video-to-image-mapping
+ """
+ vids_to_tracks = {}
+ vids_to_imgs = {}
+ vid_ids = [vid['id'] for vid in self.gt_data['videos']]
+
+ # compute an mapping from image IDs to images
+ images = {}
+ for image in self.gt_data['images']:
+ images[image['id']] = image
+
+ for ann in annotations:
+ ann["area"] = ann["bbox"][2] * ann["bbox"][3]
+
+ vid = ann["video_id"]
+ if ann["video_id"] not in vids_to_tracks.keys():
+ vids_to_tracks[ann["video_id"]] = list()
+ if ann["video_id"] not in vids_to_imgs.keys():
+ vids_to_imgs[ann["video_id"]] = list()
+
+ # Fill in vids_to_tracks
+ tid = ann["track_id"]
+ exist_tids = [track["id"] for track in vids_to_tracks[vid]]
+ try:
+ index1 = exist_tids.index(tid)
+ except ValueError:
+ index1 = -1
+ if tid not in exist_tids:
+ curr_track = {"id": tid, "category_id": ann['category_id'],
+ "video_id": vid, "annotations": [ann]}
+ vids_to_tracks[vid].append(curr_track)
+ else:
+ vids_to_tracks[vid][index1]["annotations"].append(ann)
+
+ # Fill in vids_to_imgs
+ img_id = ann['image_id']
+ exist_img_ids = [img["id"] for img in vids_to_imgs[vid]]
+ try:
+ index2 = exist_img_ids.index(img_id)
+ except ValueError:
+ index2 = -1
+ if index2 == -1:
+ curr_img = {"id": img_id, "annotations": [ann]}
+ vids_to_imgs[vid].append(curr_img)
+ else:
+ vids_to_imgs[vid][index2]["annotations"].append(ann)
+
+ # sort annotations by frame index and compute track area
+ for vid, tracks in vids_to_tracks.items():
+ for track in tracks:
+ track["annotations"] = sorted(
+ track['annotations'],
+ key=lambda x: images[x['image_id']]['frame_index'])
+ # Computer average area
+ track["area"] = (sum(x['area'] for x in track['annotations']) / len(track['annotations']))
+
+ # Ensure all videos are present
+ for vid_id in vid_ids:
+ if vid_id not in vids_to_tracks.keys():
+ vids_to_tracks[vid_id] = []
+ if vid_id not in vids_to_imgs.keys():
+ vids_to_imgs[vid_id] = []
+
+ return vids_to_tracks, vids_to_imgs
+
+ def _compute_image_to_timestep_mappings(self):
+ """
+ Computes a mapping from images to the corresponding timestep in the sequence.
+ :return: the image-to-timestep-mapping
+ """
+ images = {}
+ for image in self.gt_data['images']:
+ images[image['id']] = image
+
+ seq_to_imgs_to_timestep = {vid['id']: dict() for vid in self.gt_data['videos']}
+ for vid in seq_to_imgs_to_timestep:
+ curr_imgs = [img['id'] for img in self.videos_to_gt_images[vid]]
+ curr_imgs = sorted(curr_imgs, key=lambda x: images[x]['frame_index'])
+ seq_to_imgs_to_timestep[vid] = {curr_imgs[i]: i for i in range(len(curr_imgs))}
+
+ return seq_to_imgs_to_timestep
+
+ def _limit_dets_per_image(self, annotations):
+ """
+ Limits the number of detections for each image to config['MAX_DETECTIONS']. Adapted from
+ https://github.com/TAO-Dataset/
+ :param annotations: the annotations in which the detections should be limited
+ :return: the annotations with limited detections
+ """
+ max_dets = self.config['MAX_DETECTIONS']
+ img_ann = defaultdict(list)
+ for ann in annotations:
+ img_ann[ann["image_id"]].append(ann)
+
+ for img_id, _anns in img_ann.items():
+ if len(_anns) <= max_dets:
+ continue
+ _anns = sorted(_anns, key=lambda x: x["score"], reverse=True)
+ img_ann[img_id] = _anns[:max_dets]
+
+ return [ann for anns in img_ann.values() for ann in anns]
+
+ def _fill_video_ids_inplace(self, annotations):
+ """
+ Fills in missing video IDs inplace. Adapted from https://github.com/TAO-Dataset/
+ :param annotations: the annotations for which the videos IDs should be filled inplace
+ :return: None
+ """
+ missing_video_id = [x for x in annotations if 'video_id' not in x]
+ if missing_video_id:
+ image_id_to_video_id = {
+ x['id']: x['video_id'] for x in self.gt_data['images']
+ }
+ for x in missing_video_id:
+ x['video_id'] = image_id_to_video_id[x['image_id']]
+
+ @staticmethod
+ def _make_track_ids_unique(annotations):
+ """
+ Makes the track IDs unqiue over the whole annotation set. Adapted from https://github.com/TAO-Dataset/
+ :param annotations: the annotation set
+ :return: the number of updated IDs
+ """
+ track_id_videos = {}
+ track_ids_to_update = set()
+ max_track_id = 0
+ for ann in annotations:
+ t = ann['track_id']
+ if t not in track_id_videos:
+ track_id_videos[t] = ann['video_id']
+
+ if ann['video_id'] != track_id_videos[t]:
+ # Track id is assigned to multiple videos
+ track_ids_to_update.add(t)
+ max_track_id = max(max_track_id, t)
+
+ if track_ids_to_update:
+ print('true')
+ next_id = itertools.count(max_track_id + 1)
+ new_track_ids = defaultdict(lambda: next(next_id))
+ for ann in annotations:
+ t = ann['track_id']
+ v = ann['video_id']
+ if t in track_ids_to_update:
+ ann['track_id'] = new_track_ids[t, v]
+ return len(track_ids_to_update)
+
+ def _split_known_unknown_distractor(self):
+ all_ids = set([i for i in range(1, 2000)]) # 2000 is larger than the max category id in TAO-OW.
+ # `knowns` includes 78 TAO_category_ids that corresponds to 78 COCO classes.
+ # (The other 2 COCO classes do not have corresponding classes in TAO).
+ self.knowns = {4, 13, 1038, 544, 1057, 34, 35, 36, 41, 45, 58, 60, 579, 1091, 1097, 1099, 78, 79, 81, 91, 1115,
+ 1117, 95, 1122, 99, 1132, 621, 1135, 625, 118, 1144, 126, 642, 1155, 133, 1162, 139, 154, 174, 185,
+ 699, 1215, 714, 717, 1229, 211, 729, 221, 229, 747, 235, 237, 779, 276, 805, 299, 829, 852, 347,
+ 371, 382, 896, 392, 926, 937, 428, 429, 961, 452, 979, 980, 982, 475, 480, 993, 1001, 502, 1018}
+ # `distractors` is defined as in the paper "Opening up Open-World Tracking"
+ self.distractors = {20, 63, 108, 180, 188, 204, 212, 247, 303, 403, 407, 415, 490, 504, 507, 513, 529, 567,
+ 569, 588, 672, 691, 702, 708, 711, 720, 736, 737, 798, 813, 815, 827, 831, 851, 877, 883,
+ 912, 971, 976, 1130, 1133, 1134, 1169, 1184, 1220}
+ self.unknowns = all_ids.difference(self.knowns.union(self.distractors))
+
+ def _filter_gt_data(self, raw_gt_data):
+ """
+ Filter out irrelevant data in the raw_gt_data
+ Args:
+ raw_gt_data: directly loaded from json.
+
+ Returns:
+ filtered gt_data
+ """
+ valid_cat_ids = list()
+ if self.subset == "known":
+ valid_cat_ids = self.knowns
+ elif self.subset == "distractor":
+ valid_cat_ids = self.distractors
+ elif self.subset == "unknown":
+ valid_cat_ids = self.unknowns
+ # elif self.subset == "test_only_unknowns":
+ # valid_cat_ids = test_only_unknowns
+ else:
+ raise Exception("The parameter `SUBSET` is incorrect")
+
+ filtered = dict()
+ filtered["videos"] = raw_gt_data["videos"]
+ # filtered["videos"] = list()
+ unwanted_vid = set()
+ # for video in raw_gt_data["videos"]:
+ # datasrc = video["name"].split('/')[1]
+ # if datasrc in data_srcs:
+ # filtered["videos"].append(video)
+ # else:
+ # unwanted_vid.add(video["id"])
+
+ filtered["annotations"] = list()
+ for ann in raw_gt_data["annotations"]:
+ if (ann["video_id"] not in unwanted_vid) and (ann["category_id"] in valid_cat_ids):
+ filtered["annotations"].append(ann)
+
+ filtered["tracks"] = list()
+ for track in raw_gt_data["tracks"]:
+ if (track["video_id"] not in unwanted_vid) and (track["category_id"] in valid_cat_ids):
+ filtered["tracks"].append(track)
+
+ filtered["images"] = list()
+ for image in raw_gt_data["images"]:
+ if image["video_id"] not in unwanted_vid:
+ filtered["images"].append(image)
+
+ filtered["categories"] = list()
+ for cat in raw_gt_data["categories"]:
+ if cat["id"] in valid_cat_ids:
+ filtered["categories"].append(cat)
+
+ filtered["info"] = raw_gt_data["info"]
+ filtered["licenses"] = raw_gt_data["licenses"]
+
+ return filtered
diff --git a/val_utils/trackeval/datasets/youtube_vis.py b/val_utils/trackeval/datasets/youtube_vis.py
new file mode 100644
index 0000000000000000000000000000000000000000..6d5b54c9ed9045c5f4e2f25e4234f87a00f8e302
--- /dev/null
+++ b/val_utils/trackeval/datasets/youtube_vis.py
@@ -0,0 +1,364 @@
+import os
+import numpy as np
+import json
+from ._base_dataset import _BaseDataset
+from ..utils import TrackEvalException
+from .. import utils
+from .. import _timing
+
+
+class YouTubeVIS(_BaseDataset):
+ """Dataset class for YouTubeVIS tracking"""
+
+ @staticmethod
+ def get_default_dataset_config():
+ """Default class config values"""
+ code_path = utils.get_code_path()
+ default_config = {
+ 'GT_FOLDER': os.path.join(code_path, 'data/gt/youtube_vis/'), # Location of GT data
+ 'TRACKERS_FOLDER': os.path.join(code_path, 'data/trackers/youtube_vis/'),
+ # Trackers location
+ 'OUTPUT_FOLDER': None, # Where to save eval results (if None, same as TRACKERS_FOLDER)
+ 'TRACKERS_TO_EVAL': None, # Filenames of trackers to eval (if None, all in folder)
+ 'CLASSES_TO_EVAL': None, # Classes to eval (if None, all classes)
+ 'SPLIT_TO_EVAL': 'train_sub_split', # Valid: 'train', 'val', 'train_sub_split'
+ 'PRINT_CONFIG': True, # Whether to print current config
+ 'OUTPUT_SUB_FOLDER': '', # Output files are saved in OUTPUT_FOLDER/tracker_name/OUTPUT_SUB_FOLDER
+ 'TRACKER_SUB_FOLDER': 'data', # Tracker files are in TRACKER_FOLDER/tracker_name/TRACKER_SUB_FOLDER
+ 'TRACKER_DISPLAY_NAMES': None, # Names of trackers to display, if None: TRACKERS_TO_EVAL
+ }
+ return default_config
+
+ def __init__(self, config=None):
+ """Initialise dataset, checking that all required files are present"""
+ super().__init__()
+ # Fill non-given config values with defaults
+ self.config = utils.init_config(config, self.get_default_dataset_config(), self.get_name())
+ self.gt_fol = self.config['GT_FOLDER'] + 'youtube_vis_' + self.config['SPLIT_TO_EVAL']
+ self.tracker_fol = self.config['TRACKERS_FOLDER'] + 'youtube_vis_' + self.config['SPLIT_TO_EVAL']
+ self.use_super_categories = False
+ self.should_classes_combine = True
+
+ self.output_fol = self.config['OUTPUT_FOLDER']
+ if self.output_fol is None:
+ self.output_fol = self.tracker_fol
+ self.output_sub_fol = self.config['OUTPUT_SUB_FOLDER']
+ self.tracker_sub_fol = self.config['TRACKER_SUB_FOLDER']
+
+ if not os.path.exists(self.gt_fol):
+ print("GT folder not found: " + self.gt_fol)
+ raise TrackEvalException("GT folder not found: " + os.path.basename(self.gt_fol))
+ gt_dir_files = [file for file in os.listdir(self.gt_fol) if file.endswith('.json')]
+ if len(gt_dir_files) != 1:
+ raise TrackEvalException(self.gt_fol + ' does not contain exactly one json file.')
+
+ with open(os.path.join(self.gt_fol, gt_dir_files[0])) as f:
+ self.gt_data = json.load(f)
+
+ # Get classes to eval
+ self.valid_classes = [cls['name'] for cls in self.gt_data['categories']]
+ cls_name_to_cls_id_map = {cls['name']: cls['id'] for cls in self.gt_data['categories']}
+
+ if self.config['CLASSES_TO_EVAL']:
+ self.class_list = [cls.lower() if cls.lower() in self.valid_classes else None
+ for cls in self.config['CLASSES_TO_EVAL']]
+ if not all(self.class_list):
+ raise TrackEvalException('Attempted to evaluate an invalid class. Only classes ' +
+ ', '.join(self.valid_classes) + ' are valid.')
+ else:
+ self.class_list = [cls['name'] for cls in self.gt_data['categories']]
+ self.class_name_to_class_id = {k: v for k, v in cls_name_to_cls_id_map.items() if k in self.class_list}
+
+ # Get sequences to eval and check gt files exist
+ self.seq_list = [vid['file_names'][0].split('/')[0] for vid in self.gt_data['videos']]
+ self.seq_name_to_seq_id = {vid['file_names'][0].split('/')[0]: vid['id'] for vid in self.gt_data['videos']}
+ self.seq_lengths = {vid['id']: len(vid['file_names']) for vid in self.gt_data['videos']}
+
+ # encode masks and compute track areas
+ self._prepare_gt_annotations()
+
+ # Get trackers to eval
+ if self.config['TRACKERS_TO_EVAL'] is None:
+ self.tracker_list = os.listdir(self.tracker_fol)
+ else:
+ self.tracker_list = self.config['TRACKERS_TO_EVAL']
+
+ if self.config['TRACKER_DISPLAY_NAMES'] is None:
+ self.tracker_to_disp = dict(zip(self.tracker_list, self.tracker_list))
+ elif (self.config['TRACKERS_TO_EVAL'] is not None) and (
+ len(self.config['TRACKER_DISPLAY_NAMES']) == len(self.tracker_list)):
+ self.tracker_to_disp = dict(zip(self.tracker_list, self.config['TRACKER_DISPLAY_NAMES']))
+ else:
+ raise TrackEvalException('List of tracker files and tracker display names do not match.')
+
+ # counter for globally unique track IDs
+ self.global_tid_counter = 0
+
+ self.tracker_data = dict()
+ for tracker in self.tracker_list:
+ tracker_dir_path = os.path.join(self.tracker_fol, tracker, self.tracker_sub_fol)
+ tr_dir_files = [file for file in os.listdir(tracker_dir_path) if file.endswith('.json')]
+ if len(tr_dir_files) != 1:
+ raise TrackEvalException(tracker_dir_path + ' does not contain exactly one json file.')
+
+ with open(os.path.join(tracker_dir_path, tr_dir_files[0])) as f:
+ curr_data = json.load(f)
+
+ self.tracker_data[tracker] = curr_data
+
+ def get_display_name(self, tracker):
+ return self.tracker_to_disp[tracker]
+
+ def _load_raw_file(self, tracker, seq, is_gt):
+ """Load a file (gt or tracker) in the YouTubeVIS format
+ If is_gt, this returns a dict which contains the fields:
+ [gt_ids, gt_classes] : list (for each timestep) of 1D NDArrays (for each det).
+ [gt_dets]: list (for each timestep) of lists of detections.
+ [classes_to_gt_tracks]: dictionary with class values as keys and list of dictionaries (with frame indices as
+ keys and corresponding segmentations as values) for each track
+ [classes_to_gt_track_ids, classes_to_gt_track_areas, classes_to_gt_track_iscrowd]: dictionary with class values
+ as keys and lists (for each track) as values
+
+ if not is_gt, this returns a dict which contains the fields:
+ [tracker_ids, tracker_classes, tracker_confidences] : list (for each timestep) of 1D NDArrays (for each det).
+ [tracker_dets]: list (for each timestep) of lists of detections.
+ [classes_to_dt_tracks]: dictionary with class values as keys and list of dictionaries (with frame indices as
+ keys and corresponding segmentations as values) for each track
+ [classes_to_dt_track_ids, classes_to_dt_track_areas]: dictionary with class values as keys and lists as values
+ [classes_to_dt_track_scores]: dictionary with class values as keys and 1D numpy arrays as values
+ """
+ # select sequence tracks
+ seq_id = self.seq_name_to_seq_id[seq]
+ if is_gt:
+ tracks = [ann for ann in self.gt_data['annotations'] if ann['video_id'] == seq_id]
+ else:
+ tracks = self._get_tracker_seq_tracks(tracker, seq_id)
+
+ # Convert data to required format
+ num_timesteps = self.seq_lengths[seq_id]
+ data_keys = ['ids', 'classes', 'dets']
+ if not is_gt:
+ data_keys += ['tracker_confidences']
+ raw_data = {key: [None] * num_timesteps for key in data_keys}
+ for t in range(num_timesteps):
+ raw_data['dets'][t] = [track['segmentations'][t] for track in tracks if track['segmentations'][t]]
+ raw_data['ids'][t] = np.atleast_1d([track['id'] for track in tracks
+ if track['segmentations'][t]]).astype(int)
+ raw_data['classes'][t] = np.atleast_1d([track['category_id'] for track in tracks
+ if track['segmentations'][t]]).astype(int)
+ if not is_gt:
+ raw_data['tracker_confidences'][t] = np.atleast_1d([track['score'] for track in tracks
+ if track['segmentations'][t]]).astype(float)
+
+ if is_gt:
+ key_map = {'ids': 'gt_ids',
+ 'classes': 'gt_classes',
+ 'dets': 'gt_dets'}
+ else:
+ key_map = {'ids': 'tracker_ids',
+ 'classes': 'tracker_classes',
+ 'dets': 'tracker_dets'}
+ for k, v in key_map.items():
+ raw_data[v] = raw_data.pop(k)
+
+ all_cls_ids = {self.class_name_to_class_id[cls] for cls in self.class_list}
+ classes_to_tracks = {cls: [track for track in tracks if track['category_id'] == cls] for cls in all_cls_ids}
+
+ # mapping from classes to track representations and track information
+ raw_data['classes_to_tracks'] = {cls: [{i: track['segmentations'][i]
+ for i in range(len(track['segmentations']))} for track in tracks]
+ for cls, tracks in classes_to_tracks.items()}
+ raw_data['classes_to_track_ids'] = {cls: [track['id'] for track in tracks]
+ for cls, tracks in classes_to_tracks.items()}
+ raw_data['classes_to_track_areas'] = {cls: [track['area'] for track in tracks]
+ for cls, tracks in classes_to_tracks.items()}
+
+ if is_gt:
+ raw_data['classes_to_gt_track_iscrowd'] = {cls: [track['iscrowd'] for track in tracks]
+ for cls, tracks in classes_to_tracks.items()}
+ else:
+ raw_data['classes_to_dt_track_scores'] = {cls: np.array([track['score'] for track in tracks])
+ for cls, tracks in classes_to_tracks.items()}
+
+ if is_gt:
+ key_map = {'classes_to_tracks': 'classes_to_gt_tracks',
+ 'classes_to_track_ids': 'classes_to_gt_track_ids',
+ 'classes_to_track_areas': 'classes_to_gt_track_areas'}
+ else:
+ key_map = {'classes_to_tracks': 'classes_to_dt_tracks',
+ 'classes_to_track_ids': 'classes_to_dt_track_ids',
+ 'classes_to_track_areas': 'classes_to_dt_track_areas'}
+ for k, v in key_map.items():
+ raw_data[v] = raw_data.pop(k)
+
+ raw_data['num_timesteps'] = num_timesteps
+ raw_data['seq'] = seq
+ return raw_data
+
+ @_timing.time
+ def get_preprocessed_seq_data(self, raw_data, cls):
+ """ Preprocess data for a single sequence for a single class ready for evaluation.
+ Inputs:
+ - raw_data is a dict containing the data for the sequence already read in by get_raw_seq_data().
+ - cls is the class to be evaluated.
+ Outputs:
+ - data is a dict containing all of the information that metrics need to perform evaluation.
+ It contains the following fields:
+ [num_timesteps, num_gt_ids, num_tracker_ids, num_gt_dets, num_tracker_dets] : integers.
+ [gt_ids, tracker_ids, tracker_confidences]: list (for each timestep) of 1D NDArrays (for each det).
+ [gt_dets, tracker_dets]: list (for each timestep) of lists of detections.
+ [similarity_scores]: list (for each timestep) of 2D NDArrays.
+ Notes:
+ General preprocessing (preproc) occurs in 4 steps. Some datasets may not use all of these steps.
+ 1) Extract only detections relevant for the class to be evaluated (including distractor detections).
+ 2) Match gt dets and tracker dets. Remove tracker dets that are matched to a gt det that is of a
+ distractor class, or otherwise marked as to be removed.
+ 3) Remove unmatched tracker dets if they fall within a crowd ignore region or don't meet a certain
+ other criteria (e.g. are too small).
+ 4) Remove gt dets that were only useful for preprocessing and not for actual evaluation.
+ After the above preprocessing steps, this function also calculates the number of gt and tracker detections
+ and unique track ids. It also relabels gt and tracker ids to be contiguous and checks that ids are
+ unique within each timestep.
+ YouTubeVIS:
+ In YouTubeVIS, the 4 preproc steps are as follow:
+ 1) There are 40 classes which are evaluated separately.
+ 2) No matched tracker dets are removed.
+ 3) No unmatched tracker dets are removed.
+ 4) No gt dets are removed.
+ Further, for TrackMAP computation track representations for the given class are accessed from a dictionary
+ and the tracks from the tracker data are sorted according to the tracker confidence.
+ """
+ cls_id = self.class_name_to_class_id[cls]
+
+ data_keys = ['gt_ids', 'tracker_ids', 'gt_dets', 'tracker_dets', 'similarity_scores']
+ data = {key: [None] * raw_data['num_timesteps'] for key in data_keys}
+ unique_gt_ids = []
+ unique_tracker_ids = []
+ num_gt_dets = 0
+ num_tracker_dets = 0
+
+ for t in range(raw_data['num_timesteps']):
+
+ # Only extract relevant dets for this class for eval (cls)
+ gt_class_mask = np.atleast_1d(raw_data['gt_classes'][t] == cls_id)
+ gt_class_mask = gt_class_mask.astype(np.bool)
+ gt_ids = raw_data['gt_ids'][t][gt_class_mask]
+ gt_dets = [raw_data['gt_dets'][t][ind] for ind in range(len(gt_class_mask)) if gt_class_mask[ind]]
+
+ tracker_class_mask = np.atleast_1d(raw_data['tracker_classes'][t] == cls_id)
+ tracker_class_mask = tracker_class_mask.astype(np.bool)
+ tracker_ids = raw_data['tracker_ids'][t][tracker_class_mask]
+ tracker_dets = [raw_data['tracker_dets'][t][ind] for ind in range(len(tracker_class_mask)) if
+ tracker_class_mask[ind]]
+ similarity_scores = raw_data['similarity_scores'][t][gt_class_mask, :][:, tracker_class_mask]
+
+ data['tracker_ids'][t] = tracker_ids
+ data['tracker_dets'][t] = tracker_dets
+ data['gt_ids'][t] = gt_ids
+ data['gt_dets'][t] = gt_dets
+ data['similarity_scores'][t] = similarity_scores
+
+ unique_gt_ids += list(np.unique(data['gt_ids'][t]))
+ unique_tracker_ids += list(np.unique(data['tracker_ids'][t]))
+ num_tracker_dets += len(data['tracker_ids'][t])
+ num_gt_dets += len(data['gt_ids'][t])
+
+ # Re-label IDs such that there are no empty IDs
+ if len(unique_gt_ids) > 0:
+ unique_gt_ids = np.unique(unique_gt_ids)
+ gt_id_map = np.nan * np.ones((np.max(unique_gt_ids) + 1))
+ gt_id_map[unique_gt_ids] = np.arange(len(unique_gt_ids))
+ for t in range(raw_data['num_timesteps']):
+ if len(data['gt_ids'][t]) > 0:
+ data['gt_ids'][t] = gt_id_map[data['gt_ids'][t]].astype(np.int)
+ if len(unique_tracker_ids) > 0:
+ unique_tracker_ids = np.unique(unique_tracker_ids)
+ tracker_id_map = np.nan * np.ones((np.max(unique_tracker_ids) + 1))
+ tracker_id_map[unique_tracker_ids] = np.arange(len(unique_tracker_ids))
+ for t in range(raw_data['num_timesteps']):
+ if len(data['tracker_ids'][t]) > 0:
+ data['tracker_ids'][t] = tracker_id_map[data['tracker_ids'][t]].astype(np.int)
+
+ # Ensure that ids are unique per timestep.
+ self._check_unique_ids(data)
+
+ # Record overview statistics.
+ data['num_tracker_dets'] = num_tracker_dets
+ data['num_gt_dets'] = num_gt_dets
+ data['num_tracker_ids'] = len(unique_tracker_ids)
+ data['num_gt_ids'] = len(unique_gt_ids)
+ data['num_timesteps'] = raw_data['num_timesteps']
+ data['seq'] = raw_data['seq']
+
+ # get track representations
+ data['gt_tracks'] = raw_data['classes_to_gt_tracks'][cls_id]
+ data['gt_track_ids'] = raw_data['classes_to_gt_track_ids'][cls_id]
+ data['gt_track_areas'] = raw_data['classes_to_gt_track_areas'][cls_id]
+ data['gt_track_iscrowd'] = raw_data['classes_to_gt_track_iscrowd'][cls_id]
+ data['dt_tracks'] = raw_data['classes_to_dt_tracks'][cls_id]
+ data['dt_track_ids'] = raw_data['classes_to_dt_track_ids'][cls_id]
+ data['dt_track_areas'] = raw_data['classes_to_dt_track_areas'][cls_id]
+ data['dt_track_scores'] = raw_data['classes_to_dt_track_scores'][cls_id]
+ data['iou_type'] = 'mask'
+
+ # sort tracker data tracks by tracker confidence scores
+ if data['dt_tracks']:
+ idx = np.argsort([-score for score in data['dt_track_scores']], kind="mergesort")
+ data['dt_track_scores'] = [data['dt_track_scores'][i] for i in idx]
+ data['dt_tracks'] = [data['dt_tracks'][i] for i in idx]
+ data['dt_track_ids'] = [data['dt_track_ids'][i] for i in idx]
+ data['dt_track_areas'] = [data['dt_track_areas'][i] for i in idx]
+
+ return data
+
+ def _calculate_similarities(self, gt_dets_t, tracker_dets_t):
+ similarity_scores = self._calculate_mask_ious(gt_dets_t, tracker_dets_t, is_encoded=True, do_ioa=False)
+ return similarity_scores
+
+ def _prepare_gt_annotations(self):
+ """
+ Prepares GT data by rle encoding segmentations and computing the average track area.
+ :return: None
+ """
+ # only loaded when needed to reduce minimum requirements
+ from pycocotools import mask as mask_utils
+
+ for track in self.gt_data['annotations']:
+ h = track['height']
+ w = track['width']
+ for i, seg in enumerate(track['segmentations']):
+ if seg:
+ track['segmentations'][i] = mask_utils.frPyObjects(seg, h, w)
+ areas = [a for a in track['areas'] if a]
+ if len(areas) == 0:
+ track['area'] = 0
+ else:
+ track['area'] = np.array(areas).mean()
+
+ def _get_tracker_seq_tracks(self, tracker, seq_id):
+ """
+ Prepares tracker data for a given sequence. Extracts all annotations for given sequence ID, computes
+ average track area and assigns a track ID.
+ :param tracker: the given tracker
+ :param seq_id: the sequence ID
+ :return: the extracted tracks
+ """
+ # only loaded when needed to reduce minimum requirements
+ from pycocotools import mask as mask_utils
+
+ tracks = [ann for ann in self.tracker_data[tracker] if ann['video_id'] == seq_id]
+ for track in tracks:
+ track['areas'] = []
+ for seg in track['segmentations']:
+ if seg:
+ track['areas'].append(mask_utils.area(seg))
+ else:
+ track['areas'].append(None)
+ areas = [a for a in track['areas'] if a]
+ if len(areas) == 0:
+ track['area'] = 0
+ else:
+ track['area'] = np.array(areas).mean()
+ track['id'] = self.global_tid_counter
+ self.global_tid_counter += 1
+ return tracks
diff --git a/val_utils/trackeval/eval.py b/val_utils/trackeval/eval.py
new file mode 100644
index 0000000000000000000000000000000000000000..82d62a016d69f1786604cf5809b639d993ea7660
--- /dev/null
+++ b/val_utils/trackeval/eval.py
@@ -0,0 +1,225 @@
+import time
+import traceback
+from multiprocessing.pool import Pool
+from functools import partial
+import os
+from . import utils
+from .utils import TrackEvalException
+from . import _timing
+from .metrics import Count
+
+try:
+ import tqdm
+ TQDM_IMPORTED = True
+except ImportError as _:
+ TQDM_IMPORTED = False
+
+
+class Evaluator:
+ """Evaluator class for evaluating different metrics for different datasets"""
+
+ @staticmethod
+ def get_default_eval_config():
+ """Returns the default config values for evaluation"""
+ code_path = utils.get_code_path()
+ default_config = {
+ 'USE_PARALLEL': False,
+ 'NUM_PARALLEL_CORES': 8,
+ 'BREAK_ON_ERROR': True, # Raises exception and exits with error
+ 'RETURN_ON_ERROR': False, # if not BREAK_ON_ERROR, then returns from function on error
+ 'LOG_ON_ERROR': os.path.join(code_path, 'error_log.txt'), # if not None, save any errors into a log file.
+
+ 'PRINT_RESULTS': True,
+ 'PRINT_ONLY_COMBINED': False,
+ 'PRINT_CONFIG': True,
+ 'TIME_PROGRESS': True,
+ 'DISPLAY_LESS_PROGRESS': True,
+
+ 'OUTPUT_SUMMARY': True,
+ 'OUTPUT_EMPTY_CLASSES': True, # If False, summary files are not output for classes with no detections
+ 'OUTPUT_DETAILED': True,
+ 'PLOT_CURVES': True,
+ }
+ return default_config
+
+ def __init__(self, config=None):
+ """Initialise the evaluator with a config file"""
+ self.config = utils.init_config(config, self.get_default_eval_config(), 'Eval')
+ # Only run timing analysis if not run in parallel.
+ if self.config['TIME_PROGRESS'] and not self.config['USE_PARALLEL']:
+ _timing.DO_TIMING = True
+ if self.config['DISPLAY_LESS_PROGRESS']:
+ _timing.DISPLAY_LESS_PROGRESS = True
+
+ @_timing.time
+ def evaluate(self, dataset_list, metrics_list, show_progressbar=False):
+ """Evaluate a set of metrics on a set of datasets"""
+ config = self.config
+ metrics_list = metrics_list + [Count()] # Count metrics are always run
+ metric_names = utils.validate_metrics_list(metrics_list)
+ dataset_names = [dataset.get_name() for dataset in dataset_list]
+ output_res = {}
+ output_msg = {}
+
+ for dataset, dataset_name in zip(dataset_list, dataset_names):
+ # Get dataset info about what to evaluate
+ output_res[dataset_name] = {}
+ output_msg[dataset_name] = {}
+ tracker_list, seq_list, class_list = dataset.get_eval_info()
+ print('\nEvaluating %i tracker(s) on %i sequence(s) for %i class(es) on %s dataset using the following '
+ 'metrics: %s\n' % (len(tracker_list), len(seq_list), len(class_list), dataset_name,
+ ', '.join(metric_names)))
+
+ # Evaluate each tracker
+ for tracker in tracker_list:
+ # if not config['BREAK_ON_ERROR'] then go to next tracker without breaking
+ try:
+ # Evaluate each sequence in parallel or in series.
+ # returns a nested dict (res), indexed like: res[seq][class][metric_name][sub_metric field]
+ # e.g. res[seq_0001][pedestrian][hota][DetA]
+ print('\nEvaluating %s\n' % tracker)
+ time_start = time.time()
+ if config['USE_PARALLEL']:
+ if show_progressbar and TQDM_IMPORTED:
+ seq_list_sorted = sorted(seq_list)
+
+ with Pool(config['NUM_PARALLEL_CORES']) as pool, tqdm.tqdm(total=len(seq_list)) as pbar:
+ _eval_sequence = partial(eval_sequence, dataset=dataset, tracker=tracker,
+ class_list=class_list, metrics_list=metrics_list,
+ metric_names=metric_names)
+ results = []
+ for r in pool.imap(_eval_sequence, seq_list_sorted,
+ chunksize=20):
+ results.append(r)
+ pbar.update()
+ res = dict(zip(seq_list_sorted, results))
+
+ else:
+ with Pool(config['NUM_PARALLEL_CORES']) as pool:
+ _eval_sequence = partial(eval_sequence, dataset=dataset, tracker=tracker,
+ class_list=class_list, metrics_list=metrics_list,
+ metric_names=metric_names)
+ results = pool.map(_eval_sequence, seq_list)
+ res = dict(zip(seq_list, results))
+ else:
+ res = {}
+ if show_progressbar and TQDM_IMPORTED:
+ seq_list_sorted = sorted(seq_list)
+ for curr_seq in tqdm.tqdm(seq_list_sorted):
+ res[curr_seq] = eval_sequence(curr_seq, dataset, tracker, class_list, metrics_list,
+ metric_names)
+ else:
+ for curr_seq in sorted(seq_list):
+ res[curr_seq] = eval_sequence(curr_seq, dataset, tracker, class_list, metrics_list,
+ metric_names)
+
+ # Combine results over all sequences and then over all classes
+
+ # collecting combined cls keys (cls averaged, det averaged, super classes)
+ combined_cls_keys = []
+ res['COMBINED_SEQ'] = {}
+ # combine sequences for each class
+ for c_cls in class_list:
+ res['COMBINED_SEQ'][c_cls] = {}
+ for metric, metric_name in zip(metrics_list, metric_names):
+ curr_res = {seq_key: seq_value[c_cls][metric_name] for seq_key, seq_value in res.items() if
+ seq_key != 'COMBINED_SEQ'}
+ res['COMBINED_SEQ'][c_cls][metric_name] = metric.combine_sequences(curr_res)
+ # combine classes
+ if dataset.should_classes_combine:
+ combined_cls_keys += ['cls_comb_cls_av', 'cls_comb_det_av', 'all']
+ res['COMBINED_SEQ']['cls_comb_cls_av'] = {}
+ res['COMBINED_SEQ']['cls_comb_det_av'] = {}
+ for metric, metric_name in zip(metrics_list, metric_names):
+ cls_res = {cls_key: cls_value[metric_name] for cls_key, cls_value in
+ res['COMBINED_SEQ'].items() if cls_key not in combined_cls_keys}
+ res['COMBINED_SEQ']['cls_comb_cls_av'][metric_name] = \
+ metric.combine_classes_class_averaged(cls_res)
+ res['COMBINED_SEQ']['cls_comb_det_av'][metric_name] = \
+ metric.combine_classes_det_averaged(cls_res)
+ # combine classes to super classes
+ if dataset.use_super_categories:
+ for cat, sub_cats in dataset.super_categories.items():
+ combined_cls_keys.append(cat)
+ res['COMBINED_SEQ'][cat] = {}
+ for metric, metric_name in zip(metrics_list, metric_names):
+ cat_res = {cls_key: cls_value[metric_name] for cls_key, cls_value in
+ res['COMBINED_SEQ'].items() if cls_key in sub_cats}
+ res['COMBINED_SEQ'][cat][metric_name] = metric.combine_classes_det_averaged(cat_res)
+
+ # Print and output results in various formats
+ if config['TIME_PROGRESS']:
+ print('\nAll sequences for %s finished in %.2f seconds' % (tracker, time.time() - time_start))
+ output_fol = dataset.get_output_fol(tracker)
+ tracker_display_name = dataset.get_display_name(tracker)
+ for c_cls in res['COMBINED_SEQ'].keys(): # class_list + combined classes if calculated
+ summaries = []
+ details = []
+ num_dets = res['COMBINED_SEQ'][c_cls]['Count']['Dets']
+ if config['OUTPUT_EMPTY_CLASSES'] or num_dets > 0:
+ for metric, metric_name in zip(metrics_list, metric_names):
+ # for combined classes there is no per sequence evaluation
+ if c_cls in combined_cls_keys:
+ table_res = {'COMBINED_SEQ': res['COMBINED_SEQ'][c_cls][metric_name]}
+ else:
+ table_res = {seq_key: seq_value[c_cls][metric_name] for seq_key, seq_value
+ in res.items()}
+
+ if config['PRINT_RESULTS'] and config['PRINT_ONLY_COMBINED']:
+ dont_print = dataset.should_classes_combine and c_cls not in combined_cls_keys
+ if not dont_print:
+ metric.print_table({'COMBINED_SEQ': table_res['COMBINED_SEQ']},
+ tracker_display_name, c_cls)
+ elif config['PRINT_RESULTS']:
+ metric.print_table(table_res, tracker_display_name, c_cls)
+ if config['OUTPUT_SUMMARY']:
+ summaries.append(metric.summary_results(table_res))
+ if config['OUTPUT_DETAILED']:
+ details.append(metric.detailed_results(table_res))
+ if config['PLOT_CURVES']:
+ metric.plot_single_tracker_results(table_res, tracker_display_name, c_cls,
+ output_fol)
+ if config['OUTPUT_SUMMARY']:
+ utils.write_summary_results(summaries, c_cls, output_fol)
+ if config['OUTPUT_DETAILED']:
+ utils.write_detailed_results(details, c_cls, output_fol)
+
+ # Output for returning from function
+ output_res[dataset_name][tracker] = res
+ output_msg[dataset_name][tracker] = 'Success'
+
+ except Exception as err:
+ output_res[dataset_name][tracker] = None
+ if type(err) == TrackEvalException:
+ output_msg[dataset_name][tracker] = str(err)
+ else:
+ output_msg[dataset_name][tracker] = 'Unknown error occurred.'
+ print('Tracker %s was unable to be evaluated.' % tracker)
+ print(err)
+ traceback.print_exc()
+ if config['LOG_ON_ERROR'] is not None:
+ with open(config['LOG_ON_ERROR'], 'a') as f:
+ print(dataset_name, file=f)
+ print(tracker, file=f)
+ print(traceback.format_exc(), file=f)
+ print('\n\n\n', file=f)
+ if config['BREAK_ON_ERROR']:
+ raise err
+ elif config['RETURN_ON_ERROR']:
+ return output_res, output_msg
+
+ return output_res, output_msg
+
+
+@_timing.time
+def eval_sequence(seq, dataset, tracker, class_list, metrics_list, metric_names):
+ """Function for evaluating a single sequence"""
+
+ raw_data = dataset.get_raw_seq_data(tracker, seq)
+ seq_res = {}
+ for cls in class_list:
+ seq_res[cls] = {}
+ data = dataset.get_preprocessed_seq_data(raw_data, cls)
+ for metric, met_name in zip(metrics_list, metric_names):
+ seq_res[cls][met_name] = metric.eval_sequence(data)
+ return seq_res
diff --git a/val_utils/trackeval/metrics/__init__.py b/val_utils/trackeval/metrics/__init__.py
new file mode 100644
index 0000000000000000000000000000000000000000..1f84774682aa5ca52e98ae4424226bc3d802d3e3
--- /dev/null
+++ b/val_utils/trackeval/metrics/__init__.py
@@ -0,0 +1,8 @@
+from .hota import HOTA
+from .clear import CLEAR
+from .identity import Identity
+from .count import Count
+from .j_and_f import JAndF
+from .track_map import TrackMAP
+from .vace import VACE
+from .ideucl import IDEucl
\ No newline at end of file
diff --git a/val_utils/trackeval/metrics/_base_metric.py b/val_utils/trackeval/metrics/_base_metric.py
new file mode 100644
index 0000000000000000000000000000000000000000..ea488850c80bd1bfc7d37bea7456492d15b3641f
--- /dev/null
+++ b/val_utils/trackeval/metrics/_base_metric.py
@@ -0,0 +1,133 @@
+
+import numpy as np
+from abc import ABC, abstractmethod
+from .. import _timing
+from ..utils import TrackEvalException
+
+
+class _BaseMetric(ABC):
+ @abstractmethod
+ def __init__(self):
+ self.plottable = False
+ self.integer_fields = []
+ self.float_fields = []
+ self.array_labels = []
+ self.integer_array_fields = []
+ self.float_array_fields = []
+ self.fields = []
+ self.summary_fields = []
+ self.registered = False
+
+ #####################################################################
+ # Abstract functions for subclasses to implement
+
+ @_timing.time
+ @abstractmethod
+ def eval_sequence(self, data):
+ ...
+
+ @abstractmethod
+ def combine_sequences(self, all_res):
+ ...
+
+ @abstractmethod
+ def combine_classes_class_averaged(self, all_res, ignore_empty_classes=False):
+ ...
+
+ @ abstractmethod
+ def combine_classes_det_averaged(self, all_res):
+ ...
+
+ def plot_single_tracker_results(self, all_res, tracker, output_folder, cls):
+ """Plot results of metrics, only valid for metrics with self.plottable"""
+ if self.plottable:
+ raise NotImplementedError('plot_results is not implemented for metric %s' % self.get_name())
+ else:
+ pass
+
+ #####################################################################
+ # Helper functions which are useful for all metrics:
+
+ @classmethod
+ def get_name(cls):
+ return cls.__name__
+
+ @staticmethod
+ def _combine_sum(all_res, field):
+ """Combine sequence results via sum"""
+ return sum([all_res[k][field] for k in all_res.keys()])
+
+ @staticmethod
+ def _combine_weighted_av(all_res, field, comb_res, weight_field):
+ """Combine sequence results via weighted average"""
+ return sum([all_res[k][field] * all_res[k][weight_field] for k in all_res.keys()]) / np.maximum(1.0, comb_res[
+ weight_field])
+
+ def print_table(self, table_res, tracker, cls):
+ """Prints table of results for all sequences"""
+ print('')
+ metric_name = self.get_name()
+ self._row_print([metric_name + ': ' + tracker + '-' + cls] + self.summary_fields)
+ for seq, results in sorted(table_res.items()):
+ if seq == 'COMBINED_SEQ':
+ continue
+ summary_res = self._summary_row(results)
+ self._row_print([seq] + summary_res)
+ summary_res = self._summary_row(table_res['COMBINED_SEQ'])
+ self._row_print(['COMBINED'] + summary_res)
+
+ def _summary_row(self, results_):
+ vals = []
+ for h in self.summary_fields:
+ if h in self.float_array_fields:
+ vals.append("{0:1.5g}".format(100 * np.mean(results_[h])))
+ elif h in self.float_fields:
+ vals.append("{0:1.5g}".format(100 * float(results_[h])))
+ elif h in self.integer_fields:
+ vals.append("{0:d}".format(int(results_[h])))
+ else:
+ raise NotImplementedError("Summary function not implemented for this field type.")
+ return vals
+
+ @staticmethod
+ def _row_print(*argv):
+ """Prints results in an evenly spaced rows, with more space in first row"""
+ if len(argv) == 1:
+ argv = argv[0]
+ to_print = '%-35s' % argv[0]
+ for v in argv[1:]:
+ to_print += '%-10s' % str(v)
+ print(to_print)
+
+ def summary_results(self, table_res):
+ """Returns a simple summary of final results for a tracker"""
+ return dict(zip(self.summary_fields, self._summary_row(table_res['COMBINED_SEQ'])))
+
+ def detailed_results(self, table_res):
+ """Returns detailed final results for a tracker"""
+ # Get detailed field information
+ detailed_fields = self.float_fields + self.integer_fields
+ for h in self.float_array_fields + self.integer_array_fields:
+ for alpha in [int(100*x) for x in self.array_labels]:
+ detailed_fields.append(h + '___' + str(alpha))
+ detailed_fields.append(h + '___AUC')
+
+ # Get detailed results
+ detailed_results = {}
+ for seq, res in table_res.items():
+ detailed_row = self._detailed_row(res)
+ if len(detailed_row) != len(detailed_fields):
+ raise TrackEvalException(
+ 'Field names and data have different sizes (%i and %i)' % (len(detailed_row), len(detailed_fields)))
+ detailed_results[seq] = dict(zip(detailed_fields, detailed_row))
+ return detailed_results
+
+ def _detailed_row(self, res):
+ detailed_row = []
+ for h in self.float_fields + self.integer_fields:
+ detailed_row.append(res[h])
+ for h in self.float_array_fields + self.integer_array_fields:
+ for i, alpha in enumerate([int(100 * x) for x in self.array_labels]):
+ detailed_row.append(res[h][i])
+ detailed_row.append(np.mean(res[h]))
+ return detailed_row
diff --git a/val_utils/trackeval/metrics/clear.py b/val_utils/trackeval/metrics/clear.py
new file mode 100644
index 0000000000000000000000000000000000000000..8b5e291fc0c5375efa8b548c3ad81bad73865b1c
--- /dev/null
+++ b/val_utils/trackeval/metrics/clear.py
@@ -0,0 +1,186 @@
+
+import numpy as np
+from scipy.optimize import linear_sum_assignment
+from ._base_metric import _BaseMetric
+from .. import _timing
+from .. import utils
+
+class CLEAR(_BaseMetric):
+ """Class which implements the CLEAR metrics"""
+
+ @staticmethod
+ def get_default_config():
+ """Default class config values"""
+ default_config = {
+ 'THRESHOLD': 0.5, # Similarity score threshold required for a TP match. Default 0.5.
+ 'PRINT_CONFIG': True, # Whether to print the config information on init. Default: False.
+ }
+ return default_config
+
+ def __init__(self, config=None):
+ super().__init__()
+ main_integer_fields = ['CLR_TP', 'CLR_FN', 'CLR_FP', 'IDSW', 'MT', 'PT', 'ML', 'Frag']
+ extra_integer_fields = ['CLR_Frames']
+ self.integer_fields = main_integer_fields + extra_integer_fields
+ main_float_fields = ['MOTA', 'MOTP', 'MODA', 'CLR_Re', 'CLR_Pr', 'MTR', 'PTR', 'MLR', 'sMOTA']
+ extra_float_fields = ['CLR_F1', 'FP_per_frame', 'MOTAL', 'MOTP_sum']
+ self.float_fields = main_float_fields + extra_float_fields
+ self.fields = self.float_fields + self.integer_fields
+ self.summed_fields = self.integer_fields + ['MOTP_sum']
+ self.summary_fields = main_float_fields + main_integer_fields
+
+ # Configuration options:
+ self.config = utils.init_config(config, self.get_default_config(), self.get_name())
+ self.threshold = float(self.config['THRESHOLD'])
+
+
+ @_timing.time
+ def eval_sequence(self, data):
+ """Calculates CLEAR metrics for one sequence"""
+ # Initialise results
+ res = {}
+ for field in self.fields:
+ res[field] = 0
+
+ # Return result quickly if tracker or gt sequence is empty
+ if data['num_tracker_dets'] == 0:
+ res['CLR_FN'] = data['num_gt_dets']
+ res['ML'] = data['num_gt_ids']
+ res['MLR'] = 1.0
+ return res
+ if data['num_gt_dets'] == 0:
+ res['CLR_FP'] = data['num_tracker_dets']
+ res['MLR'] = 1.0
+ return res
+
+ # Variables counting global association
+ num_gt_ids = data['num_gt_ids']
+ gt_id_count = np.zeros(num_gt_ids) # For MT/ML/PT
+ gt_matched_count = np.zeros(num_gt_ids) # For MT/ML/PT
+ gt_frag_count = np.zeros(num_gt_ids) # For Frag
+
+ # Note that IDSWs are counted based on the last time each gt_id was present (any number of frames previously),
+ # but are only used in matching to continue current tracks based on the gt_id in the single previous timestep.
+ prev_tracker_id = np.nan * np.zeros(num_gt_ids) # For scoring IDSW
+ prev_timestep_tracker_id = np.nan * np.zeros(num_gt_ids) # For matching IDSW
+
+ # Calculate scores for each timestep
+ for t, (gt_ids_t, tracker_ids_t) in enumerate(zip(data['gt_ids'], data['tracker_ids'])):
+ # Deal with the case that there are no gt_det/tracker_det in a timestep.
+ if len(gt_ids_t) == 0:
+ res['CLR_FP'] += len(tracker_ids_t)
+ continue
+ if len(tracker_ids_t) == 0:
+ res['CLR_FN'] += len(gt_ids_t)
+ gt_id_count[gt_ids_t] += 1
+ continue
+
+ # Calc score matrix to first minimise IDSWs from previous frame, and then maximise MOTP secondarily
+ similarity = data['similarity_scores'][t]
+ score_mat = (tracker_ids_t[np.newaxis, :] == prev_timestep_tracker_id[gt_ids_t[:, np.newaxis]])
+ score_mat = 1000 * score_mat + similarity
+ score_mat[similarity < self.threshold - np.finfo('float').eps] = 0
+
+ # Hungarian algorithm to find best matches
+ match_rows, match_cols = linear_sum_assignment(-score_mat)
+ actually_matched_mask = score_mat[match_rows, match_cols] > 0 + np.finfo('float').eps
+ match_rows = match_rows[actually_matched_mask]
+ match_cols = match_cols[actually_matched_mask]
+
+ matched_gt_ids = gt_ids_t[match_rows]
+ matched_tracker_ids = tracker_ids_t[match_cols]
+
+ # Calc IDSW for MOTA
+ prev_matched_tracker_ids = prev_tracker_id[matched_gt_ids]
+ is_idsw = (np.logical_not(np.isnan(prev_matched_tracker_ids))) & (
+ np.not_equal(matched_tracker_ids, prev_matched_tracker_ids))
+ res['IDSW'] += np.sum(is_idsw)
+
+ # Update counters for MT/ML/PT/Frag and record for IDSW/Frag for next timestep
+ gt_id_count[gt_ids_t] += 1
+ gt_matched_count[matched_gt_ids] += 1
+ not_previously_tracked = np.isnan(prev_timestep_tracker_id)
+ prev_tracker_id[matched_gt_ids] = matched_tracker_ids
+ prev_timestep_tracker_id[:] = np.nan
+ prev_timestep_tracker_id[matched_gt_ids] = matched_tracker_ids
+ currently_tracked = np.logical_not(np.isnan(prev_timestep_tracker_id))
+ gt_frag_count += np.logical_and(not_previously_tracked, currently_tracked)
+
+ # Calculate and accumulate basic statistics
+ num_matches = len(matched_gt_ids)
+ res['CLR_TP'] += num_matches
+ res['CLR_FN'] += len(gt_ids_t) - num_matches
+ res['CLR_FP'] += len(tracker_ids_t) - num_matches
+ if num_matches > 0:
+ res['MOTP_sum'] += sum(similarity[match_rows, match_cols])
+
+ # Calculate MT/ML/PT/Frag/MOTP
+ tracked_ratio = gt_matched_count[gt_id_count > 0] / gt_id_count[gt_id_count > 0]
+ res['MT'] = np.sum(np.greater(tracked_ratio, 0.8))
+ res['PT'] = np.sum(np.greater_equal(tracked_ratio, 0.2)) - res['MT']
+ res['ML'] = num_gt_ids - res['MT'] - res['PT']
+ res['Frag'] = np.sum(np.subtract(gt_frag_count[gt_frag_count > 0], 1))
+ res['MOTP'] = res['MOTP_sum'] / np.maximum(1.0, res['CLR_TP'])
+
+ res['CLR_Frames'] = data['num_timesteps']
+
+ # Calculate final CLEAR scores
+ res = self._compute_final_fields(res)
+ return res
+
+ def combine_sequences(self, all_res):
+ """Combines metrics across all sequences"""
+ res = {}
+ for field in self.summed_fields:
+ res[field] = self._combine_sum(all_res, field)
+ res = self._compute_final_fields(res)
+ return res
+
+ def combine_classes_det_averaged(self, all_res):
+ """Combines metrics across all classes by averaging over the detection values"""
+ res = {}
+ for field in self.summed_fields:
+ res[field] = self._combine_sum(all_res, field)
+ res = self._compute_final_fields(res)
+ return res
+
+ def combine_classes_class_averaged(self, all_res, ignore_empty_classes=False):
+ """Combines metrics across all classes by averaging over the class values.
+ If 'ignore_empty_classes' is True, then it only sums over classes with at least one gt or predicted detection.
+ """
+ res = {}
+ for field in self.integer_fields:
+ if ignore_empty_classes:
+ res[field] = self._combine_sum(
+ {k: v for k, v in all_res.items() if v['CLR_TP'] + v['CLR_FN'] + v['CLR_FP'] > 0}, field)
+ else:
+ res[field] = self._combine_sum({k: v for k, v in all_res.items()}, field)
+ for field in self.float_fields:
+ if ignore_empty_classes:
+ res[field] = np.mean(
+ [v[field] for v in all_res.values() if v['CLR_TP'] + v['CLR_FN'] + v['CLR_FP'] > 0], axis=0)
+ else:
+ res[field] = np.mean([v[field] for v in all_res.values()], axis=0)
+ return res
+
+ @staticmethod
+ def _compute_final_fields(res):
+ """Calculate sub-metric ('field') values which only depend on other sub-metric values.
+ This function is used both for both per-sequence calculation, and in combining values across sequences.
+ """
+ num_gt_ids = res['MT'] + res['ML'] + res['PT']
+ res['MTR'] = res['MT'] / np.maximum(1.0, num_gt_ids)
+ res['MLR'] = res['ML'] / np.maximum(1.0, num_gt_ids)
+ res['PTR'] = res['PT'] / np.maximum(1.0, num_gt_ids)
+ res['CLR_Re'] = res['CLR_TP'] / np.maximum(1.0, res['CLR_TP'] + res['CLR_FN'])
+ res['CLR_Pr'] = res['CLR_TP'] / np.maximum(1.0, res['CLR_TP'] + res['CLR_FP'])
+ res['MODA'] = (res['CLR_TP'] - res['CLR_FP']) / np.maximum(1.0, res['CLR_TP'] + res['CLR_FN'])
+ res['MOTA'] = (res['CLR_TP'] - res['CLR_FP'] - res['IDSW']) / np.maximum(1.0, res['CLR_TP'] + res['CLR_FN'])
+ res['MOTP'] = res['MOTP_sum'] / np.maximum(1.0, res['CLR_TP'])
+ res['sMOTA'] = (res['MOTP_sum'] - res['CLR_FP'] - res['IDSW']) / np.maximum(1.0, res['CLR_TP'] + res['CLR_FN'])
+
+ res['CLR_F1'] = res['CLR_TP'] / np.maximum(1.0, res['CLR_TP'] + 0.5*res['CLR_FN'] + 0.5*res['CLR_FP'])
+ res['FP_per_frame'] = res['CLR_FP'] / np.maximum(1.0, res['CLR_Frames'])
+ safe_log_idsw = np.log10(res['IDSW']) if res['IDSW'] > 0 else res['IDSW']
+ res['MOTAL'] = (res['CLR_TP'] - res['CLR_FP'] - safe_log_idsw) / np.maximum(1.0, res['CLR_TP'] + res['CLR_FN'])
+ return res
diff --git a/val_utils/trackeval/metrics/count.py b/val_utils/trackeval/metrics/count.py
new file mode 100644
index 0000000000000000000000000000000000000000..49049b104f5eff57c661b3ff63fbc7c655cffe86
--- /dev/null
+++ b/val_utils/trackeval/metrics/count.py
@@ -0,0 +1,44 @@
+
+from ._base_metric import _BaseMetric
+from .. import _timing
+
+
+class Count(_BaseMetric):
+ """Class which simply counts the number of tracker and gt detections and ids."""
+ def __init__(self, config=None):
+ super().__init__()
+ self.integer_fields = ['Dets', 'GT_Dets', 'IDs', 'GT_IDs']
+ self.fields = self.integer_fields
+ self.summary_fields = self.fields
+
+ @_timing.time
+ def eval_sequence(self, data):
+ """Returns counts for one sequence"""
+ # Get results
+ res = {'Dets': data['num_tracker_dets'],
+ 'GT_Dets': data['num_gt_dets'],
+ 'IDs': data['num_tracker_ids'],
+ 'GT_IDs': data['num_gt_ids'],
+ 'Frames': data['num_timesteps']}
+ return res
+
+ def combine_sequences(self, all_res):
+ """Combines metrics across all sequences"""
+ res = {}
+ for field in self.integer_fields:
+ res[field] = self._combine_sum(all_res, field)
+ return res
+
+ def combine_classes_class_averaged(self, all_res, ignore_empty_classes=None):
+ """Combines metrics across all classes by averaging over the class values"""
+ res = {}
+ for field in self.integer_fields:
+ res[field] = self._combine_sum(all_res, field)
+ return res
+
+ def combine_classes_det_averaged(self, all_res):
+ """Combines metrics across all classes by averaging over the detection values"""
+ res = {}
+ for field in self.integer_fields:
+ res[field] = self._combine_sum(all_res, field)
+ return res
diff --git a/val_utils/trackeval/metrics/hota.py b/val_utils/trackeval/metrics/hota.py
new file mode 100644
index 0000000000000000000000000000000000000000..f551b766d1b4c3d4f4854bf99b04877dc1fa7c32
--- /dev/null
+++ b/val_utils/trackeval/metrics/hota.py
@@ -0,0 +1,203 @@
+
+import os
+import numpy as np
+from scipy.optimize import linear_sum_assignment
+from ._base_metric import _BaseMetric
+from .. import _timing
+
+
+class HOTA(_BaseMetric):
+ """Class which implements the HOTA metrics.
+ See: https://link.springer.com/article/10.1007/s11263-020-01375-2
+ """
+
+ def __init__(self, config=None):
+ super().__init__()
+ self.plottable = True
+ self.array_labels = np.arange(0.05, 0.99, 0.05)
+ self.integer_array_fields = ['HOTA_TP', 'HOTA_FN', 'HOTA_FP']
+ self.float_array_fields = ['HOTA', 'DetA', 'AssA', 'DetRe', 'DetPr', 'AssRe', 'AssPr', 'LocA', 'OWTA']
+ self.float_fields = ['HOTA(0)', 'LocA(0)', 'HOTALocA(0)']
+ self.fields = self.float_array_fields + self.integer_array_fields + self.float_fields
+ self.summary_fields = self.float_array_fields + self.float_fields
+
+ @_timing.time
+ def eval_sequence(self, data):
+ """Calculates the HOTA metrics for one sequence"""
+
+ # Initialise results
+ res = {}
+ for field in self.float_array_fields + self.integer_array_fields:
+ res[field] = np.zeros((len(self.array_labels)), dtype=np.float)
+ for field in self.float_fields:
+ res[field] = 0
+
+ # Return result quickly if tracker or gt sequence is empty
+ if data['num_tracker_dets'] == 0:
+ res['HOTA_FN'] = data['num_gt_dets'] * np.ones((len(self.array_labels)), dtype=np.float)
+ res['LocA'] = np.ones((len(self.array_labels)), dtype=np.float)
+ res['LocA(0)'] = 1.0
+ return res
+ if data['num_gt_dets'] == 0:
+ res['HOTA_FP'] = data['num_tracker_dets'] * np.ones((len(self.array_labels)), dtype=np.float)
+ res['LocA'] = np.ones((len(self.array_labels)), dtype=np.float)
+ res['LocA(0)'] = 1.0
+ return res
+
+ # Variables counting global association
+ potential_matches_count = np.zeros((data['num_gt_ids'], data['num_tracker_ids']))
+ gt_id_count = np.zeros((data['num_gt_ids'], 1))
+ tracker_id_count = np.zeros((1, data['num_tracker_ids']))
+
+ # First loop through each timestep and accumulate global track information.
+ for t, (gt_ids_t, tracker_ids_t) in enumerate(zip(data['gt_ids'], data['tracker_ids'])):
+ # Count the potential matches between ids in each timestep
+ # These are normalised, weighted by the match similarity.
+ similarity = data['similarity_scores'][t]
+ sim_iou_denom = similarity.sum(0)[np.newaxis, :] + similarity.sum(1)[:, np.newaxis] - similarity
+ sim_iou = np.zeros_like(similarity)
+ sim_iou_mask = sim_iou_denom > 0 + np.finfo('float').eps
+ sim_iou[sim_iou_mask] = similarity[sim_iou_mask] / sim_iou_denom[sim_iou_mask]
+ potential_matches_count[gt_ids_t[:, np.newaxis], tracker_ids_t[np.newaxis, :]] += sim_iou
+
+ # Calculate the total number of dets for each gt_id and tracker_id.
+ gt_id_count[gt_ids_t] += 1
+ tracker_id_count[0, tracker_ids_t] += 1
+
+ # Calculate overall jaccard alignment score (before unique matching) between IDs
+ global_alignment_score = potential_matches_count / (gt_id_count + tracker_id_count - potential_matches_count)
+ matches_counts = [np.zeros_like(potential_matches_count) for _ in self.array_labels]
+
+ # Calculate scores for each timestep
+ for t, (gt_ids_t, tracker_ids_t) in enumerate(zip(data['gt_ids'], data['tracker_ids'])):
+ # Deal with the case that there are no gt_det/tracker_det in a timestep.
+ if len(gt_ids_t) == 0:
+ for a, alpha in enumerate(self.array_labels):
+ res['HOTA_FP'][a] += len(tracker_ids_t)
+ continue
+ if len(tracker_ids_t) == 0:
+ for a, alpha in enumerate(self.array_labels):
+ res['HOTA_FN'][a] += len(gt_ids_t)
+ continue
+
+ # Get matching scores between pairs of dets for optimizing HOTA
+ similarity = data['similarity_scores'][t]
+ score_mat = global_alignment_score[gt_ids_t[:, np.newaxis], tracker_ids_t[np.newaxis, :]] * similarity
+
+ # Hungarian algorithm to find best matches
+ match_rows, match_cols = linear_sum_assignment(-score_mat)
+
+ # Calculate and accumulate basic statistics
+ for a, alpha in enumerate(self.array_labels):
+ actually_matched_mask = similarity[match_rows, match_cols] >= alpha - np.finfo('float').eps
+ alpha_match_rows = match_rows[actually_matched_mask]
+ alpha_match_cols = match_cols[actually_matched_mask]
+ num_matches = len(alpha_match_rows)
+ res['HOTA_TP'][a] += num_matches
+ res['HOTA_FN'][a] += len(gt_ids_t) - num_matches
+ res['HOTA_FP'][a] += len(tracker_ids_t) - num_matches
+ if num_matches > 0:
+ res['LocA'][a] += sum(similarity[alpha_match_rows, alpha_match_cols])
+ matches_counts[a][gt_ids_t[alpha_match_rows], tracker_ids_t[alpha_match_cols]] += 1
+
+ # Calculate association scores (AssA, AssRe, AssPr) for the alpha value.
+ # First calculate scores per gt_id/tracker_id combo and then average over the number of detections.
+ for a, alpha in enumerate(self.array_labels):
+ matches_count = matches_counts[a]
+ ass_a = matches_count / np.maximum(1, gt_id_count + tracker_id_count - matches_count)
+ res['AssA'][a] = np.sum(matches_count * ass_a) / np.maximum(1, res['HOTA_TP'][a])
+ ass_re = matches_count / np.maximum(1, gt_id_count)
+ res['AssRe'][a] = np.sum(matches_count * ass_re) / np.maximum(1, res['HOTA_TP'][a])
+ ass_pr = matches_count / np.maximum(1, tracker_id_count)
+ res['AssPr'][a] = np.sum(matches_count * ass_pr) / np.maximum(1, res['HOTA_TP'][a])
+
+ # Calculate final scores
+ res['LocA'] = np.maximum(1e-10, res['LocA']) / np.maximum(1e-10, res['HOTA_TP'])
+ res = self._compute_final_fields(res)
+ return res
+
+ def combine_sequences(self, all_res):
+ """Combines metrics across all sequences"""
+ res = {}
+ for field in self.integer_array_fields:
+ res[field] = self._combine_sum(all_res, field)
+ for field in ['AssRe', 'AssPr', 'AssA']:
+ res[field] = self._combine_weighted_av(all_res, field, res, weight_field='HOTA_TP')
+ loca_weighted_sum = sum([all_res[k]['LocA'] * all_res[k]['HOTA_TP'] for k in all_res.keys()])
+ res['LocA'] = np.maximum(1e-10, loca_weighted_sum) / np.maximum(1e-10, res['HOTA_TP'])
+ res = self._compute_final_fields(res)
+ return res
+
+ def combine_classes_class_averaged(self, all_res, ignore_empty_classes=False):
+ """Combines metrics across all classes by averaging over the class values.
+ If 'ignore_empty_classes' is True, then it only sums over classes with at least one gt or predicted detection.
+ """
+ res = {}
+ for field in self.integer_array_fields:
+ if ignore_empty_classes:
+ res[field] = self._combine_sum(
+ {k: v for k, v in all_res.items()
+ if (v['HOTA_TP'] + v['HOTA_FN'] + v['HOTA_FP'] > 0 + np.finfo('float').eps).any()}, field)
+ else:
+ res[field] = self._combine_sum({k: v for k, v in all_res.items()}, field)
+
+ for field in self.float_fields + self.float_array_fields:
+ if ignore_empty_classes:
+ res[field] = np.mean([v[field] for v in all_res.values() if
+ (v['HOTA_TP'] + v['HOTA_FN'] + v['HOTA_FP'] > 0 + np.finfo('float').eps).any()],
+ axis=0)
+ else:
+ res[field] = np.mean([v[field] for v in all_res.values()], axis=0)
+ return res
+
+ def combine_classes_det_averaged(self, all_res):
+ """Combines metrics across all classes by averaging over the detection values"""
+ res = {}
+ for field in self.integer_array_fields:
+ res[field] = self._combine_sum(all_res, field)
+ for field in ['AssRe', 'AssPr', 'AssA']:
+ res[field] = self._combine_weighted_av(all_res, field, res, weight_field='HOTA_TP')
+ loca_weighted_sum = sum([all_res[k]['LocA'] * all_res[k]['HOTA_TP'] for k in all_res.keys()])
+ res['LocA'] = np.maximum(1e-10, loca_weighted_sum) / np.maximum(1e-10, res['HOTA_TP'])
+ res = self._compute_final_fields(res)
+ return res
+
+ @staticmethod
+ def _compute_final_fields(res):
+ """Calculate sub-metric ('field') values which only depend on other sub-metric values.
+ This function is used both for both per-sequence calculation, and in combining values across sequences.
+ """
+ res['DetRe'] = res['HOTA_TP'] / np.maximum(1, res['HOTA_TP'] + res['HOTA_FN'])
+ res['DetPr'] = res['HOTA_TP'] / np.maximum(1, res['HOTA_TP'] + res['HOTA_FP'])
+ res['DetA'] = res['HOTA_TP'] / np.maximum(1, res['HOTA_TP'] + res['HOTA_FN'] + res['HOTA_FP'])
+ res['HOTA'] = np.sqrt(res['DetA'] * res['AssA'])
+ res['OWTA'] = np.sqrt(res['DetRe'] * res['AssA'])
+
+ res['HOTA(0)'] = res['HOTA'][0]
+ res['LocA(0)'] = res['LocA'][0]
+ res['HOTALocA(0)'] = res['HOTA(0)']*res['LocA(0)']
+ return res
+
+ def plot_single_tracker_results(self, table_res, tracker, cls, output_folder):
+ """Create plot of results"""
+
+ # Only loaded when run to reduce minimum requirements
+ from matplotlib import pyplot as plt
+
+ res = table_res['COMBINED_SEQ']
+ styles_to_plot = ['r', 'b', 'g', 'b--', 'b:', 'g--', 'g:', 'm']
+ for name, style in zip(self.float_array_fields, styles_to_plot):
+ plt.plot(self.array_labels, res[name], style)
+ plt.xlabel('alpha')
+ plt.ylabel('score')
+ plt.title(tracker + ' - ' + cls)
+ plt.axis([0, 1, 0, 1])
+ legend = []
+ for name in self.float_array_fields:
+ legend += [name + ' (' + str(np.round(np.mean(res[name]), 2)) + ')']
+ plt.legend(legend, loc='lower left')
+ out_file = os.path.join(output_folder, cls + '_plot.pdf')
+ os.makedirs(os.path.dirname(out_file), exist_ok=True)
+ plt.savefig(out_file)
+ plt.savefig(out_file.replace('.pdf', '.png'))
+ plt.clf()
diff --git a/val_utils/trackeval/metrics/identity.py b/val_utils/trackeval/metrics/identity.py
new file mode 100644
index 0000000000000000000000000000000000000000..c8c6c809989e5b620ceb3822941574018e605c70
--- /dev/null
+++ b/val_utils/trackeval/metrics/identity.py
@@ -0,0 +1,135 @@
+import numpy as np
+from scipy.optimize import linear_sum_assignment
+from ._base_metric import _BaseMetric
+from .. import _timing
+from .. import utils
+
+
+class Identity(_BaseMetric):
+ """Class which implements the ID metrics"""
+
+ @staticmethod
+ def get_default_config():
+ """Default class config values"""
+ default_config = {
+ 'THRESHOLD': 0.5, # Similarity score threshold required for a IDTP match. Default 0.5.
+ 'PRINT_CONFIG': True, # Whether to print the config information on init. Default: False.
+ }
+ return default_config
+
+ def __init__(self, config=None):
+ super().__init__()
+ self.integer_fields = ['IDTP', 'IDFN', 'IDFP']
+ self.float_fields = ['IDF1', 'IDR', 'IDP']
+ self.fields = self.float_fields + self.integer_fields
+ self.summary_fields = self.fields
+
+ # Configuration options:
+ self.config = utils.init_config(config, self.get_default_config(), self.get_name())
+ self.threshold = float(self.config['THRESHOLD'])
+
+ @_timing.time
+ def eval_sequence(self, data):
+ """Calculates ID metrics for one sequence"""
+ # Initialise results
+ res = {}
+ for field in self.fields:
+ res[field] = 0
+
+ # Return result quickly if tracker or gt sequence is empty
+ if data['num_tracker_dets'] == 0:
+ res['IDFN'] = data['num_gt_dets']
+ return res
+ if data['num_gt_dets'] == 0:
+ res['IDFP'] = data['num_tracker_dets']
+ return res
+
+ # Variables counting global association
+ potential_matches_count = np.zeros((data['num_gt_ids'], data['num_tracker_ids']))
+ gt_id_count = np.zeros(data['num_gt_ids'])
+ tracker_id_count = np.zeros(data['num_tracker_ids'])
+
+ # First loop through each timestep and accumulate global track information.
+ for t, (gt_ids_t, tracker_ids_t) in enumerate(zip(data['gt_ids'], data['tracker_ids'])):
+ # Count the potential matches between ids in each timestep
+ matches_mask = np.greater_equal(data['similarity_scores'][t], self.threshold)
+ match_idx_gt, match_idx_tracker = np.nonzero(matches_mask)
+ potential_matches_count[gt_ids_t[match_idx_gt], tracker_ids_t[match_idx_tracker]] += 1
+
+ # Calculate the total number of dets for each gt_id and tracker_id.
+ gt_id_count[gt_ids_t] += 1
+ tracker_id_count[tracker_ids_t] += 1
+
+ # Calculate optimal assignment cost matrix for ID metrics
+ num_gt_ids = data['num_gt_ids']
+ num_tracker_ids = data['num_tracker_ids']
+ fp_mat = np.zeros((num_gt_ids + num_tracker_ids, num_gt_ids + num_tracker_ids))
+ fn_mat = np.zeros((num_gt_ids + num_tracker_ids, num_gt_ids + num_tracker_ids))
+ fp_mat[num_gt_ids:, :num_tracker_ids] = 1e10
+ fn_mat[:num_gt_ids, num_tracker_ids:] = 1e10
+ for gt_id in range(num_gt_ids):
+ fn_mat[gt_id, :num_tracker_ids] = gt_id_count[gt_id]
+ fn_mat[gt_id, num_tracker_ids + gt_id] = gt_id_count[gt_id]
+ for tracker_id in range(num_tracker_ids):
+ fp_mat[:num_gt_ids, tracker_id] = tracker_id_count[tracker_id]
+ fp_mat[tracker_id + num_gt_ids, tracker_id] = tracker_id_count[tracker_id]
+ fn_mat[:num_gt_ids, :num_tracker_ids] -= potential_matches_count
+ fp_mat[:num_gt_ids, :num_tracker_ids] -= potential_matches_count
+
+ # Hungarian algorithm
+ match_rows, match_cols = linear_sum_assignment(fn_mat + fp_mat)
+
+ # Accumulate basic statistics
+ res['IDFN'] = fn_mat[match_rows, match_cols].sum().astype(np.int)
+ res['IDFP'] = fp_mat[match_rows, match_cols].sum().astype(np.int)
+ res['IDTP'] = (gt_id_count.sum() - res['IDFN']).astype(np.int)
+
+ # Calculate final ID scores
+ res = self._compute_final_fields(res)
+ return res
+
+ def combine_classes_class_averaged(self, all_res, ignore_empty_classes=False):
+ """Combines metrics across all classes by averaging over the class values.
+ If 'ignore_empty_classes' is True, then it only sums over classes with at least one gt or predicted detection.
+ """
+ res = {}
+ for field in self.integer_fields:
+ if ignore_empty_classes:
+ res[field] = self._combine_sum({k: v for k, v in all_res.items()
+ if v['IDTP'] + v['IDFN'] + v['IDFP'] > 0 + np.finfo('float').eps},
+ field)
+ else:
+ res[field] = self._combine_sum({k: v for k, v in all_res.items()}, field)
+ for field in self.float_fields:
+ if ignore_empty_classes:
+ res[field] = np.mean([v[field] for v in all_res.values()
+ if v['IDTP'] + v['IDFN'] + v['IDFP'] > 0 + np.finfo('float').eps], axis=0)
+ else:
+ res[field] = np.mean([v[field] for v in all_res.values()], axis=0)
+ return res
+
+ def combine_classes_det_averaged(self, all_res):
+ """Combines metrics across all classes by averaging over the detection values"""
+ res = {}
+ for field in self.integer_fields:
+ res[field] = self._combine_sum(all_res, field)
+ res = self._compute_final_fields(res)
+ return res
+
+ def combine_sequences(self, all_res):
+ """Combines metrics across all sequences"""
+ res = {}
+ for field in self.integer_fields:
+ res[field] = self._combine_sum(all_res, field)
+ res = self._compute_final_fields(res)
+ return res
+
+ @staticmethod
+ def _compute_final_fields(res):
+ """Calculate sub-metric ('field') values which only depend on other sub-metric values.
+ This function is used both for both per-sequence calculation, and in combining values across sequences.
+ """
+ res['IDR'] = res['IDTP'] / np.maximum(1.0, res['IDTP'] + res['IDFN'])
+ res['IDP'] = res['IDTP'] / np.maximum(1.0, res['IDTP'] + res['IDFP'])
+ res['IDF1'] = res['IDTP'] / np.maximum(1.0, res['IDTP'] + 0.5 * res['IDFP'] + 0.5 * res['IDFN'])
+ return res
diff --git a/val_utils/trackeval/metrics/ideucl.py b/val_utils/trackeval/metrics/ideucl.py
new file mode 100644
index 0000000000000000000000000000000000000000..db9b57b61f603f0266c92c5c0cf6f6e2e160938a
--- /dev/null
+++ b/val_utils/trackeval/metrics/ideucl.py
@@ -0,0 +1,135 @@
+import numpy as np
+from scipy.optimize import linear_sum_assignment
+from ._base_metric import _BaseMetric
+from .. import _timing
+from collections import defaultdict
+from .. import utils
+
+
+class IDEucl(_BaseMetric):
+ """Class which implements the ID metrics"""
+
+ @staticmethod
+ def get_default_config():
+ """Default class config values"""
+ default_config = {
+ 'THRESHOLD': 0.4, # Similarity score threshold required for a IDTP match. 0.4 for IDEucl.
+ 'PRINT_CONFIG': True, # Whether to print the config information on init. Default: False.
+ }
+ return default_config
+
+ def __init__(self, config=None):
+ super().__init__()
+ self.fields = ['IDEucl']
+ self.float_fields = self.fields
+ self.summary_fields = self.fields
+
+ # Configuration options:
+ self.config = utils.init_config(config, self.get_default_config(), self.get_name())
+ self.threshold = float(self.config['THRESHOLD'])
+
+
+ @_timing.time
+ def eval_sequence(self, data):
+ """Calculates IDEucl metrics for all frames"""
+ # Initialise results
+ res = {'IDEucl' : 0}
+
+ # Return result quickly if tracker or gt sequence is empty
+ if data['num_tracker_dets'] == 0 or data['num_gt_dets'] == 0.:
+ return res
+
+ data['centroid'] = []
+ for t, gt_det in enumerate(data['gt_dets']):
+ # import pdb;pdb.set_trace()
+ data['centroid'].append(self._compute_centroid(gt_det))
+
+ oid_hid_cent = defaultdict(list)
+ oid_cent = defaultdict(list)
+ for t, (gt_ids_t, tracker_ids_t) in enumerate(zip(data['gt_ids'], data['tracker_ids'])):
+ matches_mask = np.greater_equal(data['similarity_scores'][t], self.threshold)
+
+ # I hope the orders of ids and boxes are maintained in `data`
+ for ind, gid in enumerate(gt_ids_t):
+ oid_cent[gid].append(data['centroid'][t][ind])
+
+ match_idx_gt, match_idx_tracker = np.nonzero(matches_mask)
+ for m_gid, m_tid in zip(match_idx_gt, match_idx_tracker):
+ oid_hid_cent[gt_ids_t[m_gid], tracker_ids_t[m_tid]].append(data['centroid'][t][m_gid])
+
+ oid_hid_dist = {k : np.sum(np.linalg.norm(np.diff(np.array(v), axis=0), axis=1)) for k, v in oid_hid_cent.items()}
+ oid_dist = {int(k) : np.sum(np.linalg.norm(np.diff(np.array(v), axis=0), axis=1)) for k, v in oid_cent.items()}
+
+ unique_oid = np.unique([i[0] for i in oid_hid_dist.keys()]).tolist()
+ unique_hid = np.unique([i[1] for i in oid_hid_dist.keys()]).tolist()
+ o_len = len(unique_oid)
+ h_len = len(unique_hid)
+ dist_matrix = np.zeros((o_len, h_len))
+ for ((oid, hid), dist) in oid_hid_dist.items():
+ oid_ind = unique_oid.index(oid)
+ hid_ind = unique_hid.index(hid)
+ dist_matrix[oid_ind, hid_ind] = dist
+
+ # opt_hyp_dist contains GT ID : max dist covered by track
+ opt_hyp_dist = dict.fromkeys(oid_dist.keys(), 0.)
+ cost_matrix = np.max(dist_matrix) - dist_matrix
+ rows, cols = linear_sum_assignment(cost_matrix)
+ for (row, col) in zip(rows, cols):
+ value = dist_matrix[row, col]
+ opt_hyp_dist[int(unique_oid[row])] = value
+
+ assert len(opt_hyp_dist.keys()) == len(oid_dist.keys())
+ hyp_length = np.sum(list(opt_hyp_dist.values()))
+ gt_length = np.sum(list(oid_dist.values()))
+ id_eucl =np.mean([np.divide(a, b, out=np.zeros_like(a), where=b!=0) for a, b in zip(opt_hyp_dist.values(), oid_dist.values())])
+ res['IDEucl'] = np.divide(hyp_length, gt_length, out=np.zeros_like(hyp_length), where=gt_length!=0)
+ return res
+
+ def combine_classes_class_averaged(self, all_res, ignore_empty_classes=False):
+ """Combines metrics across all classes by averaging over the class values.
+ If 'ignore_empty_classes' is True, then it only sums over classes with at least one gt or predicted detection.
+ """
+ res = {}
+
+ for field in self.float_fields:
+ if ignore_empty_classes:
+ res[field] = np.mean([v[field] for v in all_res.values()
+ if v['IDEucl'] > 0 + np.finfo('float').eps], axis=0)
+ else:
+ res[field] = np.mean([v[field] for v in all_res.values()], axis=0)
+ return res
+
+ def combine_classes_det_averaged(self, all_res):
+ """Combines metrics across all classes by averaging over the detection values"""
+ res = {}
+ for field in self.float_fields:
+ res[field] = self._combine_sum(all_res, field)
+ res = self._compute_final_fields(res, len(all_res))
+ return res
+
+ def combine_sequences(self, all_res):
+ """Combines metrics across all sequences"""
+ res = {}
+ for field in self.float_fields:
+ res[field] = self._combine_sum(all_res, field)
+ res = self._compute_final_fields(res, len(all_res))
+ return res
+
+
+ @staticmethod
+ def _compute_centroid(box):
+ box = np.array(box)
+ if len(box.shape) == 1:
+ centroid = (box[0:2] + box[2:4])/2
+ else:
+ centroid = (box[:, 0:2] + box[:, 2:4])/2
+ return np.flip(centroid, axis=1)
+
+
+ @staticmethod
+ def _compute_final_fields(res, res_len):
+ """
+ Exists only to match signature with the original Identiy class.
+
+ """
+ return {k:v/res_len for k,v in res.items()}
diff --git a/val_utils/trackeval/metrics/j_and_f.py b/val_utils/trackeval/metrics/j_and_f.py
new file mode 100644
index 0000000000000000000000000000000000000000..1b18f046ba9aae48cc321d20a4831815c94c5933
--- /dev/null
+++ b/val_utils/trackeval/metrics/j_and_f.py
@@ -0,0 +1,310 @@
+
+import numpy as np
+import math
+from scipy.optimize import linear_sum_assignment
+from ..utils import TrackEvalException
+from ._base_metric import _BaseMetric
+from .. import _timing
+
+
+class JAndF(_BaseMetric):
+ """Class which implements the J&F metrics"""
+ def __init__(self, config=None):
+ super().__init__()
+ self.integer_fields = ['num_gt_tracks']
+ self.float_fields = ['J-Mean', 'J-Recall', 'J-Decay', 'F-Mean', 'F-Recall', 'F-Decay', 'J&F']
+ self.fields = self.float_fields + self.integer_fields
+ self.summary_fields = self.float_fields
+ self.optim_type = 'J' # possible values J, J&F
+
+ @_timing.time
+ def eval_sequence(self, data):
+ """Returns J&F metrics for one sequence"""
+
+ # Only loaded when run to reduce minimum requirements
+ from pycocotools import mask as mask_utils
+
+ num_timesteps = data['num_timesteps']
+ num_tracker_ids = data['num_tracker_ids']
+ num_gt_ids = data['num_gt_ids']
+ gt_dets = data['gt_dets']
+ tracker_dets = data['tracker_dets']
+ gt_ids = data['gt_ids']
+ tracker_ids = data['tracker_ids']
+
+ # get shape of frames
+ frame_shape = None
+ if num_gt_ids > 0:
+ for t in range(num_timesteps):
+ if len(gt_ids[t]) > 0:
+ frame_shape = gt_dets[t][0]['size']
+ break
+ elif num_tracker_ids > 0:
+ for t in range(num_timesteps):
+ if len(tracker_ids[t]) > 0:
+ frame_shape = tracker_dets[t][0]['size']
+ break
+
+ if frame_shape:
+ # append all zero masks for timesteps in which tracks do not have a detection
+ zero_padding = np.zeros((frame_shape), order= 'F').astype(np.uint8)
+ padding_mask = mask_utils.encode(zero_padding)
+ for t in range(num_timesteps):
+ gt_id_det_mapping = {gt_ids[t][i]: gt_dets[t][i] for i in range(len(gt_ids[t]))}
+ gt_dets[t] = [gt_id_det_mapping[index] if index in gt_ids[t] else padding_mask for index
+ in range(num_gt_ids)]
+ tracker_id_det_mapping = {tracker_ids[t][i]: tracker_dets[t][i] for i in range(len(tracker_ids[t]))}
+ tracker_dets[t] = [tracker_id_det_mapping[index] if index in tracker_ids[t] else padding_mask for index
+ in range(num_tracker_ids)]
+ # also perform zero padding if number of tracker IDs < number of ground truth IDs
+ if num_tracker_ids < num_gt_ids:
+ diff = num_gt_ids - num_tracker_ids
+ for t in range(num_timesteps):
+ tracker_dets[t] = tracker_dets[t] + [padding_mask for _ in range(diff)]
+ num_tracker_ids += diff
+
+ j = self._compute_j(gt_dets, tracker_dets, num_gt_ids, num_tracker_ids, num_timesteps)
+
+ # boundary threshold for F computation
+ bound_th = 0.008
+
+ # perform matching
+ if self.optim_type == 'J&F':
+ f = np.zeros_like(j)
+ for k in range(num_tracker_ids):
+ for i in range(num_gt_ids):
+ f[k, i, :] = self._compute_f(gt_dets, tracker_dets, k, i, bound_th)
+ optim_metrics = (np.mean(j, axis=2) + np.mean(f, axis=2)) / 2
+ row_ind, col_ind = linear_sum_assignment(- optim_metrics)
+ j_m = j[row_ind, col_ind, :]
+ f_m = f[row_ind, col_ind, :]
+ elif self.optim_type == 'J':
+ optim_metrics = np.mean(j, axis=2)
+ row_ind, col_ind = linear_sum_assignment(- optim_metrics)
+ j_m = j[row_ind, col_ind, :]
+ f_m = np.zeros_like(j_m)
+ for i, (tr_ind, gt_ind) in enumerate(zip(row_ind, col_ind)):
+ f_m[i] = self._compute_f(gt_dets, tracker_dets, tr_ind, gt_ind, bound_th)
+ else:
+ raise TrackEvalException('Unsupported optimization type %s for J&F metric.' % self.optim_type)
+
+ # append zeros for false negatives
+ if j_m.shape[0] < data['num_gt_ids']:
+ diff = data['num_gt_ids'] - j_m.shape[0]
+ j_m = np.concatenate((j_m, np.zeros((diff, j_m.shape[1]))), axis=0)
+ f_m = np.concatenate((f_m, np.zeros((diff, f_m.shape[1]))), axis=0)
+
+ # compute the metrics for each ground truth track
+ res = {
+ 'J-Mean': [np.nanmean(j_m[i, :]) for i in range(j_m.shape[0])],
+ 'J-Recall': [np.nanmean(j_m[i, :] > 0.5 + np.finfo('float').eps) for i in range(j_m.shape[0])],
+ 'F-Mean': [np.nanmean(f_m[i, :]) for i in range(f_m.shape[0])],
+ 'F-Recall': [np.nanmean(f_m[i, :] > 0.5 + np.finfo('float').eps) for i in range(f_m.shape[0])],
+ 'J-Decay': [],
+ 'F-Decay': []
+ }
+ n_bins = 4
+ ids = np.round(np.linspace(1, data['num_timesteps'], n_bins + 1) + 1e-10) - 1
+ ids = ids.astype(np.uint8)
+
+ for k in range(j_m.shape[0]):
+ d_bins_j = [j_m[k][ids[i]:ids[i + 1] + 1] for i in range(0, n_bins)]
+ res['J-Decay'].append(np.nanmean(d_bins_j[0]) - np.nanmean(d_bins_j[3]))
+ for k in range(f_m.shape[0]):
+ d_bins_f = [f_m[k][ids[i]:ids[i + 1] + 1] for i in range(0, n_bins)]
+ res['F-Decay'].append(np.nanmean(d_bins_f[0]) - np.nanmean(d_bins_f[3]))
+
+ # count number of tracks for weighting of the result
+ res['num_gt_tracks'] = len(res['J-Mean'])
+ for field in ['J-Mean', 'J-Recall', 'J-Decay', 'F-Mean', 'F-Recall', 'F-Decay']:
+ res[field] = np.mean(res[field])
+ res['J&F'] = (res['J-Mean'] + res['F-Mean']) / 2
+ return res
+
+ def combine_sequences(self, all_res):
+ """Combines metrics across all sequences"""
+ res = {'num_gt_tracks': self._combine_sum(all_res, 'num_gt_tracks')}
+ for field in self.summary_fields:
+ res[field] = self._combine_weighted_av(all_res, field, res, weight_field='num_gt_tracks')
+ return res
+
+ def combine_classes_class_averaged(self, all_res, ignore_empty_classes=False):
+ """Combines metrics across all classes by averaging over the class values
+ 'ignore empty classes' is not yet implemented here.
+ """
+ res = {'num_gt_tracks': self._combine_sum(all_res, 'num_gt_tracks')}
+ for field in self.float_fields:
+ res[field] = np.mean([v[field] for v in all_res.values()])
+ return res
+
+ def combine_classes_det_averaged(self, all_res):
+ """Combines metrics across all classes by averaging over the detection values"""
+ res = {'num_gt_tracks': self._combine_sum(all_res, 'num_gt_tracks')}
+ for field in self.float_fields:
+ res[field] = np.mean([v[field] for v in all_res.values()])
+ return res
+
+ @staticmethod
+ def _seg2bmap(seg, width=None, height=None):
+ """
+ From a segmentation, compute a binary boundary map with 1 pixel wide
+ boundaries. The boundary pixels are offset by 1/2 pixel towards the
+ origin from the actual segment boundary.
+ Arguments:
+ seg : Segments labeled from 1..k.
+ width : Width of desired bmap <= seg.shape[1]
+ height : Height of desired bmap <= seg.shape[0]
+ Returns:
+ bmap (ndarray): Binary boundary map.
+ David Martin
+ January 2003
+ """
+
+ seg = seg.astype(np.bool)
+ seg[seg > 0] = 1
+
+ assert np.atleast_3d(seg).shape[2] == 1
+
+ width = seg.shape[1] if width is None else width
+ height = seg.shape[0] if height is None else height
+
+ h, w = seg.shape[:2]
+
+ ar1 = float(width) / float(height)
+ ar2 = float(w) / float(h)
+
+ assert not (
+ width > w | height > h | abs(ar1 - ar2) > 0.01
+ ), "Can" "t convert %dx%d seg to %dx%d bmap." % (w, h, width, height)
+
+ e = np.zeros_like(seg)
+ s = np.zeros_like(seg)
+ se = np.zeros_like(seg)
+
+ e[:, :-1] = seg[:, 1:]
+ s[:-1, :] = seg[1:, :]
+ se[:-1, :-1] = seg[1:, 1:]
+
+ b = seg ^ e | seg ^ s | seg ^ se
+ b[-1, :] = seg[-1, :] ^ e[-1, :]
+ b[:, -1] = seg[:, -1] ^ s[:, -1]
+ b[-1, -1] = 0
+
+ if w == width and h == height:
+ bmap = b
+ else:
+ bmap = np.zeros((height, width))
+ for x in range(w):
+ for y in range(h):
+ if b[y, x]:
+ j = 1 + math.floor((y - 1) + height / h)
+ i = 1 + math.floor((x - 1) + width / h)
+ bmap[j, i] = 1
+
+ return bmap
+
+ @staticmethod
+ def _compute_f(gt_data, tracker_data, tracker_data_id, gt_id, bound_th):
+ """
+ Perform F computation for a given gt and a given tracker ID. Adapted from
+ https://github.com/davisvideochallenge/davis2017-evaluation
+ :param gt_data: the encoded gt masks
+ :param tracker_data: the encoded tracker masks
+ :param tracker_data_id: the tracker ID
+ :param gt_id: the ground truth ID
+ :param bound_th: boundary threshold parameter
+ :return: the F value for the given tracker and gt ID
+ """
+
+ # Only loaded when run to reduce minimum requirements
+ from pycocotools import mask as mask_utils
+ from skimage.morphology import disk
+ import cv2
+
+ f = np.zeros(len(gt_data))
+
+ for t, (gt_masks, tracker_masks) in enumerate(zip(gt_data, tracker_data)):
+ curr_tracker_mask = mask_utils.decode(tracker_masks[tracker_data_id])
+ curr_gt_mask = mask_utils.decode(gt_masks[gt_id])
+
+ bound_pix = bound_th if bound_th >= 1 - np.finfo('float').eps else \
+ np.ceil(bound_th * np.linalg.norm(curr_tracker_mask.shape))
+
+ # Get the pixel boundaries of both masks
+ fg_boundary = JAndF._seg2bmap(curr_tracker_mask)
+ gt_boundary = JAndF._seg2bmap(curr_gt_mask)
+
+ # fg_dil = binary_dilation(fg_boundary, disk(bound_pix))
+ fg_dil = cv2.dilate(fg_boundary.astype(np.uint8), disk(bound_pix).astype(np.uint8))
+ # gt_dil = binary_dilation(gt_boundary, disk(bound_pix))
+ gt_dil = cv2.dilate(gt_boundary.astype(np.uint8), disk(bound_pix).astype(np.uint8))
+
+ # Get the intersection
+ gt_match = gt_boundary * fg_dil
+ fg_match = fg_boundary * gt_dil
+
+ # Area of the intersection
+ n_fg = np.sum(fg_boundary)
+ n_gt = np.sum(gt_boundary)
+
+ # % Compute precision and recall
+ if n_fg == 0 and n_gt > 0:
+ precision = 1
+ recall = 0
+ elif n_fg > 0 and n_gt == 0:
+ precision = 0
+ recall = 1
+ elif n_fg == 0 and n_gt == 0:
+ precision = 1
+ recall = 1
+ else:
+ precision = np.sum(fg_match) / float(n_fg)
+ recall = np.sum(gt_match) / float(n_gt)
+
+ # Compute F measure
+ if precision + recall == 0:
+ f_val = 0
+ else:
+ f_val = 2 * precision * recall / (precision + recall)
+
+ f[t] = f_val
+
+ return f
+
+ @staticmethod
+ def _compute_j(gt_data, tracker_data, num_gt_ids, num_tracker_ids, num_timesteps):
+ """
+ Computation of J value for all ground truth IDs and all tracker IDs in the given sequence. Adapted from
+ https://github.com/davisvideochallenge/davis2017-evaluation
+ :param gt_data: the ground truth masks
+ :param tracker_data: the tracker masks
+ :param num_gt_ids: the number of ground truth IDs
+ :param num_tracker_ids: the number of tracker IDs
+ :param num_timesteps: the number of timesteps
+ :return: the J values
+ """
+
+ # Only loaded when run to reduce minimum requirements
+ from pycocotools import mask as mask_utils
+
+ j = np.zeros((num_tracker_ids, num_gt_ids, num_timesteps))
+
+ for t, (time_gt, time_data) in enumerate(zip(gt_data, tracker_data)):
+ # run length encoded masks with pycocotools
+ area_gt = mask_utils.area(time_gt)
+ time_data = list(time_data)
+ area_tr = mask_utils.area(time_data)
+
+ area_tr = np.repeat(area_tr[:, np.newaxis], len(area_gt), axis=1)
+ area_gt = np.repeat(area_gt[np.newaxis, :], len(area_tr), axis=0)
+
+ # mask iou computation with pycocotools
+ ious = np.atleast_2d(mask_utils.iou(time_data, time_gt, [0]*len(time_gt)))
+ # set iou to 1 if both masks are close to 0 (no ground truth and no predicted mask in timestep)
+ ious[np.isclose(area_tr, 0) & np.isclose(area_gt, 0)] = 1
+ assert (ious >= 0 - np.finfo('float').eps).all()
+ assert (ious <= 1 + np.finfo('float').eps).all()
+
+ j[..., t] = ious
+
+ return j
diff --git a/val_utils/trackeval/metrics/track_map.py b/val_utils/trackeval/metrics/track_map.py
new file mode 100644
index 0000000000000000000000000000000000000000..039f89084c8defd683c7f7d26cdd77834ecc2f23
--- /dev/null
+++ b/val_utils/trackeval/metrics/track_map.py
@@ -0,0 +1,462 @@
+import numpy as np
+from ._base_metric import _BaseMetric
+from .. import _timing
+from functools import partial
+from .. import utils
+from ..utils import TrackEvalException
+
+
+class TrackMAP(_BaseMetric):
+ """Class which implements the TrackMAP metrics"""
+
+ @staticmethod
+ def get_default_metric_config():
+ """Default class config values"""
+ default_config = {
+ 'USE_AREA_RANGES': True, # whether to evaluate for certain area ranges
+ 'AREA_RANGES': [[0 ** 2, 32 ** 2], # additional area range sets for which TrackMAP is evaluated
+ [32 ** 2, 96 ** 2], # (all area range always included), default values for TAO
+ [96 ** 2, 1e5 ** 2]], # evaluation
+ 'AREA_RANGE_LABELS': ["area_s", "area_m", "area_l"], # the labels for the area ranges
+ 'USE_TIME_RANGES': True, # whether to evaluate for certain time ranges (length of tracks)
+ 'TIME_RANGES': [[0, 3], [3, 10], [10, 1e5]], # additional time range sets for which TrackMAP is evaluated
+ # (all time range always included) , default values for TAO evaluation
+ 'TIME_RANGE_LABELS': ["time_s", "time_m", "time_l"], # the labels for the time ranges
+ 'IOU_THRESHOLDS': np.arange(0.5, 0.96, 0.05), # the IoU thresholds
+ 'RECALL_THRESHOLDS': np.linspace(0.0, 1.00, int(np.round((1.00 - 0.0) / 0.01) + 1), endpoint=True),
+ # recall thresholds at which precision is evaluated
+ 'MAX_DETECTIONS': 0, # limit the maximum number of considered tracks per sequence (0 for unlimited)
+ 'PRINT_CONFIG': True
+ }
+ return default_config
+
+ def __init__(self, config=None):
+ super().__init__()
+ self.config = utils.init_config(config, self.get_default_metric_config(), self.get_name())
+
+ self.num_ig_masks = 1
+ self.lbls = ['all']
+ self.use_area_rngs = self.config['USE_AREA_RANGES']
+ if self.use_area_rngs:
+ self.area_rngs = self.config['AREA_RANGES']
+ self.area_rng_lbls = self.config['AREA_RANGE_LABELS']
+ self.num_ig_masks += len(self.area_rng_lbls)
+ self.lbls += self.area_rng_lbls
+
+ self.use_time_rngs = self.config['USE_TIME_RANGES']
+ if self.use_time_rngs:
+ self.time_rngs = self.config['TIME_RANGES']
+ self.time_rng_lbls = self.config['TIME_RANGE_LABELS']
+ self.num_ig_masks += len(self.time_rng_lbls)
+ self.lbls += self.time_rng_lbls
+
+ self.array_labels = self.config['IOU_THRESHOLDS']
+ self.rec_thrs = self.config['RECALL_THRESHOLDS']
+
+ self.maxDet = self.config['MAX_DETECTIONS']
+ self.float_array_fields = ['AP_' + lbl for lbl in self.lbls] + ['AR_' + lbl for lbl in self.lbls]
+ self.fields = self.float_array_fields
+ self.summary_fields = self.float_array_fields
+
+ @_timing.time
+ def eval_sequence(self, data):
+ """Calculates GT and Tracker matches for one sequence for TrackMAP metrics. Adapted from
+ https://github.com/TAO-Dataset/"""
+
+ # Initialise results to zero for each sequence as the fields are only defined over the set of all sequences
+ res = {}
+ for field in self.fields:
+ res[field] = [0 for _ in self.array_labels]
+
+ gt_ids, dt_ids = data['gt_track_ids'], data['dt_track_ids']
+
+ if len(gt_ids) == 0 and len(dt_ids) == 0:
+ for idx in range(self.num_ig_masks):
+ res[idx] = None
+ return res
+
+ # get track data
+ gt_tr_areas = data.get('gt_track_areas', None) if self.use_area_rngs else None
+ gt_tr_lengths = data.get('gt_track_lengths', None) if self.use_time_rngs else None
+ gt_tr_iscrowd = data.get('gt_track_iscrowd', None)
+ dt_tr_areas = data.get('dt_track_areas', None) if self.use_area_rngs else None
+ dt_tr_lengths = data.get('dt_track_lengths', None) if self.use_time_rngs else None
+ is_nel = data.get('not_exhaustively_labeled', False)
+
+ # compute ignore masks for different track sets to eval
+ gt_ig_masks = self._compute_track_ig_masks(len(gt_ids), track_lengths=gt_tr_lengths, track_areas=gt_tr_areas,
+ iscrowd=gt_tr_iscrowd)
+ dt_ig_masks = self._compute_track_ig_masks(len(dt_ids), track_lengths=dt_tr_lengths, track_areas=dt_tr_areas,
+ is_not_exhaustively_labeled=is_nel, is_gt=False)
+
+ boxformat = data.get('boxformat', 'xywh')
+ ious = self._compute_track_ious(data['dt_tracks'], data['gt_tracks'], iou_function=data['iou_type'],
+ boxformat=boxformat)
+
+ for mask_idx in range(self.num_ig_masks):
+ gt_ig_mask = gt_ig_masks[mask_idx]
+
+ # Sort gt ignore last
+ gt_idx = np.argsort([g for g in gt_ig_mask], kind="mergesort")
+ gt_ids = [gt_ids[i] for i in gt_idx]
+
+ ious_sorted = ious[:, gt_idx] if len(ious) > 0 else ious
+
+ num_thrs = len(self.array_labels)
+ num_gt = len(gt_ids)
+ num_dt = len(dt_ids)
+
+ # Array to store the "id" of the matched dt/gt
+ gt_m = np.zeros((num_thrs, num_gt)) - 1
+ dt_m = np.zeros((num_thrs, num_dt)) - 1
+
+ gt_ig = np.array([gt_ig_mask[idx] for idx in gt_idx])
+ dt_ig = np.zeros((num_thrs, num_dt))
+
+ for iou_thr_idx, iou_thr in enumerate(self.array_labels):
+ if len(ious_sorted) == 0:
+ break
+
+ for dt_idx, _dt in enumerate(dt_ids):
+ iou = min([iou_thr, 1 - 1e-10])
+ # information about best match so far (m=-1 -> unmatched)
+ # store the gt_idx which matched for _dt
+ m = -1
+ for gt_idx, _ in enumerate(gt_ids):
+ # if this gt already matched continue
+ if gt_m[iou_thr_idx, gt_idx] > 0:
+ continue
+ # if _dt matched to reg gt, and on ignore gt, stop
+ if m > -1 and gt_ig[m] == 0 and gt_ig[gt_idx] == 1:
+ break
+ # continue to next gt unless better match made
+ if ious_sorted[dt_idx, gt_idx] < iou - np.finfo('float').eps:
+ continue
+ # if match successful and best so far, store appropriately
+ iou = ious_sorted[dt_idx, gt_idx]
+ m = gt_idx
+
+ # No match found for _dt, go to next _dt
+ if m == -1:
+ continue
+
+ # if gt to ignore for some reason update dt_ig.
+ # Should not be used in evaluation.
+ dt_ig[iou_thr_idx, dt_idx] = gt_ig[m]
+ # _dt match found, update gt_m, and dt_m with "id"
+ dt_m[iou_thr_idx, dt_idx] = gt_ids[m]
+ gt_m[iou_thr_idx, m] = _dt
+
+ dt_ig_mask = dt_ig_masks[mask_idx]
+
+ dt_ig_mask = np.array(dt_ig_mask).reshape((1, num_dt)) # 1 X num_dt
+ dt_ig_mask = np.repeat(dt_ig_mask, num_thrs, 0) # num_thrs X num_dt
+
+ # Based on dt_ig_mask ignore any unmatched detection by updating dt_ig
+ dt_ig = np.logical_or(dt_ig, np.logical_and(dt_m == -1, dt_ig_mask))
+ # store results for given video and category
+ res[mask_idx] = {
+ "dt_ids": dt_ids,
+ "gt_ids": gt_ids,
+ "dt_matches": dt_m,
+ "gt_matches": gt_m,
+ "dt_scores": data['dt_track_scores'],
+ "gt_ignore": gt_ig,
+ "dt_ignore": dt_ig,
+ }
+
+ return res
+
+ def combine_sequences(self, all_res):
+ """Combines metrics across all sequences. Computes precision and recall values based on track matches.
+ Adapted from https://github.com/TAO-Dataset/
+ """
+ num_thrs = len(self.array_labels)
+ num_recalls = len(self.rec_thrs)
+
+ # -1 for absent categories
+ precision = -np.ones(
+ (num_thrs, num_recalls, self.num_ig_masks)
+ )
+ recall = -np.ones((num_thrs, self.num_ig_masks))
+
+ for ig_idx in range(self.num_ig_masks):
+ ig_idx_results = [res[ig_idx] for res in all_res.values() if res[ig_idx] is not None]
+
+ # Remove elements which are None
+ if len(ig_idx_results) == 0:
+ continue
+
+ # Append all scores: shape (N,)
+ # limit considered tracks for each sequence if maxDet > 0
+ if self.maxDet == 0:
+ dt_scores = np.concatenate([res["dt_scores"] for res in ig_idx_results], axis=0)
+
+ dt_idx = np.argsort(-dt_scores, kind="mergesort")
+
+ dt_m = np.concatenate([e["dt_matches"] for e in ig_idx_results],
+ axis=1)[:, dt_idx]
+ dt_ig = np.concatenate([e["dt_ignore"] for e in ig_idx_results],
+ axis=1)[:, dt_idx]
+ elif self.maxDet > 0:
+ dt_scores = np.concatenate([res["dt_scores"][0:self.maxDet] for res in ig_idx_results], axis=0)
+
+ dt_idx = np.argsort(-dt_scores, kind="mergesort")
+
+ dt_m = np.concatenate([e["dt_matches"][:, 0:self.maxDet] for e in ig_idx_results],
+ axis=1)[:, dt_idx]
+ dt_ig = np.concatenate([e["dt_ignore"][:, 0:self.maxDet] for e in ig_idx_results],
+ axis=1)[:, dt_idx]
+ else:
+ raise Exception("Number of maximum detections must be >= 0, but is set to %i" % self.maxDet)
+
+ gt_ig = np.concatenate([res["gt_ignore"] for res in ig_idx_results])
+ # num gt anns to consider
+ num_gt = np.count_nonzero(gt_ig == 0)
+
+ if num_gt == 0:
+ continue
+
+ tps = np.logical_and(dt_m != -1, np.logical_not(dt_ig))
+ fps = np.logical_and(dt_m == -1, np.logical_not(dt_ig))
+
+ tp_sum = np.cumsum(tps, axis=1).astype(dtype=np.float)
+ fp_sum = np.cumsum(fps, axis=1).astype(dtype=np.float)
+
+ for iou_thr_idx, (tp, fp) in enumerate(zip(tp_sum, fp_sum)):
+ tp = np.array(tp)
+ fp = np.array(fp)
+ num_tp = len(tp)
+ rc = tp / num_gt
+ if num_tp:
+ recall[iou_thr_idx, ig_idx] = rc[-1]
+ else:
+ recall[iou_thr_idx, ig_idx] = 0
+
+ # np.spacing(1) ~= eps
+ pr = tp / (fp + tp + np.spacing(1))
+ pr = pr.tolist()
+
+ # Ensure precision values are monotonically decreasing
+ for i in range(num_tp - 1, 0, -1):
+ if pr[i] > pr[i - 1]:
+ pr[i - 1] = pr[i]
+
+ # find indices at the predefined recall values
+ rec_thrs_insert_idx = np.searchsorted(rc, self.rec_thrs, side="left")
+
+ pr_at_recall = [0.0] * num_recalls
+
+ try:
+ for _idx, pr_idx in enumerate(rec_thrs_insert_idx):
+ pr_at_recall[_idx] = pr[pr_idx]
+ except IndexError:
+ pass
+
+ precision[iou_thr_idx, :, ig_idx] = (np.array(pr_at_recall))
+
+ res = {'precision': precision, 'recall': recall}
+
+ # compute the precision and recall averages for the respective alpha thresholds and ignore masks
+ for lbl in self.lbls:
+ res['AP_' + lbl] = np.zeros((len(self.array_labels)), dtype=np.float)
+ res['AR_' + lbl] = np.zeros((len(self.array_labels)), dtype=np.float)
+
+ for a_id, alpha in enumerate(self.array_labels):
+ for lbl_idx, lbl in enumerate(self.lbls):
+ p = precision[a_id, :, lbl_idx]
+ if len(p[p > -1]) == 0:
+ mean_p = -1
+ else:
+ mean_p = np.mean(p[p > -1])
+ res['AP_' + lbl][a_id] = mean_p
+ res['AR_' + lbl][a_id] = recall[a_id, lbl_idx]
+
+ return res
+
+ def combine_classes_class_averaged(self, all_res, ignore_empty_classes=True):
+ """Combines metrics across all classes by averaging over the class values
+ Note mAP is not well defined for 'empty classes' so 'ignore empty classes' is always true here.
+ """
+ res = {}
+ for field in self.fields:
+ res[field] = np.zeros((len(self.array_labels)), dtype=np.float)
+ field_stacked = np.array([res[field] for res in all_res.values()])
+
+ for a_id, alpha in enumerate(self.array_labels):
+ values = field_stacked[:, a_id]
+ if len(values[values > -1]) == 0:
+ mean = -1
+ else:
+ mean = np.mean(values[values > -1])
+ res[field][a_id] = mean
+ return res
+
+ def combine_classes_det_averaged(self, all_res):
+ """Combines metrics across all classes by averaging over the detection values"""
+
+ res = {}
+ for field in self.fields:
+ res[field] = np.zeros((len(self.array_labels)), dtype=np.float)
+ field_stacked = np.array([res[field] for res in all_res.values()])
+
+ for a_id, alpha in enumerate(self.array_labels):
+ values = field_stacked[:, a_id]
+ if len(values[values > -1]) == 0:
+ mean = -1
+ else:
+ mean = np.mean(values[values > -1])
+ res[field][a_id] = mean
+ return res
+
+ def _compute_track_ig_masks(self, num_ids, track_lengths=None, track_areas=None, iscrowd=None,
+ is_not_exhaustively_labeled=False, is_gt=True):
+ """
+ Computes ignore masks for different track sets to evaluate
+ :param num_ids: the number of track IDs
+ :param track_lengths: the lengths of the tracks (number of timesteps)
+ :param track_areas: the average area of a track
+ :param iscrowd: whether a track is marked as crowd
+ :param is_not_exhaustively_labeled: whether the track category is not exhaustively labeled
+ :param is_gt: whether it is gt
+ :return: the track ignore masks
+ """
+ # for TAO tracks for classes which are not exhaustively labeled are not evaluated
+ if not is_gt and is_not_exhaustively_labeled:
+ track_ig_masks = [[1 for _ in range(num_ids)] for i in range(self.num_ig_masks)]
+ else:
+ # consider all tracks
+ track_ig_masks = [[0 for _ in range(num_ids)]]
+
+ # consider tracks with certain area
+ if self.use_area_rngs:
+ for rng in self.area_rngs:
+ track_ig_masks.append([0 if rng[0] - np.finfo('float').eps <= area <= rng[1] + np.finfo('float').eps
+ else 1 for area in track_areas])
+
+ # consider tracks with certain duration
+ if self.use_time_rngs:
+ for rng in self.time_rngs:
+ track_ig_masks.append([0 if rng[0] - np.finfo('float').eps <= length
+ <= rng[1] + np.finfo('float').eps else 1 for length in track_lengths])
+
+ # for YouTubeVIS evaluation tracks with crowd tag are not evaluated
+ if is_gt and iscrowd:
+ track_ig_masks = [np.logical_or(mask, iscrowd) for mask in track_ig_masks]
+
+ return track_ig_masks
+
+ @staticmethod
+ def _compute_bb_track_iou(dt_track, gt_track, boxformat='xywh'):
+ """
+ Calculates the track IoU for one detected track and one ground truth track for bounding boxes
+ :param dt_track: the detected track (format: dictionary with frame index as keys and
+ numpy arrays as values)
+ :param gt_track: the ground truth track (format: dictionary with frame index as keys and
+ numpy array as values)
+ :param boxformat: the format of the boxes
+ :return: the track IoU
+ """
+ intersect = 0
+ union = 0
+ image_ids = set(gt_track.keys()) | set(dt_track.keys())
+ for image in image_ids:
+ g = gt_track.get(image, None)
+ d = dt_track.get(image, None)
+ if boxformat == 'xywh':
+ if d is not None and g is not None:
+ dx, dy, dw, dh = d
+ gx, gy, gw, gh = g
+ w = max(min(dx + dw, gx + gw) - max(dx, gx), 0)
+ h = max(min(dy + dh, gy + gh) - max(dy, gy), 0)
+ i = w * h
+ u = dw * dh + gw * gh - i
+ intersect += i
+ union += u
+ elif d is None and g is not None:
+ union += g[2] * g[3]
+ elif d is not None and g is None:
+ union += d[2] * d[3]
+ elif boxformat == 'x0y0x1y1':
+ if d is not None and g is not None:
+ dx0, dy0, dx1, dy1 = d
+ gx0, gy0, gx1, gy1 = g
+ w = max(min(dx1, gx1) - max(dx0, gx0), 0)
+ h = max(min(dy1, gy1) - max(dy0, gy0), 0)
+ i = w * h
+ u = (dx1 - dx0) * (dy1 - dy0) + (gx1 - gx0) * (gy1 - gy0) - i
+ intersect += i
+ union += u
+ elif d is None and g is not None:
+ union += (g[2] - g[0]) * (g[3] - g[1])
+ elif d is not None and g is None:
+ union += (d[2] - d[0]) * (d[3] - d[1])
+ else:
+ raise TrackEvalException('BoxFormat not implemented')
+ if intersect > union:
+ raise TrackEvalException("Intersection value > union value. Are the box values corrupted?")
+ return intersect / union if union > 0 else 0
+
+ @staticmethod
+ def _compute_mask_track_iou(dt_track, gt_track):
+ """
+ Calculates the track IoU for one detected track and one ground truth track for segmentation masks
+ :param dt_track: the detected track (format: dictionary with frame index as keys and
+ pycocotools rle encoded masks as values)
+ :param gt_track: the ground truth track (format: dictionary with frame index as keys and
+ pycocotools rle encoded masks as values)
+ :return: the track IoU
+ """
+ # only loaded when needed to reduce minimum requirements
+ from pycocotools import mask as mask_utils
+
+ intersect = .0
+ union = .0
+ image_ids = set(gt_track.keys()) | set(dt_track.keys())
+ for image in image_ids:
+ g = gt_track.get(image, None)
+ d = dt_track.get(image, None)
+ if d and g:
+ intersect += mask_utils.area(mask_utils.merge([d, g], True))
+ union += mask_utils.area(mask_utils.merge([d, g], False))
+ elif not d and g:
+ union += mask_utils.area(g)
+ elif d and not g:
+ union += mask_utils.area(d)
+ if union < 0.0 - np.finfo('float').eps:
+ raise TrackEvalException("Union value < 0. Are the segmentaions corrupted?")
+ if intersect > union:
+ raise TrackEvalException("Intersection value > union value. Are the segmentations corrupted?")
+ iou = intersect / union if union > 0.0 + np.finfo('float').eps else 0.0
+ return iou
+
+ @staticmethod
+ def _compute_track_ious(dt, gt, iou_function='bbox', boxformat='xywh'):
+ """
+ Calculate track IoUs for a set of ground truth tracks and a set of detected tracks
+ """
+
+ if len(gt) == 0 and len(dt) == 0:
+ return []
+
+ if iou_function == 'bbox':
+ track_iou_function = partial(TrackMAP._compute_bb_track_iou, boxformat=boxformat)
+ elif iou_function == 'mask':
+ track_iou_function = partial(TrackMAP._compute_mask_track_iou)
+ else:
+ raise Exception('IoU function not implemented')
+
+ ious = np.zeros([len(dt), len(gt)])
+ for i, j in np.ndindex(ious.shape):
+ ious[i, j] = track_iou_function(dt[i], gt[j])
+ return ious
+
+ @staticmethod
+ def _row_print(*argv):
+ """Prints results in an evenly spaced rows, with more space in first row"""
+ if len(argv) == 1:
+ argv = argv[0]
+ to_print = '%-40s' % argv[0]
+ for v in argv[1:]:
+ to_print += '%-12s' % str(v)
+ print(to_print)
diff --git a/val_utils/trackeval/metrics/vace.py b/val_utils/trackeval/metrics/vace.py
new file mode 100644
index 0000000000000000000000000000000000000000..81858d429c6e4943ca8c168c6683517ec2907b98
--- /dev/null
+++ b/val_utils/trackeval/metrics/vace.py
@@ -0,0 +1,131 @@
+import numpy as np
+from scipy.optimize import linear_sum_assignment
+from ._base_metric import _BaseMetric
+from .. import _timing
+
+
+class VACE(_BaseMetric):
+ """Class which implements the VACE metrics.
+
+ The metrics are described in:
+ Manohar et al. (2006) "Performance Evaluation of Object Detection and Tracking in Video"
+ https://link.springer.com/chapter/10.1007/11612704_16
+
+ This implementation uses the "relaxed" variant of the metrics,
+ where an overlap threshold is applied in each frame.
+ """
+
+ def __init__(self, config=None):
+ super().__init__()
+ self.integer_fields = ['VACE_IDs', 'VACE_GT_IDs', 'num_non_empty_timesteps']
+ self.float_fields = ['STDA', 'ATA', 'FDA', 'SFDA']
+ self.fields = self.integer_fields + self.float_fields
+ self.summary_fields = ['SFDA', 'ATA']
+
+ # Fields that are accumulated over multiple videos.
+ self._additive_fields = self.integer_fields + ['STDA', 'FDA']
+
+ self.threshold = 0.5
+
+ @_timing.time
+ def eval_sequence(self, data):
+ """Calculates VACE metrics for one sequence.
+
+ Depends on the fields:
+ data['num_gt_ids']
+ data['num_tracker_ids']
+ data['gt_ids']
+ data['tracker_ids']
+ data['similarity_scores']
+ """
+ res = {}
+
+ # Obtain Average Tracking Accuracy (ATA) using track correspondence.
+ # Obtain counts necessary to compute temporal IOU.
+ # Assume that integer counts can be represented exactly as floats.
+ potential_matches_count = np.zeros((data['num_gt_ids'], data['num_tracker_ids']))
+ gt_id_count = np.zeros(data['num_gt_ids'])
+ tracker_id_count = np.zeros(data['num_tracker_ids'])
+ both_present_count = np.zeros((data['num_gt_ids'], data['num_tracker_ids']))
+ for t, (gt_ids_t, tracker_ids_t) in enumerate(zip(data['gt_ids'], data['tracker_ids'])):
+ # Count the number of frames in which two tracks satisfy the overlap criterion.
+ matches_mask = np.greater_equal(data['similarity_scores'][t], self.threshold)
+ match_idx_gt, match_idx_tracker = np.nonzero(matches_mask)
+ potential_matches_count[gt_ids_t[match_idx_gt], tracker_ids_t[match_idx_tracker]] += 1
+ # Count the number of frames in which the tracks are present.
+ gt_id_count[gt_ids_t] += 1
+ tracker_id_count[tracker_ids_t] += 1
+ both_present_count[gt_ids_t[:, np.newaxis], tracker_ids_t[np.newaxis, :]] += 1
+ # Number of frames in which either track is present (union of the two sets of frames).
+ union_count = (gt_id_count[:, np.newaxis]
+ + tracker_id_count[np.newaxis, :]
+ - both_present_count)
+ # The denominator should always be non-zero if all tracks are non-empty.
+ with np.errstate(divide='raise', invalid='raise'):
+ temporal_iou = potential_matches_count / union_count
+ # Find assignment that maximizes temporal IOU.
+ match_rows, match_cols = linear_sum_assignment(-temporal_iou)
+ res['STDA'] = temporal_iou[match_rows, match_cols].sum()
+ res['VACE_IDs'] = data['num_tracker_ids']
+ res['VACE_GT_IDs'] = data['num_gt_ids']
+
+ # Obtain Frame Detection Accuracy (FDA) using per-frame correspondence.
+ non_empty_count = 0
+ fda = 0
+ for t, (gt_ids_t, tracker_ids_t) in enumerate(zip(data['gt_ids'], data['tracker_ids'])):
+ n_g = len(gt_ids_t)
+ n_d = len(tracker_ids_t)
+ if not (n_g or n_d):
+ continue
+ # n_g > 0 or n_d > 0
+ non_empty_count += 1
+ if not (n_g and n_d):
+ continue
+ # n_g > 0 and n_d > 0
+ spatial_overlap = data['similarity_scores'][t]
+ match_rows, match_cols = linear_sum_assignment(-spatial_overlap)
+ overlap_ratio = spatial_overlap[match_rows, match_cols].sum()
+ fda += overlap_ratio / (0.5 * (n_g + n_d))
+ res['FDA'] = fda
+ res['num_non_empty_timesteps'] = non_empty_count
+
+ res.update(self._compute_final_fields(res))
+ return res
+
+ def combine_classes_class_averaged(self, all_res, ignore_empty_classes=True):
+ """Combines metrics across all classes by averaging over the class values.
+ If 'ignore_empty_classes' is True, then it only sums over classes with at least one gt or predicted detection.
+ """
+ res = {}
+ for field in self.fields:
+ if ignore_empty_classes:
+ res[field] = np.mean([v[field] for v in all_res.values()
+ if v['VACE_GT_IDs'] > 0 or v['VACE_IDs'] > 0], axis=0)
+ else:
+ res[field] = np.mean([v[field] for v in all_res.values()], axis=0)
+ return res
+
+ def combine_classes_det_averaged(self, all_res):
+ """Combines metrics across all classes by averaging over the detection values"""
+ res = {}
+ for field in self._additive_fields:
+ res[field] = _BaseMetric._combine_sum(all_res, field)
+ res = self._compute_final_fields(res)
+ return res
+
+ def combine_sequences(self, all_res):
+ """Combines metrics across all sequences"""
+ res = {}
+ for header in self._additive_fields:
+ res[header] = _BaseMetric._combine_sum(all_res, header)
+ res.update(self._compute_final_fields(res))
+ return res
+
+ @staticmethod
+ def _compute_final_fields(additive):
+ final = {}
+ with np.errstate(invalid='ignore'): # Permit nan results.
+ final['ATA'] = (additive['STDA'] /
+ (0.5 * (additive['VACE_IDs'] + additive['VACE_GT_IDs'])))
+ final['SFDA'] = additive['FDA'] / additive['num_non_empty_timesteps']
+ return final
diff --git a/val_utils/trackeval/plotting.py b/val_utils/trackeval/plotting.py
new file mode 100644
index 0000000000000000000000000000000000000000..e76fd08c2733011a043de5cde3f16cfa4055268c
--- /dev/null
+++ b/val_utils/trackeval/plotting.py
@@ -0,0 +1,230 @@
+
+import os
+import numpy as np
+from .utils import TrackEvalException
+
+
+def plot_compare_trackers(tracker_folder, tracker_list, cls, output_folder, plots_list=None):
+ """Create plots which compare metrics across different trackers."""
+ # Define what to plot
+ if plots_list is None:
+ plots_list = get_default_plots_list()
+
+ # Load data
+ data = load_multiple_tracker_summaries(tracker_folder, tracker_list, cls)
+ out_loc = os.path.join(output_folder, cls)
+
+ # Plot
+ for args in plots_list:
+ create_comparison_plot(data, out_loc, *args)
+
+
+def get_default_plots_list():
+ # y_label, x_label, sort_label, bg_label, bg_function
+ plots_list = [
+ ['AssA', 'DetA', 'HOTA', 'HOTA', 'geometric_mean'],
+ ['AssPr', 'AssRe', 'HOTA', 'AssA', 'jaccard'],
+ ['DetPr', 'DetRe', 'HOTA', 'DetA', 'jaccard'],
+ ['HOTA(0)', 'LocA(0)', 'HOTA', 'HOTALocA(0)', 'multiplication'],
+ ['HOTA', 'LocA', 'HOTA', None, None],
+
+ ['HOTA', 'MOTA', 'HOTA', None, None],
+ ['HOTA', 'IDF1', 'HOTA', None, None],
+ ['IDF1', 'MOTA', 'HOTA', None, None],
+ ]
+ return plots_list
+
+
+def load_multiple_tracker_summaries(tracker_folder, tracker_list, cls):
+ """Loads summary data for multiple trackers."""
+ data = {}
+ for tracker in tracker_list:
+ with open(os.path.join(tracker_folder, tracker, cls + '_summary.txt')) as f:
+ keys = next(f).split(' ')
+ done = False
+ while not done:
+ values = next(f).split(' ')
+ if len(values) == len(keys):
+ done = True
+ data[tracker] = dict(zip(keys, map(float, values)))
+ return data
+
+
+def create_comparison_plot(data, out_loc, y_label, x_label, sort_label, bg_label=None, bg_function=None, settings=None):
+ """ Creates a scatter plot comparing multiple trackers between two metric fields, with one on the x-axis and the
+ other on the y axis. Adds pareto optical lines and (optionally) a background contour.
+
+ Inputs:
+ data: dict of dicts such that data[tracker_name][metric_field_name] = float
+ y_label: the metric_field_name to be plotted on the y-axis
+ x_label: the metric_field_name to be plotted on the x-axis
+ sort_label: the metric_field_name by which trackers are ordered and ranked
+ bg_label: the metric_field_name by which (optional) background contours are plotted
+ bg_function: the (optional) function bg_function(x,y) which converts the x_label / y_label values into bg_label.
+ settings: dict of plot settings with keys:
+ 'gap_val': gap between axis ticks and bg curves.
+ 'num_to_plot': maximum number of trackers to plot
+ """
+
+ # Only loaded when run to reduce minimum requirements
+ from matplotlib import pyplot as plt
+
+ # Get plot settings
+ if settings is None:
+ gap_val = 2
+ num_to_plot = 20
+ else:
+ gap_val = settings['gap_val']
+ num_to_plot = settings['num_to_plot']
+
+ if (bg_label is None) != (bg_function is None):
+ raise TrackEvalException('bg_function and bg_label must either be both given or neither given.')
+
+ # Extract data
+ tracker_names = np.array(list(data.keys()))
+ sort_index = np.array([data[t][sort_label] for t in tracker_names]).argsort()[::-1]
+ x_values = np.array([data[t][x_label] for t in tracker_names])[sort_index][:num_to_plot]
+ y_values = np.array([data[t][y_label] for t in tracker_names])[sort_index][:num_to_plot]
+
+ # Print info on what is being plotted
+ tracker_names = tracker_names[sort_index][:num_to_plot]
+ print('\nPlotting %s vs %s, for the following (ordered) trackers:' % (y_label, x_label))
+ for i, name in enumerate(tracker_names):
+ print('%i: %s' % (i+1, name))
+
+ # Find best fitting boundaries for data
+ boundaries = _get_boundaries(x_values, y_values, round_val=gap_val/2)
+
+ fig = plt.figure()
+
+ # Plot background contour
+ if bg_function is not None:
+ _plot_bg_contour(bg_function, boundaries, gap_val)
+
+ # Plot pareto optimal lines
+ _plot_pareto_optimal_lines(x_values, y_values)
+
+ # Plot data points with number labels
+ labels = np.arange(len(y_values)) + 1
+ plt.plot(x_values, y_values, 'b.', markersize=15)
+ for xx, yy, l in zip(x_values, y_values, labels):
+ plt.text(xx, yy, str(l), color="red", fontsize=15)
+
+ # Add extra explanatory text to plots
+ plt.text(0, -0.11, 'label order:\nHOTA', horizontalalignment='left', verticalalignment='center',
+ transform=fig.axes[0].transAxes, color="red", fontsize=12)
+ if bg_label is not None:
+ plt.text(1, -0.11, 'curve values:\n' + bg_label, horizontalalignment='right', verticalalignment='center',
+ transform=fig.axes[0].transAxes, color="grey", fontsize=12)
+
+ plt.xlabel(x_label, fontsize=15)
+ plt.ylabel(y_label, fontsize=15)
+ title = y_label + ' vs ' + x_label
+ if bg_label is not None:
+ title += ' (' + bg_label + ')'
+ plt.title(title, fontsize=17)
+ plt.xticks(np.arange(0, 100, gap_val))
+ plt.yticks(np.arange(0, 100, gap_val))
+ min_x, max_x, min_y, max_y = boundaries
+ plt.xlim(min_x, max_x)
+ plt.ylim(min_y, max_y)
+ plt.gca().set_aspect('equal', adjustable='box')
+ plt.tight_layout()
+
+ os.makedirs(out_loc, exist_ok=True)
+ filename = os.path.join(out_loc, title.replace(' ', '_'))
+ plt.savefig(filename + '.pdf', bbox_inches='tight', pad_inches=0.05)
+ plt.savefig(filename + '.png', bbox_inches='tight', pad_inches=0.05)
+
+
+def _get_boundaries(x_values, y_values, round_val):
+ x1 = np.min(np.floor((x_values - 0.5) / round_val) * round_val)
+ x2 = np.max(np.ceil((x_values + 0.5) / round_val) * round_val)
+ y1 = np.min(np.floor((y_values - 0.5) / round_val) * round_val)
+ y2 = np.max(np.ceil((y_values + 0.5) / round_val) * round_val)
+ x_range = x2 - x1
+ y_range = y2 - y1
+ max_range = max(x_range, y_range)
+ x_center = (x1 + x2) / 2
+ y_center = (y1 + y2) / 2
+ min_x = max(x_center - max_range / 2, 0)
+ max_x = min(x_center + max_range / 2, 100)
+ min_y = max(y_center - max_range / 2, 0)
+ max_y = min(y_center + max_range / 2, 100)
+ return min_x, max_x, min_y, max_y
+
+
+def geometric_mean(x, y):
+ return np.sqrt(x * y)
+
+
+def jaccard(x, y):
+ x = x / 100
+ y = y / 100
+ return 100 * (x * y) / (x + y - x * y)
+
+
+def multiplication(x, y):
+ return x * y / 100
+
+
+bg_function_dict = {
+ "geometric_mean": geometric_mean,
+ "jaccard": jaccard,
+ "multiplication": multiplication,
+ }
+
+
+def _plot_bg_contour(bg_function, plot_boundaries, gap_val):
+ """ Plot background contour. """
+
+ # Only loaded when run to reduce minimum requirements
+ from matplotlib import pyplot as plt
+
+ # Plot background contour
+ min_x, max_x, min_y, max_y = plot_boundaries
+ x = np.arange(min_x, max_x, 0.1)
+ y = np.arange(min_y, max_y, 0.1)
+ x_grid, y_grid = np.meshgrid(x, y)
+ if bg_function in bg_function_dict.keys():
+ z_grid = bg_function_dict[bg_function](x_grid, y_grid)
+ else:
+ raise TrackEvalException("background plotting function '%s' is not defined." % bg_function)
+ levels = np.arange(0, 100, gap_val)
+ con = plt.contour(x_grid, y_grid, z_grid, levels, colors='grey')
+
+ def bg_format(val):
+ s = '{:1f}'.format(val)
+ return '{:.0f}'.format(val) if s[-1] == '0' else s
+
+ con.levels = [bg_format(val) for val in con.levels]
+ plt.clabel(con, con.levels, inline=True, fmt='%r', fontsize=8)
+
+
+def _plot_pareto_optimal_lines(x_values, y_values):
+ """ Plot pareto optimal lines """
+
+ # Only loaded when run to reduce minimum requirements
+ from matplotlib import pyplot as plt
+
+ # Plot pareto optimal lines
+ cxs = x_values
+ cys = y_values
+ best_y = np.argmax(cys)
+ x_pareto = [0, cxs[best_y]]
+ y_pareto = [cys[best_y], cys[best_y]]
+ t = 2
+ remaining = cxs > x_pareto[t - 1]
+ cys = cys[remaining]
+ cxs = cxs[remaining]
+ while len(cxs) > 0 and len(cys) > 0:
+ best_y = np.argmax(cys)
+ x_pareto += [x_pareto[t - 1], cxs[best_y]]
+ y_pareto += [cys[best_y], cys[best_y]]
+ t += 2
+ remaining = cxs > x_pareto[t - 1]
+ cys = cys[remaining]
+ cxs = cxs[remaining]
+ x_pareto.append(x_pareto[t - 1])
+ y_pareto.append(0)
+ plt.plot(np.array(x_pareto), np.array(y_pareto), '--r')
diff --git a/val_utils/trackeval/utils.py b/val_utils/trackeval/utils.py
new file mode 100644
index 0000000000000000000000000000000000000000..8c7c9167fe17a6d393419f14dc512c8d325b8ed2
--- /dev/null
+++ b/val_utils/trackeval/utils.py
@@ -0,0 +1,146 @@
+
+import os
+import csv
+import argparse
+from collections import OrderedDict
+
+
+def init_config(config, default_config, name=None):
+ """Initialise non-given config values with defaults"""
+ if config is None:
+ config = default_config
+ else:
+ for k in default_config.keys():
+ if k not in config.keys():
+ config[k] = default_config[k]
+ if name and config['PRINT_CONFIG']:
+ print('\n%s Config:' % name)
+ for c in config.keys():
+ print('%-20s : %-30s' % (c, config[c]))
+ return config
+
+
+def update_config(config):
+ """
+ Parse the arguments of a script and updates the config values for a given value if specified in the arguments.
+ :param config: the config to update
+ :return: the updated config
+ """
+ parser = argparse.ArgumentParser()
+ for setting in config.keys():
+ if type(config[setting]) == list or type(config[setting]) == type(None):
+ parser.add_argument("--" + setting, nargs='+')
+ else:
+ parser.add_argument("--" + setting)
+ args = parser.parse_args().__dict__
+ for setting in args.keys():
+ if args[setting] is not None:
+ if type(config[setting]) == type(True):
+ if args[setting] == 'True':
+ x = True
+ elif args[setting] == 'False':
+ x = False
+ else:
+ raise Exception('Command line parameter ' + setting + 'must be True or False')
+ elif type(config[setting]) == type(1):
+ x = int(args[setting])
+ elif type(args[setting]) == type(None):
+ x = None
+ else:
+ x = args[setting]
+ config[setting] = x
+ return config
+
+
+def get_code_path():
+ """Get base path where code is"""
+ return os.path.abspath(os.path.join(os.path.dirname(__file__), '..'))
+
+
+def validate_metrics_list(metrics_list):
+ """Get names of metric class and ensures they are unique, further checks that the fields within each metric class
+ do not have overlapping names.
+ """
+ metric_names = [metric.get_name() for metric in metrics_list]
+ # check metric names are unique
+ if len(metric_names) != len(set(metric_names)):
+ raise TrackEvalException('Code being run with multiple metrics of the same name')
+ fields = []
+ for m in metrics_list:
+ fields += m.fields
+ # check metric fields are unique
+ if len(fields) != len(set(fields)):
+ raise TrackEvalException('Code being run with multiple metrics with fields of the same name')
+ return metric_names
+
+
+def write_summary_results(summaries, cls, output_folder):
+ """Write summary results to file"""
+
+ fields = sum([list(s.keys()) for s in summaries], [])
+ values = sum([list(s.values()) for s in summaries], [])
+
+ # In order to remain consistent upon new fields being adding, for each of the following fields if they are present
+ # they will be output in the summary first in the order below. Any further fields will be output in the order each
+ # metric family is called, and within each family either in the order they were added to the dict (python >= 3.6) or
+ # randomly (python < 3.6).
+ default_order = ['HOTA', 'DetA', 'AssA', 'DetRe', 'DetPr', 'AssRe', 'AssPr', 'LocA', 'OWTA', 'HOTA(0)', 'LocA(0)',
+ 'HOTALocA(0)', 'MOTA', 'MOTP', 'MODA', 'CLR_Re', 'CLR_Pr', 'MTR', 'PTR', 'MLR', 'CLR_TP', 'CLR_FN',
+ 'CLR_FP', 'IDSW', 'MT', 'PT', 'ML', 'Frag', 'sMOTA', 'IDF1', 'IDR', 'IDP', 'IDTP', 'IDFN', 'IDFP',
+ 'Dets', 'GT_Dets', 'IDs', 'GT_IDs']
+ default_ordered_dict = OrderedDict(zip(default_order, [None for _ in default_order]))
+ for f, v in zip(fields, values):
+ default_ordered_dict[f] = v
+ for df in default_order:
+ if default_ordered_dict[df] is None:
+ del default_ordered_dict[df]
+ fields = list(default_ordered_dict.keys())
+ values = list(default_ordered_dict.values())
+
+ out_file = os.path.join(output_folder, cls + '_summary.txt')
+ os.makedirs(os.path.dirname(out_file), exist_ok=True)
+ with open(out_file, 'w', newline='') as f:
+ writer = csv.writer(f, delimiter=' ')
+ writer.writerow(fields)
+ writer.writerow(values)
+
+
+def write_detailed_results(details, cls, output_folder):
+ """Write detailed results to file"""
+ sequences = details[0].keys()
+ fields = ['seq'] + sum([list(s['COMBINED_SEQ'].keys()) for s in details], [])
+ out_file = os.path.join(output_folder, cls + '_detailed.csv')
+ os.makedirs(os.path.dirname(out_file), exist_ok=True)
+ with open(out_file, 'w', newline='') as f:
+ writer = csv.writer(f)
+ writer.writerow(fields)
+ for seq in sorted(sequences):
+ if seq == 'COMBINED_SEQ':
+ continue
+ writer.writerow([seq] + sum([list(s[seq].values()) for s in details], []))
+ writer.writerow(['COMBINED'] + sum([list(s['COMBINED_SEQ'].values()) for s in details], []))
+
+
+def load_detail(file):
+ """Loads detailed data for a tracker."""
+ data = {}
+ with open(file) as f:
+ for i, row_text in enumerate(f):
+ row = row_text.replace('\r', '').replace('\n', '').split(',')
+ if i == 0:
+ keys = row[1:]
+ continue
+ current_values = row[1:]
+ seq = row[0]
+ if seq == 'COMBINED':
+ seq = 'COMBINED_SEQ'
+ if (len(current_values) == len(keys)) and seq != '':
+ data[seq] = {}
+ for key, value in zip(keys, current_values):
+ data[seq][key] = float(value)
+ return data
+
+
+class TrackEvalException(Exception):
+ """Custom exception for catching expected errors."""
+ ...
diff --git a/video/4.mp4 b/video/4.mp4
new file mode 100644
index 0000000000000000000000000000000000000000..92274d948d7ad3794dd28efef060c393057379b3
--- /dev/null
+++ b/video/4.mp4
@@ -0,0 +1,3 @@
+version https://git-lfs.github.com/spec/v1
+oid sha256:82ca0e0d5162d0024feaaf6a106974fb39927c88b8aa7c008363847f5a20376d
+size 10121349
diff --git a/video/5.mp4 b/video/5.mp4
new file mode 100644
index 0000000000000000000000000000000000000000..70739aff733b367e6d7bb0fd6dfe8a36490246ac
--- /dev/null
+++ b/video/5.mp4
@@ -0,0 +1,3 @@
+version https://git-lfs.github.com/spec/v1
+oid sha256:55746f7b652b7f2f549db2ec7b2c94886f1b6f8378061acaacbd14770a44dcdf
+size 10144209
diff --git a/video/8.mp4 b/video/8.mp4
new file mode 100644
index 0000000000000000000000000000000000000000..5987cb986ccdf61ea737379e787c027efb9da031
Binary files /dev/null and b/video/8.mp4 differ
diff --git a/video/9.mp4 b/video/9.mp4
new file mode 100644
index 0000000000000000000000000000000000000000..4ef15b41d0b6a98cb61a546cf45070dbb1c2cb82
--- /dev/null
+++ b/video/9.mp4
@@ -0,0 +1,3 @@
+version https://git-lfs.github.com/spec/v1
+oid sha256:6f30901e2c64c4e35ffb789b95de2692d1e319c12ba3f3e40bc53da5f21af506
+size 6177301
diff --git a/video/bicyclecity.mp4 b/video/bicyclecity.mp4
new file mode 100644
index 0000000000000000000000000000000000000000..c024bc30fed7a7a2351b9e189fd04ce56adedf65
--- /dev/null
+++ b/video/bicyclecity.mp4
@@ -0,0 +1,3 @@
+version https://git-lfs.github.com/spec/v1
+oid sha256:233bd31fcaae40185e8b7e957d442c324cf9c5c8d6c864e58321f4452b57472e
+size 1099590
diff --git a/video/car.mp4 b/video/car.mp4
new file mode 100644
index 0000000000000000000000000000000000000000..c68be8e5394e247c75e9ead00f7353fcbeccf8bd
--- /dev/null
+++ b/video/car.mp4
@@ -0,0 +1,3 @@
+version https://git-lfs.github.com/spec/v1
+oid sha256:69b6792e068380e5389c1aee24ec7411e6f01b541e612faf3d516018ff364591
+size 1276768
diff --git a/yolov5/.cometml-runs/074c70a5eb3b459082fea571bc2c711b.zip b/yolov5/.cometml-runs/074c70a5eb3b459082fea571bc2c711b.zip
new file mode 100644
index 0000000000000000000000000000000000000000..3f8340ee9100c72e71befa19619f05c80041899c
--- /dev/null
+++ b/yolov5/.cometml-runs/074c70a5eb3b459082fea571bc2c711b.zip
@@ -0,0 +1,3 @@
+version https://git-lfs.github.com/spec/v1
+oid sha256:52b25243b18cfd3e8a7fa9c550120f9540ff70114bc01bce5f6d7e33a8eb130e
+size 35668
diff --git a/yolov5/.cometml-runs/0ae5485f8ae643bd8bb48922a165e199.zip b/yolov5/.cometml-runs/0ae5485f8ae643bd8bb48922a165e199.zip
new file mode 100644
index 0000000000000000000000000000000000000000..36f761ffd74ca50f477aefb3d86f4e71da2e7903
--- /dev/null
+++ b/yolov5/.cometml-runs/0ae5485f8ae643bd8bb48922a165e199.zip
@@ -0,0 +1,3 @@
+version https://git-lfs.github.com/spec/v1
+oid sha256:20752f6449aaaf99c96c2ce104aac4c187606ddf63937d9d70827bc41a361c67
+size 28380
diff --git a/yolov5/.cometml-runs/81b2b6d464c04bba8c5860481e0293b3.zip b/yolov5/.cometml-runs/81b2b6d464c04bba8c5860481e0293b3.zip
new file mode 100644
index 0000000000000000000000000000000000000000..f56b821581615644c9e462d8e3b447cefc8f0ed3
--- /dev/null
+++ b/yolov5/.cometml-runs/81b2b6d464c04bba8c5860481e0293b3.zip
@@ -0,0 +1,3 @@
+version https://git-lfs.github.com/spec/v1
+oid sha256:c9127768b47e219a3eff5346700915c9a521a8b20576c91a42b50e1a3911c997
+size 28380
diff --git a/yolov5/.dockerignore b/yolov5/.dockerignore
new file mode 100644
index 0000000000000000000000000000000000000000..3b669254e7799c0460d6be8523ed15ef1d2c3ac6
--- /dev/null
+++ b/yolov5/.dockerignore
@@ -0,0 +1,222 @@
+# Repo-specific DockerIgnore -------------------------------------------------------------------------------------------
+.git
+.cache
+.idea
+runs
+output
+coco
+storage.googleapis.com
+
+data/samples/*
+**/results*.csv
+*.jpg
+
+# Neural Network weights -----------------------------------------------------------------------------------------------
+**/*.pt
+**/*.pth
+**/*.onnx
+**/*.engine
+**/*.mlmodel
+**/*.torchscript
+**/*.torchscript.pt
+**/*.tflite
+**/*.h5
+**/*.pb
+*_saved_model/
+*_web_model/
+*_openvino_model/
+
+# Below Copied From .gitignore -----------------------------------------------------------------------------------------
+# Below Copied From .gitignore -----------------------------------------------------------------------------------------
+
+
+# GitHub Python GitIgnore ----------------------------------------------------------------------------------------------
+# Byte-compiled / optimized / DLL files
+__pycache__/
+*.py[cod]
+*$py.class
+
+# C extensions
+*.so
+
+# Distribution / packaging
+.Python
+env/
+build/
+develop-eggs/
+dist/
+downloads/
+eggs/
+.eggs/
+lib/
+lib64/
+parts/
+sdist/
+var/
+wheels/
+*.egg-info/
+wandb/
+.installed.cfg
+*.egg
+
+# PyInstaller
+# Usually these files are written by a python script from a template
+# before PyInstaller builds the exe, so as to inject date/other infos into it.
+*.manifest
+*.spec
+
+# Installer logs
+pip-log.txt
+pip-delete-this-directory.txt
+
+# Unit test / coverage reports
+htmlcov/
+.tox/
+.coverage
+.coverage.*
+.cache
+nosetests.xml
+coverage.xml
+*.cover
+.hypothesis/
+
+# Translations
+*.mo
+*.pot
+
+# Django stuff:
+*.log
+local_settings.py
+
+# Flask stuff:
+instance/
+.webassets-cache
+
+# Scrapy stuff:
+.scrapy
+
+# Sphinx documentation
+docs/_build/
+
+# PyBuilder
+target/
+
+# Jupyter Notebook
+.ipynb_checkpoints
+
+# pyenv
+.python-version
+
+# celery beat schedule file
+celerybeat-schedule
+
+# SageMath parsed files
+*.sage.py
+
+# dotenv
+.env
+
+# virtualenv
+.venv*
+venv*/
+ENV*/
+
+# Spyder project settings
+.spyderproject
+.spyproject
+
+# Rope project settings
+.ropeproject
+
+# mkdocs documentation
+/site
+
+# mypy
+.mypy_cache/
+
+
+# https://github.com/github/gitignore/blob/master/Global/macOS.gitignore -----------------------------------------------
+
+# General
+.DS_Store
+.AppleDouble
+.LSOverride
+
+# Icon must end with two \r
+Icon
+Icon?
+
+# Thumbnails
+._*
+
+# Files that might appear in the root of a volume
+.DocumentRevisions-V100
+.fseventsd
+.Spotlight-V100
+.TemporaryItems
+.Trashes
+.VolumeIcon.icns
+.com.apple.timemachine.donotpresent
+
+# Directories potentially created on remote AFP share
+.AppleDB
+.AppleDesktop
+Network Trash Folder
+Temporary Items
+.apdisk
+
+
+# https://github.com/github/gitignore/blob/master/Global/JetBrains.gitignore
+# Covers JetBrains IDEs: IntelliJ, RubyMine, PhpStorm, AppCode, PyCharm, CLion, Android Studio and WebStorm
+# Reference: https://intellij-support.jetbrains.com/hc/en-us/articles/206544839
+
+# User-specific stuff:
+.idea/*
+.idea/**/workspace.xml
+.idea/**/tasks.xml
+.idea/dictionaries
+.html # Bokeh Plots
+.pg # TensorFlow Frozen Graphs
+.avi # videos
+
+# Sensitive or high-churn files:
+.idea/**/dataSources/
+.idea/**/dataSources.ids
+.idea/**/dataSources.local.xml
+.idea/**/sqlDataSources.xml
+.idea/**/dynamic.xml
+.idea/**/uiDesigner.xml
+
+# Gradle:
+.idea/**/gradle.xml
+.idea/**/libraries
+
+# CMake
+cmake-build-debug/
+cmake-build-release/
+
+# Mongo Explorer plugin:
+.idea/**/mongoSettings.xml
+
+## File-based project format:
+*.iws
+
+## Plugin-specific files:
+
+# IntelliJ
+out/
+
+# mpeltonen/sbt-idea plugin
+.idea_modules/
+
+# JIRA plugin
+atlassian-ide-plugin.xml
+
+# Cursive Clojure plugin
+.idea/replstate.xml
+
+# Crashlytics plugin (for Android Studio and IntelliJ)
+com_crashlytics_export_strings.xml
+crashlytics.properties
+crashlytics-build.properties
+fabric.properties
diff --git a/yolov5/.gitattributes b/yolov5/.gitattributes
new file mode 100644
index 0000000000000000000000000000000000000000..dad4239ebad5b72917cbc4bba95206c1e55d519e
--- /dev/null
+++ b/yolov5/.gitattributes
@@ -0,0 +1,2 @@
+# this drop notebooks from GitHub language stats
+*.ipynb linguist-vendored
diff --git a/yolov5/.github/ISSUE_TEMPLATE/bug-report.yml b/yolov5/.github/ISSUE_TEMPLATE/bug-report.yml
new file mode 100644
index 0000000000000000000000000000000000000000..04f9c76fde1f6b9887f3145af3b89030edba223f
--- /dev/null
+++ b/yolov5/.github/ISSUE_TEMPLATE/bug-report.yml
@@ -0,0 +1,85 @@
+name: 🐛 Bug Report
+# title: " "
+description: Problems with YOLOv5
+labels: [bug, triage]
+body:
+ - type: markdown
+ attributes:
+ value: |
+ Thank you for submitting a YOLOv5 🐛 Bug Report!
+
+ - type: checkboxes
+ attributes:
+ label: Search before asking
+ description: >
+ Please search the [issues](https://github.com/ultralytics/yolov5/issues) to see if a similar bug report already exists.
+ options:
+ - label: >
+ I have searched the YOLOv5 [issues](https://github.com/ultralytics/yolov5/issues) and found no similar bug report.
+ required: true
+
+ - type: dropdown
+ attributes:
+ label: YOLOv5 Component
+ description: |
+ Please select the part of YOLOv5 where you found the bug.
+ multiple: true
+ options:
+ - "Training"
+ - "Validation"
+ - "Detection"
+ - "Export"
+ - "PyTorch Hub"
+ - "Multi-GPU"
+ - "Evolution"
+ - "Integrations"
+ - "Other"
+ validations:
+ required: false
+
+ - type: textarea
+ attributes:
+ label: Bug
+ description: Provide console output with error messages and/or screenshots of the bug.
+ placeholder: |
+ 💡 ProTip! Include as much information as possible (screenshots, logs, tracebacks etc.) to receive the most helpful response.
+ validations:
+ required: true
+
+ - type: textarea
+ attributes:
+ label: Environment
+ description: Please specify the software and hardware you used to produce the bug.
+ placeholder: |
+ - YOLO: YOLOv5 🚀 v6.0-67-g60e42e1 torch 1.9.0+cu111 CUDA:0 (A100-SXM4-40GB, 40536MiB)
+ - OS: Ubuntu 20.04
+ - Python: 3.9.0
+ validations:
+ required: false
+
+ - type: textarea
+ attributes:
+ label: Minimal Reproducible Example
+ description: >
+ When asking a question, people will be better able to provide help if you provide code that they can easily understand and use to **reproduce** the problem.
+ This is referred to by community members as creating a [minimal reproducible example](https://docs.ultralytics.com/help/minimum_reproducible_example/).
+ placeholder: |
+ ```
+ # Code to reproduce your issue here
+ ```
+ validations:
+ required: false
+
+ - type: textarea
+ attributes:
+ label: Additional
+ description: Anything else you would like to share?
+
+ - type: checkboxes
+ attributes:
+ label: Are you willing to submit a PR?
+ description: >
+ (Optional) We encourage you to submit a [Pull Request](https://github.com/ultralytics/yolov5/pulls) (PR) to help improve YOLOv5 for everyone, especially if you have a good understanding of how to implement a fix or feature.
+ See the YOLOv5 [Contributing Guide](https://docs.ultralytics.com/help/contributing) to get started.
+ options:
+ - label: Yes I'd like to help by submitting a PR!
diff --git a/yolov5/.github/ISSUE_TEMPLATE/config.yml b/yolov5/.github/ISSUE_TEMPLATE/config.yml
new file mode 100644
index 0000000000000000000000000000000000000000..743feb957ff1fea2c5fbf52910fa89d6de9392d3
--- /dev/null
+++ b/yolov5/.github/ISSUE_TEMPLATE/config.yml
@@ -0,0 +1,11 @@
+blank_issues_enabled: true
+contact_links:
+ - name: 📄 Docs
+ url: https://docs.ultralytics.com/yolov5
+ about: View Ultralytics YOLOv5 Docs
+ - name: 💬 Forum
+ url: https://community.ultralytics.com/
+ about: Ask on Ultralytics Community Forum
+ - name: 🎧 Discord
+ url: https://discord.gg/n6cFeSPZdD
+ about: Ask on Ultralytics Discord
diff --git a/yolov5/.github/ISSUE_TEMPLATE/feature-request.yml b/yolov5/.github/ISSUE_TEMPLATE/feature-request.yml
new file mode 100644
index 0000000000000000000000000000000000000000..1d3d53df217e03fddd68d35bc3017586a86b37e1
--- /dev/null
+++ b/yolov5/.github/ISSUE_TEMPLATE/feature-request.yml
@@ -0,0 +1,50 @@
+name: 🚀 Feature Request
+description: Suggest a YOLOv5 idea
+# title: " "
+labels: [enhancement]
+body:
+ - type: markdown
+ attributes:
+ value: |
+ Thank you for submitting a YOLOv5 🚀 Feature Request!
+
+ - type: checkboxes
+ attributes:
+ label: Search before asking
+ description: >
+ Please search the [issues](https://github.com/ultralytics/yolov5/issues) to see if a similar feature request already exists.
+ options:
+ - label: >
+ I have searched the YOLOv5 [issues](https://github.com/ultralytics/yolov5/issues) and found no similar feature requests.
+ required: true
+
+ - type: textarea
+ attributes:
+ label: Description
+ description: A short description of your feature.
+ placeholder: |
+ What new feature would you like to see in YOLOv5?
+ validations:
+ required: true
+
+ - type: textarea
+ attributes:
+ label: Use case
+ description: |
+ Describe the use case of your feature request. It will help us understand and prioritize the feature request.
+ placeholder: |
+ How would this feature be used, and who would use it?
+
+ - type: textarea
+ attributes:
+ label: Additional
+ description: Anything else you would like to share?
+
+ - type: checkboxes
+ attributes:
+ label: Are you willing to submit a PR?
+ description: >
+ (Optional) We encourage you to submit a [Pull Request](https://github.com/ultralytics/yolov5/pulls) (PR) to help improve YOLOv5 for everyone, especially if you have a good understanding of how to implement a fix or feature.
+ See the YOLOv5 [Contributing Guide](https://docs.ultralytics.com/help/contributing) to get started.
+ options:
+ - label: Yes I'd like to help by submitting a PR!
diff --git a/yolov5/.github/ISSUE_TEMPLATE/question.yml b/yolov5/.github/ISSUE_TEMPLATE/question.yml
new file mode 100644
index 0000000000000000000000000000000000000000..8e0993c68bab9170d972189cffeaf106c3222ae0
--- /dev/null
+++ b/yolov5/.github/ISSUE_TEMPLATE/question.yml
@@ -0,0 +1,33 @@
+name: ❓ Question
+description: Ask a YOLOv5 question
+# title: " "
+labels: [question]
+body:
+ - type: markdown
+ attributes:
+ value: |
+ Thank you for asking a YOLOv5 ❓ Question!
+
+ - type: checkboxes
+ attributes:
+ label: Search before asking
+ description: >
+ Please search the [issues](https://github.com/ultralytics/yolov5/issues) and [discussions](https://github.com/ultralytics/yolov5/discussions) to see if a similar question already exists.
+ options:
+ - label: >
+ I have searched the YOLOv5 [issues](https://github.com/ultralytics/yolov5/issues) and [discussions](https://github.com/ultralytics/yolov5/discussions) and found no similar questions.
+ required: true
+
+ - type: textarea
+ attributes:
+ label: Question
+ description: What is your question?
+ placeholder: |
+ 💡 ProTip! Include as much information as possible (screenshots, logs, tracebacks etc.) to receive the most helpful response.
+ validations:
+ required: true
+
+ - type: textarea
+ attributes:
+ label: Additional
+ description: Anything else you would like to share?
diff --git a/yolov5/.github/PULL_REQUEST_TEMPLATE.md b/yolov5/.github/PULL_REQUEST_TEMPLATE.md
new file mode 100644
index 0000000000000000000000000000000000000000..d96d5afd283618f483e28c0f29c8d8886c3520ae
--- /dev/null
+++ b/yolov5/.github/PULL_REQUEST_TEMPLATE.md
@@ -0,0 +1,13 @@
+
+
+copilot:all
diff --git a/yolov5/.github/dependabot.yml b/yolov5/.github/dependabot.yml
new file mode 100644
index 0000000000000000000000000000000000000000..c1b3d5d514c392d668238e1215e450b1d25e5ef4
--- /dev/null
+++ b/yolov5/.github/dependabot.yml
@@ -0,0 +1,23 @@
+version: 2
+updates:
+ - package-ecosystem: pip
+ directory: "/"
+ schedule:
+ interval: weekly
+ time: "04:00"
+ open-pull-requests-limit: 10
+ reviewers:
+ - glenn-jocher
+ labels:
+ - dependencies
+
+ - package-ecosystem: github-actions
+ directory: "/"
+ schedule:
+ interval: weekly
+ time: "04:00"
+ open-pull-requests-limit: 5
+ reviewers:
+ - glenn-jocher
+ labels:
+ - dependencies
diff --git a/yolov5/.github/workflows/ci-testing.yml b/yolov5/.github/workflows/ci-testing.yml
new file mode 100644
index 0000000000000000000000000000000000000000..e71a4b8f16ac124472ccf697f04212b096d29539
--- /dev/null
+++ b/yolov5/.github/workflows/ci-testing.yml
@@ -0,0 +1,167 @@
+# YOLOv5 🚀 by Ultralytics, AGPL-3.0 license
+# YOLOv5 Continuous Integration (CI) GitHub Actions tests
+
+name: YOLOv5 CI
+
+on:
+ push:
+ branches: [ master ]
+ pull_request:
+ branches: [ master ]
+ schedule:
+ - cron: '0 0 * * *' # runs at 00:00 UTC every day
+
+jobs:
+ Benchmarks:
+ runs-on: ${{ matrix.os }}
+ strategy:
+ fail-fast: false
+ matrix:
+ os: [ ubuntu-latest ]
+ python-version: [ '3.10' ] # requires python<=3.10
+ model: [ yolov5n ]
+ steps:
+ - uses: actions/checkout@v3
+ - uses: actions/setup-python@v4
+ with:
+ python-version: ${{ matrix.python-version }}
+ cache: 'pip' # caching pip dependencies
+ - name: Install requirements
+ run: |
+ python -m pip install --upgrade pip wheel
+ pip install -r requirements.txt coremltools openvino-dev tensorflow-cpu --extra-index-url https://download.pytorch.org/whl/cpu
+ python --version
+ pip --version
+ pip list
+ - name: Benchmark DetectionModel
+ run: |
+ python benchmarks.py --data coco128.yaml --weights ${{ matrix.model }}.pt --img 320 --hard-fail 0.29
+ - name: Benchmark SegmentationModel
+ run: |
+ python benchmarks.py --data coco128-seg.yaml --weights ${{ matrix.model }}-seg.pt --img 320 --hard-fail 0.22
+ - name: Test predictions
+ run: |
+ python export.py --weights ${{ matrix.model }}-cls.pt --include onnx --img 224
+ python detect.py --weights ${{ matrix.model }}.onnx --img 320
+ python segment/predict.py --weights ${{ matrix.model }}-seg.onnx --img 320
+ python classify/predict.py --weights ${{ matrix.model }}-cls.onnx --img 224
+
+ Tests:
+ timeout-minutes: 60
+ runs-on: ${{ matrix.os }}
+ strategy:
+ fail-fast: false
+ matrix:
+ os: [ ubuntu-latest, windows-latest ] # macos-latest bug https://github.com/ultralytics/yolov5/pull/9049
+ python-version: [ '3.10' ]
+ model: [ yolov5n ]
+ include:
+ - os: ubuntu-latest
+ python-version: '3.7' # '3.6.8' min
+ model: yolov5n
+ - os: ubuntu-latest
+ python-version: '3.8'
+ model: yolov5n
+ - os: ubuntu-latest
+ python-version: '3.9'
+ model: yolov5n
+ - os: ubuntu-latest
+ python-version: '3.8' # torch 1.7.0 requires python >=3.6, <=3.8
+ model: yolov5n
+ torch: '1.7.0' # min torch version CI https://pypi.org/project/torchvision/
+ steps:
+ - uses: actions/checkout@v3
+ - uses: actions/setup-python@v4
+ with:
+ python-version: ${{ matrix.python-version }}
+ cache: 'pip' # caching pip dependencies
+ - name: Install requirements
+ run: |
+ python -m pip install --upgrade pip wheel
+ if [ "${{ matrix.torch }}" == "1.7.0" ]; then
+ pip install -r requirements.txt torch==1.7.0 torchvision==0.8.1 --extra-index-url https://download.pytorch.org/whl/cpu
+ else
+ pip install -r requirements.txt --extra-index-url https://download.pytorch.org/whl/cpu
+ fi
+ shell: bash # for Windows compatibility
+ - name: Check environment
+ run: |
+ python -c "import utils; utils.notebook_init()"
+ echo "RUNNER_OS is ${{ runner.os }}"
+ echo "GITHUB_EVENT_NAME is ${{ github.event_name }}"
+ echo "GITHUB_WORKFLOW is ${{ github.workflow }}"
+ echo "GITHUB_ACTOR is ${{ github.actor }}"
+ echo "GITHUB_REPOSITORY is ${{ github.repository }}"
+ echo "GITHUB_REPOSITORY_OWNER is ${{ github.repository_owner }}"
+ python --version
+ pip --version
+ pip list
+ - name: Test detection
+ shell: bash # for Windows compatibility
+ run: |
+ # export PYTHONPATH="$PWD" # to run '$ python *.py' files in subdirectories
+ m=${{ matrix.model }} # official weights
+ b=runs/train/exp/weights/best # best.pt checkpoint
+ python train.py --imgsz 64 --batch 32 --weights $m.pt --cfg $m.yaml --epochs 1 --device cpu # train
+ for d in cpu; do # devices
+ for w in $m $b; do # weights
+ python val.py --imgsz 64 --batch 32 --weights $w.pt --device $d # val
+ python detect.py --imgsz 64 --weights $w.pt --device $d # detect
+ done
+ done
+ python hubconf.py --model $m # hub
+ # python models/tf.py --weights $m.pt # build TF model
+ python models/yolo.py --cfg $m.yaml # build PyTorch model
+ python export.py --weights $m.pt --img 64 --include torchscript # export
+ python - < GitHub Actions error for ${{ github.workflow }} ❌\n\n\n*Repository:* https://github.com/${{ github.repository }}\n*Action:* https://github.com/${{ github.repository }}/actions/runs/${{ github.run_id }}\n*Author:* ${{ github.actor }}\n*Event:* ${{ github.event_name }}\n"}
+ env:
+ SLACK_WEBHOOK_URL: ${{ secrets.SLACK_WEBHOOK_URL_YOLO }}
diff --git a/yolov5/.github/workflows/codeql-analysis.yml b/yolov5/.github/workflows/codeql-analysis.yml
new file mode 100644
index 0000000000000000000000000000000000000000..05db12dabd1ab3eea34d088daed702b4c09509c5
--- /dev/null
+++ b/yolov5/.github/workflows/codeql-analysis.yml
@@ -0,0 +1,55 @@
+# This action runs GitHub's industry-leading static analysis engine, CodeQL, against a repository's source code to find security vulnerabilities.
+# https://github.com/github/codeql-action
+
+name: "CodeQL"
+
+on:
+ schedule:
+ - cron: '0 0 1 * *' # Runs at 00:00 UTC on the 1st of every month
+ workflow_dispatch:
+
+jobs:
+ analyze:
+ name: Analyze
+ runs-on: ubuntu-latest
+
+ strategy:
+ fail-fast: false
+ matrix:
+ language: ['python']
+ # CodeQL supports [ 'cpp', 'csharp', 'go', 'java', 'javascript', 'python' ]
+ # Learn more:
+ # https://docs.github.com/en/free-pro-team@latest/github/finding-security-vulnerabilities-and-errors-in-your-code/configuring-code-scanning#changing-the-languages-that-are-analyzed
+
+ steps:
+ - name: Checkout repository
+ uses: actions/checkout@v3
+
+ # Initializes the CodeQL tools for scanning.
+ - name: Initialize CodeQL
+ uses: github/codeql-action/init@v2
+ with:
+ languages: ${{ matrix.language }}
+ # If you wish to specify custom queries, you can do so here or in a config file.
+ # By default, queries listed here will override any specified in a config file.
+ # Prefix the list here with "+" to use these queries and those in the config file.
+ # queries: ./path/to/local/query, your-org/your-repo/queries@main
+
+ # Autobuild attempts to build any compiled languages (C/C++, C#, or Java).
+ # If this step fails, then you should remove it and run the build manually (see below)
+ - name: Autobuild
+ uses: github/codeql-action/autobuild@v2
+
+ # ℹ️ Command-line programs to run using the OS shell.
+ # 📚 https://git.io/JvXDl
+
+ # ✏️ If the Autobuild fails above, remove it and uncomment the following three lines
+ # and modify them (or add more) to build your code if your project
+ # uses a compiled language
+
+ #- run: |
+ # make bootstrap
+ # make release
+
+ - name: Perform CodeQL Analysis
+ uses: github/codeql-action/analyze@v2
diff --git a/yolov5/.github/workflows/docker.yml b/yolov5/.github/workflows/docker.yml
new file mode 100644
index 0000000000000000000000000000000000000000..13e79216fc2092b6a97ce89dc35eb53f2b3ead88
--- /dev/null
+++ b/yolov5/.github/workflows/docker.yml
@@ -0,0 +1,58 @@
+# YOLOv5 🚀 by Ultralytics, AGPL-3.0 license
+# Builds ultralytics/yolov5:latest images on DockerHub https://hub.docker.com/r/ultralytics/yolov5
+
+name: Publish Docker Images
+
+on:
+ push:
+ branches: [ master ]
+ workflow_dispatch:
+
+jobs:
+ docker:
+ if: github.repository == 'ultralytics/yolov5'
+ name: Push Docker image to Docker Hub
+ runs-on: ubuntu-latest
+ steps:
+ - name: Checkout repo
+ uses: actions/checkout@v3
+
+ - name: Set up QEMU
+ uses: docker/setup-qemu-action@v2
+
+ - name: Set up Docker Buildx
+ uses: docker/setup-buildx-action@v2
+
+ - name: Login to Docker Hub
+ uses: docker/login-action@v2
+ with:
+ username: ${{ secrets.DOCKERHUB_USERNAME }}
+ password: ${{ secrets.DOCKERHUB_TOKEN }}
+
+ - name: Build and push arm64 image
+ uses: docker/build-push-action@v4
+ continue-on-error: true
+ with:
+ context: .
+ platforms: linux/arm64
+ file: utils/docker/Dockerfile-arm64
+ push: true
+ tags: ultralytics/yolov5:latest-arm64
+
+ - name: Build and push CPU image
+ uses: docker/build-push-action@v4
+ continue-on-error: true
+ with:
+ context: .
+ file: utils/docker/Dockerfile-cpu
+ push: true
+ tags: ultralytics/yolov5:latest-cpu
+
+ - name: Build and push GPU image
+ uses: docker/build-push-action@v4
+ continue-on-error: true
+ with:
+ context: .
+ file: utils/docker/Dockerfile
+ push: true
+ tags: ultralytics/yolov5:latest
diff --git a/yolov5/.github/workflows/greetings.yml b/yolov5/.github/workflows/greetings.yml
new file mode 100644
index 0000000000000000000000000000000000000000..8aca12d3c3705bc535cabe1bb9c7d2dcba00d368
--- /dev/null
+++ b/yolov5/.github/workflows/greetings.yml
@@ -0,0 +1,65 @@
+# YOLOv5 🚀 by Ultralytics, AGPL-3.0 license
+
+name: Greetings
+
+on:
+ pull_request_target:
+ types: [opened]
+ issues:
+ types: [opened]
+
+jobs:
+ greeting:
+ runs-on: ubuntu-latest
+ steps:
+ - uses: actions/first-interaction@v1
+ with:
+ repo-token: ${{ secrets.GITHUB_TOKEN }}
+ pr-message: |
+ 👋 Hello @${{ github.actor }}, thank you for submitting a YOLOv5 🚀 PR! To allow your work to be integrated as seamlessly as possible, we advise you to:
+
+ - ✅ Verify your PR is **up-to-date** with `ultralytics/yolov5` `master` branch. If your PR is behind you can update your code by clicking the 'Update branch' button or by running `git pull` and `git merge master` locally.
+ - ✅ Verify all YOLOv5 Continuous Integration (CI) **checks are passing**.
+ - ✅ Reduce changes to the absolute **minimum** required for your bug fix or feature addition. _"It is not daily increase but daily decrease, hack away the unessential. The closer to the source, the less wastage there is."_ — Bruce Lee
+
+ issue-message: |
+ 👋 Hello @${{ github.actor }}, thank you for your interest in YOLOv5 🚀! Please visit our ⭐️ [Tutorials](https://docs.ultralytics.com/yolov5/) to get started, where you can find quickstart guides for simple tasks like [Custom Data Training](https://docs.ultralytics.com/yolov5/tutorials/train_custom_data/) all the way to advanced concepts like [Hyperparameter Evolution](https://docs.ultralytics.com/yolov5/tutorials/hyperparameter_evolution/).
+
+ If this is a 🐛 Bug Report, please provide a **minimum reproducible example** to help us debug it.
+
+ If this is a custom training ❓ Question, please provide as much information as possible, including dataset image examples and training logs, and verify you are following our [Tips for Best Training Results](https://docs.ultralytics.com/yolov5/tutorials/tips_for_best_training_results/).
+
+ ## Requirements
+
+ [**Python>=3.7.0**](https://www.python.org/) with all [requirements.txt](https://github.com/ultralytics/yolov5/blob/master/requirements.txt) installed including [**PyTorch>=1.7**](https://pytorch.org/get-started/locally/). To get started:
+ ```bash
+ git clone https://github.com/ultralytics/yolov5 # clone
+ cd yolov5
+ pip install -r requirements.txt # install
+ ```
+
+ ## Environments
+
+ YOLOv5 may be run in any of the following up-to-date verified environments (with all dependencies including [CUDA](https://developer.nvidia.com/cuda)/[CUDNN](https://developer.nvidia.com/cudnn), [Python](https://www.python.org/) and [PyTorch](https://pytorch.org/) preinstalled):
+
+ - **Notebooks** with free GPU:
+ - **Google Cloud** Deep Learning VM. See [GCP Quickstart Guide](https://docs.ultralytics.com/yolov5/environments/google_cloud_quickstart_tutorial/)
+ - **Amazon** Deep Learning AMI. See [AWS Quickstart Guide](https://docs.ultralytics.com/yolov5/environments/aws_quickstart_tutorial/)
+ - **Docker Image**. See [Docker Quickstart Guide](https://docs.ultralytics.com/yolov5/environments/docker_image_quickstart_tutorial/)
+
+ ## Status
+
+
+
+ If this badge is green, all [YOLOv5 GitHub Actions](https://github.com/ultralytics/yolov5/actions) Continuous Integration (CI) tests are currently passing. CI tests verify correct operation of YOLOv5 [training](https://github.com/ultralytics/yolov5/blob/master/train.py), [validation](https://github.com/ultralytics/yolov5/blob/master/val.py), [inference](https://github.com/ultralytics/yolov5/blob/master/detect.py), [export](https://github.com/ultralytics/yolov5/blob/master/export.py) and [benchmarks](https://github.com/ultralytics/yolov5/blob/master/benchmarks.py) on macOS, Windows, and Ubuntu every 24 hours and on every commit.
+
+ ## Introducing YOLOv8 🚀
+
+ We're excited to announce the launch of our latest state-of-the-art (SOTA) object detection model for 2023 - [YOLOv8](https://github.com/ultralytics/ultralytics) 🚀!
+
+ Designed to be fast, accurate, and easy to use, YOLOv8 is an ideal choice for a wide range of object detection, image segmentation and image classification tasks. With YOLOv8, you'll be able to quickly and accurately detect objects in real-time, streamline your workflows, and achieve new levels of accuracy in your projects.
+
+ Check out our [YOLOv8 Docs](https://docs.ultralytics.com/) for details and get started with:
+ ```bash
+ pip install ultralytics
+ ```
diff --git a/yolov5/.github/workflows/links.yml b/yolov5/.github/workflows/links.yml
new file mode 100644
index 0000000000000000000000000000000000000000..306689f465078ef66c82c06e612feb203a3278ef
--- /dev/null
+++ b/yolov5/.github/workflows/links.yml
@@ -0,0 +1,44 @@
+# Ultralytics YOLO 🚀, AGPL-3.0 license
+# YOLO Continuous Integration (CI) GitHub Actions tests broken link checker
+# Accept 429(Instagram, 'too many requests'), 999(LinkedIn, 'unknown status code'), Timeout(Twitter)
+
+name: Check Broken links
+
+on:
+ push:
+ branches: [master]
+ pull_request:
+ branches: [master]
+ workflow_dispatch:
+ schedule:
+ - cron: '0 0 * * *' # runs at 00:00 UTC every day
+
+jobs:
+ Links:
+ runs-on: ubuntu-latest
+ steps:
+ - uses: actions/checkout@v3
+
+ - name: Download and install lychee
+ run: |
+ LYCHEE_URL=$(curl -s https://api.github.com/repos/lycheeverse/lychee/releases/latest | grep "browser_download_url" | grep "x86_64-unknown-linux-gnu.tar.gz" | cut -d '"' -f 4)
+ curl -L $LYCHEE_URL -o lychee.tar.gz
+ tar xzf lychee.tar.gz
+ sudo mv lychee /usr/local/bin
+
+ - name: Test Markdown and HTML links with retry
+ uses: nick-invision/retry@v2
+ with:
+ timeout_minutes: 5
+ retry_wait_seconds: 60
+ max_attempts: 3
+ command: lychee --accept 429,999 --exclude-loopback --exclude twitter.com --exclude-path '**/ci.yaml' --exclude-mail --github-token ${{ secrets.GITHUB_TOKEN }} './**/*.md' './**/*.html'
+
+ - name: Test Markdown, HTML, YAML, Python and Notebook links with retry
+ if: github.event_name == 'workflow_dispatch'
+ uses: nick-invision/retry@v2
+ with:
+ timeout_minutes: 5
+ retry_wait_seconds: 60
+ max_attempts: 3
+ command: lychee --accept 429,999 --exclude-loopback --exclude twitter.com,url.com --exclude-path '**/ci.yaml' --exclude-mail --github-token ${{ secrets.GITHUB_TOKEN }} './**/*.md' './**/*.html' './**/*.yml' './**/*.yaml' './**/*.py' './**/*.ipynb'
diff --git a/yolov5/.github/workflows/stale.yml b/yolov5/.github/workflows/stale.yml
new file mode 100644
index 0000000000000000000000000000000000000000..65c8f70798f1aeaefbe4062b0e0e231c018bdd9b
--- /dev/null
+++ b/yolov5/.github/workflows/stale.yml
@@ -0,0 +1,47 @@
+# YOLOv5 🚀 by Ultralytics, AGPL-3.0 license
+
+name: Close stale issues
+on:
+ schedule:
+ - cron: '0 0 * * *' # Runs at 00:00 UTC every day
+
+jobs:
+ stale:
+ runs-on: ubuntu-latest
+ steps:
+ - uses: actions/stale@v8
+ with:
+ repo-token: ${{ secrets.GITHUB_TOKEN }}
+
+ stale-issue-message: |
+ 👋 Hello there! We wanted to give you a friendly reminder that this issue has not had any recent activity and may be closed soon, but don't worry - you can always reopen it if needed. If you still have any questions or concerns, please feel free to let us know how we can help.
+
+ For additional resources and information, please see the links below:
+
+ - **Docs**: https://docs.ultralytics.com
+ - **HUB**: https://hub.ultralytics.com
+ - **Community**: https://community.ultralytics.com
+
+ Feel free to inform us of any other **issues** you discover or **feature requests** that come to mind in the future. Pull Requests (PRs) are also always welcomed!
+
+ Thank you for your contributions to YOLO 🚀 and Vision AI ⭐
+
+ stale-pr-message: |
+ 👋 Hello there! We wanted to let you know that we've decided to close this pull request due to inactivity. We appreciate the effort you put into contributing to our project, but unfortunately, not all contributions are suitable or aligned with our product roadmap.
+
+ We hope you understand our decision, and please don't let it discourage you from contributing to open source projects in the future. We value all of our community members and their contributions, and we encourage you to keep exploring new projects and ways to get involved.
+
+ For additional resources and information, please see the links below:
+
+ - **Docs**: https://docs.ultralytics.com
+ - **HUB**: https://hub.ultralytics.com
+ - **Community**: https://community.ultralytics.com
+
+ Thank you for your contributions to YOLO 🚀 and Vision AI ⭐
+
+ days-before-issue-stale: 30
+ days-before-issue-close: 10
+ days-before-pr-stale: 90
+ days-before-pr-close: 30
+ exempt-issue-labels: 'documentation,tutorial,TODO'
+ operations-per-run: 300 # The maximum number of operations per run, used to control rate limiting.
diff --git a/yolov5/.github/workflows/translate-readme.yml b/yolov5/.github/workflows/translate-readme.yml
new file mode 100644
index 0000000000000000000000000000000000000000..d5e2be26f52332b2ce1f7cf2ccdac286ab5ab56e
--- /dev/null
+++ b/yolov5/.github/workflows/translate-readme.yml
@@ -0,0 +1,26 @@
+# YOLOv5 🚀 by Ultralytics, AGPL-3.0 license
+# README translation action to translate README.md to Chinese as README.zh-CN.md on any change to README.md
+
+name: Translate README
+
+on:
+ push:
+ branches:
+ - translate_readme # replace with 'master' to enable action
+ paths:
+ - README.md
+
+jobs:
+ Translate:
+ runs-on: ubuntu-latest
+ steps:
+ - uses: actions/checkout@v3
+ - name: Setup Node.js
+ uses: actions/setup-node@v3
+ with:
+ node-version: 16
+ # ISO Language Codes: https://cloud.google.com/translate/docs/languages
+ - name: Adding README - Chinese Simplified
+ uses: dephraiim/translate-readme@main
+ with:
+ LANG: zh-CN
diff --git a/yolov5/.gitignore b/yolov5/.gitignore
new file mode 100644
index 0000000000000000000000000000000000000000..6bcedfac610d86354e51c3719bf48bd149f644a2
--- /dev/null
+++ b/yolov5/.gitignore
@@ -0,0 +1,257 @@
+# Repo-specific GitIgnore ----------------------------------------------------------------------------------------------
+*.jpg
+*.jpeg
+*.png
+*.bmp
+*.tif
+*.tiff
+*.heic
+*.JPG
+*.JPEG
+*.PNG
+*.BMP
+*.TIF
+*.TIFF
+*.HEIC
+*.mp4
+*.mov
+*.MOV
+*.avi
+*.data
+*.json
+*.cfg
+!setup.cfg
+!cfg/yolov3*.cfg
+
+storage.googleapis.com
+runs/*
+data/*
+data/images/*
+!data/*.yaml
+!data/hyps
+!data/scripts
+!data/images
+!data/images/zidane.jpg
+!data/images/bus.jpg
+!data/*.sh
+
+results*.csv
+
+# Datasets -------------------------------------------------------------------------------------------------------------
+coco/
+coco128/
+VOC/
+
+# MATLAB GitIgnore -----------------------------------------------------------------------------------------------------
+*.m~
+*.mat
+!targets*.mat
+
+# Neural Network weights -----------------------------------------------------------------------------------------------
+*.weights
+*.pt
+*.pb
+*.onnx
+*.engine
+*.mlmodel
+*.torchscript
+*.tflite
+*.h5
+*_saved_model/
+*_web_model/
+*_openvino_model/
+*_paddle_model/
+darknet53.conv.74
+yolov3-tiny.conv.15
+
+# GitHub Python GitIgnore ----------------------------------------------------------------------------------------------
+# Byte-compiled / optimized / DLL files
+__pycache__/
+*.py[cod]
+*$py.class
+
+# C extensions
+*.so
+
+# Distribution / packaging
+.Python
+env/
+build/
+develop-eggs/
+dist/
+downloads/
+eggs/
+.eggs/
+lib/
+lib64/
+parts/
+sdist/
+var/
+wheels/
+*.egg-info/
+/wandb/
+.installed.cfg
+*.egg
+
+
+# PyInstaller
+# Usually these files are written by a python script from a template
+# before PyInstaller builds the exe, so as to inject date/other infos into it.
+*.manifest
+*.spec
+
+# Installer logs
+pip-log.txt
+pip-delete-this-directory.txt
+
+# Unit test / coverage reports
+htmlcov/
+.tox/
+.coverage
+.coverage.*
+.cache
+nosetests.xml
+coverage.xml
+*.cover
+.hypothesis/
+
+# Translations
+*.mo
+*.pot
+
+# Django stuff:
+*.log
+local_settings.py
+
+# Flask stuff:
+instance/
+.webassets-cache
+
+# Scrapy stuff:
+.scrapy
+
+# Sphinx documentation
+docs/_build/
+
+# PyBuilder
+target/
+
+# Jupyter Notebook
+.ipynb_checkpoints
+
+# pyenv
+.python-version
+
+# celery beat schedule file
+celerybeat-schedule
+
+# SageMath parsed files
+*.sage.py
+
+# dotenv
+.env
+
+# virtualenv
+.venv*
+venv*/
+ENV*/
+
+# Spyder project settings
+.spyderproject
+.spyproject
+
+# Rope project settings
+.ropeproject
+
+# mkdocs documentation
+/site
+
+# mypy
+.mypy_cache/
+
+
+# https://github.com/github/gitignore/blob/master/Global/macOS.gitignore -----------------------------------------------
+
+# General
+.DS_Store
+.AppleDouble
+.LSOverride
+
+# Icon must end with two \r
+Icon
+Icon?
+
+# Thumbnails
+._*
+
+# Files that might appear in the root of a volume
+.DocumentRevisions-V100
+.fseventsd
+.Spotlight-V100
+.TemporaryItems
+.Trashes
+.VolumeIcon.icns
+.com.apple.timemachine.donotpresent
+
+# Directories potentially created on remote AFP share
+.AppleDB
+.AppleDesktop
+Network Trash Folder
+Temporary Items
+.apdisk
+
+
+# https://github.com/github/gitignore/blob/master/Global/JetBrains.gitignore
+# Covers JetBrains IDEs: IntelliJ, RubyMine, PhpStorm, AppCode, PyCharm, CLion, Android Studio and WebStorm
+# Reference: https://intellij-support.jetbrains.com/hc/en-us/articles/206544839
+
+# User-specific stuff:
+.idea/*
+.idea/**/workspace.xml
+.idea/**/tasks.xml
+.idea/dictionaries
+.html # Bokeh Plots
+.pg # TensorFlow Frozen Graphs
+.avi # videos
+
+# Sensitive or high-churn files:
+.idea/**/dataSources/
+.idea/**/dataSources.ids
+.idea/**/dataSources.local.xml
+.idea/**/sqlDataSources.xml
+.idea/**/dynamic.xml
+.idea/**/uiDesigner.xml
+
+# Gradle:
+.idea/**/gradle.xml
+.idea/**/libraries
+
+# CMake
+cmake-build-debug/
+cmake-build-release/
+
+# Mongo Explorer plugin:
+.idea/**/mongoSettings.xml
+
+## File-based project format:
+*.iws
+
+## Plugin-specific files:
+
+# IntelliJ
+out/
+
+# mpeltonen/sbt-idea plugin
+.idea_modules/
+
+# JIRA plugin
+atlassian-ide-plugin.xml
+
+# Cursive Clojure plugin
+.idea/replstate.xml
+
+# Crashlytics plugin (for Android Studio and IntelliJ)
+com_crashlytics_export_strings.xml
+crashlytics.properties
+crashlytics-build.properties
+fabric.properties
diff --git a/yolov5/.idea/misc.xml b/yolov5/.idea/misc.xml
new file mode 100644
index 0000000000000000000000000000000000000000..e4c68f8253267bed823c757b5e6cc0bb39a426f9
--- /dev/null
+++ b/yolov5/.idea/misc.xml
@@ -0,0 +1,4 @@
+
+
+
+
\ No newline at end of file
diff --git a/yolov5/.idea/workspace.xml b/yolov5/.idea/workspace.xml
new file mode 100644
index 0000000000000000000000000000000000000000..df7609f168e39c0dfc7d75826fbd0a4d27dceb35
--- /dev/null
+++ b/yolov5/.idea/workspace.xml
@@ -0,0 +1,99 @@
+
+
+
+
+
+
+
+
+
+
+
+
+
+
+
+
+
+
+
+
+
+
+
+
+
+
+
+
+
+
+
+
+
+
+
+
+
+
+
+
+
+
+
+
+
+
+
+
+
+
+
+
+
+
+
+
+
+
+
+
+
+
+
+
+
+
+
+
+
+
+
+
+
+
+
+
+
+
+
+
+
+
+
+
+
+
+
+
+
+
+
+ 1683810212049
+
+
+ 1683810212049
+
+
+
+
\ No newline at end of file
diff --git a/yolov5/.pre-commit-config.yaml b/yolov5/.pre-commit-config.yaml
new file mode 100644
index 0000000000000000000000000000000000000000..defb1372625e64855127cb7446daf8cf7536a4c3
--- /dev/null
+++ b/yolov5/.pre-commit-config.yaml
@@ -0,0 +1,69 @@
+# Ultralytics YOLO 🚀, AGPL-3.0 license
+# Pre-commit hooks. For more information see https://github.com/pre-commit/pre-commit-hooks/blob/main/README.md
+
+exclude: 'docs/'
+# Define bot property if installed via https://github.com/marketplace/pre-commit-ci
+ci:
+ autofix_prs: true
+ autoupdate_commit_msg: '[pre-commit.ci] pre-commit suggestions'
+ autoupdate_schedule: monthly
+ # submodules: true
+
+repos:
+ - repo: https://github.com/pre-commit/pre-commit-hooks
+ rev: v4.4.0
+ hooks:
+ - id: end-of-file-fixer
+ - id: trailing-whitespace
+ - id: check-case-conflict
+ - id: check-yaml
+ - id: check-docstring-first
+ - id: double-quote-string-fixer
+ - id: detect-private-key
+
+ - repo: https://github.com/asottile/pyupgrade
+ rev: v3.3.2
+ hooks:
+ - id: pyupgrade
+ name: Upgrade code
+ args: [--py37-plus]
+
+ - repo: https://github.com/PyCQA/isort
+ rev: 5.12.0
+ hooks:
+ - id: isort
+ name: Sort imports
+
+ - repo: https://github.com/google/yapf
+ rev: v0.33.0
+ hooks:
+ - id: yapf
+ name: YAPF formatting
+
+ - repo: https://github.com/executablebooks/mdformat
+ rev: 0.7.16
+ hooks:
+ - id: mdformat
+ name: MD formatting
+ additional_dependencies:
+ - mdformat-gfm
+ - mdformat-black
+ # exclude: "README.md|README.zh-CN.md|CONTRIBUTING.md"
+
+ - repo: https://github.com/PyCQA/flake8
+ rev: 6.0.0
+ hooks:
+ - id: flake8
+ name: PEP8
+
+ - repo: https://github.com/codespell-project/codespell
+ rev: v2.2.4
+ hooks:
+ - id: codespell
+ args:
+ - --ignore-words-list=crate,nd,strack,dota
+
+ #- repo: https://github.com/asottile/yesqa
+ # rev: v1.4.0
+ # hooks:
+ # - id: yesqa
diff --git a/yolov5/CITATION.cff b/yolov5/CITATION.cff
new file mode 100644
index 0000000000000000000000000000000000000000..c277230d922f869978cedaabc214d5236c6cb191
--- /dev/null
+++ b/yolov5/CITATION.cff
@@ -0,0 +1,14 @@
+cff-version: 1.2.0
+preferred-citation:
+ type: software
+ message: If you use YOLOv5, please cite it as below.
+ authors:
+ - family-names: Jocher
+ given-names: Glenn
+ orcid: "https://orcid.org/0000-0001-5950-6979"
+ title: "YOLOv5 by Ultralytics"
+ version: 7.0
+ doi: 10.5281/zenodo.3908559
+ date-released: 2020-5-29
+ license: AGPL-3.0
+ url: "https://github.com/ultralytics/yolov5"
diff --git a/yolov5/CONTRIBUTING.md b/yolov5/CONTRIBUTING.md
new file mode 100644
index 0000000000000000000000000000000000000000..95d88b9830d68f3bdcd621144a774c32f19a700e
--- /dev/null
+++ b/yolov5/CONTRIBUTING.md
@@ -0,0 +1,93 @@
+## Contributing to YOLOv5 🚀
+
+We love your input! We want to make contributing to YOLOv5 as easy and transparent as possible, whether it's:
+
+- Reporting a bug
+- Discussing the current state of the code
+- Submitting a fix
+- Proposing a new feature
+- Becoming a maintainer
+
+YOLOv5 works so well due to our combined community effort, and for every small improvement you contribute you will be
+helping push the frontiers of what's possible in AI 😃!
+
+## Submitting a Pull Request (PR) 🛠️
+
+Submitting a PR is easy! This example shows how to submit a PR for updating `requirements.txt` in 4 steps:
+
+### 1. Select File to Update
+
+Select `requirements.txt` to update by clicking on it in GitHub.
+
+
+
+### 2. Click 'Edit this file'
+
+The button is in the top-right corner.
+
+
+
+### 3. Make Changes
+
+Change the `matplotlib` version from `3.2.2` to `3.3`.
+
+
+
+### 4. Preview Changes and Submit PR
+
+Click on the **Preview changes** tab to verify your updates. At the bottom of the screen select 'Create a **new branch**
+for this commit', assign your branch a descriptive name such as `fix/matplotlib_version` and click the green **Propose
+changes** button. All done, your PR is now submitted to YOLOv5 for review and approval 😃!
+
+
+
+### PR recommendations
+
+To allow your work to be integrated as seamlessly as possible, we advise you to:
+
+- ✅ Verify your PR is **up-to-date** with `ultralytics/yolov5` `master` branch. If your PR is behind you can update
+ your code by clicking the 'Update branch' button or by running `git pull` and `git merge master` locally.
+
+
+
+- ✅ Verify all YOLOv5 Continuous Integration (CI) **checks are passing**.
+
+
+
+- ✅ Reduce changes to the absolute **minimum** required for your bug fix or feature addition. _"It is not daily increase
+ but daily decrease, hack away the unessential. The closer to the source, the less wastage there is."_ — Bruce Lee
+
+## Submitting a Bug Report 🐛
+
+If you spot a problem with YOLOv5 please submit a Bug Report!
+
+For us to start investigating a possible problem we need to be able to reproduce it ourselves first. We've created a few
+short guidelines below to help users provide what we need to get started.
+
+When asking a question, people will be better able to provide help if you provide **code** that they can easily
+understand and use to **reproduce** the problem. This is referred to by community members as creating
+a [minimum reproducible example](https://docs.ultralytics.com/help/minimum_reproducible_example/). Your code that reproduces
+the problem should be:
+
+- ✅ **Minimal** – Use as little code as possible that still produces the same problem
+- ✅ **Complete** – Provide **all** parts someone else needs to reproduce your problem in the question itself
+- ✅ **Reproducible** – Test the code you're about to provide to make sure it reproduces the problem
+
+In addition to the above requirements, for [Ultralytics](https://ultralytics.com/) to provide assistance your code
+should be:
+
+- ✅ **Current** – Verify that your code is up-to-date with the current
+ GitHub [master](https://github.com/ultralytics/yolov5/tree/master), and if necessary `git pull` or `git clone` a new
+ copy to ensure your problem has not already been resolved by previous commits.
+- ✅ **Unmodified** – Your problem must be reproducible without any modifications to the codebase in this
+ repository. [Ultralytics](https://ultralytics.com/) does not provide support for custom code ⚠️.
+
+If you believe your problem meets all of the above criteria, please close this issue and raise a new one using the 🐛
+**Bug Report** [template](https://github.com/ultralytics/yolov5/issues/new/choose) and provide
+a [minimum reproducible example](https://docs.ultralytics.com/help/minimum_reproducible_example/) to help us better
+understand and diagnose your problem.
+
+## License
+
+By contributing, you agree that your contributions will be licensed under
+the [AGPL-3.0 license](https://choosealicense.com/licenses/agpl-3.0/)
diff --git a/yolov5/LICENSE b/yolov5/LICENSE
new file mode 100644
index 0000000000000000000000000000000000000000..be3f7b28e564e7dd05eaf59d64adba1a4065ac0e
--- /dev/null
+++ b/yolov5/LICENSE
@@ -0,0 +1,661 @@
+ GNU AFFERO GENERAL PUBLIC LICENSE
+ Version 3, 19 November 2007
+
+ Copyright (C) 2007 Free Software Foundation, Inc.
+ Everyone is permitted to copy and distribute verbatim copies
+ of this license document, but changing it is not allowed.
+
+ Preamble
+
+ The GNU Affero General Public License is a free, copyleft license for
+software and other kinds of works, specifically designed to ensure
+cooperation with the community in the case of network server software.
+
+ The licenses for most software and other practical works are designed
+to take away your freedom to share and change the works. By contrast,
+our General Public Licenses are intended to guarantee your freedom to
+share and change all versions of a program--to make sure it remains free
+software for all its users.
+
+ When we speak of free software, we are referring to freedom, not
+price. Our General Public Licenses are designed to make sure that you
+have the freedom to distribute copies of free software (and charge for
+them if you wish), that you receive source code or can get it if you
+want it, that you can change the software or use pieces of it in new
+free programs, and that you know you can do these things.
+
+ Developers that use our General Public Licenses protect your rights
+with two steps: (1) assert copyright on the software, and (2) offer
+you this License which gives you legal permission to copy, distribute
+and/or modify the software.
+
+ A secondary benefit of defending all users' freedom is that
+improvements made in alternate versions of the program, if they
+receive widespread use, become available for other developers to
+incorporate. Many developers of free software are heartened and
+encouraged by the resulting cooperation. However, in the case of
+software used on network servers, this result may fail to come about.
+The GNU General Public License permits making a modified version and
+letting the public access it on a server without ever releasing its
+source code to the public.
+
+ The GNU Affero General Public License is designed specifically to
+ensure that, in such cases, the modified source code becomes available
+to the community. It requires the operator of a network server to
+provide the source code of the modified version running there to the
+users of that server. Therefore, public use of a modified version, on
+a publicly accessible server, gives the public access to the source
+code of the modified version.
+
+ An older license, called the Affero General Public License and
+published by Affero, was designed to accomplish similar goals. This is
+a different license, not a version of the Affero GPL, but Affero has
+released a new version of the Affero GPL which permits relicensing under
+this license.
+
+ The precise terms and conditions for copying, distribution and
+modification follow.
+
+ TERMS AND CONDITIONS
+
+ 0. Definitions.
+
+ "This License" refers to version 3 of the GNU Affero General Public License.
+
+ "Copyright" also means copyright-like laws that apply to other kinds of
+works, such as semiconductor masks.
+
+ "The Program" refers to any copyrightable work licensed under this
+License. Each licensee is addressed as "you". "Licensees" and
+"recipients" may be individuals or organizations.
+
+ To "modify" a work means to copy from or adapt all or part of the work
+in a fashion requiring copyright permission, other than the making of an
+exact copy. The resulting work is called a "modified version" of the
+earlier work or a work "based on" the earlier work.
+
+ A "covered work" means either the unmodified Program or a work based
+on the Program.
+
+ To "propagate" a work means to do anything with it that, without
+permission, would make you directly or secondarily liable for
+infringement under applicable copyright law, except executing it on a
+computer or modifying a private copy. Propagation includes copying,
+distribution (with or without modification), making available to the
+public, and in some countries other activities as well.
+
+ To "convey" a work means any kind of propagation that enables other
+parties to make or receive copies. Mere interaction with a user through
+a computer network, with no transfer of a copy, is not conveying.
+
+ An interactive user interface displays "Appropriate Legal Notices"
+to the extent that it includes a convenient and prominently visible
+feature that (1) displays an appropriate copyright notice, and (2)
+tells the user that there is no warranty for the work (except to the
+extent that warranties are provided), that licensees may convey the
+work under this License, and how to view a copy of this License. If
+the interface presents a list of user commands or options, such as a
+menu, a prominent item in the list meets this criterion.
+
+ 1. Source Code.
+
+ The "source code" for a work means the preferred form of the work
+for making modifications to it. "Object code" means any non-source
+form of a work.
+
+ A "Standard Interface" means an interface that either is an official
+standard defined by a recognized standards body, or, in the case of
+interfaces specified for a particular programming language, one that
+is widely used among developers working in that language.
+
+ The "System Libraries" of an executable work include anything, other
+than the work as a whole, that (a) is included in the normal form of
+packaging a Major Component, but which is not part of that Major
+Component, and (b) serves only to enable use of the work with that
+Major Component, or to implement a Standard Interface for which an
+implementation is available to the public in source code form. A
+"Major Component", in this context, means a major essential component
+(kernel, window system, and so on) of the specific operating system
+(if any) on which the executable work runs, or a compiler used to
+produce the work, or an object code interpreter used to run it.
+
+ The "Corresponding Source" for a work in object code form means all
+the source code needed to generate, install, and (for an executable
+work) run the object code and to modify the work, including scripts to
+control those activities. However, it does not include the work's
+System Libraries, or general-purpose tools or generally available free
+programs which are used unmodified in performing those activities but
+which are not part of the work. For example, Corresponding Source
+includes interface definition files associated with source files for
+the work, and the source code for shared libraries and dynamically
+linked subprograms that the work is specifically designed to require,
+such as by intimate data communication or control flow between those
+subprograms and other parts of the work.
+
+ The Corresponding Source need not include anything that users
+can regenerate automatically from other parts of the Corresponding
+Source.
+
+ The Corresponding Source for a work in source code form is that
+same work.
+
+ 2. Basic Permissions.
+
+ All rights granted under this License are granted for the term of
+copyright on the Program, and are irrevocable provided the stated
+conditions are met. This License explicitly affirms your unlimited
+permission to run the unmodified Program. The output from running a
+covered work is covered by this License only if the output, given its
+content, constitutes a covered work. This License acknowledges your
+rights of fair use or other equivalent, as provided by copyright law.
+
+ You may make, run and propagate covered works that you do not
+convey, without conditions so long as your license otherwise remains
+in force. You may convey covered works to others for the sole purpose
+of having them make modifications exclusively for you, or provide you
+with facilities for running those works, provided that you comply with
+the terms of this License in conveying all material for which you do
+not control copyright. Those thus making or running the covered works
+for you must do so exclusively on your behalf, under your direction
+and control, on terms that prohibit them from making any copies of
+your copyrighted material outside their relationship with you.
+
+ Conveying under any other circumstances is permitted solely under
+the conditions stated below. Sublicensing is not allowed; section 10
+makes it unnecessary.
+
+ 3. Protecting Users' Legal Rights From Anti-Circumvention Law.
+
+ No covered work shall be deemed part of an effective technological
+measure under any applicable law fulfilling obligations under article
+11 of the WIPO copyright treaty adopted on 20 December 1996, or
+similar laws prohibiting or restricting circumvention of such
+measures.
+
+ When you convey a covered work, you waive any legal power to forbid
+circumvention of technological measures to the extent such circumvention
+is effected by exercising rights under this License with respect to
+the covered work, and you disclaim any intention to limit operation or
+modification of the work as a means of enforcing, against the work's
+users, your or third parties' legal rights to forbid circumvention of
+technological measures.
+
+ 4. Conveying Verbatim Copies.
+
+ You may convey verbatim copies of the Program's source code as you
+receive it, in any medium, provided that you conspicuously and
+appropriately publish on each copy an appropriate copyright notice;
+keep intact all notices stating that this License and any
+non-permissive terms added in accord with section 7 apply to the code;
+keep intact all notices of the absence of any warranty; and give all
+recipients a copy of this License along with the Program.
+
+ You may charge any price or no price for each copy that you convey,
+and you may offer support or warranty protection for a fee.
+
+ 5. Conveying Modified Source Versions.
+
+ You may convey a work based on the Program, or the modifications to
+produce it from the Program, in the form of source code under the
+terms of section 4, provided that you also meet all of these conditions:
+
+ a) The work must carry prominent notices stating that you modified
+ it, and giving a relevant date.
+
+ b) The work must carry prominent notices stating that it is
+ released under this License and any conditions added under section
+ 7. This requirement modifies the requirement in section 4 to
+ "keep intact all notices".
+
+ c) You must license the entire work, as a whole, under this
+ License to anyone who comes into possession of a copy. This
+ License will therefore apply, along with any applicable section 7
+ additional terms, to the whole of the work, and all its parts,
+ regardless of how they are packaged. This License gives no
+ permission to license the work in any other way, but it does not
+ invalidate such permission if you have separately received it.
+
+ d) If the work has interactive user interfaces, each must display
+ Appropriate Legal Notices; however, if the Program has interactive
+ interfaces that do not display Appropriate Legal Notices, your
+ work need not make them do so.
+
+ A compilation of a covered work with other separate and independent
+works, which are not by their nature extensions of the covered work,
+and which are not combined with it such as to form a larger program,
+in or on a volume of a storage or distribution medium, is called an
+"aggregate" if the compilation and its resulting copyright are not
+used to limit the access or legal rights of the compilation's users
+beyond what the individual works permit. Inclusion of a covered work
+in an aggregate does not cause this License to apply to the other
+parts of the aggregate.
+
+ 6. Conveying Non-Source Forms.
+
+ You may convey a covered work in object code form under the terms
+of sections 4 and 5, provided that you also convey the
+machine-readable Corresponding Source under the terms of this License,
+in one of these ways:
+
+ a) Convey the object code in, or embodied in, a physical product
+ (including a physical distribution medium), accompanied by the
+ Corresponding Source fixed on a durable physical medium
+ customarily used for software interchange.
+
+ b) Convey the object code in, or embodied in, a physical product
+ (including a physical distribution medium), accompanied by a
+ written offer, valid for at least three years and valid for as
+ long as you offer spare parts or customer support for that product
+ model, to give anyone who possesses the object code either (1) a
+ copy of the Corresponding Source for all the software in the
+ product that is covered by this License, on a durable physical
+ medium customarily used for software interchange, for a price no
+ more than your reasonable cost of physically performing this
+ conveying of source, or (2) access to copy the
+ Corresponding Source from a network server at no charge.
+
+ c) Convey individual copies of the object code with a copy of the
+ written offer to provide the Corresponding Source. This
+ alternative is allowed only occasionally and noncommercially, and
+ only if you received the object code with such an offer, in accord
+ with subsection 6b.
+
+ d) Convey the object code by offering access from a designated
+ place (gratis or for a charge), and offer equivalent access to the
+ Corresponding Source in the same way through the same place at no
+ further charge. You need not require recipients to copy the
+ Corresponding Source along with the object code. If the place to
+ copy the object code is a network server, the Corresponding Source
+ may be on a different server (operated by you or a third party)
+ that supports equivalent copying facilities, provided you maintain
+ clear directions next to the object code saying where to find the
+ Corresponding Source. Regardless of what server hosts the
+ Corresponding Source, you remain obligated to ensure that it is
+ available for as long as needed to satisfy these requirements.
+
+ e) Convey the object code using peer-to-peer transmission, provided
+ you inform other peers where the object code and Corresponding
+ Source of the work are being offered to the general public at no
+ charge under subsection 6d.
+
+ A separable portion of the object code, whose source code is excluded
+from the Corresponding Source as a System Library, need not be
+included in conveying the object code work.
+
+ A "User Product" is either (1) a "consumer product", which means any
+tangible personal property which is normally used for personal, family,
+or household purposes, or (2) anything designed or sold for incorporation
+into a dwelling. In determining whether a product is a consumer product,
+doubtful cases shall be resolved in favor of coverage. For a particular
+product received by a particular user, "normally used" refers to a
+typical or common use of that class of product, regardless of the status
+of the particular user or of the way in which the particular user
+actually uses, or expects or is expected to use, the product. A product
+is a consumer product regardless of whether the product has substantial
+commercial, industrial or non-consumer uses, unless such uses represent
+the only significant mode of use of the product.
+
+ "Installation Information" for a User Product means any methods,
+procedures, authorization keys, or other information required to install
+and execute modified versions of a covered work in that User Product from
+a modified version of its Corresponding Source. The information must
+suffice to ensure that the continued functioning of the modified object
+code is in no case prevented or interfered with solely because
+modification has been made.
+
+ If you convey an object code work under this section in, or with, or
+specifically for use in, a User Product, and the conveying occurs as
+part of a transaction in which the right of possession and use of the
+User Product is transferred to the recipient in perpetuity or for a
+fixed term (regardless of how the transaction is characterized), the
+Corresponding Source conveyed under this section must be accompanied
+by the Installation Information. But this requirement does not apply
+if neither you nor any third party retains the ability to install
+modified object code on the User Product (for example, the work has
+been installed in ROM).
+
+ The requirement to provide Installation Information does not include a
+requirement to continue to provide support service, warranty, or updates
+for a work that has been modified or installed by the recipient, or for
+the User Product in which it has been modified or installed. Access to a
+network may be denied when the modification itself materially and
+adversely affects the operation of the network or violates the rules and
+protocols for communication across the network.
+
+ Corresponding Source conveyed, and Installation Information provided,
+in accord with this section must be in a format that is publicly
+documented (and with an implementation available to the public in
+source code form), and must require no special password or key for
+unpacking, reading or copying.
+
+ 7. Additional Terms.
+
+ "Additional permissions" are terms that supplement the terms of this
+License by making exceptions from one or more of its conditions.
+Additional permissions that are applicable to the entire Program shall
+be treated as though they were included in this License, to the extent
+that they are valid under applicable law. If additional permissions
+apply only to part of the Program, that part may be used separately
+under those permissions, but the entire Program remains governed by
+this License without regard to the additional permissions.
+
+ When you convey a copy of a covered work, you may at your option
+remove any additional permissions from that copy, or from any part of
+it. (Additional permissions may be written to require their own
+removal in certain cases when you modify the work.) You may place
+additional permissions on material, added by you to a covered work,
+for which you have or can give appropriate copyright permission.
+
+ Notwithstanding any other provision of this License, for material you
+add to a covered work, you may (if authorized by the copyright holders of
+that material) supplement the terms of this License with terms:
+
+ a) Disclaiming warranty or limiting liability differently from the
+ terms of sections 15 and 16 of this License; or
+
+ b) Requiring preservation of specified reasonable legal notices or
+ author attributions in that material or in the Appropriate Legal
+ Notices displayed by works containing it; or
+
+ c) Prohibiting misrepresentation of the origin of that material, or
+ requiring that modified versions of such material be marked in
+ reasonable ways as different from the original version; or
+
+ d) Limiting the use for publicity purposes of names of licensors or
+ authors of the material; or
+
+ e) Declining to grant rights under trademark law for use of some
+ trade names, trademarks, or service marks; or
+
+ f) Requiring indemnification of licensors and authors of that
+ material by anyone who conveys the material (or modified versions of
+ it) with contractual assumptions of liability to the recipient, for
+ any liability that these contractual assumptions directly impose on
+ those licensors and authors.
+
+ All other non-permissive additional terms are considered "further
+restrictions" within the meaning of section 10. If the Program as you
+received it, or any part of it, contains a notice stating that it is
+governed by this License along with a term that is a further
+restriction, you may remove that term. If a license document contains
+a further restriction but permits relicensing or conveying under this
+License, you may add to a covered work material governed by the terms
+of that license document, provided that the further restriction does
+not survive such relicensing or conveying.
+
+ If you add terms to a covered work in accord with this section, you
+must place, in the relevant source files, a statement of the
+additional terms that apply to those files, or a notice indicating
+where to find the applicable terms.
+
+ Additional terms, permissive or non-permissive, may be stated in the
+form of a separately written license, or stated as exceptions;
+the above requirements apply either way.
+
+ 8. Termination.
+
+ You may not propagate or modify a covered work except as expressly
+provided under this License. Any attempt otherwise to propagate or
+modify it is void, and will automatically terminate your rights under
+this License (including any patent licenses granted under the third
+paragraph of section 11).
+
+ However, if you cease all violation of this License, then your
+license from a particular copyright holder is reinstated (a)
+provisionally, unless and until the copyright holder explicitly and
+finally terminates your license, and (b) permanently, if the copyright
+holder fails to notify you of the violation by some reasonable means
+prior to 60 days after the cessation.
+
+ Moreover, your license from a particular copyright holder is
+reinstated permanently if the copyright holder notifies you of the
+violation by some reasonable means, this is the first time you have
+received notice of violation of this License (for any work) from that
+copyright holder, and you cure the violation prior to 30 days after
+your receipt of the notice.
+
+ Termination of your rights under this section does not terminate the
+licenses of parties who have received copies or rights from you under
+this License. If your rights have been terminated and not permanently
+reinstated, you do not qualify to receive new licenses for the same
+material under section 10.
+
+ 9. Acceptance Not Required for Having Copies.
+
+ You are not required to accept this License in order to receive or
+run a copy of the Program. Ancillary propagation of a covered work
+occurring solely as a consequence of using peer-to-peer transmission
+to receive a copy likewise does not require acceptance. However,
+nothing other than this License grants you permission to propagate or
+modify any covered work. These actions infringe copyright if you do
+not accept this License. Therefore, by modifying or propagating a
+covered work, you indicate your acceptance of this License to do so.
+
+ 10. Automatic Licensing of Downstream Recipients.
+
+ Each time you convey a covered work, the recipient automatically
+receives a license from the original licensors, to run, modify and
+propagate that work, subject to this License. You are not responsible
+for enforcing compliance by third parties with this License.
+
+ An "entity transaction" is a transaction transferring control of an
+organization, or substantially all assets of one, or subdividing an
+organization, or merging organizations. If propagation of a covered
+work results from an entity transaction, each party to that
+transaction who receives a copy of the work also receives whatever
+licenses to the work the party's predecessor in interest had or could
+give under the previous paragraph, plus a right to possession of the
+Corresponding Source of the work from the predecessor in interest, if
+the predecessor has it or can get it with reasonable efforts.
+
+ You may not impose any further restrictions on the exercise of the
+rights granted or affirmed under this License. For example, you may
+not impose a license fee, royalty, or other charge for exercise of
+rights granted under this License, and you may not initiate litigation
+(including a cross-claim or counterclaim in a lawsuit) alleging that
+any patent claim is infringed by making, using, selling, offering for
+sale, or importing the Program or any portion of it.
+
+ 11. Patents.
+
+ A "contributor" is a copyright holder who authorizes use under this
+License of the Program or a work on which the Program is based. The
+work thus licensed is called the contributor's "contributor version".
+
+ A contributor's "essential patent claims" are all patent claims
+owned or controlled by the contributor, whether already acquired or
+hereafter acquired, that would be infringed by some manner, permitted
+by this License, of making, using, or selling its contributor version,
+but do not include claims that would be infringed only as a
+consequence of further modification of the contributor version. For
+purposes of this definition, "control" includes the right to grant
+patent sublicenses in a manner consistent with the requirements of
+this License.
+
+ Each contributor grants you a non-exclusive, worldwide, royalty-free
+patent license under the contributor's essential patent claims, to
+make, use, sell, offer for sale, import and otherwise run, modify and
+propagate the contents of its contributor version.
+
+ In the following three paragraphs, a "patent license" is any express
+agreement or commitment, however denominated, not to enforce a patent
+(such as an express permission to practice a patent or covenant not to
+sue for patent infringement). To "grant" such a patent license to a
+party means to make such an agreement or commitment not to enforce a
+patent against the party.
+
+ If you convey a covered work, knowingly relying on a patent license,
+and the Corresponding Source of the work is not available for anyone
+to copy, free of charge and under the terms of this License, through a
+publicly available network server or other readily accessible means,
+then you must either (1) cause the Corresponding Source to be so
+available, or (2) arrange to deprive yourself of the benefit of the
+patent license for this particular work, or (3) arrange, in a manner
+consistent with the requirements of this License, to extend the patent
+license to downstream recipients. "Knowingly relying" means you have
+actual knowledge that, but for the patent license, your conveying the
+covered work in a country, or your recipient's use of the covered work
+in a country, would infringe one or more identifiable patents in that
+country that you have reason to believe are valid.
+
+ If, pursuant to or in connection with a single transaction or
+arrangement, you convey, or propagate by procuring conveyance of, a
+covered work, and grant a patent license to some of the parties
+receiving the covered work authorizing them to use, propagate, modify
+or convey a specific copy of the covered work, then the patent license
+you grant is automatically extended to all recipients of the covered
+work and works based on it.
+
+ A patent license is "discriminatory" if it does not include within
+the scope of its coverage, prohibits the exercise of, or is
+conditioned on the non-exercise of one or more of the rights that are
+specifically granted under this License. You may not convey a covered
+work if you are a party to an arrangement with a third party that is
+in the business of distributing software, under which you make payment
+to the third party based on the extent of your activity of conveying
+the work, and under which the third party grants, to any of the
+parties who would receive the covered work from you, a discriminatory
+patent license (a) in connection with copies of the covered work
+conveyed by you (or copies made from those copies), or (b) primarily
+for and in connection with specific products or compilations that
+contain the covered work, unless you entered into that arrangement,
+or that patent license was granted, prior to 28 March 2007.
+
+ Nothing in this License shall be construed as excluding or limiting
+any implied license or other defenses to infringement that may
+otherwise be available to you under applicable patent law.
+
+ 12. No Surrender of Others' Freedom.
+
+ If conditions are imposed on you (whether by court order, agreement or
+otherwise) that contradict the conditions of this License, they do not
+excuse you from the conditions of this License. If you cannot convey a
+covered work so as to satisfy simultaneously your obligations under this
+License and any other pertinent obligations, then as a consequence you may
+not convey it at all. For example, if you agree to terms that obligate you
+to collect a royalty for further conveying from those to whom you convey
+the Program, the only way you could satisfy both those terms and this
+License would be to refrain entirely from conveying the Program.
+
+ 13. Remote Network Interaction; Use with the GNU General Public License.
+
+ Notwithstanding any other provision of this License, if you modify the
+Program, your modified version must prominently offer all users
+interacting with it remotely through a computer network (if your version
+supports such interaction) an opportunity to receive the Corresponding
+Source of your version by providing access to the Corresponding Source
+from a network server at no charge, through some standard or customary
+means of facilitating copying of software. This Corresponding Source
+shall include the Corresponding Source for any work covered by version 3
+of the GNU General Public License that is incorporated pursuant to the
+following paragraph.
+
+ Notwithstanding any other provision of this License, you have
+permission to link or combine any covered work with a work licensed
+under version 3 of the GNU General Public License into a single
+combined work, and to convey the resulting work. The terms of this
+License will continue to apply to the part which is the covered work,
+but the work with which it is combined will remain governed by version
+3 of the GNU General Public License.
+
+ 14. Revised Versions of this License.
+
+ The Free Software Foundation may publish revised and/or new versions of
+the GNU Affero General Public License from time to time. Such new versions
+will be similar in spirit to the present version, but may differ in detail to
+address new problems or concerns.
+
+ Each version is given a distinguishing version number. If the
+Program specifies that a certain numbered version of the GNU Affero General
+Public License "or any later version" applies to it, you have the
+option of following the terms and conditions either of that numbered
+version or of any later version published by the Free Software
+Foundation. If the Program does not specify a version number of the
+GNU Affero General Public License, you may choose any version ever published
+by the Free Software Foundation.
+
+ If the Program specifies that a proxy can decide which future
+versions of the GNU Affero General Public License can be used, that proxy's
+public statement of acceptance of a version permanently authorizes you
+to choose that version for the Program.
+
+ Later license versions may give you additional or different
+permissions. However, no additional obligations are imposed on any
+author or copyright holder as a result of your choosing to follow a
+later version.
+
+ 15. Disclaimer of Warranty.
+
+ THERE IS NO WARRANTY FOR THE PROGRAM, TO THE EXTENT PERMITTED BY
+APPLICABLE LAW. EXCEPT WHEN OTHERWISE STATED IN WRITING THE COPYRIGHT
+HOLDERS AND/OR OTHER PARTIES PROVIDE THE PROGRAM "AS IS" WITHOUT WARRANTY
+OF ANY KIND, EITHER EXPRESSED OR IMPLIED, INCLUDING, BUT NOT LIMITED TO,
+THE IMPLIED WARRANTIES OF MERCHANTABILITY AND FITNESS FOR A PARTICULAR
+PURPOSE. THE ENTIRE RISK AS TO THE QUALITY AND PERFORMANCE OF THE PROGRAM
+IS WITH YOU. SHOULD THE PROGRAM PROVE DEFECTIVE, YOU ASSUME THE COST OF
+ALL NECESSARY SERVICING, REPAIR OR CORRECTION.
+
+ 16. Limitation of Liability.
+
+ IN NO EVENT UNLESS REQUIRED BY APPLICABLE LAW OR AGREED TO IN WRITING
+WILL ANY COPYRIGHT HOLDER, OR ANY OTHER PARTY WHO MODIFIES AND/OR CONVEYS
+THE PROGRAM AS PERMITTED ABOVE, BE LIABLE TO YOU FOR DAMAGES, INCLUDING ANY
+GENERAL, SPECIAL, INCIDENTAL OR CONSEQUENTIAL DAMAGES ARISING OUT OF THE
+USE OR INABILITY TO USE THE PROGRAM (INCLUDING BUT NOT LIMITED TO LOSS OF
+DATA OR DATA BEING RENDERED INACCURATE OR LOSSES SUSTAINED BY YOU OR THIRD
+PARTIES OR A FAILURE OF THE PROGRAM TO OPERATE WITH ANY OTHER PROGRAMS),
+EVEN IF SUCH HOLDER OR OTHER PARTY HAS BEEN ADVISED OF THE POSSIBILITY OF
+SUCH DAMAGES.
+
+ 17. Interpretation of Sections 15 and 16.
+
+ If the disclaimer of warranty and limitation of liability provided
+above cannot be given local legal effect according to their terms,
+reviewing courts shall apply local law that most closely approximates
+an absolute waiver of all civil liability in connection with the
+Program, unless a warranty or assumption of liability accompanies a
+copy of the Program in return for a fee.
+
+ END OF TERMS AND CONDITIONS
+
+ How to Apply These Terms to Your New Programs
+
+ If you develop a new program, and you want it to be of the greatest
+possible use to the public, the best way to achieve this is to make it
+free software which everyone can redistribute and change under these terms.
+
+ To do so, attach the following notices to the program. It is safest
+to attach them to the start of each source file to most effectively
+state the exclusion of warranty; and each file should have at least
+the "copyright" line and a pointer to where the full notice is found.
+
+
+ Copyright (C)
+
+ This program is free software: you can redistribute it and/or modify
+ it under the terms of the GNU Affero General Public License as published by
+ the Free Software Foundation, either version 3 of the License, or
+ (at your option) any later version.
+
+ This program is distributed in the hope that it will be useful,
+ but WITHOUT ANY WARRANTY; without even the implied warranty of
+ MERCHANTABILITY or FITNESS FOR A PARTICULAR PURPOSE. See the
+ GNU Affero General Public License for more details.
+
+ You should have received a copy of the GNU Affero General Public License
+ along with this program. If not, see .
+
+Also add information on how to contact you by electronic and paper mail.
+
+ If your software can interact with users remotely through a computer
+network, you should also make sure that it provides a way for users to
+get its source. For example, if your program is a web application, its
+interface could display a "Source" link that leads users to an archive
+of the code. There are many ways you could offer source, and different
+solutions will be better for different programs; see section 13 for the
+specific requirements.
+
+ You should also get your employer (if you work as a programmer) or school,
+if any, to sign a "copyright disclaimer" for the program, if necessary.
+For more information on this, and how to apply and follow the GNU AGPL, see
+.
diff --git a/yolov5/README.md b/yolov5/README.md
new file mode 100644
index 0000000000000000000000000000000000000000..37f683343f53ef962472342eb856de52909a9972
--- /dev/null
+++ b/yolov5/README.md
@@ -0,0 +1,495 @@
+
+
+
+YOLOv5 🚀 is the world's most loved vision AI, representing Ultralytics open-source research into future vision AI methods, incorporating lessons learned and best practices evolved over thousands of hours of research and development.
+
+We hope that the resources here will help you get the most out of YOLOv5. Please browse the YOLOv5 Docs for details, raise an issue on GitHub for support, and join our Discord community for questions and discussions!
+
+To request an Enterprise License please complete the form at [Ultralytics Licensing](https://ultralytics.com/license).
+
+
+
+We are thrilled to announce the launch of Ultralytics YOLOv8 🚀, our NEW cutting-edge, state-of-the-art (SOTA) model
+released at **[https://github.com/ultralytics/ultralytics](https://github.com/ultralytics/ultralytics)**.
+YOLOv8 is designed to be fast, accurate, and easy to use, making it an excellent choice for a wide range of
+object detection, image segmentation and image classification tasks.
+
+See the [YOLOv8 Docs](https://docs.ultralytics.com) for details and get started with:
+
+```commandline
+pip install ultralytics
+```
+
+
+
+| Roboflow | ClearML ⭐ NEW | Comet ⭐ NEW | Neural Magic ⭐ NEW |
+| :--------------------------------------------------------------------------------------------------------------------------: | :---------------------------------------------------------------------------------------------------------------------------------: | :--------------------------------------------------------------------------------------------------------------------------------------------------------: | :----------------------------------------------------------------------------------------------------: |
+| Label and export your custom datasets directly to YOLOv5 for training with [Roboflow](https://roboflow.com/?ref=ultralytics) | Automatically track, visualize and even remotely train YOLOv5 using [ClearML](https://cutt.ly/yolov5-readme-clearml) (open-source!) | Free forever, [Comet](https://bit.ly/yolov5-readme-comet2) lets you save YOLOv5 models, resume training, and interactively visualise and debug predictions | Run YOLOv5 inference up to 6x faster with [Neural Magic DeepSparse](https://bit.ly/yolov5-neuralmagic) |
+
+##
Ultralytics HUB
+
+Experience seamless AI with [Ultralytics HUB](https://bit.ly/ultralytics_hub) ⭐, the all-in-one solution for data visualization, YOLOv5 and YOLOv8 🚀 model training and deployment, without any coding. Transform images into actionable insights and bring your AI visions to life with ease using our cutting-edge platform and user-friendly [Ultralytics App](https://ultralytics.com/app_install). Start your journey for **Free** now!
+
+
+
+
+##
Why YOLOv5
+
+YOLOv5 has been designed to be super easy to get started and simple to learn. We prioritize real-world results.
+
+
+
+Our new YOLOv5 [release v7.0](https://github.com/ultralytics/yolov5/releases/v7.0) instance segmentation models are the fastest and most accurate in the world, beating all current [SOTA benchmarks](https://paperswithcode.com/sota/real-time-instance-segmentation-on-mscoco). We've made them super simple to train, validate and deploy. See full details in our [Release Notes](https://github.com/ultralytics/yolov5/releases/v7.0) and visit our [YOLOv5 Segmentation Colab Notebook](https://github.com/ultralytics/yolov5/blob/master/segment/tutorial.ipynb) for quickstart tutorials.
+
+
+ Segmentation Checkpoints
+
+
+
+We love your input! We want to make contributing to YOLOv5 as easy and transparent as possible. Please see our [Contributing Guide](https://docs.ultralytics.com/help/contributing/) to get started, and fill out the [YOLOv5 Survey](https://ultralytics.com/survey?utm_source=github&utm_medium=social&utm_campaign=Survey) to send us feedback on your experiences. Thank you to all our contributors!
+
+
+
+
+
+
+##
License
+
+YOLOv5 is available under two different licenses:
+
+- **AGPL-3.0 License**: See [LICENSE](https://github.com/ultralytics/yolov5/blob/master/LICENSE) file for details.
+- **Enterprise License**: Provides greater flexibility for commercial product development without the open-source requirements of AGPL-3.0. Typical use cases are embedding Ultralytics software and AI models in commercial products and applications. Request an Enterprise License at [Ultralytics Licensing](https://ultralytics.com/license).
+
+##
Contact
+
+For YOLOv5 bug reports and feature requests please visit [GitHub Issues](https://github.com/ultralytics/yolov5/issues), and join our [Discord](https://discord.gg/n6cFeSPZdD) community for questions and discussions!
+
+
+
+
+We are thrilled to announce the launch of Ultralytics YOLOv8 🚀, our NEW cutting-edge, state-of-the-art (SOTA) model
+released at **[https://github.com/ultralytics/ultralytics](https://github.com/ultralytics/ultralytics)**.
+YOLOv8 is designed to be fast, accurate, and easy to use, making it an excellent choice for a wide range of
+object detection, image segmentation and image classification tasks.
+
+See the [YOLOv8 Docs](https://docs.ultralytics.com) for details and get started with:
+
+```commandline
+pip install ultralytics
+```
+
+
+
+[tta]: https://docs.ultralytics.com/yolov5/tutorials/test_time_augmentation
diff --git a/yolov5/__pycache__/detect.cpython-38.pyc b/yolov5/__pycache__/detect.cpython-38.pyc
new file mode 100644
index 0000000000000000000000000000000000000000..89e0934df471ceac7e087c7fc767cce3d3b28e5e
Binary files /dev/null and b/yolov5/__pycache__/detect.cpython-38.pyc differ
diff --git a/yolov5/__pycache__/export.cpython-38.pyc b/yolov5/__pycache__/export.cpython-38.pyc
new file mode 100644
index 0000000000000000000000000000000000000000..173a26f08a4ac447ea74d6de1b00bc7427788c95
Binary files /dev/null and b/yolov5/__pycache__/export.cpython-38.pyc differ
diff --git a/yolov5/__pycache__/export.cpython-39.pyc b/yolov5/__pycache__/export.cpython-39.pyc
new file mode 100644
index 0000000000000000000000000000000000000000..ac788748188c08cd454365cc6e754c54e4f4b654
Binary files /dev/null and b/yolov5/__pycache__/export.cpython-39.pyc differ
diff --git a/yolov5/__pycache__/val.cpython-38.pyc b/yolov5/__pycache__/val.cpython-38.pyc
new file mode 100644
index 0000000000000000000000000000000000000000..587108c727dbb051ecc6e44d1016ca77749e5d24
Binary files /dev/null and b/yolov5/__pycache__/val.cpython-38.pyc differ
diff --git a/yolov5/benchmarks.py b/yolov5/benchmarks.py
new file mode 100644
index 0000000000000000000000000000000000000000..fc3073965ab306b99f332b954796131d3976ca31
--- /dev/null
+++ b/yolov5/benchmarks.py
@@ -0,0 +1,169 @@
+# YOLOv5 🚀 by Ultralytics, AGPL-3.0 license
+"""
+Run YOLOv5 benchmarks on all supported export formats
+
+Format | `export.py --include` | Model
+--- | --- | ---
+PyTorch | - | yolov5s.pt
+TorchScript | `torchscript` | yolov5s.torchscript
+ONNX | `onnx` | yolov5s.onnx
+OpenVINO | `openvino` | yolov5s_openvino_model/
+TensorRT | `engine` | yolov5s.engine
+CoreML | `coreml` | yolov5s.mlmodel
+TensorFlow SavedModel | `saved_model` | yolov5s_saved_model/
+TensorFlow GraphDef | `pb` | yolov5s.pb
+TensorFlow Lite | `tflite` | yolov5s.tflite
+TensorFlow Edge TPU | `edgetpu` | yolov5s_edgetpu.tflite
+TensorFlow.js | `tfjs` | yolov5s_web_model/
+
+Requirements:
+ $ pip install -r requirements.txt coremltools onnx onnx-simplifier onnxruntime openvino-dev tensorflow-cpu # CPU
+ $ pip install -r requirements.txt coremltools onnx onnx-simplifier onnxruntime-gpu openvino-dev tensorflow # GPU
+ $ pip install -U nvidia-tensorrt --index-url https://pypi.ngc.nvidia.com # TensorRT
+
+Usage:
+ $ python benchmarks.py --weights yolov5s.pt --img 640
+"""
+
+import argparse
+import platform
+import sys
+import time
+from pathlib import Path
+
+import pandas as pd
+
+FILE = Path(__file__).resolve()
+ROOT = FILE.parents[0] # YOLOv5 root directory
+if str(ROOT) not in sys.path:
+ sys.path.append(str(ROOT)) # add ROOT to PATH
+# ROOT = ROOT.relative_to(Path.cwd()) # relative
+
+import export
+from models.experimental import attempt_load
+from models.yolo import SegmentationModel
+from segment.val import run as val_seg
+from utils import notebook_init
+from utils.general import LOGGER, check_yaml, file_size, print_args
+from utils.torch_utils import select_device
+from val import run as val_det
+
+
+def run(
+ weights=ROOT / 'yolov5s.pt', # weights path
+ imgsz=640, # inference size (pixels)
+ batch_size=1, # batch size
+ data=ROOT / 'data/coco128.yaml', # dataset.yaml path
+ device='', # cuda device, i.e. 0 or 0,1,2,3 or cpu
+ half=False, # use FP16 half-precision inference
+ test=False, # test exports only
+ pt_only=False, # test PyTorch only
+ hard_fail=False, # throw error on benchmark failure
+):
+ y, t = [], time.time()
+ device = select_device(device)
+ model_type = type(attempt_load(weights, fuse=False)) # DetectionModel, SegmentationModel, etc.
+ for i, (name, f, suffix, cpu, gpu) in export.export_formats().iterrows(): # index, (name, file, suffix, CPU, GPU)
+ try:
+ assert i not in (9, 10), 'inference not supported' # Edge TPU and TF.js are unsupported
+ assert i != 5 or platform.system() == 'Darwin', 'inference only supported on macOS>=10.13' # CoreML
+ if 'cpu' in device.type:
+ assert cpu, 'inference not supported on CPU'
+ if 'cuda' in device.type:
+ assert gpu, 'inference not supported on GPU'
+
+ # Export
+ if f == '-':
+ w = weights # PyTorch format
+ else:
+ w = export.run(weights=weights, imgsz=[imgsz], include=[f], device=device, half=half)[-1] # all others
+ assert suffix in str(w), 'export failed'
+
+ # Validate
+ if model_type == SegmentationModel:
+ result = val_seg(data, w, batch_size, imgsz, plots=False, device=device, task='speed', half=half)
+ metric = result[0][7] # (box(p, r, map50, map), mask(p, r, map50, map), *loss(box, obj, cls))
+ else: # DetectionModel:
+ result = val_det(data, w, batch_size, imgsz, plots=False, device=device, task='speed', half=half)
+ metric = result[0][3] # (p, r, map50, map, *loss(box, obj, cls))
+ speed = result[2][1] # times (preprocess, inference, postprocess)
+ y.append([name, round(file_size(w), 1), round(metric, 4), round(speed, 2)]) # MB, mAP, t_inference
+ except Exception as e:
+ if hard_fail:
+ assert type(e) is AssertionError, f'Benchmark --hard-fail for {name}: {e}'
+ LOGGER.warning(f'WARNING ⚠️ Benchmark failure for {name}: {e}')
+ y.append([name, None, None, None]) # mAP, t_inference
+ if pt_only and i == 0:
+ break # break after PyTorch
+
+ # Print results
+ LOGGER.info('\n')
+ parse_opt()
+ notebook_init() # print system info
+ c = ['Format', 'Size (MB)', 'mAP50-95', 'Inference time (ms)'] if map else ['Format', 'Export', '', '']
+ py = pd.DataFrame(y, columns=c)
+ LOGGER.info(f'\nBenchmarks complete ({time.time() - t:.2f}s)')
+ LOGGER.info(str(py if map else py.iloc[:, :2]))
+ if hard_fail and isinstance(hard_fail, str):
+ metrics = py['mAP50-95'].array # values to compare to floor
+ floor = eval(hard_fail) # minimum metric floor to pass, i.e. = 0.29 mAP for YOLOv5n
+ assert all(x > floor for x in metrics if pd.notna(x)), f'HARD FAIL: mAP50-95 < floor {floor}'
+ return py
+
+
+def test(
+ weights=ROOT / 'yolov5s.pt', # weights path
+ imgsz=640, # inference size (pixels)
+ batch_size=1, # batch size
+ data=ROOT / 'data/coco128.yaml', # dataset.yaml path
+ device='', # cuda device, i.e. 0 or 0,1,2,3 or cpu
+ half=False, # use FP16 half-precision inference
+ test=False, # test exports only
+ pt_only=False, # test PyTorch only
+ hard_fail=False, # throw error on benchmark failure
+):
+ y, t = [], time.time()
+ device = select_device(device)
+ for i, (name, f, suffix, gpu) in export.export_formats().iterrows(): # index, (name, file, suffix, gpu-capable)
+ try:
+ w = weights if f == '-' else \
+ export.run(weights=weights, imgsz=[imgsz], include=[f], device=device, half=half)[-1] # weights
+ assert suffix in str(w), 'export failed'
+ y.append([name, True])
+ except Exception:
+ y.append([name, False]) # mAP, t_inference
+
+ # Print results
+ LOGGER.info('\n')
+ parse_opt()
+ notebook_init() # print system info
+ py = pd.DataFrame(y, columns=['Format', 'Export'])
+ LOGGER.info(f'\nExports complete ({time.time() - t:.2f}s)')
+ LOGGER.info(str(py))
+ return py
+
+
+def parse_opt():
+ parser = argparse.ArgumentParser()
+ parser.add_argument('--weights', type=str, default=ROOT / 'yolov5s.pt', help='weights path')
+ parser.add_argument('--imgsz', '--img', '--img-size', type=int, default=640, help='inference size (pixels)')
+ parser.add_argument('--batch-size', type=int, default=1, help='batch size')
+ parser.add_argument('--data', type=str, default=ROOT / 'data/coco128.yaml', help='dataset.yaml path')
+ parser.add_argument('--device', default='', help='cuda device, i.e. 0 or 0,1,2,3 or cpu')
+ parser.add_argument('--half', action='store_true', help='use FP16 half-precision inference')
+ parser.add_argument('--test', action='store_true', help='test exports only')
+ parser.add_argument('--pt-only', action='store_true', help='test PyTorch only')
+ parser.add_argument('--hard-fail', nargs='?', const=True, default=False, help='Exception on error or < min metric')
+ opt = parser.parse_args()
+ opt.data = check_yaml(opt.data) # check YAML
+ print_args(vars(opt))
+ return opt
+
+
+def main(opt):
+ test(**vars(opt)) if opt.test else run(**vars(opt))
+
+
+if __name__ == '__main__':
+ opt = parse_opt()
+ main(opt)
diff --git a/yolov5/classify/predict.py b/yolov5/classify/predict.py
new file mode 100644
index 0000000000000000000000000000000000000000..c1b6650d4bd0e40690f16c38ac57c2820f070361
--- /dev/null
+++ b/yolov5/classify/predict.py
@@ -0,0 +1,226 @@
+# YOLOv5 🚀 by Ultralytics, AGPL-3.0 license
+"""
+Run YOLOv5 classification inference on images, videos, directories, globs, YouTube, webcam, streams, etc.
+
+Usage - sources:
+ $ python classify/predict.py --weights yolov5s-cls.pt --source 0 # webcam
+ img.jpg # image
+ vid.mp4 # video
+ screen # screenshot
+ path/ # directory
+ list.txt # list of images
+ list.streams # list of streams
+ 'path/*.jpg' # glob
+ 'https://youtu.be/Zgi9g1ksQHc' # YouTube
+ 'rtsp://example.com/media.mp4' # RTSP, RTMP, HTTP stream
+
+Usage - formats:
+ $ python classify/predict.py --weights yolov5s-cls.pt # PyTorch
+ yolov5s-cls.torchscript # TorchScript
+ yolov5s-cls.onnx # ONNX Runtime or OpenCV DNN with --dnn
+ yolov5s-cls_openvino_model # OpenVINO
+ yolov5s-cls.engine # TensorRT
+ yolov5s-cls.mlmodel # CoreML (macOS-only)
+ yolov5s-cls_saved_model # TensorFlow SavedModel
+ yolov5s-cls.pb # TensorFlow GraphDef
+ yolov5s-cls.tflite # TensorFlow Lite
+ yolov5s-cls_edgetpu.tflite # TensorFlow Edge TPU
+ yolov5s-cls_paddle_model # PaddlePaddle
+"""
+
+import argparse
+import os
+import platform
+import sys
+from pathlib import Path
+
+import torch
+import torch.nn.functional as F
+
+FILE = Path(__file__).resolve()
+ROOT = FILE.parents[1] # YOLOv5 root directory
+if str(ROOT) not in sys.path:
+ sys.path.append(str(ROOT)) # add ROOT to PATH
+ROOT = Path(os.path.relpath(ROOT, Path.cwd())) # relative
+
+from models.common import DetectMultiBackend
+from utils.augmentations import classify_transforms
+from utils.dataloaders import IMG_FORMATS, VID_FORMATS, LoadImages, LoadScreenshots, LoadStreams
+from utils.general import (LOGGER, Profile, check_file, check_img_size, check_imshow, check_requirements, colorstr, cv2,
+ increment_path, print_args, strip_optimizer)
+from utils.plots import Annotator
+from utils.torch_utils import select_device, smart_inference_mode
+
+
+@smart_inference_mode()
+def run(
+ weights=ROOT / 'yolov5s-cls.pt', # model.pt path(s)
+ source=ROOT / 'data/images', # file/dir/URL/glob/screen/0(webcam)
+ data=ROOT / 'data/coco128.yaml', # dataset.yaml path
+ imgsz=(224, 224), # inference size (height, width)
+ device='', # cuda device, i.e. 0 or 0,1,2,3 or cpu
+ view_img=False, # show results
+ save_txt=False, # save results to *.txt
+ nosave=False, # do not save images/videos
+ augment=False, # augmented inference
+ visualize=False, # visualize features
+ update=False, # update all models
+ project=ROOT / 'runs/predict-cls', # save results to project/name
+ name='exp', # save results to project/name
+ exist_ok=False, # existing project/name ok, do not increment
+ half=False, # use FP16 half-precision inference
+ dnn=False, # use OpenCV DNN for ONNX inference
+ vid_stride=1, # video frame-rate stride
+):
+ source = str(source)
+ save_img = not nosave and not source.endswith('.txt') # save inference images
+ is_file = Path(source).suffix[1:] in (IMG_FORMATS + VID_FORMATS)
+ is_url = source.lower().startswith(('rtsp://', 'rtmp://', 'http://', 'https://'))
+ webcam = source.isnumeric() or source.endswith('.streams') or (is_url and not is_file)
+ screenshot = source.lower().startswith('screen')
+ if is_url and is_file:
+ source = check_file(source) # download
+
+ # Directories
+ save_dir = increment_path(Path(project) / name, exist_ok=exist_ok) # increment run
+ (save_dir / 'labels' if save_txt else save_dir).mkdir(parents=True, exist_ok=True) # make dir
+
+ # Load model
+ device = select_device(device)
+ model = DetectMultiBackend(weights, device=device, dnn=dnn, data=data, fp16=half)
+ stride, names, pt = model.stride, model.names, model.pt
+ imgsz = check_img_size(imgsz, s=stride) # check image size
+
+ # Dataloader
+ bs = 1 # batch_size
+ if webcam:
+ view_img = check_imshow(warn=True)
+ dataset = LoadStreams(source, img_size=imgsz, transforms=classify_transforms(imgsz[0]), vid_stride=vid_stride)
+ bs = len(dataset)
+ elif screenshot:
+ dataset = LoadScreenshots(source, img_size=imgsz, stride=stride, auto=pt)
+ else:
+ dataset = LoadImages(source, img_size=imgsz, transforms=classify_transforms(imgsz[0]), vid_stride=vid_stride)
+ vid_path, vid_writer = [None] * bs, [None] * bs
+
+ # Run inference
+ model.warmup(imgsz=(1 if pt else bs, 3, *imgsz)) # warmup
+ seen, windows, dt = 0, [], (Profile(), Profile(), Profile())
+ for path, im, im0s, vid_cap, s in dataset:
+ with dt[0]:
+ im = torch.Tensor(im).to(model.device)
+ im = im.half() if model.fp16 else im.float() # uint8 to fp16/32
+ if len(im.shape) == 3:
+ im = im[None] # expand for batch dim
+
+ # Inference
+ with dt[1]:
+ results = model(im)
+
+ # Post-process
+ with dt[2]:
+ pred = F.softmax(results, dim=1) # probabilities
+
+ # Process predictions
+ for i, prob in enumerate(pred): # per image
+ seen += 1
+ if webcam: # batch_size >= 1
+ p, im0, frame = path[i], im0s[i].copy(), dataset.count
+ s += f'{i}: '
+ else:
+ p, im0, frame = path, im0s.copy(), getattr(dataset, 'frame', 0)
+
+ p = Path(p) # to Path
+ save_path = str(save_dir / p.name) # im.jpg
+ txt_path = str(save_dir / 'labels' / p.stem) + ('' if dataset.mode == 'image' else f'_{frame}') # im.txt
+
+ s += '%gx%g ' % im.shape[2:] # print string
+ annotator = Annotator(im0, example=str(names), pil=True)
+
+ # Print results
+ top5i = prob.argsort(0, descending=True)[:5].tolist() # top 5 indices
+ s += f"{', '.join(f'{names[j]} {prob[j]:.2f}' for j in top5i)}, "
+
+ # Write results
+ text = '\n'.join(f'{prob[j]:.2f} {names[j]}' for j in top5i)
+ if save_img or view_img: # Add bbox to image
+ annotator.text((32, 32), text, txt_color=(255, 255, 255))
+ if save_txt: # Write to file
+ with open(f'{txt_path}.txt', 'a') as f:
+ f.write(text + '\n')
+
+ # Stream results
+ im0 = annotator.result()
+ if view_img:
+ if platform.system() == 'Linux' and p not in windows:
+ windows.append(p)
+ cv2.namedWindow(str(p), cv2.WINDOW_NORMAL | cv2.WINDOW_KEEPRATIO) # allow window resize (Linux)
+ cv2.resizeWindow(str(p), im0.shape[1], im0.shape[0])
+ cv2.imshow(str(p), im0)
+ cv2.waitKey(1) # 1 millisecond
+
+ # Save results (image with detections)
+ if save_img:
+ if dataset.mode == 'image':
+ cv2.imwrite(save_path, im0)
+ else: # 'video' or 'stream'
+ if vid_path[i] != save_path: # new video
+ vid_path[i] = save_path
+ if isinstance(vid_writer[i], cv2.VideoWriter):
+ vid_writer[i].release() # release previous video writer
+ if vid_cap: # video
+ fps = vid_cap.get(cv2.CAP_PROP_FPS)
+ w = int(vid_cap.get(cv2.CAP_PROP_FRAME_WIDTH))
+ h = int(vid_cap.get(cv2.CAP_PROP_FRAME_HEIGHT))
+ else: # stream
+ fps, w, h = 30, im0.shape[1], im0.shape[0]
+ save_path = str(Path(save_path).with_suffix('.mp4')) # force *.mp4 suffix on results videos
+ vid_writer[i] = cv2.VideoWriter(save_path, cv2.VideoWriter_fourcc(*'mp4v'), fps, (w, h))
+ vid_writer[i].write(im0)
+
+ # Print time (inference-only)
+ LOGGER.info(f'{s}{dt[1].dt * 1E3:.1f}ms')
+
+ # Print results
+ t = tuple(x.t / seen * 1E3 for x in dt) # speeds per image
+ LOGGER.info(f'Speed: %.1fms pre-process, %.1fms inference, %.1fms NMS per image at shape {(1, 3, *imgsz)}' % t)
+ if save_txt or save_img:
+ s = f"\n{len(list(save_dir.glob('labels/*.txt')))} labels saved to {save_dir / 'labels'}" if save_txt else ''
+ LOGGER.info(f"Results saved to {colorstr('bold', save_dir)}{s}")
+ if update:
+ strip_optimizer(weights[0]) # update model (to fix SourceChangeWarning)
+
+
+def parse_opt():
+ parser = argparse.ArgumentParser()
+ parser.add_argument('--weights', nargs='+', type=str, default=ROOT / 'yolov5s-cls.pt', help='model path(s)')
+ parser.add_argument('--source', type=str, default=ROOT / 'data/images', help='file/dir/URL/glob/screen/0(webcam)')
+ parser.add_argument('--data', type=str, default=ROOT / 'data/coco128.yaml', help='(optional) dataset.yaml path')
+ parser.add_argument('--imgsz', '--img', '--img-size', nargs='+', type=int, default=[224], help='inference size h,w')
+ parser.add_argument('--device', default='', help='cuda device, i.e. 0 or 0,1,2,3 or cpu')
+ parser.add_argument('--view-img', action='store_true', help='show results')
+ parser.add_argument('--save-txt', action='store_true', help='save results to *.txt')
+ parser.add_argument('--nosave', action='store_true', help='do not save images/videos')
+ parser.add_argument('--augment', action='store_true', help='augmented inference')
+ parser.add_argument('--visualize', action='store_true', help='visualize features')
+ parser.add_argument('--update', action='store_true', help='update all models')
+ parser.add_argument('--project', default=ROOT / 'runs/predict-cls', help='save results to project/name')
+ parser.add_argument('--name', default='exp', help='save results to project/name')
+ parser.add_argument('--exist-ok', action='store_true', help='existing project/name ok, do not increment')
+ parser.add_argument('--half', action='store_true', help='use FP16 half-precision inference')
+ parser.add_argument('--dnn', action='store_true', help='use OpenCV DNN for ONNX inference')
+ parser.add_argument('--vid-stride', type=int, default=1, help='video frame-rate stride')
+ opt = parser.parse_args()
+ opt.imgsz *= 2 if len(opt.imgsz) == 1 else 1 # expand
+ print_args(vars(opt))
+ return opt
+
+
+def main(opt):
+ check_requirements(exclude=('tensorboard', 'thop'))
+ run(**vars(opt))
+
+
+if __name__ == '__main__':
+ opt = parse_opt()
+ main(opt)
diff --git a/yolov5/classify/train.py b/yolov5/classify/train.py
new file mode 100644
index 0000000000000000000000000000000000000000..8b8327f173ef1bee725685d5a6a38c784f976ece
--- /dev/null
+++ b/yolov5/classify/train.py
@@ -0,0 +1,333 @@
+# YOLOv5 🚀 by Ultralytics, AGPL-3.0 license
+"""
+Train a YOLOv5 classifier model on a classification dataset
+
+Usage - Single-GPU training:
+ $ python classify/train.py --model yolov5s-cls.pt --data imagenette160 --epochs 5 --img 224
+
+Usage - Multi-GPU DDP training:
+ $ python -m torch.distributed.run --nproc_per_node 4 --master_port 2022 classify/train.py --model yolov5s-cls.pt --data imagenet --epochs 5 --img 224 --device 0,1,2,3
+
+Datasets: --data mnist, fashion-mnist, cifar10, cifar100, imagenette, imagewoof, imagenet, or 'path/to/data'
+YOLOv5-cls models: --model yolov5n-cls.pt, yolov5s-cls.pt, yolov5m-cls.pt, yolov5l-cls.pt, yolov5x-cls.pt
+Torchvision models: --model resnet50, efficientnet_b0, etc. See https://pytorch.org/vision/stable/models.html
+"""
+
+import argparse
+import os
+import subprocess
+import sys
+import time
+from copy import deepcopy
+from datetime import datetime
+from pathlib import Path
+
+import torch
+import torch.distributed as dist
+import torch.hub as hub
+import torch.optim.lr_scheduler as lr_scheduler
+import torchvision
+from torch.cuda import amp
+from tqdm import tqdm
+
+FILE = Path(__file__).resolve()
+ROOT = FILE.parents[1] # YOLOv5 root directory
+if str(ROOT) not in sys.path:
+ sys.path.append(str(ROOT)) # add ROOT to PATH
+ROOT = Path(os.path.relpath(ROOT, Path.cwd())) # relative
+
+from classify import val as validate
+from models.experimental import attempt_load
+from models.yolo import ClassificationModel, DetectionModel
+from utils.dataloaders import create_classification_dataloader
+from utils.general import (DATASETS_DIR, LOGGER, TQDM_BAR_FORMAT, WorkingDirectory, check_git_info, check_git_status,
+ check_requirements, colorstr, download, increment_path, init_seeds, print_args, yaml_save)
+from utils.loggers import GenericLogger
+from utils.plots import imshow_cls
+from utils.torch_utils import (ModelEMA, de_parallel, model_info, reshape_classifier_output, select_device, smart_DDP,
+ smart_optimizer, smartCrossEntropyLoss, torch_distributed_zero_first)
+
+LOCAL_RANK = int(os.getenv('LOCAL_RANK', -1)) # https://pytorch.org/docs/stable/elastic/run.html
+RANK = int(os.getenv('RANK', -1))
+WORLD_SIZE = int(os.getenv('WORLD_SIZE', 1))
+GIT_INFO = check_git_info()
+
+
+def train(opt, device):
+ init_seeds(opt.seed + 1 + RANK, deterministic=True)
+ save_dir, data, bs, epochs, nw, imgsz, pretrained = \
+ opt.save_dir, Path(opt.data), opt.batch_size, opt.epochs, min(os.cpu_count() - 1, opt.workers), \
+ opt.imgsz, str(opt.pretrained).lower() == 'true'
+ cuda = device.type != 'cpu'
+
+ # Directories
+ wdir = save_dir / 'weights'
+ wdir.mkdir(parents=True, exist_ok=True) # make dir
+ last, best = wdir / 'last.pt', wdir / 'best.pt'
+
+ # Save run settings
+ yaml_save(save_dir / 'opt.yaml', vars(opt))
+
+ # Logger
+ logger = GenericLogger(opt=opt, console_logger=LOGGER) if RANK in {-1, 0} else None
+
+ # Download Dataset
+ with torch_distributed_zero_first(LOCAL_RANK), WorkingDirectory(ROOT):
+ data_dir = data if data.is_dir() else (DATASETS_DIR / data)
+ if not data_dir.is_dir():
+ LOGGER.info(f'\nDataset not found ⚠️, missing path {data_dir}, attempting download...')
+ t = time.time()
+ if str(data) == 'imagenet':
+ subprocess.run(['bash', str(ROOT / 'data/scripts/get_imagenet.sh')], shell=True, check=True)
+ else:
+ url = f'https://github.com/ultralytics/yolov5/releases/download/v1.0/{data}.zip'
+ download(url, dir=data_dir.parent)
+ s = f"Dataset download success ✅ ({time.time() - t:.1f}s), saved to {colorstr('bold', data_dir)}\n"
+ LOGGER.info(s)
+
+ # Dataloaders
+ nc = len([x for x in (data_dir / 'train').glob('*') if x.is_dir()]) # number of classes
+ trainloader = create_classification_dataloader(path=data_dir / 'train',
+ imgsz=imgsz,
+ batch_size=bs // WORLD_SIZE,
+ augment=True,
+ cache=opt.cache,
+ rank=LOCAL_RANK,
+ workers=nw)
+
+ test_dir = data_dir / 'test' if (data_dir / 'test').exists() else data_dir / 'val' # data/test or data/val
+ if RANK in {-1, 0}:
+ testloader = create_classification_dataloader(path=test_dir,
+ imgsz=imgsz,
+ batch_size=bs // WORLD_SIZE * 2,
+ augment=False,
+ cache=opt.cache,
+ rank=-1,
+ workers=nw)
+
+ # Model
+ with torch_distributed_zero_first(LOCAL_RANK), WorkingDirectory(ROOT):
+ if Path(opt.model).is_file() or opt.model.endswith('.pt'):
+ model = attempt_load(opt.model, device='cpu', fuse=False)
+ elif opt.model in torchvision.models.__dict__: # TorchVision models i.e. resnet50, efficientnet_b0
+ model = torchvision.models.__dict__[opt.model](weights='IMAGENET1K_V1' if pretrained else None)
+ else:
+ m = hub.list('ultralytics/yolov5') # + hub.list('pytorch/vision') # models
+ raise ModuleNotFoundError(f'--model {opt.model} not found. Available models are: \n' + '\n'.join(m))
+ if isinstance(model, DetectionModel):
+ LOGGER.warning("WARNING ⚠️ pass YOLOv5 classifier model with '-cls' suffix, i.e. '--model yolov5s-cls.pt'")
+ model = ClassificationModel(model=model, nc=nc, cutoff=opt.cutoff or 10) # convert to classification model
+ reshape_classifier_output(model, nc) # update class count
+ for m in model.modules():
+ if not pretrained and hasattr(m, 'reset_parameters'):
+ m.reset_parameters()
+ if isinstance(m, torch.nn.Dropout) and opt.dropout is not None:
+ m.p = opt.dropout # set dropout
+ for p in model.parameters():
+ p.requires_grad = True # for training
+ model = model.to(device)
+
+ # Info
+ if RANK in {-1, 0}:
+ model.names = trainloader.dataset.classes # attach class names
+ model.transforms = testloader.dataset.torch_transforms # attach inference transforms
+ model_info(model)
+ if opt.verbose:
+ LOGGER.info(model)
+ images, labels = next(iter(trainloader))
+ file = imshow_cls(images[:25], labels[:25], names=model.names, f=save_dir / 'train_images.jpg')
+ logger.log_images(file, name='Train Examples')
+ logger.log_graph(model, imgsz) # log model
+
+ # Optimizer
+ optimizer = smart_optimizer(model, opt.optimizer, opt.lr0, momentum=0.9, decay=opt.decay)
+
+ # Scheduler
+ lrf = 0.01 # final lr (fraction of lr0)
+ # lf = lambda x: ((1 + math.cos(x * math.pi / epochs)) / 2) * (1 - lrf) + lrf # cosine
+ lf = lambda x: (1 - x / epochs) * (1 - lrf) + lrf # linear
+ scheduler = lr_scheduler.LambdaLR(optimizer, lr_lambda=lf)
+ # scheduler = lr_scheduler.OneCycleLR(optimizer, max_lr=lr0, total_steps=epochs, pct_start=0.1,
+ # final_div_factor=1 / 25 / lrf)
+
+ # EMA
+ ema = ModelEMA(model) if RANK in {-1, 0} else None
+
+ # DDP mode
+ if cuda and RANK != -1:
+ model = smart_DDP(model)
+
+ # Train
+ t0 = time.time()
+ criterion = smartCrossEntropyLoss(label_smoothing=opt.label_smoothing) # loss function
+ best_fitness = 0.0
+ scaler = amp.GradScaler(enabled=cuda)
+ val = test_dir.stem # 'val' or 'test'
+ LOGGER.info(f'Image sizes {imgsz} train, {imgsz} test\n'
+ f'Using {nw * WORLD_SIZE} dataloader workers\n'
+ f"Logging results to {colorstr('bold', save_dir)}\n"
+ f'Starting {opt.model} training on {data} dataset with {nc} classes for {epochs} epochs...\n\n'
+ f"{'Epoch':>10}{'GPU_mem':>10}{'train_loss':>12}{f'{val}_loss':>12}{'top1_acc':>12}{'top5_acc':>12}")
+ for epoch in range(epochs): # loop over the dataset multiple times
+ tloss, vloss, fitness = 0.0, 0.0, 0.0 # train loss, val loss, fitness
+ model.train()
+ if RANK != -1:
+ trainloader.sampler.set_epoch(epoch)
+ pbar = enumerate(trainloader)
+ if RANK in {-1, 0}:
+ pbar = tqdm(enumerate(trainloader), total=len(trainloader), bar_format=TQDM_BAR_FORMAT)
+ for i, (images, labels) in pbar: # progress bar
+ images, labels = images.to(device, non_blocking=True), labels.to(device)
+
+ # Forward
+ with amp.autocast(enabled=cuda): # stability issues when enabled
+ loss = criterion(model(images), labels)
+
+ # Backward
+ scaler.scale(loss).backward()
+
+ # Optimize
+ scaler.unscale_(optimizer) # unscale gradients
+ torch.nn.utils.clip_grad_norm_(model.parameters(), max_norm=10.0) # clip gradients
+ scaler.step(optimizer)
+ scaler.update()
+ optimizer.zero_grad()
+ if ema:
+ ema.update(model)
+
+ if RANK in {-1, 0}:
+ # Print
+ tloss = (tloss * i + loss.item()) / (i + 1) # update mean losses
+ mem = '%.3gG' % (torch.cuda.memory_reserved() / 1E9 if torch.cuda.is_available() else 0) # (GB)
+ pbar.desc = f"{f'{epoch + 1}/{epochs}':>10}{mem:>10}{tloss:>12.3g}" + ' ' * 36
+
+ # Test
+ if i == len(pbar) - 1: # last batch
+ top1, top5, vloss = validate.run(model=ema.ema,
+ dataloader=testloader,
+ criterion=criterion,
+ pbar=pbar) # test accuracy, loss
+ fitness = top1 # define fitness as top1 accuracy
+
+ # Scheduler
+ scheduler.step()
+
+ # Log metrics
+ if RANK in {-1, 0}:
+ # Best fitness
+ if fitness > best_fitness:
+ best_fitness = fitness
+
+ # Log
+ metrics = {
+ 'train/loss': tloss,
+ f'{val}/loss': vloss,
+ 'metrics/accuracy_top1': top1,
+ 'metrics/accuracy_top5': top5,
+ 'lr/0': optimizer.param_groups[0]['lr']} # learning rate
+ logger.log_metrics(metrics, epoch)
+
+ # Save model
+ final_epoch = epoch + 1 == epochs
+ if (not opt.nosave) or final_epoch:
+ ckpt = {
+ 'epoch': epoch,
+ 'best_fitness': best_fitness,
+ 'model': deepcopy(ema.ema).half(), # deepcopy(de_parallel(model)).half(),
+ 'ema': None, # deepcopy(ema.ema).half(),
+ 'updates': ema.updates,
+ 'optimizer': None, # optimizer.state_dict(),
+ 'opt': vars(opt),
+ 'git': GIT_INFO, # {remote, branch, commit} if a git repo
+ 'date': datetime.now().isoformat()}
+
+ # Save last, best and delete
+ torch.save(ckpt, last)
+ if best_fitness == fitness:
+ torch.save(ckpt, best)
+ del ckpt
+
+ # Train complete
+ if RANK in {-1, 0} and final_epoch:
+ LOGGER.info(f'\nTraining complete ({(time.time() - t0) / 3600:.3f} hours)'
+ f"\nResults saved to {colorstr('bold', save_dir)}"
+ f'\nPredict: python classify/predict.py --weights {best} --source im.jpg'
+ f'\nValidate: python classify/val.py --weights {best} --data {data_dir}'
+ f'\nExport: python export.py --weights {best} --include onnx'
+ f"\nPyTorch Hub: model = torch.hub.load('ultralytics/yolov5', 'custom', '{best}')"
+ f'\nVisualize: https://netron.app\n')
+
+ # Plot examples
+ images, labels = (x[:25] for x in next(iter(testloader))) # first 25 images and labels
+ pred = torch.max(ema.ema(images.to(device)), 1)[1]
+ file = imshow_cls(images, labels, pred, de_parallel(model).names, verbose=False, f=save_dir / 'test_images.jpg')
+
+ # Log results
+ meta = {'epochs': epochs, 'top1_acc': best_fitness, 'date': datetime.now().isoformat()}
+ logger.log_images(file, name='Test Examples (true-predicted)', epoch=epoch)
+ logger.log_model(best, epochs, metadata=meta)
+
+
+def parse_opt(known=False):
+ parser = argparse.ArgumentParser()
+ parser.add_argument('--model', type=str, default='yolov5s-cls.pt', help='initial weights path')
+ parser.add_argument('--data', type=str, default='imagenette160', help='cifar10, cifar100, mnist, imagenet, ...')
+ parser.add_argument('--epochs', type=int, default=10, help='total training epochs')
+ parser.add_argument('--batch-size', type=int, default=64, help='total batch size for all GPUs')
+ parser.add_argument('--imgsz', '--img', '--img-size', type=int, default=224, help='train, val image size (pixels)')
+ parser.add_argument('--nosave', action='store_true', help='only save final checkpoint')
+ parser.add_argument('--cache', type=str, nargs='?', const='ram', help='--cache images in "ram" (default) or "disk"')
+ parser.add_argument('--device', default='', help='cuda device, i.e. 0 or 0,1,2,3 or cpu')
+ parser.add_argument('--workers', type=int, default=8, help='max dataloader workers (per RANK in DDP mode)')
+ parser.add_argument('--project', default=ROOT / 'runs/train-cls', help='save to project/name')
+ parser.add_argument('--name', default='exp', help='save to project/name')
+ parser.add_argument('--exist-ok', action='store_true', help='existing project/name ok, do not increment')
+ parser.add_argument('--pretrained', nargs='?', const=True, default=True, help='start from i.e. --pretrained False')
+ parser.add_argument('--optimizer', choices=['SGD', 'Adam', 'AdamW', 'RMSProp'], default='Adam', help='optimizer')
+ parser.add_argument('--lr0', type=float, default=0.001, help='initial learning rate')
+ parser.add_argument('--decay', type=float, default=5e-5, help='weight decay')
+ parser.add_argument('--label-smoothing', type=float, default=0.1, help='Label smoothing epsilon')
+ parser.add_argument('--cutoff', type=int, default=None, help='Model layer cutoff index for Classify() head')
+ parser.add_argument('--dropout', type=float, default=None, help='Dropout (fraction)')
+ parser.add_argument('--verbose', action='store_true', help='Verbose mode')
+ parser.add_argument('--seed', type=int, default=0, help='Global training seed')
+ parser.add_argument('--local_rank', type=int, default=-1, help='Automatic DDP Multi-GPU argument, do not modify')
+ return parser.parse_known_args()[0] if known else parser.parse_args()
+
+
+def main(opt):
+ # Checks
+ if RANK in {-1, 0}:
+ print_args(vars(opt))
+ check_git_status()
+ check_requirements()
+
+ # DDP mode
+ device = select_device(opt.device, batch_size=opt.batch_size)
+ if LOCAL_RANK != -1:
+ assert opt.batch_size != -1, 'AutoBatch is coming soon for classification, please pass a valid --batch-size'
+ assert opt.batch_size % WORLD_SIZE == 0, f'--batch-size {opt.batch_size} must be multiple of WORLD_SIZE'
+ assert torch.cuda.device_count() > LOCAL_RANK, 'insufficient CUDA devices for DDP command'
+ torch.cuda.set_device(LOCAL_RANK)
+ device = torch.device('cuda', LOCAL_RANK)
+ dist.init_process_group(backend='nccl' if dist.is_nccl_available() else 'gloo')
+
+ # Parameters
+ opt.save_dir = increment_path(Path(opt.project) / opt.name, exist_ok=opt.exist_ok) # increment run
+
+ # Train
+ train(opt, device)
+
+
+def run(**kwargs):
+ # Usage: from yolov5 import classify; classify.train.run(data=mnist, imgsz=320, model='yolov5m')
+ opt = parse_opt(True)
+ for k, v in kwargs.items():
+ setattr(opt, k, v)
+ main(opt)
+ return opt
+
+
+if __name__ == '__main__':
+ opt = parse_opt()
+ main(opt)
diff --git a/yolov5/classify/tutorial.ipynb b/yolov5/classify/tutorial.ipynb
new file mode 100644
index 0000000000000000000000000000000000000000..75eebd8e11321da5e42d5d51f5a9066a336c177e
--- /dev/null
+++ b/yolov5/classify/tutorial.ipynb
@@ -0,0 +1,1481 @@
+{
+ "cells": [
+ {
+ "cell_type": "markdown",
+ "metadata": {
+ "id": "t6MPjfT5NrKQ"
+ },
+ "source": [
+ "
\n",
+ "\n",
+ " \n",
+ " \n",
+ "\n",
+ "\n",
+ " \n",
+ " \n",
+ " \n",
+ " \n",
+ " \n",
+ "\n",
+ "This YOLOv5 🚀 notebook by Ultralytics presents simple train, validate and predict examples to help start your AI adventure. See GitHub for community support or contact us for professional support.\n",
+ "\n",
+ "
\n",
+ "Close the active learning loop by sampling images from your inference conditions with the `roboflow` pip package\n",
+ "
\n",
+ "\n",
+ "Train a YOLOv5s Classification model on the [Imagenette](https://image-net.org/) dataset with `--data imagenet`, starting from pretrained `--pretrained yolov5s-cls.pt`.\n",
+ "\n",
+ "- **Pretrained [Models](https://github.com/ultralytics/yolov5/tree/master/models)** are downloaded\n",
+ "automatically from the [latest YOLOv5 release](https://github.com/ultralytics/yolov5/releases)\n",
+ "- **Training Results** are saved to `runs/train-cls/` with incrementing run directories, i.e. `runs/train-cls/exp2`, `runs/train-cls/exp3` etc.\n",
+ "
\n",
+ "\n",
+ "A **Mosaic Dataloader** is used for training which combines 4 images into 1 mosaic.\n",
+ "\n",
+ "## Train on Custom Data with Roboflow 🌟 NEW\n",
+ "\n",
+ "[Roboflow](https://roboflow.com/?ref=ultralytics) enables you to easily **organize, label, and prepare** a high quality dataset with your own custom data. Roboflow also makes it easy to establish an active learning pipeline, collaborate with your team on dataset improvement, and integrate directly into your model building workflow with the `roboflow` pip package.\n",
+ "\n",
+ "- Custom Training Example: [https://blog.roboflow.com/train-yolov5-classification-custom-data/](https://blog.roboflow.com/train-yolov5-classification-custom-data/?ref=ultralytics)\n",
+ "- Custom Training Notebook: [](https://colab.research.google.com/drive/1KZiKUAjtARHAfZCXbJRv14-pOnIsBLPV?usp=sharing)\n",
+ " \n",
+ "\n",
+ "
Label images lightning fast (including with model-assisted labeling)"
+ ]
+ },
+ {
+ "cell_type": "code",
+ "execution_count": null,
+ "metadata": {
+ "id": "i3oKtE4g-aNn"
+ },
+ "outputs": [],
+ "source": [
+ "#@title Select YOLOv5 🚀 logger {run: 'auto'}\n",
+ "logger = 'Comet' #@param ['Comet', 'ClearML', 'TensorBoard']\n",
+ "\n",
+ "if logger == 'Comet':\n",
+ " %pip install -q comet_ml\n",
+ " import comet_ml; comet_ml.init()\n",
+ "elif logger == 'ClearML':\n",
+ " %pip install -q clearml\n",
+ " import clearml; clearml.browser_login()\n",
+ "elif logger == 'TensorBoard':\n",
+ " %load_ext tensorboard\n",
+ " %tensorboard --logdir runs/train"
+ ]
+ },
+ {
+ "cell_type": "code",
+ "execution_count": null,
+ "metadata": {
+ "colab": {
+ "base_uri": "https://localhost:8080/"
+ },
+ "id": "1NcFxRcFdJ_O",
+ "outputId": "77c8d487-16db-4073-b3ea-06cabf2e7766"
+ },
+ "outputs": [
+ {
+ "output_type": "stream",
+ "name": "stdout",
+ "text": [
+ "\u001b[34m\u001b[1mclassify/train: \u001b[0mmodel=yolov5s-cls.pt, data=imagenette160, epochs=5, batch_size=64, imgsz=224, nosave=False, cache=ram, device=, workers=8, project=runs/train-cls, name=exp, exist_ok=False, pretrained=True, optimizer=Adam, lr0=0.001, decay=5e-05, label_smoothing=0.1, cutoff=None, dropout=None, verbose=False, seed=0, local_rank=-1\n",
+ "\u001b[34m\u001b[1mgithub: \u001b[0mup to date with https://github.com/ultralytics/yolov5 ✅\n",
+ "YOLOv5 🚀 v7.0-3-g61ebf5e Python-3.7.15 torch-1.12.1+cu113 CUDA:0 (Tesla T4, 15110MiB)\n",
+ "\n",
+ "\u001b[34m\u001b[1mTensorBoard: \u001b[0mStart with 'tensorboard --logdir runs/train-cls', view at http://localhost:6006/\n",
+ "\n",
+ "Dataset not found ⚠️, missing path /content/datasets/imagenette160, attempting download...\n",
+ "Downloading https://github.com/ultralytics/yolov5/releases/download/v1.0/imagenette160.zip to /content/datasets/imagenette160.zip...\n",
+ "100% 103M/103M [00:00<00:00, 347MB/s] \n",
+ "Unzipping /content/datasets/imagenette160.zip...\n",
+ "Dataset download success ✅ (3.3s), saved to \u001b[1m/content/datasets/imagenette160\u001b[0m\n",
+ "\n",
+ "\u001b[34m\u001b[1malbumentations: \u001b[0mRandomResizedCrop(p=1.0, height=224, width=224, scale=(0.08, 1.0), ratio=(0.75, 1.3333333333333333), interpolation=1), HorizontalFlip(p=0.5), ColorJitter(p=0.5, brightness=[0.6, 1.4], contrast=[0.6, 1.4], saturation=[0.6, 1.4], hue=[0, 0]), Normalize(p=1.0, mean=(0.485, 0.456, 0.406), std=(0.229, 0.224, 0.225), max_pixel_value=255.0), ToTensorV2(always_apply=True, p=1.0, transpose_mask=False)\n",
+ "Model summary: 149 layers, 4185290 parameters, 4185290 gradients, 10.5 GFLOPs\n",
+ "\u001b[34m\u001b[1moptimizer:\u001b[0m Adam(lr=0.001) with parameter groups 32 weight(decay=0.0), 33 weight(decay=5e-05), 33 bias\n",
+ "Image sizes 224 train, 224 test\n",
+ "Using 1 dataloader workers\n",
+ "Logging results to \u001b[1mruns/train-cls/exp\u001b[0m\n",
+ "Starting yolov5s-cls.pt training on imagenette160 dataset with 10 classes for 5 epochs...\n",
+ "\n",
+ " Epoch GPU_mem train_loss val_loss top1_acc top5_acc\n",
+ " 1/5 1.47G 1.05 0.974 0.828 0.975: 100% 148/148 [00:38<00:00, 3.82it/s]\n",
+ " 2/5 1.73G 0.895 0.766 0.911 0.994: 100% 148/148 [00:36<00:00, 4.03it/s]\n",
+ " 3/5 1.73G 0.82 0.704 0.934 0.996: 100% 148/148 [00:35<00:00, 4.20it/s]\n",
+ " 4/5 1.73G 0.766 0.664 0.951 0.998: 100% 148/148 [00:36<00:00, 4.05it/s]\n",
+ " 5/5 1.73G 0.724 0.634 0.959 0.997: 100% 148/148 [00:37<00:00, 3.94it/s]\n",
+ "\n",
+ "Training complete (0.052 hours)\n",
+ "Results saved to \u001b[1mruns/train-cls/exp\u001b[0m\n",
+ "Predict: python classify/predict.py --weights runs/train-cls/exp/weights/best.pt --source im.jpg\n",
+ "Validate: python classify/val.py --weights runs/train-cls/exp/weights/best.pt --data /content/datasets/imagenette160\n",
+ "Export: python export.py --weights runs/train-cls/exp/weights/best.pt --include onnx\n",
+ "PyTorch Hub: model = torch.hub.load('ultralytics/yolov5', 'custom', 'runs/train-cls/exp/weights/best.pt')\n",
+ "Visualize: https://netron.app\n",
+ "\n"
+ ]
+ }
+ ],
+ "source": [
+ "# Train YOLOv5s Classification on Imagenette160 for 3 epochs\n",
+ "!python classify/train.py --model yolov5s-cls.pt --data imagenette160 --epochs 5 --img 224 --cache"
+ ]
+ },
+ {
+ "cell_type": "markdown",
+ "metadata": {
+ "id": "15glLzbQx5u0"
+ },
+ "source": [
+ "# 4. Visualize"
+ ]
+ },
+ {
+ "cell_type": "markdown",
+ "metadata": {
+ "id": "nWOsI5wJR1o3"
+ },
+ "source": [
+ "## Comet Logging and Visualization 🌟 NEW\n",
+ "\n",
+ "[Comet](https://www.comet.com/site/lp/yolov5-with-comet/?utm_source=yolov5&utm_medium=partner&utm_campaign=partner_yolov5_2022&utm_content=yolov5_colab) is now fully integrated with YOLOv5. Track and visualize model metrics in real time, save your hyperparameters, datasets, and model checkpoints, and visualize your model predictions with [Comet Custom Panels](https://www.comet.com/docs/v2/guides/comet-dashboard/code-panels/about-panels/?utm_source=yolov5&utm_medium=partner&utm_campaign=partner_yolov5_2022&utm_content=yolov5_colab)! Comet makes sure you never lose track of your work and makes it easy to share results and collaborate across teams of all sizes!\n",
+ "\n",
+ "Getting started is easy:\n",
+ "```shell\n",
+ "pip install comet_ml # 1. install\n",
+ "export COMET_API_KEY= # 2. paste API key\n",
+ "python train.py --img 640 --epochs 3 --data coco128.yaml --weights yolov5s.pt # 3. train\n",
+ "```\n",
+ "To learn more about all of the supported Comet features for this integration, check out the [Comet Tutorial](https://docs.ultralytics.com/yolov5/tutorials/comet_logging_integration). If you'd like to learn more about Comet, head over to our [documentation](https://www.comet.com/docs/v2/?utm_source=yolov5&utm_medium=partner&utm_campaign=partner_yolov5_2022&utm_content=yolov5_colab). Get started by trying out the Comet Colab Notebook:\n",
+ "[](https://colab.research.google.com/drive/1RG0WOQyxlDlo5Km8GogJpIEJlg_5lyYO?usp=sharing)\n",
+ "\n",
+ "\n",
+ ""
+ ]
+ },
+ {
+ "cell_type": "markdown",
+ "metadata": {
+ "id": "Lay2WsTjNJzP"
+ },
+ "source": [
+ "## ClearML Logging and Automation 🌟 NEW\n",
+ "\n",
+ "[ClearML](https://cutt.ly/yolov5-notebook-clearml) is completely integrated into YOLOv5 to track your experimentation, manage dataset versions and even remotely execute training runs. To enable ClearML (check cells above):\n",
+ "\n",
+ "- `pip install clearml`\n",
+ "- run `clearml-init` to connect to a ClearML server (**deploy your own [open-source server](https://github.com/allegroai/clearml-server)**, or use our [free hosted server](https://cutt.ly/yolov5-notebook-clearml))\n",
+ "\n",
+ "You'll get all the great expected features from an experiment manager: live updates, model upload, experiment comparison etc. but ClearML also tracks uncommitted changes and installed packages for example. Thanks to that ClearML Tasks (which is what we call experiments) are also reproducible on different machines! With only 1 extra line, we can schedule a YOLOv5 training task on a queue to be executed by any number of ClearML Agents (workers).\n",
+ "\n",
+ "You can use ClearML Data to version your dataset and then pass it to YOLOv5 simply using its unique ID. This will help you keep track of your data without adding extra hassle. Explore the [ClearML Tutorial](https://docs.ultralytics.com/yolov5/tutorials/clearml_logging_integration) for details!\n",
+ "\n",
+ "\n",
+ ""
+ ]
+ },
+ {
+ "cell_type": "markdown",
+ "metadata": {
+ "id": "-WPvRbS5Swl6"
+ },
+ "source": [
+ "## Local Logging\n",
+ "\n",
+ "Training results are automatically logged with [Tensorboard](https://www.tensorflow.org/tensorboard) and [CSV](https://github.com/ultralytics/yolov5/pull/4148) loggers to `runs/train`, with a new experiment directory created for each new training as `runs/train/exp2`, `runs/train/exp3`, etc.\n",
+ "\n",
+ "This directory contains train and val statistics, mosaics, labels, predictions and augmentated mosaics, as well as metrics and charts including precision-recall (PR) curves and confusion matrices. \n",
+ "\n",
+ "\n"
+ ]
+ },
+ {
+ "cell_type": "markdown",
+ "metadata": {
+ "id": "Zelyeqbyt3GD"
+ },
+ "source": [
+ "# Environments\n",
+ "\n",
+ "YOLOv5 may be run in any of the following up-to-date verified environments (with all dependencies including [CUDA](https://developer.nvidia.com/cuda)/[CUDNN](https://developer.nvidia.com/cudnn), [Python](https://www.python.org/) and [PyTorch](https://pytorch.org/) preinstalled):\n",
+ "\n",
+ "- **Notebooks** with free GPU: \n",
+ "- **Google Cloud** Deep Learning VM. See [GCP Quickstart Guide](https://docs.ultralytics.com/yolov5/environments/google_cloud_quickstart_tutorial/)\n",
+ "- **Amazon** Deep Learning AMI. See [AWS Quickstart Guide](https://docs.ultralytics.com/yolov5/environments/aws_quickstart_tutorial/)\n",
+ "- **Docker Image**. See [Docker Quickstart Guide](https://docs.ultralytics.com/yolov5/environments/docker_image_quickstart_tutorial/) \n"
+ ]
+ },
+ {
+ "cell_type": "markdown",
+ "metadata": {
+ "id": "6Qu7Iesl0p54"
+ },
+ "source": [
+ "# Status\n",
+ "\n",
+ "\n",
+ "\n",
+ "If this badge is green, all [YOLOv5 GitHub Actions](https://github.com/ultralytics/yolov5/actions) Continuous Integration (CI) tests are currently passing. CI tests verify correct operation of YOLOv5 training ([train.py](https://github.com/ultralytics/yolov5/blob/master/train.py)), testing ([val.py](https://github.com/ultralytics/yolov5/blob/master/val.py)), inference ([detect.py](https://github.com/ultralytics/yolov5/blob/master/detect.py)) and export ([export.py](https://github.com/ultralytics/yolov5/blob/master/export.py)) on macOS, Windows, and Ubuntu every 24 hours and on every commit.\n"
+ ]
+ },
+ {
+ "cell_type": "markdown",
+ "metadata": {
+ "id": "IEijrePND_2I"
+ },
+ "source": [
+ "# Appendix\n",
+ "\n",
+ "Additional content below."
+ ]
+ },
+ {
+ "cell_type": "code",
+ "execution_count": null,
+ "metadata": {
+ "id": "GMusP4OAxFu6"
+ },
+ "outputs": [],
+ "source": [
+ "# YOLOv5 PyTorch HUB Inference (DetectionModels only)\n",
+ "import torch\n",
+ "\n",
+ "model = torch.hub.load('ultralytics/yolov5', 'yolov5s') # yolov5n - yolov5x6 or custom\n",
+ "im = 'https://ultralytics.com/images/zidane.jpg' # file, Path, PIL.Image, OpenCV, nparray, list\n",
+ "results = model(im) # inference\n",
+ "results.print() # or .show(), .save(), .crop(), .pandas(), etc."
+ ]
+ }
+ ],
+ "metadata": {
+ "accelerator": "GPU",
+ "colab": {
+ "name": "YOLOv5 Classification Tutorial",
+ "provenance": []
+ },
+ "kernelspec": {
+ "display_name": "Python 3 (ipykernel)",
+ "language": "python",
+ "name": "python3"
+ },
+ "language_info": {
+ "codemirror_mode": {
+ "name": "ipython",
+ "version": 3
+ },
+ "file_extension": ".py",
+ "mimetype": "text/x-python",
+ "name": "python",
+ "nbconvert_exporter": "python",
+ "pygments_lexer": "ipython3",
+ "version": "3.7.12"
+ }
+ },
+ "nbformat": 4,
+ "nbformat_minor": 0
+}
diff --git a/yolov5/classify/val.py b/yolov5/classify/val.py
new file mode 100644
index 0000000000000000000000000000000000000000..643489d64d36cb4d445d3a74c04eed747619b933
--- /dev/null
+++ b/yolov5/classify/val.py
@@ -0,0 +1,170 @@
+# YOLOv5 🚀 by Ultralytics, AGPL-3.0 license
+"""
+Validate a trained YOLOv5 classification model on a classification dataset
+
+Usage:
+ $ bash data/scripts/get_imagenet.sh --val # download ImageNet val split (6.3G, 50000 images)
+ $ python classify/val.py --weights yolov5m-cls.pt --data ../datasets/imagenet --img 224 # validate ImageNet
+
+Usage - formats:
+ $ python classify/val.py --weights yolov5s-cls.pt # PyTorch
+ yolov5s-cls.torchscript # TorchScript
+ yolov5s-cls.onnx # ONNX Runtime or OpenCV DNN with --dnn
+ yolov5s-cls_openvino_model # OpenVINO
+ yolov5s-cls.engine # TensorRT
+ yolov5s-cls.mlmodel # CoreML (macOS-only)
+ yolov5s-cls_saved_model # TensorFlow SavedModel
+ yolov5s-cls.pb # TensorFlow GraphDef
+ yolov5s-cls.tflite # TensorFlow Lite
+ yolov5s-cls_edgetpu.tflite # TensorFlow Edge TPU
+ yolov5s-cls_paddle_model # PaddlePaddle
+"""
+
+import argparse
+import os
+import sys
+from pathlib import Path
+
+import torch
+from tqdm import tqdm
+
+FILE = Path(__file__).resolve()
+ROOT = FILE.parents[1] # YOLOv5 root directory
+if str(ROOT) not in sys.path:
+ sys.path.append(str(ROOT)) # add ROOT to PATH
+ROOT = Path(os.path.relpath(ROOT, Path.cwd())) # relative
+
+from models.common import DetectMultiBackend
+from utils.dataloaders import create_classification_dataloader
+from utils.general import (LOGGER, TQDM_BAR_FORMAT, Profile, check_img_size, check_requirements, colorstr,
+ increment_path, print_args)
+from utils.torch_utils import select_device, smart_inference_mode
+
+
+@smart_inference_mode()
+def run(
+ data=ROOT / '../datasets/mnist', # dataset dir
+ weights=ROOT / 'yolov5s-cls.pt', # model.pt path(s)
+ batch_size=128, # batch size
+ imgsz=224, # inference size (pixels)
+ device='', # cuda device, i.e. 0 or 0,1,2,3 or cpu
+ workers=8, # max dataloader workers (per RANK in DDP mode)
+ verbose=False, # verbose output
+ project=ROOT / 'runs/val-cls', # save to project/name
+ name='exp', # save to project/name
+ exist_ok=False, # existing project/name ok, do not increment
+ half=False, # use FP16 half-precision inference
+ dnn=False, # use OpenCV DNN for ONNX inference
+ model=None,
+ dataloader=None,
+ criterion=None,
+ pbar=None,
+):
+ # Initialize/load model and set device
+ training = model is not None
+ if training: # called by train.py
+ device, pt, jit, engine = next(model.parameters()).device, True, False, False # get model device, PyTorch model
+ half &= device.type != 'cpu' # half precision only supported on CUDA
+ model.half() if half else model.float()
+ else: # called directly
+ device = select_device(device, batch_size=batch_size)
+
+ # Directories
+ save_dir = increment_path(Path(project) / name, exist_ok=exist_ok) # increment run
+ save_dir.mkdir(parents=True, exist_ok=True) # make dir
+
+ # Load model
+ model = DetectMultiBackend(weights, device=device, dnn=dnn, fp16=half)
+ stride, pt, jit, engine = model.stride, model.pt, model.jit, model.engine
+ imgsz = check_img_size(imgsz, s=stride) # check image size
+ half = model.fp16 # FP16 supported on limited backends with CUDA
+ if engine:
+ batch_size = model.batch_size
+ else:
+ device = model.device
+ if not (pt or jit):
+ batch_size = 1 # export.py models default to batch-size 1
+ LOGGER.info(f'Forcing --batch-size 1 square inference (1,3,{imgsz},{imgsz}) for non-PyTorch models')
+
+ # Dataloader
+ data = Path(data)
+ test_dir = data / 'test' if (data / 'test').exists() else data / 'val' # data/test or data/val
+ dataloader = create_classification_dataloader(path=test_dir,
+ imgsz=imgsz,
+ batch_size=batch_size,
+ augment=False,
+ rank=-1,
+ workers=workers)
+
+ model.eval()
+ pred, targets, loss, dt = [], [], 0, (Profile(), Profile(), Profile())
+ n = len(dataloader) # number of batches
+ action = 'validating' if dataloader.dataset.root.stem == 'val' else 'testing'
+ desc = f'{pbar.desc[:-36]}{action:>36}' if pbar else f'{action}'
+ bar = tqdm(dataloader, desc, n, not training, bar_format=TQDM_BAR_FORMAT, position=0)
+ with torch.cuda.amp.autocast(enabled=device.type != 'cpu'):
+ for images, labels in bar:
+ with dt[0]:
+ images, labels = images.to(device, non_blocking=True), labels.to(device)
+
+ with dt[1]:
+ y = model(images)
+
+ with dt[2]:
+ pred.append(y.argsort(1, descending=True)[:, :5])
+ targets.append(labels)
+ if criterion:
+ loss += criterion(y, labels)
+
+ loss /= n
+ pred, targets = torch.cat(pred), torch.cat(targets)
+ correct = (targets[:, None] == pred).float()
+ acc = torch.stack((correct[:, 0], correct.max(1).values), dim=1) # (top1, top5) accuracy
+ top1, top5 = acc.mean(0).tolist()
+
+ if pbar:
+ pbar.desc = f'{pbar.desc[:-36]}{loss:>12.3g}{top1:>12.3g}{top5:>12.3g}'
+ if verbose: # all classes
+ LOGGER.info(f"{'Class':>24}{'Images':>12}{'top1_acc':>12}{'top5_acc':>12}")
+ LOGGER.info(f"{'all':>24}{targets.shape[0]:>12}{top1:>12.3g}{top5:>12.3g}")
+ for i, c in model.names.items():
+ acc_i = acc[targets == i]
+ top1i, top5i = acc_i.mean(0).tolist()
+ LOGGER.info(f'{c:>24}{acc_i.shape[0]:>12}{top1i:>12.3g}{top5i:>12.3g}')
+
+ # Print results
+ t = tuple(x.t / len(dataloader.dataset.samples) * 1E3 for x in dt) # speeds per image
+ shape = (1, 3, imgsz, imgsz)
+ LOGGER.info(f'Speed: %.1fms pre-process, %.1fms inference, %.1fms post-process per image at shape {shape}' % t)
+ LOGGER.info(f"Results saved to {colorstr('bold', save_dir)}")
+
+ return top1, top5, loss
+
+
+def parse_opt():
+ parser = argparse.ArgumentParser()
+ parser.add_argument('--data', type=str, default=ROOT / '../datasets/mnist', help='dataset path')
+ parser.add_argument('--weights', nargs='+', type=str, default=ROOT / 'yolov5s-cls.pt', help='model.pt path(s)')
+ parser.add_argument('--batch-size', type=int, default=128, help='batch size')
+ parser.add_argument('--imgsz', '--img', '--img-size', type=int, default=224, help='inference size (pixels)')
+ parser.add_argument('--device', default='', help='cuda device, i.e. 0 or 0,1,2,3 or cpu')
+ parser.add_argument('--workers', type=int, default=8, help='max dataloader workers (per RANK in DDP mode)')
+ parser.add_argument('--verbose', nargs='?', const=True, default=True, help='verbose output')
+ parser.add_argument('--project', default=ROOT / 'runs/val-cls', help='save to project/name')
+ parser.add_argument('--name', default='exp', help='save to project/name')
+ parser.add_argument('--exist-ok', action='store_true', help='existing project/name ok, do not increment')
+ parser.add_argument('--half', action='store_true', help='use FP16 half-precision inference')
+ parser.add_argument('--dnn', action='store_true', help='use OpenCV DNN for ONNX inference')
+ opt = parser.parse_args()
+ print_args(vars(opt))
+ return opt
+
+
+def main(opt):
+ check_requirements(exclude=('tensorboard', 'thop'))
+ run(**vars(opt))
+
+
+if __name__ == '__main__':
+ opt = parse_opt()
+ main(opt)
diff --git a/yolov5/data/Argoverse.yaml b/yolov5/data/Argoverse.yaml
new file mode 100644
index 0000000000000000000000000000000000000000..8a65407a633371210feed2779f1476186b9185bc
--- /dev/null
+++ b/yolov5/data/Argoverse.yaml
@@ -0,0 +1,74 @@
+# YOLOv5 🚀 by Ultralytics, AGPL-3.0 license
+# Argoverse-HD dataset (ring-front-center camera) http://www.cs.cmu.edu/~mengtial/proj/streaming/ by Argo AI
+# Example usage: python train.py --data Argoverse.yaml
+# parent
+# ├── yolov5
+# └── datasets
+# └── Argoverse ← downloads here (31.3 GB)
+
+
+# Train/val/test sets as 1) dir: path/to/imgs, 2) file: path/to/imgs.txt, or 3) list: [path/to/imgs1, path/to/imgs2, ..]
+path: ../datasets/Argoverse # dataset root dir
+train: Argoverse-1.1/images/train/ # train images (relative to 'path') 39384 images
+val: Argoverse-1.1/images/val/ # val images (relative to 'path') 15062 images
+test: Argoverse-1.1/images/test/ # test images (optional) https://eval.ai/web/challenges/challenge-page/800/overview
+
+# Classes
+names:
+ 0: person
+ 1: bicycle
+ 2: car
+ 3: motorcycle
+ 4: bus
+ 5: truck
+ 6: traffic_light
+ 7: stop_sign
+
+
+# Download script/URL (optional) ---------------------------------------------------------------------------------------
+download: |
+ import json
+
+ from tqdm import tqdm
+ from utils.general import download, Path
+
+
+ def argoverse2yolo(set):
+ labels = {}
+ a = json.load(open(set, "rb"))
+ for annot in tqdm(a['annotations'], desc=f"Converting {set} to YOLOv5 format..."):
+ img_id = annot['image_id']
+ img_name = a['images'][img_id]['name']
+ img_label_name = f'{img_name[:-3]}txt'
+
+ cls = annot['category_id'] # instance class id
+ x_center, y_center, width, height = annot['bbox']
+ x_center = (x_center + width / 2) / 1920.0 # offset and scale
+ y_center = (y_center + height / 2) / 1200.0 # offset and scale
+ width /= 1920.0 # scale
+ height /= 1200.0 # scale
+
+ img_dir = set.parents[2] / 'Argoverse-1.1' / 'labels' / a['seq_dirs'][a['images'][annot['image_id']]['sid']]
+ if not img_dir.exists():
+ img_dir.mkdir(parents=True, exist_ok=True)
+
+ k = str(img_dir / img_label_name)
+ if k not in labels:
+ labels[k] = []
+ labels[k].append(f"{cls} {x_center} {y_center} {width} {height}\n")
+
+ for k in labels:
+ with open(k, "w") as f:
+ f.writelines(labels[k])
+
+
+ # Download
+ dir = Path(yaml['path']) # dataset root dir
+ urls = ['https://argoverse-hd.s3.us-east-2.amazonaws.com/Argoverse-HD-Full.zip']
+ download(urls, dir=dir, delete=False)
+
+ # Convert
+ annotations_dir = 'Argoverse-HD/annotations/'
+ (dir / 'Argoverse-1.1' / 'tracking').rename(dir / 'Argoverse-1.1' / 'images') # rename 'tracking' to 'images'
+ for d in "train.json", "val.json":
+ argoverse2yolo(dir / annotations_dir / d) # convert VisDrone annotations to YOLO labels
diff --git a/yolov5/data/FLIR.yaml b/yolov5/data/FLIR.yaml
new file mode 100644
index 0000000000000000000000000000000000000000..89da77a742da141897bc21b26d31e2a368912de4
--- /dev/null
+++ b/yolov5/data/FLIR.yaml
@@ -0,0 +1,28 @@
+train: ../VOCdevkit/images/train/ # 8493 images 训练集
+val: ../VOCdevkit/images/val/ # 2249 images 验证集
+
+
+# number of classes 类别数
+nc: 15
+
+
+# Classes
+names:
+ 0: person
+ 1: bike
+ 2: car
+ 3: motor
+ 4: bus
+ 5: train
+ 6: truck
+ 7: light
+ 8: hydrant
+ 9: sign
+ 10: dog
+ 11: skateboard
+ 12: stroller
+ 13: scooter
+ 14: other vehicle
+
+
+
diff --git a/yolov5/data/GlobalWheat2020.yaml b/yolov5/data/GlobalWheat2020.yaml
new file mode 100644
index 0000000000000000000000000000000000000000..7b02ac95dd95d1b8fc466b268775f33b5f60ce88
--- /dev/null
+++ b/yolov5/data/GlobalWheat2020.yaml
@@ -0,0 +1,54 @@
+# YOLOv5 🚀 by Ultralytics, AGPL-3.0 license
+# Global Wheat 2020 dataset http://www.global-wheat.com/ by University of Saskatchewan
+# Example usage: python train.py --data GlobalWheat2020.yaml
+# parent
+# ├── yolov5
+# └── datasets
+# └── GlobalWheat2020 ← downloads here (7.0 GB)
+
+
+# Train/val/test sets as 1) dir: path/to/imgs, 2) file: path/to/imgs.txt, or 3) list: [path/to/imgs1, path/to/imgs2, ..]
+path: ../datasets/GlobalWheat2020 # dataset root dir
+train: # train images (relative to 'path') 3422 images
+ - images/arvalis_1
+ - images/arvalis_2
+ - images/arvalis_3
+ - images/ethz_1
+ - images/rres_1
+ - images/inrae_1
+ - images/usask_1
+val: # val images (relative to 'path') 748 images (WARNING: train set contains ethz_1)
+ - images/ethz_1
+test: # test images (optional) 1276 images
+ - images/utokyo_1
+ - images/utokyo_2
+ - images/nau_1
+ - images/uq_1
+
+# Classes
+names:
+ 0: wheat_head
+
+
+# Download script/URL (optional) ---------------------------------------------------------------------------------------
+download: |
+ from utils.general import download, Path
+
+
+ # Download
+ dir = Path(yaml['path']) # dataset root dir
+ urls = ['https://zenodo.org/record/4298502/files/global-wheat-codalab-official.zip',
+ 'https://github.com/ultralytics/yolov5/releases/download/v1.0/GlobalWheat2020_labels.zip']
+ download(urls, dir=dir)
+
+ # Make Directories
+ for p in 'annotations', 'images', 'labels':
+ (dir / p).mkdir(parents=True, exist_ok=True)
+
+ # Move
+ for p in 'arvalis_1', 'arvalis_2', 'arvalis_3', 'ethz_1', 'rres_1', 'inrae_1', 'usask_1', \
+ 'utokyo_1', 'utokyo_2', 'nau_1', 'uq_1':
+ (dir / p).rename(dir / 'images' / p) # move to /images
+ f = (dir / p).with_suffix('.json') # json file
+ if f.exists():
+ f.rename((dir / 'annotations' / p).with_suffix('.json')) # move to /annotations
diff --git a/yolov5/data/ImageNet.yaml b/yolov5/data/ImageNet.yaml
new file mode 100644
index 0000000000000000000000000000000000000000..5fdcb63f89a5c4a0e4a579fc0b3bf995e8777e87
--- /dev/null
+++ b/yolov5/data/ImageNet.yaml
@@ -0,0 +1,1022 @@
+# YOLOv5 🚀 by Ultralytics, AGPL-3.0 license
+# ImageNet-1k dataset https://www.image-net.org/index.php by Stanford University
+# Simplified class names from https://github.com/anishathalye/imagenet-simple-labels
+# Example usage: python classify/train.py --data imagenet
+# parent
+# ├── yolov5
+# └── datasets
+# └── imagenet ← downloads here (144 GB)
+
+
+# Train/val/test sets as 1) dir: path/to/imgs, 2) file: path/to/imgs.txt, or 3) list: [path/to/imgs1, path/to/imgs2, ..]
+path: ../datasets/imagenet # dataset root dir
+train: train # train images (relative to 'path') 1281167 images
+val: val # val images (relative to 'path') 50000 images
+test: # test images (optional)
+
+# Classes
+names:
+ 0: tench
+ 1: goldfish
+ 2: great white shark
+ 3: tiger shark
+ 4: hammerhead shark
+ 5: electric ray
+ 6: stingray
+ 7: cock
+ 8: hen
+ 9: ostrich
+ 10: brambling
+ 11: goldfinch
+ 12: house finch
+ 13: junco
+ 14: indigo bunting
+ 15: American robin
+ 16: bulbul
+ 17: jay
+ 18: magpie
+ 19: chickadee
+ 20: American dipper
+ 21: kite
+ 22: bald eagle
+ 23: vulture
+ 24: great grey owl
+ 25: fire salamander
+ 26: smooth newt
+ 27: newt
+ 28: spotted salamander
+ 29: axolotl
+ 30: American bullfrog
+ 31: tree frog
+ 32: tailed frog
+ 33: loggerhead sea turtle
+ 34: leatherback sea turtle
+ 35: mud turtle
+ 36: terrapin
+ 37: box turtle
+ 38: banded gecko
+ 39: green iguana
+ 40: Carolina anole
+ 41: desert grassland whiptail lizard
+ 42: agama
+ 43: frilled-necked lizard
+ 44: alligator lizard
+ 45: Gila monster
+ 46: European green lizard
+ 47: chameleon
+ 48: Komodo dragon
+ 49: Nile crocodile
+ 50: American alligator
+ 51: triceratops
+ 52: worm snake
+ 53: ring-necked snake
+ 54: eastern hog-nosed snake
+ 55: smooth green snake
+ 56: kingsnake
+ 57: garter snake
+ 58: water snake
+ 59: vine snake
+ 60: night snake
+ 61: boa constrictor
+ 62: African rock python
+ 63: Indian cobra
+ 64: green mamba
+ 65: sea snake
+ 66: Saharan horned viper
+ 67: eastern diamondback rattlesnake
+ 68: sidewinder
+ 69: trilobite
+ 70: harvestman
+ 71: scorpion
+ 72: yellow garden spider
+ 73: barn spider
+ 74: European garden spider
+ 75: southern black widow
+ 76: tarantula
+ 77: wolf spider
+ 78: tick
+ 79: centipede
+ 80: black grouse
+ 81: ptarmigan
+ 82: ruffed grouse
+ 83: prairie grouse
+ 84: peacock
+ 85: quail
+ 86: partridge
+ 87: grey parrot
+ 88: macaw
+ 89: sulphur-crested cockatoo
+ 90: lorikeet
+ 91: coucal
+ 92: bee eater
+ 93: hornbill
+ 94: hummingbird
+ 95: jacamar
+ 96: toucan
+ 97: duck
+ 98: red-breasted merganser
+ 99: goose
+ 100: black swan
+ 101: tusker
+ 102: echidna
+ 103: platypus
+ 104: wallaby
+ 105: koala
+ 106: wombat
+ 107: jellyfish
+ 108: sea anemone
+ 109: brain coral
+ 110: flatworm
+ 111: nematode
+ 112: conch
+ 113: snail
+ 114: slug
+ 115: sea slug
+ 116: chiton
+ 117: chambered nautilus
+ 118: Dungeness crab
+ 119: rock crab
+ 120: fiddler crab
+ 121: red king crab
+ 122: American lobster
+ 123: spiny lobster
+ 124: crayfish
+ 125: hermit crab
+ 126: isopod
+ 127: white stork
+ 128: black stork
+ 129: spoonbill
+ 130: flamingo
+ 131: little blue heron
+ 132: great egret
+ 133: bittern
+ 134: crane (bird)
+ 135: limpkin
+ 136: common gallinule
+ 137: American coot
+ 138: bustard
+ 139: ruddy turnstone
+ 140: dunlin
+ 141: common redshank
+ 142: dowitcher
+ 143: oystercatcher
+ 144: pelican
+ 145: king penguin
+ 146: albatross
+ 147: grey whale
+ 148: killer whale
+ 149: dugong
+ 150: sea lion
+ 151: Chihuahua
+ 152: Japanese Chin
+ 153: Maltese
+ 154: Pekingese
+ 155: Shih Tzu
+ 156: King Charles Spaniel
+ 157: Papillon
+ 158: toy terrier
+ 159: Rhodesian Ridgeback
+ 160: Afghan Hound
+ 161: Basset Hound
+ 162: Beagle
+ 163: Bloodhound
+ 164: Bluetick Coonhound
+ 165: Black and Tan Coonhound
+ 166: Treeing Walker Coonhound
+ 167: English foxhound
+ 168: Redbone Coonhound
+ 169: borzoi
+ 170: Irish Wolfhound
+ 171: Italian Greyhound
+ 172: Whippet
+ 173: Ibizan Hound
+ 174: Norwegian Elkhound
+ 175: Otterhound
+ 176: Saluki
+ 177: Scottish Deerhound
+ 178: Weimaraner
+ 179: Staffordshire Bull Terrier
+ 180: American Staffordshire Terrier
+ 181: Bedlington Terrier
+ 182: Border Terrier
+ 183: Kerry Blue Terrier
+ 184: Irish Terrier
+ 185: Norfolk Terrier
+ 186: Norwich Terrier
+ 187: Yorkshire Terrier
+ 188: Wire Fox Terrier
+ 189: Lakeland Terrier
+ 190: Sealyham Terrier
+ 191: Airedale Terrier
+ 192: Cairn Terrier
+ 193: Australian Terrier
+ 194: Dandie Dinmont Terrier
+ 195: Boston Terrier
+ 196: Miniature Schnauzer
+ 197: Giant Schnauzer
+ 198: Standard Schnauzer
+ 199: Scottish Terrier
+ 200: Tibetan Terrier
+ 201: Australian Silky Terrier
+ 202: Soft-coated Wheaten Terrier
+ 203: West Highland White Terrier
+ 204: Lhasa Apso
+ 205: Flat-Coated Retriever
+ 206: Curly-coated Retriever
+ 207: Golden Retriever
+ 208: Labrador Retriever
+ 209: Chesapeake Bay Retriever
+ 210: German Shorthaired Pointer
+ 211: Vizsla
+ 212: English Setter
+ 213: Irish Setter
+ 214: Gordon Setter
+ 215: Brittany
+ 216: Clumber Spaniel
+ 217: English Springer Spaniel
+ 218: Welsh Springer Spaniel
+ 219: Cocker Spaniels
+ 220: Sussex Spaniel
+ 221: Irish Water Spaniel
+ 222: Kuvasz
+ 223: Schipperke
+ 224: Groenendael
+ 225: Malinois
+ 226: Briard
+ 227: Australian Kelpie
+ 228: Komondor
+ 229: Old English Sheepdog
+ 230: Shetland Sheepdog
+ 231: collie
+ 232: Border Collie
+ 233: Bouvier des Flandres
+ 234: Rottweiler
+ 235: German Shepherd Dog
+ 236: Dobermann
+ 237: Miniature Pinscher
+ 238: Greater Swiss Mountain Dog
+ 239: Bernese Mountain Dog
+ 240: Appenzeller Sennenhund
+ 241: Entlebucher Sennenhund
+ 242: Boxer
+ 243: Bullmastiff
+ 244: Tibetan Mastiff
+ 245: French Bulldog
+ 246: Great Dane
+ 247: St. Bernard
+ 248: husky
+ 249: Alaskan Malamute
+ 250: Siberian Husky
+ 251: Dalmatian
+ 252: Affenpinscher
+ 253: Basenji
+ 254: pug
+ 255: Leonberger
+ 256: Newfoundland
+ 257: Pyrenean Mountain Dog
+ 258: Samoyed
+ 259: Pomeranian
+ 260: Chow Chow
+ 261: Keeshond
+ 262: Griffon Bruxellois
+ 263: Pembroke Welsh Corgi
+ 264: Cardigan Welsh Corgi
+ 265: Toy Poodle
+ 266: Miniature Poodle
+ 267: Standard Poodle
+ 268: Mexican hairless dog
+ 269: grey wolf
+ 270: Alaskan tundra wolf
+ 271: red wolf
+ 272: coyote
+ 273: dingo
+ 274: dhole
+ 275: African wild dog
+ 276: hyena
+ 277: red fox
+ 278: kit fox
+ 279: Arctic fox
+ 280: grey fox
+ 281: tabby cat
+ 282: tiger cat
+ 283: Persian cat
+ 284: Siamese cat
+ 285: Egyptian Mau
+ 286: cougar
+ 287: lynx
+ 288: leopard
+ 289: snow leopard
+ 290: jaguar
+ 291: lion
+ 292: tiger
+ 293: cheetah
+ 294: brown bear
+ 295: American black bear
+ 296: polar bear
+ 297: sloth bear
+ 298: mongoose
+ 299: meerkat
+ 300: tiger beetle
+ 301: ladybug
+ 302: ground beetle
+ 303: longhorn beetle
+ 304: leaf beetle
+ 305: dung beetle
+ 306: rhinoceros beetle
+ 307: weevil
+ 308: fly
+ 309: bee
+ 310: ant
+ 311: grasshopper
+ 312: cricket
+ 313: stick insect
+ 314: cockroach
+ 315: mantis
+ 316: cicada
+ 317: leafhopper
+ 318: lacewing
+ 319: dragonfly
+ 320: damselfly
+ 321: red admiral
+ 322: ringlet
+ 323: monarch butterfly
+ 324: small white
+ 325: sulphur butterfly
+ 326: gossamer-winged butterfly
+ 327: starfish
+ 328: sea urchin
+ 329: sea cucumber
+ 330: cottontail rabbit
+ 331: hare
+ 332: Angora rabbit
+ 333: hamster
+ 334: porcupine
+ 335: fox squirrel
+ 336: marmot
+ 337: beaver
+ 338: guinea pig
+ 339: common sorrel
+ 340: zebra
+ 341: pig
+ 342: wild boar
+ 343: warthog
+ 344: hippopotamus
+ 345: ox
+ 346: water buffalo
+ 347: bison
+ 348: ram
+ 349: bighorn sheep
+ 350: Alpine ibex
+ 351: hartebeest
+ 352: impala
+ 353: gazelle
+ 354: dromedary
+ 355: llama
+ 356: weasel
+ 357: mink
+ 358: European polecat
+ 359: black-footed ferret
+ 360: otter
+ 361: skunk
+ 362: badger
+ 363: armadillo
+ 364: three-toed sloth
+ 365: orangutan
+ 366: gorilla
+ 367: chimpanzee
+ 368: gibbon
+ 369: siamang
+ 370: guenon
+ 371: patas monkey
+ 372: baboon
+ 373: macaque
+ 374: langur
+ 375: black-and-white colobus
+ 376: proboscis monkey
+ 377: marmoset
+ 378: white-headed capuchin
+ 379: howler monkey
+ 380: titi
+ 381: Geoffroy's spider monkey
+ 382: common squirrel monkey
+ 383: ring-tailed lemur
+ 384: indri
+ 385: Asian elephant
+ 386: African bush elephant
+ 387: red panda
+ 388: giant panda
+ 389: snoek
+ 390: eel
+ 391: coho salmon
+ 392: rock beauty
+ 393: clownfish
+ 394: sturgeon
+ 395: garfish
+ 396: lionfish
+ 397: pufferfish
+ 398: abacus
+ 399: abaya
+ 400: academic gown
+ 401: accordion
+ 402: acoustic guitar
+ 403: aircraft carrier
+ 404: airliner
+ 405: airship
+ 406: altar
+ 407: ambulance
+ 408: amphibious vehicle
+ 409: analog clock
+ 410: apiary
+ 411: apron
+ 412: waste container
+ 413: assault rifle
+ 414: backpack
+ 415: bakery
+ 416: balance beam
+ 417: balloon
+ 418: ballpoint pen
+ 419: Band-Aid
+ 420: banjo
+ 421: baluster
+ 422: barbell
+ 423: barber chair
+ 424: barbershop
+ 425: barn
+ 426: barometer
+ 427: barrel
+ 428: wheelbarrow
+ 429: baseball
+ 430: basketball
+ 431: bassinet
+ 432: bassoon
+ 433: swimming cap
+ 434: bath towel
+ 435: bathtub
+ 436: station wagon
+ 437: lighthouse
+ 438: beaker
+ 439: military cap
+ 440: beer bottle
+ 441: beer glass
+ 442: bell-cot
+ 443: bib
+ 444: tandem bicycle
+ 445: bikini
+ 446: ring binder
+ 447: binoculars
+ 448: birdhouse
+ 449: boathouse
+ 450: bobsleigh
+ 451: bolo tie
+ 452: poke bonnet
+ 453: bookcase
+ 454: bookstore
+ 455: bottle cap
+ 456: bow
+ 457: bow tie
+ 458: brass
+ 459: bra
+ 460: breakwater
+ 461: breastplate
+ 462: broom
+ 463: bucket
+ 464: buckle
+ 465: bulletproof vest
+ 466: high-speed train
+ 467: butcher shop
+ 468: taxicab
+ 469: cauldron
+ 470: candle
+ 471: cannon
+ 472: canoe
+ 473: can opener
+ 474: cardigan
+ 475: car mirror
+ 476: carousel
+ 477: tool kit
+ 478: carton
+ 479: car wheel
+ 480: automated teller machine
+ 481: cassette
+ 482: cassette player
+ 483: castle
+ 484: catamaran
+ 485: CD player
+ 486: cello
+ 487: mobile phone
+ 488: chain
+ 489: chain-link fence
+ 490: chain mail
+ 491: chainsaw
+ 492: chest
+ 493: chiffonier
+ 494: chime
+ 495: china cabinet
+ 496: Christmas stocking
+ 497: church
+ 498: movie theater
+ 499: cleaver
+ 500: cliff dwelling
+ 501: cloak
+ 502: clogs
+ 503: cocktail shaker
+ 504: coffee mug
+ 505: coffeemaker
+ 506: coil
+ 507: combination lock
+ 508: computer keyboard
+ 509: confectionery store
+ 510: container ship
+ 511: convertible
+ 512: corkscrew
+ 513: cornet
+ 514: cowboy boot
+ 515: cowboy hat
+ 516: cradle
+ 517: crane (machine)
+ 518: crash helmet
+ 519: crate
+ 520: infant bed
+ 521: Crock Pot
+ 522: croquet ball
+ 523: crutch
+ 524: cuirass
+ 525: dam
+ 526: desk
+ 527: desktop computer
+ 528: rotary dial telephone
+ 529: diaper
+ 530: digital clock
+ 531: digital watch
+ 532: dining table
+ 533: dishcloth
+ 534: dishwasher
+ 535: disc brake
+ 536: dock
+ 537: dog sled
+ 538: dome
+ 539: doormat
+ 540: drilling rig
+ 541: drum
+ 542: drumstick
+ 543: dumbbell
+ 544: Dutch oven
+ 545: electric fan
+ 546: electric guitar
+ 547: electric locomotive
+ 548: entertainment center
+ 549: envelope
+ 550: espresso machine
+ 551: face powder
+ 552: feather boa
+ 553: filing cabinet
+ 554: fireboat
+ 555: fire engine
+ 556: fire screen sheet
+ 557: flagpole
+ 558: flute
+ 559: folding chair
+ 560: football helmet
+ 561: forklift
+ 562: fountain
+ 563: fountain pen
+ 564: four-poster bed
+ 565: freight car
+ 566: French horn
+ 567: frying pan
+ 568: fur coat
+ 569: garbage truck
+ 570: gas mask
+ 571: gas pump
+ 572: goblet
+ 573: go-kart
+ 574: golf ball
+ 575: golf cart
+ 576: gondola
+ 577: gong
+ 578: gown
+ 579: grand piano
+ 580: greenhouse
+ 581: grille
+ 582: grocery store
+ 583: guillotine
+ 584: barrette
+ 585: hair spray
+ 586: half-track
+ 587: hammer
+ 588: hamper
+ 589: hair dryer
+ 590: hand-held computer
+ 591: handkerchief
+ 592: hard disk drive
+ 593: harmonica
+ 594: harp
+ 595: harvester
+ 596: hatchet
+ 597: holster
+ 598: home theater
+ 599: honeycomb
+ 600: hook
+ 601: hoop skirt
+ 602: horizontal bar
+ 603: horse-drawn vehicle
+ 604: hourglass
+ 605: iPod
+ 606: clothes iron
+ 607: jack-o'-lantern
+ 608: jeans
+ 609: jeep
+ 610: T-shirt
+ 611: jigsaw puzzle
+ 612: pulled rickshaw
+ 613: joystick
+ 614: kimono
+ 615: knee pad
+ 616: knot
+ 617: lab coat
+ 618: ladle
+ 619: lampshade
+ 620: laptop computer
+ 621: lawn mower
+ 622: lens cap
+ 623: paper knife
+ 624: library
+ 625: lifeboat
+ 626: lighter
+ 627: limousine
+ 628: ocean liner
+ 629: lipstick
+ 630: slip-on shoe
+ 631: lotion
+ 632: speaker
+ 633: loupe
+ 634: sawmill
+ 635: magnetic compass
+ 636: mail bag
+ 637: mailbox
+ 638: tights
+ 639: tank suit
+ 640: manhole cover
+ 641: maraca
+ 642: marimba
+ 643: mask
+ 644: match
+ 645: maypole
+ 646: maze
+ 647: measuring cup
+ 648: medicine chest
+ 649: megalith
+ 650: microphone
+ 651: microwave oven
+ 652: military uniform
+ 653: milk can
+ 654: minibus
+ 655: miniskirt
+ 656: minivan
+ 657: missile
+ 658: mitten
+ 659: mixing bowl
+ 660: mobile home
+ 661: Model T
+ 662: modem
+ 663: monastery
+ 664: monitor
+ 665: moped
+ 666: mortar
+ 667: square academic cap
+ 668: mosque
+ 669: mosquito net
+ 670: scooter
+ 671: mountain bike
+ 672: tent
+ 673: computer mouse
+ 674: mousetrap
+ 675: moving van
+ 676: muzzle
+ 677: nail
+ 678: neck brace
+ 679: necklace
+ 680: nipple
+ 681: notebook computer
+ 682: obelisk
+ 683: oboe
+ 684: ocarina
+ 685: odometer
+ 686: oil filter
+ 687: organ
+ 688: oscilloscope
+ 689: overskirt
+ 690: bullock cart
+ 691: oxygen mask
+ 692: packet
+ 693: paddle
+ 694: paddle wheel
+ 695: padlock
+ 696: paintbrush
+ 697: pajamas
+ 698: palace
+ 699: pan flute
+ 700: paper towel
+ 701: parachute
+ 702: parallel bars
+ 703: park bench
+ 704: parking meter
+ 705: passenger car
+ 706: patio
+ 707: payphone
+ 708: pedestal
+ 709: pencil case
+ 710: pencil sharpener
+ 711: perfume
+ 712: Petri dish
+ 713: photocopier
+ 714: plectrum
+ 715: Pickelhaube
+ 716: picket fence
+ 717: pickup truck
+ 718: pier
+ 719: piggy bank
+ 720: pill bottle
+ 721: pillow
+ 722: ping-pong ball
+ 723: pinwheel
+ 724: pirate ship
+ 725: pitcher
+ 726: hand plane
+ 727: planetarium
+ 728: plastic bag
+ 729: plate rack
+ 730: plow
+ 731: plunger
+ 732: Polaroid camera
+ 733: pole
+ 734: police van
+ 735: poncho
+ 736: billiard table
+ 737: soda bottle
+ 738: pot
+ 739: potter's wheel
+ 740: power drill
+ 741: prayer rug
+ 742: printer
+ 743: prison
+ 744: projectile
+ 745: projector
+ 746: hockey puck
+ 747: punching bag
+ 748: purse
+ 749: quill
+ 750: quilt
+ 751: race car
+ 752: racket
+ 753: radiator
+ 754: radio
+ 755: radio telescope
+ 756: rain barrel
+ 757: recreational vehicle
+ 758: reel
+ 759: reflex camera
+ 760: refrigerator
+ 761: remote control
+ 762: restaurant
+ 763: revolver
+ 764: rifle
+ 765: rocking chair
+ 766: rotisserie
+ 767: eraser
+ 768: rugby ball
+ 769: ruler
+ 770: running shoe
+ 771: safe
+ 772: safety pin
+ 773: salt shaker
+ 774: sandal
+ 775: sarong
+ 776: saxophone
+ 777: scabbard
+ 778: weighing scale
+ 779: school bus
+ 780: schooner
+ 781: scoreboard
+ 782: CRT screen
+ 783: screw
+ 784: screwdriver
+ 785: seat belt
+ 786: sewing machine
+ 787: shield
+ 788: shoe store
+ 789: shoji
+ 790: shopping basket
+ 791: shopping cart
+ 792: shovel
+ 793: shower cap
+ 794: shower curtain
+ 795: ski
+ 796: ski mask
+ 797: sleeping bag
+ 798: slide rule
+ 799: sliding door
+ 800: slot machine
+ 801: snorkel
+ 802: snowmobile
+ 803: snowplow
+ 804: soap dispenser
+ 805: soccer ball
+ 806: sock
+ 807: solar thermal collector
+ 808: sombrero
+ 809: soup bowl
+ 810: space bar
+ 811: space heater
+ 812: space shuttle
+ 813: spatula
+ 814: motorboat
+ 815: spider web
+ 816: spindle
+ 817: sports car
+ 818: spotlight
+ 819: stage
+ 820: steam locomotive
+ 821: through arch bridge
+ 822: steel drum
+ 823: stethoscope
+ 824: scarf
+ 825: stone wall
+ 826: stopwatch
+ 827: stove
+ 828: strainer
+ 829: tram
+ 830: stretcher
+ 831: couch
+ 832: stupa
+ 833: submarine
+ 834: suit
+ 835: sundial
+ 836: sunglass
+ 837: sunglasses
+ 838: sunscreen
+ 839: suspension bridge
+ 840: mop
+ 841: sweatshirt
+ 842: swimsuit
+ 843: swing
+ 844: switch
+ 845: syringe
+ 846: table lamp
+ 847: tank
+ 848: tape player
+ 849: teapot
+ 850: teddy bear
+ 851: television
+ 852: tennis ball
+ 853: thatched roof
+ 854: front curtain
+ 855: thimble
+ 856: threshing machine
+ 857: throne
+ 858: tile roof
+ 859: toaster
+ 860: tobacco shop
+ 861: toilet seat
+ 862: torch
+ 863: totem pole
+ 864: tow truck
+ 865: toy store
+ 866: tractor
+ 867: semi-trailer truck
+ 868: tray
+ 869: trench coat
+ 870: tricycle
+ 871: trimaran
+ 872: tripod
+ 873: triumphal arch
+ 874: trolleybus
+ 875: trombone
+ 876: tub
+ 877: turnstile
+ 878: typewriter keyboard
+ 879: umbrella
+ 880: unicycle
+ 881: upright piano
+ 882: vacuum cleaner
+ 883: vase
+ 884: vault
+ 885: velvet
+ 886: vending machine
+ 887: vestment
+ 888: viaduct
+ 889: violin
+ 890: volleyball
+ 891: waffle iron
+ 892: wall clock
+ 893: wallet
+ 894: wardrobe
+ 895: military aircraft
+ 896: sink
+ 897: washing machine
+ 898: water bottle
+ 899: water jug
+ 900: water tower
+ 901: whiskey jug
+ 902: whistle
+ 903: wig
+ 904: window screen
+ 905: window shade
+ 906: Windsor tie
+ 907: wine bottle
+ 908: wing
+ 909: wok
+ 910: wooden spoon
+ 911: wool
+ 912: split-rail fence
+ 913: shipwreck
+ 914: yawl
+ 915: yurt
+ 916: website
+ 917: comic book
+ 918: crossword
+ 919: traffic sign
+ 920: traffic light
+ 921: dust jacket
+ 922: menu
+ 923: plate
+ 924: guacamole
+ 925: consomme
+ 926: hot pot
+ 927: trifle
+ 928: ice cream
+ 929: ice pop
+ 930: baguette
+ 931: bagel
+ 932: pretzel
+ 933: cheeseburger
+ 934: hot dog
+ 935: mashed potato
+ 936: cabbage
+ 937: broccoli
+ 938: cauliflower
+ 939: zucchini
+ 940: spaghetti squash
+ 941: acorn squash
+ 942: butternut squash
+ 943: cucumber
+ 944: artichoke
+ 945: bell pepper
+ 946: cardoon
+ 947: mushroom
+ 948: Granny Smith
+ 949: strawberry
+ 950: orange
+ 951: lemon
+ 952: fig
+ 953: pineapple
+ 954: banana
+ 955: jackfruit
+ 956: custard apple
+ 957: pomegranate
+ 958: hay
+ 959: carbonara
+ 960: chocolate syrup
+ 961: dough
+ 962: meatloaf
+ 963: pizza
+ 964: pot pie
+ 965: burrito
+ 966: red wine
+ 967: espresso
+ 968: cup
+ 969: eggnog
+ 970: alp
+ 971: bubble
+ 972: cliff
+ 973: coral reef
+ 974: geyser
+ 975: lakeshore
+ 976: promontory
+ 977: shoal
+ 978: seashore
+ 979: valley
+ 980: volcano
+ 981: baseball player
+ 982: bridegroom
+ 983: scuba diver
+ 984: rapeseed
+ 985: daisy
+ 986: yellow lady's slipper
+ 987: corn
+ 988: acorn
+ 989: rose hip
+ 990: horse chestnut seed
+ 991: coral fungus
+ 992: agaric
+ 993: gyromitra
+ 994: stinkhorn mushroom
+ 995: earth star
+ 996: hen-of-the-woods
+ 997: bolete
+ 998: ear
+ 999: toilet paper
+
+
+# Download script/URL (optional)
+download: data/scripts/get_imagenet.sh
diff --git a/yolov5/data/Objects365.yaml b/yolov5/data/Objects365.yaml
new file mode 100644
index 0000000000000000000000000000000000000000..bb2aa34cd4a4f85870747039485695893b3e4c2b
--- /dev/null
+++ b/yolov5/data/Objects365.yaml
@@ -0,0 +1,438 @@
+# YOLOv5 🚀 by Ultralytics, AGPL-3.0 license
+# Objects365 dataset https://www.objects365.org/ by Megvii
+# Example usage: python train.py --data Objects365.yaml
+# parent
+# ├── yolov5
+# └── datasets
+# └── Objects365 ← downloads here (712 GB = 367G data + 345G zips)
+
+
+# Train/val/test sets as 1) dir: path/to/imgs, 2) file: path/to/imgs.txt, or 3) list: [path/to/imgs1, path/to/imgs2, ..]
+path: ../datasets/Objects365 # dataset root dir
+train: images/train # train images (relative to 'path') 1742289 images
+val: images/val # val images (relative to 'path') 80000 images
+test: # test images (optional)
+
+# Classes
+names:
+ 0: Person
+ 1: Sneakers
+ 2: Chair
+ 3: Other Shoes
+ 4: Hat
+ 5: Car
+ 6: Lamp
+ 7: Glasses
+ 8: Bottle
+ 9: Desk
+ 10: Cup
+ 11: Street Lights
+ 12: Cabinet/shelf
+ 13: Handbag/Satchel
+ 14: Bracelet
+ 15: Plate
+ 16: Picture/Frame
+ 17: Helmet
+ 18: Book
+ 19: Gloves
+ 20: Storage box
+ 21: Boat
+ 22: Leather Shoes
+ 23: Flower
+ 24: Bench
+ 25: Potted Plant
+ 26: Bowl/Basin
+ 27: Flag
+ 28: Pillow
+ 29: Boots
+ 30: Vase
+ 31: Microphone
+ 32: Necklace
+ 33: Ring
+ 34: SUV
+ 35: Wine Glass
+ 36: Belt
+ 37: Monitor/TV
+ 38: Backpack
+ 39: Umbrella
+ 40: Traffic Light
+ 41: Speaker
+ 42: Watch
+ 43: Tie
+ 44: Trash bin Can
+ 45: Slippers
+ 46: Bicycle
+ 47: Stool
+ 48: Barrel/bucket
+ 49: Van
+ 50: Couch
+ 51: Sandals
+ 52: Basket
+ 53: Drum
+ 54: Pen/Pencil
+ 55: Bus
+ 56: Wild Bird
+ 57: High Heels
+ 58: Motorcycle
+ 59: Guitar
+ 60: Carpet
+ 61: Cell Phone
+ 62: Bread
+ 63: Camera
+ 64: Canned
+ 65: Truck
+ 66: Traffic cone
+ 67: Cymbal
+ 68: Lifesaver
+ 69: Towel
+ 70: Stuffed Toy
+ 71: Candle
+ 72: Sailboat
+ 73: Laptop
+ 74: Awning
+ 75: Bed
+ 76: Faucet
+ 77: Tent
+ 78: Horse
+ 79: Mirror
+ 80: Power outlet
+ 81: Sink
+ 82: Apple
+ 83: Air Conditioner
+ 84: Knife
+ 85: Hockey Stick
+ 86: Paddle
+ 87: Pickup Truck
+ 88: Fork
+ 89: Traffic Sign
+ 90: Balloon
+ 91: Tripod
+ 92: Dog
+ 93: Spoon
+ 94: Clock
+ 95: Pot
+ 96: Cow
+ 97: Cake
+ 98: Dinning Table
+ 99: Sheep
+ 100: Hanger
+ 101: Blackboard/Whiteboard
+ 102: Napkin
+ 103: Other Fish
+ 104: Orange/Tangerine
+ 105: Toiletry
+ 106: Keyboard
+ 107: Tomato
+ 108: Lantern
+ 109: Machinery Vehicle
+ 110: Fan
+ 111: Green Vegetables
+ 112: Banana
+ 113: Baseball Glove
+ 114: Airplane
+ 115: Mouse
+ 116: Train
+ 117: Pumpkin
+ 118: Soccer
+ 119: Skiboard
+ 120: Luggage
+ 121: Nightstand
+ 122: Tea pot
+ 123: Telephone
+ 124: Trolley
+ 125: Head Phone
+ 126: Sports Car
+ 127: Stop Sign
+ 128: Dessert
+ 129: Scooter
+ 130: Stroller
+ 131: Crane
+ 132: Remote
+ 133: Refrigerator
+ 134: Oven
+ 135: Lemon
+ 136: Duck
+ 137: Baseball Bat
+ 138: Surveillance Camera
+ 139: Cat
+ 140: Jug
+ 141: Broccoli
+ 142: Piano
+ 143: Pizza
+ 144: Elephant
+ 145: Skateboard
+ 146: Surfboard
+ 147: Gun
+ 148: Skating and Skiing shoes
+ 149: Gas stove
+ 150: Donut
+ 151: Bow Tie
+ 152: Carrot
+ 153: Toilet
+ 154: Kite
+ 155: Strawberry
+ 156: Other Balls
+ 157: Shovel
+ 158: Pepper
+ 159: Computer Box
+ 160: Toilet Paper
+ 161: Cleaning Products
+ 162: Chopsticks
+ 163: Microwave
+ 164: Pigeon
+ 165: Baseball
+ 166: Cutting/chopping Board
+ 167: Coffee Table
+ 168: Side Table
+ 169: Scissors
+ 170: Marker
+ 171: Pie
+ 172: Ladder
+ 173: Snowboard
+ 174: Cookies
+ 175: Radiator
+ 176: Fire Hydrant
+ 177: Basketball
+ 178: Zebra
+ 179: Grape
+ 180: Giraffe
+ 181: Potato
+ 182: Sausage
+ 183: Tricycle
+ 184: Violin
+ 185: Egg
+ 186: Fire Extinguisher
+ 187: Candy
+ 188: Fire Truck
+ 189: Billiards
+ 190: Converter
+ 191: Bathtub
+ 192: Wheelchair
+ 193: Golf Club
+ 194: Briefcase
+ 195: Cucumber
+ 196: Cigar/Cigarette
+ 197: Paint Brush
+ 198: Pear
+ 199: Heavy Truck
+ 200: Hamburger
+ 201: Extractor
+ 202: Extension Cord
+ 203: Tong
+ 204: Tennis Racket
+ 205: Folder
+ 206: American Football
+ 207: earphone
+ 208: Mask
+ 209: Kettle
+ 210: Tennis
+ 211: Ship
+ 212: Swing
+ 213: Coffee Machine
+ 214: Slide
+ 215: Carriage
+ 216: Onion
+ 217: Green beans
+ 218: Projector
+ 219: Frisbee
+ 220: Washing Machine/Drying Machine
+ 221: Chicken
+ 222: Printer
+ 223: Watermelon
+ 224: Saxophone
+ 225: Tissue
+ 226: Toothbrush
+ 227: Ice cream
+ 228: Hot-air balloon
+ 229: Cello
+ 230: French Fries
+ 231: Scale
+ 232: Trophy
+ 233: Cabbage
+ 234: Hot dog
+ 235: Blender
+ 236: Peach
+ 237: Rice
+ 238: Wallet/Purse
+ 239: Volleyball
+ 240: Deer
+ 241: Goose
+ 242: Tape
+ 243: Tablet
+ 244: Cosmetics
+ 245: Trumpet
+ 246: Pineapple
+ 247: Golf Ball
+ 248: Ambulance
+ 249: Parking meter
+ 250: Mango
+ 251: Key
+ 252: Hurdle
+ 253: Fishing Rod
+ 254: Medal
+ 255: Flute
+ 256: Brush
+ 257: Penguin
+ 258: Megaphone
+ 259: Corn
+ 260: Lettuce
+ 261: Garlic
+ 262: Swan
+ 263: Helicopter
+ 264: Green Onion
+ 265: Sandwich
+ 266: Nuts
+ 267: Speed Limit Sign
+ 268: Induction Cooker
+ 269: Broom
+ 270: Trombone
+ 271: Plum
+ 272: Rickshaw
+ 273: Goldfish
+ 274: Kiwi fruit
+ 275: Router/modem
+ 276: Poker Card
+ 277: Toaster
+ 278: Shrimp
+ 279: Sushi
+ 280: Cheese
+ 281: Notepaper
+ 282: Cherry
+ 283: Pliers
+ 284: CD
+ 285: Pasta
+ 286: Hammer
+ 287: Cue
+ 288: Avocado
+ 289: Hamimelon
+ 290: Flask
+ 291: Mushroom
+ 292: Screwdriver
+ 293: Soap
+ 294: Recorder
+ 295: Bear
+ 296: Eggplant
+ 297: Board Eraser
+ 298: Coconut
+ 299: Tape Measure/Ruler
+ 300: Pig
+ 301: Showerhead
+ 302: Globe
+ 303: Chips
+ 304: Steak
+ 305: Crosswalk Sign
+ 306: Stapler
+ 307: Camel
+ 308: Formula 1
+ 309: Pomegranate
+ 310: Dishwasher
+ 311: Crab
+ 312: Hoverboard
+ 313: Meat ball
+ 314: Rice Cooker
+ 315: Tuba
+ 316: Calculator
+ 317: Papaya
+ 318: Antelope
+ 319: Parrot
+ 320: Seal
+ 321: Butterfly
+ 322: Dumbbell
+ 323: Donkey
+ 324: Lion
+ 325: Urinal
+ 326: Dolphin
+ 327: Electric Drill
+ 328: Hair Dryer
+ 329: Egg tart
+ 330: Jellyfish
+ 331: Treadmill
+ 332: Lighter
+ 333: Grapefruit
+ 334: Game board
+ 335: Mop
+ 336: Radish
+ 337: Baozi
+ 338: Target
+ 339: French
+ 340: Spring Rolls
+ 341: Monkey
+ 342: Rabbit
+ 343: Pencil Case
+ 344: Yak
+ 345: Red Cabbage
+ 346: Binoculars
+ 347: Asparagus
+ 348: Barbell
+ 349: Scallop
+ 350: Noddles
+ 351: Comb
+ 352: Dumpling
+ 353: Oyster
+ 354: Table Tennis paddle
+ 355: Cosmetics Brush/Eyeliner Pencil
+ 356: Chainsaw
+ 357: Eraser
+ 358: Lobster
+ 359: Durian
+ 360: Okra
+ 361: Lipstick
+ 362: Cosmetics Mirror
+ 363: Curling
+ 364: Table Tennis
+
+
+# Download script/URL (optional) ---------------------------------------------------------------------------------------
+download: |
+ from tqdm import tqdm
+
+ from utils.general import Path, check_requirements, download, np, xyxy2xywhn
+
+ check_requirements(('pycocotools>=2.0',))
+ from pycocotools.coco import COCO
+
+ # Make Directories
+ dir = Path(yaml['path']) # dataset root dir
+ for p in 'images', 'labels':
+ (dir / p).mkdir(parents=True, exist_ok=True)
+ for q in 'train', 'val':
+ (dir / p / q).mkdir(parents=True, exist_ok=True)
+
+ # Train, Val Splits
+ for split, patches in [('train', 50 + 1), ('val', 43 + 1)]:
+ print(f"Processing {split} in {patches} patches ...")
+ images, labels = dir / 'images' / split, dir / 'labels' / split
+
+ # Download
+ url = f"https://dorc.ks3-cn-beijing.ksyun.com/data-set/2020Objects365%E6%95%B0%E6%8D%AE%E9%9B%86/{split}/"
+ if split == 'train':
+ download([f'{url}zhiyuan_objv2_{split}.tar.gz'], dir=dir, delete=False) # annotations json
+ download([f'{url}patch{i}.tar.gz' for i in range(patches)], dir=images, curl=True, delete=False, threads=8)
+ elif split == 'val':
+ download([f'{url}zhiyuan_objv2_{split}.json'], dir=dir, delete=False) # annotations json
+ download([f'{url}images/v1/patch{i}.tar.gz' for i in range(15 + 1)], dir=images, curl=True, delete=False, threads=8)
+ download([f'{url}images/v2/patch{i}.tar.gz' for i in range(16, patches)], dir=images, curl=True, delete=False, threads=8)
+
+ # Move
+ for f in tqdm(images.rglob('*.jpg'), desc=f'Moving {split} images'):
+ f.rename(images / f.name) # move to /images/{split}
+
+ # Labels
+ coco = COCO(dir / f'zhiyuan_objv2_{split}.json')
+ names = [x["name"] for x in coco.loadCats(coco.getCatIds())]
+ for cid, cat in enumerate(names):
+ catIds = coco.getCatIds(catNms=[cat])
+ imgIds = coco.getImgIds(catIds=catIds)
+ for im in tqdm(coco.loadImgs(imgIds), desc=f'Class {cid + 1}/{len(names)} {cat}'):
+ width, height = im["width"], im["height"]
+ path = Path(im["file_name"]) # image filename
+ try:
+ with open(labels / path.with_suffix('.txt').name, 'a') as file:
+ annIds = coco.getAnnIds(imgIds=im["id"], catIds=catIds, iscrowd=None)
+ for a in coco.loadAnns(annIds):
+ x, y, w, h = a['bbox'] # bounding box in xywh (xy top-left corner)
+ xyxy = np.array([x, y, x + w, y + h])[None] # pixels(1,4)
+ x, y, w, h = xyxy2xywhn(xyxy, w=width, h=height, clip=True)[0] # normalized and clipped
+ file.write(f"{cid} {x:.5f} {y:.5f} {w:.5f} {h:.5f}\n")
+ except Exception as e:
+ print(e)
diff --git a/yolov5/data/SKU-110K.yaml b/yolov5/data/SKU-110K.yaml
new file mode 100644
index 0000000000000000000000000000000000000000..a943eecdeee699c6f85d08311b925b2ededc8836
--- /dev/null
+++ b/yolov5/data/SKU-110K.yaml
@@ -0,0 +1,53 @@
+# YOLOv5 🚀 by Ultralytics, AGPL-3.0 license
+# SKU-110K retail items dataset https://github.com/eg4000/SKU110K_CVPR19 by Trax Retail
+# Example usage: python train.py --data SKU-110K.yaml
+# parent
+# ├── yolov5
+# └── datasets
+# └── SKU-110K ← downloads here (13.6 GB)
+
+
+# Train/val/test sets as 1) dir: path/to/imgs, 2) file: path/to/imgs.txt, or 3) list: [path/to/imgs1, path/to/imgs2, ..]
+path: ../datasets/SKU-110K # dataset root dir
+train: train.txt # train images (relative to 'path') 8219 images
+val: val.txt # val images (relative to 'path') 588 images
+test: test.txt # test images (optional) 2936 images
+
+# Classes
+names:
+ 0: object
+
+
+# Download script/URL (optional) ---------------------------------------------------------------------------------------
+download: |
+ import shutil
+ from tqdm import tqdm
+ from utils.general import np, pd, Path, download, xyxy2xywh
+
+
+ # Download
+ dir = Path(yaml['path']) # dataset root dir
+ parent = Path(dir.parent) # download dir
+ urls = ['http://trax-geometry.s3.amazonaws.com/cvpr_challenge/SKU110K_fixed.tar.gz']
+ download(urls, dir=parent, delete=False)
+
+ # Rename directories
+ if dir.exists():
+ shutil.rmtree(dir)
+ (parent / 'SKU110K_fixed').rename(dir) # rename dir
+ (dir / 'labels').mkdir(parents=True, exist_ok=True) # create labels dir
+
+ # Convert labels
+ names = 'image', 'x1', 'y1', 'x2', 'y2', 'class', 'image_width', 'image_height' # column names
+ for d in 'annotations_train.csv', 'annotations_val.csv', 'annotations_test.csv':
+ x = pd.read_csv(dir / 'annotations' / d, names=names).values # annotations
+ images, unique_images = x[:, 0], np.unique(x[:, 0])
+ with open((dir / d).with_suffix('.txt').__str__().replace('annotations_', ''), 'w') as f:
+ f.writelines(f'./images/{s}\n' for s in unique_images)
+ for im in tqdm(unique_images, desc=f'Converting {dir / d}'):
+ cls = 0 # single-class dataset
+ with open((dir / 'labels' / im).with_suffix('.txt'), 'a') as f:
+ for r in x[images == im]:
+ w, h = r[6], r[7] # image width, height
+ xywh = xyxy2xywh(np.array([[r[1] / w, r[2] / h, r[3] / w, r[4] / h]]))[0] # instance
+ f.write(f"{cls} {xywh[0]:.5f} {xywh[1]:.5f} {xywh[2]:.5f} {xywh[3]:.5f}\n") # write label
diff --git a/yolov5/data/VOC.yaml b/yolov5/data/VOC.yaml
new file mode 100644
index 0000000000000000000000000000000000000000..104856f0c9c77dbcccff866d0d0677be5c08c2a0
--- /dev/null
+++ b/yolov5/data/VOC.yaml
@@ -0,0 +1,100 @@
+# YOLOv5 🚀 by Ultralytics, AGPL-3.0 license
+# PASCAL VOC dataset http://host.robots.ox.ac.uk/pascal/VOC by University of Oxford
+# Example usage: python train.py --data VOC.yaml
+# parent
+# ├── yolov5
+# └── datasets
+# └── VOC ← downloads here (2.8 GB)
+
+
+# Train/val/test sets as 1) dir: path/to/imgs, 2) file: path/to/imgs.txt, or 3) list: [path/to/imgs1, path/to/imgs2, ..]
+path: ../datasets/VOC
+train: # train images (relative to 'path') 16551 images
+ - images/train2012
+ - images/train2007
+ - images/val2012
+ - images/val2007
+val: # val images (relative to 'path') 4952 images
+ - images/test2007
+test: # test images (optional)
+ - images/test2007
+
+# Classes
+names:
+ 0: aeroplane
+ 1: bicycle
+ 2: bird
+ 3: boat
+ 4: bottle
+ 5: bus
+ 6: car
+ 7: cat
+ 8: chair
+ 9: cow
+ 10: diningtable
+ 11: dog
+ 12: horse
+ 13: motorbike
+ 14: person
+ 15: pottedplant
+ 16: sheep
+ 17: sofa
+ 18: train
+ 19: tvmonitor
+
+
+# Download script/URL (optional) ---------------------------------------------------------------------------------------
+download: |
+ import xml.etree.ElementTree as ET
+
+ from tqdm import tqdm
+ from utils.general import download, Path
+
+
+ def convert_label(path, lb_path, year, image_id):
+ def convert_box(size, box):
+ dw, dh = 1. / size[0], 1. / size[1]
+ x, y, w, h = (box[0] + box[1]) / 2.0 - 1, (box[2] + box[3]) / 2.0 - 1, box[1] - box[0], box[3] - box[2]
+ return x * dw, y * dh, w * dw, h * dh
+
+ in_file = open(path / f'VOC{year}/Annotations/{image_id}.xml')
+ out_file = open(lb_path, 'w')
+ tree = ET.parse(in_file)
+ root = tree.getroot()
+ size = root.find('size')
+ w = int(size.find('width').text)
+ h = int(size.find('height').text)
+
+ names = list(yaml['names'].values()) # names list
+ for obj in root.iter('object'):
+ cls = obj.find('name').text
+ if cls in names and int(obj.find('difficult').text) != 1:
+ xmlbox = obj.find('bndbox')
+ bb = convert_box((w, h), [float(xmlbox.find(x).text) for x in ('xmin', 'xmax', 'ymin', 'ymax')])
+ cls_id = names.index(cls) # class id
+ out_file.write(" ".join([str(a) for a in (cls_id, *bb)]) + '\n')
+
+
+ # Download
+ dir = Path(yaml['path']) # dataset root dir
+ url = 'https://github.com/ultralytics/yolov5/releases/download/v1.0/'
+ urls = [f'{url}VOCtrainval_06-Nov-2007.zip', # 446MB, 5012 images
+ f'{url}VOCtest_06-Nov-2007.zip', # 438MB, 4953 images
+ f'{url}VOCtrainval_11-May-2012.zip'] # 1.95GB, 17126 images
+ download(urls, dir=dir / 'images', delete=False, curl=True, threads=3)
+
+ # Convert
+ path = dir / 'images/VOCdevkit'
+ for year, image_set in ('2012', 'train'), ('2012', 'val'), ('2007', 'train'), ('2007', 'val'), ('2007', 'test'):
+ imgs_path = dir / 'images' / f'{image_set}{year}'
+ lbs_path = dir / 'labels' / f'{image_set}{year}'
+ imgs_path.mkdir(exist_ok=True, parents=True)
+ lbs_path.mkdir(exist_ok=True, parents=True)
+
+ with open(path / f'VOC{year}/ImageSets/Main/{image_set}.txt') as f:
+ image_ids = f.read().strip().split()
+ for id in tqdm(image_ids, desc=f'{image_set}{year}'):
+ f = path / f'VOC{year}/JPEGImages/{id}.jpg' # old img path
+ lb_path = (lbs_path / f.name).with_suffix('.txt') # new label path
+ f.rename(imgs_path / f.name) # move image
+ convert_label(path, lb_path, year, id) # convert labels to YOLO format
diff --git a/yolov5/data/VisDrone.yaml b/yolov5/data/VisDrone.yaml
new file mode 100644
index 0000000000000000000000000000000000000000..2a13904dc8dd50c3906b18cc414a6975b2c28dd6
--- /dev/null
+++ b/yolov5/data/VisDrone.yaml
@@ -0,0 +1,70 @@
+# YOLOv5 🚀 by Ultralytics, AGPL-3.0 license
+# VisDrone2019-DET dataset https://github.com/VisDrone/VisDrone-Dataset by Tianjin University
+# Example usage: python train.py --data VisDrone.yaml
+# parent
+# ├── yolov5
+# └── datasets
+# └── VisDrone ← downloads here (2.3 GB)
+
+
+# Train/val/test sets as 1) dir: path/to/imgs, 2) file: path/to/imgs.txt, or 3) list: [path/to/imgs1, path/to/imgs2, ..]
+path: ../datasets/VisDrone # dataset root dir
+train: VisDrone2019-DET-train/images # train images (relative to 'path') 6471 images
+val: VisDrone2019-DET-val/images # val images (relative to 'path') 548 images
+test: VisDrone2019-DET-test-dev/images # test images (optional) 1610 images
+
+# Classes
+names:
+ 0: pedestrian
+ 1: people
+ 2: bicycle
+ 3: car
+ 4: van
+ 5: truck
+ 6: tricycle
+ 7: awning-tricycle
+ 8: bus
+ 9: motor
+
+
+# Download script/URL (optional) ---------------------------------------------------------------------------------------
+download: |
+ from utils.general import download, os, Path
+
+ def visdrone2yolo(dir):
+ from PIL import Image
+ from tqdm import tqdm
+
+ def convert_box(size, box):
+ # Convert VisDrone box to YOLO xywh box
+ dw = 1. / size[0]
+ dh = 1. / size[1]
+ return (box[0] + box[2] / 2) * dw, (box[1] + box[3] / 2) * dh, box[2] * dw, box[3] * dh
+
+ (dir / 'labels').mkdir(parents=True, exist_ok=True) # make labels directory
+ pbar = tqdm((dir / 'annotations').glob('*.txt'), desc=f'Converting {dir}')
+ for f in pbar:
+ img_size = Image.open((dir / 'images' / f.name).with_suffix('.jpg')).size
+ lines = []
+ with open(f, 'r') as file: # read annotation.txt
+ for row in [x.split(',') for x in file.read().strip().splitlines()]:
+ if row[4] == '0': # VisDrone 'ignored regions' class 0
+ continue
+ cls = int(row[5]) - 1
+ box = convert_box(img_size, tuple(map(int, row[:4])))
+ lines.append(f"{cls} {' '.join(f'{x:.6f}' for x in box)}\n")
+ with open(str(f).replace(os.sep + 'annotations' + os.sep, os.sep + 'labels' + os.sep), 'w') as fl:
+ fl.writelines(lines) # write label.txt
+
+
+ # Download
+ dir = Path(yaml['path']) # dataset root dir
+ urls = ['https://github.com/ultralytics/yolov5/releases/download/v1.0/VisDrone2019-DET-train.zip',
+ 'https://github.com/ultralytics/yolov5/releases/download/v1.0/VisDrone2019-DET-val.zip',
+ 'https://github.com/ultralytics/yolov5/releases/download/v1.0/VisDrone2019-DET-test-dev.zip',
+ 'https://github.com/ultralytics/yolov5/releases/download/v1.0/VisDrone2019-DET-test-challenge.zip']
+ download(urls, dir=dir, curl=True, threads=4)
+
+ # Convert
+ for d in 'VisDrone2019-DET-train', 'VisDrone2019-DET-val', 'VisDrone2019-DET-test-dev':
+ visdrone2yolo(dir / d) # convert VisDrone annotations to YOLO labels
diff --git a/yolov5/data/coco.yaml b/yolov5/data/coco.yaml
new file mode 100644
index 0000000000000000000000000000000000000000..ea32cb6269a362886c7283e4a3d543115891eec8
--- /dev/null
+++ b/yolov5/data/coco.yaml
@@ -0,0 +1,116 @@
+# YOLOv5 🚀 by Ultralytics, AGPL-3.0 license
+# COCO 2017 dataset http://cocodataset.org by Microsoft
+# Example usage: python train.py --data coco.yaml
+# parent
+# ├── yolov5
+# └── datasets
+# └── coco ← downloads here (20.1 GB)
+
+
+# Train/val/test sets as 1) dir: path/to/imgs, 2) file: path/to/imgs.txt, or 3) list: [path/to/imgs1, path/to/imgs2, ..]
+path: ../datasets/coco # dataset root dir
+train: train2017.txt # train images (relative to 'path') 118287 images
+val: val2017.txt # val images (relative to 'path') 5000 images
+test: test-dev2017.txt # 20288 of 40670 images, submit to https://competitions.codalab.org/competitions/20794
+
+# Classes
+names:
+ 0: person
+ 1: bicycle
+ 2: car
+ 3: motorcycle
+ 4: airplane
+ 5: bus
+ 6: train
+ 7: truck
+ 8: boat
+ 9: traffic light
+ 10: fire hydrant
+ 11: stop sign
+ 12: parking meter
+ 13: bench
+ 14: bird
+ 15: cat
+ 16: dog
+ 17: horse
+ 18: sheep
+ 19: cow
+ 20: elephant
+ 21: bear
+ 22: zebra
+ 23: giraffe
+ 24: backpack
+ 25: umbrella
+ 26: handbag
+ 27: tie
+ 28: suitcase
+ 29: frisbee
+ 30: skis
+ 31: snowboard
+ 32: sports ball
+ 33: kite
+ 34: baseball bat
+ 35: baseball glove
+ 36: skateboard
+ 37: surfboard
+ 38: tennis racket
+ 39: bottle
+ 40: wine glass
+ 41: cup
+ 42: fork
+ 43: knife
+ 44: spoon
+ 45: bowl
+ 46: banana
+ 47: apple
+ 48: sandwich
+ 49: orange
+ 50: broccoli
+ 51: carrot
+ 52: hot dog
+ 53: pizza
+ 54: donut
+ 55: cake
+ 56: chair
+ 57: couch
+ 58: potted plant
+ 59: bed
+ 60: dining table
+ 61: toilet
+ 62: tv
+ 63: laptop
+ 64: mouse
+ 65: remote
+ 66: keyboard
+ 67: cell phone
+ 68: microwave
+ 69: oven
+ 70: toaster
+ 71: sink
+ 72: refrigerator
+ 73: book
+ 74: clock
+ 75: vase
+ 76: scissors
+ 77: teddy bear
+ 78: hair drier
+ 79: toothbrush
+
+
+# Download script/URL (optional)
+download: |
+ from utils.general import download, Path
+
+
+ # Download labels
+ segments = False # segment or box labels
+ dir = Path(yaml['path']) # dataset root dir
+ url = 'https://github.com/ultralytics/yolov5/releases/download/v1.0/'
+ urls = [url + ('coco2017labels-segments.zip' if segments else 'coco2017labels.zip')] # labels
+ download(urls, dir=dir.parent)
+
+ # Download data
+ urls = ['http://images.cocodataset.org/zips/train2017.zip', # 19G, 118k images
+ 'http://images.cocodataset.org/zips/val2017.zip', # 1G, 5k images
+ 'http://images.cocodataset.org/zips/test2017.zip'] # 7G, 41k images (optional)
+ download(urls, dir=dir / 'images', threads=3)
diff --git a/yolov5/data/coco128-seg.yaml b/yolov5/data/coco128-seg.yaml
new file mode 100644
index 0000000000000000000000000000000000000000..0a2499c00a1aa012b0d1a031714977843874b29d
--- /dev/null
+++ b/yolov5/data/coco128-seg.yaml
@@ -0,0 +1,101 @@
+# YOLOv5 🚀 by Ultralytics, AGPL-3.0 license
+# COCO128-seg dataset https://www.kaggle.com/ultralytics/coco128 (first 128 images from COCO train2017) by Ultralytics
+# Example usage: python train.py --data coco128.yaml
+# parent
+# ├── yolov5
+# └── datasets
+# └── coco128-seg ← downloads here (7 MB)
+
+
+# Train/val/test sets as 1) dir: path/to/imgs, 2) file: path/to/imgs.txt, or 3) list: [path/to/imgs1, path/to/imgs2, ..]
+path: ../datasets/coco128-seg # dataset root dir
+train: images/train2017 # train images (relative to 'path') 128 images
+val: images/train2017 # val images (relative to 'path') 128 images
+test: # test images (optional)
+
+# Classes
+names:
+ 0: person
+ 1: bicycle
+ 2: car
+ 3: motorcycle
+ 4: airplane
+ 5: bus
+ 6: train
+ 7: truck
+ 8: boat
+ 9: traffic light
+ 10: fire hydrant
+ 11: stop sign
+ 12: parking meter
+ 13: bench
+ 14: bird
+ 15: cat
+ 16: dog
+ 17: horse
+ 18: sheep
+ 19: cow
+ 20: elephant
+ 21: bear
+ 22: zebra
+ 23: giraffe
+ 24: backpack
+ 25: umbrella
+ 26: handbag
+ 27: tie
+ 28: suitcase
+ 29: frisbee
+ 30: skis
+ 31: snowboard
+ 32: sports ball
+ 33: kite
+ 34: baseball bat
+ 35: baseball glove
+ 36: skateboard
+ 37: surfboard
+ 38: tennis racket
+ 39: bottle
+ 40: wine glass
+ 41: cup
+ 42: fork
+ 43: knife
+ 44: spoon
+ 45: bowl
+ 46: banana
+ 47: apple
+ 48: sandwich
+ 49: orange
+ 50: broccoli
+ 51: carrot
+ 52: hot dog
+ 53: pizza
+ 54: donut
+ 55: cake
+ 56: chair
+ 57: couch
+ 58: potted plant
+ 59: bed
+ 60: dining table
+ 61: toilet
+ 62: tv
+ 63: laptop
+ 64: mouse
+ 65: remote
+ 66: keyboard
+ 67: cell phone
+ 68: microwave
+ 69: oven
+ 70: toaster
+ 71: sink
+ 72: refrigerator
+ 73: book
+ 74: clock
+ 75: vase
+ 76: scissors
+ 77: teddy bear
+ 78: hair drier
+ 79: toothbrush
+
+
+# Download script/URL (optional)
+download: https://ultralytics.com/assets/coco128-seg.zip
diff --git a/yolov5/data/coco128.yaml b/yolov5/data/coco128.yaml
new file mode 100644
index 0000000000000000000000000000000000000000..0cb53120be2c88db1543e9600576d200c4775a41
--- /dev/null
+++ b/yolov5/data/coco128.yaml
@@ -0,0 +1,101 @@
+# YOLOv5 🚀 by Ultralytics, AGPL-3.0 license
+# COCO128 dataset https://www.kaggle.com/ultralytics/coco128 (first 128 images from COCO train2017) by Ultralytics
+# Example usage: python train.py --data coco128.yaml
+# parent
+# ├── yolov5
+# └── datasets
+# └── coco128 ← downloads here (7 MB)
+
+
+# Train/val/test sets as 1) dir: path/to/imgs, 2) file: path/to/imgs.txt, or 3) list: [path/to/imgs1, path/to/imgs2, ..]
+path: ../datasets/coco128 # dataset root dir
+train: images/train2017 # train images (relative to 'path') 128 images
+val: images/train2017 # val images (relative to 'path') 128 images
+test: # test images (optional)
+
+# Classes
+names:
+ 0: person
+ 1: bicycle
+ 2: car
+ 3: motorcycle
+ 4: airplane
+ 5: bus
+ 6: train
+ 7: truck
+ 8: boat
+ 9: traffic light
+ 10: fire hydrant
+ 11: stop sign
+ 12: parking meter
+ 13: bench
+ 14: bird
+ 15: cat
+ 16: dog
+ 17: horse
+ 18: sheep
+ 19: cow
+ 20: elephant
+ 21: bear
+ 22: zebra
+ 23: giraffe
+ 24: backpack
+ 25: umbrella
+ 26: handbag
+ 27: tie
+ 28: suitcase
+ 29: frisbee
+ 30: skis
+ 31: snowboard
+ 32: sports ball
+ 33: kite
+ 34: baseball bat
+ 35: baseball glove
+ 36: skateboard
+ 37: surfboard
+ 38: tennis racket
+ 39: bottle
+ 40: wine glass
+ 41: cup
+ 42: fork
+ 43: knife
+ 44: spoon
+ 45: bowl
+ 46: banana
+ 47: apple
+ 48: sandwich
+ 49: orange
+ 50: broccoli
+ 51: carrot
+ 52: hot dog
+ 53: pizza
+ 54: donut
+ 55: cake
+ 56: chair
+ 57: couch
+ 58: potted plant
+ 59: bed
+ 60: dining table
+ 61: toilet
+ 62: tv
+ 63: laptop
+ 64: mouse
+ 65: remote
+ 66: keyboard
+ 67: cell phone
+ 68: microwave
+ 69: oven
+ 70: toaster
+ 71: sink
+ 72: refrigerator
+ 73: book
+ 74: clock
+ 75: vase
+ 76: scissors
+ 77: teddy bear
+ 78: hair drier
+ 79: toothbrush
+
+
+# Download script/URL (optional)
+download: https://ultralytics.com/assets/coco128.zip
diff --git a/yolov5/data/hyps/hyp.Objects365.yaml b/yolov5/data/hyps/hyp.Objects365.yaml
new file mode 100644
index 0000000000000000000000000000000000000000..c4b6e8051d7bafd93155c8e03e1b264b468f68a7
--- /dev/null
+++ b/yolov5/data/hyps/hyp.Objects365.yaml
@@ -0,0 +1,34 @@
+# YOLOv5 🚀 by Ultralytics, AGPL-3.0 license
+# Hyperparameters for Objects365 training
+# python train.py --weights yolov5m.pt --data Objects365.yaml --evolve
+# See Hyperparameter Evolution tutorial for details https://github.com/ultralytics/yolov5#tutorials
+
+lr0: 0.00258
+lrf: 0.17
+momentum: 0.779
+weight_decay: 0.00058
+warmup_epochs: 1.33
+warmup_momentum: 0.86
+warmup_bias_lr: 0.0711
+box: 0.0539
+cls: 0.299
+cls_pw: 0.825
+obj: 0.632
+obj_pw: 1.0
+iou_t: 0.2
+anchor_t: 3.44
+anchors: 3.2
+fl_gamma: 0.0
+hsv_h: 0.0188
+hsv_s: 0.704
+hsv_v: 0.36
+degrees: 0.0
+translate: 0.0902
+scale: 0.491
+shear: 0.0
+perspective: 0.0
+flipud: 0.0
+fliplr: 0.5
+mosaic: 1.0
+mixup: 0.0
+copy_paste: 0.0
diff --git a/yolov5/data/hyps/hyp.VOC.yaml b/yolov5/data/hyps/hyp.VOC.yaml
new file mode 100644
index 0000000000000000000000000000000000000000..ce20dbbddbdbdb7228ca3262fade10b64b798087
--- /dev/null
+++ b/yolov5/data/hyps/hyp.VOC.yaml
@@ -0,0 +1,40 @@
+# YOLOv5 🚀 by Ultralytics, AGPL-3.0 license
+# Hyperparameters for VOC training
+# python train.py --batch 128 --weights yolov5m6.pt --data VOC.yaml --epochs 50 --img 512 --hyp hyp.scratch-med.yaml --evolve
+# See Hyperparameter Evolution tutorial for details https://github.com/ultralytics/yolov5#tutorials
+
+# YOLOv5 Hyperparameter Evolution Results
+# Best generation: 467
+# Last generation: 996
+# metrics/precision, metrics/recall, metrics/mAP_0.5, metrics/mAP_0.5:0.95, val/box_loss, val/obj_loss, val/cls_loss
+# 0.87729, 0.85125, 0.91286, 0.72664, 0.0076739, 0.0042529, 0.0013865
+
+lr0: 0.00334
+lrf: 0.15135
+momentum: 0.74832
+weight_decay: 0.00025
+warmup_epochs: 3.3835
+warmup_momentum: 0.59462
+warmup_bias_lr: 0.18657
+box: 0.02
+cls: 0.21638
+cls_pw: 0.5
+obj: 0.51728
+obj_pw: 0.67198
+iou_t: 0.2
+anchor_t: 3.3744
+fl_gamma: 0.0
+hsv_h: 0.01041
+hsv_s: 0.54703
+hsv_v: 0.27739
+degrees: 0.0
+translate: 0.04591
+scale: 0.75544
+shear: 0.0
+perspective: 0.0
+flipud: 0.0
+fliplr: 0.5
+mosaic: 0.85834
+mixup: 0.04266
+copy_paste: 0.0
+anchors: 3.412
diff --git a/yolov5/data/hyps/hyp.no-augmentation.yaml b/yolov5/data/hyps/hyp.no-augmentation.yaml
new file mode 100644
index 0000000000000000000000000000000000000000..8da18150538b9918e6140f12ed0740bbc8e95955
--- /dev/null
+++ b/yolov5/data/hyps/hyp.no-augmentation.yaml
@@ -0,0 +1,35 @@
+# YOLOv5 🚀 by Ultralytics, AGPL-3.0 license
+# Hyperparameters when using Albumentations frameworks
+# python train.py --hyp hyp.no-augmentation.yaml
+# See https://github.com/ultralytics/yolov5/pull/3882 for YOLOv5 + Albumentations Usage examples
+
+lr0: 0.01 # initial learning rate (SGD=1E-2, Adam=1E-3)
+lrf: 0.1 # final OneCycleLR learning rate (lr0 * lrf)
+momentum: 0.937 # SGD momentum/Adam beta1
+weight_decay: 0.0005 # optimizer weight decay 5e-4
+warmup_epochs: 3.0 # warmup epochs (fractions ok)
+warmup_momentum: 0.8 # warmup initial momentum
+warmup_bias_lr: 0.1 # warmup initial bias lr
+box: 0.05 # box loss gain
+cls: 0.3 # cls loss gain
+cls_pw: 1.0 # cls BCELoss positive_weight
+obj: 0.7 # obj loss gain (scale with pixels)
+obj_pw: 1.0 # obj BCELoss positive_weight
+iou_t: 0.20 # IoU training threshold
+anchor_t: 4.0 # anchor-multiple threshold
+# anchors: 3 # anchors per output layer (0 to ignore)
+# this parameters are all zero since we want to use albumentation framework
+fl_gamma: 0.0 # focal loss gamma (efficientDet default gamma=1.5)
+hsv_h: 0 # image HSV-Hue augmentation (fraction)
+hsv_s: 0 # image HSV-Saturation augmentation (fraction)
+hsv_v: 0 # image HSV-Value augmentation (fraction)
+degrees: 0.0 # image rotation (+/- deg)
+translate: 0 # image translation (+/- fraction)
+scale: 0 # image scale (+/- gain)
+shear: 0 # image shear (+/- deg)
+perspective: 0.0 # image perspective (+/- fraction), range 0-0.001
+flipud: 0.0 # image flip up-down (probability)
+fliplr: 0.0 # image flip left-right (probability)
+mosaic: 0.0 # image mosaic (probability)
+mixup: 0.0 # image mixup (probability)
+copy_paste: 0.0 # segment copy-paste (probability)
diff --git a/yolov5/data/hyps/hyp.scratch-high.yaml b/yolov5/data/hyps/hyp.scratch-high.yaml
new file mode 100644
index 0000000000000000000000000000000000000000..0a0f4ec216219890f93f457ce7bc31036490d96d
--- /dev/null
+++ b/yolov5/data/hyps/hyp.scratch-high.yaml
@@ -0,0 +1,34 @@
+# YOLOv5 🚀 by Ultralytics, AGPL-3.0 license
+# Hyperparameters for high-augmentation COCO training from scratch
+# python train.py --batch 32 --cfg yolov5m6.yaml --weights '' --data coco.yaml --img 1280 --epochs 300
+# See tutorials for hyperparameter evolution https://github.com/ultralytics/yolov5#tutorials
+
+lr0: 0.01 # initial learning rate (SGD=1E-2, Adam=1E-3)
+lrf: 0.1 # final OneCycleLR learning rate (lr0 * lrf)
+momentum: 0.937 # SGD momentum/Adam beta1
+weight_decay: 0.0005 # optimizer weight decay 5e-4
+warmup_epochs: 3.0 # warmup epochs (fractions ok)
+warmup_momentum: 0.8 # warmup initial momentum
+warmup_bias_lr: 0.1 # warmup initial bias lr
+box: 0.05 # box loss gain
+cls: 0.3 # cls loss gain
+cls_pw: 1.0 # cls BCELoss positive_weight
+obj: 0.7 # obj loss gain (scale with pixels)
+obj_pw: 1.0 # obj BCELoss positive_weight
+iou_t: 0.20 # IoU training threshold
+anchor_t: 4.0 # anchor-multiple threshold
+# anchors: 3 # anchors per output layer (0 to ignore)
+fl_gamma: 0.0 # focal loss gamma (efficientDet default gamma=1.5)
+hsv_h: 0.015 # image HSV-Hue augmentation (fraction)
+hsv_s: 0.7 # image HSV-Saturation augmentation (fraction)
+hsv_v: 0.4 # image HSV-Value augmentation (fraction)
+degrees: 0.0 # image rotation (+/- deg)
+translate: 0.1 # image translation (+/- fraction)
+scale: 0.9 # image scale (+/- gain)
+shear: 0.0 # image shear (+/- deg)
+perspective: 0.0 # image perspective (+/- fraction), range 0-0.001
+flipud: 0.0 # image flip up-down (probability)
+fliplr: 0.5 # image flip left-right (probability)
+mosaic: 1.0 # image mosaic (probability)
+mixup: 0.1 # image mixup (probability)
+copy_paste: 0.1 # segment copy-paste (probability)
diff --git a/yolov5/data/hyps/hyp.scratch-low.yaml b/yolov5/data/hyps/hyp.scratch-low.yaml
new file mode 100644
index 0000000000000000000000000000000000000000..9d722568f5266f60b6fc4a6cd1d126be6fd0c146
--- /dev/null
+++ b/yolov5/data/hyps/hyp.scratch-low.yaml
@@ -0,0 +1,34 @@
+# YOLOv5 🚀 by Ultralytics, AGPL-3.0 license
+# Hyperparameters for low-augmentation COCO training from scratch
+# python train.py --batch 64 --cfg yolov5n6.yaml --weights '' --data coco.yaml --img 640 --epochs 300 --linear
+# See tutorials for hyperparameter evolution https://github.com/ultralytics/yolov5#tutorials
+
+lr0: 0.01 # initial learning rate (SGD=1E-2, Adam=1E-3)
+lrf: 0.01 # final OneCycleLR learning rate (lr0 * lrf)
+momentum: 0.937 # SGD momentum/Adam beta1
+weight_decay: 0.0005 # optimizer weight decay 5e-4
+warmup_epochs: 3.0 # warmup epochs (fractions ok)
+warmup_momentum: 0.8 # warmup initial momentum
+warmup_bias_lr: 0.1 # warmup initial bias lr
+box: 0.05 # box loss gain
+cls: 0.5 # cls loss gain
+cls_pw: 1.0 # cls BCELoss positive_weight
+obj: 1.0 # obj loss gain (scale with pixels)
+obj_pw: 1.0 # obj BCELoss positive_weight
+iou_t: 0.20 # IoU training threshold
+anchor_t: 4.0 # anchor-multiple threshold
+# anchors: 3 # anchors per output layer (0 to ignore)
+fl_gamma: 0.0 # focal loss gamma (efficientDet default gamma=1.5)
+hsv_h: 0.015 # image HSV-Hue augmentation (fraction)
+hsv_s: 0.7 # image HSV-Saturation augmentation (fraction)
+hsv_v: 0.4 # image HSV-Value augmentation (fraction)
+degrees: 0.0 # image rotation (+/- deg)
+translate: 0.1 # image translation (+/- fraction)
+scale: 0.5 # image scale (+/- gain)
+shear: 0.0 # image shear (+/- deg)
+perspective: 0.0 # image perspective (+/- fraction), range 0-0.001
+flipud: 0.0 # image flip up-down (probability)
+fliplr: 0.5 # image flip left-right (probability)
+mosaic: 1.0 # image mosaic (probability)
+mixup: 0.0 # image mixup (probability)
+copy_paste: 0.0 # segment copy-paste (probability)
diff --git a/yolov5/data/hyps/hyp.scratch-med.yaml b/yolov5/data/hyps/hyp.scratch-med.yaml
new file mode 100644
index 0000000000000000000000000000000000000000..f6abb090bb0420a92e571e6d83c12d37f206a8fc
--- /dev/null
+++ b/yolov5/data/hyps/hyp.scratch-med.yaml
@@ -0,0 +1,34 @@
+# YOLOv5 🚀 by Ultralytics, AGPL-3.0 license
+# Hyperparameters for medium-augmentation COCO training from scratch
+# python train.py --batch 32 --cfg yolov5m6.yaml --weights '' --data coco.yaml --img 1280 --epochs 300
+# See tutorials for hyperparameter evolution https://github.com/ultralytics/yolov5#tutorials
+
+lr0: 0.01 # initial learning rate (SGD=1E-2, Adam=1E-3)
+lrf: 0.1 # final OneCycleLR learning rate (lr0 * lrf)
+momentum: 0.937 # SGD momentum/Adam beta1
+weight_decay: 0.0005 # optimizer weight decay 5e-4
+warmup_epochs: 3.0 # warmup epochs (fractions ok)
+warmup_momentum: 0.8 # warmup initial momentum
+warmup_bias_lr: 0.1 # warmup initial bias lr
+box: 0.05 # box loss gain
+cls: 0.3 # cls loss gain
+cls_pw: 1.0 # cls BCELoss positive_weight
+obj: 0.7 # obj loss gain (scale with pixels)
+obj_pw: 1.0 # obj BCELoss positive_weight
+iou_t: 0.20 # IoU training threshold
+anchor_t: 4.0 # anchor-multiple threshold
+# anchors: 3 # anchors per output layer (0 to ignore)
+fl_gamma: 0.0 # focal loss gamma (efficientDet default gamma=1.5)
+hsv_h: 0.015 # image HSV-Hue augmentation (fraction)
+hsv_s: 0.7 # image HSV-Saturation augmentation (fraction)
+hsv_v: 0.4 # image HSV-Value augmentation (fraction)
+degrees: 0.0 # image rotation (+/- deg)
+translate: 0.1 # image translation (+/- fraction)
+scale: 0.9 # image scale (+/- gain)
+shear: 0.0 # image shear (+/- deg)
+perspective: 0.0 # image perspective (+/- fraction), range 0-0.001
+flipud: 0.0 # image flip up-down (probability)
+fliplr: 0.5 # image flip left-right (probability)
+mosaic: 1.0 # image mosaic (probability)
+mixup: 0.1 # image mixup (probability)
+copy_paste: 0.0 # segment copy-paste (probability)
diff --git a/yolov5/data/images/bus.jpg b/yolov5/data/images/bus.jpg
new file mode 100644
index 0000000000000000000000000000000000000000..b43e311165c785f000eb7493ff8fb662d06a3f83
Binary files /dev/null and b/yolov5/data/images/bus.jpg differ
diff --git a/yolov5/data/images/video-4FRnNpmSmwktFJKjg-frame-000850-4EXx8Fi3iE4nWNcCn.jpg b/yolov5/data/images/video-4FRnNpmSmwktFJKjg-frame-000850-4EXx8Fi3iE4nWNcCn.jpg
new file mode 100644
index 0000000000000000000000000000000000000000..d6d21400d9112ce5239d1ae597d6b161bc437d27
Binary files /dev/null and b/yolov5/data/images/video-4FRnNpmSmwktFJKjg-frame-000850-4EXx8Fi3iE4nWNcCn.jpg differ
diff --git a/yolov5/data/images/video-6tLtjdkv5K5BuhB37-frame-000010-KRtKNoxRWwwgfz62F.jpg b/yolov5/data/images/video-6tLtjdkv5K5BuhB37-frame-000010-KRtKNoxRWwwgfz62F.jpg
new file mode 100644
index 0000000000000000000000000000000000000000..a4faea6dec2aadf2eb17f3b7cb7c6ce8083848c6
Binary files /dev/null and b/yolov5/data/images/video-6tLtjdkv5K5BuhB37-frame-000010-KRtKNoxRWwwgfz62F.jpg differ
diff --git a/yolov5/data/images/video-6tLtjdkv5K5BuhB37-frame-000043-Qo8ZHanDJ7p8epxpX.jpg b/yolov5/data/images/video-6tLtjdkv5K5BuhB37-frame-000043-Qo8ZHanDJ7p8epxpX.jpg
new file mode 100644
index 0000000000000000000000000000000000000000..2bde486d59af93ec7f7628250522c89686d674f6
Binary files /dev/null and b/yolov5/data/images/video-6tLtjdkv5K5BuhB37-frame-000043-Qo8ZHanDJ7p8epxpX.jpg differ
diff --git a/yolov5/data/images/video-6tLtjdkv5K5BuhB37-frame-000044-Z5MfsCQMgR3mMAeYX.jpg b/yolov5/data/images/video-6tLtjdkv5K5BuhB37-frame-000044-Z5MfsCQMgR3mMAeYX.jpg
new file mode 100644
index 0000000000000000000000000000000000000000..32cb2ab81db52309c0a9bf352e740a0539e7c891
Binary files /dev/null and b/yolov5/data/images/video-6tLtjdkv5K5BuhB37-frame-000044-Z5MfsCQMgR3mMAeYX.jpg differ
diff --git a/yolov5/data/images/video-6tLtjdkv5K5BuhB37-frame-000045-yQ8azA2FYFFJpscM8.jpg b/yolov5/data/images/video-6tLtjdkv5K5BuhB37-frame-000045-yQ8azA2FYFFJpscM8.jpg
new file mode 100644
index 0000000000000000000000000000000000000000..645dff8e07602bd13d431bcd23c2ed474be4f2ec
Binary files /dev/null and b/yolov5/data/images/video-6tLtjdkv5K5BuhB37-frame-000045-yQ8azA2FYFFJpscM8.jpg differ
diff --git a/yolov5/data/images/video-6tLtjdkv5K5BuhB37-frame-000046-mz6XiyZJZeRtyFQvu.jpg b/yolov5/data/images/video-6tLtjdkv5K5BuhB37-frame-000046-mz6XiyZJZeRtyFQvu.jpg
new file mode 100644
index 0000000000000000000000000000000000000000..fec2fd90e78d7a96bb5fcc4190f0b3d9379938dd
Binary files /dev/null and b/yolov5/data/images/video-6tLtjdkv5K5BuhB37-frame-000046-mz6XiyZJZeRtyFQvu.jpg differ
diff --git a/yolov5/data/images/video-6tLtjdkv5K5BuhB37-frame-000047-yiafE6z9N5PtfHnSH.jpg b/yolov5/data/images/video-6tLtjdkv5K5BuhB37-frame-000047-yiafE6z9N5PtfHnSH.jpg
new file mode 100644
index 0000000000000000000000000000000000000000..6603af4b505874c8b841dd259a77cb7d8578a76c
Binary files /dev/null and b/yolov5/data/images/video-6tLtjdkv5K5BuhB37-frame-000047-yiafE6z9N5PtfHnSH.jpg differ
diff --git a/yolov5/data/images/video-6tLtjdkv5K5BuhB37-frame-000048-uZnkEjQhZvpj9wqS7.jpg b/yolov5/data/images/video-6tLtjdkv5K5BuhB37-frame-000048-uZnkEjQhZvpj9wqS7.jpg
new file mode 100644
index 0000000000000000000000000000000000000000..afd7465346b77555e627ccf1f34f1766077e7a7b
Binary files /dev/null and b/yolov5/data/images/video-6tLtjdkv5K5BuhB37-frame-000048-uZnkEjQhZvpj9wqS7.jpg differ
diff --git a/yolov5/data/images/video-6tLtjdkv5K5BuhB37-frame-000049-ZXap8fc92GtrTZPbW.jpg b/yolov5/data/images/video-6tLtjdkv5K5BuhB37-frame-000049-ZXap8fc92GtrTZPbW.jpg
new file mode 100644
index 0000000000000000000000000000000000000000..9e4940aeba10608dbdae4ca828c68fd6a0f8c761
Binary files /dev/null and b/yolov5/data/images/video-6tLtjdkv5K5BuhB37-frame-000049-ZXap8fc92GtrTZPbW.jpg differ
diff --git a/yolov5/data/images/video-6tLtjdkv5K5BuhB37-frame-000050-hSZ786SKo3GhdfPcA.jpg b/yolov5/data/images/video-6tLtjdkv5K5BuhB37-frame-000050-hSZ786SKo3GhdfPcA.jpg
new file mode 100644
index 0000000000000000000000000000000000000000..12ecfad9645be49be6f51efc5ce43042680edf3d
Binary files /dev/null and b/yolov5/data/images/video-6tLtjdkv5K5BuhB37-frame-000050-hSZ786SKo3GhdfPcA.jpg differ
diff --git a/yolov5/data/images/video-6tLtjdkv5K5BuhB37-frame-000051-p6qknbBumgWvxToxT.jpg b/yolov5/data/images/video-6tLtjdkv5K5BuhB37-frame-000051-p6qknbBumgWvxToxT.jpg
new file mode 100644
index 0000000000000000000000000000000000000000..71ccd6c35792b9e44e4ef768f108f57cabfd1646
Binary files /dev/null and b/yolov5/data/images/video-6tLtjdkv5K5BuhB37-frame-000051-p6qknbBumgWvxToxT.jpg differ
diff --git a/yolov5/data/images/video-6tLtjdkv5K5BuhB37-frame-000052-afEMvoJQLzkgehnax.jpg b/yolov5/data/images/video-6tLtjdkv5K5BuhB37-frame-000052-afEMvoJQLzkgehnax.jpg
new file mode 100644
index 0000000000000000000000000000000000000000..6b8b9198ecb27448827a8ef10464b1c69a8fadcd
Binary files /dev/null and b/yolov5/data/images/video-6tLtjdkv5K5BuhB37-frame-000052-afEMvoJQLzkgehnax.jpg differ
diff --git a/yolov5/data/images/video-6tLtjdkv5K5BuhB37-frame-000053-3DtD28HJ4uGDaEcAS.jpg b/yolov5/data/images/video-6tLtjdkv5K5BuhB37-frame-000053-3DtD28HJ4uGDaEcAS.jpg
new file mode 100644
index 0000000000000000000000000000000000000000..57bdc3d7703982b0907d349b64e6f8315a9c7984
Binary files /dev/null and b/yolov5/data/images/video-6tLtjdkv5K5BuhB37-frame-000053-3DtD28HJ4uGDaEcAS.jpg differ
diff --git a/yolov5/data/images/video-6tLtjdkv5K5BuhB37-frame-000054-j7JbDL2yDt4KNHGDZ.jpg b/yolov5/data/images/video-6tLtjdkv5K5BuhB37-frame-000054-j7JbDL2yDt4KNHGDZ.jpg
new file mode 100644
index 0000000000000000000000000000000000000000..f0b27a8d03622e8eba57b78db29bab780774a5df
Binary files /dev/null and b/yolov5/data/images/video-6tLtjdkv5K5BuhB37-frame-000054-j7JbDL2yDt4KNHGDZ.jpg differ
diff --git a/yolov5/data/images/video-6tLtjdkv5K5BuhB37-frame-000055-n3vz85ez27B8BX9Qd.jpg b/yolov5/data/images/video-6tLtjdkv5K5BuhB37-frame-000055-n3vz85ez27B8BX9Qd.jpg
new file mode 100644
index 0000000000000000000000000000000000000000..632fe9fd1bd18809fe91740aac504175892b124f
Binary files /dev/null and b/yolov5/data/images/video-6tLtjdkv5K5BuhB37-frame-000055-n3vz85ez27B8BX9Qd.jpg differ
diff --git a/yolov5/data/images/video-6tLtjdkv5K5BuhB37-frame-000056-XxAJfrE3rBn6ETSTS.jpg b/yolov5/data/images/video-6tLtjdkv5K5BuhB37-frame-000056-XxAJfrE3rBn6ETSTS.jpg
new file mode 100644
index 0000000000000000000000000000000000000000..b8f95d5c57d574b29238d7129eb1a968165e559c
Binary files /dev/null and b/yolov5/data/images/video-6tLtjdkv5K5BuhB37-frame-000056-XxAJfrE3rBn6ETSTS.jpg differ
diff --git a/yolov5/data/images/video-6tLtjdkv5K5BuhB37-frame-000057-ba65sSn2Gm3zqr3GD.jpg b/yolov5/data/images/video-6tLtjdkv5K5BuhB37-frame-000057-ba65sSn2Gm3zqr3GD.jpg
new file mode 100644
index 0000000000000000000000000000000000000000..531a371c744235e9274dc2332d6712f5d964cfd6
Binary files /dev/null and b/yolov5/data/images/video-6tLtjdkv5K5BuhB37-frame-000057-ba65sSn2Gm3zqr3GD.jpg differ
diff --git a/yolov5/data/images/video-6tLtjdkv5K5BuhB37-frame-000058-XnbHMehqXsxpNdcRi.jpg b/yolov5/data/images/video-6tLtjdkv5K5BuhB37-frame-000058-XnbHMehqXsxpNdcRi.jpg
new file mode 100644
index 0000000000000000000000000000000000000000..d2aba55f7e86257ff49240ce4fe58216c2a4ad53
Binary files /dev/null and b/yolov5/data/images/video-6tLtjdkv5K5BuhB37-frame-000058-XnbHMehqXsxpNdcRi.jpg differ
diff --git a/yolov5/data/images/video-6tLtjdkv5K5BuhB37-frame-000059-hY7B5y9Frdi5FauHJ.jpg b/yolov5/data/images/video-6tLtjdkv5K5BuhB37-frame-000059-hY7B5y9Frdi5FauHJ.jpg
new file mode 100644
index 0000000000000000000000000000000000000000..e2978e9f1fc440780a55c67aebe2ccc4d3d98c33
Binary files /dev/null and b/yolov5/data/images/video-6tLtjdkv5K5BuhB37-frame-000059-hY7B5y9Frdi5FauHJ.jpg differ
diff --git a/yolov5/data/images/video-6tLtjdkv5K5BuhB37-frame-000060-HxavCNELiqedirhFo.jpg b/yolov5/data/images/video-6tLtjdkv5K5BuhB37-frame-000060-HxavCNELiqedirhFo.jpg
new file mode 100644
index 0000000000000000000000000000000000000000..79df7fafe7f0530f5d284ad90e6965b9cb06551d
Binary files /dev/null and b/yolov5/data/images/video-6tLtjdkv5K5BuhB37-frame-000060-HxavCNELiqedirhFo.jpg differ
diff --git a/yolov5/data/images/video-6tLtjdkv5K5BuhB37-frame-000191-HnXJKowwSKf9AtA9K.jpg b/yolov5/data/images/video-6tLtjdkv5K5BuhB37-frame-000191-HnXJKowwSKf9AtA9K.jpg
new file mode 100644
index 0000000000000000000000000000000000000000..5fd1bf7366dd4db5ac562ee582d7e52610f65d79
Binary files /dev/null and b/yolov5/data/images/video-6tLtjdkv5K5BuhB37-frame-000191-HnXJKowwSKf9AtA9K.jpg differ
diff --git a/yolov5/data/images/video-ePoikf5LyTTfqchga-frame-000397-wvZieNFhvhBzzzJuT.jpg b/yolov5/data/images/video-ePoikf5LyTTfqchga-frame-000397-wvZieNFhvhBzzzJuT.jpg
new file mode 100644
index 0000000000000000000000000000000000000000..5c5ef29c7fdca555b5a05c44f3e98ba5f66e0a73
Binary files /dev/null and b/yolov5/data/images/video-ePoikf5LyTTfqchga-frame-000397-wvZieNFhvhBzzzJuT.jpg differ
diff --git a/yolov5/data/images/zidane.jpg b/yolov5/data/images/zidane.jpg
new file mode 100644
index 0000000000000000000000000000000000000000..92d72ea124760ce5dbf9425e3aa8f371e7481328
Binary files /dev/null and b/yolov5/data/images/zidane.jpg differ
diff --git a/yolov5/data/scripts/download_weights.sh b/yolov5/data/scripts/download_weights.sh
new file mode 100644
index 0000000000000000000000000000000000000000..e408959b32b245f5a6bb1291db16afd138c56a37
--- /dev/null
+++ b/yolov5/data/scripts/download_weights.sh
@@ -0,0 +1,22 @@
+#!/bin/bash
+# YOLOv5 🚀 by Ultralytics, AGPL-3.0 license
+# Download latest models from https://github.com/ultralytics/yolov5/releases
+# Example usage: bash data/scripts/download_weights.sh
+# parent
+# └── yolov5
+# ├── yolov5s.pt ← downloads here
+# ├── yolov5m.pt
+# └── ...
+
+python - <= cls >= 0, f'incorrect class index {cls}'
+
+ # Write YOLO label
+ if id not in shapes:
+ shapes[id] = Image.open(file).size
+ box = xyxy2xywhn(box[None].astype(np.float), w=shapes[id][0], h=shapes[id][1], clip=True)
+ with open((labels / id).with_suffix('.txt'), 'a') as f:
+ f.write(f"{cls} {' '.join(f'{x:.6f}' for x in box[0])}\n") # write label.txt
+ except Exception as e:
+ print(f'WARNING: skipping one label for {file}: {e}')
+
+
+ # Download manually from https://challenge.xviewdataset.org
+ dir = Path(yaml['path']) # dataset root dir
+ # urls = ['https://d307kc0mrhucc3.cloudfront.net/train_labels.zip', # train labels
+ # 'https://d307kc0mrhucc3.cloudfront.net/train_images.zip', # 15G, 847 train images
+ # 'https://d307kc0mrhucc3.cloudfront.net/val_images.zip'] # 5G, 282 val images (no labels)
+ # download(urls, dir=dir, delete=False)
+
+ # Convert labels
+ convert_labels(dir / 'xView_train.geojson')
+
+ # Move images
+ images = Path(dir / 'images')
+ images.mkdir(parents=True, exist_ok=True)
+ Path(dir / 'train_images').rename(dir / 'images' / 'train')
+ Path(dir / 'val_images').rename(dir / 'images' / 'val')
+
+ # Split
+ autosplit(dir / 'images' / 'train')
diff --git a/yolov5/datasets/runs/May17_09-16-11_DESKTOP-NGLUKKBtest_tensorboard/_images_arctanx/events.out.tfevents.1684286171.DESKTOP-NGLUKKB.8816.3 b/yolov5/datasets/runs/May17_09-16-11_DESKTOP-NGLUKKBtest_tensorboard/_images_arctanx/events.out.tfevents.1684286171.DESKTOP-NGLUKKB.8816.3
new file mode 100644
index 0000000000000000000000000000000000000000..38e90391fd651b3ec4a87f9b5e9e6af0618a78eb
--- /dev/null
+++ b/yolov5/datasets/runs/May17_09-16-11_DESKTOP-NGLUKKBtest_tensorboard/_images_arctanx/events.out.tfevents.1684286171.DESKTOP-NGLUKKB.8816.3
@@ -0,0 +1,3 @@
+version https://git-lfs.github.com/spec/v1
+oid sha256:76c711e70801814eb48f287989d6077d1a0f3008ca25f06ca80be7ca6d775def
+size 4586
diff --git a/yolov5/datasets/runs/May17_09-16-11_DESKTOP-NGLUKKBtest_tensorboard/_images_xcosx/events.out.tfevents.1684286171.DESKTOP-NGLUKKB.8816.2 b/yolov5/datasets/runs/May17_09-16-11_DESKTOP-NGLUKKBtest_tensorboard/_images_xcosx/events.out.tfevents.1684286171.DESKTOP-NGLUKKB.8816.2
new file mode 100644
index 0000000000000000000000000000000000000000..46e58ae67b28171ad7f80231c52e8fd6b1f4a6be
--- /dev/null
+++ b/yolov5/datasets/runs/May17_09-16-11_DESKTOP-NGLUKKBtest_tensorboard/_images_xcosx/events.out.tfevents.1684286171.DESKTOP-NGLUKKB.8816.2
@@ -0,0 +1,3 @@
+version https://git-lfs.github.com/spec/v1
+oid sha256:52d31bebe5cdaef5644eb8188e5d1bebf868077e2d167be31104fd36cc193676
+size 4586
diff --git a/yolov5/datasets/runs/May17_09-16-11_DESKTOP-NGLUKKBtest_tensorboard/_images_xsinx/events.out.tfevents.1684286171.DESKTOP-NGLUKKB.8816.1 b/yolov5/datasets/runs/May17_09-16-11_DESKTOP-NGLUKKBtest_tensorboard/_images_xsinx/events.out.tfevents.1684286171.DESKTOP-NGLUKKB.8816.1
new file mode 100644
index 0000000000000000000000000000000000000000..9029f9bb0922c5efb6e3b07fae4b58ec24be7dfb
--- /dev/null
+++ b/yolov5/datasets/runs/May17_09-16-11_DESKTOP-NGLUKKBtest_tensorboard/_images_xsinx/events.out.tfevents.1684286171.DESKTOP-NGLUKKB.8816.1
@@ -0,0 +1,3 @@
+version https://git-lfs.github.com/spec/v1
+oid sha256:255d8dccc227f37333e6c61f37e41b6e2cf0664a6ee8be046a75b7a7cb485803
+size 4586
diff --git a/yolov5/datasets/runs/May17_09-16-11_DESKTOP-NGLUKKBtest_tensorboard/events.out.tfevents.1684286171.DESKTOP-NGLUKKB.8816.0 b/yolov5/datasets/runs/May17_09-16-11_DESKTOP-NGLUKKBtest_tensorboard/events.out.tfevents.1684286171.DESKTOP-NGLUKKB.8816.0
new file mode 100644
index 0000000000000000000000000000000000000000..74f3d315128d1daf70ef5387376d91965b3a6b52
--- /dev/null
+++ b/yolov5/datasets/runs/May17_09-16-11_DESKTOP-NGLUKKBtest_tensorboard/events.out.tfevents.1684286171.DESKTOP-NGLUKKB.8816.0
@@ -0,0 +1,3 @@
+version https://git-lfs.github.com/spec/v1
+oid sha256:ebb31737cfc931f7b31ae1ad2dfe62f59e1f226ce62ab3df94728cc316d5dfaf
+size 9184
diff --git a/yolov5/datasets/test.py b/yolov5/datasets/test.py
new file mode 100644
index 0000000000000000000000000000000000000000..fab959aa99ace4169bb37e36fc47efccaa335e79
--- /dev/null
+++ b/yolov5/datasets/test.py
@@ -0,0 +1,12 @@
+from torch.utils.tensorboard import SummaryWriter
+import numpy as np
+writer = SummaryWriter(comment='test_tensorboard')
+for x in range(100):
+ writer.add_scalar('y=2x', x * 2, x)
+ writer.add_scalar('y=pow(2, x)', 2 ** x, x)
+
+ writer.add_scalars('/images', {"xsinx": x * np.sin(x),
+ "xcosx": x * np.cos(x),
+ "arctanx": np.arctan(x)}, x)
+writer.close()
+
diff --git a/yolov5/detect.py b/yolov5/detect.py
new file mode 100644
index 0000000000000000000000000000000000000000..792e12473d6ee06da4f9706957d3f992d98b9e2e
--- /dev/null
+++ b/yolov5/detect.py
@@ -0,0 +1,261 @@
+# YOLOv5 🚀 by Ultralytics, AGPL-3.0 license
+"""
+Run YOLOv5 detection inference on images, videos, directories, globs, YouTube, webcam, streams, etc.
+
+Usage - sources:
+ $ python detect.py --weights yolov5s.pt --source 0 # webcam
+ img.jpg # image
+ vid.mp4 # video
+ screen # screenshot
+ path/ # directory
+ list.txt # list of images
+ list.streams # list of streams
+ 'path/*.jpg' # glob
+ 'https://youtu.be/Zgi9g1ksQHc' # YouTube
+ 'rtsp://example.com/media.mp4' # RTSP, RTMP, HTTP stream
+
+Usage - formats:
+ $ python detect.py --weights yolov5s.pt # PyTorch
+ yolov5s.torchscript # TorchScript
+ yolov5s.onnx # ONNX Runtime or OpenCV DNN with --dnn
+ yolov5s_openvino_model # OpenVINO
+ yolov5s.engine # TensorRT
+ yolov5s.mlmodel # CoreML (macOS-only)
+ yolov5s_saved_model # TensorFlow SavedModel
+ yolov5s.pb # TensorFlow GraphDef
+ yolov5s.tflite # TensorFlow Lite
+ yolov5s_edgetpu.tflite # TensorFlow Edge TPU
+ yolov5s_paddle_model # PaddlePaddle
+"""
+
+import argparse
+import os
+import platform
+import sys
+from pathlib import Path
+
+import torch
+
+FILE = Path(__file__).resolve()
+ROOT = FILE.parents[0] # YOLOv5 root directory
+if str(ROOT) not in sys.path:
+ sys.path.append(str(ROOT)) # add ROOT to PATH
+ROOT = Path(os.path.relpath(ROOT, Path.cwd())) # relative
+
+from models.common import DetectMultiBackend
+from utils.dataloaders import IMG_FORMATS, VID_FORMATS, LoadImages, LoadScreenshots, LoadStreams
+from utils.general import (LOGGER, Profile, check_file, check_img_size, check_imshow, check_requirements, colorstr, cv2,
+ increment_path, non_max_suppression, print_args, scale_boxes, strip_optimizer, xyxy2xywh)
+from utils.plots import Annotator, colors, save_one_box
+from utils.torch_utils import select_device, smart_inference_mode
+
+
+@smart_inference_mode()
+def run(
+ weights=ROOT / 'yolov5s.pt', # model path or triton URL
+ source=ROOT / 'data/images', # file/dir/URL/glob/screen/0(webcam)
+ data=ROOT / 'data/coco128.yaml', # dataset.yaml path
+ imgsz=(640, 640), # inference size (height, width)
+ conf_thres=0.25, # confidence threshold
+ iou_thres=0.45, # NMS IOU threshold
+ max_det=1000, # maximum detections per image
+ device='', # cuda device, i.e. 0 or 0,1,2,3 or cpu
+ view_img=False, # show results
+ save_txt=False, # save results to *.txt
+ save_conf=False, # save confidences in --save-txt labels
+ save_crop=False, # save cropped prediction boxes
+ nosave=False, # do not save images/videos
+ classes=None, # filter by class: --class 0, or --class 0 2 3
+ agnostic_nms=False, # class-agnostic NMS
+ augment=False, # augmented inference
+ visualize=False, # visualize features
+ update=False, # update all models
+ project=ROOT / 'runs/detect', # save results to project/name
+ name='exp', # save results to project/name
+ exist_ok=False, # existing project/name ok, do not increment
+ line_thickness=1, # bounding box thickness (pixels)
+ hide_labels=False, # hide labels
+ hide_conf=False, # hide confidences
+ half=False, # use FP16 half-precision inference
+ dnn=False, # use OpenCV DNN for ONNX inference
+ vid_stride=1, # video frame-rate stride
+):
+ source = str(source)
+ save_img = not nosave and not source.endswith('.txt') # save inference images
+ is_file = Path(source).suffix[1:] in (IMG_FORMATS + VID_FORMATS)
+ is_url = source.lower().startswith(('rtsp://', 'rtmp://', 'http://', 'https://'))
+ webcam = source.isnumeric() or source.endswith('.streams') or (is_url and not is_file)
+ screenshot = source.lower().startswith('screen')
+ if is_url and is_file:
+ source = check_file(source) # download
+
+ # Directories
+ save_dir = increment_path(Path(project) / name, exist_ok=exist_ok) # increment run
+ (save_dir / 'labels' if save_txt else save_dir).mkdir(parents=True, exist_ok=True) # make dir
+
+ # Load model
+ device = select_device(device)
+ model = DetectMultiBackend(weights, device=device, dnn=dnn, data=data, fp16=half)
+ stride, names, pt = model.stride, model.names, model.pt
+ imgsz = check_img_size(imgsz, s=stride) # check image size
+
+ # Dataloader
+ bs = 1 # batch_size
+ if webcam:
+ view_img = check_imshow(warn=True)
+ dataset = LoadStreams(source, img_size=imgsz, stride=stride, auto=pt, vid_stride=vid_stride)
+ bs = len(dataset)
+ elif screenshot:
+ dataset = LoadScreenshots(source, img_size=imgsz, stride=stride, auto=pt)
+ else:
+ dataset = LoadImages(source, img_size=imgsz, stride=stride, auto=pt, vid_stride=vid_stride)
+ vid_path, vid_writer = [None] * bs, [None] * bs
+
+ # Run inference
+ model.warmup(imgsz=(1 if pt or model.triton else bs, 3, *imgsz)) # warmup
+ seen, windows, dt = 0, [], (Profile(), Profile(), Profile())
+ for path, im, im0s, vid_cap, s in dataset:
+ with dt[0]:
+ im = torch.from_numpy(im).to(model.device)
+ im = im.half() if model.fp16 else im.float() # uint8 to fp16/32
+ im /= 255 # 0 - 255 to 0.0 - 1.0
+ if len(im.shape) == 3:
+ im = im[None] # expand for batch dim
+
+ # Inference
+ with dt[1]:
+ visualize = increment_path(save_dir / Path(path).stem, mkdir=True) if visualize else False
+ pred = model(im, augment=augment, visualize=visualize)
+
+ # NMS
+ with dt[2]:
+ pred = non_max_suppression(pred, conf_thres, iou_thres, classes, agnostic_nms, max_det=max_det)
+
+ # Second-stage classifier (optional)
+ # pred = utils.general.apply_classifier(pred, classifier_model, im, im0s)
+
+ # Process predictions
+ for i, det in enumerate(pred): # per image
+ seen += 1
+ if webcam: # batch_size >= 1
+ p, im0, frame = path[i], im0s[i].copy(), dataset.count
+ s += f'{i}: '
+ else:
+ p, im0, frame = path, im0s.copy(), getattr(dataset, 'frame', 0)
+
+ p = Path(p) # to Path
+ save_path = str(save_dir / p.name) # im.jpg
+ txt_path = str(save_dir / 'labels' / p.stem) + ('' if dataset.mode == 'image' else f'_{frame}') # im.txt
+ s += '%gx%g ' % im.shape[2:] # print string
+ gn = torch.tensor(im0.shape)[[1, 0, 1, 0]] # normalization gain whwh
+ imc = im0.copy() if save_crop else im0 # for save_crop
+ annotator = Annotator(im0, line_width=line_thickness, example=str(names))
+ if len(det):
+ # Rescale boxes from img_size to im0 size
+ det[:, :4] = scale_boxes(im.shape[2:], det[:, :4], im0.shape).round()
+
+ # Print results
+ for c in det[:, 5].unique():
+ n = (det[:, 5] == c).sum() # detections per class
+ s += f"{n} {names[int(c)]}{'s' * (n > 1)}, " # add to string
+
+ # Write results
+ for *xyxy, conf, cls in reversed(det):
+ if save_txt: # Write to file
+ xywh = (xyxy2xywh(torch.tensor(xyxy).view(1, 4)) / gn).view(-1).tolist() # normalized xywh
+ line = (cls, *xywh, conf) if save_conf else (cls, *xywh) # label format
+ with open(f'{txt_path}.txt', 'a') as f:
+ f.write(('%g ' * len(line)).rstrip() % line + '\n')
+
+ if save_img or save_crop or view_img: # Add bbox to image
+ c = int(cls) # integer class
+ label = None if hide_labels else (names[c] if hide_conf else f'{names[c]} {conf:.2f}')
+ annotator.box_label(xyxy, label, color=colors(c, True))
+ if save_crop:
+ save_one_box(xyxy, imc, file=save_dir / 'crops' / names[c] / f'{p.stem}.jpg', BGR=True)
+
+ # Stream results
+ im0 = annotator.result()
+ if view_img:
+ if platform.system() == 'Linux' and p not in windows:
+ windows.append(p)
+ cv2.namedWindow(str(p), cv2.WINDOW_NORMAL | cv2.WINDOW_KEEPRATIO) # allow window resize (Linux)
+ cv2.resizeWindow(str(p), im0.shape[1], im0.shape[0])
+ cv2.imshow(str(p), im0)
+ cv2.waitKey(1) # 1 millisecond
+
+ # Save results (image with detections)
+ if save_img:
+ if dataset.mode == 'image':
+ cv2.imwrite(save_path, im0)
+ else: # 'video' or 'stream'
+ if vid_path[i] != save_path: # new video
+ vid_path[i] = save_path
+ if isinstance(vid_writer[i], cv2.VideoWriter):
+ vid_writer[i].release() # release previous video writer
+ if vid_cap: # video
+ fps = vid_cap.get(cv2.CAP_PROP_FPS)
+ w = int(vid_cap.get(cv2.CAP_PROP_FRAME_WIDTH))
+ h = int(vid_cap.get(cv2.CAP_PROP_FRAME_HEIGHT))
+ else: # stream
+ fps, w, h = 30, im0.shape[1], im0.shape[0]
+ save_path = str(Path(save_path).with_suffix('.mp4')) # force *.mp4 suffix on results videos
+ vid_writer[i] = cv2.VideoWriter(save_path, cv2.VideoWriter_fourcc(*'mp4v'), fps, (w, h))
+ vid_writer[i].write(im0)
+
+ # Print time (inference-only)
+ LOGGER.info(f"{s}{'' if len(det) else '(no detections), '}{dt[1].dt * 1E3:.1f}ms")
+
+ # Print results
+ t = tuple(x.t / seen * 1E3 for x in dt) # speeds per image
+ LOGGER.info(f'Speed: %.1fms pre-process, %.1fms inference, %.1fms NMS per image at shape {(1, 3, *imgsz)}' % t)
+ if save_txt or save_img:
+ s = f"\n{len(list(save_dir.glob('labels/*.txt')))} labels saved to {save_dir / 'labels'}" if save_txt else ''
+ LOGGER.info(f"Results saved to {colorstr('bold', save_dir)}{s}")
+ if update:
+ strip_optimizer(weights[0]) # update model (to fix SourceChangeWarning)
+
+
+def parse_opt():
+ parser = argparse.ArgumentParser()
+ parser.add_argument('--weights', nargs='+', type=str, default=ROOT / 'yolov5s.pt', help='model path or triton URL')
+ parser.add_argument('--source', type=str, default=ROOT / 'data/images', help='file/dir/URL/glob/screen/0(webcam)')
+ parser.add_argument('--data', type=str, default=ROOT / 'data/coco128.yaml', help='(optional) dataset.yaml path')
+ parser.add_argument('--imgsz', '--img', '--img-size', nargs='+', type=int, default=[640], help='inference size h,w')
+ parser.add_argument('--conf-thres', type=float, default=0.25, help='confidence threshold')
+ parser.add_argument('--iou-thres', type=float, default=0.45, help='NMS IoU threshold')
+ parser.add_argument('--max-det', type=int, default=1000, help='maximum detections per image')
+ parser.add_argument('--device', default='', help='cuda device, i.e. 0 or 0,1,2,3 or cpu')
+ parser.add_argument('--view-img', action='store_true', help='show results')
+ parser.add_argument('--save-txt', action='store_true', help='save results to *.txt')
+ parser.add_argument('--save-conf', action='store_true', help='save confidences in --save-txt labels')
+ parser.add_argument('--save-crop', action='store_true', help='save cropped prediction boxes')
+ parser.add_argument('--nosave', action='store_true', help='do not save images/videos')
+ parser.add_argument('--classes', nargs='+', type=int, help='filter by class: --classes 0, or --classes 0 2 3')
+ parser.add_argument('--agnostic-nms', action='store_true', help='class-agnostic NMS')
+ parser.add_argument('--augment', action='store_true', help='augmented inference')
+ parser.add_argument('--visualize', action='store_true', help='visualize features')
+ parser.add_argument('--update', action='store_true', help='update all models')
+ parser.add_argument('--project', default=ROOT / 'runs/detect', help='save results to project/name')
+ parser.add_argument('--name', default='exp', help='save results to project/name')
+ parser.add_argument('--exist-ok', action='store_true', help='existing project/name ok, do not increment')
+ parser.add_argument('--line-thickness', default=3, type=int, help='bounding box thickness (pixels)')
+ parser.add_argument('--hide-labels', default=False, action='store_true', help='hide labels')
+ parser.add_argument('--hide-conf', default=False, action='store_true', help='hide confidences')
+ parser.add_argument('--half', action='store_true', help='use FP16 half-precision inference')
+ parser.add_argument('--dnn', action='store_true', help='use OpenCV DNN for ONNX inference')
+ parser.add_argument('--vid-stride', type=int, default=1, help='video frame-rate stride')
+ opt = parser.parse_args()
+ opt.imgsz *= 2 if len(opt.imgsz) == 1 else 1 # expand
+ print_args(vars(opt))
+ return opt
+
+
+def main(opt):
+ check_requirements(exclude=('tensorboard', 'thop'))
+ run(**vars(opt))
+
+
+if __name__ == '__main__':
+ opt = parse_opt()
+ main(opt)
diff --git a/yolov5/export.py b/yolov5/export.py
new file mode 100644
index 0000000000000000000000000000000000000000..067d9a22f292bf53004f9470f0198977a6880fab
--- /dev/null
+++ b/yolov5/export.py
@@ -0,0 +1,818 @@
+# YOLOv5 🚀 by Ultralytics, AGPL-3.0 license
+"""
+Export a YOLOv5 PyTorch model to other formats. TensorFlow exports authored by https://github.com/zldrobit
+
+Format | `export.py --include` | Model
+--- | --- | ---
+PyTorch | - | yolov5s.pt
+TorchScript | `torchscript` | yolov5s.torchscript
+ONNX | `onnx` | yolov5s.onnx
+OpenVINO | `openvino` | yolov5s_openvino_model/
+TensorRT | `engine` | yolov5s.engine
+CoreML | `coreml` | yolov5s.mlmodel
+TensorFlow SavedModel | `saved_model` | yolov5s_saved_model/
+TensorFlow GraphDef | `pb` | yolov5s.pb
+TensorFlow Lite | `tflite` | yolov5s.tflite
+TensorFlow Edge TPU | `edgetpu` | yolov5s_edgetpu.tflite
+TensorFlow.js | `tfjs` | yolov5s_web_model/
+PaddlePaddle | `paddle` | yolov5s_paddle_model/
+
+Requirements:
+ $ pip install -r requirements.txt coremltools onnx onnx-simplifier onnxruntime openvino-dev tensorflow-cpu # CPU
+ $ pip install -r requirements.txt coremltools onnx onnx-simplifier onnxruntime-gpu openvino-dev tensorflow # GPU
+
+Usage:
+ $ python export.py --weights yolov5s.pt --include torchscript onnx openvino engine coreml tflite ...
+
+Inference:
+ $ python detect.py --weights yolov5s.pt # PyTorch
+ yolov5s.torchscript # TorchScript
+ yolov5s.onnx # ONNX Runtime or OpenCV DNN with --dnn
+ yolov5s_openvino_model # OpenVINO
+ yolov5s.engine # TensorRT
+ yolov5s.mlmodel # CoreML (macOS-only)
+ yolov5s_saved_model # TensorFlow SavedModel
+ yolov5s.pb # TensorFlow GraphDef
+ yolov5s.tflite # TensorFlow Lite
+ yolov5s_edgetpu.tflite # TensorFlow Edge TPU
+ yolov5s_paddle_model # PaddlePaddle
+
+TensorFlow.js:
+ $ cd .. && git clone https://github.com/zldrobit/tfjs-yolov5-example.git && cd tfjs-yolov5-example
+ $ npm install
+ $ ln -s ../../yolov5/yolov5s_web_model public/yolov5s_web_model
+ $ npm start
+"""
+
+import argparse
+import contextlib
+import json
+import os
+import platform
+import re
+import subprocess
+import sys
+import time
+import warnings
+from pathlib import Path
+
+import pandas as pd
+import torch
+from torch.utils.mobile_optimizer import optimize_for_mobile
+
+FILE = Path(__file__).resolve()
+ROOT = FILE.parents[0] # YOLOv5 root directory
+if str(ROOT) not in sys.path:
+ sys.path.append(str(ROOT)) # add ROOT to PATH
+if platform.system() != 'Windows':
+ ROOT = Path(os.path.relpath(ROOT, Path.cwd())) # relative
+
+from yolov5.models.experimental import attempt_load
+from yolov5.models.yolo import ClassificationModel, Detect, DetectionModel, SegmentationModel
+from yolov5.utils.dataloaders import LoadImages
+from yolov5.utils.general import (LOGGER, Profile, check_dataset, check_img_size, check_requirements, check_version,
+ check_yaml, colorstr, file_size, get_default_args, print_args, url2file, yaml_save)
+from yolov5.utils.torch_utils import select_device, smart_inference_mode
+
+MACOS = platform.system() == 'Darwin' # macOS environment
+
+
+class iOSModel(torch.nn.Module):
+
+ def __init__(self, model, im):
+ super().__init__()
+ b, c, h, w = im.shape # batch, channel, height, width
+ self.model = model
+ self.nc = model.nc # number of classes
+ if w == h:
+ self.normalize = 1. / w
+ else:
+ self.normalize = torch.tensor([1. / w, 1. / h, 1. / w, 1. / h]) # broadcast (slower, smaller)
+ # np = model(im)[0].shape[1] # number of points
+ # self.normalize = torch.tensor([1. / w, 1. / h, 1. / w, 1. / h]).expand(np, 4) # explicit (faster, larger)
+
+ def forward(self, x):
+ xywh, conf, cls = self.model(x)[0].squeeze().split((4, 1, self.nc), 1)
+ return cls * conf, xywh * self.normalize # confidence (3780, 80), coordinates (3780, 4)
+
+
+def export_formats():
+ # YOLOv5 export formats
+ x = [
+ ['PyTorch', '-', '.pt', True, True],
+ ['TorchScript', 'torchscript', '.torchscript', True, True],
+ ['ONNX', 'onnx', '.onnx', True, True],
+ ['OpenVINO', 'openvino', '_openvino_model', True, False],
+ ['TensorRT', 'engine', '.engine', False, True],
+ ['CoreML', 'coreml', '.mlmodel', True, False],
+ ['TensorFlow SavedModel', 'saved_model', '_saved_model', True, True],
+ ['TensorFlow GraphDef', 'pb', '.pb', True, True],
+ ['TensorFlow Lite', 'tflite', '.tflite', True, False],
+ ['TensorFlow Edge TPU', 'edgetpu', '_edgetpu.tflite', False, False],
+ ['TensorFlow.js', 'tfjs', '_web_model', False, False],
+ ['PaddlePaddle', 'paddle', '_paddle_model', True, True],]
+ return pd.DataFrame(x, columns=['Format', 'Argument', 'Suffix', 'CPU', 'GPU'])
+
+
+def try_export(inner_func):
+ # YOLOv5 export decorator, i..e @try_export
+ inner_args = get_default_args(inner_func)
+
+ def outer_func(*args, **kwargs):
+ prefix = inner_args['prefix']
+ try:
+ with Profile() as dt:
+ f, model = inner_func(*args, **kwargs)
+ LOGGER.info(f'{prefix} export success ✅ {dt.t:.1f}s, saved as {f} ({file_size(f):.1f} MB)')
+ return f, model
+ except Exception as e:
+ LOGGER.info(f'{prefix} export failure ❌ {dt.t:.1f}s: {e}')
+ return None, None
+
+ return outer_func
+
+
+@try_export
+def export_torchscript(model, im, file, optimize, prefix=colorstr('TorchScript:')):
+ # YOLOv5 TorchScript model export
+ LOGGER.info(f'\n{prefix} starting export with torch {torch.__version__}...')
+ f = file.with_suffix('.torchscript')
+
+ ts = torch.jit.trace(model, im, strict=False)
+ d = {'shape': im.shape, 'stride': int(max(model.stride)), 'names': model.names}
+ extra_files = {'config.txt': json.dumps(d)} # torch._C.ExtraFilesMap()
+ if optimize: # https://pytorch.org/tutorials/recipes/mobile_interpreter.html
+ optimize_for_mobile(ts)._save_for_lite_interpreter(str(f), _extra_files=extra_files)
+ else:
+ ts.save(str(f), _extra_files=extra_files)
+ return f, None
+
+
+@try_export
+def export_onnx(model, im, file, opset, dynamic, simplify, prefix=colorstr('ONNX:')):
+ # YOLOv5 ONNX export
+ check_requirements('onnx>=1.12.0')
+ import onnx
+
+ LOGGER.info(f'\n{prefix} starting export with onnx {onnx.__version__}...')
+ f = file.with_suffix('.onnx')
+
+ output_names = ['output0', 'output1'] if isinstance(model, SegmentationModel) else ['output0']
+ if dynamic:
+ dynamic = {'images': {0: 'batch', 2: 'height', 3: 'width'}} # shape(1,3,640,640)
+ if isinstance(model, SegmentationModel):
+ dynamic['output0'] = {0: 'batch', 1: 'anchors'} # shape(1,25200,85)
+ dynamic['output1'] = {0: 'batch', 2: 'mask_height', 3: 'mask_width'} # shape(1,32,160,160)
+ elif isinstance(model, DetectionModel):
+ dynamic['output0'] = {0: 'batch', 1: 'anchors'} # shape(1,25200,85)
+
+ torch.onnx.export(
+ model.cpu() if dynamic else model, # --dynamic only compatible with cpu
+ im.cpu() if dynamic else im,
+ f,
+ verbose=False,
+ opset_version=opset,
+ do_constant_folding=True, # WARNING: DNN inference with torch>=1.12 may require do_constant_folding=False
+ input_names=['images'],
+ output_names=output_names,
+ dynamic_axes=dynamic or None)
+
+ # Checks
+ model_onnx = onnx.load(f) # load onnx model
+ onnx.checker.check_model(model_onnx) # check onnx model
+
+ # Metadata
+ d = {'stride': int(max(model.stride)), 'names': model.names}
+ for k, v in d.items():
+ meta = model_onnx.metadata_props.add()
+ meta.key, meta.value = k, str(v)
+ onnx.save(model_onnx, f)
+
+ # Simplify
+ if simplify:
+ try:
+ cuda = torch.cuda.is_available()
+ check_requirements(('onnxruntime-gpu' if cuda else 'onnxruntime', 'onnx-simplifier>=0.4.1'))
+ import onnxsim
+
+ LOGGER.info(f'{prefix} simplifying with onnx-simplifier {onnxsim.__version__}...')
+ model_onnx, check = onnxsim.simplify(model_onnx)
+ assert check, 'assert check failed'
+ onnx.save(model_onnx, f)
+ except Exception as e:
+ LOGGER.info(f'{prefix} simplifier failure: {e}')
+ return f, model_onnx
+
+
+@try_export
+def export_openvino(file, metadata, half, prefix=colorstr('OpenVINO:')):
+ # YOLOv5 OpenVINO export
+ check_requirements('openvino-dev') # requires openvino-dev: https://pypi.org/project/openvino-dev/
+ import openvino.inference_engine as ie
+
+ LOGGER.info(f'\n{prefix} starting export with openvino {ie.__version__}...')
+ f = str(file).replace('.pt', f'_openvino_model{os.sep}')
+
+ args = [
+ 'mo',
+ '--input_model',
+ str(file.with_suffix('.onnx')),
+ '--output_dir',
+ f,
+ '--data_type',
+ ('FP16' if half else 'FP32'),]
+ subprocess.run(args, check=True, env=os.environ) # export
+ yaml_save(Path(f) / file.with_suffix('.yaml').name, metadata) # add metadata.yaml
+ return f, None
+
+
+@try_export
+def export_paddle(model, im, file, metadata, prefix=colorstr('PaddlePaddle:')):
+ # YOLOv5 Paddle export
+ check_requirements(('paddlepaddle', 'x2paddle'))
+ import x2paddle
+ from x2paddle.convert import pytorch2paddle
+
+ LOGGER.info(f'\n{prefix} starting export with X2Paddle {x2paddle.__version__}...')
+ f = str(file).replace('.pt', f'_paddle_model{os.sep}')
+
+ pytorch2paddle(module=model, save_dir=f, jit_type='trace', input_examples=[im]) # export
+ yaml_save(Path(f) / file.with_suffix('.yaml').name, metadata) # add metadata.yaml
+ return f, None
+
+
+@try_export
+def export_coreml(model, im, file, int8, half, nms, prefix=colorstr('CoreML:')):
+ # YOLOv5 CoreML export
+ check_requirements('coremltools')
+ import coremltools as ct
+
+ LOGGER.info(f'\n{prefix} starting export with coremltools {ct.__version__}...')
+ f = file.with_suffix('.mlmodel')
+
+ if nms:
+ model = iOSModel(model, im)
+ ts = torch.jit.trace(model, im, strict=False) # TorchScript model
+ ct_model = ct.convert(ts, inputs=[ct.ImageType('image', shape=im.shape, scale=1 / 255, bias=[0, 0, 0])])
+ bits, mode = (8, 'kmeans_lut') if int8 else (16, 'linear') if half else (32, None)
+ if bits < 32:
+ if MACOS: # quantization only supported on macOS
+ with warnings.catch_warnings():
+ warnings.filterwarnings('ignore', category=DeprecationWarning) # suppress numpy==1.20 float warning
+ ct_model = ct.models.neural_network.quantization_utils.quantize_weights(ct_model, bits, mode)
+ else:
+ print(f'{prefix} quantization only supported on macOS, skipping...')
+ ct_model.save(f)
+ return f, ct_model
+
+
+@try_export
+def export_engine(model, im, file, half, dynamic, simplify, workspace=4, verbose=False, prefix=colorstr('TensorRT:')):
+ # YOLOv5 TensorRT export https://developer.nvidia.com/tensorrt
+ assert im.device.type != 'cpu', 'export running on CPU but must be on GPU, i.e. `python export.py --device 0`'
+ try:
+ import tensorrt as trt
+ except Exception:
+ if platform.system() == 'Linux':
+ check_requirements('nvidia-tensorrt', cmds='-U --index-url https://pypi.ngc.nvidia.com')
+ import tensorrt as trt
+
+ if trt.__version__[0] == '7': # TensorRT 7 handling https://github.com/ultralytics/yolov5/issues/6012
+ grid = model.model[-1].anchor_grid
+ model.model[-1].anchor_grid = [a[..., :1, :1, :] for a in grid]
+ export_onnx(model, im, file, 12, dynamic, simplify) # opset 12
+ model.model[-1].anchor_grid = grid
+ else: # TensorRT >= 8
+ check_version(trt.__version__, '8.0.0', hard=True) # require tensorrt>=8.0.0
+ export_onnx(model, im, file, 12, dynamic, simplify) # opset 12
+ onnx = file.with_suffix('.onnx')
+
+ LOGGER.info(f'\n{prefix} starting export with TensorRT {trt.__version__}...')
+ assert onnx.exists(), f'failed to export ONNX file: {onnx}'
+ f = file.with_suffix('.engine') # TensorRT engine file
+ logger = trt.Logger(trt.Logger.INFO)
+ if verbose:
+ logger.min_severity = trt.Logger.Severity.VERBOSE
+
+ builder = trt.Builder(logger)
+ config = builder.create_builder_config()
+ config.max_workspace_size = workspace * 1 << 30
+ # config.set_memory_pool_limit(trt.MemoryPoolType.WORKSPACE, workspace << 30) # fix TRT 8.4 deprecation notice
+
+ flag = (1 << int(trt.NetworkDefinitionCreationFlag.EXPLICIT_BATCH))
+ network = builder.create_network(flag)
+ parser = trt.OnnxParser(network, logger)
+ if not parser.parse_from_file(str(onnx)):
+ raise RuntimeError(f'failed to load ONNX file: {onnx}')
+
+ inputs = [network.get_input(i) for i in range(network.num_inputs)]
+ outputs = [network.get_output(i) for i in range(network.num_outputs)]
+ for inp in inputs:
+ LOGGER.info(f'{prefix} input "{inp.name}" with shape{inp.shape} {inp.dtype}')
+ for out in outputs:
+ LOGGER.info(f'{prefix} output "{out.name}" with shape{out.shape} {out.dtype}')
+
+ if dynamic:
+ if im.shape[0] <= 1:
+ LOGGER.warning(f'{prefix} WARNING ⚠️ --dynamic model requires maximum --batch-size argument')
+ profile = builder.create_optimization_profile()
+ for inp in inputs:
+ profile.set_shape(inp.name, (1, *im.shape[1:]), (max(1, im.shape[0] // 2), *im.shape[1:]), im.shape)
+ config.add_optimization_profile(profile)
+
+ LOGGER.info(f'{prefix} building FP{16 if builder.platform_has_fast_fp16 and half else 32} engine as {f}')
+ if builder.platform_has_fast_fp16 and half:
+ config.set_flag(trt.BuilderFlag.FP16)
+ with builder.build_engine(network, config) as engine, open(f, 'wb') as t:
+ t.write(engine.serialize())
+ return f, None
+
+
+@try_export
+def export_saved_model(model,
+ im,
+ file,
+ dynamic,
+ tf_nms=False,
+ agnostic_nms=False,
+ topk_per_class=100,
+ topk_all=100,
+ iou_thres=0.45,
+ conf_thres=0.25,
+ keras=False,
+ prefix=colorstr('TensorFlow SavedModel:')):
+ # YOLOv5 TensorFlow SavedModel export
+ try:
+ import tensorflow as tf
+ except Exception:
+ check_requirements(f"tensorflow{'' if torch.cuda.is_available() else '-macos' if MACOS else '-cpu'}")
+ import tensorflow as tf
+ from tensorflow.python.framework.convert_to_constants import convert_variables_to_constants_v2
+
+ from models.tf import TFModel
+
+ LOGGER.info(f'\n{prefix} starting export with tensorflow {tf.__version__}...')
+ f = str(file).replace('.pt', '_saved_model')
+ batch_size, ch, *imgsz = list(im.shape) # BCHW
+
+ tf_model = TFModel(cfg=model.yaml, model=model, nc=model.nc, imgsz=imgsz)
+ im = tf.zeros((batch_size, *imgsz, ch)) # BHWC order for TensorFlow
+ _ = tf_model.predict(im, tf_nms, agnostic_nms, topk_per_class, topk_all, iou_thres, conf_thres)
+ inputs = tf.keras.Input(shape=(*imgsz, ch), batch_size=None if dynamic else batch_size)
+ outputs = tf_model.predict(inputs, tf_nms, agnostic_nms, topk_per_class, topk_all, iou_thres, conf_thres)
+ keras_model = tf.keras.Model(inputs=inputs, outputs=outputs)
+ keras_model.trainable = False
+ keras_model.summary()
+ if keras:
+ keras_model.save(f, save_format='tf')
+ else:
+ spec = tf.TensorSpec(keras_model.inputs[0].shape, keras_model.inputs[0].dtype)
+ m = tf.function(lambda x: keras_model(x)) # full model
+ m = m.get_concrete_function(spec)
+ frozen_func = convert_variables_to_constants_v2(m)
+ tfm = tf.Module()
+ tfm.__call__ = tf.function(lambda x: frozen_func(x)[:4] if tf_nms else frozen_func(x), [spec])
+ tfm.__call__(im)
+ tf.saved_model.save(tfm,
+ f,
+ options=tf.saved_model.SaveOptions(experimental_custom_gradients=False) if check_version(
+ tf.__version__, '2.6') else tf.saved_model.SaveOptions())
+ return f, keras_model
+
+
+@try_export
+def export_pb(keras_model, file, prefix=colorstr('TensorFlow GraphDef:')):
+ # YOLOv5 TensorFlow GraphDef *.pb export https://github.com/leimao/Frozen_Graph_TensorFlow
+ import tensorflow as tf
+ from tensorflow.python.framework.convert_to_constants import convert_variables_to_constants_v2
+
+ LOGGER.info(f'\n{prefix} starting export with tensorflow {tf.__version__}...')
+ f = file.with_suffix('.pb')
+
+ m = tf.function(lambda x: keras_model(x)) # full model
+ m = m.get_concrete_function(tf.TensorSpec(keras_model.inputs[0].shape, keras_model.inputs[0].dtype))
+ frozen_func = convert_variables_to_constants_v2(m)
+ frozen_func.graph.as_graph_def()
+ tf.io.write_graph(graph_or_graph_def=frozen_func.graph, logdir=str(f.parent), name=f.name, as_text=False)
+ return f, None
+
+
+@try_export
+def export_tflite(keras_model, im, file, int8, data, nms, agnostic_nms, prefix=colorstr('TensorFlow Lite:')):
+ # YOLOv5 TensorFlow Lite export
+ import tensorflow as tf
+
+ LOGGER.info(f'\n{prefix} starting export with tensorflow {tf.__version__}...')
+ batch_size, ch, *imgsz = list(im.shape) # BCHW
+ f = str(file).replace('.pt', '-fp16.tflite')
+
+ converter = tf.lite.TFLiteConverter.from_keras_model(keras_model)
+ converter.target_spec.supported_ops = [tf.lite.OpsSet.TFLITE_BUILTINS]
+ converter.target_spec.supported_types = [tf.float16]
+ converter.optimizations = [tf.lite.Optimize.DEFAULT]
+ if int8:
+ from models.tf import representative_dataset_gen
+ dataset = LoadImages(check_dataset(check_yaml(data))['train'], img_size=imgsz, auto=False)
+ converter.representative_dataset = lambda: representative_dataset_gen(dataset, ncalib=100)
+ converter.target_spec.supported_ops = [tf.lite.OpsSet.TFLITE_BUILTINS_INT8]
+ converter.target_spec.supported_types = []
+ converter.inference_input_type = tf.uint8 # or tf.int8
+ converter.inference_output_type = tf.uint8 # or tf.int8
+ converter.experimental_new_quantizer = True
+ f = str(file).replace('.pt', '-int8.tflite')
+ if nms or agnostic_nms:
+ converter.target_spec.supported_ops.append(tf.lite.OpsSet.SELECT_TF_OPS)
+
+ tflite_model = converter.convert()
+ open(f, 'wb').write(tflite_model)
+ return f, None
+
+
+@try_export
+def export_edgetpu(file, prefix=colorstr('Edge TPU:')):
+ # YOLOv5 Edge TPU export https://coral.ai/docs/edgetpu/models-intro/
+ cmd = 'edgetpu_compiler --version'
+ help_url = 'https://coral.ai/docs/edgetpu/compiler/'
+ assert platform.system() == 'Linux', f'export only supported on Linux. See {help_url}'
+ if subprocess.run(f'{cmd} > /dev/null 2>&1', shell=True).returncode != 0:
+ LOGGER.info(f'\n{prefix} export requires Edge TPU compiler. Attempting install from {help_url}')
+ sudo = subprocess.run('sudo --version >/dev/null', shell=True).returncode == 0 # sudo installed on system
+ for c in (
+ 'curl https://packages.cloud.google.com/apt/doc/apt-key.gpg | sudo apt-key add -',
+ 'echo "deb https://packages.cloud.google.com/apt coral-edgetpu-stable main" | sudo tee /etc/apt/sources.list.d/coral-edgetpu.list',
+ 'sudo apt-get update', 'sudo apt-get install edgetpu-compiler'):
+ subprocess.run(c if sudo else c.replace('sudo ', ''), shell=True, check=True)
+ ver = subprocess.run(cmd, shell=True, capture_output=True, check=True).stdout.decode().split()[-1]
+
+ LOGGER.info(f'\n{prefix} starting export with Edge TPU compiler {ver}...')
+ f = str(file).replace('.pt', '-int8_edgetpu.tflite') # Edge TPU model
+ f_tfl = str(file).replace('.pt', '-int8.tflite') # TFLite model
+
+ subprocess.run([
+ 'edgetpu_compiler',
+ '-s',
+ '-d',
+ '-k',
+ '10',
+ '--out_dir',
+ str(file.parent),
+ f_tfl,], check=True)
+ return f, None
+
+
+@try_export
+def export_tfjs(file, int8, prefix=colorstr('TensorFlow.js:')):
+ # YOLOv5 TensorFlow.js export
+ check_requirements('tensorflowjs')
+ import tensorflowjs as tfjs
+
+ LOGGER.info(f'\n{prefix} starting export with tensorflowjs {tfjs.__version__}...')
+ f = str(file).replace('.pt', '_web_model') # js dir
+ f_pb = file.with_suffix('.pb') # *.pb path
+ f_json = f'{f}/model.json' # *.json path
+
+ args = [
+ 'tensorflowjs_converter',
+ '--input_format=tf_frozen_model',
+ '--quantize_uint8' if int8 else '',
+ '--output_node_names=Identity,Identity_1,Identity_2,Identity_3',
+ str(f_pb),
+ str(f),]
+ subprocess.run([arg for arg in args if arg], check=True)
+
+ json = Path(f_json).read_text()
+ with open(f_json, 'w') as j: # sort JSON Identity_* in ascending order
+ subst = re.sub(
+ r'{"outputs": {"Identity.?.?": {"name": "Identity.?.?"}, '
+ r'"Identity.?.?": {"name": "Identity.?.?"}, '
+ r'"Identity.?.?": {"name": "Identity.?.?"}, '
+ r'"Identity.?.?": {"name": "Identity.?.?"}}}', r'{"outputs": {"Identity": {"name": "Identity"}, '
+ r'"Identity_1": {"name": "Identity_1"}, '
+ r'"Identity_2": {"name": "Identity_2"}, '
+ r'"Identity_3": {"name": "Identity_3"}}}', json)
+ j.write(subst)
+ return f, None
+
+
+def add_tflite_metadata(file, metadata, num_outputs):
+ # Add metadata to *.tflite models per https://www.tensorflow.org/lite/models/convert/metadata
+ with contextlib.suppress(ImportError):
+ # check_requirements('tflite_support')
+ from tflite_support import flatbuffers
+ from tflite_support import metadata as _metadata
+ from tflite_support import metadata_schema_py_generated as _metadata_fb
+
+ tmp_file = Path('/tmp/meta.txt')
+ with open(tmp_file, 'w') as meta_f:
+ meta_f.write(str(metadata))
+
+ model_meta = _metadata_fb.ModelMetadataT()
+ label_file = _metadata_fb.AssociatedFileT()
+ label_file.name = tmp_file.name
+ model_meta.associatedFiles = [label_file]
+
+ subgraph = _metadata_fb.SubGraphMetadataT()
+ subgraph.inputTensorMetadata = [_metadata_fb.TensorMetadataT()]
+ subgraph.outputTensorMetadata = [_metadata_fb.TensorMetadataT()] * num_outputs
+ model_meta.subgraphMetadata = [subgraph]
+
+ b = flatbuffers.Builder(0)
+ b.Finish(model_meta.Pack(b), _metadata.MetadataPopulator.METADATA_FILE_IDENTIFIER)
+ metadata_buf = b.Output()
+
+ populator = _metadata.MetadataPopulator.with_model_file(file)
+ populator.load_metadata_buffer(metadata_buf)
+ populator.load_associated_files([str(tmp_file)])
+ populator.populate()
+ tmp_file.unlink()
+
+
+def pipeline_coreml(model, im, file, names, y, prefix=colorstr('CoreML Pipeline:')):
+ # YOLOv5 CoreML pipeline
+ import coremltools as ct
+ from PIL import Image
+
+ print(f'{prefix} starting pipeline with coremltools {ct.__version__}...')
+ batch_size, ch, h, w = list(im.shape) # BCHW
+ t = time.time()
+
+ # Output shapes
+ spec = model.get_spec()
+ out0, out1 = iter(spec.description.output)
+ if platform.system() == 'Darwin':
+ img = Image.new('RGB', (w, h)) # img(192 width, 320 height)
+ # img = torch.zeros((*opt.img_size, 3)).numpy() # img size(320,192,3) iDetection
+ out = model.predict({'image': img})
+ out0_shape, out1_shape = out[out0.name].shape, out[out1.name].shape
+ else: # linux and windows can not run model.predict(), get sizes from pytorch output y
+ s = tuple(y[0].shape)
+ out0_shape, out1_shape = (s[1], s[2] - 5), (s[1], 4) # (3780, 80), (3780, 4)
+
+ # Checks
+ nx, ny = spec.description.input[0].type.imageType.width, spec.description.input[0].type.imageType.height
+ na, nc = out0_shape
+ # na, nc = out0.type.multiArrayType.shape # number anchors, classes
+ assert len(names) == nc, f'{len(names)} names found for nc={nc}' # check
+
+ # Define output shapes (missing)
+ out0.type.multiArrayType.shape[:] = out0_shape # (3780, 80)
+ out1.type.multiArrayType.shape[:] = out1_shape # (3780, 4)
+ # spec.neuralNetwork.preprocessing[0].featureName = '0'
+
+ # Flexible input shapes
+ # from coremltools.models.neural_network import flexible_shape_utils
+ # s = [] # shapes
+ # s.append(flexible_shape_utils.NeuralNetworkImageSize(320, 192))
+ # s.append(flexible_shape_utils.NeuralNetworkImageSize(640, 384)) # (height, width)
+ # flexible_shape_utils.add_enumerated_image_sizes(spec, feature_name='image', sizes=s)
+ # r = flexible_shape_utils.NeuralNetworkImageSizeRange() # shape ranges
+ # r.add_height_range((192, 640))
+ # r.add_width_range((192, 640))
+ # flexible_shape_utils.update_image_size_range(spec, feature_name='image', size_range=r)
+
+ # Print
+ print(spec.description)
+
+ # Model from spec
+ model = ct.models.MLModel(spec)
+
+ # 3. Create NMS protobuf
+ nms_spec = ct.proto.Model_pb2.Model()
+ nms_spec.specificationVersion = 5
+ for i in range(2):
+ decoder_output = model._spec.description.output[i].SerializeToString()
+ nms_spec.description.input.add()
+ nms_spec.description.input[i].ParseFromString(decoder_output)
+ nms_spec.description.output.add()
+ nms_spec.description.output[i].ParseFromString(decoder_output)
+
+ nms_spec.description.output[0].name = 'confidence'
+ nms_spec.description.output[1].name = 'coordinates'
+
+ output_sizes = [nc, 4]
+ for i in range(2):
+ ma_type = nms_spec.description.output[i].type.multiArrayType
+ ma_type.shapeRange.sizeRanges.add()
+ ma_type.shapeRange.sizeRanges[0].lowerBound = 0
+ ma_type.shapeRange.sizeRanges[0].upperBound = -1
+ ma_type.shapeRange.sizeRanges.add()
+ ma_type.shapeRange.sizeRanges[1].lowerBound = output_sizes[i]
+ ma_type.shapeRange.sizeRanges[1].upperBound = output_sizes[i]
+ del ma_type.shape[:]
+
+ nms = nms_spec.nonMaximumSuppression
+ nms.confidenceInputFeatureName = out0.name # 1x507x80
+ nms.coordinatesInputFeatureName = out1.name # 1x507x4
+ nms.confidenceOutputFeatureName = 'confidence'
+ nms.coordinatesOutputFeatureName = 'coordinates'
+ nms.iouThresholdInputFeatureName = 'iouThreshold'
+ nms.confidenceThresholdInputFeatureName = 'confidenceThreshold'
+ nms.iouThreshold = 0.45
+ nms.confidenceThreshold = 0.25
+ nms.pickTop.perClass = True
+ nms.stringClassLabels.vector.extend(names.values())
+ nms_model = ct.models.MLModel(nms_spec)
+
+ # 4. Pipeline models together
+ pipeline = ct.models.pipeline.Pipeline(input_features=[('image', ct.models.datatypes.Array(3, ny, nx)),
+ ('iouThreshold', ct.models.datatypes.Double()),
+ ('confidenceThreshold', ct.models.datatypes.Double())],
+ output_features=['confidence', 'coordinates'])
+ pipeline.add_model(model)
+ pipeline.add_model(nms_model)
+
+ # Correct datatypes
+ pipeline.spec.description.input[0].ParseFromString(model._spec.description.input[0].SerializeToString())
+ pipeline.spec.description.output[0].ParseFromString(nms_model._spec.description.output[0].SerializeToString())
+ pipeline.spec.description.output[1].ParseFromString(nms_model._spec.description.output[1].SerializeToString())
+
+ # Update metadata
+ pipeline.spec.specificationVersion = 5
+ pipeline.spec.description.metadata.versionString = 'https://github.com/ultralytics/yolov5'
+ pipeline.spec.description.metadata.shortDescription = 'https://github.com/ultralytics/yolov5'
+ pipeline.spec.description.metadata.author = 'glenn.jocher@ultralytics.com'
+ pipeline.spec.description.metadata.license = 'https://github.com/ultralytics/yolov5/blob/master/LICENSE'
+ pipeline.spec.description.metadata.userDefined.update({
+ 'classes': ','.join(names.values()),
+ 'iou_threshold': str(nms.iouThreshold),
+ 'confidence_threshold': str(nms.confidenceThreshold)})
+
+ # Save the model
+ f = file.with_suffix('.mlmodel') # filename
+ model = ct.models.MLModel(pipeline.spec)
+ model.input_description['image'] = 'Input image'
+ model.input_description['iouThreshold'] = f'(optional) IOU Threshold override (default: {nms.iouThreshold})'
+ model.input_description['confidenceThreshold'] = \
+ f'(optional) Confidence Threshold override (default: {nms.confidenceThreshold})'
+ model.output_description['confidence'] = 'Boxes × Class confidence (see user-defined metadata "classes")'
+ model.output_description['coordinates'] = 'Boxes × [x, y, width, height] (relative to image size)'
+ model.save(f) # pipelined
+ print(f'{prefix} pipeline success ({time.time() - t:.2f}s), saved as {f} ({file_size(f):.1f} MB)')
+
+
+@smart_inference_mode()
+def run(
+ data=ROOT / 'data/coco128.yaml', # 'dataset.yaml path'
+ weights=ROOT / 'yolov5s.pt', # weights path
+ imgsz=(640, 640), # image (height, width)
+ batch_size=1, # batch size
+ device='cpu', # cuda device, i.e. 0 or 0,1,2,3 or cpu
+ include=('torchscript', 'onnx'), # include formats
+ half=False, # FP16 half-precision export
+ inplace=False, # set YOLOv5 Detect() inplace=True
+ keras=False, # use Keras
+ optimize=False, # TorchScript: optimize for mobile
+ int8=False, # CoreML/TF INT8 quantization
+ dynamic=False, # ONNX/TF/TensorRT: dynamic axes
+ simplify=False, # ONNX: simplify model
+ opset=12, # ONNX: opset version
+ verbose=False, # TensorRT: verbose log
+ workspace=4, # TensorRT: workspace size (GB)
+ nms=False, # TF: add NMS to model
+ agnostic_nms=False, # TF: add agnostic NMS to model
+ topk_per_class=100, # TF.js NMS: topk per class to keep
+ topk_all=100, # TF.js NMS: topk for all classes to keep
+ iou_thres=0.45, # TF.js NMS: IoU threshold
+ conf_thres=0.25, # TF.js NMS: confidence threshold
+):
+ t = time.time()
+ include = [x.lower() for x in include] # to lowercase
+ fmts = tuple(export_formats()['Argument'][1:]) # --include arguments
+ flags = [x in include for x in fmts]
+ assert sum(flags) == len(include), f'ERROR: Invalid --include {include}, valid --include arguments are {fmts}'
+ jit, onnx, xml, engine, coreml, saved_model, pb, tflite, edgetpu, tfjs, paddle = flags # export booleans
+ file = Path(url2file(weights) if str(weights).startswith(('http:/', 'https:/')) else weights) # PyTorch weights
+
+ # Load PyTorch model
+ device = select_device(device)
+ if half:
+ assert device.type != 'cpu' or coreml, '--half only compatible with GPU export, i.e. use --device 0'
+ assert not dynamic, '--half not compatible with --dynamic, i.e. use either --half or --dynamic but not both'
+ model = attempt_load(weights, device=device, inplace=True, fuse=True) # load FP32 model
+
+ # Checks
+ imgsz *= 2 if len(imgsz) == 1 else 1 # expand
+ if optimize:
+ assert device.type == 'cpu', '--optimize not compatible with cuda devices, i.e. use --device cpu'
+
+ # Input
+ gs = int(max(model.stride)) # grid size (max stride)
+ imgsz = [check_img_size(x, gs) for x in imgsz] # verify img_size are gs-multiples
+ im = torch.zeros(batch_size, 3, *imgsz).to(device) # image size(1,3,320,192) BCHW iDetection
+
+ # Update model
+ model.eval()
+ for k, m in model.named_modules():
+ if isinstance(m, Detect):
+ m.inplace = inplace
+ m.dynamic = dynamic
+ m.export = True
+
+ for _ in range(2):
+ y = model(im) # dry runs
+ if half and not coreml:
+ im, model = im.half(), model.half() # to FP16
+ shape = tuple((y[0] if isinstance(y, tuple) else y).shape) # model output shape
+ metadata = {'stride': int(max(model.stride)), 'names': model.names} # model metadata
+ LOGGER.info(f"\n{colorstr('PyTorch:')} starting from {file} with output shape {shape} ({file_size(file):.1f} MB)")
+
+ # Exports
+ f = [''] * len(fmts) # exported filenames
+ warnings.filterwarnings(action='ignore', category=torch.jit.TracerWarning) # suppress TracerWarning
+ if jit: # TorchScript
+ f[0], _ = export_torchscript(model, im, file, optimize)
+ if engine: # TensorRT required before ONNX
+ f[1], _ = export_engine(model, im, file, half, dynamic, simplify, workspace, verbose)
+ if onnx or xml: # OpenVINO requires ONNX
+ f[2], _ = export_onnx(model, im, file, opset, dynamic, simplify)
+ if xml: # OpenVINO
+ f[3], _ = export_openvino(file, metadata, half)
+ if coreml: # CoreML
+ f[4], ct_model = export_coreml(model, im, file, int8, half, nms)
+ if nms:
+ pipeline_coreml(ct_model, im, file, model.names, y)
+ if any((saved_model, pb, tflite, edgetpu, tfjs)): # TensorFlow formats
+ assert not tflite or not tfjs, 'TFLite and TF.js models must be exported separately, please pass only one type.'
+ assert not isinstance(model, ClassificationModel), 'ClassificationModel export to TF formats not yet supported.'
+ f[5], s_model = export_saved_model(model.cpu(),
+ im,
+ file,
+ dynamic,
+ tf_nms=nms or agnostic_nms or tfjs,
+ agnostic_nms=agnostic_nms or tfjs,
+ topk_per_class=topk_per_class,
+ topk_all=topk_all,
+ iou_thres=iou_thres,
+ conf_thres=conf_thres,
+ keras=keras)
+ if pb or tfjs: # pb prerequisite to tfjs
+ f[6], _ = export_pb(s_model, file)
+ if tflite or edgetpu:
+ f[7], _ = export_tflite(s_model, im, file, int8 or edgetpu, data=data, nms=nms, agnostic_nms=agnostic_nms)
+ if edgetpu:
+ f[8], _ = export_edgetpu(file)
+ add_tflite_metadata(f[8] or f[7], metadata, num_outputs=len(s_model.outputs))
+ if tfjs:
+ f[9], _ = export_tfjs(file, int8)
+ if paddle: # PaddlePaddle
+ f[10], _ = export_paddle(model, im, file, metadata)
+
+ # Finish
+ f = [str(x) for x in f if x] # filter out '' and None
+ if any(f):
+ cls, det, seg = (isinstance(model, x) for x in (ClassificationModel, DetectionModel, SegmentationModel)) # type
+ det &= not seg # segmentation models inherit from SegmentationModel(DetectionModel)
+ dir = Path('segment' if seg else 'classify' if cls else '')
+ h = '--half' if half else '' # --half FP16 inference arg
+ s = '# WARNING ⚠️ ClassificationModel not yet supported for PyTorch Hub AutoShape inference' if cls else \
+ '# WARNING ⚠️ SegmentationModel not yet supported for PyTorch Hub AutoShape inference' if seg else ''
+ LOGGER.info(f'\nExport complete ({time.time() - t:.1f}s)'
+ f"\nResults saved to {colorstr('bold', file.parent.resolve())}"
+ f"\nDetect: python {dir / ('detect.py' if det else 'predict.py')} --weights {f[-1]} {h}"
+ f"\nValidate: python {dir / 'val.py'} --weights {f[-1]} {h}"
+ f"\nPyTorch Hub: model = torch.hub.load('ultralytics/yolov5', 'custom', '{f[-1]}') {s}"
+ f'\nVisualize: https://netron.app')
+ return f # return list of exported files/dirs
+
+
+def parse_opt(known=False):
+ parser = argparse.ArgumentParser()
+ parser.add_argument('--data', type=str, default=ROOT / 'data/coco128.yaml', help='dataset.yaml path')
+ parser.add_argument('--weights', nargs='+', type=str, default=ROOT / 'yolov5s.pt', help='model.pt path(s)')
+ parser.add_argument('--imgsz', '--img', '--img-size', nargs='+', type=int, default=[640, 640], help='image (h, w)')
+ parser.add_argument('--batch-size', type=int, default=1, help='batch size')
+ parser.add_argument('--device', default='cpu', help='cuda device, i.e. 0 or 0,1,2,3 or cpu')
+ parser.add_argument('--half', action='store_true', help='FP16 half-precision export')
+ parser.add_argument('--inplace', action='store_true', help='set YOLOv5 Detect() inplace=True')
+ parser.add_argument('--keras', action='store_true', help='TF: use Keras')
+ parser.add_argument('--optimize', action='store_true', help='TorchScript: optimize for mobile')
+ parser.add_argument('--int8', action='store_true', help='CoreML/TF INT8 quantization')
+ parser.add_argument('--dynamic', action='store_true', help='ONNX/TF/TensorRT: dynamic axes')
+ parser.add_argument('--simplify', action='store_true', help='ONNX: simplify model')
+ parser.add_argument('--opset', type=int, default=17, help='ONNX: opset version')
+ parser.add_argument('--verbose', action='store_true', help='TensorRT: verbose log')
+ parser.add_argument('--workspace', type=int, default=4, help='TensorRT: workspace size (GB)')
+ parser.add_argument('--nms', action='store_true', help='TF: add NMS to model')
+ parser.add_argument('--agnostic-nms', action='store_true', help='TF: add agnostic NMS to model')
+ parser.add_argument('--topk-per-class', type=int, default=100, help='TF.js NMS: topk per class to keep')
+ parser.add_argument('--topk-all', type=int, default=100, help='TF.js NMS: topk for all classes to keep')
+ parser.add_argument('--iou-thres', type=float, default=0.45, help='TF.js NMS: IoU threshold')
+ parser.add_argument('--conf-thres', type=float, default=0.25, help='TF.js NMS: confidence threshold')
+ parser.add_argument(
+ '--include',
+ nargs='+',
+ default=['torchscript'],
+ help='torchscript, onnx, openvino, engine, coreml, saved_model, pb, tflite, edgetpu, tfjs, paddle')
+ opt = parser.parse_known_args()[0] if known else parser.parse_args()
+ print_args(vars(opt))
+ return opt
+
+
+def main(opt):
+ for opt.weights in (opt.weights if isinstance(opt.weights, list) else [opt.weights]):
+ run(**vars(opt))
+
+
+if __name__ == '__main__':
+ opt = parse_opt()
+ main(opt)
diff --git a/yolov5/hubconf.py b/yolov5/hubconf.py
new file mode 100644
index 0000000000000000000000000000000000000000..73caf06685da77db0c63cd4e392d86b949c00a97
--- /dev/null
+++ b/yolov5/hubconf.py
@@ -0,0 +1,169 @@
+# YOLOv5 🚀 by Ultralytics, AGPL-3.0 license
+"""
+PyTorch Hub models https://pytorch.org/hub/ultralytics_yolov5
+
+Usage:
+ import torch
+ model = torch.hub.load('ultralytics/yolov5', 'yolov5s') # official model
+ model = torch.hub.load('ultralytics/yolov5:master', 'yolov5s') # from branch
+ model = torch.hub.load('ultralytics/yolov5', 'custom', 'yolov5s.pt') # custom/local model
+ model = torch.hub.load('.', 'custom', 'yolov5s.pt', source='local') # local repo
+"""
+
+import torch
+
+
+def _create(name, pretrained=True, channels=3, classes=80, autoshape=True, verbose=True, device=None):
+ """Creates or loads a YOLOv5 model
+
+ Arguments:
+ name (str): model name 'yolov5s' or path 'path/to/best.pt'
+ pretrained (bool): load pretrained weights into the model
+ channels (int): number of input channels
+ classes (int): number of model classes
+ autoshape (bool): apply YOLOv5 .autoshape() wrapper to model
+ verbose (bool): print all information to screen
+ device (str, torch.device, None): device to use for model parameters
+
+ Returns:
+ YOLOv5 model
+ """
+ from pathlib import Path
+
+ from models.common import AutoShape, DetectMultiBackend
+ from models.experimental import attempt_load
+ from models.yolo import ClassificationModel, DetectionModel, SegmentationModel
+ from utils.downloads import attempt_download
+ from utils.general import LOGGER, check_requirements, intersect_dicts, logging
+ from utils.torch_utils import select_device
+
+ if not verbose:
+ LOGGER.setLevel(logging.WARNING)
+ check_requirements(exclude=('opencv-python', 'tensorboard', 'thop'))
+ name = Path(name)
+ path = name.with_suffix('.pt') if name.suffix == '' and not name.is_dir() else name # checkpoint path
+ try:
+ device = select_device(device)
+ if pretrained and channels == 3 and classes == 80:
+ try:
+ model = DetectMultiBackend(path, device=device, fuse=autoshape) # detection model
+ if autoshape:
+ if model.pt and isinstance(model.model, ClassificationModel):
+ LOGGER.warning('WARNING ⚠️ YOLOv5 ClassificationModel is not yet AutoShape compatible. '
+ 'You must pass torch tensors in BCHW to this model, i.e. shape(1,3,224,224).')
+ elif model.pt and isinstance(model.model, SegmentationModel):
+ LOGGER.warning('WARNING ⚠️ YOLOv5 SegmentationModel is not yet AutoShape compatible. '
+ 'You will not be able to run inference with this model.')
+ else:
+ model = AutoShape(model) # for file/URI/PIL/cv2/np inputs and NMS
+ except Exception:
+ model = attempt_load(path, device=device, fuse=False) # arbitrary model
+ else:
+ cfg = list((Path(__file__).parent / 'models').rglob(f'{path.stem}.yaml'))[0] # model.yaml path
+ model = DetectionModel(cfg, channels, classes) # create model
+ if pretrained:
+ ckpt = torch.load(attempt_download(path), map_location=device) # load
+ csd = ckpt['model'].float().state_dict() # checkpoint state_dict as FP32
+ csd = intersect_dicts(csd, model.state_dict(), exclude=['anchors']) # intersect
+ model.load_state_dict(csd, strict=False) # load
+ if len(ckpt['model'].names) == classes:
+ model.names = ckpt['model'].names # set class names attribute
+ if not verbose:
+ LOGGER.setLevel(logging.INFO) # reset to default
+ return model.to(device)
+
+ except Exception as e:
+ help_url = 'https://docs.ultralytics.com/yolov5/tutorials/pytorch_hub_model_loading'
+ s = f'{e}. Cache may be out of date, try `force_reload=True` or see {help_url} for help.'
+ raise Exception(s) from e
+
+
+def custom(path='path/to/model.pt', autoshape=True, _verbose=True, device=None):
+ # YOLOv5 custom or local model
+ return _create(path, autoshape=autoshape, verbose=_verbose, device=device)
+
+
+def yolov5n(pretrained=True, channels=3, classes=80, autoshape=True, _verbose=True, device=None):
+ # YOLOv5-nano model https://github.com/ultralytics/yolov5
+ return _create('yolov5n', pretrained, channels, classes, autoshape, _verbose, device)
+
+
+def yolov5s(pretrained=True, channels=3, classes=80, autoshape=True, _verbose=True, device=None):
+ # YOLOv5-small model https://github.com/ultralytics/yolov5
+ return _create('yolov5s', pretrained, channels, classes, autoshape, _verbose, device)
+
+
+def yolov5m(pretrained=True, channels=3, classes=80, autoshape=True, _verbose=True, device=None):
+ # YOLOv5-medium model https://github.com/ultralytics/yolov5
+ return _create('yolov5m', pretrained, channels, classes, autoshape, _verbose, device)
+
+
+def yolov5l(pretrained=True, channels=3, classes=80, autoshape=True, _verbose=True, device=None):
+ # YOLOv5-large model https://github.com/ultralytics/yolov5
+ return _create('yolov5l', pretrained, channels, classes, autoshape, _verbose, device)
+
+
+def yolov5x(pretrained=True, channels=3, classes=80, autoshape=True, _verbose=True, device=None):
+ # YOLOv5-xlarge model https://github.com/ultralytics/yolov5
+ return _create('yolov5x', pretrained, channels, classes, autoshape, _verbose, device)
+
+
+def yolov5n6(pretrained=True, channels=3, classes=80, autoshape=True, _verbose=True, device=None):
+ # YOLOv5-nano-P6 model https://github.com/ultralytics/yolov5
+ return _create('yolov5n6', pretrained, channels, classes, autoshape, _verbose, device)
+
+
+def yolov5s6(pretrained=True, channels=3, classes=80, autoshape=True, _verbose=True, device=None):
+ # YOLOv5-small-P6 model https://github.com/ultralytics/yolov5
+ return _create('yolov5s6', pretrained, channels, classes, autoshape, _verbose, device)
+
+
+def yolov5m6(pretrained=True, channels=3, classes=80, autoshape=True, _verbose=True, device=None):
+ # YOLOv5-medium-P6 model https://github.com/ultralytics/yolov5
+ return _create('yolov5m6', pretrained, channels, classes, autoshape, _verbose, device)
+
+
+def yolov5l6(pretrained=True, channels=3, classes=80, autoshape=True, _verbose=True, device=None):
+ # YOLOv5-large-P6 model https://github.com/ultralytics/yolov5
+ return _create('yolov5l6', pretrained, channels, classes, autoshape, _verbose, device)
+
+
+def yolov5x6(pretrained=True, channels=3, classes=80, autoshape=True, _verbose=True, device=None):
+ # YOLOv5-xlarge-P6 model https://github.com/ultralytics/yolov5
+ return _create('yolov5x6', pretrained, channels, classes, autoshape, _verbose, device)
+
+
+if __name__ == '__main__':
+ import argparse
+ from pathlib import Path
+
+ import numpy as np
+ from PIL import Image
+
+ from utils.general import cv2, print_args
+
+ # Argparser
+ parser = argparse.ArgumentParser()
+ parser.add_argument('--model', type=str, default='yolov5s', help='model name')
+ opt = parser.parse_args()
+ print_args(vars(opt))
+
+ # Model
+ model = _create(name=opt.model, pretrained=True, channels=3, classes=80, autoshape=True, verbose=True)
+ # model = custom(path='path/to/model.pt') # custom
+
+ # Images
+ imgs = [
+ 'data/images/zidane.jpg', # filename
+ Path('data/images/zidane.jpg'), # Path
+ 'https://ultralytics.com/images/zidane.jpg', # URI
+ cv2.imread('data/images/bus.jpg')[:, :, ::-1], # OpenCV
+ Image.open('data/images/bus.jpg'), # PIL
+ np.zeros((320, 640, 3))] # numpy
+
+ # Inference
+ results = model(imgs, size=320) # batched inference
+
+ # Results
+ results.print()
+ results.save()
diff --git a/yolov5/models/__init__.py b/yolov5/models/__init__.py
new file mode 100644
index 0000000000000000000000000000000000000000..e69de29bb2d1d6434b8b29ae775ad8c2e48c5391
diff --git a/yolov5/models/__pycache__/__init__.cpython-38.pyc b/yolov5/models/__pycache__/__init__.cpython-38.pyc
new file mode 100644
index 0000000000000000000000000000000000000000..201b0be1e7b16b0da38c04b7821c4270d8660afa
Binary files /dev/null and b/yolov5/models/__pycache__/__init__.cpython-38.pyc differ
diff --git a/yolov5/models/__pycache__/__init__.cpython-39.pyc b/yolov5/models/__pycache__/__init__.cpython-39.pyc
new file mode 100644
index 0000000000000000000000000000000000000000..1e2934992bf072ae51810e1a233430da994a619a
Binary files /dev/null and b/yolov5/models/__pycache__/__init__.cpython-39.pyc differ
diff --git a/yolov5/models/__pycache__/common.cpython-38.pyc b/yolov5/models/__pycache__/common.cpython-38.pyc
new file mode 100644
index 0000000000000000000000000000000000000000..70840eab1adda4358a1bb42aa122c01be5ab2deb
Binary files /dev/null and b/yolov5/models/__pycache__/common.cpython-38.pyc differ
diff --git a/yolov5/models/__pycache__/common.cpython-39.pyc b/yolov5/models/__pycache__/common.cpython-39.pyc
new file mode 100644
index 0000000000000000000000000000000000000000..cc4d87ba7fc3585e43ebcc5d59e4322bec3dfa70
Binary files /dev/null and b/yolov5/models/__pycache__/common.cpython-39.pyc differ
diff --git a/yolov5/models/__pycache__/experimental.cpython-38.pyc b/yolov5/models/__pycache__/experimental.cpython-38.pyc
new file mode 100644
index 0000000000000000000000000000000000000000..04ea5916faadc4be3af14ff694036a616b004a63
Binary files /dev/null and b/yolov5/models/__pycache__/experimental.cpython-38.pyc differ
diff --git a/yolov5/models/__pycache__/experimental.cpython-39.pyc b/yolov5/models/__pycache__/experimental.cpython-39.pyc
new file mode 100644
index 0000000000000000000000000000000000000000..4a6ecb9e6caff1ec001af5bc33211aa7789d30dd
Binary files /dev/null and b/yolov5/models/__pycache__/experimental.cpython-39.pyc differ
diff --git a/yolov5/models/__pycache__/yolo.cpython-38.pyc b/yolov5/models/__pycache__/yolo.cpython-38.pyc
new file mode 100644
index 0000000000000000000000000000000000000000..86681e5c39429e7bcefebc8749870dd9b7fe69ea
Binary files /dev/null and b/yolov5/models/__pycache__/yolo.cpython-38.pyc differ
diff --git a/yolov5/models/__pycache__/yolo.cpython-39.pyc b/yolov5/models/__pycache__/yolo.cpython-39.pyc
new file mode 100644
index 0000000000000000000000000000000000000000..3d54a1a671e81b0cf71b257ab3ae9c74c93a20f8
Binary files /dev/null and b/yolov5/models/__pycache__/yolo.cpython-39.pyc differ
diff --git a/yolov5/models/common.py b/yolov5/models/common.py
new file mode 100644
index 0000000000000000000000000000000000000000..195ccd63a10c64de45de57fc2fe1653d3e745a0e
--- /dev/null
+++ b/yolov5/models/common.py
@@ -0,0 +1,871 @@
+# YOLOv5 🚀 by Ultralytics, AGPL-3.0 license
+"""
+Common modules
+"""
+
+import ast
+import contextlib
+import json
+import math
+import platform
+import warnings
+import zipfile
+from collections import OrderedDict, namedtuple
+from copy import copy
+from pathlib import Path
+from urllib.parse import urlparse
+
+import cv2
+import numpy as np
+import pandas as pd
+import requests
+import torch
+import torch.nn as nn
+from PIL import Image
+from torch.cuda import amp
+
+from yolov5.utils import TryExcept
+from yolov5.utils.dataloaders import exif_transpose, letterbox
+from yolov5.utils.general import (LOGGER, ROOT, Profile, check_requirements, check_suffix, check_version, colorstr,
+ increment_path, is_jupyter, make_divisible, non_max_suppression, scale_boxes, xywh2xyxy,
+ xyxy2xywh, yaml_load)
+from yolov5.utils.plots import Annotator, colors, save_one_box
+from yolov5.utils.torch_utils import copy_attr, smart_inference_mode
+
+
+def autopad(k, p=None, d=1): # kernel, padding, dilation
+ # Pad to 'same' shape outputs
+ if d > 1:
+ k = d * (k - 1) + 1 if isinstance(k, int) else [d * (x - 1) + 1 for x in k] # actual kernel-size
+ if p is None:
+ p = k // 2 if isinstance(k, int) else [x // 2 for x in k] # auto-pad
+ return p
+
+
+class Conv(nn.Module):
+ # Standard convolution with args(ch_in, ch_out, kernel, stride, padding, groups, dilation, activation)
+ default_act = nn.SiLU() # default activation
+
+ def __init__(self, c1, c2, k=1, s=1, p=None, g=1, d=1, act=True):
+ super().__init__()
+ self.conv = nn.Conv2d(c1, c2, k, s, autopad(k, p, d), groups=g, dilation=d, bias=False)
+ self.bn = nn.BatchNorm2d(c2)
+ self.act = self.default_act if act is True else act if isinstance(act, nn.Module) else nn.Identity()
+
+ def forward(self, x):
+ return self.act(self.bn(self.conv(x)))
+
+ def forward_fuse(self, x):
+ return self.act(self.conv(x))
+
+
+class DWConv(Conv):
+ # Depth-wise convolution
+ def __init__(self, c1, c2, k=1, s=1, d=1, act=True): # ch_in, ch_out, kernel, stride, dilation, activation
+ super().__init__(c1, c2, k, s, g=math.gcd(c1, c2), d=d, act=act)
+
+
+class DWConvTranspose2d(nn.ConvTranspose2d):
+ # Depth-wise transpose convolution
+ def __init__(self, c1, c2, k=1, s=1, p1=0, p2=0): # ch_in, ch_out, kernel, stride, padding, padding_out
+ super().__init__(c1, c2, k, s, p1, p2, groups=math.gcd(c1, c2))
+
+
+class TransformerLayer(nn.Module):
+ # Transformer layer https://arxiv.org/abs/2010.11929 (LayerNorm layers removed for better performance)
+ def __init__(self, c, num_heads):
+ super().__init__()
+ self.q = nn.Linear(c, c, bias=False)
+ self.k = nn.Linear(c, c, bias=False)
+ self.v = nn.Linear(c, c, bias=False)
+ self.ma = nn.MultiheadAttention(embed_dim=c, num_heads=num_heads)
+ self.fc1 = nn.Linear(c, c, bias=False)
+ self.fc2 = nn.Linear(c, c, bias=False)
+
+ def forward(self, x):
+ x = self.ma(self.q(x), self.k(x), self.v(x))[0] + x
+ x = self.fc2(self.fc1(x)) + x
+ return x
+
+
+class TransformerBlock(nn.Module):
+ # Vision Transformer https://arxiv.org/abs/2010.11929
+ def __init__(self, c1, c2, num_heads, num_layers):
+ super().__init__()
+ self.conv = None
+ if c1 != c2:
+ self.conv = Conv(c1, c2)
+ self.linear = nn.Linear(c2, c2) # learnable position embedding
+ self.tr = nn.Sequential(*(TransformerLayer(c2, num_heads) for _ in range(num_layers)))
+ self.c2 = c2
+
+ def forward(self, x):
+ if self.conv is not None:
+ x = self.conv(x)
+ b, _, w, h = x.shape
+ p = x.flatten(2).permute(2, 0, 1)
+ return self.tr(p + self.linear(p)).permute(1, 2, 0).reshape(b, self.c2, w, h)
+
+
+class Bottleneck(nn.Module):
+ # Standard bottleneck
+ def __init__(self, c1, c2, shortcut=True, g=1, e=0.5): # ch_in, ch_out, shortcut, groups, expansion
+ super().__init__()
+ c_ = int(c2 * e) # hidden channels
+ self.cv1 = Conv(c1, c_, 1, 1)
+ self.cv2 = Conv(c_, c2, 3, 1, g=g)
+ self.add = shortcut and c1 == c2
+
+ def forward(self, x):
+ return x + self.cv2(self.cv1(x)) if self.add else self.cv2(self.cv1(x))
+
+
+class BottleneckCSP(nn.Module):
+ # CSP Bottleneck https://github.com/WongKinYiu/CrossStagePartialNetworks
+ def __init__(self, c1, c2, n=1, shortcut=True, g=1, e=0.5): # ch_in, ch_out, number, shortcut, groups, expansion
+ super().__init__()
+ c_ = int(c2 * e) # hidden channels
+ self.cv1 = Conv(c1, c_, 1, 1)
+ self.cv2 = nn.Conv2d(c1, c_, 1, 1, bias=False)
+ self.cv3 = nn.Conv2d(c_, c_, 1, 1, bias=False)
+ self.cv4 = Conv(2 * c_, c2, 1, 1)
+ self.bn = nn.BatchNorm2d(2 * c_) # applied to cat(cv2, cv3)
+ self.act = nn.SiLU()
+ self.m = nn.Sequential(*(Bottleneck(c_, c_, shortcut, g, e=1.0) for _ in range(n)))
+
+ def forward(self, x):
+ y1 = self.cv3(self.m(self.cv1(x)))
+ y2 = self.cv2(x)
+ return self.cv4(self.act(self.bn(torch.cat((y1, y2), 1))))
+
+
+class CrossConv(nn.Module):
+ # Cross Convolution Downsample
+ def __init__(self, c1, c2, k=3, s=1, g=1, e=1.0, shortcut=False):
+ # ch_in, ch_out, kernel, stride, groups, expansion, shortcut
+ super().__init__()
+ c_ = int(c2 * e) # hidden channels
+ self.cv1 = Conv(c1, c_, (1, k), (1, s))
+ self.cv2 = Conv(c_, c2, (k, 1), (s, 1), g=g)
+ self.add = shortcut and c1 == c2
+
+ def forward(self, x):
+ return x + self.cv2(self.cv1(x)) if self.add else self.cv2(self.cv1(x))
+
+
+class C3(nn.Module):
+ # CSP Bottleneck with 3 convolutions
+ def __init__(self, c1, c2, n=1, shortcut=True, g=1, e=0.5): # ch_in, ch_out, number, shortcut, groups, expansion
+ super().__init__()
+ c_ = int(c2 * e) # hidden channels
+ self.cv1 = Conv(c1, c_, 1, 1)
+ self.cv2 = Conv(c1, c_, 1, 1)
+ self.cv3 = Conv(2 * c_, c2, 1) # optional act=FReLU(c2)
+ self.m = nn.Sequential(*(Bottleneck(c_, c_, shortcut, g, e=1.0) for _ in range(n)))
+
+ def forward(self, x):
+ return self.cv3(torch.cat((self.m(self.cv1(x)), self.cv2(x)), 1))
+
+
+class C3x(C3):
+ # C3 module with cross-convolutions
+ def __init__(self, c1, c2, n=1, shortcut=True, g=1, e=0.5):
+ super().__init__(c1, c2, n, shortcut, g, e)
+ c_ = int(c2 * e)
+ self.m = nn.Sequential(*(CrossConv(c_, c_, 3, 1, g, 1.0, shortcut) for _ in range(n)))
+
+
+class C3TR(C3):
+ # C3 module with TransformerBlock()
+ def __init__(self, c1, c2, n=1, shortcut=True, g=1, e=0.5):
+ super().__init__(c1, c2, n, shortcut, g, e)
+ c_ = int(c2 * e)
+ self.m = TransformerBlock(c_, c_, 4, n)
+
+
+class C3SPP(C3):
+ # C3 module with SPP()
+ def __init__(self, c1, c2, k=(5, 9, 13), n=1, shortcut=True, g=1, e=0.5):
+ super().__init__(c1, c2, n, shortcut, g, e)
+ c_ = int(c2 * e)
+ self.m = SPP(c_, c_, k)
+
+
+class C3Ghost(C3):
+ # C3 module with GhostBottleneck()
+ def __init__(self, c1, c2, n=1, shortcut=True, g=1, e=0.5):
+ super().__init__(c1, c2, n, shortcut, g, e)
+ c_ = int(c2 * e) # hidden channels
+ self.m = nn.Sequential(*(GhostBottleneck(c_, c_) for _ in range(n)))
+
+
+class SPP(nn.Module):
+ # Spatial Pyramid Pooling (SPP) layer https://arxiv.org/abs/1406.4729
+ def __init__(self, c1, c2, k=(5, 9, 13)):
+ super().__init__()
+ c_ = c1 // 2 # hidden channels
+ self.cv1 = Conv(c1, c_, 1, 1)
+ self.cv2 = Conv(c_ * (len(k) + 1), c2, 1, 1)
+ self.m = nn.ModuleList([nn.MaxPool2d(kernel_size=x, stride=1, padding=x // 2) for x in k])
+
+ def forward(self, x):
+ x = self.cv1(x)
+ with warnings.catch_warnings():
+ warnings.simplefilter('ignore') # suppress torch 1.9.0 max_pool2d() warning
+ return self.cv2(torch.cat([x] + [m(x) for m in self.m], 1))
+
+
+class SPPF(nn.Module):
+ # Spatial Pyramid Pooling - Fast (SPPF) layer for YOLOv5 by Glenn Jocher
+ def __init__(self, c1, c2, k=5): # equivalent to SPP(k=(5, 9, 13))
+ super().__init__()
+ c_ = c1 // 2 # hidden channels
+ self.cv1 = Conv(c1, c_, 1, 1)
+ self.cv2 = Conv(c_ * 4, c2, 1, 1)
+ self.m = nn.MaxPool2d(kernel_size=k, stride=1, padding=k // 2)
+
+ def forward(self, x):
+ x = self.cv1(x)
+ with warnings.catch_warnings():
+ warnings.simplefilter('ignore') # suppress torch 1.9.0 max_pool2d() warning
+ y1 = self.m(x)
+ y2 = self.m(y1)
+ return self.cv2(torch.cat((x, y1, y2, self.m(y2)), 1))
+
+
+class Focus(nn.Module):
+ # Focus wh information into c-space
+ def __init__(self, c1, c2, k=1, s=1, p=None, g=1, act=True): # ch_in, ch_out, kernel, stride, padding, groups
+ super().__init__()
+ self.conv = Conv(c1 * 4, c2, k, s, p, g, act=act)
+ # self.contract = Contract(gain=2)
+
+ def forward(self, x): # x(b,c,w,h) -> y(b,4c,w/2,h/2)
+ return self.conv(torch.cat((x[..., ::2, ::2], x[..., 1::2, ::2], x[..., ::2, 1::2], x[..., 1::2, 1::2]), 1))
+ # return self.conv(self.contract(x))
+
+
+class GhostConv(nn.Module):
+ # Ghost Convolution https://github.com/huawei-noah/ghostnet
+ def __init__(self, c1, c2, k=1, s=1, g=1, act=True): # ch_in, ch_out, kernel, stride, groups
+ super().__init__()
+ c_ = c2 // 2 # hidden channels
+ self.cv1 = Conv(c1, c_, k, s, None, g, act=act)
+ self.cv2 = Conv(c_, c_, 5, 1, None, c_, act=act)
+
+ def forward(self, x):
+ y = self.cv1(x)
+ return torch.cat((y, self.cv2(y)), 1)
+
+
+class GhostBottleneck(nn.Module):
+ # Ghost Bottleneck https://github.com/huawei-noah/ghostnet
+ def __init__(self, c1, c2, k=3, s=1): # ch_in, ch_out, kernel, stride
+ super().__init__()
+ c_ = c2 // 2
+ self.conv = nn.Sequential(
+ GhostConv(c1, c_, 1, 1), # pw
+ DWConv(c_, c_, k, s, act=False) if s == 2 else nn.Identity(), # dw
+ GhostConv(c_, c2, 1, 1, act=False)) # pw-linear
+ self.shortcut = nn.Sequential(DWConv(c1, c1, k, s, act=False), Conv(c1, c2, 1, 1,
+ act=False)) if s == 2 else nn.Identity()
+
+ def forward(self, x):
+ return self.conv(x) + self.shortcut(x)
+
+
+class Contract(nn.Module):
+ # Contract width-height into channels, i.e. x(1,64,80,80) to x(1,256,40,40)
+ def __init__(self, gain=2):
+ super().__init__()
+ self.gain = gain
+
+ def forward(self, x):
+ b, c, h, w = x.size() # assert (h / s == 0) and (W / s == 0), 'Indivisible gain'
+ s = self.gain
+ x = x.view(b, c, h // s, s, w // s, s) # x(1,64,40,2,40,2)
+ x = x.permute(0, 3, 5, 1, 2, 4).contiguous() # x(1,2,2,64,40,40)
+ return x.view(b, c * s * s, h // s, w // s) # x(1,256,40,40)
+
+
+class Expand(nn.Module):
+ # Expand channels into width-height, i.e. x(1,64,80,80) to x(1,16,160,160)
+ def __init__(self, gain=2):
+ super().__init__()
+ self.gain = gain
+
+ def forward(self, x):
+ b, c, h, w = x.size() # assert C / s ** 2 == 0, 'Indivisible gain'
+ s = self.gain
+ x = x.view(b, s, s, c // s ** 2, h, w) # x(1,2,2,16,80,80)
+ x = x.permute(0, 3, 4, 1, 5, 2).contiguous() # x(1,16,80,2,80,2)
+ return x.view(b, c // s ** 2, h * s, w * s) # x(1,16,160,160)
+
+
+class Concat(nn.Module):
+ # Concatenate a list of tensors along dimension
+ def __init__(self, dimension=1):
+ super().__init__()
+ self.d = dimension
+
+ def forward(self, x):
+ return torch.cat(x, self.d)
+
+
+class DetectMultiBackend(nn.Module):
+ # YOLOv5 MultiBackend class for python inference on various backends
+ def __init__(self, weights='yolov5s.pt', device=torch.device('cpu'), dnn=False, data=None, fp16=False, fuse=True):
+ # Usage:
+ # PyTorch: weights = *.pt
+ # TorchScript: *.torchscript
+ # ONNX Runtime: *.onnx
+ # ONNX OpenCV DNN: *.onnx --dnn
+ # OpenVINO: *_openvino_model
+ # CoreML: *.mlmodel
+ # TensorRT: *.engine
+ # TensorFlow SavedModel: *_saved_model
+ # TensorFlow GraphDef: *.pb
+ # TensorFlow Lite: *.tflite
+ # TensorFlow Edge TPU: *_edgetpu.tflite
+ # PaddlePaddle: *_paddle_model
+ from models.experimental import attempt_download, attempt_load # scoped to avoid circular import
+
+ super().__init__()
+ w = str(weights[0] if isinstance(weights, list) else weights)
+ pt, jit, onnx, xml, engine, coreml, saved_model, pb, tflite, edgetpu, tfjs, paddle, triton = self._model_type(w)
+ fp16 &= pt or jit or onnx or engine # FP16
+ nhwc = coreml or saved_model or pb or tflite or edgetpu # BHWC formats (vs torch BCWH)
+ stride = 32 # default stride
+ cuda = torch.cuda.is_available() and device.type != 'cpu' # use CUDA
+ if not (pt or triton):
+ w = attempt_download(w) # download if not local
+
+ if pt: # PyTorch
+ model = attempt_load(weights if isinstance(weights, list) else w, device=device, inplace=True, fuse=fuse)
+ stride = max(int(model.stride.max()), 32) # model stride
+ names = model.module.names if hasattr(model, 'module') else model.names # get class names
+ model.half() if fp16 else model.float()
+ self.model = model # explicitly assign for to(), cpu(), cuda(), half()
+ elif jit: # TorchScript
+ LOGGER.info(f'Loading {w} for TorchScript inference...')
+ extra_files = {'config.txt': ''} # model metadata
+ model = torch.jit.load(w, _extra_files=extra_files, map_location=device)
+ model.half() if fp16 else model.float()
+ if extra_files['config.txt']: # load metadata dict
+ d = json.loads(extra_files['config.txt'],
+ object_hook=lambda d: {
+ int(k) if k.isdigit() else k: v
+ for k, v in d.items()})
+ stride, names = int(d['stride']), d['names']
+ elif dnn: # ONNX OpenCV DNN
+ LOGGER.info(f'Loading {w} for ONNX OpenCV DNN inference...')
+ check_requirements('opencv-python>=4.5.4')
+ net = cv2.dnn.readNetFromONNX(w)
+ elif onnx: # ONNX Runtime
+ LOGGER.info(f'Loading {w} for ONNX Runtime inference...')
+ check_requirements(('onnx', 'onnxruntime-gpu' if cuda else 'onnxruntime'))
+ import onnxruntime
+ providers = ['CUDAExecutionProvider', 'CPUExecutionProvider'] if cuda else ['CPUExecutionProvider']
+ session = onnxruntime.InferenceSession(w, providers=providers)
+ output_names = [x.name for x in session.get_outputs()]
+ meta = session.get_modelmeta().custom_metadata_map # metadata
+ if 'stride' in meta:
+ stride, names = int(meta['stride']), eval(meta['names'])
+ elif xml: # OpenVINO
+ LOGGER.info(f'Loading {w} for OpenVINO inference...')
+ check_requirements('openvino') # requires openvino-dev: https://pypi.org/project/openvino-dev/
+ from openvino.runtime import Core, Layout, get_batch
+ ie = Core()
+ if not Path(w).is_file(): # if not *.xml
+ w = next(Path(w).glob('*.xml')) # get *.xml file from *_openvino_model dir
+ network = ie.read_model(model=w, weights=Path(w).with_suffix('.bin'))
+ if network.get_parameters()[0].get_layout().empty:
+ network.get_parameters()[0].set_layout(Layout('NCHW'))
+ batch_dim = get_batch(network)
+ if batch_dim.is_static:
+ batch_size = batch_dim.get_length()
+ executable_network = ie.compile_model(network, device_name='CPU') # device_name="MYRIAD" for Intel NCS2
+ stride, names = self._load_metadata(Path(w).with_suffix('.yaml')) # load metadata
+ elif engine: # TensorRT
+ LOGGER.info(f'Loading {w} for TensorRT inference...')
+ import tensorrt as trt # https://developer.nvidia.com/nvidia-tensorrt-download
+ check_version(trt.__version__, '7.0.0', hard=True) # require tensorrt>=7.0.0
+ if device.type == 'cpu':
+ device = torch.device('cuda:0')
+ Binding = namedtuple('Binding', ('name', 'dtype', 'shape', 'data', 'ptr'))
+ logger = trt.Logger(trt.Logger.INFO)
+ with open(w, 'rb') as f, trt.Runtime(logger) as runtime:
+ model = runtime.deserialize_cuda_engine(f.read())
+ context = model.create_execution_context()
+ bindings = OrderedDict()
+ output_names = []
+ fp16 = False # default updated below
+ dynamic = False
+ for i in range(model.num_bindings):
+ name = model.get_binding_name(i)
+ dtype = trt.nptype(model.get_binding_dtype(i))
+ if model.binding_is_input(i):
+ if -1 in tuple(model.get_binding_shape(i)): # dynamic
+ dynamic = True
+ context.set_binding_shape(i, tuple(model.get_profile_shape(0, i)[2]))
+ if dtype == np.float16:
+ fp16 = True
+ else: # output
+ output_names.append(name)
+ shape = tuple(context.get_binding_shape(i))
+ im = torch.from_numpy(np.empty(shape, dtype=dtype)).to(device)
+ bindings[name] = Binding(name, dtype, shape, im, int(im.data_ptr()))
+ binding_addrs = OrderedDict((n, d.ptr) for n, d in bindings.items())
+ batch_size = bindings['images'].shape[0] # if dynamic, this is instead max batch size
+ elif coreml: # CoreML
+ LOGGER.info(f'Loading {w} for CoreML inference...')
+ import coremltools as ct
+ model = ct.models.MLModel(w)
+ elif saved_model: # TF SavedModel
+ LOGGER.info(f'Loading {w} for TensorFlow SavedModel inference...')
+ import tensorflow as tf
+ keras = False # assume TF1 saved_model
+ model = tf.keras.models.load_model(w) if keras else tf.saved_model.load(w)
+ elif pb: # GraphDef https://www.tensorflow.org/guide/migrate#a_graphpb_or_graphpbtxt
+ LOGGER.info(f'Loading {w} for TensorFlow GraphDef inference...')
+ import tensorflow as tf
+
+ def wrap_frozen_graph(gd, inputs, outputs):
+ x = tf.compat.v1.wrap_function(lambda: tf.compat.v1.import_graph_def(gd, name=''), []) # wrapped
+ ge = x.graph.as_graph_element
+ return x.prune(tf.nest.map_structure(ge, inputs), tf.nest.map_structure(ge, outputs))
+
+ def gd_outputs(gd):
+ name_list, input_list = [], []
+ for node in gd.node: # tensorflow.core.framework.node_def_pb2.NodeDef
+ name_list.append(node.name)
+ input_list.extend(node.input)
+ return sorted(f'{x}:0' for x in list(set(name_list) - set(input_list)) if not x.startswith('NoOp'))
+
+ gd = tf.Graph().as_graph_def() # TF GraphDef
+ with open(w, 'rb') as f:
+ gd.ParseFromString(f.read())
+ frozen_func = wrap_frozen_graph(gd, inputs='x:0', outputs=gd_outputs(gd))
+ elif tflite or edgetpu: # https://www.tensorflow.org/lite/guide/python#install_tensorflow_lite_for_python
+ try: # https://coral.ai/docs/edgetpu/tflite-python/#update-existing-tf-lite-code-for-the-edge-tpu
+ from tflite_runtime.interpreter import Interpreter, load_delegate
+ except ImportError:
+ import tensorflow as tf
+ Interpreter, load_delegate = tf.lite.Interpreter, tf.lite.experimental.load_delegate,
+ if edgetpu: # TF Edge TPU https://coral.ai/software/#edgetpu-runtime
+ LOGGER.info(f'Loading {w} for TensorFlow Lite Edge TPU inference...')
+ delegate = {
+ 'Linux': 'libedgetpu.so.1',
+ 'Darwin': 'libedgetpu.1.dylib',
+ 'Windows': 'edgetpu.dll'}[platform.system()]
+ interpreter = Interpreter(model_path=w, experimental_delegates=[load_delegate(delegate)])
+ else: # TFLite
+ LOGGER.info(f'Loading {w} for TensorFlow Lite inference...')
+ interpreter = Interpreter(model_path=w) # load TFLite model
+ interpreter.allocate_tensors() # allocate
+ input_details = interpreter.get_input_details() # inputs
+ output_details = interpreter.get_output_details() # outputs
+ # load metadata
+ with contextlib.suppress(zipfile.BadZipFile):
+ with zipfile.ZipFile(w, 'r') as model:
+ meta_file = model.namelist()[0]
+ meta = ast.literal_eval(model.read(meta_file).decode('utf-8'))
+ stride, names = int(meta['stride']), meta['names']
+ elif tfjs: # TF.js
+ raise NotImplementedError('ERROR: YOLOv5 TF.js inference is not supported')
+ elif paddle: # PaddlePaddle
+ LOGGER.info(f'Loading {w} for PaddlePaddle inference...')
+ check_requirements('paddlepaddle-gpu' if cuda else 'paddlepaddle')
+ import paddle.inference as pdi
+ if not Path(w).is_file(): # if not *.pdmodel
+ w = next(Path(w).rglob('*.pdmodel')) # get *.pdmodel file from *_paddle_model dir
+ weights = Path(w).with_suffix('.pdiparams')
+ config = pdi.Config(str(w), str(weights))
+ if cuda:
+ config.enable_use_gpu(memory_pool_init_size_mb=2048, device_id=0)
+ predictor = pdi.create_predictor(config)
+ input_handle = predictor.get_input_handle(predictor.get_input_names()[0])
+ output_names = predictor.get_output_names()
+ elif triton: # NVIDIA Triton Inference Server
+ LOGGER.info(f'Using {w} as Triton Inference Server...')
+ check_requirements('tritonclient[all]')
+ from utils.triton import TritonRemoteModel
+ model = TritonRemoteModel(url=w)
+ nhwc = model.runtime.startswith('tensorflow')
+ else:
+ raise NotImplementedError(f'ERROR: {w} is not a supported format')
+
+ # class names
+ if 'names' not in locals():
+ names = yaml_load(data)['names'] if data else {i: f'class{i}' for i in range(999)}
+ if names[0] == 'n01440764' and len(names) == 1000: # ImageNet
+ names = yaml_load(ROOT / 'data/ImageNet.yaml')['names'] # human-readable names
+
+ self.__dict__.update(locals()) # assign all variables to self
+
+ def forward(self, im, augment=False, visualize=False):
+ # YOLOv5 MultiBackend inference
+ b, ch, h, w = im.shape # batch, channel, height, width
+ if self.fp16 and im.dtype != torch.float16:
+ im = im.half() # to FP16
+ if self.nhwc:
+ im = im.permute(0, 2, 3, 1) # torch BCHW to numpy BHWC shape(1,320,192,3)
+
+ if self.pt: # PyTorch
+ y = self.model(im, augment=augment, visualize=visualize) if augment or visualize else self.model(im)
+ elif self.jit: # TorchScript
+ y = self.model(im)
+ elif self.dnn: # ONNX OpenCV DNN
+ im = im.cpu().numpy() # torch to numpy
+ self.net.setInput(im)
+ y = self.net.forward()
+ elif self.onnx: # ONNX Runtime
+ im = im.cpu().numpy() # torch to numpy
+ y = self.session.run(self.output_names, {self.session.get_inputs()[0].name: im})
+ elif self.xml: # OpenVINO
+ im = im.cpu().numpy() # FP32
+ y = list(self.executable_network([im]).values())
+ elif self.engine: # TensorRT
+ if self.dynamic and im.shape != self.bindings['images'].shape:
+ i = self.model.get_binding_index('images')
+ self.context.set_binding_shape(i, im.shape) # reshape if dynamic
+ self.bindings['images'] = self.bindings['images']._replace(shape=im.shape)
+ for name in self.output_names:
+ i = self.model.get_binding_index(name)
+ self.bindings[name].data.resize_(tuple(self.context.get_binding_shape(i)))
+ s = self.bindings['images'].shape
+ assert im.shape == s, f"input size {im.shape} {'>' if self.dynamic else 'not equal to'} max model size {s}"
+ self.binding_addrs['images'] = int(im.data_ptr())
+ self.context.execute_v2(list(self.binding_addrs.values()))
+ y = [self.bindings[x].data for x in sorted(self.output_names)]
+ elif self.coreml: # CoreML
+ im = im.cpu().numpy()
+ im = Image.fromarray((im[0] * 255).astype('uint8'))
+ # im = im.resize((192, 320), Image.ANTIALIAS)
+ y = self.model.predict({'image': im}) # coordinates are xywh normalized
+ if 'confidence' in y:
+ box = xywh2xyxy(y['coordinates'] * [[w, h, w, h]]) # xyxy pixels
+ conf, cls = y['confidence'].max(1), y['confidence'].argmax(1).astype(np.float)
+ y = np.concatenate((box, conf.reshape(-1, 1), cls.reshape(-1, 1)), 1)
+ else:
+ y = list(reversed(y.values())) # reversed for segmentation models (pred, proto)
+ elif self.paddle: # PaddlePaddle
+ im = im.cpu().numpy().astype(np.float32)
+ self.input_handle.copy_from_cpu(im)
+ self.predictor.run()
+ y = [self.predictor.get_output_handle(x).copy_to_cpu() for x in self.output_names]
+ elif self.triton: # NVIDIA Triton Inference Server
+ y = self.model(im)
+ else: # TensorFlow (SavedModel, GraphDef, Lite, Edge TPU)
+ im = im.cpu().numpy()
+ if self.saved_model: # SavedModel
+ y = self.model(im, training=False) if self.keras else self.model(im)
+ elif self.pb: # GraphDef
+ y = self.frozen_func(x=self.tf.constant(im))
+ else: # Lite or Edge TPU
+ input = self.input_details[0]
+ int8 = input['dtype'] == np.uint8 # is TFLite quantized uint8 model
+ if int8:
+ scale, zero_point = input['quantization']
+ im = (im / scale + zero_point).astype(np.uint8) # de-scale
+ self.interpreter.set_tensor(input['index'], im)
+ self.interpreter.invoke()
+ y = []
+ for output in self.output_details:
+ x = self.interpreter.get_tensor(output['index'])
+ if int8:
+ scale, zero_point = output['quantization']
+ x = (x.astype(np.float32) - zero_point) * scale # re-scale
+ y.append(x)
+ y = [x if isinstance(x, np.ndarray) else x.numpy() for x in y]
+ y[0][..., :4] *= [w, h, w, h] # xywh normalized to pixels
+
+ if isinstance(y, (list, tuple)):
+ return self.from_numpy(y[0]) if len(y) == 1 else [self.from_numpy(x) for x in y]
+ else:
+ return self.from_numpy(y)
+
+ def from_numpy(self, x):
+ return torch.from_numpy(x).to(self.device) if isinstance(x, np.ndarray) else x
+
+ def warmup(self, imgsz=(1, 3, 640, 640)):
+ # Warmup model by running inference once
+ warmup_types = self.pt, self.jit, self.onnx, self.engine, self.saved_model, self.pb, self.triton
+ if any(warmup_types) and (self.device.type != 'cpu' or self.triton):
+ im = torch.empty(*imgsz, dtype=torch.half if self.fp16 else torch.float, device=self.device) # input
+ for _ in range(2 if self.jit else 1): #
+ self.forward(im) # warmup
+
+ @staticmethod
+ def _model_type(p='path/to/model.pt'):
+ # Return model type from model path, i.e. path='path/to/model.onnx' -> type=onnx
+ # types = [pt, jit, onnx, xml, engine, coreml, saved_model, pb, tflite, edgetpu, tfjs, paddle]
+ from yolov5.export import export_formats
+ from yolov5.utils.downloads import is_url
+ sf = list(export_formats().Suffix) # export suffixes
+ if not is_url(p, check=False):
+ check_suffix(p, sf) # checks
+ url = urlparse(p) # if url may be Triton inference server
+ types = [s in Path(p).name for s in sf]
+ types[8] &= not types[9] # tflite &= not edgetpu
+ triton = not any(types) and all([any(s in url.scheme for s in ['http', 'grpc']), url.netloc])
+ return types + [triton]
+
+ @staticmethod
+ def _load_metadata(f=Path('path/to/meta.yaml')):
+ # Load metadata from meta.yaml if it exists
+ if f.exists():
+ d = yaml_load(f)
+ return d['stride'], d['names'] # assign stride, names
+ return None, None
+
+
+class AutoShape(nn.Module):
+ # YOLOv5 input-robust model wrapper for passing cv2/np/PIL/torch inputs. Includes preprocessing, inference and NMS
+ conf = 0.25 # NMS confidence threshold
+ iou = 0.45 # NMS IoU threshold
+ agnostic = False # NMS class-agnostic
+ multi_label = False # NMS multiple labels per box
+ classes = None # (optional list) filter by class, i.e. = [0, 15, 16] for COCO persons, cats and dogs
+ max_det = 1000 # maximum number of detections per image
+ amp = False # Automatic Mixed Precision (AMP) inference
+
+ def __init__(self, model, verbose=True):
+ super().__init__()
+ if verbose:
+ LOGGER.info('Adding AutoShape... ')
+ copy_attr(self, model, include=('yaml', 'nc', 'hyp', 'names', 'stride', 'abc'), exclude=()) # copy attributes
+ self.dmb = isinstance(model, DetectMultiBackend) # DetectMultiBackend() instance
+ self.pt = not self.dmb or model.pt # PyTorch model
+ self.model = model.eval()
+ if self.pt:
+ m = self.model.model.model[-1] if self.dmb else self.model.model[-1] # Detect()
+ m.inplace = False # Detect.inplace=False for safe multithread inference
+ m.export = True # do not output loss values
+
+ def _apply(self, fn):
+ # Apply to(), cpu(), cuda(), half() to model tensors that are not parameters or registered buffers
+ self = super()._apply(fn)
+ if self.pt:
+ m = self.model.model.model[-1] if self.dmb else self.model.model[-1] # Detect()
+ m.stride = fn(m.stride)
+ m.grid = list(map(fn, m.grid))
+ if isinstance(m.anchor_grid, list):
+ m.anchor_grid = list(map(fn, m.anchor_grid))
+ return self
+
+ @smart_inference_mode()
+ def forward(self, ims, size=640, augment=False, profile=False):
+ # Inference from various sources. For size(height=640, width=1280), RGB images example inputs are:
+ # file: ims = 'data/images/zidane.jpg' # str or PosixPath
+ # URI: = 'https://ultralytics.com/images/zidane.jpg'
+ # OpenCV: = cv2.imread('image.jpg')[:,:,::-1] # HWC BGR to RGB x(640,1280,3)
+ # PIL: = Image.open('image.jpg') or ImageGrab.grab() # HWC x(640,1280,3)
+ # numpy: = np.zeros((640,1280,3)) # HWC
+ # torch: = torch.zeros(16,3,320,640) # BCHW (scaled to size=640, 0-1 values)
+ # multiple: = [Image.open('image1.jpg'), Image.open('image2.jpg'), ...] # list of images
+
+ dt = (Profile(), Profile(), Profile())
+ with dt[0]:
+ if isinstance(size, int): # expand
+ size = (size, size)
+ p = next(self.model.parameters()) if self.pt else torch.empty(1, device=self.model.device) # param
+ autocast = self.amp and (p.device.type != 'cpu') # Automatic Mixed Precision (AMP) inference
+ if isinstance(ims, torch.Tensor): # torch
+ with amp.autocast(autocast):
+ return self.model(ims.to(p.device).type_as(p), augment=augment) # inference
+
+ # Pre-process
+ n, ims = (len(ims), list(ims)) if isinstance(ims, (list, tuple)) else (1, [ims]) # number, list of images
+ shape0, shape1, files = [], [], [] # image and inference shapes, filenames
+ for i, im in enumerate(ims):
+ f = f'image{i}' # filename
+ if isinstance(im, (str, Path)): # filename or uri
+ im, f = Image.open(requests.get(im, stream=True).raw if str(im).startswith('http') else im), im
+ im = np.asarray(exif_transpose(im))
+ elif isinstance(im, Image.Image): # PIL Image
+ im, f = np.asarray(exif_transpose(im)), getattr(im, 'filename', f) or f
+ files.append(Path(f).with_suffix('.jpg').name)
+ if im.shape[0] < 5: # image in CHW
+ im = im.transpose((1, 2, 0)) # reverse dataloader .transpose(2, 0, 1)
+ im = im[..., :3] if im.ndim == 3 else cv2.cvtColor(im, cv2.COLOR_GRAY2BGR) # enforce 3ch input
+ s = im.shape[:2] # HWC
+ shape0.append(s) # image shape
+ g = max(size) / max(s) # gain
+ shape1.append([int(y * g) for y in s])
+ ims[i] = im if im.data.contiguous else np.ascontiguousarray(im) # update
+ shape1 = [make_divisible(x, self.stride) for x in np.array(shape1).max(0)] # inf shape
+ x = [letterbox(im, shape1, auto=False)[0] for im in ims] # pad
+ x = np.ascontiguousarray(np.array(x).transpose((0, 3, 1, 2))) # stack and BHWC to BCHW
+ x = torch.from_numpy(x).to(p.device).type_as(p) / 255 # uint8 to fp16/32
+
+ with amp.autocast(autocast):
+ # Inference
+ with dt[1]:
+ y = self.model(x, augment=augment) # forward
+
+ # Post-process
+ with dt[2]:
+ y = non_max_suppression(y if self.dmb else y[0],
+ self.conf,
+ self.iou,
+ self.classes,
+ self.agnostic,
+ self.multi_label,
+ max_det=self.max_det) # NMS
+ for i in range(n):
+ scale_boxes(shape1, y[i][:, :4], shape0[i])
+
+ return Detections(ims, y, files, dt, self.names, x.shape)
+
+
+class Detections:
+ # YOLOv5 detections class for inference results
+ def __init__(self, ims, pred, files, times=(0, 0, 0), names=None, shape=None):
+ super().__init__()
+ d = pred[0].device # device
+ gn = [torch.tensor([*(im.shape[i] for i in [1, 0, 1, 0]), 1, 1], device=d) for im in ims] # normalizations
+ self.ims = ims # list of images as numpy arrays
+ self.pred = pred # list of tensors pred[0] = (xyxy, conf, cls)
+ self.names = names # class names
+ self.files = files # image filenames
+ self.times = times # profiling times
+ self.xyxy = pred # xyxy pixels
+ self.xywh = [xyxy2xywh(x) for x in pred] # xywh pixels
+ self.xyxyn = [x / g for x, g in zip(self.xyxy, gn)] # xyxy normalized
+ self.xywhn = [x / g for x, g in zip(self.xywh, gn)] # xywh normalized
+ self.n = len(self.pred) # number of images (batch size)
+ self.t = tuple(x.t / self.n * 1E3 for x in times) # timestamps (ms)
+ self.s = tuple(shape) # inference BCHW shape
+
+ def _run(self, pprint=False, show=False, save=False, crop=False, render=False, labels=True, save_dir=Path('')):
+ s, crops = '', []
+ for i, (im, pred) in enumerate(zip(self.ims, self.pred)):
+ s += f'\nimage {i + 1}/{len(self.pred)}: {im.shape[0]}x{im.shape[1]} ' # string
+ if pred.shape[0]:
+ for c in pred[:, -1].unique():
+ n = (pred[:, -1] == c).sum() # detections per class
+ s += f"{n} {self.names[int(c)]}{'s' * (n > 1)}, " # add to string
+ s = s.rstrip(', ')
+ if show or save or render or crop:
+ annotator = Annotator(im, example=str(self.names))
+ for *box, conf, cls in reversed(pred): # xyxy, confidence, class
+ label = f'{self.names[int(cls)]} {conf:.2f}'
+ if crop:
+ file = save_dir / 'crops' / self.names[int(cls)] / self.files[i] if save else None
+ crops.append({
+ 'box': box,
+ 'conf': conf,
+ 'cls': cls,
+ 'label': label,
+ 'im': save_one_box(box, im, file=file, save=save)})
+ else: # all others
+ annotator.box_label(box, label if labels else '', color=colors(cls))
+ im = annotator.im
+ else:
+ s += '(no detections)'
+
+ im = Image.fromarray(im.astype(np.uint8)) if isinstance(im, np.ndarray) else im # from np
+ if show:
+ if is_jupyter():
+ from IPython.display import display
+ display(im)
+ else:
+ im.show(self.files[i])
+ if save:
+ f = self.files[i]
+ im.save(save_dir / f) # save
+ if i == self.n - 1:
+ LOGGER.info(f"Saved {self.n} image{'s' * (self.n > 1)} to {colorstr('bold', save_dir)}")
+ if render:
+ self.ims[i] = np.asarray(im)
+ if pprint:
+ s = s.lstrip('\n')
+ return f'{s}\nSpeed: %.1fms pre-process, %.1fms inference, %.1fms NMS per image at shape {self.s}' % self.t
+ if crop:
+ if save:
+ LOGGER.info(f'Saved results to {save_dir}\n')
+ return crops
+
+ @TryExcept('Showing images is not supported in this environment')
+ def show(self, labels=True):
+ self._run(show=True, labels=labels) # show results
+
+ def save(self, labels=True, save_dir='runs/detect/exp', exist_ok=False):
+ save_dir = increment_path(save_dir, exist_ok, mkdir=True) # increment save_dir
+ self._run(save=True, labels=labels, save_dir=save_dir) # save results
+
+ def crop(self, save=True, save_dir='runs/detect/exp', exist_ok=False):
+ save_dir = increment_path(save_dir, exist_ok, mkdir=True) if save else None
+ return self._run(crop=True, save=save, save_dir=save_dir) # crop results
+
+ def render(self, labels=True):
+ self._run(render=True, labels=labels) # render results
+ return self.ims
+
+ def pandas(self):
+ # return detections as pandas DataFrames, i.e. print(results.pandas().xyxy[0])
+ new = copy(self) # return copy
+ ca = 'xmin', 'ymin', 'xmax', 'ymax', 'confidence', 'class', 'name' # xyxy columns
+ cb = 'xcenter', 'ycenter', 'width', 'height', 'confidence', 'class', 'name' # xywh columns
+ for k, c in zip(['xyxy', 'xyxyn', 'xywh', 'xywhn'], [ca, ca, cb, cb]):
+ a = [[x[:5] + [int(x[5]), self.names[int(x[5])]] for x in x.tolist()] for x in getattr(self, k)] # update
+ setattr(new, k, [pd.DataFrame(x, columns=c) for x in a])
+ return new
+
+ def tolist(self):
+ # return a list of Detections objects, i.e. 'for result in results.tolist():'
+ r = range(self.n) # iterable
+ x = [Detections([self.ims[i]], [self.pred[i]], [self.files[i]], self.times, self.names, self.s) for i in r]
+ # for d in x:
+ # for k in ['ims', 'pred', 'xyxy', 'xyxyn', 'xywh', 'xywhn']:
+ # setattr(d, k, getattr(d, k)[0]) # pop out of list
+ return x
+
+ def print(self):
+ LOGGER.info(self.__str__())
+
+ def __len__(self): # override len(results)
+ return self.n
+
+ def __str__(self): # override print(results)
+ return self._run(pprint=True) # print results
+
+ def __repr__(self):
+ return f'YOLOv5 {self.__class__} instance\n' + self.__str__()
+
+
+class Proto(nn.Module):
+ # YOLOv5 mask Proto module for segmentation models
+ def __init__(self, c1, c_=256, c2=32): # ch_in, number of protos, number of masks
+ super().__init__()
+ self.cv1 = Conv(c1, c_, k=3)
+ self.upsample = nn.Upsample(scale_factor=2, mode='nearest')
+ self.cv2 = Conv(c_, c_, k=3)
+ self.cv3 = Conv(c_, c2)
+
+ def forward(self, x):
+ return self.cv3(self.cv2(self.upsample(self.cv1(x))))
+
+
+class Classify(nn.Module):
+ # YOLOv5 classification head, i.e. x(b,c1,20,20) to x(b,c2)
+ def __init__(self,
+ c1,
+ c2,
+ k=1,
+ s=1,
+ p=None,
+ g=1,
+ dropout_p=0.0): # ch_in, ch_out, kernel, stride, padding, groups, dropout probability
+ super().__init__()
+ c_ = 1280 # efficientnet_b0 size
+ self.conv = Conv(c1, c_, k, s, autopad(k, p), g)
+ self.pool = nn.AdaptiveAvgPool2d(1) # to x(b,c_,1,1)
+ self.drop = nn.Dropout(p=dropout_p, inplace=True)
+ self.linear = nn.Linear(c_, c2) # to x(b,c2)
+
+ def forward(self, x):
+ if isinstance(x, list):
+ x = torch.cat(x, 1)
+ return self.linear(self.drop(self.pool(self.conv(x)).flatten(1)))
diff --git a/yolov5/models/experimental.py b/yolov5/models/experimental.py
new file mode 100644
index 0000000000000000000000000000000000000000..b554401b133438a7d38a927c6fdd263503e78c35
--- /dev/null
+++ b/yolov5/models/experimental.py
@@ -0,0 +1,111 @@
+# YOLOv5 🚀 by Ultralytics, AGPL-3.0 license
+"""
+Experimental modules
+"""
+import math
+
+import numpy as np
+import torch
+import torch.nn as nn
+
+from yolov5.utils.downloads import attempt_download
+
+
+class Sum(nn.Module):
+ # Weighted sum of 2 or more layers https://arxiv.org/abs/1911.09070
+ def __init__(self, n, weight=False): # n: number of inputs
+ super().__init__()
+ self.weight = weight # apply weights boolean
+ self.iter = range(n - 1) # iter object
+ if weight:
+ self.w = nn.Parameter(-torch.arange(1.0, n) / 2, requires_grad=True) # layer weights
+
+ def forward(self, x):
+ y = x[0] # no weight
+ if self.weight:
+ w = torch.sigmoid(self.w) * 2
+ for i in self.iter:
+ y = y + x[i + 1] * w[i]
+ else:
+ for i in self.iter:
+ y = y + x[i + 1]
+ return y
+
+
+class MixConv2d(nn.Module):
+ # Mixed Depth-wise Conv https://arxiv.org/abs/1907.09595
+ def __init__(self, c1, c2, k=(1, 3), s=1, equal_ch=True): # ch_in, ch_out, kernel, stride, ch_strategy
+ super().__init__()
+ n = len(k) # number of convolutions
+ if equal_ch: # equal c_ per group
+ i = torch.linspace(0, n - 1E-6, c2).floor() # c2 indices
+ c_ = [(i == g).sum() for g in range(n)] # intermediate channels
+ else: # equal weight.numel() per group
+ b = [c2] + [0] * n
+ a = np.eye(n + 1, n, k=-1)
+ a -= np.roll(a, 1, axis=1)
+ a *= np.array(k) ** 2
+ a[0] = 1
+ c_ = np.linalg.lstsq(a, b, rcond=None)[0].round() # solve for equal weight indices, ax = b
+
+ self.m = nn.ModuleList([
+ nn.Conv2d(c1, int(c_), k, s, k // 2, groups=math.gcd(c1, int(c_)), bias=False) for k, c_ in zip(k, c_)])
+ self.bn = nn.BatchNorm2d(c2)
+ self.act = nn.SiLU()
+
+ def forward(self, x):
+ return self.act(self.bn(torch.cat([m(x) for m in self.m], 1)))
+
+
+class Ensemble(nn.ModuleList):
+ # Ensemble of models
+ def __init__(self):
+ super().__init__()
+
+ def forward(self, x, augment=False, profile=False, visualize=False):
+ y = [module(x, augment, profile, visualize)[0] for module in self]
+ # y = torch.stack(y).max(0)[0] # max ensemble
+ # y = torch.stack(y).mean(0) # mean ensemble
+ y = torch.cat(y, 1) # nms ensemble
+ return y, None # inference, train output
+
+
+def attempt_load(weights, device=None, inplace=True, fuse=True):
+ # Loads an ensemble of models weights=[a,b,c] or a single model weights=[a] or weights=a
+ from models.yolo import Detect, Model
+
+ model = Ensemble()
+ for w in weights if isinstance(weights, list) else [weights]:
+ ckpt = torch.load(attempt_download(w), map_location='cpu') # load
+ ckpt = (ckpt.get('ema') or ckpt['model']).to(device).float() # FP32 model
+
+ # Model compatibility updates
+ if not hasattr(ckpt, 'stride'):
+ ckpt.stride = torch.tensor([32.])
+ if hasattr(ckpt, 'names') and isinstance(ckpt.names, (list, tuple)):
+ ckpt.names = dict(enumerate(ckpt.names)) # convert to dict
+
+ model.append(ckpt.fuse().eval() if fuse and hasattr(ckpt, 'fuse') else ckpt.eval()) # model in eval mode
+
+ # Module compatibility updates
+ for m in model.modules():
+ t = type(m)
+ if t in (nn.Hardswish, nn.LeakyReLU, nn.ReLU, nn.ReLU6, nn.SiLU, Detect, Model):
+ m.inplace = inplace # torch 1.7.0 compatibility
+ if t is Detect and not isinstance(m.anchor_grid, list):
+ delattr(m, 'anchor_grid')
+ setattr(m, 'anchor_grid', [torch.zeros(1)] * m.nl)
+ elif t is nn.Upsample and not hasattr(m, 'recompute_scale_factor'):
+ m.recompute_scale_factor = None # torch 1.11.0 compatibility
+
+ # Return model
+ if len(model) == 1:
+ return model[-1]
+
+ # Return detection ensemble
+ print(f'Ensemble created with {weights}\n')
+ for k in 'names', 'nc', 'yaml':
+ setattr(model, k, getattr(model[0], k))
+ model.stride = model[torch.argmax(torch.tensor([m.stride.max() for m in model])).int()].stride # max stride
+ assert all(model[0].nc == m.nc for m in model), f'Models have different class counts: {[m.nc for m in model]}'
+ return model
diff --git a/yolov5/models/hub/anchors.yaml b/yolov5/models/hub/anchors.yaml
new file mode 100644
index 0000000000000000000000000000000000000000..df2f668b022ca363b47e62ef95bdf20d418fe0e3
--- /dev/null
+++ b/yolov5/models/hub/anchors.yaml
@@ -0,0 +1,59 @@
+# YOLOv5 🚀 by Ultralytics, AGPL-3.0 license
+# Default anchors for COCO data
+
+
+# P5 -------------------------------------------------------------------------------------------------------------------
+# P5-640:
+anchors_p5_640:
+ - [10,13, 16,30, 33,23] # P3/8
+ - [30,61, 62,45, 59,119] # P4/16
+ - [116,90, 156,198, 373,326] # P5/32
+
+
+# P6 -------------------------------------------------------------------------------------------------------------------
+# P6-640: thr=0.25: 0.9964 BPR, 5.54 anchors past thr, n=12, img_size=640, metric_all=0.281/0.716-mean/best, past_thr=0.469-mean: 9,11, 21,19, 17,41, 43,32, 39,70, 86,64, 65,131, 134,130, 120,265, 282,180, 247,354, 512,387
+anchors_p6_640:
+ - [9,11, 21,19, 17,41] # P3/8
+ - [43,32, 39,70, 86,64] # P4/16
+ - [65,131, 134,130, 120,265] # P5/32
+ - [282,180, 247,354, 512,387] # P6/64
+
+# P6-1280: thr=0.25: 0.9950 BPR, 5.55 anchors past thr, n=12, img_size=1280, metric_all=0.281/0.714-mean/best, past_thr=0.468-mean: 19,27, 44,40, 38,94, 96,68, 86,152, 180,137, 140,301, 303,264, 238,542, 436,615, 739,380, 925,792
+anchors_p6_1280:
+ - [19,27, 44,40, 38,94] # P3/8
+ - [96,68, 86,152, 180,137] # P4/16
+ - [140,301, 303,264, 238,542] # P5/32
+ - [436,615, 739,380, 925,792] # P6/64
+
+# P6-1920: thr=0.25: 0.9950 BPR, 5.55 anchors past thr, n=12, img_size=1920, metric_all=0.281/0.714-mean/best, past_thr=0.468-mean: 28,41, 67,59, 57,141, 144,103, 129,227, 270,205, 209,452, 455,396, 358,812, 653,922, 1109,570, 1387,1187
+anchors_p6_1920:
+ - [28,41, 67,59, 57,141] # P3/8
+ - [144,103, 129,227, 270,205] # P4/16
+ - [209,452, 455,396, 358,812] # P5/32
+ - [653,922, 1109,570, 1387,1187] # P6/64
+
+
+# P7 -------------------------------------------------------------------------------------------------------------------
+# P7-640: thr=0.25: 0.9962 BPR, 6.76 anchors past thr, n=15, img_size=640, metric_all=0.275/0.733-mean/best, past_thr=0.466-mean: 11,11, 13,30, 29,20, 30,46, 61,38, 39,92, 78,80, 146,66, 79,163, 149,150, 321,143, 157,303, 257,402, 359,290, 524,372
+anchors_p7_640:
+ - [11,11, 13,30, 29,20] # P3/8
+ - [30,46, 61,38, 39,92] # P4/16
+ - [78,80, 146,66, 79,163] # P5/32
+ - [149,150, 321,143, 157,303] # P6/64
+ - [257,402, 359,290, 524,372] # P7/128
+
+# P7-1280: thr=0.25: 0.9968 BPR, 6.71 anchors past thr, n=15, img_size=1280, metric_all=0.273/0.732-mean/best, past_thr=0.463-mean: 19,22, 54,36, 32,77, 70,83, 138,71, 75,173, 165,159, 148,334, 375,151, 334,317, 251,626, 499,474, 750,326, 534,814, 1079,818
+anchors_p7_1280:
+ - [19,22, 54,36, 32,77] # P3/8
+ - [70,83, 138,71, 75,173] # P4/16
+ - [165,159, 148,334, 375,151] # P5/32
+ - [334,317, 251,626, 499,474] # P6/64
+ - [750,326, 534,814, 1079,818] # P7/128
+
+# P7-1920: thr=0.25: 0.9968 BPR, 6.71 anchors past thr, n=15, img_size=1920, metric_all=0.273/0.732-mean/best, past_thr=0.463-mean: 29,34, 81,55, 47,115, 105,124, 207,107, 113,259, 247,238, 222,500, 563,227, 501,476, 376,939, 749,711, 1126,489, 801,1222, 1618,1227
+anchors_p7_1920:
+ - [29,34, 81,55, 47,115] # P3/8
+ - [105,124, 207,107, 113,259] # P4/16
+ - [247,238, 222,500, 563,227] # P5/32
+ - [501,476, 376,939, 749,711] # P6/64
+ - [1126,489, 801,1222, 1618,1227] # P7/128
diff --git a/yolov5/models/hub/yolov3-spp.yaml b/yolov5/models/hub/yolov3-spp.yaml
new file mode 100644
index 0000000000000000000000000000000000000000..4a71ed405277ce10a3c3f386834764ff0a82d53c
--- /dev/null
+++ b/yolov5/models/hub/yolov3-spp.yaml
@@ -0,0 +1,51 @@
+# YOLOv5 🚀 by Ultralytics, AGPL-3.0 license
+
+# Parameters
+nc: 80 # number of classes
+depth_multiple: 1.0 # model depth multiple
+width_multiple: 1.0 # layer channel multiple
+anchors:
+ - [10,13, 16,30, 33,23] # P3/8
+ - [30,61, 62,45, 59,119] # P4/16
+ - [116,90, 156,198, 373,326] # P5/32
+
+# darknet53 backbone
+backbone:
+ # [from, number, module, args]
+ [[-1, 1, Conv, [32, 3, 1]], # 0
+ [-1, 1, Conv, [64, 3, 2]], # 1-P1/2
+ [-1, 1, Bottleneck, [64]],
+ [-1, 1, Conv, [128, 3, 2]], # 3-P2/4
+ [-1, 2, Bottleneck, [128]],
+ [-1, 1, Conv, [256, 3, 2]], # 5-P3/8
+ [-1, 8, Bottleneck, [256]],
+ [-1, 1, Conv, [512, 3, 2]], # 7-P4/16
+ [-1, 8, Bottleneck, [512]],
+ [-1, 1, Conv, [1024, 3, 2]], # 9-P5/32
+ [-1, 4, Bottleneck, [1024]], # 10
+ ]
+
+# YOLOv3-SPP head
+head:
+ [[-1, 1, Bottleneck, [1024, False]],
+ [-1, 1, SPP, [512, [5, 9, 13]]],
+ [-1, 1, Conv, [1024, 3, 1]],
+ [-1, 1, Conv, [512, 1, 1]],
+ [-1, 1, Conv, [1024, 3, 1]], # 15 (P5/32-large)
+
+ [-2, 1, Conv, [256, 1, 1]],
+ [-1, 1, nn.Upsample, [None, 2, 'nearest']],
+ [[-1, 8], 1, Concat, [1]], # cat backbone P4
+ [-1, 1, Bottleneck, [512, False]],
+ [-1, 1, Bottleneck, [512, False]],
+ [-1, 1, Conv, [256, 1, 1]],
+ [-1, 1, Conv, [512, 3, 1]], # 22 (P4/16-medium)
+
+ [-2, 1, Conv, [128, 1, 1]],
+ [-1, 1, nn.Upsample, [None, 2, 'nearest']],
+ [[-1, 6], 1, Concat, [1]], # cat backbone P3
+ [-1, 1, Bottleneck, [256, False]],
+ [-1, 2, Bottleneck, [256, False]], # 27 (P3/8-small)
+
+ [[27, 22, 15], 1, Detect, [nc, anchors]], # Detect(P3, P4, P5)
+ ]
diff --git a/yolov5/models/hub/yolov3-tiny.yaml b/yolov5/models/hub/yolov3-tiny.yaml
new file mode 100644
index 0000000000000000000000000000000000000000..50b47e282df482b6f4f8dfb485c914ab1cbf6274
--- /dev/null
+++ b/yolov5/models/hub/yolov3-tiny.yaml
@@ -0,0 +1,41 @@
+# YOLOv5 🚀 by Ultralytics, AGPL-3.0 license
+
+# Parameters
+nc: 80 # number of classes
+depth_multiple: 1.0 # model depth multiple
+width_multiple: 1.0 # layer channel multiple
+anchors:
+ - [10,14, 23,27, 37,58] # P4/16
+ - [81,82, 135,169, 344,319] # P5/32
+
+# YOLOv3-tiny backbone
+backbone:
+ # [from, number, module, args]
+ [[-1, 1, Conv, [16, 3, 1]], # 0
+ [-1, 1, nn.MaxPool2d, [2, 2, 0]], # 1-P1/2
+ [-1, 1, Conv, [32, 3, 1]],
+ [-1, 1, nn.MaxPool2d, [2, 2, 0]], # 3-P2/4
+ [-1, 1, Conv, [64, 3, 1]],
+ [-1, 1, nn.MaxPool2d, [2, 2, 0]], # 5-P3/8
+ [-1, 1, Conv, [128, 3, 1]],
+ [-1, 1, nn.MaxPool2d, [2, 2, 0]], # 7-P4/16
+ [-1, 1, Conv, [256, 3, 1]],
+ [-1, 1, nn.MaxPool2d, [2, 2, 0]], # 9-P5/32
+ [-1, 1, Conv, [512, 3, 1]],
+ [-1, 1, nn.ZeroPad2d, [[0, 1, 0, 1]]], # 11
+ [-1, 1, nn.MaxPool2d, [2, 1, 0]], # 12
+ ]
+
+# YOLOv3-tiny head
+head:
+ [[-1, 1, Conv, [1024, 3, 1]],
+ [-1, 1, Conv, [256, 1, 1]],
+ [-1, 1, Conv, [512, 3, 1]], # 15 (P5/32-large)
+
+ [-2, 1, Conv, [128, 1, 1]],
+ [-1, 1, nn.Upsample, [None, 2, 'nearest']],
+ [[-1, 8], 1, Concat, [1]], # cat backbone P4
+ [-1, 1, Conv, [256, 3, 1]], # 19 (P4/16-medium)
+
+ [[19, 15], 1, Detect, [nc, anchors]], # Detect(P4, P5)
+ ]
diff --git a/yolov5/models/hub/yolov3.yaml b/yolov5/models/hub/yolov3.yaml
new file mode 100644
index 0000000000000000000000000000000000000000..c5e21098f89379487dde6f236b78667fad0ad57f
--- /dev/null
+++ b/yolov5/models/hub/yolov3.yaml
@@ -0,0 +1,51 @@
+# YOLOv5 🚀 by Ultralytics, AGPL-3.0 license
+
+# Parameters
+nc: 80 # number of classes
+depth_multiple: 1.0 # model depth multiple
+width_multiple: 1.0 # layer channel multiple
+anchors:
+ - [10,13, 16,30, 33,23] # P3/8
+ - [30,61, 62,45, 59,119] # P4/16
+ - [116,90, 156,198, 373,326] # P5/32
+
+# darknet53 backbone
+backbone:
+ # [from, number, module, args]
+ [[-1, 1, Conv, [32, 3, 1]], # 0
+ [-1, 1, Conv, [64, 3, 2]], # 1-P1/2
+ [-1, 1, Bottleneck, [64]],
+ [-1, 1, Conv, [128, 3, 2]], # 3-P2/4
+ [-1, 2, Bottleneck, [128]],
+ [-1, 1, Conv, [256, 3, 2]], # 5-P3/8
+ [-1, 8, Bottleneck, [256]],
+ [-1, 1, Conv, [512, 3, 2]], # 7-P4/16
+ [-1, 8, Bottleneck, [512]],
+ [-1, 1, Conv, [1024, 3, 2]], # 9-P5/32
+ [-1, 4, Bottleneck, [1024]], # 10
+ ]
+
+# YOLOv3 head
+head:
+ [[-1, 1, Bottleneck, [1024, False]],
+ [-1, 1, Conv, [512, 1, 1]],
+ [-1, 1, Conv, [1024, 3, 1]],
+ [-1, 1, Conv, [512, 1, 1]],
+ [-1, 1, Conv, [1024, 3, 1]], # 15 (P5/32-large)
+
+ [-2, 1, Conv, [256, 1, 1]],
+ [-1, 1, nn.Upsample, [None, 2, 'nearest']],
+ [[-1, 8], 1, Concat, [1]], # cat backbone P4
+ [-1, 1, Bottleneck, [512, False]],
+ [-1, 1, Bottleneck, [512, False]],
+ [-1, 1, Conv, [256, 1, 1]],
+ [-1, 1, Conv, [512, 3, 1]], # 22 (P4/16-medium)
+
+ [-2, 1, Conv, [128, 1, 1]],
+ [-1, 1, nn.Upsample, [None, 2, 'nearest']],
+ [[-1, 6], 1, Concat, [1]], # cat backbone P3
+ [-1, 1, Bottleneck, [256, False]],
+ [-1, 2, Bottleneck, [256, False]], # 27 (P3/8-small)
+
+ [[27, 22, 15], 1, Detect, [nc, anchors]], # Detect(P3, P4, P5)
+ ]
diff --git a/yolov5/models/hub/yolov5-bifpn.yaml b/yolov5/models/hub/yolov5-bifpn.yaml
new file mode 100644
index 0000000000000000000000000000000000000000..9dbdd4ee0580c4a5613548607b6970aefda8c03e
--- /dev/null
+++ b/yolov5/models/hub/yolov5-bifpn.yaml
@@ -0,0 +1,48 @@
+# YOLOv5 🚀 by Ultralytics, AGPL-3.0 license
+
+# Parameters
+nc: 80 # number of classes
+depth_multiple: 1.0 # model depth multiple
+width_multiple: 1.0 # layer channel multiple
+anchors:
+ - [10,13, 16,30, 33,23] # P3/8
+ - [30,61, 62,45, 59,119] # P4/16
+ - [116,90, 156,198, 373,326] # P5/32
+
+# YOLOv5 v6.0 backbone
+backbone:
+ # [from, number, module, args]
+ [[-1, 1, Conv, [64, 6, 2, 2]], # 0-P1/2
+ [-1, 1, Conv, [128, 3, 2]], # 1-P2/4
+ [-1, 3, C3, [128]],
+ [-1, 1, Conv, [256, 3, 2]], # 3-P3/8
+ [-1, 6, C3, [256]],
+ [-1, 1, Conv, [512, 3, 2]], # 5-P4/16
+ [-1, 9, C3, [512]],
+ [-1, 1, Conv, [1024, 3, 2]], # 7-P5/32
+ [-1, 3, C3, [1024]],
+ [-1, 1, SPPF, [1024, 5]], # 9
+ ]
+
+# YOLOv5 v6.0 BiFPN head
+head:
+ [[-1, 1, Conv, [512, 1, 1]],
+ [-1, 1, nn.Upsample, [None, 2, 'nearest']],
+ [[-1, 6], 1, Concat, [1]], # cat backbone P4
+ [-1, 3, C3, [512, False]], # 13
+
+ [-1, 1, Conv, [256, 1, 1]],
+ [-1, 1, nn.Upsample, [None, 2, 'nearest']],
+ [[-1, 4], 1, Concat, [1]], # cat backbone P3
+ [-1, 3, C3, [256, False]], # 17 (P3/8-small)
+
+ [-1, 1, Conv, [256, 3, 2]],
+ [[-1, 14, 6], 1, Concat, [1]], # cat P4 <--- BiFPN change
+ [-1, 3, C3, [512, False]], # 20 (P4/16-medium)
+
+ [-1, 1, Conv, [512, 3, 2]],
+ [[-1, 10], 1, Concat, [1]], # cat head P5
+ [-1, 3, C3, [1024, False]], # 23 (P5/32-large)
+
+ [[17, 20, 23], 1, Detect, [nc, anchors]], # Detect(P3, P4, P5)
+ ]
diff --git a/yolov5/models/hub/yolov5-fpn.yaml b/yolov5/models/hub/yolov5-fpn.yaml
new file mode 100644
index 0000000000000000000000000000000000000000..2292eb1185a0d4c14985c3039d01fcffa26b32fd
--- /dev/null
+++ b/yolov5/models/hub/yolov5-fpn.yaml
@@ -0,0 +1,42 @@
+# YOLOv5 🚀 by Ultralytics, AGPL-3.0 license
+
+# Parameters
+nc: 80 # number of classes
+depth_multiple: 1.0 # model depth multiple
+width_multiple: 1.0 # layer channel multiple
+anchors:
+ - [10,13, 16,30, 33,23] # P3/8
+ - [30,61, 62,45, 59,119] # P4/16
+ - [116,90, 156,198, 373,326] # P5/32
+
+# YOLOv5 v6.0 backbone
+backbone:
+ # [from, number, module, args]
+ [[-1, 1, Conv, [64, 6, 2, 2]], # 0-P1/2
+ [-1, 1, Conv, [128, 3, 2]], # 1-P2/4
+ [-1, 3, C3, [128]],
+ [-1, 1, Conv, [256, 3, 2]], # 3-P3/8
+ [-1, 6, C3, [256]],
+ [-1, 1, Conv, [512, 3, 2]], # 5-P4/16
+ [-1, 9, C3, [512]],
+ [-1, 1, Conv, [1024, 3, 2]], # 7-P5/32
+ [-1, 3, C3, [1024]],
+ [-1, 1, SPPF, [1024, 5]], # 9
+ ]
+
+# YOLOv5 v6.0 FPN head
+head:
+ [[-1, 3, C3, [1024, False]], # 10 (P5/32-large)
+
+ [-1, 1, nn.Upsample, [None, 2, 'nearest']],
+ [[-1, 6], 1, Concat, [1]], # cat backbone P4
+ [-1, 1, Conv, [512, 1, 1]],
+ [-1, 3, C3, [512, False]], # 14 (P4/16-medium)
+
+ [-1, 1, nn.Upsample, [None, 2, 'nearest']],
+ [[-1, 4], 1, Concat, [1]], # cat backbone P3
+ [-1, 1, Conv, [256, 1, 1]],
+ [-1, 3, C3, [256, False]], # 18 (P3/8-small)
+
+ [[18, 14, 10], 1, Detect, [nc, anchors]], # Detect(P3, P4, P5)
+ ]
diff --git a/yolov5/models/hub/yolov5-p2.yaml b/yolov5/models/hub/yolov5-p2.yaml
new file mode 100644
index 0000000000000000000000000000000000000000..2c0ae44841cc967bceee310c3b5606c7d191c6f7
--- /dev/null
+++ b/yolov5/models/hub/yolov5-p2.yaml
@@ -0,0 +1,54 @@
+# YOLOv5 🚀 by Ultralytics, AGPL-3.0 license
+
+# Parameters
+nc: 80 # number of classes
+depth_multiple: 1.0 # model depth multiple
+width_multiple: 1.0 # layer channel multiple
+anchors: 3 # AutoAnchor evolves 3 anchors per P output layer
+
+# YOLOv5 v6.0 backbone
+backbone:
+ # [from, number, module, args]
+ [[-1, 1, Conv, [64, 6, 2, 2]], # 0-P1/2
+ [-1, 1, Conv, [128, 3, 2]], # 1-P2/4
+ [-1, 3, C3, [128]],
+ [-1, 1, Conv, [256, 3, 2]], # 3-P3/8
+ [-1, 6, C3, [256]],
+ [-1, 1, Conv, [512, 3, 2]], # 5-P4/16
+ [-1, 9, C3, [512]],
+ [-1, 1, Conv, [1024, 3, 2]], # 7-P5/32
+ [-1, 3, C3, [1024]],
+ [-1, 1, SPPF, [1024, 5]], # 9
+ ]
+
+# YOLOv5 v6.0 head with (P2, P3, P4, P5) outputs
+head:
+ [[-1, 1, Conv, [512, 1, 1]],
+ [-1, 1, nn.Upsample, [None, 2, 'nearest']],
+ [[-1, 6], 1, Concat, [1]], # cat backbone P4
+ [-1, 3, C3, [512, False]], # 13
+
+ [-1, 1, Conv, [256, 1, 1]],
+ [-1, 1, nn.Upsample, [None, 2, 'nearest']],
+ [[-1, 4], 1, Concat, [1]], # cat backbone P3
+ [-1, 3, C3, [256, False]], # 17 (P3/8-small)
+
+ [-1, 1, Conv, [128, 1, 1]],
+ [-1, 1, nn.Upsample, [None, 2, 'nearest']],
+ [[-1, 2], 1, Concat, [1]], # cat backbone P2
+ [-1, 1, C3, [128, False]], # 21 (P2/4-xsmall)
+
+ [-1, 1, Conv, [128, 3, 2]],
+ [[-1, 18], 1, Concat, [1]], # cat head P3
+ [-1, 3, C3, [256, False]], # 24 (P3/8-small)
+
+ [-1, 1, Conv, [256, 3, 2]],
+ [[-1, 14], 1, Concat, [1]], # cat head P4
+ [-1, 3, C3, [512, False]], # 27 (P4/16-medium)
+
+ [-1, 1, Conv, [512, 3, 2]],
+ [[-1, 10], 1, Concat, [1]], # cat head P5
+ [-1, 3, C3, [1024, False]], # 30 (P5/32-large)
+
+ [[21, 24, 27, 30], 1, Detect, [nc, anchors]], # Detect(P2, P3, P4, P5)
+ ]
diff --git a/yolov5/models/hub/yolov5-p34.yaml b/yolov5/models/hub/yolov5-p34.yaml
new file mode 100644
index 0000000000000000000000000000000000000000..60ae3b4b6f30d0b1a5ba901d021de77d14953161
--- /dev/null
+++ b/yolov5/models/hub/yolov5-p34.yaml
@@ -0,0 +1,41 @@
+# YOLOv5 🚀 by Ultralytics, AGPL-3.0 license
+
+# Parameters
+nc: 80 # number of classes
+depth_multiple: 0.33 # model depth multiple
+width_multiple: 0.50 # layer channel multiple
+anchors: 3 # AutoAnchor evolves 3 anchors per P output layer
+
+# YOLOv5 v6.0 backbone
+backbone:
+ # [from, number, module, args]
+ [ [ -1, 1, Conv, [ 64, 6, 2, 2 ] ], # 0-P1/2
+ [ -1, 1, Conv, [ 128, 3, 2 ] ], # 1-P2/4
+ [ -1, 3, C3, [ 128 ] ],
+ [ -1, 1, Conv, [ 256, 3, 2 ] ], # 3-P3/8
+ [ -1, 6, C3, [ 256 ] ],
+ [ -1, 1, Conv, [ 512, 3, 2 ] ], # 5-P4/16
+ [ -1, 9, C3, [ 512 ] ],
+ [ -1, 1, Conv, [ 1024, 3, 2 ] ], # 7-P5/32
+ [ -1, 3, C3, [ 1024 ] ],
+ [ -1, 1, SPPF, [ 1024, 5 ] ], # 9
+ ]
+
+# YOLOv5 v6.0 head with (P3, P4) outputs
+head:
+ [ [ -1, 1, Conv, [ 512, 1, 1 ] ],
+ [ -1, 1, nn.Upsample, [ None, 2, 'nearest' ] ],
+ [ [ -1, 6 ], 1, Concat, [ 1 ] ], # cat backbone P4
+ [ -1, 3, C3, [ 512, False ] ], # 13
+
+ [ -1, 1, Conv, [ 256, 1, 1 ] ],
+ [ -1, 1, nn.Upsample, [ None, 2, 'nearest' ] ],
+ [ [ -1, 4 ], 1, Concat, [ 1 ] ], # cat backbone P3
+ [ -1, 3, C3, [ 256, False ] ], # 17 (P3/8-small)
+
+ [ -1, 1, Conv, [ 256, 3, 2 ] ],
+ [ [ -1, 14 ], 1, Concat, [ 1 ] ], # cat head P4
+ [ -1, 3, C3, [ 512, False ] ], # 20 (P4/16-medium)
+
+ [ [ 17, 20 ], 1, Detect, [ nc, anchors ] ], # Detect(P3, P4)
+ ]
diff --git a/yolov5/models/hub/yolov5-p6.yaml b/yolov5/models/hub/yolov5-p6.yaml
new file mode 100644
index 0000000000000000000000000000000000000000..a9e1b5f90c72dbe0c7257001761da7190c7b235b
--- /dev/null
+++ b/yolov5/models/hub/yolov5-p6.yaml
@@ -0,0 +1,56 @@
+# YOLOv5 🚀 by Ultralytics, AGPL-3.0 license
+
+# Parameters
+nc: 80 # number of classes
+depth_multiple: 1.0 # model depth multiple
+width_multiple: 1.0 # layer channel multiple
+anchors: 3 # AutoAnchor evolves 3 anchors per P output layer
+
+# YOLOv5 v6.0 backbone
+backbone:
+ # [from, number, module, args]
+ [[-1, 1, Conv, [64, 6, 2, 2]], # 0-P1/2
+ [-1, 1, Conv, [128, 3, 2]], # 1-P2/4
+ [-1, 3, C3, [128]],
+ [-1, 1, Conv, [256, 3, 2]], # 3-P3/8
+ [-1, 6, C3, [256]],
+ [-1, 1, Conv, [512, 3, 2]], # 5-P4/16
+ [-1, 9, C3, [512]],
+ [-1, 1, Conv, [768, 3, 2]], # 7-P5/32
+ [-1, 3, C3, [768]],
+ [-1, 1, Conv, [1024, 3, 2]], # 9-P6/64
+ [-1, 3, C3, [1024]],
+ [-1, 1, SPPF, [1024, 5]], # 11
+ ]
+
+# YOLOv5 v6.0 head with (P3, P4, P5, P6) outputs
+head:
+ [[-1, 1, Conv, [768, 1, 1]],
+ [-1, 1, nn.Upsample, [None, 2, 'nearest']],
+ [[-1, 8], 1, Concat, [1]], # cat backbone P5
+ [-1, 3, C3, [768, False]], # 15
+
+ [-1, 1, Conv, [512, 1, 1]],
+ [-1, 1, nn.Upsample, [None, 2, 'nearest']],
+ [[-1, 6], 1, Concat, [1]], # cat backbone P4
+ [-1, 3, C3, [512, False]], # 19
+
+ [-1, 1, Conv, [256, 1, 1]],
+ [-1, 1, nn.Upsample, [None, 2, 'nearest']],
+ [[-1, 4], 1, Concat, [1]], # cat backbone P3
+ [-1, 3, C3, [256, False]], # 23 (P3/8-small)
+
+ [-1, 1, Conv, [256, 3, 2]],
+ [[-1, 20], 1, Concat, [1]], # cat head P4
+ [-1, 3, C3, [512, False]], # 26 (P4/16-medium)
+
+ [-1, 1, Conv, [512, 3, 2]],
+ [[-1, 16], 1, Concat, [1]], # cat head P5
+ [-1, 3, C3, [768, False]], # 29 (P5/32-large)
+
+ [-1, 1, Conv, [768, 3, 2]],
+ [[-1, 12], 1, Concat, [1]], # cat head P6
+ [-1, 3, C3, [1024, False]], # 32 (P6/64-xlarge)
+
+ [[23, 26, 29, 32], 1, Detect, [nc, anchors]], # Detect(P3, P4, P5, P6)
+ ]
diff --git a/yolov5/models/hub/yolov5-p7.yaml b/yolov5/models/hub/yolov5-p7.yaml
new file mode 100644
index 0000000000000000000000000000000000000000..a502412f08877bd233580bf048558758f8d0c1c4
--- /dev/null
+++ b/yolov5/models/hub/yolov5-p7.yaml
@@ -0,0 +1,67 @@
+# YOLOv5 🚀 by Ultralytics, AGPL-3.0 license
+
+# Parameters
+nc: 80 # number of classes
+depth_multiple: 1.0 # model depth multiple
+width_multiple: 1.0 # layer channel multiple
+anchors: 3 # AutoAnchor evolves 3 anchors per P output layer
+
+# YOLOv5 v6.0 backbone
+backbone:
+ # [from, number, module, args]
+ [[-1, 1, Conv, [64, 6, 2, 2]], # 0-P1/2
+ [-1, 1, Conv, [128, 3, 2]], # 1-P2/4
+ [-1, 3, C3, [128]],
+ [-1, 1, Conv, [256, 3, 2]], # 3-P3/8
+ [-1, 6, C3, [256]],
+ [-1, 1, Conv, [512, 3, 2]], # 5-P4/16
+ [-1, 9, C3, [512]],
+ [-1, 1, Conv, [768, 3, 2]], # 7-P5/32
+ [-1, 3, C3, [768]],
+ [-1, 1, Conv, [1024, 3, 2]], # 9-P6/64
+ [-1, 3, C3, [1024]],
+ [-1, 1, Conv, [1280, 3, 2]], # 11-P7/128
+ [-1, 3, C3, [1280]],
+ [-1, 1, SPPF, [1280, 5]], # 13
+ ]
+
+# YOLOv5 v6.0 head with (P3, P4, P5, P6, P7) outputs
+head:
+ [[-1, 1, Conv, [1024, 1, 1]],
+ [-1, 1, nn.Upsample, [None, 2, 'nearest']],
+ [[-1, 10], 1, Concat, [1]], # cat backbone P6
+ [-1, 3, C3, [1024, False]], # 17
+
+ [-1, 1, Conv, [768, 1, 1]],
+ [-1, 1, nn.Upsample, [None, 2, 'nearest']],
+ [[-1, 8], 1, Concat, [1]], # cat backbone P5
+ [-1, 3, C3, [768, False]], # 21
+
+ [-1, 1, Conv, [512, 1, 1]],
+ [-1, 1, nn.Upsample, [None, 2, 'nearest']],
+ [[-1, 6], 1, Concat, [1]], # cat backbone P4
+ [-1, 3, C3, [512, False]], # 25
+
+ [-1, 1, Conv, [256, 1, 1]],
+ [-1, 1, nn.Upsample, [None, 2, 'nearest']],
+ [[-1, 4], 1, Concat, [1]], # cat backbone P3
+ [-1, 3, C3, [256, False]], # 29 (P3/8-small)
+
+ [-1, 1, Conv, [256, 3, 2]],
+ [[-1, 26], 1, Concat, [1]], # cat head P4
+ [-1, 3, C3, [512, False]], # 32 (P4/16-medium)
+
+ [-1, 1, Conv, [512, 3, 2]],
+ [[-1, 22], 1, Concat, [1]], # cat head P5
+ [-1, 3, C3, [768, False]], # 35 (P5/32-large)
+
+ [-1, 1, Conv, [768, 3, 2]],
+ [[-1, 18], 1, Concat, [1]], # cat head P6
+ [-1, 3, C3, [1024, False]], # 38 (P6/64-xlarge)
+
+ [-1, 1, Conv, [1024, 3, 2]],
+ [[-1, 14], 1, Concat, [1]], # cat head P7
+ [-1, 3, C3, [1280, False]], # 41 (P7/128-xxlarge)
+
+ [[29, 32, 35, 38, 41], 1, Detect, [nc, anchors]], # Detect(P3, P4, P5, P6, P7)
+ ]
diff --git a/yolov5/models/hub/yolov5-panet.yaml b/yolov5/models/hub/yolov5-panet.yaml
new file mode 100644
index 0000000000000000000000000000000000000000..5595e25738235718d5b4eb8672fc50301f0c043d
--- /dev/null
+++ b/yolov5/models/hub/yolov5-panet.yaml
@@ -0,0 +1,48 @@
+# YOLOv5 🚀 by Ultralytics, AGPL-3.0 license
+
+# Parameters
+nc: 80 # number of classes
+depth_multiple: 1.0 # model depth multiple
+width_multiple: 1.0 # layer channel multiple
+anchors:
+ - [10,13, 16,30, 33,23] # P3/8
+ - [30,61, 62,45, 59,119] # P4/16
+ - [116,90, 156,198, 373,326] # P5/32
+
+# YOLOv5 v6.0 backbone
+backbone:
+ # [from, number, module, args]
+ [[-1, 1, Conv, [64, 6, 2, 2]], # 0-P1/2
+ [-1, 1, Conv, [128, 3, 2]], # 1-P2/4
+ [-1, 3, C3, [128]],
+ [-1, 1, Conv, [256, 3, 2]], # 3-P3/8
+ [-1, 6, C3, [256]],
+ [-1, 1, Conv, [512, 3, 2]], # 5-P4/16
+ [-1, 9, C3, [512]],
+ [-1, 1, Conv, [1024, 3, 2]], # 7-P5/32
+ [-1, 3, C3, [1024]],
+ [-1, 1, SPPF, [1024, 5]], # 9
+ ]
+
+# YOLOv5 v6.0 PANet head
+head:
+ [[-1, 1, Conv, [512, 1, 1]],
+ [-1, 1, nn.Upsample, [None, 2, 'nearest']],
+ [[-1, 6], 1, Concat, [1]], # cat backbone P4
+ [-1, 3, C3, [512, False]], # 13
+
+ [-1, 1, Conv, [256, 1, 1]],
+ [-1, 1, nn.Upsample, [None, 2, 'nearest']],
+ [[-1, 4], 1, Concat, [1]], # cat backbone P3
+ [-1, 3, C3, [256, False]], # 17 (P3/8-small)
+
+ [-1, 1, Conv, [256, 3, 2]],
+ [[-1, 14], 1, Concat, [1]], # cat head P4
+ [-1, 3, C3, [512, False]], # 20 (P4/16-medium)
+
+ [-1, 1, Conv, [512, 3, 2]],
+ [[-1, 10], 1, Concat, [1]], # cat head P5
+ [-1, 3, C3, [1024, False]], # 23 (P5/32-large)
+
+ [[17, 20, 23], 1, Detect, [nc, anchors]], # Detect(P3, P4, P5)
+ ]
diff --git a/yolov5/models/hub/yolov5l6.yaml b/yolov5/models/hub/yolov5l6.yaml
new file mode 100644
index 0000000000000000000000000000000000000000..651dbb0251ae89817e6292e215e57ab7ddc9a92a
--- /dev/null
+++ b/yolov5/models/hub/yolov5l6.yaml
@@ -0,0 +1,60 @@
+# YOLOv5 🚀 by Ultralytics, AGPL-3.0 license
+
+# Parameters
+nc: 80 # number of classes
+depth_multiple: 1.0 # model depth multiple
+width_multiple: 1.0 # layer channel multiple
+anchors:
+ - [19,27, 44,40, 38,94] # P3/8
+ - [96,68, 86,152, 180,137] # P4/16
+ - [140,301, 303,264, 238,542] # P5/32
+ - [436,615, 739,380, 925,792] # P6/64
+
+# YOLOv5 v6.0 backbone
+backbone:
+ # [from, number, module, args]
+ [[-1, 1, Conv, [64, 6, 2, 2]], # 0-P1/2
+ [-1, 1, Conv, [128, 3, 2]], # 1-P2/4
+ [-1, 3, C3, [128]],
+ [-1, 1, Conv, [256, 3, 2]], # 3-P3/8
+ [-1, 6, C3, [256]],
+ [-1, 1, Conv, [512, 3, 2]], # 5-P4/16
+ [-1, 9, C3, [512]],
+ [-1, 1, Conv, [768, 3, 2]], # 7-P5/32
+ [-1, 3, C3, [768]],
+ [-1, 1, Conv, [1024, 3, 2]], # 9-P6/64
+ [-1, 3, C3, [1024]],
+ [-1, 1, SPPF, [1024, 5]], # 11
+ ]
+
+# YOLOv5 v6.0 head
+head:
+ [[-1, 1, Conv, [768, 1, 1]],
+ [-1, 1, nn.Upsample, [None, 2, 'nearest']],
+ [[-1, 8], 1, Concat, [1]], # cat backbone P5
+ [-1, 3, C3, [768, False]], # 15
+
+ [-1, 1, Conv, [512, 1, 1]],
+ [-1, 1, nn.Upsample, [None, 2, 'nearest']],
+ [[-1, 6], 1, Concat, [1]], # cat backbone P4
+ [-1, 3, C3, [512, False]], # 19
+
+ [-1, 1, Conv, [256, 1, 1]],
+ [-1, 1, nn.Upsample, [None, 2, 'nearest']],
+ [[-1, 4], 1, Concat, [1]], # cat backbone P3
+ [-1, 3, C3, [256, False]], # 23 (P3/8-small)
+
+ [-1, 1, Conv, [256, 3, 2]],
+ [[-1, 20], 1, Concat, [1]], # cat head P4
+ [-1, 3, C3, [512, False]], # 26 (P4/16-medium)
+
+ [-1, 1, Conv, [512, 3, 2]],
+ [[-1, 16], 1, Concat, [1]], # cat head P5
+ [-1, 3, C3, [768, False]], # 29 (P5/32-large)
+
+ [-1, 1, Conv, [768, 3, 2]],
+ [[-1, 12], 1, Concat, [1]], # cat head P6
+ [-1, 3, C3, [1024, False]], # 32 (P6/64-xlarge)
+
+ [[23, 26, 29, 32], 1, Detect, [nc, anchors]], # Detect(P3, P4, P5, P6)
+ ]
diff --git a/yolov5/models/hub/yolov5m6.yaml b/yolov5/models/hub/yolov5m6.yaml
new file mode 100644
index 0000000000000000000000000000000000000000..059b12b46929cc481a014298a5ab5ae2b2bdaf68
--- /dev/null
+++ b/yolov5/models/hub/yolov5m6.yaml
@@ -0,0 +1,60 @@
+# YOLOv5 🚀 by Ultralytics, AGPL-3.0 license
+
+# Parameters
+nc: 80 # number of classes
+depth_multiple: 0.67 # model depth multiple
+width_multiple: 0.75 # layer channel multiple
+anchors:
+ - [19,27, 44,40, 38,94] # P3/8
+ - [96,68, 86,152, 180,137] # P4/16
+ - [140,301, 303,264, 238,542] # P5/32
+ - [436,615, 739,380, 925,792] # P6/64
+
+# YOLOv5 v6.0 backbone
+backbone:
+ # [from, number, module, args]
+ [[-1, 1, Conv, [64, 6, 2, 2]], # 0-P1/2
+ [-1, 1, Conv, [128, 3, 2]], # 1-P2/4
+ [-1, 3, C3, [128]],
+ [-1, 1, Conv, [256, 3, 2]], # 3-P3/8
+ [-1, 6, C3, [256]],
+ [-1, 1, Conv, [512, 3, 2]], # 5-P4/16
+ [-1, 9, C3, [512]],
+ [-1, 1, Conv, [768, 3, 2]], # 7-P5/32
+ [-1, 3, C3, [768]],
+ [-1, 1, Conv, [1024, 3, 2]], # 9-P6/64
+ [-1, 3, C3, [1024]],
+ [-1, 1, SPPF, [1024, 5]], # 11
+ ]
+
+# YOLOv5 v6.0 head
+head:
+ [[-1, 1, Conv, [768, 1, 1]],
+ [-1, 1, nn.Upsample, [None, 2, 'nearest']],
+ [[-1, 8], 1, Concat, [1]], # cat backbone P5
+ [-1, 3, C3, [768, False]], # 15
+
+ [-1, 1, Conv, [512, 1, 1]],
+ [-1, 1, nn.Upsample, [None, 2, 'nearest']],
+ [[-1, 6], 1, Concat, [1]], # cat backbone P4
+ [-1, 3, C3, [512, False]], # 19
+
+ [-1, 1, Conv, [256, 1, 1]],
+ [-1, 1, nn.Upsample, [None, 2, 'nearest']],
+ [[-1, 4], 1, Concat, [1]], # cat backbone P3
+ [-1, 3, C3, [256, False]], # 23 (P3/8-small)
+
+ [-1, 1, Conv, [256, 3, 2]],
+ [[-1, 20], 1, Concat, [1]], # cat head P4
+ [-1, 3, C3, [512, False]], # 26 (P4/16-medium)
+
+ [-1, 1, Conv, [512, 3, 2]],
+ [[-1, 16], 1, Concat, [1]], # cat head P5
+ [-1, 3, C3, [768, False]], # 29 (P5/32-large)
+
+ [-1, 1, Conv, [768, 3, 2]],
+ [[-1, 12], 1, Concat, [1]], # cat head P6
+ [-1, 3, C3, [1024, False]], # 32 (P6/64-xlarge)
+
+ [[23, 26, 29, 32], 1, Detect, [nc, anchors]], # Detect(P3, P4, P5, P6)
+ ]
diff --git a/yolov5/models/hub/yolov5n6.yaml b/yolov5/models/hub/yolov5n6.yaml
new file mode 100644
index 0000000000000000000000000000000000000000..5052e7cbfc8b972a577af8c1668e0d475728268c
--- /dev/null
+++ b/yolov5/models/hub/yolov5n6.yaml
@@ -0,0 +1,60 @@
+# YOLOv5 🚀 by Ultralytics, AGPL-3.0 license
+
+# Parameters
+nc: 80 # number of classes
+depth_multiple: 0.33 # model depth multiple
+width_multiple: 0.25 # layer channel multiple
+anchors:
+ - [19,27, 44,40, 38,94] # P3/8
+ - [96,68, 86,152, 180,137] # P4/16
+ - [140,301, 303,264, 238,542] # P5/32
+ - [436,615, 739,380, 925,792] # P6/64
+
+# YOLOv5 v6.0 backbone
+backbone:
+ # [from, number, module, args]
+ [[-1, 1, Conv, [64, 6, 2, 2]], # 0-P1/2
+ [-1, 1, Conv, [128, 3, 2]], # 1-P2/4
+ [-1, 3, C3, [128]],
+ [-1, 1, Conv, [256, 3, 2]], # 3-P3/8
+ [-1, 6, C3, [256]],
+ [-1, 1, Conv, [512, 3, 2]], # 5-P4/16
+ [-1, 9, C3, [512]],
+ [-1, 1, Conv, [768, 3, 2]], # 7-P5/32
+ [-1, 3, C3, [768]],
+ [-1, 1, Conv, [1024, 3, 2]], # 9-P6/64
+ [-1, 3, C3, [1024]],
+ [-1, 1, SPPF, [1024, 5]], # 11
+ ]
+
+# YOLOv5 v6.0 head
+head:
+ [[-1, 1, Conv, [768, 1, 1]],
+ [-1, 1, nn.Upsample, [None, 2, 'nearest']],
+ [[-1, 8], 1, Concat, [1]], # cat backbone P5
+ [-1, 3, C3, [768, False]], # 15
+
+ [-1, 1, Conv, [512, 1, 1]],
+ [-1, 1, nn.Upsample, [None, 2, 'nearest']],
+ [[-1, 6], 1, Concat, [1]], # cat backbone P4
+ [-1, 3, C3, [512, False]], # 19
+
+ [-1, 1, Conv, [256, 1, 1]],
+ [-1, 1, nn.Upsample, [None, 2, 'nearest']],
+ [[-1, 4], 1, Concat, [1]], # cat backbone P3
+ [-1, 3, C3, [256, False]], # 23 (P3/8-small)
+
+ [-1, 1, Conv, [256, 3, 2]],
+ [[-1, 20], 1, Concat, [1]], # cat head P4
+ [-1, 3, C3, [512, False]], # 26 (P4/16-medium)
+
+ [-1, 1, Conv, [512, 3, 2]],
+ [[-1, 16], 1, Concat, [1]], # cat head P5
+ [-1, 3, C3, [768, False]], # 29 (P5/32-large)
+
+ [-1, 1, Conv, [768, 3, 2]],
+ [[-1, 12], 1, Concat, [1]], # cat head P6
+ [-1, 3, C3, [1024, False]], # 32 (P6/64-xlarge)
+
+ [[23, 26, 29, 32], 1, Detect, [nc, anchors]], # Detect(P3, P4, P5, P6)
+ ]
diff --git a/yolov5/models/hub/yolov5s-LeakyReLU.yaml b/yolov5/models/hub/yolov5s-LeakyReLU.yaml
new file mode 100644
index 0000000000000000000000000000000000000000..0368a78dcbb42c690a2ee81789c248d41009d665
--- /dev/null
+++ b/yolov5/models/hub/yolov5s-LeakyReLU.yaml
@@ -0,0 +1,49 @@
+# YOLOv5 🚀 by Ultralytics, AGPL-3.0 license
+
+# Parameters
+nc: 80 # number of classes
+activation: nn.LeakyReLU(0.1) # <----- Conv() activation used throughout entire YOLOv5 model
+depth_multiple: 0.33 # model depth multiple
+width_multiple: 0.50 # layer channel multiple
+anchors:
+ - [10,13, 16,30, 33,23] # P3/8
+ - [30,61, 62,45, 59,119] # P4/16
+ - [116,90, 156,198, 373,326] # P5/32
+
+# YOLOv5 v6.0 backbone
+backbone:
+ # [from, number, module, args]
+ [[-1, 1, Conv, [64, 6, 2, 2]], # 0-P1/2
+ [-1, 1, Conv, [128, 3, 2]], # 1-P2/4
+ [-1, 3, C3, [128]],
+ [-1, 1, Conv, [256, 3, 2]], # 3-P3/8
+ [-1, 6, C3, [256]],
+ [-1, 1, Conv, [512, 3, 2]], # 5-P4/16
+ [-1, 9, C3, [512]],
+ [-1, 1, Conv, [1024, 3, 2]], # 7-P5/32
+ [-1, 3, C3, [1024]],
+ [-1, 1, SPPF, [1024, 5]], # 9
+ ]
+
+# YOLOv5 v6.0 head
+head:
+ [[-1, 1, Conv, [512, 1, 1]],
+ [-1, 1, nn.Upsample, [None, 2, 'nearest']],
+ [[-1, 6], 1, Concat, [1]], # cat backbone P4
+ [-1, 3, C3, [512, False]], # 13
+
+ [-1, 1, Conv, [256, 1, 1]],
+ [-1, 1, nn.Upsample, [None, 2, 'nearest']],
+ [[-1, 4], 1, Concat, [1]], # cat backbone P3
+ [-1, 3, C3, [256, False]], # 17 (P3/8-small)
+
+ [-1, 1, Conv, [256, 3, 2]],
+ [[-1, 14], 1, Concat, [1]], # cat head P4
+ [-1, 3, C3, [512, False]], # 20 (P4/16-medium)
+
+ [-1, 1, Conv, [512, 3, 2]],
+ [[-1, 10], 1, Concat, [1]], # cat head P5
+ [-1, 3, C3, [1024, False]], # 23 (P5/32-large)
+
+ [[17, 20, 23], 1, Detect, [nc, anchors]], # Detect(P3, P4, P5)
+ ]
diff --git a/yolov5/models/hub/yolov5s-ghost.yaml b/yolov5/models/hub/yolov5s-ghost.yaml
new file mode 100644
index 0000000000000000000000000000000000000000..ce5238fa5dfcd629b01d5a4a29388d6a7646d6ec
--- /dev/null
+++ b/yolov5/models/hub/yolov5s-ghost.yaml
@@ -0,0 +1,48 @@
+# YOLOv5 🚀 by Ultralytics, AGPL-3.0 license
+
+# Parameters
+nc: 80 # number of classes
+depth_multiple: 0.33 # model depth multiple
+width_multiple: 0.50 # layer channel multiple
+anchors:
+ - [10,13, 16,30, 33,23] # P3/8
+ - [30,61, 62,45, 59,119] # P4/16
+ - [116,90, 156,198, 373,326] # P5/32
+
+# YOLOv5 v6.0 backbone
+backbone:
+ # [from, number, module, args]
+ [[-1, 1, Conv, [64, 6, 2, 2]], # 0-P1/2
+ [-1, 1, GhostConv, [128, 3, 2]], # 1-P2/4
+ [-1, 3, C3Ghost, [128]],
+ [-1, 1, GhostConv, [256, 3, 2]], # 3-P3/8
+ [-1, 6, C3Ghost, [256]],
+ [-1, 1, GhostConv, [512, 3, 2]], # 5-P4/16
+ [-1, 9, C3Ghost, [512]],
+ [-1, 1, GhostConv, [1024, 3, 2]], # 7-P5/32
+ [-1, 3, C3Ghost, [1024]],
+ [-1, 1, SPPF, [1024, 5]], # 9
+ ]
+
+# YOLOv5 v6.0 head
+head:
+ [[-1, 1, GhostConv, [512, 1, 1]],
+ [-1, 1, nn.Upsample, [None, 2, 'nearest']],
+ [[-1, 6], 1, Concat, [1]], # cat backbone P4
+ [-1, 3, C3Ghost, [512, False]], # 13
+
+ [-1, 1, GhostConv, [256, 1, 1]],
+ [-1, 1, nn.Upsample, [None, 2, 'nearest']],
+ [[-1, 4], 1, Concat, [1]], # cat backbone P3
+ [-1, 3, C3Ghost, [256, False]], # 17 (P3/8-small)
+
+ [-1, 1, GhostConv, [256, 3, 2]],
+ [[-1, 14], 1, Concat, [1]], # cat head P4
+ [-1, 3, C3Ghost, [512, False]], # 20 (P4/16-medium)
+
+ [-1, 1, GhostConv, [512, 3, 2]],
+ [[-1, 10], 1, Concat, [1]], # cat head P5
+ [-1, 3, C3Ghost, [1024, False]], # 23 (P5/32-large)
+
+ [[17, 20, 23], 1, Detect, [nc, anchors]], # Detect(P3, P4, P5)
+ ]
diff --git a/yolov5/models/hub/yolov5s-transformer.yaml b/yolov5/models/hub/yolov5s-transformer.yaml
new file mode 100644
index 0000000000000000000000000000000000000000..f5267163453c059a6b948567ec1fe5a9af18f7e5
--- /dev/null
+++ b/yolov5/models/hub/yolov5s-transformer.yaml
@@ -0,0 +1,48 @@
+# YOLOv5 🚀 by Ultralytics, AGPL-3.0 license
+
+# Parameters
+nc: 80 # number of classes
+depth_multiple: 0.33 # model depth multiple
+width_multiple: 0.50 # layer channel multiple
+anchors:
+ - [10,13, 16,30, 33,23] # P3/8
+ - [30,61, 62,45, 59,119] # P4/16
+ - [116,90, 156,198, 373,326] # P5/32
+
+# YOLOv5 v6.0 backbone
+backbone:
+ # [from, number, module, args]
+ [[-1, 1, Conv, [64, 6, 2, 2]], # 0-P1/2
+ [-1, 1, Conv, [128, 3, 2]], # 1-P2/4
+ [-1, 3, C3, [128]],
+ [-1, 1, Conv, [256, 3, 2]], # 3-P3/8
+ [-1, 6, C3, [256]],
+ [-1, 1, Conv, [512, 3, 2]], # 5-P4/16
+ [-1, 9, C3, [512]],
+ [-1, 1, Conv, [1024, 3, 2]], # 7-P5/32
+ [-1, 3, C3TR, [1024]], # 9 <--- C3TR() Transformer module
+ [-1, 1, SPPF, [1024, 5]], # 9
+ ]
+
+# YOLOv5 v6.0 head
+head:
+ [[-1, 1, Conv, [512, 1, 1]],
+ [-1, 1, nn.Upsample, [None, 2, 'nearest']],
+ [[-1, 6], 1, Concat, [1]], # cat backbone P4
+ [-1, 3, C3, [512, False]], # 13
+
+ [-1, 1, Conv, [256, 1, 1]],
+ [-1, 1, nn.Upsample, [None, 2, 'nearest']],
+ [[-1, 4], 1, Concat, [1]], # cat backbone P3
+ [-1, 3, C3, [256, False]], # 17 (P3/8-small)
+
+ [-1, 1, Conv, [256, 3, 2]],
+ [[-1, 14], 1, Concat, [1]], # cat head P4
+ [-1, 3, C3, [512, False]], # 20 (P4/16-medium)
+
+ [-1, 1, Conv, [512, 3, 2]],
+ [[-1, 10], 1, Concat, [1]], # cat head P5
+ [-1, 3, C3, [1024, False]], # 23 (P5/32-large)
+
+ [[17, 20, 23], 1, Detect, [nc, anchors]], # Detect(P3, P4, P5)
+ ]
diff --git a/yolov5/models/hub/yolov5s6.yaml b/yolov5/models/hub/yolov5s6.yaml
new file mode 100644
index 0000000000000000000000000000000000000000..2f39b0379e74dbfdd584896561337572d47ee580
--- /dev/null
+++ b/yolov5/models/hub/yolov5s6.yaml
@@ -0,0 +1,60 @@
+# YOLOv5 🚀 by Ultralytics, AGPL-3.0 license
+
+# Parameters
+nc: 80 # number of classes
+depth_multiple: 0.33 # model depth multiple
+width_multiple: 0.50 # layer channel multiple
+anchors:
+ - [19,27, 44,40, 38,94] # P3/8
+ - [96,68, 86,152, 180,137] # P4/16
+ - [140,301, 303,264, 238,542] # P5/32
+ - [436,615, 739,380, 925,792] # P6/64
+
+# YOLOv5 v6.0 backbone
+backbone:
+ # [from, number, module, args]
+ [[-1, 1, Conv, [64, 6, 2, 2]], # 0-P1/2
+ [-1, 1, Conv, [128, 3, 2]], # 1-P2/4
+ [-1, 3, C3, [128]],
+ [-1, 1, Conv, [256, 3, 2]], # 3-P3/8
+ [-1, 6, C3, [256]],
+ [-1, 1, Conv, [512, 3, 2]], # 5-P4/16
+ [-1, 9, C3, [512]],
+ [-1, 1, Conv, [768, 3, 2]], # 7-P5/32
+ [-1, 3, C3, [768]],
+ [-1, 1, Conv, [1024, 3, 2]], # 9-P6/64
+ [-1, 3, C3, [1024]],
+ [-1, 1, SPPF, [1024, 5]], # 11
+ ]
+
+# YOLOv5 v6.0 head
+head:
+ [[-1, 1, Conv, [768, 1, 1]],
+ [-1, 1, nn.Upsample, [None, 2, 'nearest']],
+ [[-1, 8], 1, Concat, [1]], # cat backbone P5
+ [-1, 3, C3, [768, False]], # 15
+
+ [-1, 1, Conv, [512, 1, 1]],
+ [-1, 1, nn.Upsample, [None, 2, 'nearest']],
+ [[-1, 6], 1, Concat, [1]], # cat backbone P4
+ [-1, 3, C3, [512, False]], # 19
+
+ [-1, 1, Conv, [256, 1, 1]],
+ [-1, 1, nn.Upsample, [None, 2, 'nearest']],
+ [[-1, 4], 1, Concat, [1]], # cat backbone P3
+ [-1, 3, C3, [256, False]], # 23 (P3/8-small)
+
+ [-1, 1, Conv, [256, 3, 2]],
+ [[-1, 20], 1, Concat, [1]], # cat head P4
+ [-1, 3, C3, [512, False]], # 26 (P4/16-medium)
+
+ [-1, 1, Conv, [512, 3, 2]],
+ [[-1, 16], 1, Concat, [1]], # cat head P5
+ [-1, 3, C3, [768, False]], # 29 (P5/32-large)
+
+ [-1, 1, Conv, [768, 3, 2]],
+ [[-1, 12], 1, Concat, [1]], # cat head P6
+ [-1, 3, C3, [1024, False]], # 32 (P6/64-xlarge)
+
+ [[23, 26, 29, 32], 1, Detect, [nc, anchors]], # Detect(P3, P4, P5, P6)
+ ]
diff --git a/yolov5/models/hub/yolov5x6.yaml b/yolov5/models/hub/yolov5x6.yaml
new file mode 100644
index 0000000000000000000000000000000000000000..e1edbcb8634c7c8abc68fa99bf53a2106700129c
--- /dev/null
+++ b/yolov5/models/hub/yolov5x6.yaml
@@ -0,0 +1,60 @@
+# YOLOv5 🚀 by Ultralytics, AGPL-3.0 license
+
+# Parameters
+nc: 80 # number of classes
+depth_multiple: 1.33 # model depth multiple
+width_multiple: 1.25 # layer channel multiple
+anchors:
+ - [19,27, 44,40, 38,94] # P3/8
+ - [96,68, 86,152, 180,137] # P4/16
+ - [140,301, 303,264, 238,542] # P5/32
+ - [436,615, 739,380, 925,792] # P6/64
+
+# YOLOv5 v6.0 backbone
+backbone:
+ # [from, number, module, args]
+ [[-1, 1, Conv, [64, 6, 2, 2]], # 0-P1/2
+ [-1, 1, Conv, [128, 3, 2]], # 1-P2/4
+ [-1, 3, C3, [128]],
+ [-1, 1, Conv, [256, 3, 2]], # 3-P3/8
+ [-1, 6, C3, [256]],
+ [-1, 1, Conv, [512, 3, 2]], # 5-P4/16
+ [-1, 9, C3, [512]],
+ [-1, 1, Conv, [768, 3, 2]], # 7-P5/32
+ [-1, 3, C3, [768]],
+ [-1, 1, Conv, [1024, 3, 2]], # 9-P6/64
+ [-1, 3, C3, [1024]],
+ [-1, 1, SPPF, [1024, 5]], # 11
+ ]
+
+# YOLOv5 v6.0 head
+head:
+ [[-1, 1, Conv, [768, 1, 1]],
+ [-1, 1, nn.Upsample, [None, 2, 'nearest']],
+ [[-1, 8], 1, Concat, [1]], # cat backbone P5
+ [-1, 3, C3, [768, False]], # 15
+
+ [-1, 1, Conv, [512, 1, 1]],
+ [-1, 1, nn.Upsample, [None, 2, 'nearest']],
+ [[-1, 6], 1, Concat, [1]], # cat backbone P4
+ [-1, 3, C3, [512, False]], # 19
+
+ [-1, 1, Conv, [256, 1, 1]],
+ [-1, 1, nn.Upsample, [None, 2, 'nearest']],
+ [[-1, 4], 1, Concat, [1]], # cat backbone P3
+ [-1, 3, C3, [256, False]], # 23 (P3/8-small)
+
+ [-1, 1, Conv, [256, 3, 2]],
+ [[-1, 20], 1, Concat, [1]], # cat head P4
+ [-1, 3, C3, [512, False]], # 26 (P4/16-medium)
+
+ [-1, 1, Conv, [512, 3, 2]],
+ [[-1, 16], 1, Concat, [1]], # cat head P5
+ [-1, 3, C3, [768, False]], # 29 (P5/32-large)
+
+ [-1, 1, Conv, [768, 3, 2]],
+ [[-1, 12], 1, Concat, [1]], # cat head P6
+ [-1, 3, C3, [1024, False]], # 32 (P6/64-xlarge)
+
+ [[23, 26, 29, 32], 1, Detect, [nc, anchors]], # Detect(P3, P4, P5, P6)
+ ]
diff --git a/yolov5/models/segment/yolov5l-seg.yaml b/yolov5/models/segment/yolov5l-seg.yaml
new file mode 100644
index 0000000000000000000000000000000000000000..71f80cc0805490c21adbb27bc093a04c4bc7b882
--- /dev/null
+++ b/yolov5/models/segment/yolov5l-seg.yaml
@@ -0,0 +1,48 @@
+# YOLOv5 🚀 by Ultralytics, AGPL-3.0 license
+
+# Parameters
+nc: 80 # number of classes
+depth_multiple: 1.0 # model depth multiple
+width_multiple: 1.0 # layer channel multiple
+anchors:
+ - [10,13, 16,30, 33,23] # P3/8
+ - [30,61, 62,45, 59,119] # P4/16
+ - [116,90, 156,198, 373,326] # P5/32
+
+# YOLOv5 v6.0 backbone
+backbone:
+ # [from, number, module, args]
+ [[-1, 1, Conv, [64, 6, 2, 2]], # 0-P1/2
+ [-1, 1, Conv, [128, 3, 2]], # 1-P2/4
+ [-1, 3, C3, [128]],
+ [-1, 1, Conv, [256, 3, 2]], # 3-P3/8
+ [-1, 6, C3, [256]],
+ [-1, 1, Conv, [512, 3, 2]], # 5-P4/16
+ [-1, 9, C3, [512]],
+ [-1, 1, Conv, [1024, 3, 2]], # 7-P5/32
+ [-1, 3, C3, [1024]],
+ [-1, 1, SPPF, [1024, 5]], # 9
+ ]
+
+# YOLOv5 v6.0 head
+head:
+ [[-1, 1, Conv, [512, 1, 1]],
+ [-1, 1, nn.Upsample, [None, 2, 'nearest']],
+ [[-1, 6], 1, Concat, [1]], # cat backbone P4
+ [-1, 3, C3, [512, False]], # 13
+
+ [-1, 1, Conv, [256, 1, 1]],
+ [-1, 1, nn.Upsample, [None, 2, 'nearest']],
+ [[-1, 4], 1, Concat, [1]], # cat backbone P3
+ [-1, 3, C3, [256, False]], # 17 (P3/8-small)
+
+ [-1, 1, Conv, [256, 3, 2]],
+ [[-1, 14], 1, Concat, [1]], # cat head P4
+ [-1, 3, C3, [512, False]], # 20 (P4/16-medium)
+
+ [-1, 1, Conv, [512, 3, 2]],
+ [[-1, 10], 1, Concat, [1]], # cat head P5
+ [-1, 3, C3, [1024, False]], # 23 (P5/32-large)
+
+ [[17, 20, 23], 1, Segment, [nc, anchors, 32, 256]], # Detect(P3, P4, P5)
+ ]
diff --git a/yolov5/models/segment/yolov5m-seg.yaml b/yolov5/models/segment/yolov5m-seg.yaml
new file mode 100644
index 0000000000000000000000000000000000000000..2b8e1db2818acbbcd6d2b340c2d950fc3108d4d4
--- /dev/null
+++ b/yolov5/models/segment/yolov5m-seg.yaml
@@ -0,0 +1,48 @@
+# YOLOv5 🚀 by Ultralytics, AGPL-3.0 license
+
+# Parameters
+nc: 80 # number of classes
+depth_multiple: 0.67 # model depth multiple
+width_multiple: 0.75 # layer channel multiple
+anchors:
+ - [10,13, 16,30, 33,23] # P3/8
+ - [30,61, 62,45, 59,119] # P4/16
+ - [116,90, 156,198, 373,326] # P5/32
+
+# YOLOv5 v6.0 backbone
+backbone:
+ # [from, number, module, args]
+ [[-1, 1, Conv, [64, 6, 2, 2]], # 0-P1/2
+ [-1, 1, Conv, [128, 3, 2]], # 1-P2/4
+ [-1, 3, C3, [128]],
+ [-1, 1, Conv, [256, 3, 2]], # 3-P3/8
+ [-1, 6, C3, [256]],
+ [-1, 1, Conv, [512, 3, 2]], # 5-P4/16
+ [-1, 9, C3, [512]],
+ [-1, 1, Conv, [1024, 3, 2]], # 7-P5/32
+ [-1, 3, C3, [1024]],
+ [-1, 1, SPPF, [1024, 5]], # 9
+ ]
+
+# YOLOv5 v6.0 head
+head:
+ [[-1, 1, Conv, [512, 1, 1]],
+ [-1, 1, nn.Upsample, [None, 2, 'nearest']],
+ [[-1, 6], 1, Concat, [1]], # cat backbone P4
+ [-1, 3, C3, [512, False]], # 13
+
+ [-1, 1, Conv, [256, 1, 1]],
+ [-1, 1, nn.Upsample, [None, 2, 'nearest']],
+ [[-1, 4], 1, Concat, [1]], # cat backbone P3
+ [-1, 3, C3, [256, False]], # 17 (P3/8-small)
+
+ [-1, 1, Conv, [256, 3, 2]],
+ [[-1, 14], 1, Concat, [1]], # cat head P4
+ [-1, 3, C3, [512, False]], # 20 (P4/16-medium)
+
+ [-1, 1, Conv, [512, 3, 2]],
+ [[-1, 10], 1, Concat, [1]], # cat head P5
+ [-1, 3, C3, [1024, False]], # 23 (P5/32-large)
+
+ [[17, 20, 23], 1, Segment, [nc, anchors, 32, 256]], # Detect(P3, P4, P5)
+ ]
diff --git a/yolov5/models/segment/yolov5n-seg.yaml b/yolov5/models/segment/yolov5n-seg.yaml
new file mode 100644
index 0000000000000000000000000000000000000000..1f67f8e3dfb05af091943100d7578e5f30770455
--- /dev/null
+++ b/yolov5/models/segment/yolov5n-seg.yaml
@@ -0,0 +1,48 @@
+# YOLOv5 🚀 by Ultralytics, AGPL-3.0 license
+
+# Parameters
+nc: 80 # number of classes
+depth_multiple: 0.33 # model depth multiple
+width_multiple: 0.25 # layer channel multiple
+anchors:
+ - [10,13, 16,30, 33,23] # P3/8
+ - [30,61, 62,45, 59,119] # P4/16
+ - [116,90, 156,198, 373,326] # P5/32
+
+# YOLOv5 v6.0 backbone
+backbone:
+ # [from, number, module, args]
+ [[-1, 1, Conv, [64, 6, 2, 2]], # 0-P1/2
+ [-1, 1, Conv, [128, 3, 2]], # 1-P2/4
+ [-1, 3, C3, [128]],
+ [-1, 1, Conv, [256, 3, 2]], # 3-P3/8
+ [-1, 6, C3, [256]],
+ [-1, 1, Conv, [512, 3, 2]], # 5-P4/16
+ [-1, 9, C3, [512]],
+ [-1, 1, Conv, [1024, 3, 2]], # 7-P5/32
+ [-1, 3, C3, [1024]],
+ [-1, 1, SPPF, [1024, 5]], # 9
+ ]
+
+# YOLOv5 v6.0 head
+head:
+ [[-1, 1, Conv, [512, 1, 1]],
+ [-1, 1, nn.Upsample, [None, 2, 'nearest']],
+ [[-1, 6], 1, Concat, [1]], # cat backbone P4
+ [-1, 3, C3, [512, False]], # 13
+
+ [-1, 1, Conv, [256, 1, 1]],
+ [-1, 1, nn.Upsample, [None, 2, 'nearest']],
+ [[-1, 4], 1, Concat, [1]], # cat backbone P3
+ [-1, 3, C3, [256, False]], # 17 (P3/8-small)
+
+ [-1, 1, Conv, [256, 3, 2]],
+ [[-1, 14], 1, Concat, [1]], # cat head P4
+ [-1, 3, C3, [512, False]], # 20 (P4/16-medium)
+
+ [-1, 1, Conv, [512, 3, 2]],
+ [[-1, 10], 1, Concat, [1]], # cat head P5
+ [-1, 3, C3, [1024, False]], # 23 (P5/32-large)
+
+ [[17, 20, 23], 1, Segment, [nc, anchors, 32, 256]], # Detect(P3, P4, P5)
+ ]
diff --git a/yolov5/models/segment/yolov5s-seg.yaml b/yolov5/models/segment/yolov5s-seg.yaml
new file mode 100644
index 0000000000000000000000000000000000000000..2ff2524ca9b559fa416854dba8af9d3e16eb8323
--- /dev/null
+++ b/yolov5/models/segment/yolov5s-seg.yaml
@@ -0,0 +1,48 @@
+# YOLOv5 🚀 by Ultralytics, AGPL-3.0 license
+
+# Parameters
+nc: 80 # number of classes
+depth_multiple: 0.33 # model depth multiple
+width_multiple: 0.5 # layer channel multiple
+anchors:
+ - [10,13, 16,30, 33,23] # P3/8
+ - [30,61, 62,45, 59,119] # P4/16
+ - [116,90, 156,198, 373,326] # P5/32
+
+# YOLOv5 v6.0 backbone
+backbone:
+ # [from, number, module, args]
+ [[-1, 1, Conv, [64, 6, 2, 2]], # 0-P1/2
+ [-1, 1, Conv, [128, 3, 2]], # 1-P2/4
+ [-1, 3, C3, [128]],
+ [-1, 1, Conv, [256, 3, 2]], # 3-P3/8
+ [-1, 6, C3, [256]],
+ [-1, 1, Conv, [512, 3, 2]], # 5-P4/16
+ [-1, 9, C3, [512]],
+ [-1, 1, Conv, [1024, 3, 2]], # 7-P5/32
+ [-1, 3, C3, [1024]],
+ [-1, 1, SPPF, [1024, 5]], # 9
+ ]
+
+# YOLOv5 v6.0 head
+head:
+ [[-1, 1, Conv, [512, 1, 1]],
+ [-1, 1, nn.Upsample, [None, 2, 'nearest']],
+ [[-1, 6], 1, Concat, [1]], # cat backbone P4
+ [-1, 3, C3, [512, False]], # 13
+
+ [-1, 1, Conv, [256, 1, 1]],
+ [-1, 1, nn.Upsample, [None, 2, 'nearest']],
+ [[-1, 4], 1, Concat, [1]], # cat backbone P3
+ [-1, 3, C3, [256, False]], # 17 (P3/8-small)
+
+ [-1, 1, Conv, [256, 3, 2]],
+ [[-1, 14], 1, Concat, [1]], # cat head P4
+ [-1, 3, C3, [512, False]], # 20 (P4/16-medium)
+
+ [-1, 1, Conv, [512, 3, 2]],
+ [[-1, 10], 1, Concat, [1]], # cat head P5
+ [-1, 3, C3, [1024, False]], # 23 (P5/32-large)
+
+ [[17, 20, 23], 1, Segment, [nc, anchors, 32, 256]], # Detect(P3, P4, P5)
+ ]
diff --git a/yolov5/models/segment/yolov5x-seg.yaml b/yolov5/models/segment/yolov5x-seg.yaml
new file mode 100644
index 0000000000000000000000000000000000000000..589f65c76f954c4db6bc88e4fb0a6d26be70556e
--- /dev/null
+++ b/yolov5/models/segment/yolov5x-seg.yaml
@@ -0,0 +1,48 @@
+# YOLOv5 🚀 by Ultralytics, AGPL-3.0 license
+
+# Parameters
+nc: 80 # number of classes
+depth_multiple: 1.33 # model depth multiple
+width_multiple: 1.25 # layer channel multiple
+anchors:
+ - [10,13, 16,30, 33,23] # P3/8
+ - [30,61, 62,45, 59,119] # P4/16
+ - [116,90, 156,198, 373,326] # P5/32
+
+# YOLOv5 v6.0 backbone
+backbone:
+ # [from, number, module, args]
+ [[-1, 1, Conv, [64, 6, 2, 2]], # 0-P1/2
+ [-1, 1, Conv, [128, 3, 2]], # 1-P2/4
+ [-1, 3, C3, [128]],
+ [-1, 1, Conv, [256, 3, 2]], # 3-P3/8
+ [-1, 6, C3, [256]],
+ [-1, 1, Conv, [512, 3, 2]], # 5-P4/16
+ [-1, 9, C3, [512]],
+ [-1, 1, Conv, [1024, 3, 2]], # 7-P5/32
+ [-1, 3, C3, [1024]],
+ [-1, 1, SPPF, [1024, 5]], # 9
+ ]
+
+# YOLOv5 v6.0 head
+head:
+ [[-1, 1, Conv, [512, 1, 1]],
+ [-1, 1, nn.Upsample, [None, 2, 'nearest']],
+ [[-1, 6], 1, Concat, [1]], # cat backbone P4
+ [-1, 3, C3, [512, False]], # 13
+
+ [-1, 1, Conv, [256, 1, 1]],
+ [-1, 1, nn.Upsample, [None, 2, 'nearest']],
+ [[-1, 4], 1, Concat, [1]], # cat backbone P3
+ [-1, 3, C3, [256, False]], # 17 (P3/8-small)
+
+ [-1, 1, Conv, [256, 3, 2]],
+ [[-1, 14], 1, Concat, [1]], # cat head P4
+ [-1, 3, C3, [512, False]], # 20 (P4/16-medium)
+
+ [-1, 1, Conv, [512, 3, 2]],
+ [[-1, 10], 1, Concat, [1]], # cat head P5
+ [-1, 3, C3, [1024, False]], # 23 (P5/32-large)
+
+ [[17, 20, 23], 1, Segment, [nc, anchors, 32, 256]], # Detect(P3, P4, P5)
+ ]
diff --git a/yolov5/models/tf.py b/yolov5/models/tf.py
new file mode 100644
index 0000000000000000000000000000000000000000..bc0a465d7edd094231bd7d2230eb9fe9270ed27e
--- /dev/null
+++ b/yolov5/models/tf.py
@@ -0,0 +1,608 @@
+# YOLOv5 🚀 by Ultralytics, AGPL-3.0 license
+"""
+TensorFlow, Keras and TFLite versions of YOLOv5
+Authored by https://github.com/zldrobit in PR https://github.com/ultralytics/yolov5/pull/1127
+
+Usage:
+ $ python models/tf.py --weights yolov5s.pt
+
+Export:
+ $ python export.py --weights yolov5s.pt --include saved_model pb tflite tfjs
+"""
+
+import argparse
+import sys
+from copy import deepcopy
+from pathlib import Path
+
+FILE = Path(__file__).resolve()
+ROOT = FILE.parents[1] # YOLOv5 root directory
+if str(ROOT) not in sys.path:
+ sys.path.append(str(ROOT)) # add ROOT to PATH
+# ROOT = ROOT.relative_to(Path.cwd()) # relative
+
+import numpy as np
+import tensorflow as tf
+import torch
+import torch.nn as nn
+from tensorflow import keras
+
+from models.common import (C3, SPP, SPPF, Bottleneck, BottleneckCSP, C3x, Concat, Conv, CrossConv, DWConv,
+ DWConvTranspose2d, Focus, autopad)
+from models.experimental import MixConv2d, attempt_load
+from models.yolo import Detect, Segment
+from utils.activations import SiLU
+from utils.general import LOGGER, make_divisible, print_args
+
+
+class TFBN(keras.layers.Layer):
+ # TensorFlow BatchNormalization wrapper
+ def __init__(self, w=None):
+ super().__init__()
+ self.bn = keras.layers.BatchNormalization(
+ beta_initializer=keras.initializers.Constant(w.bias.numpy()),
+ gamma_initializer=keras.initializers.Constant(w.weight.numpy()),
+ moving_mean_initializer=keras.initializers.Constant(w.running_mean.numpy()),
+ moving_variance_initializer=keras.initializers.Constant(w.running_var.numpy()),
+ epsilon=w.eps)
+
+ def call(self, inputs):
+ return self.bn(inputs)
+
+
+class TFPad(keras.layers.Layer):
+ # Pad inputs in spatial dimensions 1 and 2
+ def __init__(self, pad):
+ super().__init__()
+ if isinstance(pad, int):
+ self.pad = tf.constant([[0, 0], [pad, pad], [pad, pad], [0, 0]])
+ else: # tuple/list
+ self.pad = tf.constant([[0, 0], [pad[0], pad[0]], [pad[1], pad[1]], [0, 0]])
+
+ def call(self, inputs):
+ return tf.pad(inputs, self.pad, mode='constant', constant_values=0)
+
+
+class TFConv(keras.layers.Layer):
+ # Standard convolution
+ def __init__(self, c1, c2, k=1, s=1, p=None, g=1, act=True, w=None):
+ # ch_in, ch_out, weights, kernel, stride, padding, groups
+ super().__init__()
+ assert g == 1, "TF v2.2 Conv2D does not support 'groups' argument"
+ # TensorFlow convolution padding is inconsistent with PyTorch (e.g. k=3 s=2 'SAME' padding)
+ # see https://stackoverflow.com/questions/52975843/comparing-conv2d-with-padding-between-tensorflow-and-pytorch
+ conv = keras.layers.Conv2D(
+ filters=c2,
+ kernel_size=k,
+ strides=s,
+ padding='SAME' if s == 1 else 'VALID',
+ use_bias=not hasattr(w, 'bn'),
+ kernel_initializer=keras.initializers.Constant(w.conv.weight.permute(2, 3, 1, 0).numpy()),
+ bias_initializer='zeros' if hasattr(w, 'bn') else keras.initializers.Constant(w.conv.bias.numpy()))
+ self.conv = conv if s == 1 else keras.Sequential([TFPad(autopad(k, p)), conv])
+ self.bn = TFBN(w.bn) if hasattr(w, 'bn') else tf.identity
+ self.act = activations(w.act) if act else tf.identity
+
+ def call(self, inputs):
+ return self.act(self.bn(self.conv(inputs)))
+
+
+class TFDWConv(keras.layers.Layer):
+ # Depthwise convolution
+ def __init__(self, c1, c2, k=1, s=1, p=None, act=True, w=None):
+ # ch_in, ch_out, weights, kernel, stride, padding, groups
+ super().__init__()
+ assert c2 % c1 == 0, f'TFDWConv() output={c2} must be a multiple of input={c1} channels'
+ conv = keras.layers.DepthwiseConv2D(
+ kernel_size=k,
+ depth_multiplier=c2 // c1,
+ strides=s,
+ padding='SAME' if s == 1 else 'VALID',
+ use_bias=not hasattr(w, 'bn'),
+ depthwise_initializer=keras.initializers.Constant(w.conv.weight.permute(2, 3, 1, 0).numpy()),
+ bias_initializer='zeros' if hasattr(w, 'bn') else keras.initializers.Constant(w.conv.bias.numpy()))
+ self.conv = conv if s == 1 else keras.Sequential([TFPad(autopad(k, p)), conv])
+ self.bn = TFBN(w.bn) if hasattr(w, 'bn') else tf.identity
+ self.act = activations(w.act) if act else tf.identity
+
+ def call(self, inputs):
+ return self.act(self.bn(self.conv(inputs)))
+
+
+class TFDWConvTranspose2d(keras.layers.Layer):
+ # Depthwise ConvTranspose2d
+ def __init__(self, c1, c2, k=1, s=1, p1=0, p2=0, w=None):
+ # ch_in, ch_out, weights, kernel, stride, padding, groups
+ super().__init__()
+ assert c1 == c2, f'TFDWConv() output={c2} must be equal to input={c1} channels'
+ assert k == 4 and p1 == 1, 'TFDWConv() only valid for k=4 and p1=1'
+ weight, bias = w.weight.permute(2, 3, 1, 0).numpy(), w.bias.numpy()
+ self.c1 = c1
+ self.conv = [
+ keras.layers.Conv2DTranspose(filters=1,
+ kernel_size=k,
+ strides=s,
+ padding='VALID',
+ output_padding=p2,
+ use_bias=True,
+ kernel_initializer=keras.initializers.Constant(weight[..., i:i + 1]),
+ bias_initializer=keras.initializers.Constant(bias[i])) for i in range(c1)]
+
+ def call(self, inputs):
+ return tf.concat([m(x) for m, x in zip(self.conv, tf.split(inputs, self.c1, 3))], 3)[:, 1:-1, 1:-1]
+
+
+class TFFocus(keras.layers.Layer):
+ # Focus wh information into c-space
+ def __init__(self, c1, c2, k=1, s=1, p=None, g=1, act=True, w=None):
+ # ch_in, ch_out, kernel, stride, padding, groups
+ super().__init__()
+ self.conv = TFConv(c1 * 4, c2, k, s, p, g, act, w.conv)
+
+ def call(self, inputs): # x(b,w,h,c) -> y(b,w/2,h/2,4c)
+ # inputs = inputs / 255 # normalize 0-255 to 0-1
+ inputs = [inputs[:, ::2, ::2, :], inputs[:, 1::2, ::2, :], inputs[:, ::2, 1::2, :], inputs[:, 1::2, 1::2, :]]
+ return self.conv(tf.concat(inputs, 3))
+
+
+class TFBottleneck(keras.layers.Layer):
+ # Standard bottleneck
+ def __init__(self, c1, c2, shortcut=True, g=1, e=0.5, w=None): # ch_in, ch_out, shortcut, groups, expansion
+ super().__init__()
+ c_ = int(c2 * e) # hidden channels
+ self.cv1 = TFConv(c1, c_, 1, 1, w=w.cv1)
+ self.cv2 = TFConv(c_, c2, 3, 1, g=g, w=w.cv2)
+ self.add = shortcut and c1 == c2
+
+ def call(self, inputs):
+ return inputs + self.cv2(self.cv1(inputs)) if self.add else self.cv2(self.cv1(inputs))
+
+
+class TFCrossConv(keras.layers.Layer):
+ # Cross Convolution
+ def __init__(self, c1, c2, k=3, s=1, g=1, e=1.0, shortcut=False, w=None):
+ super().__init__()
+ c_ = int(c2 * e) # hidden channels
+ self.cv1 = TFConv(c1, c_, (1, k), (1, s), w=w.cv1)
+ self.cv2 = TFConv(c_, c2, (k, 1), (s, 1), g=g, w=w.cv2)
+ self.add = shortcut and c1 == c2
+
+ def call(self, inputs):
+ return inputs + self.cv2(self.cv1(inputs)) if self.add else self.cv2(self.cv1(inputs))
+
+
+class TFConv2d(keras.layers.Layer):
+ # Substitution for PyTorch nn.Conv2D
+ def __init__(self, c1, c2, k, s=1, g=1, bias=True, w=None):
+ super().__init__()
+ assert g == 1, "TF v2.2 Conv2D does not support 'groups' argument"
+ self.conv = keras.layers.Conv2D(filters=c2,
+ kernel_size=k,
+ strides=s,
+ padding='VALID',
+ use_bias=bias,
+ kernel_initializer=keras.initializers.Constant(
+ w.weight.permute(2, 3, 1, 0).numpy()),
+ bias_initializer=keras.initializers.Constant(w.bias.numpy()) if bias else None)
+
+ def call(self, inputs):
+ return self.conv(inputs)
+
+
+class TFBottleneckCSP(keras.layers.Layer):
+ # CSP Bottleneck https://github.com/WongKinYiu/CrossStagePartialNetworks
+ def __init__(self, c1, c2, n=1, shortcut=True, g=1, e=0.5, w=None):
+ # ch_in, ch_out, number, shortcut, groups, expansion
+ super().__init__()
+ c_ = int(c2 * e) # hidden channels
+ self.cv1 = TFConv(c1, c_, 1, 1, w=w.cv1)
+ self.cv2 = TFConv2d(c1, c_, 1, 1, bias=False, w=w.cv2)
+ self.cv3 = TFConv2d(c_, c_, 1, 1, bias=False, w=w.cv3)
+ self.cv4 = TFConv(2 * c_, c2, 1, 1, w=w.cv4)
+ self.bn = TFBN(w.bn)
+ self.act = lambda x: keras.activations.swish(x)
+ self.m = keras.Sequential([TFBottleneck(c_, c_, shortcut, g, e=1.0, w=w.m[j]) for j in range(n)])
+
+ def call(self, inputs):
+ y1 = self.cv3(self.m(self.cv1(inputs)))
+ y2 = self.cv2(inputs)
+ return self.cv4(self.act(self.bn(tf.concat((y1, y2), axis=3))))
+
+
+class TFC3(keras.layers.Layer):
+ # CSP Bottleneck with 3 convolutions
+ def __init__(self, c1, c2, n=1, shortcut=True, g=1, e=0.5, w=None):
+ # ch_in, ch_out, number, shortcut, groups, expansion
+ super().__init__()
+ c_ = int(c2 * e) # hidden channels
+ self.cv1 = TFConv(c1, c_, 1, 1, w=w.cv1)
+ self.cv2 = TFConv(c1, c_, 1, 1, w=w.cv2)
+ self.cv3 = TFConv(2 * c_, c2, 1, 1, w=w.cv3)
+ self.m = keras.Sequential([TFBottleneck(c_, c_, shortcut, g, e=1.0, w=w.m[j]) for j in range(n)])
+
+ def call(self, inputs):
+ return self.cv3(tf.concat((self.m(self.cv1(inputs)), self.cv2(inputs)), axis=3))
+
+
+class TFC3x(keras.layers.Layer):
+ # 3 module with cross-convolutions
+ def __init__(self, c1, c2, n=1, shortcut=True, g=1, e=0.5, w=None):
+ # ch_in, ch_out, number, shortcut, groups, expansion
+ super().__init__()
+ c_ = int(c2 * e) # hidden channels
+ self.cv1 = TFConv(c1, c_, 1, 1, w=w.cv1)
+ self.cv2 = TFConv(c1, c_, 1, 1, w=w.cv2)
+ self.cv3 = TFConv(2 * c_, c2, 1, 1, w=w.cv3)
+ self.m = keras.Sequential([
+ TFCrossConv(c_, c_, k=3, s=1, g=g, e=1.0, shortcut=shortcut, w=w.m[j]) for j in range(n)])
+
+ def call(self, inputs):
+ return self.cv3(tf.concat((self.m(self.cv1(inputs)), self.cv2(inputs)), axis=3))
+
+
+class TFSPP(keras.layers.Layer):
+ # Spatial pyramid pooling layer used in YOLOv3-SPP
+ def __init__(self, c1, c2, k=(5, 9, 13), w=None):
+ super().__init__()
+ c_ = c1 // 2 # hidden channels
+ self.cv1 = TFConv(c1, c_, 1, 1, w=w.cv1)
+ self.cv2 = TFConv(c_ * (len(k) + 1), c2, 1, 1, w=w.cv2)
+ self.m = [keras.layers.MaxPool2D(pool_size=x, strides=1, padding='SAME') for x in k]
+
+ def call(self, inputs):
+ x = self.cv1(inputs)
+ return self.cv2(tf.concat([x] + [m(x) for m in self.m], 3))
+
+
+class TFSPPF(keras.layers.Layer):
+ # Spatial pyramid pooling-Fast layer
+ def __init__(self, c1, c2, k=5, w=None):
+ super().__init__()
+ c_ = c1 // 2 # hidden channels
+ self.cv1 = TFConv(c1, c_, 1, 1, w=w.cv1)
+ self.cv2 = TFConv(c_ * 4, c2, 1, 1, w=w.cv2)
+ self.m = keras.layers.MaxPool2D(pool_size=k, strides=1, padding='SAME')
+
+ def call(self, inputs):
+ x = self.cv1(inputs)
+ y1 = self.m(x)
+ y2 = self.m(y1)
+ return self.cv2(tf.concat([x, y1, y2, self.m(y2)], 3))
+
+
+class TFDetect(keras.layers.Layer):
+ # TF YOLOv5 Detect layer
+ def __init__(self, nc=80, anchors=(), ch=(), imgsz=(640, 640), w=None): # detection layer
+ super().__init__()
+ self.stride = tf.convert_to_tensor(w.stride.numpy(), dtype=tf.float32)
+ self.nc = nc # number of classes
+ self.no = nc + 5 # number of outputs per anchor
+ self.nl = len(anchors) # number of detection layers
+ self.na = len(anchors[0]) // 2 # number of anchors
+ self.grid = [tf.zeros(1)] * self.nl # init grid
+ self.anchors = tf.convert_to_tensor(w.anchors.numpy(), dtype=tf.float32)
+ self.anchor_grid = tf.reshape(self.anchors * tf.reshape(self.stride, [self.nl, 1, 1]), [self.nl, 1, -1, 1, 2])
+ self.m = [TFConv2d(x, self.no * self.na, 1, w=w.m[i]) for i, x in enumerate(ch)]
+ self.training = False # set to False after building model
+ self.imgsz = imgsz
+ for i in range(self.nl):
+ ny, nx = self.imgsz[0] // self.stride[i], self.imgsz[1] // self.stride[i]
+ self.grid[i] = self._make_grid(nx, ny)
+
+ def call(self, inputs):
+ z = [] # inference output
+ x = []
+ for i in range(self.nl):
+ x.append(self.m[i](inputs[i]))
+ # x(bs,20,20,255) to x(bs,3,20,20,85)
+ ny, nx = self.imgsz[0] // self.stride[i], self.imgsz[1] // self.stride[i]
+ x[i] = tf.reshape(x[i], [-1, ny * nx, self.na, self.no])
+
+ if not self.training: # inference
+ y = x[i]
+ grid = tf.transpose(self.grid[i], [0, 2, 1, 3]) - 0.5
+ anchor_grid = tf.transpose(self.anchor_grid[i], [0, 2, 1, 3]) * 4
+ xy = (tf.sigmoid(y[..., 0:2]) * 2 + grid) * self.stride[i] # xy
+ wh = tf.sigmoid(y[..., 2:4]) ** 2 * anchor_grid
+ # Normalize xywh to 0-1 to reduce calibration error
+ xy /= tf.constant([[self.imgsz[1], self.imgsz[0]]], dtype=tf.float32)
+ wh /= tf.constant([[self.imgsz[1], self.imgsz[0]]], dtype=tf.float32)
+ y = tf.concat([xy, wh, tf.sigmoid(y[..., 4:5 + self.nc]), y[..., 5 + self.nc:]], -1)
+ z.append(tf.reshape(y, [-1, self.na * ny * nx, self.no]))
+
+ return tf.transpose(x, [0, 2, 1, 3]) if self.training else (tf.concat(z, 1),)
+
+ @staticmethod
+ def _make_grid(nx=20, ny=20):
+ # yv, xv = torch.meshgrid([torch.arange(ny), torch.arange(nx)])
+ # return torch.stack((xv, yv), 2).view((1, 1, ny, nx, 2)).float()
+ xv, yv = tf.meshgrid(tf.range(nx), tf.range(ny))
+ return tf.cast(tf.reshape(tf.stack([xv, yv], 2), [1, 1, ny * nx, 2]), dtype=tf.float32)
+
+
+class TFSegment(TFDetect):
+ # YOLOv5 Segment head for segmentation models
+ def __init__(self, nc=80, anchors=(), nm=32, npr=256, ch=(), imgsz=(640, 640), w=None):
+ super().__init__(nc, anchors, ch, imgsz, w)
+ self.nm = nm # number of masks
+ self.npr = npr # number of protos
+ self.no = 5 + nc + self.nm # number of outputs per anchor
+ self.m = [TFConv2d(x, self.no * self.na, 1, w=w.m[i]) for i, x in enumerate(ch)] # output conv
+ self.proto = TFProto(ch[0], self.npr, self.nm, w=w.proto) # protos
+ self.detect = TFDetect.call
+
+ def call(self, x):
+ p = self.proto(x[0])
+ # p = TFUpsample(None, scale_factor=4, mode='nearest')(self.proto(x[0])) # (optional) full-size protos
+ p = tf.transpose(p, [0, 3, 1, 2]) # from shape(1,160,160,32) to shape(1,32,160,160)
+ x = self.detect(self, x)
+ return (x, p) if self.training else (x[0], p)
+
+
+class TFProto(keras.layers.Layer):
+
+ def __init__(self, c1, c_=256, c2=32, w=None):
+ super().__init__()
+ self.cv1 = TFConv(c1, c_, k=3, w=w.cv1)
+ self.upsample = TFUpsample(None, scale_factor=2, mode='nearest')
+ self.cv2 = TFConv(c_, c_, k=3, w=w.cv2)
+ self.cv3 = TFConv(c_, c2, w=w.cv3)
+
+ def call(self, inputs):
+ return self.cv3(self.cv2(self.upsample(self.cv1(inputs))))
+
+
+class TFUpsample(keras.layers.Layer):
+ # TF version of torch.nn.Upsample()
+ def __init__(self, size, scale_factor, mode, w=None): # warning: all arguments needed including 'w'
+ super().__init__()
+ assert scale_factor % 2 == 0, 'scale_factor must be multiple of 2'
+ self.upsample = lambda x: tf.image.resize(x, (x.shape[1] * scale_factor, x.shape[2] * scale_factor), mode)
+ # self.upsample = keras.layers.UpSampling2D(size=scale_factor, interpolation=mode)
+ # with default arguments: align_corners=False, half_pixel_centers=False
+ # self.upsample = lambda x: tf.raw_ops.ResizeNearestNeighbor(images=x,
+ # size=(x.shape[1] * 2, x.shape[2] * 2))
+
+ def call(self, inputs):
+ return self.upsample(inputs)
+
+
+class TFConcat(keras.layers.Layer):
+ # TF version of torch.concat()
+ def __init__(self, dimension=1, w=None):
+ super().__init__()
+ assert dimension == 1, 'convert only NCHW to NHWC concat'
+ self.d = 3
+
+ def call(self, inputs):
+ return tf.concat(inputs, self.d)
+
+
+def parse_model(d, ch, model, imgsz): # model_dict, input_channels(3)
+ LOGGER.info(f"\n{'':>3}{'from':>18}{'n':>3}{'params':>10} {'module':<40}{'arguments':<30}")
+ anchors, nc, gd, gw = d['anchors'], d['nc'], d['depth_multiple'], d['width_multiple']
+ na = (len(anchors[0]) // 2) if isinstance(anchors, list) else anchors # number of anchors
+ no = na * (nc + 5) # number of outputs = anchors * (classes + 5)
+
+ layers, save, c2 = [], [], ch[-1] # layers, savelist, ch out
+ for i, (f, n, m, args) in enumerate(d['backbone'] + d['head']): # from, number, module, args
+ m_str = m
+ m = eval(m) if isinstance(m, str) else m # eval strings
+ for j, a in enumerate(args):
+ try:
+ args[j] = eval(a) if isinstance(a, str) else a # eval strings
+ except NameError:
+ pass
+
+ n = max(round(n * gd), 1) if n > 1 else n # depth gain
+ if m in [
+ nn.Conv2d, Conv, DWConv, DWConvTranspose2d, Bottleneck, SPP, SPPF, MixConv2d, Focus, CrossConv,
+ BottleneckCSP, C3, C3x]:
+ c1, c2 = ch[f], args[0]
+ c2 = make_divisible(c2 * gw, 8) if c2 != no else c2
+
+ args = [c1, c2, *args[1:]]
+ if m in [BottleneckCSP, C3, C3x]:
+ args.insert(2, n)
+ n = 1
+ elif m is nn.BatchNorm2d:
+ args = [ch[f]]
+ elif m is Concat:
+ c2 = sum(ch[-1 if x == -1 else x + 1] for x in f)
+ elif m in [Detect, Segment]:
+ args.append([ch[x + 1] for x in f])
+ if isinstance(args[1], int): # number of anchors
+ args[1] = [list(range(args[1] * 2))] * len(f)
+ if m is Segment:
+ args[3] = make_divisible(args[3] * gw, 8)
+ args.append(imgsz)
+ else:
+ c2 = ch[f]
+
+ tf_m = eval('TF' + m_str.replace('nn.', ''))
+ m_ = keras.Sequential([tf_m(*args, w=model.model[i][j]) for j in range(n)]) if n > 1 \
+ else tf_m(*args, w=model.model[i]) # module
+
+ torch_m_ = nn.Sequential(*(m(*args) for _ in range(n))) if n > 1 else m(*args) # module
+ t = str(m)[8:-2].replace('__main__.', '') # module type
+ np = sum(x.numel() for x in torch_m_.parameters()) # number params
+ m_.i, m_.f, m_.type, m_.np = i, f, t, np # attach index, 'from' index, type, number params
+ LOGGER.info(f'{i:>3}{str(f):>18}{str(n):>3}{np:>10} {t:<40}{str(args):<30}') # print
+ save.extend(x % i for x in ([f] if isinstance(f, int) else f) if x != -1) # append to savelist
+ layers.append(m_)
+ ch.append(c2)
+ return keras.Sequential(layers), sorted(save)
+
+
+class TFModel:
+ # TF YOLOv5 model
+ def __init__(self, cfg='yolov5s.yaml', ch=3, nc=None, model=None, imgsz=(640, 640)): # model, channels, classes
+ super().__init__()
+ if isinstance(cfg, dict):
+ self.yaml = cfg # model dict
+ else: # is *.yaml
+ import yaml # for torch hub
+ self.yaml_file = Path(cfg).name
+ with open(cfg) as f:
+ self.yaml = yaml.load(f, Loader=yaml.FullLoader) # model dict
+
+ # Define model
+ if nc and nc != self.yaml['nc']:
+ LOGGER.info(f"Overriding {cfg} nc={self.yaml['nc']} with nc={nc}")
+ self.yaml['nc'] = nc # override yaml value
+ self.model, self.savelist = parse_model(deepcopy(self.yaml), ch=[ch], model=model, imgsz=imgsz)
+
+ def predict(self,
+ inputs,
+ tf_nms=False,
+ agnostic_nms=False,
+ topk_per_class=100,
+ topk_all=100,
+ iou_thres=0.45,
+ conf_thres=0.25):
+ y = [] # outputs
+ x = inputs
+ for m in self.model.layers:
+ if m.f != -1: # if not from previous layer
+ x = y[m.f] if isinstance(m.f, int) else [x if j == -1 else y[j] for j in m.f] # from earlier layers
+
+ x = m(x) # run
+ y.append(x if m.i in self.savelist else None) # save output
+
+ # Add TensorFlow NMS
+ if tf_nms:
+ boxes = self._xywh2xyxy(x[0][..., :4])
+ probs = x[0][:, :, 4:5]
+ classes = x[0][:, :, 5:]
+ scores = probs * classes
+ if agnostic_nms:
+ nms = AgnosticNMS()((boxes, classes, scores), topk_all, iou_thres, conf_thres)
+ else:
+ boxes = tf.expand_dims(boxes, 2)
+ nms = tf.image.combined_non_max_suppression(boxes,
+ scores,
+ topk_per_class,
+ topk_all,
+ iou_thres,
+ conf_thres,
+ clip_boxes=False)
+ return (nms,)
+ return x # output [1,6300,85] = [xywh, conf, class0, class1, ...]
+ # x = x[0] # [x(1,6300,85), ...] to x(6300,85)
+ # xywh = x[..., :4] # x(6300,4) boxes
+ # conf = x[..., 4:5] # x(6300,1) confidences
+ # cls = tf.reshape(tf.cast(tf.argmax(x[..., 5:], axis=1), tf.float32), (-1, 1)) # x(6300,1) classes
+ # return tf.concat([conf, cls, xywh], 1)
+
+ @staticmethod
+ def _xywh2xyxy(xywh):
+ # Convert nx4 boxes from [x, y, w, h] to [x1, y1, x2, y2] where xy1=top-left, xy2=bottom-right
+ x, y, w, h = tf.split(xywh, num_or_size_splits=4, axis=-1)
+ return tf.concat([x - w / 2, y - h / 2, x + w / 2, y + h / 2], axis=-1)
+
+
+class AgnosticNMS(keras.layers.Layer):
+ # TF Agnostic NMS
+ def call(self, input, topk_all, iou_thres, conf_thres):
+ # wrap map_fn to avoid TypeSpec related error https://stackoverflow.com/a/65809989/3036450
+ return tf.map_fn(lambda x: self._nms(x, topk_all, iou_thres, conf_thres),
+ input,
+ fn_output_signature=(tf.float32, tf.float32, tf.float32, tf.int32),
+ name='agnostic_nms')
+
+ @staticmethod
+ def _nms(x, topk_all=100, iou_thres=0.45, conf_thres=0.25): # agnostic NMS
+ boxes, classes, scores = x
+ class_inds = tf.cast(tf.argmax(classes, axis=-1), tf.float32)
+ scores_inp = tf.reduce_max(scores, -1)
+ selected_inds = tf.image.non_max_suppression(boxes,
+ scores_inp,
+ max_output_size=topk_all,
+ iou_threshold=iou_thres,
+ score_threshold=conf_thres)
+ selected_boxes = tf.gather(boxes, selected_inds)
+ padded_boxes = tf.pad(selected_boxes,
+ paddings=[[0, topk_all - tf.shape(selected_boxes)[0]], [0, 0]],
+ mode='CONSTANT',
+ constant_values=0.0)
+ selected_scores = tf.gather(scores_inp, selected_inds)
+ padded_scores = tf.pad(selected_scores,
+ paddings=[[0, topk_all - tf.shape(selected_boxes)[0]]],
+ mode='CONSTANT',
+ constant_values=-1.0)
+ selected_classes = tf.gather(class_inds, selected_inds)
+ padded_classes = tf.pad(selected_classes,
+ paddings=[[0, topk_all - tf.shape(selected_boxes)[0]]],
+ mode='CONSTANT',
+ constant_values=-1.0)
+ valid_detections = tf.shape(selected_inds)[0]
+ return padded_boxes, padded_scores, padded_classes, valid_detections
+
+
+def activations(act=nn.SiLU):
+ # Returns TF activation from input PyTorch activation
+ if isinstance(act, nn.LeakyReLU):
+ return lambda x: keras.activations.relu(x, alpha=0.1)
+ elif isinstance(act, nn.Hardswish):
+ return lambda x: x * tf.nn.relu6(x + 3) * 0.166666667
+ elif isinstance(act, (nn.SiLU, SiLU)):
+ return lambda x: keras.activations.swish(x)
+ else:
+ raise Exception(f'no matching TensorFlow activation found for PyTorch activation {act}')
+
+
+def representative_dataset_gen(dataset, ncalib=100):
+ # Representative dataset generator for use with converter.representative_dataset, returns a generator of np arrays
+ for n, (path, img, im0s, vid_cap, string) in enumerate(dataset):
+ im = np.transpose(img, [1, 2, 0])
+ im = np.expand_dims(im, axis=0).astype(np.float32)
+ im /= 255
+ yield [im]
+ if n >= ncalib:
+ break
+
+
+def run(
+ weights=ROOT / 'yolov5s.pt', # weights path
+ imgsz=(640, 640), # inference size h,w
+ batch_size=1, # batch size
+ dynamic=False, # dynamic batch size
+):
+ # PyTorch model
+ im = torch.zeros((batch_size, 3, *imgsz)) # BCHW image
+ model = attempt_load(weights, device=torch.device('cpu'), inplace=True, fuse=False)
+ _ = model(im) # inference
+ model.info()
+
+ # TensorFlow model
+ im = tf.zeros((batch_size, *imgsz, 3)) # BHWC image
+ tf_model = TFModel(cfg=model.yaml, model=model, nc=model.nc, imgsz=imgsz)
+ _ = tf_model.predict(im) # inference
+
+ # Keras model
+ im = keras.Input(shape=(*imgsz, 3), batch_size=None if dynamic else batch_size)
+ keras_model = keras.Model(inputs=im, outputs=tf_model.predict(im))
+ keras_model.summary()
+
+ LOGGER.info('PyTorch, TensorFlow and Keras models successfully verified.\nUse export.py for TF model export.')
+
+
+def parse_opt():
+ parser = argparse.ArgumentParser()
+ parser.add_argument('--weights', type=str, default=ROOT / 'yolov5s.pt', help='weights path')
+ parser.add_argument('--imgsz', '--img', '--img-size', nargs='+', type=int, default=[640], help='inference size h,w')
+ parser.add_argument('--batch-size', type=int, default=1, help='batch size')
+ parser.add_argument('--dynamic', action='store_true', help='dynamic batch size')
+ opt = parser.parse_args()
+ opt.imgsz *= 2 if len(opt.imgsz) == 1 else 1 # expand
+ print_args(vars(opt))
+ return opt
+
+
+def main(opt):
+ run(**vars(opt))
+
+
+if __name__ == '__main__':
+ opt = parse_opt()
+ main(opt)
diff --git a/yolov5/models/yolo.py b/yolov5/models/yolo.py
new file mode 100644
index 0000000000000000000000000000000000000000..cf4ac8c8da0ad90df26413852c519b031559f8c6
--- /dev/null
+++ b/yolov5/models/yolo.py
@@ -0,0 +1,391 @@
+# YOLOv5 🚀 by Ultralytics, AGPL-3.0 license
+"""
+YOLO-specific modules
+
+Usage:
+ $ python models/yolo.py --cfg yolov5s.yaml
+"""
+
+import argparse
+import contextlib
+import os
+import platform
+import sys
+from copy import deepcopy
+from pathlib import Path
+
+FILE = Path(__file__).resolve()
+ROOT = FILE.parents[1] # YOLOv5 root directory
+if str(ROOT) not in sys.path:
+ sys.path.append(str(ROOT)) # add ROOT to PATH
+if platform.system() != 'Windows':
+ ROOT = Path(os.path.relpath(ROOT, Path.cwd())) # relative
+
+from yolov5.models.common import *
+from yolov5.models.experimental import *
+from yolov5.utils.autoanchor import check_anchor_order
+from yolov5.utils.general import LOGGER, check_version, check_yaml, make_divisible, print_args
+from yolov5.utils.plots import feature_visualization
+from yolov5.utils.torch_utils import (fuse_conv_and_bn, initialize_weights, model_info, profile, scale_img, select_device,
+ time_sync)
+
+try:
+ import thop # for FLOPs computation
+except ImportError:
+ thop = None
+
+
+class Detect(nn.Module):
+ # YOLOv5 Detect head for detection models
+ stride = None # strides computed during build
+ dynamic = False # force grid reconstruction
+ export = False # export mode
+
+ def __init__(self, nc=80, anchors=(), ch=(), inplace=True): # detection layer
+ super().__init__()
+ self.nc = nc # number of classes
+ self.no = nc + 5 # number of outputs per anchor
+ self.nl = len(anchors) # number of detection layers
+ self.na = len(anchors[0]) // 2 # number of anchors
+ self.grid = [torch.empty(0) for _ in range(self.nl)] # init grid
+ self.anchor_grid = [torch.empty(0) for _ in range(self.nl)] # init anchor grid
+ self.register_buffer('anchors', torch.tensor(anchors).float().view(self.nl, -1, 2)) # shape(nl,na,2)
+ self.m = nn.ModuleList(nn.Conv2d(x, self.no * self.na, 1) for x in ch) # output conv
+ self.inplace = inplace # use inplace ops (e.g. slice assignment)
+
+ def forward(self, x):
+ z = [] # inference output
+ for i in range(self.nl):
+ x[i] = self.m[i](x[i]) # conv
+ bs, _, ny, nx = x[i].shape # x(bs,255,20,20) to x(bs,3,20,20,85)
+ x[i] = x[i].view(bs, self.na, self.no, ny, nx).permute(0, 1, 3, 4, 2).contiguous()
+
+ if not self.training: # inference
+ if self.dynamic or self.grid[i].shape[2:4] != x[i].shape[2:4]:
+ self.grid[i], self.anchor_grid[i] = self._make_grid(nx, ny, i)
+
+ if isinstance(self, Segment): # (boxes + masks)
+ xy, wh, conf, mask = x[i].split((2, 2, self.nc + 1, self.no - self.nc - 5), 4)
+ xy = (xy.sigmoid() * 2 + self.grid[i]) * self.stride[i] # xy
+ wh = (wh.sigmoid() * 2) ** 2 * self.anchor_grid[i] # wh
+ y = torch.cat((xy, wh, conf.sigmoid(), mask), 4)
+ else: # Detect (boxes only)
+ xy, wh, conf = x[i].sigmoid().split((2, 2, self.nc + 1), 4)
+ xy = (xy * 2 + self.grid[i]) * self.stride[i] # xy
+ wh = (wh * 2) ** 2 * self.anchor_grid[i] # wh
+ y = torch.cat((xy, wh, conf), 4)
+ z.append(y.view(bs, self.na * nx * ny, self.no))
+
+ return x if self.training else (torch.cat(z, 1),) if self.export else (torch.cat(z, 1), x)
+
+ def _make_grid(self, nx=20, ny=20, i=0, torch_1_10=check_version(torch.__version__, '1.10.0')):
+ d = self.anchors[i].device
+ t = self.anchors[i].dtype
+ shape = 1, self.na, ny, nx, 2 # grid shape
+ y, x = torch.arange(ny, device=d, dtype=t), torch.arange(nx, device=d, dtype=t)
+ yv, xv = torch.meshgrid(y, x, indexing='ij') if torch_1_10 else torch.meshgrid(y, x) # torch>=0.7 compatibility
+ grid = torch.stack((xv, yv), 2).expand(shape) - 0.5 # add grid offset, i.e. y = 2.0 * x - 0.5
+ anchor_grid = (self.anchors[i] * self.stride[i]).view((1, self.na, 1, 1, 2)).expand(shape)
+ return grid, anchor_grid
+
+
+class Segment(Detect):
+ # YOLOv5 Segment head for segmentation models
+ def __init__(self, nc=80, anchors=(), nm=32, npr=256, ch=(), inplace=True):
+ super().__init__(nc, anchors, ch, inplace)
+ self.nm = nm # number of masks
+ self.npr = npr # number of protos
+ self.no = 5 + nc + self.nm # number of outputs per anchor
+ self.m = nn.ModuleList(nn.Conv2d(x, self.no * self.na, 1) for x in ch) # output conv
+ self.proto = Proto(ch[0], self.npr, self.nm) # protos
+ self.detect = Detect.forward
+
+ def forward(self, x):
+ p = self.proto(x[0])
+ x = self.detect(self, x)
+ return (x, p) if self.training else (x[0], p) if self.export else (x[0], p, x[1])
+
+
+class BaseModel(nn.Module):
+ # YOLOv5 base model
+ def forward(self, x, profile=False, visualize=False):
+ return self._forward_once(x, profile, visualize) # single-scale inference, train
+
+ def _forward_once(self, x, profile=False, visualize=False):
+ y, dt = [], [] # outputs
+ for m in self.model:
+ if m.f != -1: # if not from previous layer
+ x = y[m.f] if isinstance(m.f, int) else [x if j == -1 else y[j] for j in m.f] # from earlier layers
+ if profile:
+ self._profile_one_layer(m, x, dt)
+ x = m(x) # run
+ y.append(x if m.i in self.save else None) # save output
+ if visualize:
+ feature_visualization(x, m.type, m.i, save_dir=visualize)
+ return x
+
+ def _profile_one_layer(self, m, x, dt):
+ c = m == self.model[-1] # is final layer, copy input as inplace fix
+ o = thop.profile(m, inputs=(x.copy() if c else x,), verbose=False)[0] / 1E9 * 2 if thop else 0 # FLOPs
+ t = time_sync()
+ for _ in range(10):
+ m(x.copy() if c else x)
+ dt.append((time_sync() - t) * 100)
+ if m == self.model[0]:
+ LOGGER.info(f"{'time (ms)':>10s} {'GFLOPs':>10s} {'params':>10s} module")
+ LOGGER.info(f'{dt[-1]:10.2f} {o:10.2f} {m.np:10.0f} {m.type}')
+ if c:
+ LOGGER.info(f"{sum(dt):10.2f} {'-':>10s} {'-':>10s} Total")
+
+ def fuse(self): # fuse model Conv2d() + BatchNorm2d() layers
+ LOGGER.info('Fusing layers... ')
+ for m in self.model.modules():
+ if isinstance(m, (Conv, DWConv)) and hasattr(m, 'bn'):
+ m.conv = fuse_conv_and_bn(m.conv, m.bn) # update conv
+ delattr(m, 'bn') # remove batchnorm
+ m.forward = m.forward_fuse # update forward
+ self.info()
+ return self
+
+ def info(self, verbose=False, img_size=640): # print model information
+ model_info(self, verbose, img_size)
+
+ def _apply(self, fn):
+ # Apply to(), cpu(), cuda(), half() to model tensors that are not parameters or registered buffers
+ self = super()._apply(fn)
+ m = self.model[-1] # Detect()
+ if isinstance(m, (Detect, Segment)):
+ m.stride = fn(m.stride)
+ m.grid = list(map(fn, m.grid))
+ if isinstance(m.anchor_grid, list):
+ m.anchor_grid = list(map(fn, m.anchor_grid))
+ return self
+
+
+class DetectionModel(BaseModel):
+ # YOLOv5 detection model
+ def __init__(self, cfg='yolov5s.yaml', ch=3, nc=None, anchors=None): # model, input channels, number of classes
+ super().__init__()
+ if isinstance(cfg, dict):
+ self.yaml = cfg # model dict
+ else: # is *.yaml
+ import yaml # for torch hub
+ self.yaml_file = Path(cfg).name
+ with open(cfg, encoding='ascii', errors='ignore') as f:
+ self.yaml = yaml.safe_load(f) # model dict
+
+ # Define model
+ ch = self.yaml['ch'] = self.yaml.get('ch', ch) # input channels
+ if nc and nc != self.yaml['nc']:
+ LOGGER.info(f"Overriding model.yaml nc={self.yaml['nc']} with nc={nc}")
+ self.yaml['nc'] = nc # override yaml value
+ if anchors:
+ LOGGER.info(f'Overriding model.yaml anchors with anchors={anchors}')
+ self.yaml['anchors'] = round(anchors) # override yaml value
+ self.model, self.save = parse_model(deepcopy(self.yaml), ch=[ch]) # model, savelist
+ self.names = [str(i) for i in range(self.yaml['nc'])] # default names
+ self.inplace = self.yaml.get('inplace', True)
+
+ # Build strides, anchors
+ m = self.model[-1] # Detect()
+ if isinstance(m, (Detect, Segment)):
+ s = 256 # 2x min stride
+ m.inplace = self.inplace
+ forward = lambda x: self.forward(x)[0] if isinstance(m, Segment) else self.forward(x)
+ m.stride = torch.tensor([s / x.shape[-2] for x in forward(torch.zeros(1, ch, s, s))]) # forward
+ check_anchor_order(m)
+ m.anchors /= m.stride.view(-1, 1, 1)
+ self.stride = m.stride
+ self._initialize_biases() # only run once
+
+ # Init weights, biases
+ initialize_weights(self)
+ self.info()
+ LOGGER.info('')
+
+ def forward(self, x, augment=False, profile=False, visualize=False):
+ if augment:
+ return self._forward_augment(x) # augmented inference, None
+ return self._forward_once(x, profile, visualize) # single-scale inference, train
+
+ def _forward_augment(self, x):
+ img_size = x.shape[-2:] # height, width
+ s = [1, 0.83, 0.67] # scales
+ f = [None, 3, None] # flips (2-ud, 3-lr)
+ y = [] # outputs
+ for si, fi in zip(s, f):
+ xi = scale_img(x.flip(fi) if fi else x, si, gs=int(self.stride.max()))
+ yi = self._forward_once(xi)[0] # forward
+ # cv2.imwrite(f'img_{si}.jpg', 255 * xi[0].cpu().numpy().transpose((1, 2, 0))[:, :, ::-1]) # save
+ yi = self._descale_pred(yi, fi, si, img_size)
+ y.append(yi)
+ y = self._clip_augmented(y) # clip augmented tails
+ return torch.cat(y, 1), None # augmented inference, train
+
+ def _descale_pred(self, p, flips, scale, img_size):
+ # de-scale predictions following augmented inference (inverse operation)
+ if self.inplace:
+ p[..., :4] /= scale # de-scale
+ if flips == 2:
+ p[..., 1] = img_size[0] - p[..., 1] # de-flip ud
+ elif flips == 3:
+ p[..., 0] = img_size[1] - p[..., 0] # de-flip lr
+ else:
+ x, y, wh = p[..., 0:1] / scale, p[..., 1:2] / scale, p[..., 2:4] / scale # de-scale
+ if flips == 2:
+ y = img_size[0] - y # de-flip ud
+ elif flips == 3:
+ x = img_size[1] - x # de-flip lr
+ p = torch.cat((x, y, wh, p[..., 4:]), -1)
+ return p
+
+ def _clip_augmented(self, y):
+ # Clip YOLOv5 augmented inference tails
+ nl = self.model[-1].nl # number of detection layers (P3-P5)
+ g = sum(4 ** x for x in range(nl)) # grid points
+ e = 1 # exclude layer count
+ i = (y[0].shape[1] // g) * sum(4 ** x for x in range(e)) # indices
+ y[0] = y[0][:, :-i] # large
+ i = (y[-1].shape[1] // g) * sum(4 ** (nl - 1 - x) for x in range(e)) # indices
+ y[-1] = y[-1][:, i:] # small
+ return y
+
+ def _initialize_biases(self, cf=None): # initialize biases into Detect(), cf is class frequency
+ # https://arxiv.org/abs/1708.02002 section 3.3
+ # cf = torch.bincount(torch.tensor(np.concatenate(dataset.labels, 0)[:, 0]).long(), minlength=nc) + 1.
+ m = self.model[-1] # Detect() module
+ for mi, s in zip(m.m, m.stride): # from
+ b = mi.bias.view(m.na, -1) # conv.bias(255) to (3,85)
+ b.data[:, 4] += math.log(8 / (640 / s) ** 2) # obj (8 objects per 640 image)
+ b.data[:, 5:5 + m.nc] += math.log(0.6 / (m.nc - 0.99999)) if cf is None else torch.log(cf / cf.sum()) # cls
+ mi.bias = torch.nn.Parameter(b.view(-1), requires_grad=True)
+
+
+Model = DetectionModel # retain YOLOv5 'Model' class for backwards compatibility
+
+
+class SegmentationModel(DetectionModel):
+ # YOLOv5 segmentation model
+ def __init__(self, cfg='yolov5s-seg.yaml', ch=3, nc=None, anchors=None):
+ super().__init__(cfg, ch, nc, anchors)
+
+
+class ClassificationModel(BaseModel):
+ # YOLOv5 classification model
+ def __init__(self, cfg=None, model=None, nc=1000, cutoff=10): # yaml, model, number of classes, cutoff index
+ super().__init__()
+ self._from_detection_model(model, nc, cutoff) if model is not None else self._from_yaml(cfg)
+
+ def _from_detection_model(self, model, nc=1000, cutoff=10):
+ # Create a YOLOv5 classification model from a YOLOv5 detection model
+ if isinstance(model, DetectMultiBackend):
+ model = model.model # unwrap DetectMultiBackend
+ model.model = model.model[:cutoff] # backbone
+ m = model.model[-1] # last layer
+ ch = m.conv.in_channels if hasattr(m, 'conv') else m.cv1.conv.in_channels # ch into module
+ c = Classify(ch, nc) # Classify()
+ c.i, c.f, c.type = m.i, m.f, 'models.common.Classify' # index, from, type
+ model.model[-1] = c # replace
+ self.model = model.model
+ self.stride = model.stride
+ self.save = []
+ self.nc = nc
+
+ def _from_yaml(self, cfg):
+ # Create a YOLOv5 classification model from a *.yaml file
+ self.model = None
+
+
+def parse_model(d, ch): # model_dict, input_channels(3)
+ # Parse a YOLOv5 model.yaml dictionary
+ LOGGER.info(f"\n{'':>3}{'from':>18}{'n':>3}{'params':>10} {'module':<40}{'arguments':<30}")
+ anchors, nc, gd, gw, act = d['anchors'], d['nc'], d['depth_multiple'], d['width_multiple'], d.get('activation')
+ if act:
+ Conv.default_act = eval(act) # redefine default activation, i.e. Conv.default_act = nn.SiLU()
+ LOGGER.info(f"{colorstr('activation:')} {act}") # print
+ na = (len(anchors[0]) // 2) if isinstance(anchors, list) else anchors # number of anchors
+ no = na * (nc + 5) # number of outputs = anchors * (classes + 5)
+
+ layers, save, c2 = [], [], ch[-1] # layers, savelist, ch out
+ for i, (f, n, m, args) in enumerate(d['backbone'] + d['head']): # from, number, module, args
+ m = eval(m) if isinstance(m, str) else m # eval strings
+ for j, a in enumerate(args):
+ with contextlib.suppress(NameError):
+ args[j] = eval(a) if isinstance(a, str) else a # eval strings
+
+ n = n_ = max(round(n * gd), 1) if n > 1 else n # depth gain
+ if m in {
+ Conv, GhostConv, Bottleneck, GhostBottleneck, SPP, SPPF, DWConv, MixConv2d, Focus, CrossConv,
+ BottleneckCSP, C3, C3TR, C3SPP, C3Ghost, nn.ConvTranspose2d, DWConvTranspose2d, C3x}:
+ c1, c2 = ch[f], args[0]
+ if c2 != no: # if not output
+ c2 = make_divisible(c2 * gw, 8)
+
+ args = [c1, c2, *args[1:]]
+ if m in {BottleneckCSP, C3, C3TR, C3Ghost, C3x}:
+ args.insert(2, n) # number of repeats
+ n = 1
+ elif m is nn.BatchNorm2d:
+ args = [ch[f]]
+ elif m is Concat:
+ c2 = sum(ch[x] for x in f)
+ # TODO: channel, gw, gd
+ elif m in {Detect, Segment}:
+ args.append([ch[x] for x in f])
+ if isinstance(args[1], int): # number of anchors
+ args[1] = [list(range(args[1] * 2))] * len(f)
+ if m is Segment:
+ args[3] = make_divisible(args[3] * gw, 8)
+ elif m is Contract:
+ c2 = ch[f] * args[0] ** 2
+ elif m is Expand:
+ c2 = ch[f] // args[0] ** 2
+ else:
+ c2 = ch[f]
+
+ m_ = nn.Sequential(*(m(*args) for _ in range(n))) if n > 1 else m(*args) # module
+ t = str(m)[8:-2].replace('__main__.', '') # module type
+ np = sum(x.numel() for x in m_.parameters()) # number params
+ m_.i, m_.f, m_.type, m_.np = i, f, t, np # attach index, 'from' index, type, number params
+ LOGGER.info(f'{i:>3}{str(f):>18}{n_:>3}{np:10.0f} {t:<40}{str(args):<30}') # print
+ save.extend(x % i for x in ([f] if isinstance(f, int) else f) if x != -1) # append to savelist
+ layers.append(m_)
+ if i == 0:
+ ch = []
+ ch.append(c2)
+ return nn.Sequential(*layers), sorted(save)
+
+
+if __name__ == '__main__':
+ parser = argparse.ArgumentParser()
+ parser.add_argument('--cfg', type=str, default='yolov5s.yaml', help='model.yaml')
+ parser.add_argument('--batch-size', type=int, default=1, help='total batch size for all GPUs')
+ parser.add_argument('--device', default='', help='cuda device, i.e. 0 or 0,1,2,3 or cpu')
+ parser.add_argument('--profile', action='store_true', help='profile model speed')
+ parser.add_argument('--line-profile', action='store_true', help='profile model speed layer by layer')
+ parser.add_argument('--test', action='store_true', help='test all yolo*.yaml')
+ opt = parser.parse_args()
+ opt.cfg = check_yaml(opt.cfg) # check YAML
+ print_args(vars(opt))
+ device = select_device(opt.device)
+
+ # Create model
+ im = torch.rand(opt.batch_size, 3, 640, 640).to(device)
+ model = Model(opt.cfg).to(device)
+
+ # Options
+ if opt.line_profile: # profile layer by layer
+ model(im, profile=True)
+
+ elif opt.profile: # profile forward-backward
+ results = profile(input=im, ops=[model], n=3)
+
+ elif opt.test: # test all models
+ for cfg in Path(ROOT / 'models').rglob('yolo*.yaml'):
+ try:
+ _ = Model(cfg)
+ except Exception as e:
+ print(f'Error in {cfg}: {e}')
+
+ else: # report fused model summary
+ model.fuse()
diff --git a/yolov5/models/yolov5l.yaml b/yolov5/models/yolov5l.yaml
new file mode 100644
index 0000000000000000000000000000000000000000..31362f8769327bad3afdb65d39a3b940397ecfae
--- /dev/null
+++ b/yolov5/models/yolov5l.yaml
@@ -0,0 +1,48 @@
+# YOLOv5 🚀 by Ultralytics, AGPL-3.0 license
+
+# Parameters
+nc: 80 # number of classes
+depth_multiple: 1.0 # model depth multiple
+width_multiple: 1.0 # layer channel multiple
+anchors:
+ - [10,13, 16,30, 33,23] # P3/8
+ - [30,61, 62,45, 59,119] # P4/16
+ - [116,90, 156,198, 373,326] # P5/32
+
+# YOLOv5 v6.0 backbone
+backbone:
+ # [from, number, module, args]
+ [[-1, 1, Conv, [64, 6, 2, 2]], # 0-P1/2
+ [-1, 1, Conv, [128, 3, 2]], # 1-P2/4
+ [-1, 3, C3, [128]],
+ [-1, 1, Conv, [256, 3, 2]], # 3-P3/8
+ [-1, 6, C3, [256]],
+ [-1, 1, Conv, [512, 3, 2]], # 5-P4/16
+ [-1, 9, C3, [512]],
+ [-1, 1, Conv, [1024, 3, 2]], # 7-P5/32
+ [-1, 3, C3, [1024]],
+ [-1, 1, SPPF, [1024, 5]], # 9
+ ]
+
+# YOLOv5 v6.0 head
+head:
+ [[-1, 1, Conv, [512, 1, 1]],
+ [-1, 1, nn.Upsample, [None, 2, 'nearest']],
+ [[-1, 6], 1, Concat, [1]], # cat backbone P4
+ [-1, 3, C3, [512, False]], # 13
+
+ [-1, 1, Conv, [256, 1, 1]],
+ [-1, 1, nn.Upsample, [None, 2, 'nearest']],
+ [[-1, 4], 1, Concat, [1]], # cat backbone P3
+ [-1, 3, C3, [256, False]], # 17 (P3/8-small)
+
+ [-1, 1, Conv, [256, 3, 2]],
+ [[-1, 14], 1, Concat, [1]], # cat head P4
+ [-1, 3, C3, [512, False]], # 20 (P4/16-medium)
+
+ [-1, 1, Conv, [512, 3, 2]],
+ [[-1, 10], 1, Concat, [1]], # cat head P5
+ [-1, 3, C3, [1024, False]], # 23 (P5/32-large)
+
+ [[17, 20, 23], 1, Detect, [nc, anchors]], # Detect(P3, P4, P5)
+ ]
diff --git a/yolov5/models/yolov5m.yaml b/yolov5/models/yolov5m.yaml
new file mode 100644
index 0000000000000000000000000000000000000000..a76900c5a2e2602d59ece8645bbd67e0dc454311
--- /dev/null
+++ b/yolov5/models/yolov5m.yaml
@@ -0,0 +1,48 @@
+# YOLOv5 🚀 by Ultralytics, AGPL-3.0 license
+
+# Parameters
+nc: 80 # number of classes
+depth_multiple: 0.67 # model depth multiple
+width_multiple: 0.75 # layer channel multiple
+anchors:
+ - [10,13, 16,30, 33,23] # P3/8
+ - [30,61, 62,45, 59,119] # P4/16
+ - [116,90, 156,198, 373,326] # P5/32
+
+# YOLOv5 v6.0 backbone
+backbone:
+ # [from, number, module, args]
+ [[-1, 1, Conv, [64, 6, 2, 2]], # 0-P1/2
+ [-1, 1, Conv, [128, 3, 2]], # 1-P2/4
+ [-1, 3, C3, [128]],
+ [-1, 1, Conv, [256, 3, 2]], # 3-P3/8
+ [-1, 6, C3, [256]],
+ [-1, 1, Conv, [512, 3, 2]], # 5-P4/16
+ [-1, 9, C3, [512]],
+ [-1, 1, Conv, [1024, 3, 2]], # 7-P5/32
+ [-1, 3, C3, [1024]],
+ [-1, 1, SPPF, [1024, 5]], # 9
+ ]
+
+# YOLOv5 v6.0 head
+head:
+ [[-1, 1, Conv, [512, 1, 1]],
+ [-1, 1, nn.Upsample, [None, 2, 'nearest']],
+ [[-1, 6], 1, Concat, [1]], # cat backbone P4
+ [-1, 3, C3, [512, False]], # 13
+
+ [-1, 1, Conv, [256, 1, 1]],
+ [-1, 1, nn.Upsample, [None, 2, 'nearest']],
+ [[-1, 4], 1, Concat, [1]], # cat backbone P3
+ [-1, 3, C3, [256, False]], # 17 (P3/8-small)
+
+ [-1, 1, Conv, [256, 3, 2]],
+ [[-1, 14], 1, Concat, [1]], # cat head P4
+ [-1, 3, C3, [512, False]], # 20 (P4/16-medium)
+
+ [-1, 1, Conv, [512, 3, 2]],
+ [[-1, 10], 1, Concat, [1]], # cat head P5
+ [-1, 3, C3, [1024, False]], # 23 (P5/32-large)
+
+ [[17, 20, 23], 1, Detect, [nc, anchors]], # Detect(P3, P4, P5)
+ ]
diff --git a/yolov5/models/yolov5n.yaml b/yolov5/models/yolov5n.yaml
new file mode 100644
index 0000000000000000000000000000000000000000..aba96cfc54f48c2cb2fe16aae2b0b94e38826c9d
--- /dev/null
+++ b/yolov5/models/yolov5n.yaml
@@ -0,0 +1,48 @@
+# YOLOv5 🚀 by Ultralytics, AGPL-3.0 license
+
+# Parameters
+nc: 80 # number of classes
+depth_multiple: 0.33 # model depth multiple
+width_multiple: 0.25 # layer channel multiple
+anchors:
+ - [10,13, 16,30, 33,23] # P3/8
+ - [30,61, 62,45, 59,119] # P4/16
+ - [116,90, 156,198, 373,326] # P5/32
+
+# YOLOv5 v6.0 backbone
+backbone:
+ # [from, number, module, args]
+ [[-1, 1, Conv, [64, 6, 2, 2]], # 0-P1/2
+ [-1, 1, Conv, [128, 3, 2]], # 1-P2/4
+ [-1, 3, C3, [128]],
+ [-1, 1, Conv, [256, 3, 2]], # 3-P3/8
+ [-1, 6, C3, [256]],
+ [-1, 1, Conv, [512, 3, 2]], # 5-P4/16
+ [-1, 9, C3, [512]],
+ [-1, 1, Conv, [1024, 3, 2]], # 7-P5/32
+ [-1, 3, C3, [1024]],
+ [-1, 1, SPPF, [1024, 5]], # 9
+ ]
+
+# YOLOv5 v6.0 head
+head:
+ [[-1, 1, Conv, [512, 1, 1]],
+ [-1, 1, nn.Upsample, [None, 2, 'nearest']],
+ [[-1, 6], 1, Concat, [1]], # cat backbone P4
+ [-1, 3, C3, [512, False]], # 13
+
+ [-1, 1, Conv, [256, 1, 1]],
+ [-1, 1, nn.Upsample, [None, 2, 'nearest']],
+ [[-1, 4], 1, Concat, [1]], # cat backbone P3
+ [-1, 3, C3, [256, False]], # 17 (P3/8-small)
+
+ [-1, 1, Conv, [256, 3, 2]],
+ [[-1, 14], 1, Concat, [1]], # cat head P4
+ [-1, 3, C3, [512, False]], # 20 (P4/16-medium)
+
+ [-1, 1, Conv, [512, 3, 2]],
+ [[-1, 10], 1, Concat, [1]], # cat head P5
+ [-1, 3, C3, [1024, False]], # 23 (P5/32-large)
+
+ [[17, 20, 23], 1, Detect, [nc, anchors]], # Detect(P3, P4, P5)
+ ]
diff --git a/yolov5/models/yolov5s.yaml b/yolov5/models/yolov5s.yaml
new file mode 100644
index 0000000000000000000000000000000000000000..5d05364c49363adb2b863ecf163e45516679dc03
--- /dev/null
+++ b/yolov5/models/yolov5s.yaml
@@ -0,0 +1,48 @@
+# YOLOv5 🚀 by Ultralytics, AGPL-3.0 license
+
+# Parameters
+nc: 80 # number of classes
+depth_multiple: 0.33 # model depth multiple
+width_multiple: 0.50 # layer channel multiple
+anchors:
+ - [10,13, 16,30, 33,23] # P3/8
+ - [30,61, 62,45, 59,119] # P4/16
+ - [116,90, 156,198, 373,326] # P5/32
+
+# YOLOv5 v6.0 backbone
+backbone:
+ # [from, number, module, args]
+ [[-1, 1, Conv, [64, 6, 2, 2]], # 0-P1/2
+ [-1, 1, Conv, [128, 3, 2]], # 1-P2/4
+ [-1, 3, C3, [128]],
+ [-1, 1, Conv, [256, 3, 2]], # 3-P3/8
+ [-1, 6, C3, [256]],
+ [-1, 1, Conv, [512, 3, 2]], # 5-P4/16
+ [-1, 9, C3, [512]],
+ [-1, 1, Conv, [1024, 3, 2]], # 7-P5/32
+ [-1, 3, C3, [1024]],
+ [-1, 1, SPPF, [1024, 5]], # 9
+ ]
+
+# YOLOv5 v6.0 head
+head:
+ [[-1, 1, Conv, [512, 1, 1]],
+ [-1, 1, nn.Upsample, [None, 2, 'nearest']],
+ [[-1, 6], 1, Concat, [1]], # cat backbone P4
+ [-1, 3, C3, [512, False]], # 13
+
+ [-1, 1, Conv, [256, 1, 1]],
+ [-1, 1, nn.Upsample, [None, 2, 'nearest']],
+ [[-1, 4], 1, Concat, [1]], # cat backbone P3
+ [-1, 3, C3, [256, False]], # 17 (P3/8-small)
+
+ [-1, 1, Conv, [256, 3, 2]],
+ [[-1, 14], 1, Concat, [1]], # cat head P4
+ [-1, 3, C3, [512, False]], # 20 (P4/16-medium)
+
+ [-1, 1, Conv, [512, 3, 2]],
+ [[-1, 10], 1, Concat, [1]], # cat head P5
+ [-1, 3, C3, [1024, False]], # 23 (P5/32-large)
+
+ [[17, 20, 23], 1, Detect, [nc, anchors]], # Detect(P3, P4, P5)
+ ]
diff --git a/yolov5/models/yolov5x.yaml b/yolov5/models/yolov5x.yaml
new file mode 100644
index 0000000000000000000000000000000000000000..4bdd93915da550d62c4ada3684c5660ff5034404
--- /dev/null
+++ b/yolov5/models/yolov5x.yaml
@@ -0,0 +1,48 @@
+# YOLOv5 🚀 by Ultralytics, AGPL-3.0 license
+
+# Parameters
+nc: 80 # number of classes
+depth_multiple: 1.33 # model depth multiple
+width_multiple: 1.25 # layer channel multiple
+anchors:
+ - [10,13, 16,30, 33,23] # P3/8
+ - [30,61, 62,45, 59,119] # P4/16
+ - [116,90, 156,198, 373,326] # P5/32
+
+# YOLOv5 v6.0 backbone
+backbone:
+ # [from, number, module, args]
+ [[-1, 1, Conv, [64, 6, 2, 2]], # 0-P1/2
+ [-1, 1, Conv, [128, 3, 2]], # 1-P2/4
+ [-1, 3, C3, [128]],
+ [-1, 1, Conv, [256, 3, 2]], # 3-P3/8
+ [-1, 6, C3, [256]],
+ [-1, 1, Conv, [512, 3, 2]], # 5-P4/16
+ [-1, 9, C3, [512]],
+ [-1, 1, Conv, [1024, 3, 2]], # 7-P5/32
+ [-1, 3, C3, [1024]],
+ [-1, 1, SPPF, [1024, 5]], # 9
+ ]
+
+# YOLOv5 v6.0 head
+head:
+ [[-1, 1, Conv, [512, 1, 1]],
+ [-1, 1, nn.Upsample, [None, 2, 'nearest']],
+ [[-1, 6], 1, Concat, [1]], # cat backbone P4
+ [-1, 3, C3, [512, False]], # 13
+
+ [-1, 1, Conv, [256, 1, 1]],
+ [-1, 1, nn.Upsample, [None, 2, 'nearest']],
+ [[-1, 4], 1, Concat, [1]], # cat backbone P3
+ [-1, 3, C3, [256, False]], # 17 (P3/8-small)
+
+ [-1, 1, Conv, [256, 3, 2]],
+ [[-1, 14], 1, Concat, [1]], # cat head P4
+ [-1, 3, C3, [512, False]], # 20 (P4/16-medium)
+
+ [-1, 1, Conv, [512, 3, 2]],
+ [[-1, 10], 1, Concat, [1]], # cat head P5
+ [-1, 3, C3, [1024, False]], # 23 (P5/32-large)
+
+ [[17, 20, 23], 1, Detect, [nc, anchors]], # Detect(P3, P4, P5)
+ ]
diff --git a/yolov5/requirements.txt b/yolov5/requirements.txt
new file mode 100644
index 0000000000000000000000000000000000000000..65924c9feec41273a8a4d2bb238e5622ac928a96
--- /dev/null
+++ b/yolov5/requirements.txt
@@ -0,0 +1,49 @@
+# YOLOv5 requirements
+# Usage: pip install -r requirements.txt
+
+# Base ------------------------------------------------------------------------
+gitpython>=3.1.30
+matplotlib>=3.3
+numpy>=1.18.5
+opencv-python>=4.1.1
+Pillow>=7.1.2
+psutil # system resources
+PyYAML>=5.3.1
+requests>=2.23.0
+scipy>=1.4.1
+thop>=0.1.1 # FLOPs computation
+torch>=1.7.0 # see https://pytorch.org/get-started/locally (recommended)
+torchvision>=0.8.1
+tqdm>=4.64.0
+# protobuf<=3.20.1 # https://github.com/ultralytics/yolov5/issues/8012
+
+# Logging ---------------------------------------------------------------------
+# tensorboard>=2.4.1
+# clearml>=1.2.0
+# comet
+
+# Plotting --------------------------------------------------------------------
+pandas>=1.1.4
+seaborn>=0.11.0
+
+# Export ----------------------------------------------------------------------
+# coremltools>=6.0 # CoreML export
+# onnx>=1.10.0 # ONNX export
+# onnx-simplifier>=0.4.1 # ONNX simplifier
+# nvidia-pyindex # TensorRT export
+# nvidia-tensorrt # TensorRT export
+# scikit-learn<=1.1.2 # CoreML quantization
+# tensorflow>=2.4.0 # TF exports (-cpu, -aarch64, -macos)
+# tensorflowjs>=3.9.0 # TF.js export
+# openvino-dev # OpenVINO export
+
+# Deploy ----------------------------------------------------------------------
+setuptools>=65.5.1 # Snyk vulnerability fix
+# tritonclient[all]~=2.24.0
+
+# Extras ----------------------------------------------------------------------
+# ipython # interactive notebook
+# mss # screenshots
+# albumentations>=1.0.3
+# pycocotools>=2.0.6 # COCO mAP
+# ultralytics # HUB https://hub.ultralytics.com
diff --git a/yolov5/segment/predict.py b/yolov5/segment/predict.py
new file mode 100644
index 0000000000000000000000000000000000000000..4d4d6036358a755e297cbc83e2579242712b128a
--- /dev/null
+++ b/yolov5/segment/predict.py
@@ -0,0 +1,284 @@
+# YOLOv5 🚀 by Ultralytics, AGPL-3.0 license
+"""
+Run YOLOv5 segmentation inference on images, videos, directories, streams, etc.
+
+Usage - sources:
+ $ python segment/predict.py --weights yolov5s-seg.pt --source 0 # webcam
+ img.jpg # image
+ vid.mp4 # video
+ screen # screenshot
+ path/ # directory
+ list.txt # list of images
+ list.streams # list of streams
+ 'path/*.jpg' # glob
+ 'https://youtu.be/Zgi9g1ksQHc' # YouTube
+ 'rtsp://example.com/media.mp4' # RTSP, RTMP, HTTP stream
+
+Usage - formats:
+ $ python segment/predict.py --weights yolov5s-seg.pt # PyTorch
+ yolov5s-seg.torchscript # TorchScript
+ yolov5s-seg.onnx # ONNX Runtime or OpenCV DNN with --dnn
+ yolov5s-seg_openvino_model # OpenVINO
+ yolov5s-seg.engine # TensorRT
+ yolov5s-seg.mlmodel # CoreML (macOS-only)
+ yolov5s-seg_saved_model # TensorFlow SavedModel
+ yolov5s-seg.pb # TensorFlow GraphDef
+ yolov5s-seg.tflite # TensorFlow Lite
+ yolov5s-seg_edgetpu.tflite # TensorFlow Edge TPU
+ yolov5s-seg_paddle_model # PaddlePaddle
+"""
+
+import argparse
+import os
+import platform
+import sys
+from pathlib import Path
+
+import torch
+
+FILE = Path(__file__).resolve()
+ROOT = FILE.parents[1] # YOLOv5 root directory
+if str(ROOT) not in sys.path:
+ sys.path.append(str(ROOT)) # add ROOT to PATH
+ROOT = Path(os.path.relpath(ROOT, Path.cwd())) # relative
+
+from models.common import DetectMultiBackend
+from utils.dataloaders import IMG_FORMATS, VID_FORMATS, LoadImages, LoadScreenshots, LoadStreams
+from utils.general import (LOGGER, Profile, check_file, check_img_size, check_imshow, check_requirements, colorstr, cv2,
+ increment_path, non_max_suppression, print_args, scale_boxes, scale_segments,
+ strip_optimizer)
+from utils.plots import Annotator, colors, save_one_box
+from utils.segment.general import masks2segments, process_mask, process_mask_native
+from utils.torch_utils import select_device, smart_inference_mode
+
+
+@smart_inference_mode()
+def run(
+ weights=ROOT / 'yolov5s-seg.pt', # model.pt path(s)
+ source=ROOT / 'data/images', # file/dir/URL/glob/screen/0(webcam)
+ data=ROOT / 'data/coco128.yaml', # dataset.yaml path
+ imgsz=(640, 640), # inference size (height, width)
+ conf_thres=0.25, # confidence threshold
+ iou_thres=0.45, # NMS IOU threshold
+ max_det=1000, # maximum detections per image
+ device='', # cuda device, i.e. 0 or 0,1,2,3 or cpu
+ view_img=False, # show results
+ save_txt=False, # save results to *.txt
+ save_conf=False, # save confidences in --save-txt labels
+ save_crop=False, # save cropped prediction boxes
+ nosave=False, # do not save images/videos
+ classes=None, # filter by class: --class 0, or --class 0 2 3
+ agnostic_nms=False, # class-agnostic NMS
+ augment=False, # augmented inference
+ visualize=False, # visualize features
+ update=False, # update all models
+ project=ROOT / 'runs/predict-seg', # save results to project/name
+ name='exp', # save results to project/name
+ exist_ok=False, # existing project/name ok, do not increment
+ line_thickness=3, # bounding box thickness (pixels)
+ hide_labels=False, # hide labels
+ hide_conf=False, # hide confidences
+ half=False, # use FP16 half-precision inference
+ dnn=False, # use OpenCV DNN for ONNX inference
+ vid_stride=1, # video frame-rate stride
+ retina_masks=False,
+):
+ source = str(source)
+ save_img = not nosave and not source.endswith('.txt') # save inference images
+ is_file = Path(source).suffix[1:] in (IMG_FORMATS + VID_FORMATS)
+ is_url = source.lower().startswith(('rtsp://', 'rtmp://', 'http://', 'https://'))
+ webcam = source.isnumeric() or source.endswith('.streams') or (is_url and not is_file)
+ screenshot = source.lower().startswith('screen')
+ if is_url and is_file:
+ source = check_file(source) # download
+
+ # Directories
+ save_dir = increment_path(Path(project) / name, exist_ok=exist_ok) # increment run
+ (save_dir / 'labels' if save_txt else save_dir).mkdir(parents=True, exist_ok=True) # make dir
+
+ # Load model
+ device = select_device(device)
+ model = DetectMultiBackend(weights, device=device, dnn=dnn, data=data, fp16=half)
+ stride, names, pt = model.stride, model.names, model.pt
+ imgsz = check_img_size(imgsz, s=stride) # check image size
+
+ # Dataloader
+ bs = 1 # batch_size
+ if webcam:
+ view_img = check_imshow(warn=True)
+ dataset = LoadStreams(source, img_size=imgsz, stride=stride, auto=pt, vid_stride=vid_stride)
+ bs = len(dataset)
+ elif screenshot:
+ dataset = LoadScreenshots(source, img_size=imgsz, stride=stride, auto=pt)
+ else:
+ dataset = LoadImages(source, img_size=imgsz, stride=stride, auto=pt, vid_stride=vid_stride)
+ vid_path, vid_writer = [None] * bs, [None] * bs
+
+ # Run inference
+ model.warmup(imgsz=(1 if pt else bs, 3, *imgsz)) # warmup
+ seen, windows, dt = 0, [], (Profile(), Profile(), Profile())
+ for path, im, im0s, vid_cap, s in dataset:
+ with dt[0]:
+ im = torch.from_numpy(im).to(model.device)
+ im = im.half() if model.fp16 else im.float() # uint8 to fp16/32
+ im /= 255 # 0 - 255 to 0.0 - 1.0
+ if len(im.shape) == 3:
+ im = im[None] # expand for batch dim
+
+ # Inference
+ with dt[1]:
+ visualize = increment_path(save_dir / Path(path).stem, mkdir=True) if visualize else False
+ pred, proto = model(im, augment=augment, visualize=visualize)[:2]
+
+ # NMS
+ with dt[2]:
+ pred = non_max_suppression(pred, conf_thres, iou_thres, classes, agnostic_nms, max_det=max_det, nm=32)
+
+ # Second-stage classifier (optional)
+ # pred = utils.general.apply_classifier(pred, classifier_model, im, im0s)
+
+ # Process predictions
+ for i, det in enumerate(pred): # per image
+ seen += 1
+ if webcam: # batch_size >= 1
+ p, im0, frame = path[i], im0s[i].copy(), dataset.count
+ s += f'{i}: '
+ else:
+ p, im0, frame = path, im0s.copy(), getattr(dataset, 'frame', 0)
+
+ p = Path(p) # to Path
+ save_path = str(save_dir / p.name) # im.jpg
+ txt_path = str(save_dir / 'labels' / p.stem) + ('' if dataset.mode == 'image' else f'_{frame}') # im.txt
+ s += '%gx%g ' % im.shape[2:] # print string
+ imc = im0.copy() if save_crop else im0 # for save_crop
+ annotator = Annotator(im0, line_width=line_thickness, example=str(names))
+ if len(det):
+ if retina_masks:
+ # scale bbox first the crop masks
+ det[:, :4] = scale_boxes(im.shape[2:], det[:, :4], im0.shape).round() # rescale boxes to im0 size
+ masks = process_mask_native(proto[i], det[:, 6:], det[:, :4], im0.shape[:2]) # HWC
+ else:
+ masks = process_mask(proto[i], det[:, 6:], det[:, :4], im.shape[2:], upsample=True) # HWC
+ det[:, :4] = scale_boxes(im.shape[2:], det[:, :4], im0.shape).round() # rescale boxes to im0 size
+
+ # Segments
+ if save_txt:
+ segments = [
+ scale_segments(im0.shape if retina_masks else im.shape[2:], x, im0.shape, normalize=True)
+ for x in reversed(masks2segments(masks))]
+
+ # Print results
+ for c in det[:, 5].unique():
+ n = (det[:, 5] == c).sum() # detections per class
+ s += f"{n} {names[int(c)]}{'s' * (n > 1)}, " # add to string
+
+ # Mask plotting
+ annotator.masks(
+ masks,
+ colors=[colors(x, True) for x in det[:, 5]],
+ im_gpu=torch.as_tensor(im0, dtype=torch.float16).to(device).permute(2, 0, 1).flip(0).contiguous() /
+ 255 if retina_masks else im[i])
+
+ # Write results
+ for j, (*xyxy, conf, cls) in enumerate(reversed(det[:, :6])):
+ if save_txt: # Write to file
+ seg = segments[j].reshape(-1) # (n,2) to (n*2)
+ line = (cls, *seg, conf) if save_conf else (cls, *seg) # label format
+ with open(f'{txt_path}.txt', 'a') as f:
+ f.write(('%g ' * len(line)).rstrip() % line + '\n')
+
+ if save_img or save_crop or view_img: # Add bbox to image
+ c = int(cls) # integer class
+ label = None if hide_labels else (names[c] if hide_conf else f'{names[c]} {conf:.2f}')
+ annotator.box_label(xyxy, label, color=colors(c, True))
+ # annotator.draw.polygon(segments[j], outline=colors(c, True), width=3)
+ if save_crop:
+ save_one_box(xyxy, imc, file=save_dir / 'crops' / names[c] / f'{p.stem}.jpg', BGR=True)
+
+ # Stream results
+ im0 = annotator.result()
+ if view_img:
+ if platform.system() == 'Linux' and p not in windows:
+ windows.append(p)
+ cv2.namedWindow(str(p), cv2.WINDOW_NORMAL | cv2.WINDOW_KEEPRATIO) # allow window resize (Linux)
+ cv2.resizeWindow(str(p), im0.shape[1], im0.shape[0])
+ cv2.imshow(str(p), im0)
+ if cv2.waitKey(1) == ord('q'): # 1 millisecond
+ exit()
+
+ # Save results (image with detections)
+ if save_img:
+ if dataset.mode == 'image':
+ cv2.imwrite(save_path, im0)
+ else: # 'video' or 'stream'
+ if vid_path[i] != save_path: # new video
+ vid_path[i] = save_path
+ if isinstance(vid_writer[i], cv2.VideoWriter):
+ vid_writer[i].release() # release previous video writer
+ if vid_cap: # video
+ fps = vid_cap.get(cv2.CAP_PROP_FPS)
+ w = int(vid_cap.get(cv2.CAP_PROP_FRAME_WIDTH))
+ h = int(vid_cap.get(cv2.CAP_PROP_FRAME_HEIGHT))
+ else: # stream
+ fps, w, h = 30, im0.shape[1], im0.shape[0]
+ save_path = str(Path(save_path).with_suffix('.mp4')) # force *.mp4 suffix on results videos
+ vid_writer[i] = cv2.VideoWriter(save_path, cv2.VideoWriter_fourcc(*'mp4v'), fps, (w, h))
+ vid_writer[i].write(im0)
+
+ # Print time (inference-only)
+ LOGGER.info(f"{s}{'' if len(det) else '(no detections), '}{dt[1].dt * 1E3:.1f}ms")
+
+ # Print results
+ t = tuple(x.t / seen * 1E3 for x in dt) # speeds per image
+ LOGGER.info(f'Speed: %.1fms pre-process, %.1fms inference, %.1fms NMS per image at shape {(1, 3, *imgsz)}' % t)
+ if save_txt or save_img:
+ s = f"\n{len(list(save_dir.glob('labels/*.txt')))} labels saved to {save_dir / 'labels'}" if save_txt else ''
+ LOGGER.info(f"Results saved to {colorstr('bold', save_dir)}{s}")
+ if update:
+ strip_optimizer(weights[0]) # update model (to fix SourceChangeWarning)
+
+
+def parse_opt():
+ parser = argparse.ArgumentParser()
+ parser.add_argument('--weights', nargs='+', type=str, default=ROOT / 'yolov5s-seg.pt', help='model path(s)')
+ parser.add_argument('--source', type=str, default=ROOT / 'data/images', help='file/dir/URL/glob/screen/0(webcam)')
+ parser.add_argument('--data', type=str, default=ROOT / 'data/coco128.yaml', help='(optional) dataset.yaml path')
+ parser.add_argument('--imgsz', '--img', '--img-size', nargs='+', type=int, default=[640], help='inference size h,w')
+ parser.add_argument('--conf-thres', type=float, default=0.25, help='confidence threshold')
+ parser.add_argument('--iou-thres', type=float, default=0.45, help='NMS IoU threshold')
+ parser.add_argument('--max-det', type=int, default=1000, help='maximum detections per image')
+ parser.add_argument('--device', default='', help='cuda device, i.e. 0 or 0,1,2,3 or cpu')
+ parser.add_argument('--view-img', action='store_true', help='show results')
+ parser.add_argument('--save-txt', action='store_true', help='save results to *.txt')
+ parser.add_argument('--save-conf', action='store_true', help='save confidences in --save-txt labels')
+ parser.add_argument('--save-crop', action='store_true', help='save cropped prediction boxes')
+ parser.add_argument('--nosave', action='store_true', help='do not save images/videos')
+ parser.add_argument('--classes', nargs='+', type=int, help='filter by class: --classes 0, or --classes 0 2 3')
+ parser.add_argument('--agnostic-nms', action='store_true', help='class-agnostic NMS')
+ parser.add_argument('--augment', action='store_true', help='augmented inference')
+ parser.add_argument('--visualize', action='store_true', help='visualize features')
+ parser.add_argument('--update', action='store_true', help='update all models')
+ parser.add_argument('--project', default=ROOT / 'runs/predict-seg', help='save results to project/name')
+ parser.add_argument('--name', default='exp', help='save results to project/name')
+ parser.add_argument('--exist-ok', action='store_true', help='existing project/name ok, do not increment')
+ parser.add_argument('--line-thickness', default=3, type=int, help='bounding box thickness (pixels)')
+ parser.add_argument('--hide-labels', default=False, action='store_true', help='hide labels')
+ parser.add_argument('--hide-conf', default=False, action='store_true', help='hide confidences')
+ parser.add_argument('--half', action='store_true', help='use FP16 half-precision inference')
+ parser.add_argument('--dnn', action='store_true', help='use OpenCV DNN for ONNX inference')
+ parser.add_argument('--vid-stride', type=int, default=1, help='video frame-rate stride')
+ parser.add_argument('--retina-masks', action='store_true', help='whether to plot masks in native resolution')
+ opt = parser.parse_args()
+ opt.imgsz *= 2 if len(opt.imgsz) == 1 else 1 # expand
+ print_args(vars(opt))
+ return opt
+
+
+def main(opt):
+ check_requirements(exclude=('tensorboard', 'thop'))
+ run(**vars(opt))
+
+
+if __name__ == '__main__':
+ opt = parse_opt()
+ main(opt)
diff --git a/yolov5/segment/train.py b/yolov5/segment/train.py
new file mode 100644
index 0000000000000000000000000000000000000000..073fc742005b856992f853af2a54093c638d79b6
--- /dev/null
+++ b/yolov5/segment/train.py
@@ -0,0 +1,666 @@
+# YOLOv5 🚀 by Ultralytics, AGPL-3.0 license
+"""
+Train a YOLOv5 segment model on a segment dataset
+Models and datasets download automatically from the latest YOLOv5 release.
+
+Usage - Single-GPU training:
+ $ python segment/train.py --data coco128-seg.yaml --weights yolov5s-seg.pt --img 640 # from pretrained (recommended)
+ $ python segment/train.py --data coco128-seg.yaml --weights '' --cfg yolov5s-seg.yaml --img 640 # from scratch
+
+Usage - Multi-GPU DDP training:
+ $ python -m torch.distributed.run --nproc_per_node 4 --master_port 1 segment/train.py --data coco128-seg.yaml --weights yolov5s-seg.pt --img 640 --device 0,1,2,3
+
+Models: https://github.com/ultralytics/yolov5/tree/master/models
+Datasets: https://github.com/ultralytics/yolov5/tree/master/data
+Tutorial: https://docs.ultralytics.com/yolov5/tutorials/train_custom_data
+"""
+
+import argparse
+import math
+import os
+import random
+import subprocess
+import sys
+import time
+from copy import deepcopy
+from datetime import datetime
+from pathlib import Path
+
+import numpy as np
+import torch
+import torch.distributed as dist
+import torch.nn as nn
+import yaml
+from torch.optim import lr_scheduler
+from tqdm import tqdm
+
+FILE = Path(__file__).resolve()
+ROOT = FILE.parents[1] # YOLOv5 root directory
+if str(ROOT) not in sys.path:
+ sys.path.append(str(ROOT)) # add ROOT to PATH
+ROOT = Path(os.path.relpath(ROOT, Path.cwd())) # relative
+
+import segment.val as validate # for end-of-epoch mAP
+from models.experimental import attempt_load
+from models.yolo import SegmentationModel
+from utils.autoanchor import check_anchors
+from utils.autobatch import check_train_batch_size
+from utils.callbacks import Callbacks
+from utils.downloads import attempt_download, is_url
+from utils.general import (LOGGER, TQDM_BAR_FORMAT, check_amp, check_dataset, check_file, check_git_info,
+ check_git_status, check_img_size, check_requirements, check_suffix, check_yaml, colorstr,
+ get_latest_run, increment_path, init_seeds, intersect_dicts, labels_to_class_weights,
+ labels_to_image_weights, one_cycle, print_args, print_mutation, strip_optimizer, yaml_save)
+from utils.loggers import GenericLogger
+from utils.plots import plot_evolve, plot_labels
+from utils.segment.dataloaders import create_dataloader
+from utils.segment.loss import ComputeLoss
+from utils.segment.metrics import KEYS, fitness
+from utils.segment.plots import plot_images_and_masks, plot_results_with_masks
+from utils.torch_utils import (EarlyStopping, ModelEMA, de_parallel, select_device, smart_DDP, smart_optimizer,
+ smart_resume, torch_distributed_zero_first)
+
+LOCAL_RANK = int(os.getenv('LOCAL_RANK', -1)) # https://pytorch.org/docs/stable/elastic/run.html
+RANK = int(os.getenv('RANK', -1))
+WORLD_SIZE = int(os.getenv('WORLD_SIZE', 1))
+GIT_INFO = check_git_info()
+
+
+def train(hyp, opt, device, callbacks): # hyp is path/to/hyp.yaml or hyp dictionary
+ save_dir, epochs, batch_size, weights, single_cls, evolve, data, cfg, resume, noval, nosave, workers, freeze, mask_ratio = \
+ Path(opt.save_dir), opt.epochs, opt.batch_size, opt.weights, opt.single_cls, opt.evolve, opt.data, opt.cfg, \
+ opt.resume, opt.noval, opt.nosave, opt.workers, opt.freeze, opt.mask_ratio
+ # callbacks.run('on_pretrain_routine_start')
+
+ # Directories
+ w = save_dir / 'weights' # weights dir
+ (w.parent if evolve else w).mkdir(parents=True, exist_ok=True) # make dir
+ last, best = w / 'last.pt', w / 'best.pt'
+
+ # Hyperparameters
+ if isinstance(hyp, str):
+ with open(hyp, errors='ignore') as f:
+ hyp = yaml.safe_load(f) # load hyps dict
+ LOGGER.info(colorstr('hyperparameters: ') + ', '.join(f'{k}={v}' for k, v in hyp.items()))
+ opt.hyp = hyp.copy() # for saving hyps to checkpoints
+
+ # Save run settings
+ if not evolve:
+ yaml_save(save_dir / 'hyp.yaml', hyp)
+ yaml_save(save_dir / 'opt.yaml', vars(opt))
+
+ # Loggers
+ data_dict = None
+ if RANK in {-1, 0}:
+ logger = GenericLogger(opt=opt, console_logger=LOGGER)
+
+ # Config
+ plots = not evolve and not opt.noplots # create plots
+ overlap = not opt.no_overlap
+ cuda = device.type != 'cpu'
+ init_seeds(opt.seed + 1 + RANK, deterministic=True)
+ with torch_distributed_zero_first(LOCAL_RANK):
+ data_dict = data_dict or check_dataset(data) # check if None
+ train_path, val_path = data_dict['train'], data_dict['val']
+ nc = 1 if single_cls else int(data_dict['nc']) # number of classes
+ names = {0: 'item'} if single_cls and len(data_dict['names']) != 1 else data_dict['names'] # class names
+ is_coco = isinstance(val_path, str) and val_path.endswith('coco/val2017.txt') # COCO dataset
+
+ # Model
+ check_suffix(weights, '.pt') # check weights
+ pretrained = weights.endswith('.pt')
+ if pretrained:
+ with torch_distributed_zero_first(LOCAL_RANK):
+ weights = attempt_download(weights) # download if not found locally
+ ckpt = torch.load(weights, map_location='cpu') # load checkpoint to CPU to avoid CUDA memory leak
+ model = SegmentationModel(cfg or ckpt['model'].yaml, ch=3, nc=nc, anchors=hyp.get('anchors')).to(device)
+ exclude = ['anchor'] if (cfg or hyp.get('anchors')) and not resume else [] # exclude keys
+ csd = ckpt['model'].float().state_dict() # checkpoint state_dict as FP32
+ csd = intersect_dicts(csd, model.state_dict(), exclude=exclude) # intersect
+ model.load_state_dict(csd, strict=False) # load
+ LOGGER.info(f'Transferred {len(csd)}/{len(model.state_dict())} items from {weights}') # report
+ else:
+ model = SegmentationModel(cfg, ch=3, nc=nc, anchors=hyp.get('anchors')).to(device) # create
+ amp = check_amp(model) # check AMP
+
+ # Freeze
+ freeze = [f'model.{x}.' for x in (freeze if len(freeze) > 1 else range(freeze[0]))] # layers to freeze
+ for k, v in model.named_parameters():
+ v.requires_grad = True # train all layers
+ # v.register_hook(lambda x: torch.nan_to_num(x)) # NaN to 0 (commented for erratic training results)
+ if any(x in k for x in freeze):
+ LOGGER.info(f'freezing {k}')
+ v.requires_grad = False
+
+ # Image size
+ gs = max(int(model.stride.max()), 32) # grid size (max stride)
+ imgsz = check_img_size(opt.imgsz, gs, floor=gs * 2) # verify imgsz is gs-multiple
+
+ # Batch size
+ if RANK == -1 and batch_size == -1: # single-GPU only, estimate best batch size
+ batch_size = check_train_batch_size(model, imgsz, amp)
+ logger.update_params({'batch_size': batch_size})
+ # loggers.on_params_update({"batch_size": batch_size})
+
+ # Optimizer
+ nbs = 64 # nominal batch size
+ accumulate = max(round(nbs / batch_size), 1) # accumulate loss before optimizing
+ hyp['weight_decay'] *= batch_size * accumulate / nbs # scale weight_decay
+ optimizer = smart_optimizer(model, opt.optimizer, hyp['lr0'], hyp['momentum'], hyp['weight_decay'])
+
+ # Scheduler
+ if opt.cos_lr:
+ lf = one_cycle(1, hyp['lrf'], epochs) # cosine 1->hyp['lrf']
+ else:
+ lf = lambda x: (1 - x / epochs) * (1.0 - hyp['lrf']) + hyp['lrf'] # linear
+ scheduler = lr_scheduler.LambdaLR(optimizer, lr_lambda=lf) # plot_lr_scheduler(optimizer, scheduler, epochs)
+
+ # EMA
+ ema = ModelEMA(model) if RANK in {-1, 0} else None
+
+ # Resume
+ best_fitness, start_epoch = 0.0, 0
+ if pretrained:
+ if resume:
+ best_fitness, start_epoch, epochs = smart_resume(ckpt, optimizer, ema, weights, epochs, resume)
+ del ckpt, csd
+
+ # DP mode
+ if cuda and RANK == -1 and torch.cuda.device_count() > 1:
+ LOGGER.warning(
+ 'WARNING ⚠️ DP not recommended, use torch.distributed.run for best DDP Multi-GPU results.\n'
+ 'See Multi-GPU Tutorial at https://docs.ultralytics.com/yolov5/tutorials/multi_gpu_training to get started.'
+ )
+ model = torch.nn.DataParallel(model)
+
+ # SyncBatchNorm
+ if opt.sync_bn and cuda and RANK != -1:
+ model = torch.nn.SyncBatchNorm.convert_sync_batchnorm(model).to(device)
+ LOGGER.info('Using SyncBatchNorm()')
+
+ # Trainloader
+ train_loader, dataset = create_dataloader(
+ train_path,
+ imgsz,
+ batch_size // WORLD_SIZE,
+ gs,
+ single_cls,
+ hyp=hyp,
+ augment=True,
+ cache=None if opt.cache == 'val' else opt.cache,
+ rect=opt.rect,
+ rank=LOCAL_RANK,
+ workers=workers,
+ image_weights=opt.image_weights,
+ quad=opt.quad,
+ prefix=colorstr('train: '),
+ shuffle=True,
+ mask_downsample_ratio=mask_ratio,
+ overlap_mask=overlap,
+ )
+ labels = np.concatenate(dataset.labels, 0)
+ mlc = int(labels[:, 0].max()) # max label class
+ assert mlc < nc, f'Label class {mlc} exceeds nc={nc} in {data}. Possible class labels are 0-{nc - 1}'
+
+ # Process 0
+ if RANK in {-1, 0}:
+ val_loader = create_dataloader(val_path,
+ imgsz,
+ batch_size // WORLD_SIZE * 2,
+ gs,
+ single_cls,
+ hyp=hyp,
+ cache=None if noval else opt.cache,
+ rect=True,
+ rank=-1,
+ workers=workers * 2,
+ pad=0.5,
+ mask_downsample_ratio=mask_ratio,
+ overlap_mask=overlap,
+ prefix=colorstr('val: '))[0]
+
+ if not resume:
+ if not opt.noautoanchor:
+ check_anchors(dataset, model=model, thr=hyp['anchor_t'], imgsz=imgsz) # run AutoAnchor
+ model.half().float() # pre-reduce anchor precision
+
+ if plots:
+ plot_labels(labels, names, save_dir)
+ # callbacks.run('on_pretrain_routine_end', labels, names)
+
+ # DDP mode
+ if cuda and RANK != -1:
+ model = smart_DDP(model)
+
+ # Model attributes
+ nl = de_parallel(model).model[-1].nl # number of detection layers (to scale hyps)
+ hyp['box'] *= 3 / nl # scale to layers
+ hyp['cls'] *= nc / 80 * 3 / nl # scale to classes and layers
+ hyp['obj'] *= (imgsz / 640) ** 2 * 3 / nl # scale to image size and layers
+ hyp['label_smoothing'] = opt.label_smoothing
+ model.nc = nc # attach number of classes to model
+ model.hyp = hyp # attach hyperparameters to model
+ model.class_weights = labels_to_class_weights(dataset.labels, nc).to(device) * nc # attach class weights
+ model.names = names
+
+ # Start training
+ t0 = time.time()
+ nb = len(train_loader) # number of batches
+ nw = max(round(hyp['warmup_epochs'] * nb), 100) # number of warmup iterations, max(3 epochs, 100 iterations)
+ # nw = min(nw, (epochs - start_epoch) / 2 * nb) # limit warmup to < 1/2 of training
+ last_opt_step = -1
+ maps = np.zeros(nc) # mAP per class
+ results = (0, 0, 0, 0, 0, 0, 0, 0, 0, 0, 0, 0) # P, R, mAP@.5, mAP@.5-.95, val_loss(box, obj, cls)
+ scheduler.last_epoch = start_epoch - 1 # do not move
+ scaler = torch.cuda.amp.GradScaler(enabled=amp)
+ stopper, stop = EarlyStopping(patience=opt.patience), False
+ compute_loss = ComputeLoss(model, overlap=overlap) # init loss class
+ # callbacks.run('on_train_start')
+ LOGGER.info(f'Image sizes {imgsz} train, {imgsz} val\n'
+ f'Using {train_loader.num_workers * WORLD_SIZE} dataloader workers\n'
+ f"Logging results to {colorstr('bold', save_dir)}\n"
+ f'Starting training for {epochs} epochs...')
+ for epoch in range(start_epoch, epochs): # epoch ------------------------------------------------------------------
+ # callbacks.run('on_train_epoch_start')
+ model.train()
+
+ # Update image weights (optional, single-GPU only)
+ if opt.image_weights:
+ cw = model.class_weights.cpu().numpy() * (1 - maps) ** 2 / nc # class weights
+ iw = labels_to_image_weights(dataset.labels, nc=nc, class_weights=cw) # image weights
+ dataset.indices = random.choices(range(dataset.n), weights=iw, k=dataset.n) # rand weighted idx
+
+ # Update mosaic border (optional)
+ # b = int(random.uniform(0.25 * imgsz, 0.75 * imgsz + gs) // gs * gs)
+ # dataset.mosaic_border = [b - imgsz, -b] # height, width borders
+
+ mloss = torch.zeros(4, device=device) # mean losses
+ if RANK != -1:
+ train_loader.sampler.set_epoch(epoch)
+ pbar = enumerate(train_loader)
+ LOGGER.info(('\n' + '%11s' * 8) %
+ ('Epoch', 'GPU_mem', 'box_loss', 'seg_loss', 'obj_loss', 'cls_loss', 'Instances', 'Size'))
+ if RANK in {-1, 0}:
+ pbar = tqdm(pbar, total=nb, bar_format=TQDM_BAR_FORMAT) # progress bar
+ optimizer.zero_grad()
+ for i, (imgs, targets, paths, _, masks) in pbar: # batch ------------------------------------------------------
+ # callbacks.run('on_train_batch_start')
+ ni = i + nb * epoch # number integrated batches (since train start)
+ imgs = imgs.to(device, non_blocking=True).float() / 255 # uint8 to float32, 0-255 to 0.0-1.0
+
+ # Warmup
+ if ni <= nw:
+ xi = [0, nw] # x interp
+ # compute_loss.gr = np.interp(ni, xi, [0.0, 1.0]) # iou loss ratio (obj_loss = 1.0 or iou)
+ accumulate = max(1, np.interp(ni, xi, [1, nbs / batch_size]).round())
+ for j, x in enumerate(optimizer.param_groups):
+ # bias lr falls from 0.1 to lr0, all other lrs rise from 0.0 to lr0
+ x['lr'] = np.interp(ni, xi, [hyp['warmup_bias_lr'] if j == 0 else 0.0, x['initial_lr'] * lf(epoch)])
+ if 'momentum' in x:
+ x['momentum'] = np.interp(ni, xi, [hyp['warmup_momentum'], hyp['momentum']])
+
+ # Multi-scale
+ if opt.multi_scale:
+ sz = random.randrange(int(imgsz * 0.5), int(imgsz * 1.5) + gs) // gs * gs # size
+ sf = sz / max(imgs.shape[2:]) # scale factor
+ if sf != 1:
+ ns = [math.ceil(x * sf / gs) * gs for x in imgs.shape[2:]] # new shape (stretched to gs-multiple)
+ imgs = nn.functional.interpolate(imgs, size=ns, mode='bilinear', align_corners=False)
+
+ # Forward
+ with torch.cuda.amp.autocast(amp):
+ pred = model(imgs) # forward
+ loss, loss_items = compute_loss(pred, targets.to(device), masks=masks.to(device).float())
+ if RANK != -1:
+ loss *= WORLD_SIZE # gradient averaged between devices in DDP mode
+ if opt.quad:
+ loss *= 4.
+
+ # Backward
+ scaler.scale(loss).backward()
+
+ # Optimize - https://pytorch.org/docs/master/notes/amp_examples.html
+ if ni - last_opt_step >= accumulate:
+ scaler.unscale_(optimizer) # unscale gradients
+ torch.nn.utils.clip_grad_norm_(model.parameters(), max_norm=10.0) # clip gradients
+ scaler.step(optimizer) # optimizer.step
+ scaler.update()
+ optimizer.zero_grad()
+ if ema:
+ ema.update(model)
+ last_opt_step = ni
+
+ # Log
+ if RANK in {-1, 0}:
+ mloss = (mloss * i + loss_items) / (i + 1) # update mean losses
+ mem = f'{torch.cuda.memory_reserved() / 1E9 if torch.cuda.is_available() else 0:.3g}G' # (GB)
+ pbar.set_description(('%11s' * 2 + '%11.4g' * 6) %
+ (f'{epoch}/{epochs - 1}', mem, *mloss, targets.shape[0], imgs.shape[-1]))
+ # callbacks.run('on_train_batch_end', model, ni, imgs, targets, paths)
+ # if callbacks.stop_training:
+ # return
+
+ # Mosaic plots
+ if plots:
+ if ni < 3:
+ plot_images_and_masks(imgs, targets, masks, paths, save_dir / f'train_batch{ni}.jpg')
+ if ni == 10:
+ files = sorted(save_dir.glob('train*.jpg'))
+ logger.log_images(files, 'Mosaics', epoch)
+ # end batch ------------------------------------------------------------------------------------------------
+
+ # Scheduler
+ lr = [x['lr'] for x in optimizer.param_groups] # for loggers
+ scheduler.step()
+
+ if RANK in {-1, 0}:
+ # mAP
+ # callbacks.run('on_train_epoch_end', epoch=epoch)
+ ema.update_attr(model, include=['yaml', 'nc', 'hyp', 'names', 'stride', 'class_weights'])
+ final_epoch = (epoch + 1 == epochs) or stopper.possible_stop
+ if not noval or final_epoch: # Calculate mAP
+ results, maps, _ = validate.run(data_dict,
+ batch_size=batch_size // WORLD_SIZE * 2,
+ imgsz=imgsz,
+ half=amp,
+ model=ema.ema,
+ single_cls=single_cls,
+ dataloader=val_loader,
+ save_dir=save_dir,
+ plots=False,
+ callbacks=callbacks,
+ compute_loss=compute_loss,
+ mask_downsample_ratio=mask_ratio,
+ overlap=overlap)
+
+ # Update best mAP
+ fi = fitness(np.array(results).reshape(1, -1)) # weighted combination of [P, R, mAP@.5, mAP@.5-.95]
+ stop = stopper(epoch=epoch, fitness=fi) # early stop check
+ if fi > best_fitness:
+ best_fitness = fi
+ log_vals = list(mloss) + list(results) + lr
+ # callbacks.run('on_fit_epoch_end', log_vals, epoch, best_fitness, fi)
+ # Log val metrics and media
+ metrics_dict = dict(zip(KEYS, log_vals))
+ logger.log_metrics(metrics_dict, epoch)
+
+ # Save model
+ if (not nosave) or (final_epoch and not evolve): # if save
+ ckpt = {
+ 'epoch': epoch,
+ 'best_fitness': best_fitness,
+ 'model': deepcopy(de_parallel(model)).half(),
+ 'ema': deepcopy(ema.ema).half(),
+ 'updates': ema.updates,
+ 'optimizer': optimizer.state_dict(),
+ 'opt': vars(opt),
+ 'git': GIT_INFO, # {remote, branch, commit} if a git repo
+ 'date': datetime.now().isoformat()}
+
+ # Save last, best and delete
+ torch.save(ckpt, last)
+ if best_fitness == fi:
+ torch.save(ckpt, best)
+ if opt.save_period > 0 and epoch % opt.save_period == 0:
+ torch.save(ckpt, w / f'epoch{epoch}.pt')
+ logger.log_model(w / f'epoch{epoch}.pt')
+ del ckpt
+ # callbacks.run('on_model_save', last, epoch, final_epoch, best_fitness, fi)
+
+ # EarlyStopping
+ if RANK != -1: # if DDP training
+ broadcast_list = [stop if RANK == 0 else None]
+ dist.broadcast_object_list(broadcast_list, 0) # broadcast 'stop' to all ranks
+ if RANK != 0:
+ stop = broadcast_list[0]
+ if stop:
+ break # must break all DDP ranks
+
+ # end epoch ----------------------------------------------------------------------------------------------------
+ # end training -----------------------------------------------------------------------------------------------------
+ if RANK in {-1, 0}:
+ LOGGER.info(f'\n{epoch - start_epoch + 1} epochs completed in {(time.time() - t0) / 3600:.3f} hours.')
+ for f in last, best:
+ if f.exists():
+ strip_optimizer(f) # strip optimizers
+ if f is best:
+ LOGGER.info(f'\nValidating {f}...')
+ results, _, _ = validate.run(
+ data_dict,
+ batch_size=batch_size // WORLD_SIZE * 2,
+ imgsz=imgsz,
+ model=attempt_load(f, device).half(),
+ iou_thres=0.65 if is_coco else 0.60, # best pycocotools at iou 0.65
+ single_cls=single_cls,
+ dataloader=val_loader,
+ save_dir=save_dir,
+ save_json=is_coco,
+ verbose=True,
+ plots=plots,
+ callbacks=callbacks,
+ compute_loss=compute_loss,
+ mask_downsample_ratio=mask_ratio,
+ overlap=overlap) # val best model with plots
+ if is_coco:
+ # callbacks.run('on_fit_epoch_end', list(mloss) + list(results) + lr, epoch, best_fitness, fi)
+ metrics_dict = dict(zip(KEYS, list(mloss) + list(results) + lr))
+ logger.log_metrics(metrics_dict, epoch)
+
+ # callbacks.run('on_train_end', last, best, epoch, results)
+ # on train end callback using genericLogger
+ logger.log_metrics(dict(zip(KEYS[4:16], results)), epochs)
+ if not opt.evolve:
+ logger.log_model(best, epoch)
+ if plots:
+ plot_results_with_masks(file=save_dir / 'results.csv') # save results.png
+ files = ['results.png', 'confusion_matrix.png', *(f'{x}_curve.png' for x in ('F1', 'PR', 'P', 'R'))]
+ files = [(save_dir / f) for f in files if (save_dir / f).exists()] # filter
+ LOGGER.info(f"Results saved to {colorstr('bold', save_dir)}")
+ logger.log_images(files, 'Results', epoch + 1)
+ logger.log_images(sorted(save_dir.glob('val*.jpg')), 'Validation', epoch + 1)
+ torch.cuda.empty_cache()
+ return results
+
+
+def parse_opt(known=False):
+ parser = argparse.ArgumentParser()
+ parser.add_argument('--weights', type=str, default=ROOT / 'yolov5s-seg.pt', help='initial weights path')
+ parser.add_argument('--cfg', type=str, default='', help='model.yaml path')
+ parser.add_argument('--data', type=str, default=ROOT / 'data/coco128-seg.yaml', help='dataset.yaml path')
+ parser.add_argument('--hyp', type=str, default=ROOT / 'data/hyps/hyp.scratch-low.yaml', help='hyperparameters path')
+ parser.add_argument('--epochs', type=int, default=100, help='total training epochs')
+ parser.add_argument('--batch-size', type=int, default=16, help='total batch size for all GPUs, -1 for autobatch')
+ parser.add_argument('--imgsz', '--img', '--img-size', type=int, default=640, help='train, val image size (pixels)')
+ parser.add_argument('--rect', action='store_true', help='rectangular training')
+ parser.add_argument('--resume', nargs='?', const=True, default=False, help='resume most recent training')
+ parser.add_argument('--nosave', action='store_true', help='only save final checkpoint')
+ parser.add_argument('--noval', action='store_true', help='only validate final epoch')
+ parser.add_argument('--noautoanchor', action='store_true', help='disable AutoAnchor')
+ parser.add_argument('--noplots', action='store_true', help='save no plot files')
+ parser.add_argument('--evolve', type=int, nargs='?', const=300, help='evolve hyperparameters for x generations')
+ parser.add_argument('--bucket', type=str, default='', help='gsutil bucket')
+ parser.add_argument('--cache', type=str, nargs='?', const='ram', help='image --cache ram/disk')
+ parser.add_argument('--image-weights', action='store_true', help='use weighted image selection for training')
+ parser.add_argument('--device', default='', help='cuda device, i.e. 0 or 0,1,2,3 or cpu')
+ parser.add_argument('--multi-scale', action='store_true', help='vary img-size +/- 50%%')
+ parser.add_argument('--single-cls', action='store_true', help='train multi-class data as single-class')
+ parser.add_argument('--optimizer', type=str, choices=['SGD', 'Adam', 'AdamW'], default='SGD', help='optimizer')
+ parser.add_argument('--sync-bn', action='store_true', help='use SyncBatchNorm, only available in DDP mode')
+ parser.add_argument('--workers', type=int, default=8, help='max dataloader workers (per RANK in DDP mode)')
+ parser.add_argument('--project', default=ROOT / 'runs/train-seg', help='save to project/name')
+ parser.add_argument('--name', default='exp', help='save to project/name')
+ parser.add_argument('--exist-ok', action='store_true', help='existing project/name ok, do not increment')
+ parser.add_argument('--quad', action='store_true', help='quad dataloader')
+ parser.add_argument('--cos-lr', action='store_true', help='cosine LR scheduler')
+ parser.add_argument('--label-smoothing', type=float, default=0.0, help='Label smoothing epsilon')
+ parser.add_argument('--patience', type=int, default=100, help='EarlyStopping patience (epochs without improvement)')
+ parser.add_argument('--freeze', nargs='+', type=int, default=[0], help='Freeze layers: backbone=10, first3=0 1 2')
+ parser.add_argument('--save-period', type=int, default=-1, help='Save checkpoint every x epochs (disabled if < 1)')
+ parser.add_argument('--seed', type=int, default=0, help='Global training seed')
+ parser.add_argument('--local_rank', type=int, default=-1, help='Automatic DDP Multi-GPU argument, do not modify')
+
+ # Instance Segmentation Args
+ parser.add_argument('--mask-ratio', type=int, default=4, help='Downsample the truth masks to saving memory')
+ parser.add_argument('--no-overlap', action='store_true', help='Overlap masks train faster at slightly less mAP')
+
+ return parser.parse_known_args()[0] if known else parser.parse_args()
+
+
+def main(opt, callbacks=Callbacks()):
+ # Checks
+ if RANK in {-1, 0}:
+ print_args(vars(opt))
+ check_git_status()
+ check_requirements()
+
+ # Resume
+ if opt.resume and not opt.evolve: # resume from specified or most recent last.pt
+ last = Path(check_file(opt.resume) if isinstance(opt.resume, str) else get_latest_run())
+ opt_yaml = last.parent.parent / 'opt.yaml' # train options yaml
+ opt_data = opt.data # original dataset
+ if opt_yaml.is_file():
+ with open(opt_yaml, errors='ignore') as f:
+ d = yaml.safe_load(f)
+ else:
+ d = torch.load(last, map_location='cpu')['opt']
+ opt = argparse.Namespace(**d) # replace
+ opt.cfg, opt.weights, opt.resume = '', str(last), True # reinstate
+ if is_url(opt_data):
+ opt.data = check_file(opt_data) # avoid HUB resume auth timeout
+ else:
+ opt.data, opt.cfg, opt.hyp, opt.weights, opt.project = \
+ check_file(opt.data), check_yaml(opt.cfg), check_yaml(opt.hyp), str(opt.weights), str(opt.project) # checks
+ assert len(opt.cfg) or len(opt.weights), 'either --cfg or --weights must be specified'
+ if opt.evolve:
+ if opt.project == str(ROOT / 'runs/train-seg'): # if default project name, rename to runs/evolve-seg
+ opt.project = str(ROOT / 'runs/evolve-seg')
+ opt.exist_ok, opt.resume = opt.resume, False # pass resume to exist_ok and disable resume
+ if opt.name == 'cfg':
+ opt.name = Path(opt.cfg).stem # use model.yaml as name
+ opt.save_dir = str(increment_path(Path(opt.project) / opt.name, exist_ok=opt.exist_ok))
+
+ # DDP mode
+ device = select_device(opt.device, batch_size=opt.batch_size)
+ if LOCAL_RANK != -1:
+ msg = 'is not compatible with YOLOv5 Multi-GPU DDP training'
+ assert not opt.image_weights, f'--image-weights {msg}'
+ assert not opt.evolve, f'--evolve {msg}'
+ assert opt.batch_size != -1, f'AutoBatch with --batch-size -1 {msg}, please pass a valid --batch-size'
+ assert opt.batch_size % WORLD_SIZE == 0, f'--batch-size {opt.batch_size} must be multiple of WORLD_SIZE'
+ assert torch.cuda.device_count() > LOCAL_RANK, 'insufficient CUDA devices for DDP command'
+ torch.cuda.set_device(LOCAL_RANK)
+ device = torch.device('cuda', LOCAL_RANK)
+ dist.init_process_group(backend='nccl' if dist.is_nccl_available() else 'gloo')
+
+ # Train
+ if not opt.evolve:
+ train(opt.hyp, opt, device, callbacks)
+
+ # Evolve hyperparameters (optional)
+ else:
+ # Hyperparameter evolution metadata (mutation scale 0-1, lower_limit, upper_limit)
+ meta = {
+ 'lr0': (1, 1e-5, 1e-1), # initial learning rate (SGD=1E-2, Adam=1E-3)
+ 'lrf': (1, 0.01, 1.0), # final OneCycleLR learning rate (lr0 * lrf)
+ 'momentum': (0.3, 0.6, 0.98), # SGD momentum/Adam beta1
+ 'weight_decay': (1, 0.0, 0.001), # optimizer weight decay
+ 'warmup_epochs': (1, 0.0, 5.0), # warmup epochs (fractions ok)
+ 'warmup_momentum': (1, 0.0, 0.95), # warmup initial momentum
+ 'warmup_bias_lr': (1, 0.0, 0.2), # warmup initial bias lr
+ 'box': (1, 0.02, 0.2), # box loss gain
+ 'cls': (1, 0.2, 4.0), # cls loss gain
+ 'cls_pw': (1, 0.5, 2.0), # cls BCELoss positive_weight
+ 'obj': (1, 0.2, 4.0), # obj loss gain (scale with pixels)
+ 'obj_pw': (1, 0.5, 2.0), # obj BCELoss positive_weight
+ 'iou_t': (0, 0.1, 0.7), # IoU training threshold
+ 'anchor_t': (1, 2.0, 8.0), # anchor-multiple threshold
+ 'anchors': (2, 2.0, 10.0), # anchors per output grid (0 to ignore)
+ 'fl_gamma': (0, 0.0, 2.0), # focal loss gamma (efficientDet default gamma=1.5)
+ 'hsv_h': (1, 0.0, 0.1), # image HSV-Hue augmentation (fraction)
+ 'hsv_s': (1, 0.0, 0.9), # image HSV-Saturation augmentation (fraction)
+ 'hsv_v': (1, 0.0, 0.9), # image HSV-Value augmentation (fraction)
+ 'degrees': (1, 0.0, 45.0), # image rotation (+/- deg)
+ 'translate': (1, 0.0, 0.9), # image translation (+/- fraction)
+ 'scale': (1, 0.0, 0.9), # image scale (+/- gain)
+ 'shear': (1, 0.0, 10.0), # image shear (+/- deg)
+ 'perspective': (0, 0.0, 0.001), # image perspective (+/- fraction), range 0-0.001
+ 'flipud': (1, 0.0, 1.0), # image flip up-down (probability)
+ 'fliplr': (0, 0.0, 1.0), # image flip left-right (probability)
+ 'mosaic': (1, 0.0, 1.0), # image mixup (probability)
+ 'mixup': (1, 0.0, 1.0), # image mixup (probability)
+ 'copy_paste': (1, 0.0, 1.0)} # segment copy-paste (probability)
+
+ with open(opt.hyp, errors='ignore') as f:
+ hyp = yaml.safe_load(f) # load hyps dict
+ if 'anchors' not in hyp: # anchors commented in hyp.yaml
+ hyp['anchors'] = 3
+ if opt.noautoanchor:
+ del hyp['anchors'], meta['anchors']
+ opt.noval, opt.nosave, save_dir = True, True, Path(opt.save_dir) # only val/save final epoch
+ # ei = [isinstance(x, (int, float)) for x in hyp.values()] # evolvable indices
+ evolve_yaml, evolve_csv = save_dir / 'hyp_evolve.yaml', save_dir / 'evolve.csv'
+ if opt.bucket:
+ # download evolve.csv if exists
+ subprocess.run([
+ 'gsutil',
+ 'cp',
+ f'gs://{opt.bucket}/evolve.csv',
+ str(evolve_csv),])
+
+ for _ in range(opt.evolve): # generations to evolve
+ if evolve_csv.exists(): # if evolve.csv exists: select best hyps and mutate
+ # Select parent(s)
+ parent = 'single' # parent selection method: 'single' or 'weighted'
+ x = np.loadtxt(evolve_csv, ndmin=2, delimiter=',', skiprows=1)
+ n = min(5, len(x)) # number of previous results to consider
+ x = x[np.argsort(-fitness(x))][:n] # top n mutations
+ w = fitness(x) - fitness(x).min() + 1E-6 # weights (sum > 0)
+ if parent == 'single' or len(x) == 1:
+ # x = x[random.randint(0, n - 1)] # random selection
+ x = x[random.choices(range(n), weights=w)[0]] # weighted selection
+ elif parent == 'weighted':
+ x = (x * w.reshape(n, 1)).sum(0) / w.sum() # weighted combination
+
+ # Mutate
+ mp, s = 0.8, 0.2 # mutation probability, sigma
+ npr = np.random
+ npr.seed(int(time.time()))
+ g = np.array([meta[k][0] for k in hyp.keys()]) # gains 0-1
+ ng = len(meta)
+ v = np.ones(ng)
+ while all(v == 1): # mutate until a change occurs (prevent duplicates)
+ v = (g * (npr.random(ng) < mp) * npr.randn(ng) * npr.random() * s + 1).clip(0.3, 3.0)
+ for i, k in enumerate(hyp.keys()): # plt.hist(v.ravel(), 300)
+ hyp[k] = float(x[i + 12] * v[i]) # mutate
+
+ # Constrain to limits
+ for k, v in meta.items():
+ hyp[k] = max(hyp[k], v[1]) # lower limit
+ hyp[k] = min(hyp[k], v[2]) # upper limit
+ hyp[k] = round(hyp[k], 5) # significant digits
+
+ # Train mutation
+ results = train(hyp.copy(), opt, device, callbacks)
+ callbacks = Callbacks()
+ # Write mutation results
+ print_mutation(KEYS[4:16], results, hyp.copy(), save_dir, opt.bucket)
+
+ # Plot results
+ plot_evolve(evolve_csv)
+ LOGGER.info(f'Hyperparameter evolution finished {opt.evolve} generations\n'
+ f"Results saved to {colorstr('bold', save_dir)}\n"
+ f'Usage example: $ python train.py --hyp {evolve_yaml}')
+
+
+def run(**kwargs):
+ # Usage: import train; train.run(data='coco128.yaml', imgsz=320, weights='yolov5m.pt')
+ opt = parse_opt(True)
+ for k, v in kwargs.items():
+ setattr(opt, k, v)
+ main(opt)
+ return opt
+
+
+if __name__ == '__main__':
+ opt = parse_opt()
+ main(opt)
diff --git a/yolov5/segment/tutorial.ipynb b/yolov5/segment/tutorial.ipynb
new file mode 100644
index 0000000000000000000000000000000000000000..f2aee9e26b336a66cc0b5f6d3c30006fdedc4b82
--- /dev/null
+++ b/yolov5/segment/tutorial.ipynb
@@ -0,0 +1,595 @@
+{
+ "cells": [
+ {
+ "cell_type": "markdown",
+ "metadata": {
+ "id": "t6MPjfT5NrKQ"
+ },
+ "source": [
+ "
\n",
+ "\n",
+ " \n",
+ " \n",
+ "\n",
+ "\n",
+ " \n",
+ " \n",
+ " \n",
+ " \n",
+ " \n",
+ "\n",
+ "This YOLOv5 🚀 notebook by Ultralytics presents simple train, validate and predict examples to help start your AI adventure. See GitHub for community support or contact us for professional support.\n",
+ "\n",
+ "
\n",
+ "Close the active learning loop by sampling images from your inference conditions with the `roboflow` pip package\n",
+ "
\n",
+ "\n",
+ "Train a YOLOv5s-seg model on the [COCO128](https://www.kaggle.com/ultralytics/coco128) dataset with `--data coco128-seg.yaml`, starting from pretrained `--weights yolov5s-seg.pt`, or from randomly initialized `--weights '' --cfg yolov5s-seg.yaml`.\n",
+ "\n",
+ "- **Pretrained [Models](https://github.com/ultralytics/yolov5/tree/master/models)** are downloaded\n",
+ "automatically from the [latest YOLOv5 release](https://github.com/ultralytics/yolov5/releases)\n",
+ "- **[Datasets](https://github.com/ultralytics/yolov5/tree/master/data)** available for autodownload include: [COCO](https://github.com/ultralytics/yolov5/blob/master/data/coco.yaml), [COCO128](https://github.com/ultralytics/yolov5/blob/master/data/coco128.yaml), [VOC](https://github.com/ultralytics/yolov5/blob/master/data/VOC.yaml), [Argoverse](https://github.com/ultralytics/yolov5/blob/master/data/Argoverse.yaml), [VisDrone](https://github.com/ultralytics/yolov5/blob/master/data/VisDrone.yaml), [GlobalWheat](https://github.com/ultralytics/yolov5/blob/master/data/GlobalWheat2020.yaml), [xView](https://github.com/ultralytics/yolov5/blob/master/data/xView.yaml), [Objects365](https://github.com/ultralytics/yolov5/blob/master/data/Objects365.yaml), [SKU-110K](https://github.com/ultralytics/yolov5/blob/master/data/SKU-110K.yaml).\n",
+ "- **Training Results** are saved to `runs/train-seg/` with incrementing run directories, i.e. `runs/train-seg/exp2`, `runs/train-seg/exp3` etc.\n",
+ "
\n",
+ "\n",
+ "A **Mosaic Dataloader** is used for training which combines 4 images into 1 mosaic.\n",
+ "\n",
+ "## Train on Custom Data with Roboflow 🌟 NEW\n",
+ "\n",
+ "[Roboflow](https://roboflow.com/?ref=ultralytics) enables you to easily **organize, label, and prepare** a high quality dataset with your own custom data. Roboflow also makes it easy to establish an active learning pipeline, collaborate with your team on dataset improvement, and integrate directly into your model building workflow with the `roboflow` pip package.\n",
+ "\n",
+ "- Custom Training Example: [https://blog.roboflow.com/train-yolov5-instance-segmentation-custom-dataset/](https://blog.roboflow.com/train-yolov5-instance-segmentation-custom-dataset/?ref=ultralytics)\n",
+ "- Custom Training Notebook: [](https://colab.research.google.com/drive/1JTz7kpmHsg-5qwVz2d2IH3AaenI1tv0N?usp=sharing)\n",
+ " \n",
+ "\n",
+ "
Label images lightning fast (including with model-assisted labeling)"
+ ]
+ },
+ {
+ "cell_type": "code",
+ "execution_count": null,
+ "metadata": {
+ "id": "i3oKtE4g-aNn"
+ },
+ "outputs": [],
+ "source": [
+ "#@title Select YOLOv5 🚀 logger {run: 'auto'}\n",
+ "logger = 'Comet' #@param ['Comet', 'ClearML', 'TensorBoard']\n",
+ "\n",
+ "if logger == 'Comet':\n",
+ " %pip install -q comet_ml\n",
+ " import comet_ml; comet_ml.init()\n",
+ "elif logger == 'ClearML':\n",
+ " %pip install -q clearml\n",
+ " import clearml; clearml.browser_login()\n",
+ "elif logger == 'TensorBoard':\n",
+ " %load_ext tensorboard\n",
+ " %tensorboard --logdir runs/train"
+ ]
+ },
+ {
+ "cell_type": "code",
+ "execution_count": null,
+ "metadata": {
+ "colab": {
+ "base_uri": "https://localhost:8080/"
+ },
+ "id": "1NcFxRcFdJ_O",
+ "outputId": "3a3e0cf7-e79c-47a5-c8e7-2d26eeeab988"
+ },
+ "outputs": [
+ {
+ "output_type": "stream",
+ "name": "stdout",
+ "text": [
+ "\u001b[34m\u001b[1msegment/train: \u001b[0mweights=yolov5s-seg.pt, cfg=, data=coco128-seg.yaml, hyp=data/hyps/hyp.scratch-low.yaml, epochs=3, batch_size=16, imgsz=640, rect=False, resume=False, nosave=False, noval=False, noautoanchor=False, noplots=False, evolve=None, bucket=, cache=ram, image_weights=False, device=, multi_scale=False, single_cls=False, optimizer=SGD, sync_bn=False, workers=8, project=runs/train-seg, name=exp, exist_ok=False, quad=False, cos_lr=False, label_smoothing=0.0, patience=100, freeze=[0], save_period=-1, seed=0, local_rank=-1, mask_ratio=4, no_overlap=False\n",
+ "\u001b[34m\u001b[1mgithub: \u001b[0mup to date with https://github.com/ultralytics/yolov5 ✅\n",
+ "YOLOv5 🚀 v7.0-2-gc9d47ae Python-3.7.15 torch-1.12.1+cu113 CUDA:0 (Tesla T4, 15110MiB)\n",
+ "\n",
+ "\u001b[34m\u001b[1mhyperparameters: \u001b[0mlr0=0.01, lrf=0.01, momentum=0.937, weight_decay=0.0005, warmup_epochs=3.0, warmup_momentum=0.8, warmup_bias_lr=0.1, box=0.05, cls=0.5, cls_pw=1.0, obj=1.0, obj_pw=1.0, iou_t=0.2, anchor_t=4.0, fl_gamma=0.0, hsv_h=0.015, hsv_s=0.7, hsv_v=0.4, degrees=0.0, translate=0.1, scale=0.5, shear=0.0, perspective=0.0, flipud=0.0, fliplr=0.5, mosaic=1.0, mixup=0.0, copy_paste=0.0\n",
+ "\u001b[34m\u001b[1mTensorBoard: \u001b[0mStart with 'tensorboard --logdir runs/train-seg', view at http://localhost:6006/\n",
+ "\n",
+ "Dataset not found ⚠️, missing paths ['/content/datasets/coco128-seg/images/train2017']\n",
+ "Downloading https://ultralytics.com/assets/coco128-seg.zip to coco128-seg.zip...\n",
+ "100% 6.79M/6.79M [00:01<00:00, 6.73MB/s]\n",
+ "Dataset download success ✅ (1.9s), saved to \u001b[1m/content/datasets\u001b[0m\n",
+ "\n",
+ " from n params module arguments \n",
+ " 0 -1 1 3520 models.common.Conv [3, 32, 6, 2, 2] \n",
+ " 1 -1 1 18560 models.common.Conv [32, 64, 3, 2] \n",
+ " 2 -1 1 18816 models.common.C3 [64, 64, 1] \n",
+ " 3 -1 1 73984 models.common.Conv [64, 128, 3, 2] \n",
+ " 4 -1 2 115712 models.common.C3 [128, 128, 2] \n",
+ " 5 -1 1 295424 models.common.Conv [128, 256, 3, 2] \n",
+ " 6 -1 3 625152 models.common.C3 [256, 256, 3] \n",
+ " 7 -1 1 1180672 models.common.Conv [256, 512, 3, 2] \n",
+ " 8 -1 1 1182720 models.common.C3 [512, 512, 1] \n",
+ " 9 -1 1 656896 models.common.SPPF [512, 512, 5] \n",
+ " 10 -1 1 131584 models.common.Conv [512, 256, 1, 1] \n",
+ " 11 -1 1 0 torch.nn.modules.upsampling.Upsample [None, 2, 'nearest'] \n",
+ " 12 [-1, 6] 1 0 models.common.Concat [1] \n",
+ " 13 -1 1 361984 models.common.C3 [512, 256, 1, False] \n",
+ " 14 -1 1 33024 models.common.Conv [256, 128, 1, 1] \n",
+ " 15 -1 1 0 torch.nn.modules.upsampling.Upsample [None, 2, 'nearest'] \n",
+ " 16 [-1, 4] 1 0 models.common.Concat [1] \n",
+ " 17 -1 1 90880 models.common.C3 [256, 128, 1, False] \n",
+ " 18 -1 1 147712 models.common.Conv [128, 128, 3, 2] \n",
+ " 19 [-1, 14] 1 0 models.common.Concat [1] \n",
+ " 20 -1 1 296448 models.common.C3 [256, 256, 1, False] \n",
+ " 21 -1 1 590336 models.common.Conv [256, 256, 3, 2] \n",
+ " 22 [-1, 10] 1 0 models.common.Concat [1] \n",
+ " 23 -1 1 1182720 models.common.C3 [512, 512, 1, False] \n",
+ " 24 [17, 20, 23] 1 615133 models.yolo.Segment [80, [[10, 13, 16, 30, 33, 23], [30, 61, 62, 45, 59, 119], [116, 90, 156, 198, 373, 326]], 32, 128, [128, 256, 512]]\n",
+ "Model summary: 225 layers, 7621277 parameters, 7621277 gradients, 26.6 GFLOPs\n",
+ "\n",
+ "Transferred 367/367 items from yolov5s-seg.pt\n",
+ "\u001b[34m\u001b[1mAMP: \u001b[0mchecks passed ✅\n",
+ "\u001b[34m\u001b[1moptimizer:\u001b[0m SGD(lr=0.01) with parameter groups 60 weight(decay=0.0), 63 weight(decay=0.0005), 63 bias\n",
+ "\u001b[34m\u001b[1malbumentations: \u001b[0mBlur(p=0.01, blur_limit=(3, 7)), MedianBlur(p=0.01, blur_limit=(3, 7)), ToGray(p=0.01), CLAHE(p=0.01, clip_limit=(1, 4.0), tile_grid_size=(8, 8))\n",
+ "\u001b[34m\u001b[1mtrain: \u001b[0mScanning /content/datasets/coco128-seg/labels/train2017... 126 images, 2 backgrounds, 0 corrupt: 100% 128/128 [00:00<00:00, 1389.59it/s]\n",
+ "\u001b[34m\u001b[1mtrain: \u001b[0mNew cache created: /content/datasets/coco128-seg/labels/train2017.cache\n",
+ "\u001b[34m\u001b[1mtrain: \u001b[0mCaching images (0.1GB ram): 100% 128/128 [00:00<00:00, 238.86it/s]\n",
+ "\u001b[34m\u001b[1mval: \u001b[0mScanning /content/datasets/coco128-seg/labels/train2017.cache... 126 images, 2 backgrounds, 0 corrupt: 100% 128/128 [00:00, ?it/s]\n",
+ "\u001b[34m\u001b[1mval: \u001b[0mCaching images (0.1GB ram): 100% 128/128 [00:01<00:00, 98.90it/s]\n",
+ "\n",
+ "\u001b[34m\u001b[1mAutoAnchor: \u001b[0m4.27 anchors/target, 0.994 Best Possible Recall (BPR). Current anchors are a good fit to dataset ✅\n",
+ "Plotting labels to runs/train-seg/exp/labels.jpg... \n",
+ "Image sizes 640 train, 640 val\n",
+ "Using 2 dataloader workers\n",
+ "Logging results to \u001b[1mruns/train-seg/exp\u001b[0m\n",
+ "Starting training for 3 epochs...\n",
+ "\n",
+ " Epoch GPU_mem box_loss seg_loss obj_loss cls_loss Instances Size\n",
+ " 0/2 4.92G 0.0417 0.04646 0.06066 0.02126 192 640: 100% 8/8 [00:08<00:00, 1.10s/it]\n",
+ " Class Images Instances Box(P R mAP50 mAP50-95) Mask(P R mAP50 mAP50-95): 100% 4/4 [00:02<00:00, 1.81it/s]\n",
+ " all 128 929 0.737 0.649 0.715 0.492 0.719 0.617 0.658 0.408\n",
+ "\n",
+ " Epoch GPU_mem box_loss seg_loss obj_loss cls_loss Instances Size\n",
+ " 1/2 6.29G 0.04157 0.04503 0.05772 0.01777 208 640: 100% 8/8 [00:09<00:00, 1.21s/it]\n",
+ " Class Images Instances Box(P R mAP50 mAP50-95) Mask(P R mAP50 mAP50-95): 100% 4/4 [00:02<00:00, 1.87it/s]\n",
+ " all 128 929 0.756 0.674 0.738 0.506 0.725 0.64 0.68 0.422\n",
+ "\n",
+ " Epoch GPU_mem box_loss seg_loss obj_loss cls_loss Instances Size\n",
+ " 2/2 6.29G 0.0425 0.04793 0.06784 0.01863 161 640: 100% 8/8 [00:03<00:00, 2.02it/s]\n",
+ " Class Images Instances Box(P R mAP50 mAP50-95) Mask(P R mAP50 mAP50-95): 100% 4/4 [00:02<00:00, 1.88it/s]\n",
+ " all 128 929 0.736 0.694 0.747 0.522 0.769 0.622 0.683 0.427\n",
+ "\n",
+ "3 epochs completed in 0.009 hours.\n",
+ "Optimizer stripped from runs/train-seg/exp/weights/last.pt, 15.6MB\n",
+ "Optimizer stripped from runs/train-seg/exp/weights/best.pt, 15.6MB\n",
+ "\n",
+ "Validating runs/train-seg/exp/weights/best.pt...\n",
+ "Fusing layers... \n",
+ "Model summary: 165 layers, 7611485 parameters, 0 gradients, 26.4 GFLOPs\n",
+ " Class Images Instances Box(P R mAP50 mAP50-95) Mask(P R mAP50 mAP50-95): 100% 4/4 [00:06<00:00, 1.59s/it]\n",
+ " all 128 929 0.738 0.694 0.746 0.522 0.759 0.625 0.682 0.426\n",
+ " person 128 254 0.845 0.756 0.836 0.55 0.861 0.669 0.759 0.407\n",
+ " bicycle 128 6 0.475 0.333 0.549 0.341 0.711 0.333 0.526 0.322\n",
+ " car 128 46 0.612 0.565 0.539 0.257 0.555 0.435 0.477 0.171\n",
+ " motorcycle 128 5 0.73 0.8 0.752 0.571 0.747 0.8 0.752 0.42\n",
+ " airplane 128 6 1 0.943 0.995 0.732 0.92 0.833 0.839 0.555\n",
+ " bus 128 7 0.677 0.714 0.722 0.653 0.711 0.714 0.722 0.593\n",
+ " train 128 3 1 0.951 0.995 0.551 1 0.884 0.995 0.781\n",
+ " truck 128 12 0.555 0.417 0.457 0.285 0.624 0.417 0.397 0.277\n",
+ " boat 128 6 0.624 0.5 0.584 0.186 1 0.326 0.412 0.133\n",
+ " traffic light 128 14 0.513 0.302 0.411 0.247 0.435 0.214 0.376 0.251\n",
+ " stop sign 128 2 0.824 1 0.995 0.796 0.906 1 0.995 0.747\n",
+ " bench 128 9 0.75 0.667 0.763 0.367 0.724 0.585 0.698 0.209\n",
+ " bird 128 16 0.961 1 0.995 0.686 0.918 0.938 0.91 0.525\n",
+ " cat 128 4 0.771 0.857 0.945 0.752 0.76 0.8 0.945 0.728\n",
+ " dog 128 9 0.987 0.778 0.963 0.681 1 0.705 0.89 0.574\n",
+ " horse 128 2 0.703 1 0.995 0.697 0.759 1 0.995 0.249\n",
+ " elephant 128 17 0.916 0.882 0.93 0.691 0.811 0.765 0.829 0.537\n",
+ " bear 128 1 0.664 1 0.995 0.995 0.701 1 0.995 0.895\n",
+ " zebra 128 4 0.864 1 0.995 0.921 0.879 1 0.995 0.804\n",
+ " giraffe 128 9 0.883 0.889 0.94 0.683 0.845 0.778 0.78 0.463\n",
+ " backpack 128 6 1 0.59 0.701 0.372 1 0.474 0.52 0.252\n",
+ " umbrella 128 18 0.654 0.839 0.887 0.52 0.517 0.556 0.427 0.229\n",
+ " handbag 128 19 0.54 0.211 0.408 0.221 0.796 0.206 0.396 0.196\n",
+ " tie 128 7 0.864 0.857 0.857 0.577 0.925 0.857 0.857 0.534\n",
+ " suitcase 128 4 0.716 1 0.945 0.647 0.767 1 0.945 0.634\n",
+ " frisbee 128 5 0.708 0.8 0.761 0.643 0.737 0.8 0.761 0.501\n",
+ " skis 128 1 0.691 1 0.995 0.796 0.761 1 0.995 0.199\n",
+ " snowboard 128 7 0.918 0.857 0.904 0.604 0.32 0.286 0.235 0.137\n",
+ " sports ball 128 6 0.902 0.667 0.701 0.466 0.727 0.5 0.497 0.471\n",
+ " kite 128 10 0.586 0.4 0.511 0.231 0.663 0.394 0.417 0.139\n",
+ " baseball bat 128 4 0.359 0.5 0.401 0.169 0.631 0.5 0.526 0.133\n",
+ " baseball glove 128 7 1 0.519 0.58 0.327 0.687 0.286 0.455 0.328\n",
+ " skateboard 128 5 0.729 0.8 0.862 0.631 0.599 0.6 0.604 0.379\n",
+ " tennis racket 128 7 0.57 0.714 0.645 0.448 0.608 0.714 0.645 0.412\n",
+ " bottle 128 18 0.469 0.393 0.537 0.357 0.661 0.389 0.543 0.349\n",
+ " wine glass 128 16 0.677 0.938 0.866 0.441 0.53 0.625 0.67 0.334\n",
+ " cup 128 36 0.777 0.722 0.812 0.466 0.725 0.583 0.762 0.467\n",
+ " fork 128 6 0.948 0.333 0.425 0.27 0.527 0.167 0.18 0.102\n",
+ " knife 128 16 0.757 0.587 0.669 0.458 0.79 0.5 0.552 0.34\n",
+ " spoon 128 22 0.74 0.364 0.559 0.269 0.925 0.364 0.513 0.213\n",
+ " bowl 128 28 0.766 0.714 0.725 0.559 0.803 0.584 0.665 0.353\n",
+ " banana 128 1 0.408 1 0.995 0.398 0.539 1 0.995 0.497\n",
+ " sandwich 128 2 1 0 0.695 0.536 1 0 0.498 0.448\n",
+ " orange 128 4 0.467 1 0.995 0.693 0.518 1 0.995 0.663\n",
+ " broccoli 128 11 0.462 0.455 0.383 0.259 0.548 0.455 0.384 0.256\n",
+ " carrot 128 24 0.631 0.875 0.77 0.533 0.757 0.909 0.853 0.499\n",
+ " hot dog 128 2 0.555 1 0.995 0.995 0.578 1 0.995 0.796\n",
+ " pizza 128 5 0.89 0.8 0.962 0.796 1 0.778 0.962 0.766\n",
+ " donut 128 14 0.695 1 0.893 0.772 0.704 1 0.893 0.696\n",
+ " cake 128 4 0.826 1 0.995 0.92 0.862 1 0.995 0.846\n",
+ " chair 128 35 0.53 0.571 0.613 0.336 0.67 0.6 0.538 0.271\n",
+ " couch 128 6 0.972 0.667 0.833 0.627 1 0.62 0.696 0.394\n",
+ " potted plant 128 14 0.7 0.857 0.883 0.552 0.836 0.857 0.883 0.473\n",
+ " bed 128 3 0.979 0.667 0.83 0.366 1 0 0.83 0.373\n",
+ " dining table 128 13 0.775 0.308 0.505 0.364 0.644 0.231 0.25 0.0804\n",
+ " toilet 128 2 0.836 1 0.995 0.846 0.887 1 0.995 0.797\n",
+ " tv 128 2 0.6 1 0.995 0.846 0.655 1 0.995 0.896\n",
+ " laptop 128 3 0.822 0.333 0.445 0.307 1 0 0.392 0.12\n",
+ " mouse 128 2 1 0 0 0 1 0 0 0\n",
+ " remote 128 8 0.745 0.5 0.62 0.459 0.821 0.5 0.624 0.449\n",
+ " cell phone 128 8 0.686 0.375 0.502 0.272 0.488 0.25 0.28 0.132\n",
+ " microwave 128 3 0.831 1 0.995 0.722 0.867 1 0.995 0.592\n",
+ " oven 128 5 0.439 0.4 0.435 0.294 0.823 0.6 0.645 0.418\n",
+ " sink 128 6 0.677 0.5 0.565 0.448 0.722 0.5 0.46 0.362\n",
+ " refrigerator 128 5 0.533 0.8 0.783 0.524 0.558 0.8 0.783 0.527\n",
+ " book 128 29 0.732 0.379 0.423 0.196 0.69 0.207 0.38 0.131\n",
+ " clock 128 9 0.889 0.778 0.917 0.677 0.908 0.778 0.875 0.604\n",
+ " vase 128 2 0.375 1 0.995 0.995 0.455 1 0.995 0.796\n",
+ " scissors 128 1 1 0 0.0166 0.00166 1 0 0 0\n",
+ " teddy bear 128 21 0.813 0.829 0.841 0.457 0.826 0.678 0.786 0.422\n",
+ " toothbrush 128 5 0.806 1 0.995 0.733 0.991 1 0.995 0.628\n",
+ "Results saved to \u001b[1mruns/train-seg/exp\u001b[0m\n"
+ ]
+ }
+ ],
+ "source": [
+ "# Train YOLOv5s on COCO128 for 3 epochs\n",
+ "!python segment/train.py --img 640 --batch 16 --epochs 3 --data coco128-seg.yaml --weights yolov5s-seg.pt --cache"
+ ]
+ },
+ {
+ "cell_type": "markdown",
+ "metadata": {
+ "id": "15glLzbQx5u0"
+ },
+ "source": [
+ "# 4. Visualize"
+ ]
+ },
+ {
+ "cell_type": "markdown",
+ "metadata": {
+ "id": "nWOsI5wJR1o3"
+ },
+ "source": [
+ "## Comet Logging and Visualization 🌟 NEW\n",
+ "\n",
+ "[Comet](https://www.comet.com/site/lp/yolov5-with-comet/?utm_source=yolov5&utm_medium=partner&utm_campaign=partner_yolov5_2022&utm_content=yolov5_colab) is now fully integrated with YOLOv5. Track and visualize model metrics in real time, save your hyperparameters, datasets, and model checkpoints, and visualize your model predictions with [Comet Custom Panels](https://www.comet.com/docs/v2/guides/comet-dashboard/code-panels/about-panels/?utm_source=yolov5&utm_medium=partner&utm_campaign=partner_yolov5_2022&utm_content=yolov5_colab)! Comet makes sure you never lose track of your work and makes it easy to share results and collaborate across teams of all sizes!\n",
+ "\n",
+ "Getting started is easy:\n",
+ "```shell\n",
+ "pip install comet_ml # 1. install\n",
+ "export COMET_API_KEY= # 2. paste API key\n",
+ "python train.py --img 640 --epochs 3 --data coco128.yaml --weights yolov5s.pt # 3. train\n",
+ "```\n",
+ "To learn more about all of the supported Comet features for this integration, check out the [Comet Tutorial](https://docs.ultralytics.com/yolov5/tutorials/comet_logging_integration). If you'd like to learn more about Comet, head over to our [documentation](https://www.comet.com/docs/v2/?utm_source=yolov5&utm_medium=partner&utm_campaign=partner_yolov5_2022&utm_content=yolov5_colab). Get started by trying out the Comet Colab Notebook:\n",
+ "[](https://colab.research.google.com/drive/1RG0WOQyxlDlo5Km8GogJpIEJlg_5lyYO?usp=sharing)\n",
+ "\n",
+ "\n",
+ ""
+ ]
+ },
+ {
+ "cell_type": "markdown",
+ "metadata": {
+ "id": "Lay2WsTjNJzP"
+ },
+ "source": [
+ "## ClearML Logging and Automation 🌟 NEW\n",
+ "\n",
+ "[ClearML](https://cutt.ly/yolov5-notebook-clearml) is completely integrated into YOLOv5 to track your experimentation, manage dataset versions and even remotely execute training runs. To enable ClearML (check cells above):\n",
+ "\n",
+ "- `pip install clearml`\n",
+ "- run `clearml-init` to connect to a ClearML server (**deploy your own [open-source server](https://github.com/allegroai/clearml-server)**, or use our [free hosted server](https://cutt.ly/yolov5-notebook-clearml))\n",
+ "\n",
+ "You'll get all the great expected features from an experiment manager: live updates, model upload, experiment comparison etc. but ClearML also tracks uncommitted changes and installed packages for example. Thanks to that ClearML Tasks (which is what we call experiments) are also reproducible on different machines! With only 1 extra line, we can schedule a YOLOv5 training task on a queue to be executed by any number of ClearML Agents (workers).\n",
+ "\n",
+ "You can use ClearML Data to version your dataset and then pass it to YOLOv5 simply using its unique ID. This will help you keep track of your data without adding extra hassle. Explore the [ClearML Tutorial](https://docs.ultralytics.com/yolov5/tutorials/clearml_logging_integration) for details!\n",
+ "\n",
+ "\n",
+ ""
+ ]
+ },
+ {
+ "cell_type": "markdown",
+ "metadata": {
+ "id": "-WPvRbS5Swl6"
+ },
+ "source": [
+ "## Local Logging\n",
+ "\n",
+ "Training results are automatically logged with [Tensorboard](https://www.tensorflow.org/tensorboard) and [CSV](https://github.com/ultralytics/yolov5/pull/4148) loggers to `runs/train`, with a new experiment directory created for each new training as `runs/train/exp2`, `runs/train/exp3`, etc.\n",
+ "\n",
+ "This directory contains train and val statistics, mosaics, labels, predictions and augmentated mosaics, as well as metrics and charts including precision-recall (PR) curves and confusion matrices. \n",
+ "\n",
+ "\n"
+ ]
+ },
+ {
+ "cell_type": "markdown",
+ "metadata": {
+ "id": "Zelyeqbyt3GD"
+ },
+ "source": [
+ "# Environments\n",
+ "\n",
+ "YOLOv5 may be run in any of the following up-to-date verified environments (with all dependencies including [CUDA](https://developer.nvidia.com/cuda)/[CUDNN](https://developer.nvidia.com/cudnn), [Python](https://www.python.org/) and [PyTorch](https://pytorch.org/) preinstalled):\n",
+ "\n",
+ "- **Notebooks** with free GPU: \n",
+ "- **Google Cloud** Deep Learning VM. See [GCP Quickstart Guide](https://docs.ultralytics.com/yolov5/environments/google_cloud_quickstart_tutorial/)\n",
+ "- **Amazon** Deep Learning AMI. See [AWS Quickstart Guide](https://docs.ultralytics.com/yolov5/environments/aws_quickstart_tutorial/)\n",
+ "- **Docker Image**. See [Docker Quickstart Guide](https://docs.ultralytics.com/yolov5/environments/docker_image_quickstart_tutorial/) \n"
+ ]
+ },
+ {
+ "cell_type": "markdown",
+ "metadata": {
+ "id": "6Qu7Iesl0p54"
+ },
+ "source": [
+ "# Status\n",
+ "\n",
+ "\n",
+ "\n",
+ "If this badge is green, all [YOLOv5 GitHub Actions](https://github.com/ultralytics/yolov5/actions) Continuous Integration (CI) tests are currently passing. CI tests verify correct operation of YOLOv5 training ([train.py](https://github.com/ultralytics/yolov5/blob/master/train.py)), testing ([val.py](https://github.com/ultralytics/yolov5/blob/master/val.py)), inference ([detect.py](https://github.com/ultralytics/yolov5/blob/master/detect.py)) and export ([export.py](https://github.com/ultralytics/yolov5/blob/master/export.py)) on macOS, Windows, and Ubuntu every 24 hours and on every commit.\n"
+ ]
+ },
+ {
+ "cell_type": "markdown",
+ "metadata": {
+ "id": "IEijrePND_2I"
+ },
+ "source": [
+ "# Appendix\n",
+ "\n",
+ "Additional content below."
+ ]
+ },
+ {
+ "cell_type": "code",
+ "execution_count": null,
+ "metadata": {
+ "id": "GMusP4OAxFu6"
+ },
+ "outputs": [],
+ "source": [
+ "# YOLOv5 PyTorch HUB Inference (DetectionModels only)\n",
+ "import torch\n",
+ "\n",
+ "model = torch.hub.load('ultralytics/yolov5', 'yolov5s-seg') # yolov5n - yolov5x6 or custom\n",
+ "im = 'https://ultralytics.com/images/zidane.jpg' # file, Path, PIL.Image, OpenCV, nparray, list\n",
+ "results = model(im) # inference\n",
+ "results.print() # or .show(), .save(), .crop(), .pandas(), etc."
+ ]
+ }
+ ],
+ "metadata": {
+ "accelerator": "GPU",
+ "colab": {
+ "name": "YOLOv5 Segmentation Tutorial",
+ "provenance": [],
+ "toc_visible": true
+ },
+ "kernelspec": {
+ "display_name": "Python 3 (ipykernel)",
+ "language": "python",
+ "name": "python3"
+ },
+ "language_info": {
+ "codemirror_mode": {
+ "name": "ipython",
+ "version": 3
+ },
+ "file_extension": ".py",
+ "mimetype": "text/x-python",
+ "name": "python",
+ "nbconvert_exporter": "python",
+ "pygments_lexer": "ipython3",
+ "version": "3.7.12"
+ }
+ },
+ "nbformat": 4,
+ "nbformat_minor": 0
+}
diff --git a/yolov5/segment/val.py b/yolov5/segment/val.py
new file mode 100644
index 0000000000000000000000000000000000000000..c0575fd59a91632af67082bd561d9b4171df50e5
--- /dev/null
+++ b/yolov5/segment/val.py
@@ -0,0 +1,473 @@
+# YOLOv5 🚀 by Ultralytics, AGPL-3.0 license
+"""
+Validate a trained YOLOv5 segment model on a segment dataset
+
+Usage:
+ $ bash data/scripts/get_coco.sh --val --segments # download COCO-segments val split (1G, 5000 images)
+ $ python segment/val.py --weights yolov5s-seg.pt --data coco.yaml --img 640 # validate COCO-segments
+
+Usage - formats:
+ $ python segment/val.py --weights yolov5s-seg.pt # PyTorch
+ yolov5s-seg.torchscript # TorchScript
+ yolov5s-seg.onnx # ONNX Runtime or OpenCV DNN with --dnn
+ yolov5s-seg_openvino_label # OpenVINO
+ yolov5s-seg.engine # TensorRT
+ yolov5s-seg.mlmodel # CoreML (macOS-only)
+ yolov5s-seg_saved_model # TensorFlow SavedModel
+ yolov5s-seg.pb # TensorFlow GraphDef
+ yolov5s-seg.tflite # TensorFlow Lite
+ yolov5s-seg_edgetpu.tflite # TensorFlow Edge TPU
+ yolov5s-seg_paddle_model # PaddlePaddle
+"""
+
+import argparse
+import json
+import os
+import subprocess
+import sys
+from multiprocessing.pool import ThreadPool
+from pathlib import Path
+
+import numpy as np
+import torch
+from tqdm import tqdm
+
+FILE = Path(__file__).resolve()
+ROOT = FILE.parents[1] # YOLOv5 root directory
+if str(ROOT) not in sys.path:
+ sys.path.append(str(ROOT)) # add ROOT to PATH
+ROOT = Path(os.path.relpath(ROOT, Path.cwd())) # relative
+
+import torch.nn.functional as F
+
+from models.common import DetectMultiBackend
+from models.yolo import SegmentationModel
+from utils.callbacks import Callbacks
+from utils.general import (LOGGER, NUM_THREADS, TQDM_BAR_FORMAT, Profile, check_dataset, check_img_size,
+ check_requirements, check_yaml, coco80_to_coco91_class, colorstr, increment_path,
+ non_max_suppression, print_args, scale_boxes, xywh2xyxy, xyxy2xywh)
+from utils.metrics import ConfusionMatrix, box_iou
+from utils.plots import output_to_target, plot_val_study
+from utils.segment.dataloaders import create_dataloader
+from utils.segment.general import mask_iou, process_mask, process_mask_native, scale_image
+from utils.segment.metrics import Metrics, ap_per_class_box_and_mask
+from utils.segment.plots import plot_images_and_masks
+from utils.torch_utils import de_parallel, select_device, smart_inference_mode
+
+
+def save_one_txt(predn, save_conf, shape, file):
+ # Save one txt result
+ gn = torch.tensor(shape)[[1, 0, 1, 0]] # normalization gain whwh
+ for *xyxy, conf, cls in predn.tolist():
+ xywh = (xyxy2xywh(torch.tensor(xyxy).view(1, 4)) / gn).view(-1).tolist() # normalized xywh
+ line = (cls, *xywh, conf) if save_conf else (cls, *xywh) # label format
+ with open(file, 'a') as f:
+ f.write(('%g ' * len(line)).rstrip() % line + '\n')
+
+
+def save_one_json(predn, jdict, path, class_map, pred_masks):
+ # Save one JSON result {"image_id": 42, "category_id": 18, "bbox": [258.15, 41.29, 348.26, 243.78], "score": 0.236}
+ from pycocotools.mask import encode
+
+ def single_encode(x):
+ rle = encode(np.asarray(x[:, :, None], order='F', dtype='uint8'))[0]
+ rle['counts'] = rle['counts'].decode('utf-8')
+ return rle
+
+ image_id = int(path.stem) if path.stem.isnumeric() else path.stem
+ box = xyxy2xywh(predn[:, :4]) # xywh
+ box[:, :2] -= box[:, 2:] / 2 # xy center to top-left corner
+ pred_masks = np.transpose(pred_masks, (2, 0, 1))
+ with ThreadPool(NUM_THREADS) as pool:
+ rles = pool.map(single_encode, pred_masks)
+ for i, (p, b) in enumerate(zip(predn.tolist(), box.tolist())):
+ jdict.append({
+ 'image_id': image_id,
+ 'category_id': class_map[int(p[5])],
+ 'bbox': [round(x, 3) for x in b],
+ 'score': round(p[4], 5),
+ 'segmentation': rles[i]})
+
+
+def process_batch(detections, labels, iouv, pred_masks=None, gt_masks=None, overlap=False, masks=False):
+ """
+ Return correct prediction matrix
+ Arguments:
+ detections (array[N, 6]), x1, y1, x2, y2, conf, class
+ labels (array[M, 5]), class, x1, y1, x2, y2
+ Returns:
+ correct (array[N, 10]), for 10 IoU levels
+ """
+ if masks:
+ if overlap:
+ nl = len(labels)
+ index = torch.arange(nl, device=gt_masks.device).view(nl, 1, 1) + 1
+ gt_masks = gt_masks.repeat(nl, 1, 1) # shape(1,640,640) -> (n,640,640)
+ gt_masks = torch.where(gt_masks == index, 1.0, 0.0)
+ if gt_masks.shape[1:] != pred_masks.shape[1:]:
+ gt_masks = F.interpolate(gt_masks[None], pred_masks.shape[1:], mode='bilinear', align_corners=False)[0]
+ gt_masks = gt_masks.gt_(0.5)
+ iou = mask_iou(gt_masks.view(gt_masks.shape[0], -1), pred_masks.view(pred_masks.shape[0], -1))
+ else: # boxes
+ iou = box_iou(labels[:, 1:], detections[:, :4])
+
+ correct = np.zeros((detections.shape[0], iouv.shape[0])).astype(bool)
+ correct_class = labels[:, 0:1] == detections[:, 5]
+ for i in range(len(iouv)):
+ x = torch.where((iou >= iouv[i]) & correct_class) # IoU > threshold and classes match
+ if x[0].shape[0]:
+ matches = torch.cat((torch.stack(x, 1), iou[x[0], x[1]][:, None]), 1).cpu().numpy() # [label, detect, iou]
+ if x[0].shape[0] > 1:
+ matches = matches[matches[:, 2].argsort()[::-1]]
+ matches = matches[np.unique(matches[:, 1], return_index=True)[1]]
+ # matches = matches[matches[:, 2].argsort()[::-1]]
+ matches = matches[np.unique(matches[:, 0], return_index=True)[1]]
+ correct[matches[:, 1].astype(int), i] = True
+ return torch.tensor(correct, dtype=torch.bool, device=iouv.device)
+
+
+@smart_inference_mode()
+def run(
+ data,
+ weights=None, # model.pt path(s)
+ batch_size=32, # batch size
+ imgsz=640, # inference size (pixels)
+ conf_thres=0.001, # confidence threshold
+ iou_thres=0.6, # NMS IoU threshold
+ max_det=300, # maximum detections per image
+ task='val', # train, val, test, speed or study
+ device='', # cuda device, i.e. 0 or 0,1,2,3 or cpu
+ workers=8, # max dataloader workers (per RANK in DDP mode)
+ single_cls=False, # treat as single-class dataset
+ augment=False, # augmented inference
+ verbose=False, # verbose output
+ save_txt=False, # save results to *.txt
+ save_hybrid=False, # save label+prediction hybrid results to *.txt
+ save_conf=False, # save confidences in --save-txt labels
+ save_json=False, # save a COCO-JSON results file
+ project=ROOT / 'runs/val-seg', # save to project/name
+ name='exp', # save to project/name
+ exist_ok=False, # existing project/name ok, do not increment
+ half=True, # use FP16 half-precision inference
+ dnn=False, # use OpenCV DNN for ONNX inference
+ model=None,
+ dataloader=None,
+ save_dir=Path(''),
+ plots=True,
+ overlap=False,
+ mask_downsample_ratio=1,
+ compute_loss=None,
+ callbacks=Callbacks(),
+):
+ if save_json:
+ check_requirements('pycocotools>=2.0.6')
+ process = process_mask_native # more accurate
+ else:
+ process = process_mask # faster
+
+ # Initialize/load model and set device
+ training = model is not None
+ if training: # called by train.py
+ device, pt, jit, engine = next(model.parameters()).device, True, False, False # get model device, PyTorch model
+ half &= device.type != 'cpu' # half precision only supported on CUDA
+ model.half() if half else model.float()
+ nm = de_parallel(model).model[-1].nm # number of masks
+ else: # called directly
+ device = select_device(device, batch_size=batch_size)
+
+ # Directories
+ save_dir = increment_path(Path(project) / name, exist_ok=exist_ok) # increment run
+ (save_dir / 'labels' if save_txt else save_dir).mkdir(parents=True, exist_ok=True) # make dir
+
+ # Load model
+ model = DetectMultiBackend(weights, device=device, dnn=dnn, data=data, fp16=half)
+ stride, pt, jit, engine = model.stride, model.pt, model.jit, model.engine
+ imgsz = check_img_size(imgsz, s=stride) # check image size
+ half = model.fp16 # FP16 supported on limited backends with CUDA
+ nm = de_parallel(model).model.model[-1].nm if isinstance(model, SegmentationModel) else 32 # number of masks
+ if engine:
+ batch_size = model.batch_size
+ else:
+ device = model.device
+ if not (pt or jit):
+ batch_size = 1 # export.py models default to batch-size 1
+ LOGGER.info(f'Forcing --batch-size 1 square inference (1,3,{imgsz},{imgsz}) for non-PyTorch models')
+
+ # Data
+ data = check_dataset(data) # check
+
+ # Configure
+ model.eval()
+ cuda = device.type != 'cpu'
+ is_coco = isinstance(data.get('val'), str) and data['val'].endswith(f'coco{os.sep}val2017.txt') # COCO dataset
+ nc = 1 if single_cls else int(data['nc']) # number of classes
+ iouv = torch.linspace(0.5, 0.95, 10, device=device) # iou vector for mAP@0.5:0.95
+ niou = iouv.numel()
+
+ # Dataloader
+ if not training:
+ if pt and not single_cls: # check --weights are trained on --data
+ ncm = model.model.nc
+ assert ncm == nc, f'{weights} ({ncm} classes) trained on different --data than what you passed ({nc} ' \
+ f'classes). Pass correct combination of --weights and --data that are trained together.'
+ model.warmup(imgsz=(1 if pt else batch_size, 3, imgsz, imgsz)) # warmup
+ pad, rect = (0.0, False) if task == 'speed' else (0.5, pt) # square inference for benchmarks
+ task = task if task in ('train', 'val', 'test') else 'val' # path to train/val/test images
+ dataloader = create_dataloader(data[task],
+ imgsz,
+ batch_size,
+ stride,
+ single_cls,
+ pad=pad,
+ rect=rect,
+ workers=workers,
+ prefix=colorstr(f'{task}: '),
+ overlap_mask=overlap,
+ mask_downsample_ratio=mask_downsample_ratio)[0]
+
+ seen = 0
+ confusion_matrix = ConfusionMatrix(nc=nc)
+ names = model.names if hasattr(model, 'names') else model.module.names # get class names
+ if isinstance(names, (list, tuple)): # old format
+ names = dict(enumerate(names))
+ class_map = coco80_to_coco91_class() if is_coco else list(range(1000))
+ s = ('%22s' + '%11s' * 10) % ('Class', 'Images', 'Instances', 'Box(P', 'R', 'mAP50', 'mAP50-95)', 'Mask(P', 'R',
+ 'mAP50', 'mAP50-95)')
+ dt = Profile(), Profile(), Profile()
+ metrics = Metrics()
+ loss = torch.zeros(4, device=device)
+ jdict, stats = [], []
+ # callbacks.run('on_val_start')
+ pbar = tqdm(dataloader, desc=s, bar_format=TQDM_BAR_FORMAT) # progress bar
+ for batch_i, (im, targets, paths, shapes, masks) in enumerate(pbar):
+ # callbacks.run('on_val_batch_start')
+ with dt[0]:
+ if cuda:
+ im = im.to(device, non_blocking=True)
+ targets = targets.to(device)
+ masks = masks.to(device)
+ masks = masks.float()
+ im = im.half() if half else im.float() # uint8 to fp16/32
+ im /= 255 # 0 - 255 to 0.0 - 1.0
+ nb, _, height, width = im.shape # batch size, channels, height, width
+
+ # Inference
+ with dt[1]:
+ preds, protos, train_out = model(im) if compute_loss else (*model(im, augment=augment)[:2], None)
+
+ # Loss
+ if compute_loss:
+ loss += compute_loss((train_out, protos), targets, masks)[1] # box, obj, cls
+
+ # NMS
+ targets[:, 2:] *= torch.tensor((width, height, width, height), device=device) # to pixels
+ lb = [targets[targets[:, 0] == i, 1:] for i in range(nb)] if save_hybrid else [] # for autolabelling
+ with dt[2]:
+ preds = non_max_suppression(preds,
+ conf_thres,
+ iou_thres,
+ labels=lb,
+ multi_label=True,
+ agnostic=single_cls,
+ max_det=max_det,
+ nm=nm)
+
+ # Metrics
+ plot_masks = [] # masks for plotting
+ for si, (pred, proto) in enumerate(zip(preds, protos)):
+ labels = targets[targets[:, 0] == si, 1:]
+ nl, npr = labels.shape[0], pred.shape[0] # number of labels, predictions
+ path, shape = Path(paths[si]), shapes[si][0]
+ correct_masks = torch.zeros(npr, niou, dtype=torch.bool, device=device) # init
+ correct_bboxes = torch.zeros(npr, niou, dtype=torch.bool, device=device) # init
+ seen += 1
+
+ if npr == 0:
+ if nl:
+ stats.append((correct_masks, correct_bboxes, *torch.zeros((2, 0), device=device), labels[:, 0]))
+ if plots:
+ confusion_matrix.process_batch(detections=None, labels=labels[:, 0])
+ continue
+
+ # Masks
+ midx = [si] if overlap else targets[:, 0] == si
+ gt_masks = masks[midx]
+ pred_masks = process(proto, pred[:, 6:], pred[:, :4], shape=im[si].shape[1:])
+
+ # Predictions
+ if single_cls:
+ pred[:, 5] = 0
+ predn = pred.clone()
+ scale_boxes(im[si].shape[1:], predn[:, :4], shape, shapes[si][1]) # native-space pred
+
+ # Evaluate
+ if nl:
+ tbox = xywh2xyxy(labels[:, 1:5]) # target boxes
+ scale_boxes(im[si].shape[1:], tbox, shape, shapes[si][1]) # native-space labels
+ labelsn = torch.cat((labels[:, 0:1], tbox), 1) # native-space labels
+ correct_bboxes = process_batch(predn, labelsn, iouv)
+ correct_masks = process_batch(predn, labelsn, iouv, pred_masks, gt_masks, overlap=overlap, masks=True)
+ if plots:
+ confusion_matrix.process_batch(predn, labelsn)
+ stats.append((correct_masks, correct_bboxes, pred[:, 4], pred[:, 5], labels[:, 0])) # (conf, pcls, tcls)
+
+ pred_masks = torch.as_tensor(pred_masks, dtype=torch.uint8)
+ if plots and batch_i < 3:
+ plot_masks.append(pred_masks[:15]) # filter top 15 to plot
+
+ # Save/log
+ if save_txt:
+ save_one_txt(predn, save_conf, shape, file=save_dir / 'labels' / f'{path.stem}.txt')
+ if save_json:
+ pred_masks = scale_image(im[si].shape[1:],
+ pred_masks.permute(1, 2, 0).contiguous().cpu().numpy(), shape, shapes[si][1])
+ save_one_json(predn, jdict, path, class_map, pred_masks) # append to COCO-JSON dictionary
+ # callbacks.run('on_val_image_end', pred, predn, path, names, im[si])
+
+ # Plot images
+ if plots and batch_i < 3:
+ if len(plot_masks):
+ plot_masks = torch.cat(plot_masks, dim=0)
+ plot_images_and_masks(im, targets, masks, paths, save_dir / f'val_batch{batch_i}_labels.jpg', names)
+ plot_images_and_masks(im, output_to_target(preds, max_det=15), plot_masks, paths,
+ save_dir / f'val_batch{batch_i}_pred.jpg', names) # pred
+
+ # callbacks.run('on_val_batch_end')
+
+ # Compute metrics
+ stats = [torch.cat(x, 0).cpu().numpy() for x in zip(*stats)] # to numpy
+ if len(stats) and stats[0].any():
+ results = ap_per_class_box_and_mask(*stats, plot=plots, save_dir=save_dir, names=names)
+ metrics.update(results)
+ nt = np.bincount(stats[4].astype(int), minlength=nc) # number of targets per class
+
+ # Print results
+ pf = '%22s' + '%11i' * 2 + '%11.3g' * 8 # print format
+ LOGGER.info(pf % ('all', seen, nt.sum(), *metrics.mean_results()))
+ if nt.sum() == 0:
+ LOGGER.warning(f'WARNING ⚠️ no labels found in {task} set, can not compute metrics without labels')
+
+ # Print results per class
+ if (verbose or (nc < 50 and not training)) and nc > 1 and len(stats):
+ for i, c in enumerate(metrics.ap_class_index):
+ LOGGER.info(pf % (names[c], seen, nt[c], *metrics.class_result(i)))
+
+ # Print speeds
+ t = tuple(x.t / seen * 1E3 for x in dt) # speeds per image
+ if not training:
+ shape = (batch_size, 3, imgsz, imgsz)
+ LOGGER.info(f'Speed: %.1fms pre-process, %.1fms inference, %.1fms NMS per image at shape {shape}' % t)
+
+ # Plots
+ if plots:
+ confusion_matrix.plot(save_dir=save_dir, names=list(names.values()))
+ # callbacks.run('on_val_end')
+
+ mp_bbox, mr_bbox, map50_bbox, map_bbox, mp_mask, mr_mask, map50_mask, map_mask = metrics.mean_results()
+
+ # Save JSON
+ if save_json and len(jdict):
+ w = Path(weights[0] if isinstance(weights, list) else weights).stem if weights is not None else '' # weights
+ anno_json = str(Path('../datasets/coco/annotations/instances_val2017.json')) # annotations
+ pred_json = str(save_dir / f'{w}_predictions.json') # predictions
+ LOGGER.info(f'\nEvaluating pycocotools mAP... saving {pred_json}...')
+ with open(pred_json, 'w') as f:
+ json.dump(jdict, f)
+
+ try: # https://github.com/cocodataset/cocoapi/blob/master/PythonAPI/pycocoEvalDemo.ipynb
+ from pycocotools.coco import COCO
+ from pycocotools.cocoeval import COCOeval
+
+ anno = COCO(anno_json) # init annotations api
+ pred = anno.loadRes(pred_json) # init predictions api
+ results = []
+ for eval in COCOeval(anno, pred, 'bbox'), COCOeval(anno, pred, 'segm'):
+ if is_coco:
+ eval.params.imgIds = [int(Path(x).stem) for x in dataloader.dataset.im_files] # img ID to evaluate
+ eval.evaluate()
+ eval.accumulate()
+ eval.summarize()
+ results.extend(eval.stats[:2]) # update results (mAP@0.5:0.95, mAP@0.5)
+ map_bbox, map50_bbox, map_mask, map50_mask = results
+ except Exception as e:
+ LOGGER.info(f'pycocotools unable to run: {e}')
+
+ # Return results
+ model.float() # for training
+ if not training:
+ s = f"\n{len(list(save_dir.glob('labels/*.txt')))} labels saved to {save_dir / 'labels'}" if save_txt else ''
+ LOGGER.info(f"Results saved to {colorstr('bold', save_dir)}{s}")
+ final_metric = mp_bbox, mr_bbox, map50_bbox, map_bbox, mp_mask, mr_mask, map50_mask, map_mask
+ return (*final_metric, *(loss.cpu() / len(dataloader)).tolist()), metrics.get_maps(nc), t
+
+
+def parse_opt():
+ parser = argparse.ArgumentParser()
+ parser.add_argument('--data', type=str, default=ROOT / 'data/coco128-seg.yaml', help='dataset.yaml path')
+ parser.add_argument('--weights', nargs='+', type=str, default=ROOT / 'yolov5s-seg.pt', help='model path(s)')
+ parser.add_argument('--batch-size', type=int, default=32, help='batch size')
+ parser.add_argument('--imgsz', '--img', '--img-size', type=int, default=640, help='inference size (pixels)')
+ parser.add_argument('--conf-thres', type=float, default=0.001, help='confidence threshold')
+ parser.add_argument('--iou-thres', type=float, default=0.6, help='NMS IoU threshold')
+ parser.add_argument('--max-det', type=int, default=300, help='maximum detections per image')
+ parser.add_argument('--task', default='val', help='train, val, test, speed or study')
+ parser.add_argument('--device', default='', help='cuda device, i.e. 0 or 0,1,2,3 or cpu')
+ parser.add_argument('--workers', type=int, default=8, help='max dataloader workers (per RANK in DDP mode)')
+ parser.add_argument('--single-cls', action='store_true', help='treat as single-class dataset')
+ parser.add_argument('--augment', action='store_true', help='augmented inference')
+ parser.add_argument('--verbose', action='store_true', help='report mAP by class')
+ parser.add_argument('--save-txt', action='store_true', help='save results to *.txt')
+ parser.add_argument('--save-hybrid', action='store_true', help='save label+prediction hybrid results to *.txt')
+ parser.add_argument('--save-conf', action='store_true', help='save confidences in --save-txt labels')
+ parser.add_argument('--save-json', action='store_true', help='save a COCO-JSON results file')
+ parser.add_argument('--project', default=ROOT / 'runs/val-seg', help='save results to project/name')
+ parser.add_argument('--name', default='exp', help='save to project/name')
+ parser.add_argument('--exist-ok', action='store_true', help='existing project/name ok, do not increment')
+ parser.add_argument('--half', action='store_true', help='use FP16 half-precision inference')
+ parser.add_argument('--dnn', action='store_true', help='use OpenCV DNN for ONNX inference')
+ opt = parser.parse_args()
+ opt.data = check_yaml(opt.data) # check YAML
+ # opt.save_json |= opt.data.endswith('coco.yaml')
+ opt.save_txt |= opt.save_hybrid
+ print_args(vars(opt))
+ return opt
+
+
+def main(opt):
+ check_requirements(requirements=ROOT / 'requirements.txt', exclude=('tensorboard', 'thop'))
+
+ if opt.task in ('train', 'val', 'test'): # run normally
+ if opt.conf_thres > 0.001: # https://github.com/ultralytics/yolov5/issues/1466
+ LOGGER.warning(f'WARNING ⚠️ confidence threshold {opt.conf_thres} > 0.001 produces invalid results')
+ if opt.save_hybrid:
+ LOGGER.warning('WARNING ⚠️ --save-hybrid returns high mAP from hybrid labels, not from predictions alone')
+ run(**vars(opt))
+
+ else:
+ weights = opt.weights if isinstance(opt.weights, list) else [opt.weights]
+ opt.half = torch.cuda.is_available() and opt.device != 'cpu' # FP16 for fastest results
+ if opt.task == 'speed': # speed benchmarks
+ # python val.py --task speed --data coco.yaml --batch 1 --weights yolov5n.pt yolov5s.pt...
+ opt.conf_thres, opt.iou_thres, opt.save_json = 0.25, 0.45, False
+ for opt.weights in weights:
+ run(**vars(opt), plots=False)
+
+ elif opt.task == 'study': # speed vs mAP benchmarks
+ # python val.py --task study --data coco.yaml --iou 0.7 --weights yolov5n.pt yolov5s.pt...
+ for opt.weights in weights:
+ f = f'study_{Path(opt.data).stem}_{Path(opt.weights).stem}.txt' # filename to save to
+ x, y = list(range(256, 1536 + 128, 128)), [] # x axis (image sizes), y axis
+ for opt.imgsz in x: # img-size
+ LOGGER.info(f'\nRunning {f} --imgsz {opt.imgsz}...')
+ r, _, t = run(**vars(opt), plots=False)
+ y.append(r + t) # results and times
+ np.savetxt(f, y, fmt='%10.4g') # save
+ subprocess.run(['zip', '-r', 'study.zip', 'study_*.txt'])
+ plot_val_study(x=x) # plot
+ else:
+ raise NotImplementedError(f'--task {opt.task} not in ("train", "val", "test", "speed", "study")')
+
+
+if __name__ == '__main__':
+ opt = parse_opt()
+ main(opt)
diff --git a/yolov5/setup.cfg b/yolov5/setup.cfg
new file mode 100644
index 0000000000000000000000000000000000000000..d7c4cb3e1a4d34291816835b071ba0d75243d79b
--- /dev/null
+++ b/yolov5/setup.cfg
@@ -0,0 +1,54 @@
+# Project-wide configuration file, can be used for package metadata and other toll configurations
+# Example usage: global configuration for PEP8 (via flake8) setting or default pytest arguments
+# Local usage: pip install pre-commit, pre-commit run --all-files
+
+[metadata]
+license_file = LICENSE
+description_file = README.md
+
+[tool:pytest]
+norecursedirs =
+ .git
+ dist
+ build
+addopts =
+ --doctest-modules
+ --durations=25
+ --color=yes
+
+[flake8]
+max-line-length = 120
+exclude = .tox,*.egg,build,temp
+select = E,W,F
+doctests = True
+verbose = 2
+# https://pep8.readthedocs.io/en/latest/intro.html#error-codes
+format = pylint
+# see: https://www.flake8rules.com/
+ignore = E731,F405,E402,F401,W504,E127,E231,E501,F403
+ # E731: Do not assign a lambda expression, use a def
+ # F405: name may be undefined, or defined from star imports: module
+ # E402: module level import not at top of file
+ # F401: module imported but unused
+ # W504: line break after binary operator
+ # E127: continuation line over-indented for visual indent
+ # E231: missing whitespace after ‘,’, ‘;’, or ‘:’
+ # E501: line too long
+ # F403: ‘from module import *’ used; unable to detect undefined names
+
+[isort]
+# https://pycqa.github.io/isort/docs/configuration/options.html
+line_length = 120
+# see: https://pycqa.github.io/isort/docs/configuration/multi_line_output_modes.html
+multi_line_output = 0
+
+[yapf]
+based_on_style = pep8
+spaces_before_comment = 2
+COLUMN_LIMIT = 120
+COALESCE_BRACKETS = True
+SPACES_AROUND_POWER_OPERATOR = True
+SPACE_BETWEEN_ENDING_COMMA_AND_CLOSING_BRACKET = False
+SPLIT_BEFORE_CLOSING_BRACKET = False
+SPLIT_BEFORE_FIRST_ARGUMENT = False
+# EACH_DICT_ENTRY_ON_SEPARATE_LINE = False
diff --git a/yolov5/train.py b/yolov5/train.py
new file mode 100644
index 0000000000000000000000000000000000000000..216da6399028bd3f6ed399bfdd377d25e3356a6e
--- /dev/null
+++ b/yolov5/train.py
@@ -0,0 +1,642 @@
+# YOLOv5 🚀 by Ultralytics, AGPL-3.0 license
+"""
+Train a YOLOv5 model on a custom dataset.
+Models and datasets download automatically from the latest YOLOv5 release.
+
+Usage - Single-GPU training:
+ $ python train.py --data coco128.yaml --weights yolov5s.pt --img 640 # from pretrained (recommended)
+ $ python train.py --data coco128.yaml --weights '' --cfg yolov5s.yaml --img 640 # from scratch
+
+Usage - Multi-GPU DDP training:
+ $ python -m torch.distributed.run --nproc_per_node 4 --master_port 1 train.py --data coco128.yaml --weights yolov5s.pt --img 640 --device 0,1,2,3
+
+Models: https://github.com/ultralytics/yolov5/tree/master/models
+Datasets: https://github.com/ultralytics/yolov5/tree/master/data
+Tutorial: https://docs.ultralytics.com/yolov5/tutorials/train_custom_data
+"""
+
+import argparse
+import math
+import os
+import random
+import subprocess
+import sys
+import time
+from copy import deepcopy
+from datetime import datetime
+from pathlib import Path
+
+import numpy as np
+import torch
+import torch.distributed as dist
+import torch.nn as nn
+import yaml
+from torch.optim import lr_scheduler
+from tqdm import tqdm
+
+FILE = Path(__file__).resolve()
+ROOT = FILE.parents[0] # YOLOv5 root directory
+if str(ROOT) not in sys.path:
+ sys.path.append(str(ROOT)) # add ROOT to PATH
+ROOT = Path(os.path.relpath(ROOT, Path.cwd())) # relative
+
+import val as validate # for end-of-epoch mAP
+from models.experimental import attempt_load
+from models.yolo import Model
+from utils.autoanchor import check_anchors
+from utils.autobatch import check_train_batch_size
+from utils.callbacks import Callbacks
+from utils.dataloaders import create_dataloader
+from utils.downloads import attempt_download, is_url
+from utils.general import (LOGGER, TQDM_BAR_FORMAT, check_amp, check_dataset, check_file, check_git_info,
+ check_git_status, check_img_size, check_requirements, check_suffix, check_yaml, colorstr,
+ get_latest_run, increment_path, init_seeds, intersect_dicts, labels_to_class_weights,
+ labels_to_image_weights, methods, one_cycle, print_args, print_mutation, strip_optimizer,
+ yaml_save)
+from utils.loggers import Loggers
+from utils.loggers.comet.comet_utils import check_comet_resume
+from utils.loss import ComputeLoss
+from utils.metrics import fitness
+from utils.plots import plot_evolve
+from utils.torch_utils import (EarlyStopping, ModelEMA, de_parallel, select_device, smart_DDP, smart_optimizer,
+ smart_resume, torch_distributed_zero_first)
+
+LOCAL_RANK = int(os.getenv('LOCAL_RANK', -1)) # https://pytorch.org/docs/stable/elastic/run.html
+RANK = int(os.getenv('RANK', -1))
+WORLD_SIZE = int(os.getenv('WORLD_SIZE', 1))
+GIT_INFO = check_git_info()
+
+
+def train(hyp, opt, device, callbacks): # hyp is path/to/hyp.yaml or hyp dictionary
+ save_dir, epochs, batch_size, weights, single_cls, evolve, data, cfg, resume, noval, nosave, workers, freeze = \
+ Path(opt.save_dir), opt.epochs, opt.batch_size, opt.weights, opt.single_cls, opt.evolve, opt.data, opt.cfg, \
+ opt.resume, opt.noval, opt.nosave, opt.workers, opt.freeze
+ callbacks.run('on_pretrain_routine_start')
+
+ # Directories
+ w = save_dir / 'weights' # weights dir
+ (w.parent if evolve else w).mkdir(parents=True, exist_ok=True) # make dir
+ last, best = w / 'last.pt', w / 'best.pt'
+
+ # Hyperparameters
+ if isinstance(hyp, str):
+ with open(hyp, errors='ignore') as f:
+ hyp = yaml.safe_load(f) # load hyps dict
+ LOGGER.info(colorstr('hyperparameters: ') + ', '.join(f'{k}={v}' for k, v in hyp.items()))
+ opt.hyp = hyp.copy() # for saving hyps to checkpoints
+
+ # Save run settings
+ if not evolve:
+ yaml_save(save_dir / 'hyp.yaml', hyp)
+ yaml_save(save_dir / 'opt.yaml', vars(opt))
+
+ # Loggers
+ data_dict = None
+ if RANK in {-1, 0}:
+ loggers = Loggers(save_dir, weights, opt, hyp, LOGGER) # loggers instance
+
+ # Register actions
+ for k in methods(loggers):
+ callbacks.register_action(k, callback=getattr(loggers, k))
+
+ # Process custom dataset artifact link
+ data_dict = loggers.remote_dataset
+ if resume: # If resuming runs from remote artifact
+ weights, epochs, hyp, batch_size = opt.weights, opt.epochs, opt.hyp, opt.batch_size
+
+ # Config
+ plots = not evolve and not opt.noplots # create plots
+ cuda = device.type != 'cpu'
+ init_seeds(opt.seed + 1 + RANK, deterministic=True)
+ with torch_distributed_zero_first(LOCAL_RANK):
+ data_dict = data_dict or check_dataset(data) # check if None
+ train_path, val_path = data_dict['train'], data_dict['val']
+ nc = 1 if single_cls else int(data_dict['nc']) # number of classes
+ names = {0: 'item'} if single_cls and len(data_dict['names']) != 1 else data_dict['names'] # class names
+ is_coco = isinstance(val_path, str) and val_path.endswith('coco/val2017.txt') # COCO dataset
+
+ # Model
+ check_suffix(weights, '.pt') # check weights
+ pretrained = weights.endswith('.pt')
+ if pretrained:
+ with torch_distributed_zero_first(LOCAL_RANK):
+ weights = attempt_download(weights) # download if not found locally
+ ckpt = torch.load(weights, map_location='cpu') # load checkpoint to CPU to avoid CUDA memory leak
+ model = Model(cfg or ckpt['model'].yaml, ch=3, nc=nc, anchors=hyp.get('anchors')).to(device) # create
+ exclude = ['anchor'] if (cfg or hyp.get('anchors')) and not resume else [] # exclude keys
+ csd = ckpt['model'].float().state_dict() # checkpoint state_dict as FP32
+ csd = intersect_dicts(csd, model.state_dict(), exclude=exclude) # intersect
+ model.load_state_dict(csd, strict=False) # load
+ LOGGER.info(f'Transferred {len(csd)}/{len(model.state_dict())} items from {weights}') # report
+ else:
+ model = Model(cfg, ch=3, nc=nc, anchors=hyp.get('anchors')).to(device) # create
+ amp = check_amp(model) # check AMP
+
+ # Freeze
+ freeze = [f'model.{x}.' for x in (freeze if len(freeze) > 1 else range(freeze[0]))] # layers to freeze
+ for k, v in model.named_parameters():
+ v.requires_grad = True # train all layers
+ # v.register_hook(lambda x: torch.nan_to_num(x)) # NaN to 0 (commented for erratic training results)
+ if any(x in k for x in freeze):
+ LOGGER.info(f'freezing {k}')
+ v.requires_grad = False
+
+ # Image size
+ gs = max(int(model.stride.max()), 32) # grid size (max stride)
+ imgsz = check_img_size(opt.imgsz, gs, floor=gs * 2) # verify imgsz is gs-multiple
+
+ # Batch size
+ if RANK == -1 and batch_size == -1: # single-GPU only, estimate best batch size
+ batch_size = check_train_batch_size(model, imgsz, amp)
+ loggers.on_params_update({'batch_size': batch_size})
+
+ # Optimizer
+ nbs = 64 # nominal batch size
+ accumulate = max(round(nbs / batch_size), 1) # accumulate loss before optimizing
+ hyp['weight_decay'] *= batch_size * accumulate / nbs # scale weight_decay
+ optimizer = smart_optimizer(model, opt.optimizer, hyp['lr0'], hyp['momentum'], hyp['weight_decay'])
+
+ # Scheduler
+ if opt.cos_lr:
+ lf = one_cycle(1, hyp['lrf'], epochs) # cosine 1->hyp['lrf']
+ else:
+ lf = lambda x: (1 - x / epochs) * (1.0 - hyp['lrf']) + hyp['lrf'] # linear
+ scheduler = lr_scheduler.LambdaLR(optimizer, lr_lambda=lf) # plot_lr_scheduler(optimizer, scheduler, epochs)
+
+ # EMA
+ ema = ModelEMA(model) if RANK in {-1, 0} else None
+
+ # Resume
+ best_fitness, start_epoch = 0.0, 0
+ if pretrained:
+ if resume:
+ best_fitness, start_epoch, epochs = smart_resume(ckpt, optimizer, ema, weights, epochs, resume)
+ del ckpt, csd
+
+ # DP mode
+ if cuda and RANK == -1 and torch.cuda.device_count() > 1:
+ LOGGER.warning(
+ 'WARNING ⚠️ DP not recommended, use torch.distributed.run for best DDP Multi-GPU results.\n'
+ 'See Multi-GPU Tutorial at https://docs.ultralytics.com/yolov5/tutorials/multi_gpu_training to get started.'
+ )
+ model = torch.nn.DataParallel(model)
+
+ # SyncBatchNorm
+ if opt.sync_bn and cuda and RANK != -1:
+ model = torch.nn.SyncBatchNorm.convert_sync_batchnorm(model).to(device)
+ LOGGER.info('Using SyncBatchNorm()')
+
+ # Trainloader
+ train_loader, dataset = create_dataloader(train_path,
+ imgsz,
+ batch_size // WORLD_SIZE,
+ gs,
+ single_cls,
+ hyp=hyp,
+ augment=True,
+ cache=None if opt.cache == 'val' else opt.cache,
+ rect=opt.rect,
+ rank=LOCAL_RANK,
+ workers=workers,
+ image_weights=opt.image_weights,
+ quad=opt.quad,
+ prefix=colorstr('train: '),
+ shuffle=True,
+ seed=opt.seed)
+ labels = np.concatenate(dataset.labels, 0)
+ mlc = int(labels[:, 0].max()) # max label class
+ assert mlc < nc, f'Label class {mlc} exceeds nc={nc} in {data}. Possible class labels are 0-{nc - 1}'
+
+ # Process 0
+ if RANK in {-1, 0}:
+ val_loader = create_dataloader(val_path,
+ imgsz,
+ batch_size // WORLD_SIZE * 2,
+ gs,
+ single_cls,
+ hyp=hyp,
+ cache=None if noval else opt.cache,
+ rect=True,
+ rank=-1,
+ workers=workers * 2,
+ pad=0.5,
+ prefix=colorstr('val: '))[0]
+
+ if not resume:
+ if not opt.noautoanchor:
+ check_anchors(dataset, model=model, thr=hyp['anchor_t'], imgsz=imgsz) # run AutoAnchor
+ model.half().float() # pre-reduce anchor precision
+
+ callbacks.run('on_pretrain_routine_end', labels, names)
+
+ # DDP mode
+ if cuda and RANK != -1:
+ model = smart_DDP(model)
+
+ # Model attributes
+ nl = de_parallel(model).model[-1].nl # number of detection layers (to scale hyps)
+ hyp['box'] *= 3 / nl # scale to layers
+ hyp['cls'] *= nc / 80 * 3 / nl # scale to classes and layers
+ hyp['obj'] *= (imgsz / 640) ** 2 * 3 / nl # scale to image size and layers
+ hyp['label_smoothing'] = opt.label_smoothing
+ model.nc = nc # attach number of classes to model
+ model.hyp = hyp # attach hyperparameters to model
+ model.class_weights = labels_to_class_weights(dataset.labels, nc).to(device) * nc # attach class weights
+ model.names = names
+
+ # Start training
+ t0 = time.time()
+ nb = len(train_loader) # number of batches
+ nw = max(round(hyp['warmup_epochs'] * nb), 100) # number of warmup iterations, max(3 epochs, 100 iterations)
+ # nw = min(nw, (epochs - start_epoch) / 2 * nb) # limit warmup to < 1/2 of training
+ last_opt_step = -1
+ maps = np.zeros(nc) # mAP per class
+ results = (0, 0, 0, 0, 0, 0, 0) # P, R, mAP@.5, mAP@.5-.95, val_loss(box, obj, cls)
+ scheduler.last_epoch = start_epoch - 1 # do not move
+ scaler = torch.cuda.amp.GradScaler(enabled=amp)
+ stopper, stop = EarlyStopping(patience=opt.patience), False
+ compute_loss = ComputeLoss(model) # init loss class
+ callbacks.run('on_train_start')
+ LOGGER.info(f'Image sizes {imgsz} train, {imgsz} val\n'
+ f'Using {train_loader.num_workers * WORLD_SIZE} dataloader workers\n'
+ f"Logging results to {colorstr('bold', save_dir)}\n"
+ f'Starting training for {epochs} epochs...')
+ for epoch in range(start_epoch, epochs): # epoch ------------------------------------------------------------------
+ callbacks.run('on_train_epoch_start')
+ model.train()
+
+ # Update image weights (optional, single-GPU only)
+ if opt.image_weights:
+ cw = model.class_weights.cpu().numpy() * (1 - maps) ** 2 / nc # class weights
+ iw = labels_to_image_weights(dataset.labels, nc=nc, class_weights=cw) # image weights
+ dataset.indices = random.choices(range(dataset.n), weights=iw, k=dataset.n) # rand weighted idx
+
+ # Update mosaic border (optional)
+ # b = int(random.uniform(0.25 * imgsz, 0.75 * imgsz + gs) // gs * gs)
+ # dataset.mosaic_border = [b - imgsz, -b] # height, width borders
+
+ mloss = torch.zeros(3, device=device) # mean losses
+ if RANK != -1:
+ train_loader.sampler.set_epoch(epoch)
+ pbar = enumerate(train_loader)
+ LOGGER.info(('\n' + '%11s' * 7) % ('Epoch', 'GPU_mem', 'box_loss', 'obj_loss', 'cls_loss', 'Instances', 'Size'))
+ if RANK in {-1, 0}:
+ pbar = tqdm(pbar, total=nb, bar_format=TQDM_BAR_FORMAT) # progress bar
+ optimizer.zero_grad()
+ for i, (imgs, targets, paths, _) in pbar: # batch -------------------------------------------------------------
+ callbacks.run('on_train_batch_start')
+ ni = i + nb * epoch # number integrated batches (since train start)
+ imgs = imgs.to(device, non_blocking=True).float() / 255 # uint8 to float32, 0-255 to 0.0-1.0
+
+ # Warmup
+ if ni <= nw:
+ xi = [0, nw] # x interp
+ # compute_loss.gr = np.interp(ni, xi, [0.0, 1.0]) # iou loss ratio (obj_loss = 1.0 or iou)
+ accumulate = max(1, np.interp(ni, xi, [1, nbs / batch_size]).round())
+ for j, x in enumerate(optimizer.param_groups):
+ # bias lr falls from 0.1 to lr0, all other lrs rise from 0.0 to lr0
+ x['lr'] = np.interp(ni, xi, [hyp['warmup_bias_lr'] if j == 0 else 0.0, x['initial_lr'] * lf(epoch)])
+ if 'momentum' in x:
+ x['momentum'] = np.interp(ni, xi, [hyp['warmup_momentum'], hyp['momentum']])
+
+ # Multi-scale
+ if opt.multi_scale:
+ sz = random.randrange(int(imgsz * 0.5), int(imgsz * 1.5) + gs) // gs * gs # size
+ sf = sz / max(imgs.shape[2:]) # scale factor
+ if sf != 1:
+ ns = [math.ceil(x * sf / gs) * gs for x in imgs.shape[2:]] # new shape (stretched to gs-multiple)
+ imgs = nn.functional.interpolate(imgs, size=ns, mode='bilinear', align_corners=False)
+
+ # Forward
+ with torch.cuda.amp.autocast(amp):
+ pred = model(imgs) # forward
+ loss, loss_items = compute_loss(pred, targets.to(device)) # loss scaled by batch_size
+ if RANK != -1:
+ loss *= WORLD_SIZE # gradient averaged between devices in DDP mode
+ if opt.quad:
+ loss *= 4.
+
+ # Backward
+ scaler.scale(loss).backward()
+
+ # Optimize - https://pytorch.org/docs/master/notes/amp_examples.html
+ if ni - last_opt_step >= accumulate:
+ scaler.unscale_(optimizer) # unscale gradients
+ torch.nn.utils.clip_grad_norm_(model.parameters(), max_norm=10.0) # clip gradients
+ scaler.step(optimizer) # optimizer.step
+ scaler.update()
+ optimizer.zero_grad()
+ if ema:
+ ema.update(model)
+ last_opt_step = ni
+
+ # Log
+ if RANK in {-1, 0}:
+ mloss = (mloss * i + loss_items) / (i + 1) # update mean losses
+ mem = f'{torch.cuda.memory_reserved() / 1E9 if torch.cuda.is_available() else 0:.3g}G' # (GB)
+ pbar.set_description(('%11s' * 2 + '%11.4g' * 5) %
+ (f'{epoch}/{epochs - 1}', mem, *mloss, targets.shape[0], imgs.shape[-1]))
+ callbacks.run('on_train_batch_end', model, ni, imgs, targets, paths, list(mloss))
+ if callbacks.stop_training:
+ return
+ # end batch ------------------------------------------------------------------------------------------------
+
+ # Scheduler
+ lr = [x['lr'] for x in optimizer.param_groups] # for loggers
+ scheduler.step()
+
+ if RANK in {-1, 0}:
+ # mAP
+ callbacks.run('on_train_epoch_end', epoch=epoch)
+ ema.update_attr(model, include=['yaml', 'nc', 'hyp', 'names', 'stride', 'class_weights'])
+ final_epoch = (epoch + 1 == epochs) or stopper.possible_stop
+ if not noval or final_epoch: # Calculate mAP
+ results, maps, _ = validate.run(data_dict,
+ batch_size=batch_size // WORLD_SIZE * 2,
+ imgsz=imgsz,
+ half=amp,
+ model=ema.ema,
+ single_cls=single_cls,
+ dataloader=val_loader,
+ save_dir=save_dir,
+ plots=False,
+ callbacks=callbacks,
+ compute_loss=compute_loss)
+
+ # Update best mAP
+ fi = fitness(np.array(results).reshape(1, -1)) # weighted combination of [P, R, mAP@.5, mAP@.5-.95]
+ stop = stopper(epoch=epoch, fitness=fi) # early stop check
+ if fi > best_fitness:
+ best_fitness = fi
+ log_vals = list(mloss) + list(results) + lr
+ callbacks.run('on_fit_epoch_end', log_vals, epoch, best_fitness, fi)
+
+ # Save model
+ if (not nosave) or (final_epoch and not evolve): # if save
+ ckpt = {
+ 'epoch': epoch,
+ 'best_fitness': best_fitness,
+ 'model': deepcopy(de_parallel(model)).half(),
+ 'ema': deepcopy(ema.ema).half(),
+ 'updates': ema.updates,
+ 'optimizer': optimizer.state_dict(),
+ 'opt': vars(opt),
+ 'git': GIT_INFO, # {remote, branch, commit} if a git repo
+ 'date': datetime.now().isoformat()}
+
+ # Save last, best and delete
+ torch.save(ckpt, last)
+ if best_fitness == fi:
+ torch.save(ckpt, best)
+ if opt.save_period > 0 and epoch % opt.save_period == 0:
+ torch.save(ckpt, w / f'epoch{epoch}.pt')
+ del ckpt
+ callbacks.run('on_model_save', last, epoch, final_epoch, best_fitness, fi)
+
+ # EarlyStopping
+ if RANK != -1: # if DDP training
+ broadcast_list = [stop if RANK == 0 else None]
+ dist.broadcast_object_list(broadcast_list, 0) # broadcast 'stop' to all ranks
+ if RANK != 0:
+ stop = broadcast_list[0]
+ if stop:
+ break # must break all DDP ranks
+
+ # end epoch ----------------------------------------------------------------------------------------------------
+ # end training -----------------------------------------------------------------------------------------------------
+ if RANK in {-1, 0}:
+ LOGGER.info(f'\n{epoch - start_epoch + 1} epochs completed in {(time.time() - t0) / 3600:.3f} hours.')
+ for f in last, best:
+ if f.exists():
+ strip_optimizer(f) # strip optimizers
+ if f is best:
+ LOGGER.info(f'\nValidating {f}...')
+ results, _, _ = validate.run(
+ data_dict,
+ batch_size=batch_size // WORLD_SIZE * 2,
+ imgsz=imgsz,
+ model=attempt_load(f, device).half(),
+ iou_thres=0.65 if is_coco else 0.60, # best pycocotools at iou 0.65
+ single_cls=single_cls,
+ dataloader=val_loader,
+ save_dir=save_dir,
+ save_json=is_coco,
+ verbose=True,
+ plots=plots,
+ callbacks=callbacks,
+ compute_loss=compute_loss) # val best model with plots
+ if is_coco:
+ callbacks.run('on_fit_epoch_end', list(mloss) + list(results) + lr, epoch, best_fitness, fi)
+
+ callbacks.run('on_train_end', last, best, epoch, results)
+
+ torch.cuda.empty_cache()
+ return results
+
+
+def parse_opt(known=False):
+ parser = argparse.ArgumentParser()
+ parser.add_argument('--weights', type=str, default=ROOT / 'yolov5s.pt', help='initial weights path')
+ parser.add_argument('--cfg', type=str, default='', help='model.yaml path')
+ parser.add_argument('--data', type=str, default=ROOT / 'data/coco128.yaml', help='dataset.yaml path')
+ parser.add_argument('--hyp', type=str, default=ROOT / 'data/hyps/hyp.scratch-low.yaml', help='hyperparameters path')
+ parser.add_argument('--epochs', type=int, default=100, help='total training epochs')
+ parser.add_argument('--batch-size', type=int, default=16, help='total batch size for all GPUs, -1 for autobatch')
+ parser.add_argument('--imgsz', '--img', '--img-size', type=int, default=640, help='train, val image size (pixels)')
+ parser.add_argument('--rect', action='store_true', help='rectangular training')
+ parser.add_argument('--resume', nargs='?', const=True, default=False, help='resume most recent training')
+ parser.add_argument('--nosave', action='store_true', help='only save final checkpoint')
+ parser.add_argument('--noval', action='store_true', help='only validate final epoch')
+ parser.add_argument('--noautoanchor', action='store_true', help='disable AutoAnchor')
+ parser.add_argument('--noplots', action='store_true', help='save no plot files')
+ parser.add_argument('--evolve', type=int, nargs='?', const=300, help='evolve hyperparameters for x generations')
+ parser.add_argument('--bucket', type=str, default='', help='gsutil bucket')
+ parser.add_argument('--cache', type=str, nargs='?', const='ram', help='image --cache ram/disk')
+ parser.add_argument('--image-weights', action='store_true', help='use weighted image selection for training')
+ parser.add_argument('--device', default='', help='cuda device, i.e. 0 or 0,1,2,3 or cpu')
+ parser.add_argument('--multi-scale', action='store_true', help='vary img-size +/- 50%%')
+ parser.add_argument('--single-cls', action='store_true', help='train multi-class data as single-class')
+ parser.add_argument('--optimizer', type=str, choices=['SGD', 'Adam', 'AdamW'], default='SGD', help='optimizer')
+ parser.add_argument('--sync-bn', action='store_true', help='use SyncBatchNorm, only available in DDP mode')
+ parser.add_argument('--workers', type=int, default=8, help='max dataloader workers (per RANK in DDP mode)')
+ parser.add_argument('--project', default=ROOT / 'runs/train', help='save to project/name')
+ parser.add_argument('--name', default='exp', help='save to project/name')
+ parser.add_argument('--exist-ok', action='store_true', help='existing project/name ok, do not increment')
+ parser.add_argument('--quad', action='store_true', help='quad dataloader')
+ parser.add_argument('--cos-lr', action='store_true', help='cosine LR scheduler')
+ parser.add_argument('--label-smoothing', type=float, default=0.0, help='Label smoothing epsilon')
+ parser.add_argument('--patience', type=int, default=100, help='EarlyStopping patience (epochs without improvement)')
+ parser.add_argument('--freeze', nargs='+', type=int, default=[0], help='Freeze layers: backbone=10, first3=0 1 2')
+ parser.add_argument('--save-period', type=int, default=-1, help='Save checkpoint every x epochs (disabled if < 1)')
+ parser.add_argument('--seed', type=int, default=0, help='Global training seed')
+ parser.add_argument('--local_rank', type=int, default=-1, help='Automatic DDP Multi-GPU argument, do not modify')
+
+ # Logger arguments
+ parser.add_argument('--entity', default=None, help='Entity')
+ parser.add_argument('--upload_dataset', nargs='?', const=True, default=False, help='Upload data, "val" option')
+ parser.add_argument('--bbox_interval', type=int, default=-1, help='Set bounding-box image logging interval')
+ parser.add_argument('--artifact_alias', type=str, default='latest', help='Version of dataset artifact to use')
+
+ return parser.parse_known_args()[0] if known else parser.parse_args()
+
+
+def main(opt, callbacks=Callbacks()):
+ # Checks
+ if RANK in {-1, 0}:
+ print_args(vars(opt))
+ check_git_status()
+ check_requirements()
+
+ # Resume (from specified or most recent last.pt)
+ if opt.resume and not check_comet_resume(opt) and not opt.evolve:
+ last = Path(check_file(opt.resume) if isinstance(opt.resume, str) else get_latest_run())
+ opt_yaml = last.parent.parent / 'opt.yaml' # train options yaml
+ opt_data = opt.data # original dataset
+ if opt_yaml.is_file():
+ with open(opt_yaml, errors='ignore') as f:
+ d = yaml.safe_load(f)
+ else:
+ d = torch.load(last, map_location='cpu')['opt']
+ opt = argparse.Namespace(**d) # replace
+ opt.cfg, opt.weights, opt.resume = '', str(last), True # reinstate
+ if is_url(opt_data):
+ opt.data = check_file(opt_data) # avoid HUB resume auth timeout
+ else:
+ opt.data, opt.cfg, opt.hyp, opt.weights, opt.project = \
+ check_file(opt.data), check_yaml(opt.cfg), check_yaml(opt.hyp), str(opt.weights), str(opt.project) # checks
+ assert len(opt.cfg) or len(opt.weights), 'either --cfg or --weights must be specified'
+ if opt.evolve:
+ if opt.project == str(ROOT / 'runs/train'): # if default project name, rename to runs/evolve
+ opt.project = str(ROOT / 'runs/evolve')
+ opt.exist_ok, opt.resume = opt.resume, False # pass resume to exist_ok and disable resume
+ if opt.name == 'cfg':
+ opt.name = Path(opt.cfg).stem # use model.yaml as name
+ opt.save_dir = str(increment_path(Path(opt.project) / opt.name, exist_ok=opt.exist_ok))
+
+ # DDP mode
+ device = select_device(opt.device, batch_size=opt.batch_size)
+ if LOCAL_RANK != -1:
+ msg = 'is not compatible with YOLOv5 Multi-GPU DDP training'
+ assert not opt.image_weights, f'--image-weights {msg}'
+ assert not opt.evolve, f'--evolve {msg}'
+ assert opt.batch_size != -1, f'AutoBatch with --batch-size -1 {msg}, please pass a valid --batch-size'
+ assert opt.batch_size % WORLD_SIZE == 0, f'--batch-size {opt.batch_size} must be multiple of WORLD_SIZE'
+ assert torch.cuda.device_count() > LOCAL_RANK, 'insufficient CUDA devices for DDP command'
+ torch.cuda.set_device(LOCAL_RANK)
+ device = torch.device('cuda', LOCAL_RANK)
+ dist.init_process_group(backend='nccl' if dist.is_nccl_available() else 'gloo')
+
+ # Train
+ if not opt.evolve:
+ train(opt.hyp, opt, device, callbacks)
+
+ # Evolve hyperparameters (optional)
+ else:
+ # Hyperparameter evolution metadata (mutation scale 0-1, lower_limit, upper_limit)
+ meta = {
+ 'lr0': (1, 1e-5, 1e-1), # initial learning rate (SGD=1E-2, Adam=1E-3)
+ 'lrf': (1, 0.01, 1.0), # final OneCycleLR learning rate (lr0 * lrf)
+ 'momentum': (0.3, 0.6, 0.98), # SGD momentum/Adam beta1
+ 'weight_decay': (1, 0.0, 0.001), # optimizer weight decay
+ 'warmup_epochs': (1, 0.0, 5.0), # warmup epochs (fractions ok)
+ 'warmup_momentum': (1, 0.0, 0.95), # warmup initial momentum
+ 'warmup_bias_lr': (1, 0.0, 0.2), # warmup initial bias lr
+ 'box': (1, 0.02, 0.2), # box loss gain
+ 'cls': (1, 0.2, 4.0), # cls loss gain
+ 'cls_pw': (1, 0.5, 2.0), # cls BCELoss positive_weight
+ 'obj': (1, 0.2, 4.0), # obj loss gain (scale with pixels)
+ 'obj_pw': (1, 0.5, 2.0), # obj BCELoss positive_weight
+ 'iou_t': (0, 0.1, 0.7), # IoU training threshold
+ 'anchor_t': (1, 2.0, 8.0), # anchor-multiple threshold
+ 'anchors': (2, 2.0, 10.0), # anchors per output grid (0 to ignore)
+ 'fl_gamma': (0, 0.0, 2.0), # focal loss gamma (efficientDet default gamma=1.5)
+ 'hsv_h': (1, 0.0, 0.1), # image HSV-Hue augmentation (fraction)
+ 'hsv_s': (1, 0.0, 0.9), # image HSV-Saturation augmentation (fraction)
+ 'hsv_v': (1, 0.0, 0.9), # image HSV-Value augmentation (fraction)
+ 'degrees': (1, 0.0, 45.0), # image rotation (+/- deg)
+ 'translate': (1, 0.0, 0.9), # image translation (+/- fraction)
+ 'scale': (1, 0.0, 0.9), # image scale (+/- gain)
+ 'shear': (1, 0.0, 10.0), # image shear (+/- deg)
+ 'perspective': (0, 0.0, 0.001), # image perspective (+/- fraction), range 0-0.001
+ 'flipud': (1, 0.0, 1.0), # image flip up-down (probability)
+ 'fliplr': (0, 0.0, 1.0), # image flip left-right (probability)
+ 'mosaic': (1, 0.0, 1.0), # image mixup (probability)
+ 'mixup': (1, 0.0, 1.0), # image mixup (probability)
+ 'copy_paste': (1, 0.0, 1.0)} # segment copy-paste (probability)
+
+ with open(opt.hyp, errors='ignore') as f:
+ hyp = yaml.safe_load(f) # load hyps dict
+ if 'anchors' not in hyp: # anchors commented in hyp.yaml
+ hyp['anchors'] = 3
+ if opt.noautoanchor:
+ del hyp['anchors'], meta['anchors']
+ opt.noval, opt.nosave, save_dir = True, True, Path(opt.save_dir) # only val/save final epoch
+ # ei = [isinstance(x, (int, float)) for x in hyp.values()] # evolvable indices
+ evolve_yaml, evolve_csv = save_dir / 'hyp_evolve.yaml', save_dir / 'evolve.csv'
+ if opt.bucket:
+ # download evolve.csv if exists
+ subprocess.run([
+ 'gsutil',
+ 'cp',
+ f'gs://{opt.bucket}/evolve.csv',
+ str(evolve_csv),])
+
+ for _ in range(opt.evolve): # generations to evolve
+ if evolve_csv.exists(): # if evolve.csv exists: select best hyps and mutate
+ # Select parent(s)
+ parent = 'single' # parent selection method: 'single' or 'weighted'
+ x = np.loadtxt(evolve_csv, ndmin=2, delimiter=',', skiprows=1)
+ n = min(5, len(x)) # number of previous results to consider
+ x = x[np.argsort(-fitness(x))][:n] # top n mutations
+ w = fitness(x) - fitness(x).min() + 1E-6 # weights (sum > 0)
+ if parent == 'single' or len(x) == 1:
+ # x = x[random.randint(0, n - 1)] # random selection
+ x = x[random.choices(range(n), weights=w)[0]] # weighted selection
+ elif parent == 'weighted':
+ x = (x * w.reshape(n, 1)).sum(0) / w.sum() # weighted combination
+
+ # Mutate
+ mp, s = 0.8, 0.2 # mutation probability, sigma
+ npr = np.random
+ npr.seed(int(time.time()))
+ g = np.array([meta[k][0] for k in hyp.keys()]) # gains 0-1
+ ng = len(meta)
+ v = np.ones(ng)
+ while all(v == 1): # mutate until a change occurs (prevent duplicates)
+ v = (g * (npr.random(ng) < mp) * npr.randn(ng) * npr.random() * s + 1).clip(0.3, 3.0)
+ for i, k in enumerate(hyp.keys()): # plt.hist(v.ravel(), 300)
+ hyp[k] = float(x[i + 7] * v[i]) # mutate
+
+ # Constrain to limits
+ for k, v in meta.items():
+ hyp[k] = max(hyp[k], v[1]) # lower limit
+ hyp[k] = min(hyp[k], v[2]) # upper limit
+ hyp[k] = round(hyp[k], 5) # significant digits
+
+ # Train mutation
+ results = train(hyp.copy(), opt, device, callbacks)
+ callbacks = Callbacks()
+ # Write mutation results
+ keys = ('metrics/precision', 'metrics/recall', 'metrics/mAP_0.5', 'metrics/mAP_0.5:0.95', 'val/box_loss',
+ 'val/obj_loss', 'val/cls_loss')
+ print_mutation(keys, results, hyp.copy(), save_dir, opt.bucket)
+
+ # Plot results
+ plot_evolve(evolve_csv)
+ LOGGER.info(f'Hyperparameter evolution finished {opt.evolve} generations\n'
+ f"Results saved to {colorstr('bold', save_dir)}\n"
+ f'Usage example: $ python train.py --hyp {evolve_yaml}')
+
+
+def run(**kwargs):
+ # Usage: import train; train.run(data='coco128.yaml', imgsz=320, weights='yolov5m.pt')
+ opt = parse_opt(True)
+ for k, v in kwargs.items():
+ setattr(opt, k, v)
+ main(opt)
+ return opt
+
+
+if __name__ == '__main__':
+ opt = parse_opt()
+ main(opt)
diff --git a/yolov5/tutorial.ipynb b/yolov5/tutorial.ipynb
new file mode 100644
index 0000000000000000000000000000000000000000..be87068822af223ba8383b6912b55e9f79181b0b
--- /dev/null
+++ b/yolov5/tutorial.ipynb
@@ -0,0 +1,605 @@
+{
+ "nbformat": 4,
+ "nbformat_minor": 0,
+ "metadata": {
+ "colab": {
+ "name": "YOLOv5 Tutorial",
+ "provenance": []
+ },
+ "kernelspec": {
+ "name": "python3",
+ "display_name": "Python 3"
+ },
+ "accelerator": "GPU"
+ },
+ "cells": [
+ {
+ "cell_type": "markdown",
+ "metadata": {
+ "id": "t6MPjfT5NrKQ"
+ },
+ "source": [
+ "
\n",
+ "\n",
+ " \n",
+ " \n",
+ "\n",
+ "\n",
+ " \n",
+ " \n",
+ " \n",
+ " \n",
+ " \n",
+ "\n",
+ "This YOLOv5 🚀 notebook by Ultralytics presents simple train, validate and predict examples to help start your AI adventure. We hope that the resources in this notebook will help you get the most out of YOLOv5. Please browse the YOLOv5 Docs for details, raise an issue on GitHub for support, and join our Discord community for questions and discussions!\n",
+ "\n",
+ "