File size: 12,896 Bytes
43b7e92 |
1 2 3 4 5 6 7 8 9 10 11 12 13 14 15 16 17 18 19 20 21 22 23 24 25 26 27 28 29 30 31 32 33 34 35 36 37 38 39 40 41 42 43 44 45 46 47 48 49 50 51 52 53 54 55 56 57 58 59 60 61 62 63 64 65 66 67 68 69 70 71 72 73 74 75 76 77 78 79 80 81 82 83 84 85 86 87 88 89 90 91 92 93 94 95 96 97 98 99 100 101 102 103 104 105 106 107 108 109 110 111 112 113 114 115 116 117 118 119 120 121 122 123 124 125 126 127 128 129 130 131 132 133 134 135 136 137 138 139 140 141 142 143 144 145 146 147 148 149 150 151 152 153 154 155 156 157 158 159 160 161 162 163 164 165 166 167 168 169 170 171 172 173 174 175 176 177 178 179 180 181 182 183 184 185 186 187 188 189 190 191 192 193 194 195 196 197 198 199 200 201 202 203 204 205 206 207 208 209 210 211 212 213 214 215 216 217 218 219 220 221 222 223 224 225 226 227 228 229 230 231 232 |
# Community Scripts
**Community scripts** consist of inference examples using Diffusers pipelines that have been added by the community.
Please have a look at the following table to get an overview of all community examples. Click on the **Code Example** to get a copy-and-paste code example that you can try out.
If a community script doesn't work as expected, please open an issue and ping the author on it.
| Example | Description | Code Example | Colab | Author |
|:--------------------------------------------------------------------------------------------------------------------------------------|:---------------------------------------------------------------------------------------------------------------------------------------------------------------------------------------------------------------------------------------------------------------------------------------------------------------------------------------------------------------------------------------------------------------------------------------------------------------------------------------------------------|:------------------------------------------------------------------------------------------|:-------------------------------------------------------------------------------------------------------------------------------------------------------------------------------------------------------------------|--------------------------------------------------------------:|
| Using IP-Adapter with negative noise | Using negative noise with IP-adapter to better control the generation (see the [original post](https://github.com/huggingface/diffusers/discussions/7167) on the forum for more details) | [IP-Adapter Negative Noise](#ip-adapter-negative-noise) | | [Álvaro Somoza](https://github.com/asomoza)|
| asymmetric tiling |configure seamless image tiling independently for the X and Y axes | [Asymmetric Tiling](#asymmetric-tiling ) | | [alexisrolland](https://github.com/alexisrolland)|
## Example usages
### IP Adapter Negative Noise
Diffusers pipelines are fully integrated with IP-Adapter, which allows you to prompt the diffusion model with an image. However, it does not support negative image prompts (there is no `negative_ip_adapter_image` argument) the same way it supports negative text prompts. When you pass an `ip_adapter_image,` it will create a zero-filled tensor as a negative image. This script shows you how to create a negative noise from `ip_adapter_image` and use it to significantly improve the generation quality while preserving the composition of images.
[cubiq](https://github.com/cubiq) initially developed this feature in his [repository](https://github.com/cubiq/ComfyUI_IPAdapter_plus). The community script was contributed by [asomoza](https://github.com/Somoza). You can find more details about this experimentation [this discussion](https://github.com/huggingface/diffusers/discussions/7167)
IP-Adapter without negative noise
|source|result|
|---|---|
|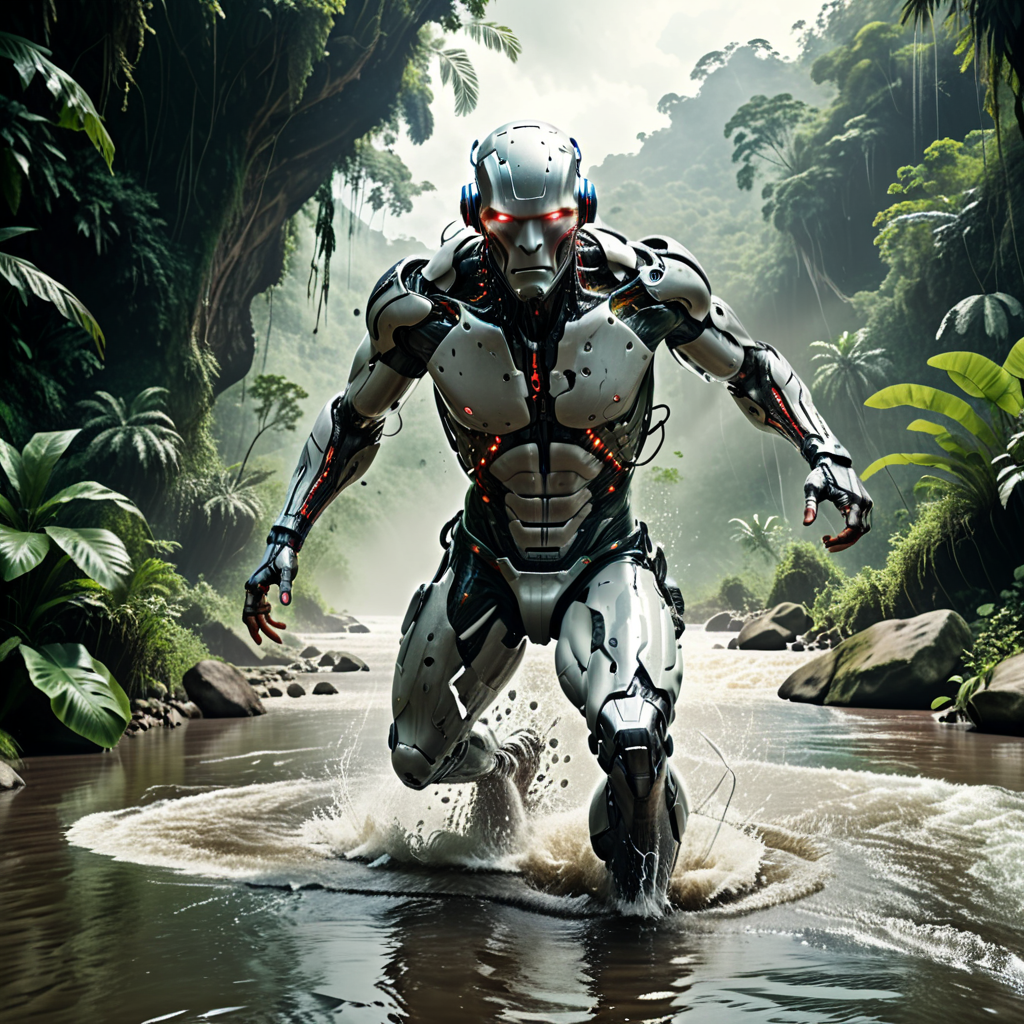|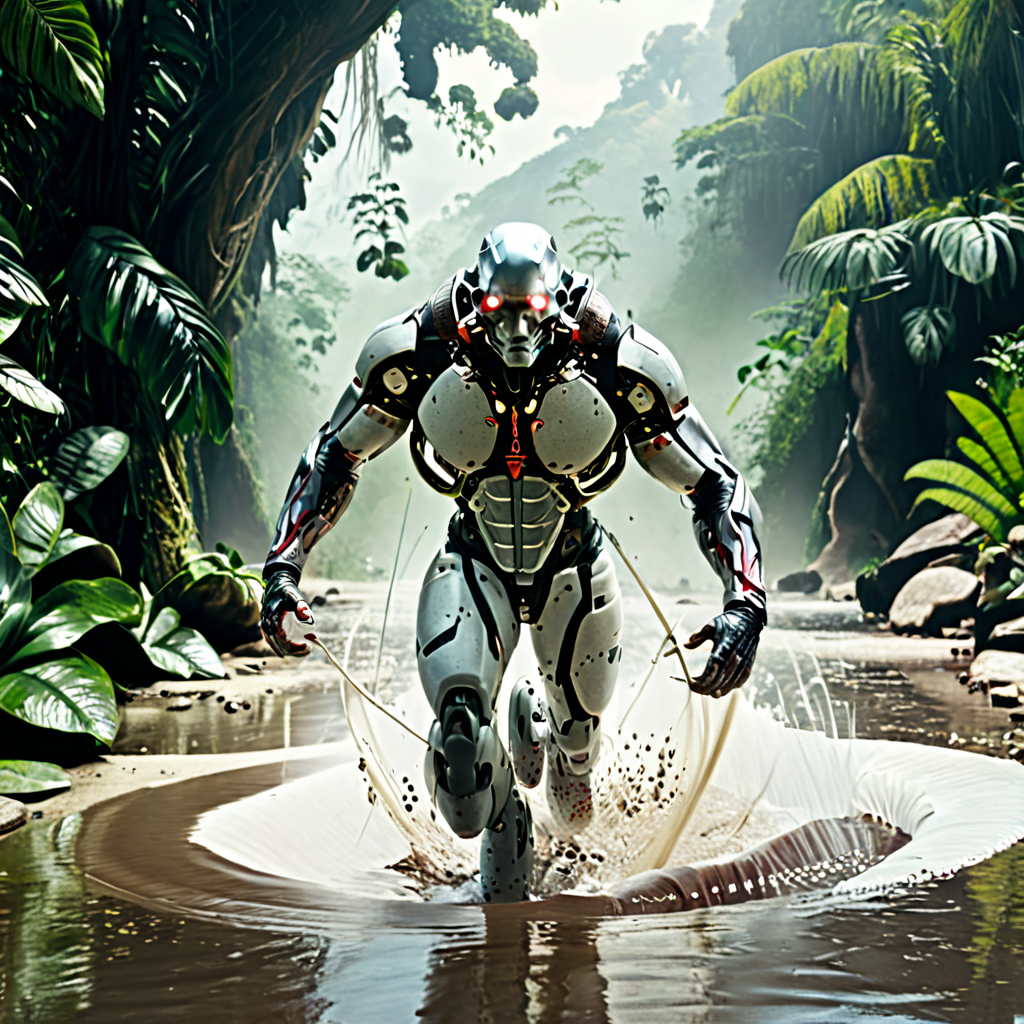|
IP-Adapter with negative noise
|source|result|
|---|---|
|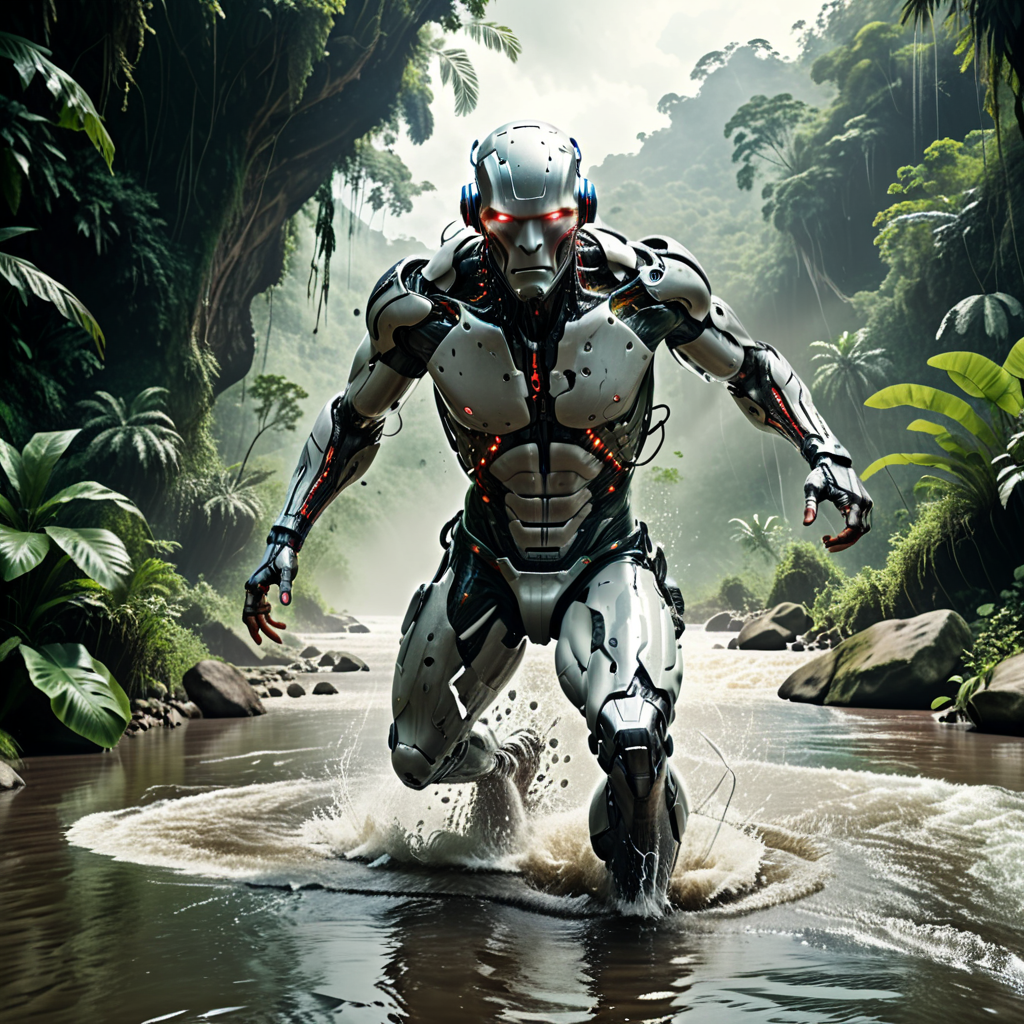|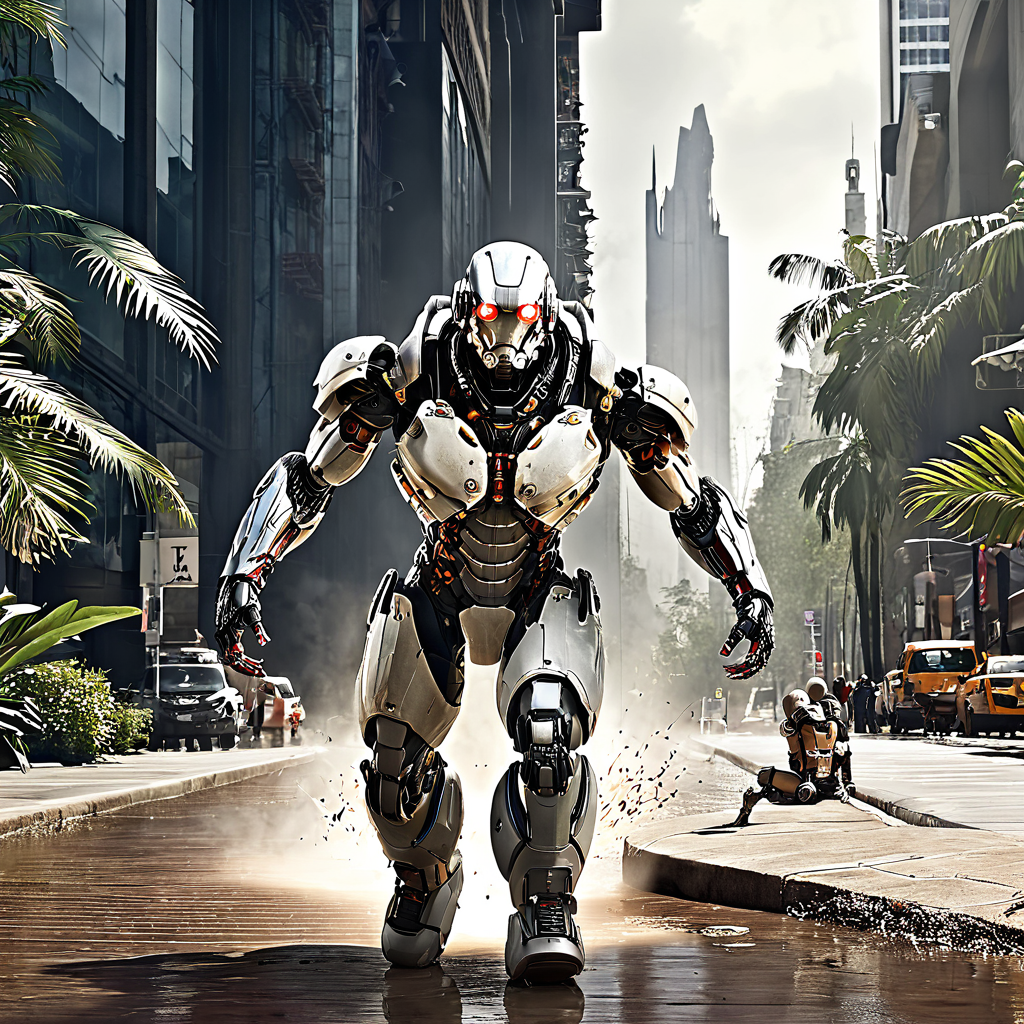|
```python
import torch
from diffusers import AutoencoderKL, DPMSolverMultistepScheduler, StableDiffusionXLPipeline
from diffusers.models import ImageProjection
from diffusers.utils import load_image
def encode_image(
image_encoder,
feature_extractor,
image,
device,
num_images_per_prompt,
output_hidden_states=None,
negative_image=None,
):
dtype = next(image_encoder.parameters()).dtype
if not isinstance(image, torch.Tensor):
image = feature_extractor(image, return_tensors="pt").pixel_values
image = image.to(device=device, dtype=dtype)
if output_hidden_states:
image_enc_hidden_states = image_encoder(image, output_hidden_states=True).hidden_states[-2]
image_enc_hidden_states = image_enc_hidden_states.repeat_interleave(num_images_per_prompt, dim=0)
if negative_image is None:
uncond_image_enc_hidden_states = image_encoder(
torch.zeros_like(image), output_hidden_states=True
).hidden_states[-2]
else:
if not isinstance(negative_image, torch.Tensor):
negative_image = feature_extractor(negative_image, return_tensors="pt").pixel_values
negative_image = negative_image.to(device=device, dtype=dtype)
uncond_image_enc_hidden_states = image_encoder(negative_image, output_hidden_states=True).hidden_states[-2]
uncond_image_enc_hidden_states = uncond_image_enc_hidden_states.repeat_interleave(num_images_per_prompt, dim=0)
return image_enc_hidden_states, uncond_image_enc_hidden_states
else:
image_embeds = image_encoder(image).image_embeds
image_embeds = image_embeds.repeat_interleave(num_images_per_prompt, dim=0)
uncond_image_embeds = torch.zeros_like(image_embeds)
return image_embeds, uncond_image_embeds
@torch.no_grad()
def prepare_ip_adapter_image_embeds(
unet,
image_encoder,
feature_extractor,
ip_adapter_image,
do_classifier_free_guidance,
device,
num_images_per_prompt,
ip_adapter_negative_image=None,
):
if not isinstance(ip_adapter_image, list):
ip_adapter_image = [ip_adapter_image]
if len(ip_adapter_image) != len(unet.encoder_hid_proj.image_projection_layers):
raise ValueError(
f"`ip_adapter_image` must have same length as the number of IP Adapters. Got {len(ip_adapter_image)} images and {len(unet.encoder_hid_proj.image_projection_layers)} IP Adapters."
)
image_embeds = []
for single_ip_adapter_image, image_proj_layer in zip(
ip_adapter_image, unet.encoder_hid_proj.image_projection_layers
):
output_hidden_state = not isinstance(image_proj_layer, ImageProjection)
single_image_embeds, single_negative_image_embeds = encode_image(
image_encoder,
feature_extractor,
single_ip_adapter_image,
device,
1,
output_hidden_state,
negative_image=ip_adapter_negative_image,
)
single_image_embeds = torch.stack([single_image_embeds] * num_images_per_prompt, dim=0)
single_negative_image_embeds = torch.stack([single_negative_image_embeds] * num_images_per_prompt, dim=0)
if do_classifier_free_guidance:
single_image_embeds = torch.cat([single_negative_image_embeds, single_image_embeds])
single_image_embeds = single_image_embeds.to(device)
image_embeds.append(single_image_embeds)
return image_embeds
vae = AutoencoderKL.from_pretrained(
"madebyollin/sdxl-vae-fp16-fix",
torch_dtype=torch.float16,
).to("cuda")
pipeline = StableDiffusionXLPipeline.from_pretrained(
"RunDiffusion/Juggernaut-XL-v9",
torch_dtype=torch.float16,
vae=vae,
variant="fp16",
).to("cuda")
pipeline.scheduler = DPMSolverMultistepScheduler.from_config(pipeline.scheduler.config)
pipeline.scheduler.config.use_karras_sigmas = True
pipeline.load_ip_adapter(
"h94/IP-Adapter",
subfolder="sdxl_models",
weight_name="ip-adapter-plus_sdxl_vit-h.safetensors",
image_encoder_folder="models/image_encoder",
)
pipeline.set_ip_adapter_scale(0.7)
ip_image = load_image("source.png")
negative_ip_image = load_image("noise.png")
image_embeds = prepare_ip_adapter_image_embeds(
unet=pipeline.unet,
image_encoder=pipeline.image_encoder,
feature_extractor=pipeline.feature_extractor,
ip_adapter_image=[[ip_image]],
do_classifier_free_guidance=True,
device="cuda",
num_images_per_prompt=1,
ip_adapter_negative_image=negative_ip_image,
)
prompt = "cinematic photo of a cyborg in the city, 4k, high quality, intricate, highly detailed"
negative_prompt = "blurry, smooth, plastic"
image = pipeline(
prompt=prompt,
negative_prompt=negative_prompt,
ip_adapter_image_embeds=image_embeds,
guidance_scale=6.0,
num_inference_steps=25,
generator=torch.Generator(device="cpu").manual_seed(1556265306),
).images[0]
image.save("result.png")
```
### Asymmetric Tiling
Stable Diffusion is not trained to generate seamless textures. However, you can use this simple script to add tiling to your generation. This script is contributed by [alexisrolland](https://github.com/alexisrolland). See more details in the [this issue](https://github.com/huggingface/diffusers/issues/556)
|Generated|Tiled|
|---|---|
|||
```py
import torch
from typing import Optional
from diffusers import StableDiffusionPipeline
from diffusers.models.lora import LoRACompatibleConv
def seamless_tiling(pipeline, x_axis, y_axis):
def asymmetric_conv2d_convforward(self, input: torch.Tensor, weight: torch.Tensor, bias: Optional[torch.Tensor] = None):
self.paddingX = (self._reversed_padding_repeated_twice[0], self._reversed_padding_repeated_twice[1], 0, 0)
self.paddingY = (0, 0, self._reversed_padding_repeated_twice[2], self._reversed_padding_repeated_twice[3])
working = torch.nn.functional.pad(input, self.paddingX, mode=x_mode)
working = torch.nn.functional.pad(working, self.paddingY, mode=y_mode)
return torch.nn.functional.conv2d(working, weight, bias, self.stride, torch.nn.modules.utils._pair(0), self.dilation, self.groups)
x_mode = 'circular' if x_axis else 'constant'
y_mode = 'circular' if y_axis else 'constant'
targets = [pipeline.vae, pipeline.text_encoder, pipeline.unet]
convolution_layers = []
for target in targets:
for module in target.modules():
if isinstance(module, torch.nn.Conv2d):
convolution_layers.append(module)
for layer in convolution_layers:
if isinstance(layer, LoRACompatibleConv) and layer.lora_layer is None:
layer.lora_layer = lambda * x: 0
layer._conv_forward = asymmetric_conv2d_convforward.__get__(layer, torch.nn.Conv2d)
return pipeline
pipeline = StableDiffusionPipeline.from_pretrained("runwayml/stable-diffusion-v1-5", torch_dtype=torch.float16, use_safetensors=True)
pipeline.enable_model_cpu_offload()
prompt = ["texture of a red brick wall"]
seed = 123456
generator = torch.Generator(device='cuda').manual_seed(seed)
pipeline = seamless_tiling(pipeline=pipeline, x_axis=True, y_axis=True)
image = pipeline(
prompt=prompt,
width=512,
height=512,
num_inference_steps=20,
guidance_scale=7,
num_images_per_prompt=1,
generator=generator
).images[0]
seamless_tiling(pipeline=pipeline, x_axis=False, y_axis=False)
torch.cuda.empty_cache()
image.save('image.png')
``` |