|
--- |
|
language: |
|
- ar |
|
datasets: |
|
- arabic QA |
|
widget: |
|
- text: "answer: 7 سنوات ونصف context: الثورة الجزائرية أو ثورة المليون شهيد، اندلعت في 1 نوفمبر 1954 ضد المستعمر الفرنسي ودامت 7 سنوات ونصف. استشهد فيها أكثر من مليون ونصف مليون جزائري" |
|
--- |
|
|
|
# Arabic Question generation Model |
|
[AraT5-Base Model](https://huggingface.co/UBC-NLP/AraT5-base) fine-tuned on Arabic Question-Answering Dataset for **Question generation** by just prepending the *answer* to the context* |
|
|
|
## Details of T5 |
|
|
|
The **Ara-T5** model was presented in [AraT5: Text-to-Text Transformers for Arabic Language Generation](https://arxiv.org/abs/2109.12068) by *El Moatez Billah Nagoudi, AbdelRahim Elmadany, Muhammad Abdul-Mageed* in Here the abstract: |
|
|
|
Transfer learning with a unified Transformer framework (T5) that converts all language problems into a text-to-text format was recently proposed as a simple and effective transfer learning approach. Although a multilingual version of the T5 model (mT5) was also introduced, it is not clear how well it can fare on non-English tasks involving diverse data. To investigate this question, we apply mT5 on a language with a wide variety of dialects--Arabic. For evaluation, we introduce a novel benchmark for ARabic language GENeration (ARGEN), covering seven important tasks. For model comparison, we pre-train three powerful Arabic T5-style models and evaluate them on ARGEN. Although pre-trained with ~49 less data, our new models perform significantly better than mT5 on all ARGEN tasks (in 52 out of 59 test sets) and set several new SOTAs. Our models also establish new SOTA on the recently-proposed, large Arabic language understanding evaluation benchmark ARLUE (Abdul-Mageed et al., 2021). Our new models are publicly available. We also link to ARGEN datasets through our repository |
|
|
|
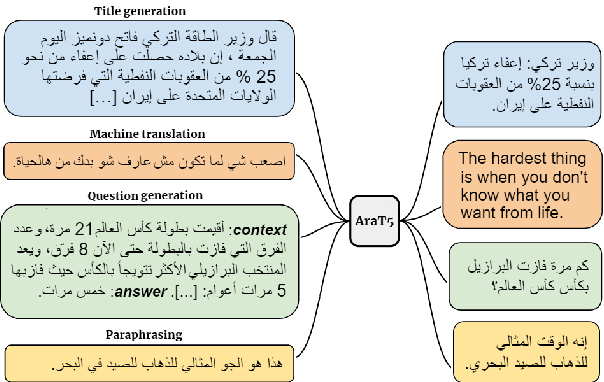 |
|
|
|
|
|
## Model in Action 🚀 |
|
```python |
|
from transformers import AutoTokenizer,AutoModelForSeq2SeqLM |
|
|
|
model = AutoModelForSeq2SeqLM.from_pretrained("Mihakram/Arabic_Question_Generation") |
|
tokenizer = AutoTokenizer.from_pretrained("Mihakram/Arabic_Question_Generation") |
|
|
|
def get_question(context,answer): |
|
text="context: " +context + " " + "answer: " + answer + " </s>" |
|
text_encoding = tokenizer.encode_plus( |
|
text,return_tensors="pt" |
|
) |
|
model.eval() |
|
output = model.generate( |
|
input_ids=text_encoding['input_ids'], |
|
attention_mask=text_encoding['attention_mask'], |
|
max_length=64, |
|
num_beams=5, |
|
num_return_sequences=1 |
|
) |
|
|
|
preds = [ |
|
tokenizer.decode(gen_id,skip_special_tokens=True,clean_up_tokenization_spaces=True) |
|
for gen_id in generated_ids |
|
] |
|
return tokenizer.decode(output[0],skip_special_tokens=True,clean_up_tokenization_spaces=True) |
|
|
|
context="الثورة الجزائرية أو ثورة المليون شهيد، اندلعت في 1 نوفمبر 1954 ضد المستعمر الفرنسي ودامت 7 سنوات ونصف. استشهد فيها أكثر من مليون ونصف مليون جزائري" |
|
answer =" 7 سنوات ونصف" |
|
|
|
get_question(answer,context) |
|
|
|
#output : question="كم استمرت الثورة الجزائرية؟ " |
|
|
|
``` |
|
|
|
## Citation |
|
If you want to cite this model you can use this: |
|
|
|
```bibtex |
|
@misc{Mihakram/, |
|
title={}, |
|
author={Mihoubi, Ibrir}, |
|
publisher={Hugging Face}, |
|
journal={Hugging Face Hub}, |
|
howpublished={\url{https://huggingface.co/}}, |
|
year={2022} |
|
} |
|
``` |
|
|
|
> Created by [LinkedIn]() | [LinkedIn](https://www.linkedin.com/in/mihoubi-akram/) |