Virtual Artist (InstructG2I: Synthesizing Images from Multimodal Attributed Graphs - NeurIPs 2024)
Introduction
We propose a graph context-conditioned diffusion model called InstructG2I to generate images from multimodal attributed graphs (MMAGs). InstructG2I first exploits the graph structure and multimodal information to conduct informative neighbor sampling by combining personalized page rank and re-ranking based on vision-language features. Then, a Graph-QFormer encoder adaptively encodes the graph nodes into an auxiliary set of graph prompts to guide the denoising process of diffusion. Finally, we propose graph classifier-free guidance, enabling controllable generation by varying the strength of graph guidance and multiple connected edges to a node.
Installation
conda create --name instructg2i python==3.10
conda activate instructg2i
git clone https://github.com/PeterGriffinJin/InstructG2I.git
conda install pytorch==2.0.1 torchvision==0.15.2 torchaudio==2.0.2 pytorch-cuda=11.8 -c pytorch -c nvidia
pip install -e .
Quick Start
Generate a picture called a mountain in the blue sky under Claude Monet's style.
import os
from PIL import Image
from instructg2i import InstructG2IPipeline, get_neighbor_transforms
text_prompt = 'a mountain in the blue sky' # a man playing soccer, a man playing piano
neighbor_pic_dir = 'examples/monet_pictures'
neighbor_transforms = get_neighbor_transforms(resolution=256)
pipeline = InstructG2IPipeline.from_pretrained("PeterJinGo/VirtualArtist", neighbor_num=5, device='cuda:0')
neighbor_image = [neighbor_transforms(Image.open(f'{neighbor_pic_dir}/{n_file}').convert("RGB")) for n_file in os.listdir(neighbor_pic_dir)]
image_gen = pipeline(prompt=text_prompt, neighbor_image=neighbor_image, neighbor_mask=[1] * len(neighbor_image), num_inference_steps=100).images[0]
image_gen.show()
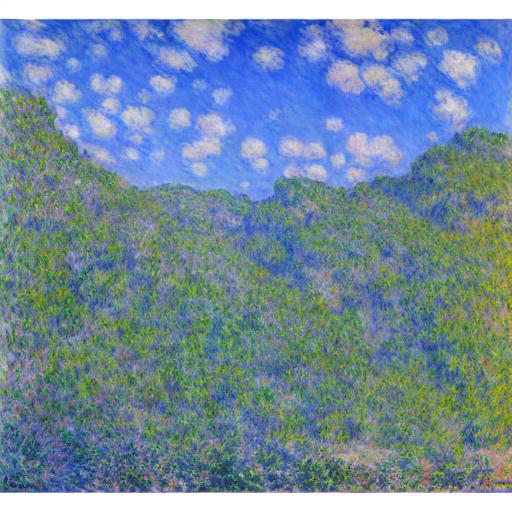
Generate a picture called a house in the snow combining the style of Claude Monet and my little brother.
import os
from PIL import Image
from instructg2i import image_grid, InstructG2IMultiGuidePipeline, get_neighbor_transforms
# load the model
pipeline = InstructG2IMultiGuidePipeline.from_pretrained("PeterJinGo/VirtualArtist", neighbor_num=5, device='cuda:0')
# configuration
text_prompt = 'a house in the snow' # a man playing soccer, a man playing piano
scale_as = [0, 3, 10]
scale_bs = [0, 5, 15]
# read the sampled neighbors
path1 = "examples/monet_pictures"
path2 = "examples/children_pictures"
neighbor_images = [[neighbor_transforms(Image.open(os.path.join(path1, n_file)).convert("RGB")) for n_file in os.listdir(path1)],
[neighbor_transforms(Image.open(os.path.join(path2, n_file)).convert("RGB")) for n_file in os.listdir(path2)]]
neighbor_masks = [[1,1,1,1,1],
[1,1,1,1,1]]
# generation
image_gens = []
neighbor_transforms = get_neighbor_transforms(resolution=256)
for scale_a in scale_as:
for scale_b in scale_bs:
graph_guidance_scales = [scale_a, scale_b]
image_gen = pipeline(prompt=text_prompt,
neighbor_images=neighbor_images,
neighbor_masks=neighbor_masks,
graph_guidance_scales=graph_guidance_scales,
num_inference_steps=100).images[0]
image_gens.append(image_gen)
res_grid = image_grid(image_gens, len(scale_as), len(scale_bs))
res_grid.show()

Download Models
Image Encoder
Create an image_encoder folder by mkdir image_encoder
, then place the files downloaded here into the folder.
InstructG2I checkpoints
The virtual artist InstructG2I checkpoint which is trained on Artwork graphs can be downloaded here or here.
from huggingface_hub import snapshot_download
snapshot_download(repo_id="PeterJinGo/VirtualArtist", local_dir=your_local_path)
The InstructG2I checkpoints for the Amazon graph and Goodreads graph can be found here.
Citations
If you find InstructG2I useful for your research and applications, please cite using this BibTeX:
@article{jin2024instructg2i,
title={InstructG2I: Synthesizing Images from Multimodal Attributed Graphs},
author={Jin, Bowen and Pang, Ziqi and Guo, Bingjun and Wang, Yu-Xiong and You, Jiaxuan and Han, Jiawei},
journal={arXiv preprint arXiv:2410.07157},
year={2024}
}
- Downloads last month
- 15