Model Details
We introduce a suite of neural language model tools for pre-training, fine-tuning SMILES-based molecular language models. Furthermore, we also provide recipes for semi-supervised recipes for fine-tuning these languages in low-data settings using Semi-supervised learning.
Enumeration-aware Molecular Transformers
Introduces contrastive learning alongside multi-task regression, and masked language modelling as pre-training objectives to inject enumeration knowledge into pre-trained language models.
a. Molecular Domain Adaptation (Contrastive Encoder-based)
i. Architecture
ii. Contrastive Learning
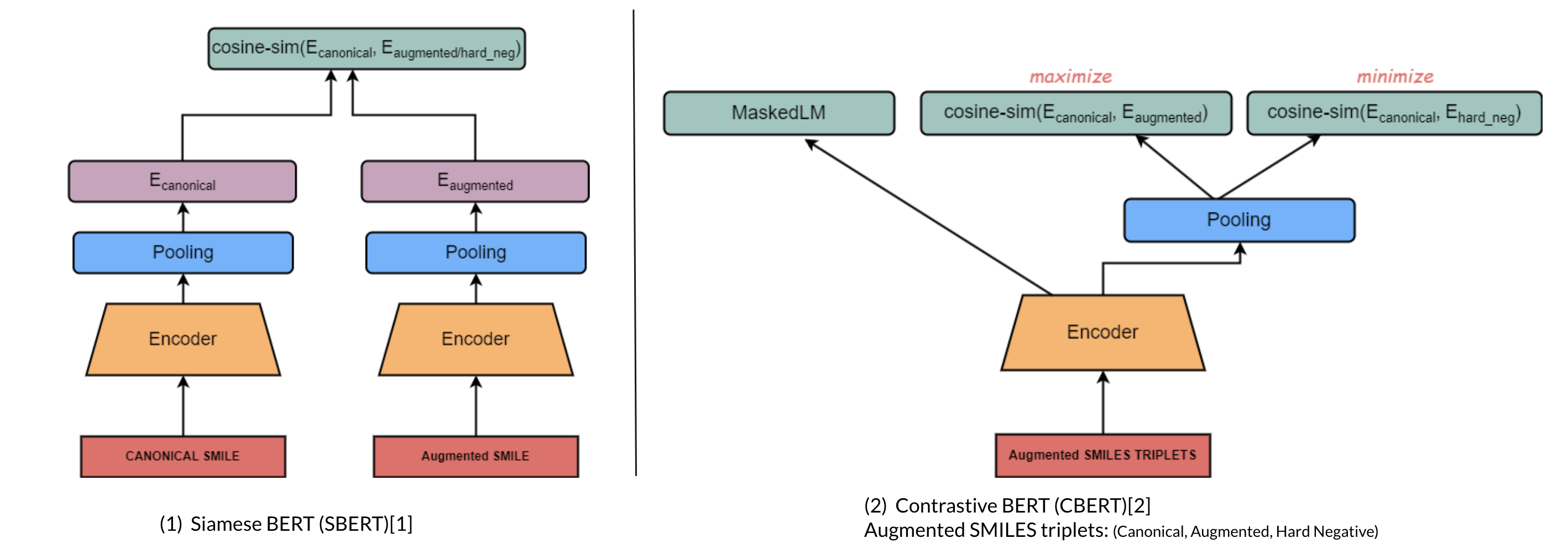
b. Canonicalization Encoder-decoder (Denoising Encoder-decoder)
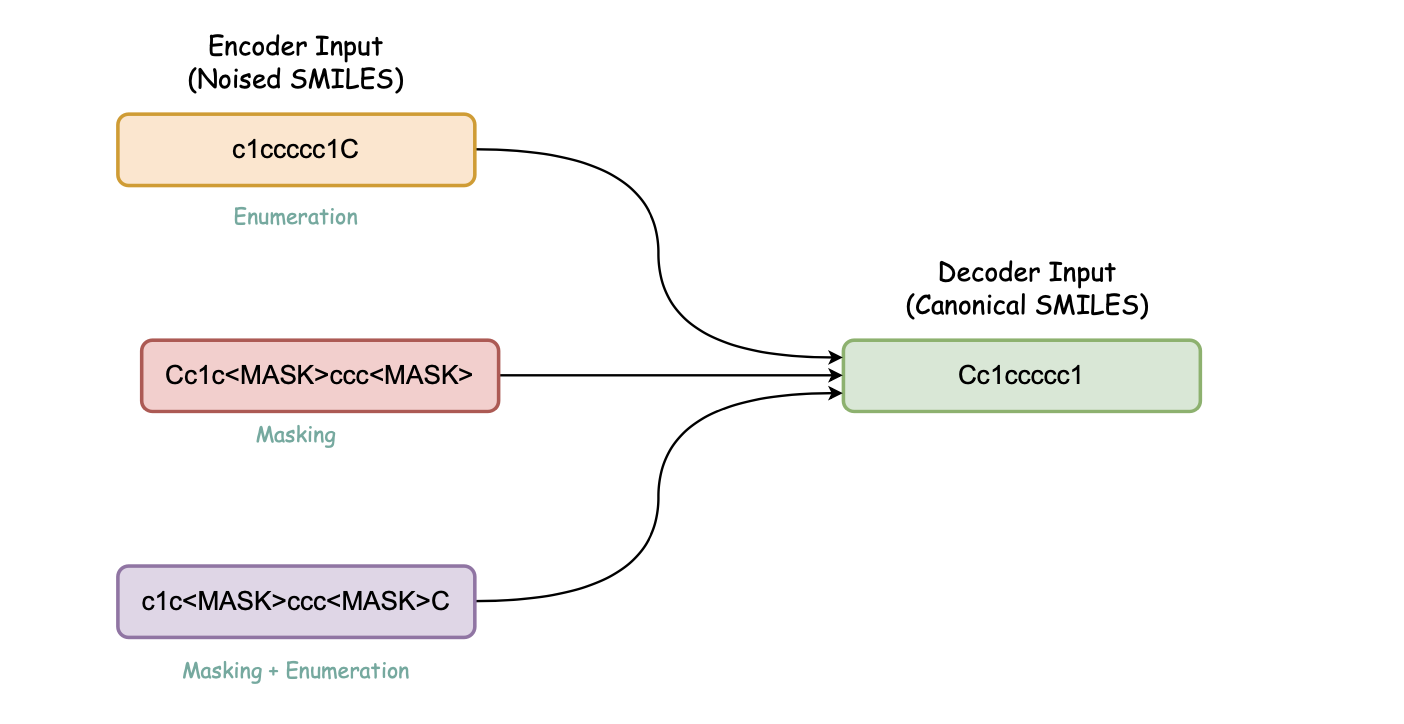
Pretraining steps for this model:
Pretrain BERT model with Masked language modeling with masked proportion set to 15% on Guacamol datasetFore more details please see our github repository.
Virtual Screening Benchmark (Github Repository)
original version presented in S. Riniker, G. Landrum, J. Cheminf., 5, 26 (2013), DOI: 10.1186/1758-2946-5-26, URL: http://www.jcheminf.com/content/5/1/26
extended version presented in S. Riniker, N. Fechner, G. Landrum, J. Chem. Inf. Model., 53, 2829, (2013), DOI: 10.1021/ci400466r, URL: http://pubs.acs.org/doi/abs/10.1021/ci400466r
Model List
Our released models are listed as following. You can import these models by using the smiles-featurizers
package or using HuggingFace's Transformers.
Model | Type | AUROC | BEDROC |
---|---|---|---|
UdS-LSV/smole-bert | Bert |
0.615 | 0.225 |
UdS-LSV/smole-bert-mtr | Bert |
0.621 | 0.262 |
UdS-LSV/smole-bart | Bart |
0.660 | 0.263 |
UdS-LSV/muv2x-simcse-smole-bart | Simcse |
0.697 | 0.270 |
UdS-LSV/siamese-smole-bert-muv-1x | SentenceTransformer |
0.673 | 0.274 |
- Downloads last month
- 13