Exllamav2 quant (exl2 / 3.75 bpw) made with ExLlamaV2 v0.1.3
Other EXL2 quants:
Quant | Model Size | lm_head |
---|---|---|
Master Files for Ultra High Quality Remasters of "Psyonic-Cetacean" 20B
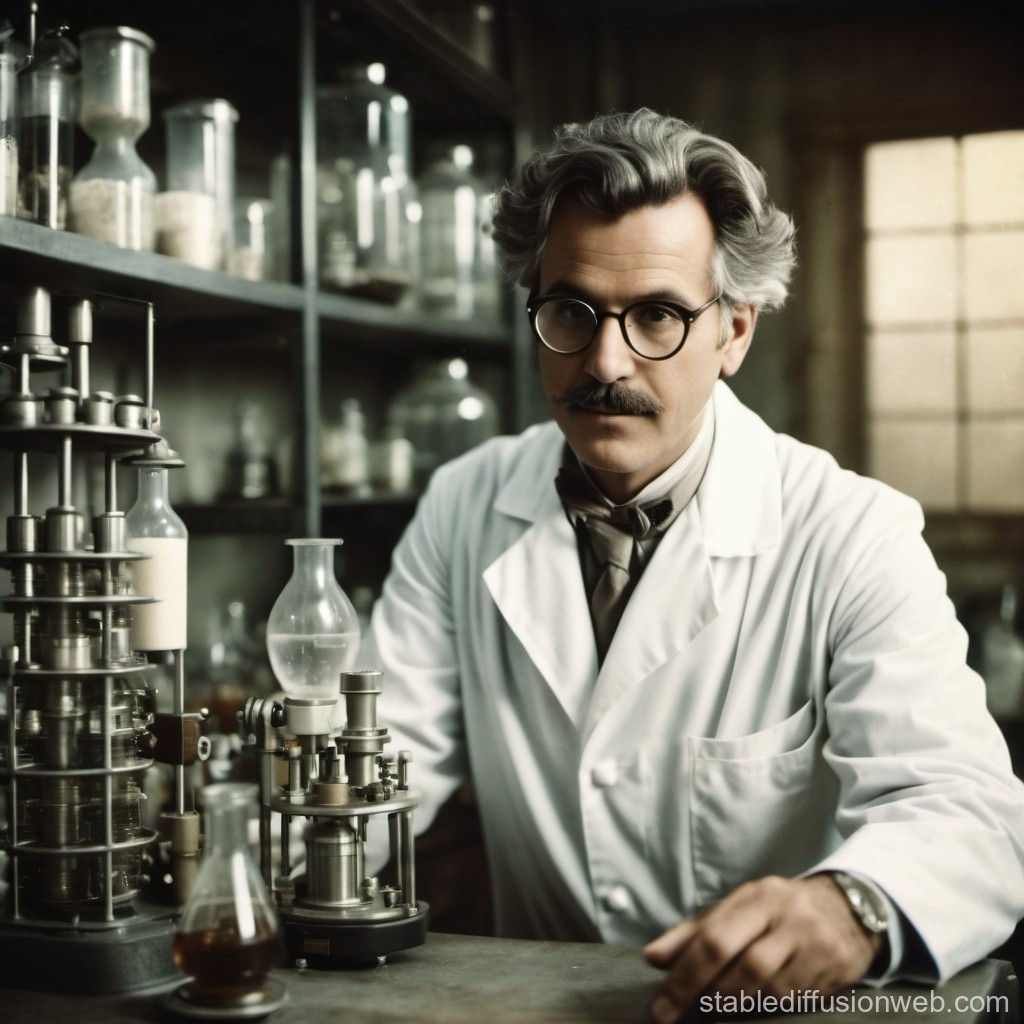
May "Space Whale" swim in the oceans of the universe forever!
This repo contains the full precision (32 bit) master files for 32 bit upscales created by "DavidAU" of:
https://huggingface.co/DavidAU/Psyonic-Cetacean-Ultra-Quality-20b-GGUF-imatrix
And
https://huggingface.co/DavidAU/Psyonic-Cetacean-Ultra-Quality-20b-GGUF
Please view either repo for details on the remaster's results, and other important infomation.
IMPORTANT NOTES For Maximum Results:
These are "final" result files of the full precision rebuild (including end result merge(s)) minus GGUF and Imatrix level upscaling / adjustments which occuring during "GGUFing" processes.
If you use these to create your own GGUFs, please use "outfile" at F32 for best results. If you use F16 this will reduce the quality by a factor of 2 or higher.
Imatrix processes should use a stable dataset(s) of at least 500 "chunks" or more. If smaller dataset(s) are used this may corrupt or reduce the quality of the Imatrix builds.
Due to the precision remaster there will be "greater" distance between each quant - both non imatrix and imatrix.
IE: The jump in quality, instruction following, "ai brainpower", nuance and output between Q4 and Q5 and likewise Q5 and Q6 will be larger than normal.
Same applies to "Imatrix" quants.
In addition there will also be differences between exact Imatrix and non-imatrix quants especially in terms of "creative uses" and/or uses where there is no "right answer".
Finally, in terms of prompts:
You may find longer prompts are no longer required and/or you may need to reduce the size of prompts in usage. This is a factor due to the precision upscale.
Doing this will ensure the quality of the upscale is maximized in the GGUFs.
/* GPTQers:
Suggest 4bit-Act32 TRUE for best results.
/* EXL2ers:
Suggest Min 4.5 BPW or higher ; 6 BPW and up is especially potent. Strongly suggest you do not reduce layer bit count, as this will affect depth and nuance. The more BPW the better.
Happy GGUFing, EXL2ing, GPTQing, AWQing, HQQing and of course "Merging".
LONG LIVE OPEN SOURCE!
DavidAU
/* Drop me a note when up, and I will link the masters to your repos.
- Downloads last month
- 6