html_url
stringlengths 48
51
| title
stringlengths 5
280
| comments
stringlengths 63
51.8k
| body
stringlengths 0
36.2k
β | comment_length
int64 16
1.52k
| text
stringlengths 163
54.1k
|
---|---|---|---|---|---|
https://github.com/huggingface/datasets/issues/514 | dataset.shuffle(keep_in_memory=True) is never allowed | These are the steps needed to fix this issue:
1. add the following check to `Dataset.shuffle`:
```python
if keep_in_memory and indices_cache_file_name is not None:
raise ValueError("Please use either `keep_in_memory` or `indices_cache_file_name` but not both.")
```
2. set `indices_cache_file_name` to `None` if `keep_in_memory` is True in the call to `select`
3. add a test with `shuffle(keep_in_memory=True)` | As of commit ef4aac2, the usage of the parameter `keep_in_memory=True` is never possible: `dataset.select(keep_in_memory=True)`
The commit added the lines
```python
# lines 994-996 in src/nlp/arrow_dataset.py
assert (
not keep_in_memory or cache_file_name is None
), "Please use either `keep_in_memory` or `cache_file_name` but not both."
```
This affects both `shuffle()` as `select()` is a sub-routine, and `map()` that has the same check.
I'd love to fix this myself, but unsure what the intention of the assert is given the rest of the logic in the function concerning `ccache_file_name` and `keep_in_memory`. | 55 | dataset.shuffle(keep_in_memory=True) is never allowed
As of commit ef4aac2, the usage of the parameter `keep_in_memory=True` is never possible: `dataset.select(keep_in_memory=True)`
The commit added the lines
```python
# lines 994-996 in src/nlp/arrow_dataset.py
assert (
not keep_in_memory or cache_file_name is None
), "Please use either `keep_in_memory` or `cache_file_name` but not both."
```
This affects both `shuffle()` as `select()` is a sub-routine, and `map()` that has the same check.
I'd love to fix this myself, but unsure what the intention of the assert is given the rest of the logic in the function concerning `ccache_file_name` and `keep_in_memory`.
These are the steps needed to fix this issue:
1. add the following check to `Dataset.shuffle`:
```python
if keep_in_memory and indices_cache_file_name is not None:
raise ValueError("Please use either `keep_in_memory` or `indices_cache_file_name` but not both.")
```
2. set `indices_cache_file_name` to `None` if `keep_in_memory` is True in the call to `select`
3. add a test with `shuffle(keep_in_memory=True)` |
https://github.com/huggingface/datasets/issues/511 | dataset.shuffle() and select() resets format. Intended? | Hi @vegarab yes feel free to open a discussion here.
This design choice was not very much thought about.
Since `dataset.select()` (like all the method without a trailing underscore) is non-destructive and returns a new dataset it has most of its properties initialized from scratch (except the table and infos).
Thinking about it I don't see a strong reason against transmitting the format from the parent dataset to its newly created child. It's probably what's expected by the user in most cases. What do you think @lhoestq?
By the way, I've been working today on a refactoring of all the samples re-ordering/selection methods (`select`, `sort`, `shuffle`, `shard`, `train_test_split`). The idea is to speed them up by a lot (like, really a lot) by working as much as possible with an indices mapping table instead of doing a deep copy of the full dataset as we've been doing currently. You can give it a look and try it here: https://github.com/huggingface/nlp/pull/513
Feedbacks are very much welcome | Calling `dataset.shuffle()` or `dataset.select()` on a dataset resets its format set by `dataset.set_format()`. Is this intended or an oversight?
When working on quite large datasets that require a lot of preprocessing I find it convenient to save the processed dataset to file using `torch.save("dataset.pt")`. Later loading the dataset object using `torch.load("dataset.pt")`, which conserves the defined format before saving.
I do shuffling and selecting (for controlling dataset size) after loading the data from .pt-file, as it's convenient whenever you train multiple models with varying sizes of the same dataset.
The obvious workaround for this is to set the format again after using `dataset.select()` or `dataset.shuffle()`.
_I guess this is more of a discussion on the design philosophy of the functions. Please let me know if this is not the right channel for these kinds of discussions or if they are not wanted at all!_
#### How to reproduce:
```python
import nlp
from transformers import T5Tokenizer
tokenizer = T5Tokenizer.from_pretrained("t5-base")
def create_features(batch):
context_encoding = tokenizer.batch_encode_plus(batch["context"])
return {"input_ids": context_encoding["input_ids"]}
dataset = nlp.load_dataset("cosmos_qa", split="train")
dataset = dataset.map(create_features, batched=True)
dataset.set_format(type="torch", columns=["input_ids"])
dataset[0]
# {'input_ids': tensor([ 1804, 3525, 1602, ... 0, 0])}
dataset = dataset.shuffle()
dataset[0]
# {'id': '3Q9(...)20', 'context': "Good Old War an (...) play ?', 'answer0': 'None of the above choices .', 'answer1': 'This person likes music and likes to see the show , they will see other bands play .', (...) 'input_ids': [1804, 3525, 1602, ... , 0, 0]}
``` | 164 | dataset.shuffle() and select() resets format. Intended?
Calling `dataset.shuffle()` or `dataset.select()` on a dataset resets its format set by `dataset.set_format()`. Is this intended or an oversight?
When working on quite large datasets that require a lot of preprocessing I find it convenient to save the processed dataset to file using `torch.save("dataset.pt")`. Later loading the dataset object using `torch.load("dataset.pt")`, which conserves the defined format before saving.
I do shuffling and selecting (for controlling dataset size) after loading the data from .pt-file, as it's convenient whenever you train multiple models with varying sizes of the same dataset.
The obvious workaround for this is to set the format again after using `dataset.select()` or `dataset.shuffle()`.
_I guess this is more of a discussion on the design philosophy of the functions. Please let me know if this is not the right channel for these kinds of discussions or if they are not wanted at all!_
#### How to reproduce:
```python
import nlp
from transformers import T5Tokenizer
tokenizer = T5Tokenizer.from_pretrained("t5-base")
def create_features(batch):
context_encoding = tokenizer.batch_encode_plus(batch["context"])
return {"input_ids": context_encoding["input_ids"]}
dataset = nlp.load_dataset("cosmos_qa", split="train")
dataset = dataset.map(create_features, batched=True)
dataset.set_format(type="torch", columns=["input_ids"])
dataset[0]
# {'input_ids': tensor([ 1804, 3525, 1602, ... 0, 0])}
dataset = dataset.shuffle()
dataset[0]
# {'id': '3Q9(...)20', 'context': "Good Old War an (...) play ?', 'answer0': 'None of the above choices .', 'answer1': 'This person likes music and likes to see the show , they will see other bands play .', (...) 'input_ids': [1804, 3525, 1602, ... , 0, 0]}
```
Hi @vegarab yes feel free to open a discussion here.
This design choice was not very much thought about.
Since `dataset.select()` (like all the method without a trailing underscore) is non-destructive and returns a new dataset it has most of its properties initialized from scratch (except the table and infos).
Thinking about it I don't see a strong reason against transmitting the format from the parent dataset to its newly created child. It's probably what's expected by the user in most cases. What do you think @lhoestq?
By the way, I've been working today on a refactoring of all the samples re-ordering/selection methods (`select`, `sort`, `shuffle`, `shard`, `train_test_split`). The idea is to speed them up by a lot (like, really a lot) by working as much as possible with an indices mapping table instead of doing a deep copy of the full dataset as we've been doing currently. You can give it a look and try it here: https://github.com/huggingface/nlp/pull/513
Feedbacks are very much welcome |
https://github.com/huggingface/datasets/issues/511 | dataset.shuffle() and select() resets format. Intended? | I think it's ok to keep the format.
If we want to have this behavior for `.map` too we just have to make sure it doesn't keep a column that's been removed. | Calling `dataset.shuffle()` or `dataset.select()` on a dataset resets its format set by `dataset.set_format()`. Is this intended or an oversight?
When working on quite large datasets that require a lot of preprocessing I find it convenient to save the processed dataset to file using `torch.save("dataset.pt")`. Later loading the dataset object using `torch.load("dataset.pt")`, which conserves the defined format before saving.
I do shuffling and selecting (for controlling dataset size) after loading the data from .pt-file, as it's convenient whenever you train multiple models with varying sizes of the same dataset.
The obvious workaround for this is to set the format again after using `dataset.select()` or `dataset.shuffle()`.
_I guess this is more of a discussion on the design philosophy of the functions. Please let me know if this is not the right channel for these kinds of discussions or if they are not wanted at all!_
#### How to reproduce:
```python
import nlp
from transformers import T5Tokenizer
tokenizer = T5Tokenizer.from_pretrained("t5-base")
def create_features(batch):
context_encoding = tokenizer.batch_encode_plus(batch["context"])
return {"input_ids": context_encoding["input_ids"]}
dataset = nlp.load_dataset("cosmos_qa", split="train")
dataset = dataset.map(create_features, batched=True)
dataset.set_format(type="torch", columns=["input_ids"])
dataset[0]
# {'input_ids': tensor([ 1804, 3525, 1602, ... 0, 0])}
dataset = dataset.shuffle()
dataset[0]
# {'id': '3Q9(...)20', 'context': "Good Old War an (...) play ?', 'answer0': 'None of the above choices .', 'answer1': 'This person likes music and likes to see the show , they will see other bands play .', (...) 'input_ids': [1804, 3525, 1602, ... , 0, 0]}
``` | 32 | dataset.shuffle() and select() resets format. Intended?
Calling `dataset.shuffle()` or `dataset.select()` on a dataset resets its format set by `dataset.set_format()`. Is this intended or an oversight?
When working on quite large datasets that require a lot of preprocessing I find it convenient to save the processed dataset to file using `torch.save("dataset.pt")`. Later loading the dataset object using `torch.load("dataset.pt")`, which conserves the defined format before saving.
I do shuffling and selecting (for controlling dataset size) after loading the data from .pt-file, as it's convenient whenever you train multiple models with varying sizes of the same dataset.
The obvious workaround for this is to set the format again after using `dataset.select()` or `dataset.shuffle()`.
_I guess this is more of a discussion on the design philosophy of the functions. Please let me know if this is not the right channel for these kinds of discussions or if they are not wanted at all!_
#### How to reproduce:
```python
import nlp
from transformers import T5Tokenizer
tokenizer = T5Tokenizer.from_pretrained("t5-base")
def create_features(batch):
context_encoding = tokenizer.batch_encode_plus(batch["context"])
return {"input_ids": context_encoding["input_ids"]}
dataset = nlp.load_dataset("cosmos_qa", split="train")
dataset = dataset.map(create_features, batched=True)
dataset.set_format(type="torch", columns=["input_ids"])
dataset[0]
# {'input_ids': tensor([ 1804, 3525, 1602, ... 0, 0])}
dataset = dataset.shuffle()
dataset[0]
# {'id': '3Q9(...)20', 'context': "Good Old War an (...) play ?', 'answer0': 'None of the above choices .', 'answer1': 'This person likes music and likes to see the show , they will see other bands play .', (...) 'input_ids': [1804, 3525, 1602, ... , 0, 0]}
```
I think it's ok to keep the format.
If we want to have this behavior for `.map` too we just have to make sure it doesn't keep a column that's been removed. |
https://github.com/huggingface/datasets/issues/511 | dataset.shuffle() and select() resets format. Intended? | Since datasets 1.0.0 the format is not reset anymore.
Closing this one, but feel free to re-open if you have other questions | Calling `dataset.shuffle()` or `dataset.select()` on a dataset resets its format set by `dataset.set_format()`. Is this intended or an oversight?
When working on quite large datasets that require a lot of preprocessing I find it convenient to save the processed dataset to file using `torch.save("dataset.pt")`. Later loading the dataset object using `torch.load("dataset.pt")`, which conserves the defined format before saving.
I do shuffling and selecting (for controlling dataset size) after loading the data from .pt-file, as it's convenient whenever you train multiple models with varying sizes of the same dataset.
The obvious workaround for this is to set the format again after using `dataset.select()` or `dataset.shuffle()`.
_I guess this is more of a discussion on the design philosophy of the functions. Please let me know if this is not the right channel for these kinds of discussions or if they are not wanted at all!_
#### How to reproduce:
```python
import nlp
from transformers import T5Tokenizer
tokenizer = T5Tokenizer.from_pretrained("t5-base")
def create_features(batch):
context_encoding = tokenizer.batch_encode_plus(batch["context"])
return {"input_ids": context_encoding["input_ids"]}
dataset = nlp.load_dataset("cosmos_qa", split="train")
dataset = dataset.map(create_features, batched=True)
dataset.set_format(type="torch", columns=["input_ids"])
dataset[0]
# {'input_ids': tensor([ 1804, 3525, 1602, ... 0, 0])}
dataset = dataset.shuffle()
dataset[0]
# {'id': '3Q9(...)20', 'context': "Good Old War an (...) play ?', 'answer0': 'None of the above choices .', 'answer1': 'This person likes music and likes to see the show , they will see other bands play .', (...) 'input_ids': [1804, 3525, 1602, ... , 0, 0]}
``` | 22 | dataset.shuffle() and select() resets format. Intended?
Calling `dataset.shuffle()` or `dataset.select()` on a dataset resets its format set by `dataset.set_format()`. Is this intended or an oversight?
When working on quite large datasets that require a lot of preprocessing I find it convenient to save the processed dataset to file using `torch.save("dataset.pt")`. Later loading the dataset object using `torch.load("dataset.pt")`, which conserves the defined format before saving.
I do shuffling and selecting (for controlling dataset size) after loading the data from .pt-file, as it's convenient whenever you train multiple models with varying sizes of the same dataset.
The obvious workaround for this is to set the format again after using `dataset.select()` or `dataset.shuffle()`.
_I guess this is more of a discussion on the design philosophy of the functions. Please let me know if this is not the right channel for these kinds of discussions or if they are not wanted at all!_
#### How to reproduce:
```python
import nlp
from transformers import T5Tokenizer
tokenizer = T5Tokenizer.from_pretrained("t5-base")
def create_features(batch):
context_encoding = tokenizer.batch_encode_plus(batch["context"])
return {"input_ids": context_encoding["input_ids"]}
dataset = nlp.load_dataset("cosmos_qa", split="train")
dataset = dataset.map(create_features, batched=True)
dataset.set_format(type="torch", columns=["input_ids"])
dataset[0]
# {'input_ids': tensor([ 1804, 3525, 1602, ... 0, 0])}
dataset = dataset.shuffle()
dataset[0]
# {'id': '3Q9(...)20', 'context': "Good Old War an (...) play ?', 'answer0': 'None of the above choices .', 'answer1': 'This person likes music and likes to see the show , they will see other bands play .', (...) 'input_ids': [1804, 3525, 1602, ... , 0, 0]}
```
Since datasets 1.0.0 the format is not reset anymore.
Closing this one, but feel free to re-open if you have other questions |
https://github.com/huggingface/datasets/issues/509 | Converting TensorFlow dataset example | Do you want to convert a dataset script to the tfds format ?
If so, we currently have a comversion script nlp/commands/convert.py but it is a conversion script that goes from tfds to nlp.
I think it shouldn't be too hard to do the changes in reverse (at some manual adjustments).
If you manage to make it work in reverse, feel free to open a PR to share it with the community :) | Hi,
I want to use TensorFlow datasets with this repo, I noticed you made some conversion script,
can you give a simple example of using it?
Thanks
| 73 | Converting TensorFlow dataset example
Hi,
I want to use TensorFlow datasets with this repo, I noticed you made some conversion script,
can you give a simple example of using it?
Thanks
Do you want to convert a dataset script to the tfds format ?
If so, we currently have a comversion script nlp/commands/convert.py but it is a conversion script that goes from tfds to nlp.
I think it shouldn't be too hard to do the changes in reverse (at some manual adjustments).
If you manage to make it work in reverse, feel free to open a PR to share it with the community :) |
https://github.com/huggingface/datasets/issues/508 | TypeError: Receiver() takes no arguments | Which version of Apache Beam do you have (can you copy your full environment info here)? | I am trying to load a wikipedia data set
```
import nlp
from nlp import load_dataset
dataset = load_dataset("wikipedia", "20200501.en", split="train", cache_dir=data_path, beam_runner='DirectRunner')
#dataset = load_dataset('wikipedia', '20200501.sv', cache_dir=data_path, beam_runner='DirectRunner')
```
This fails in the apache beam runner.
```
Traceback (most recent call last):
File "D:/ML/wikiembedding/gpt2_sv.py", line 36, in <module>
dataset = load_dataset("wikipedia", "20200501.en", split="train", cache_dir=my_cache_dir, beam_runner='DirectRunner')
File "C:\Users\seto\AppData\Local\Programs\Python\Python38\lib\site-packages\nlp\load.py", line 548, in load_dataset
builder_instance.download_and_prepare(
File "C:\Users\seto\AppData\Local\Programs\Python\Python38\lib\site-packages\nlp\builder.py", line 462, in download_and_prepare
self._download_and_prepare(
File "C:\Users\seto\AppData\Local\Programs\Python\Python38\lib\site-packages\nlp\builder.py", line 969, in _download_and_prepare
pipeline_results = pipeline.run()
File "C:\Users\seto\AppData\Local\Programs\Python\Python38\lib\site-packages\apache_beam\pipeline.py", line 534, in run
return self.runner.run_pipeline(self, self._options)
....
File "C:\Users\seto\AppData\Local\Programs\Python\Python38\lib\site-packages\apache_beam\runners\worker\bundle_processor.py", line 218, in process_encoded
self.output(decoded_value)
File "C:\Users\seto\AppData\Local\Programs\Python\Python38\lib\site-packages\apache_beam\runners\worker\operations.py", line 332, in output
cython.cast(Receiver, self.receivers[output_index]).receive(windowed_value)
File "C:\Users\seto\AppData\Local\Programs\Python\Python38\lib\site-packages\Cython\Shadow.py", line 167, in cast
return type(*args)
TypeError: Receiver() takes no arguments
```
This is run on a Windows 10 machine with python 3.8. I get the same error loading the swedish wikipedia dump. | 16 | TypeError: Receiver() takes no arguments
I am trying to load a wikipedia data set
```
import nlp
from nlp import load_dataset
dataset = load_dataset("wikipedia", "20200501.en", split="train", cache_dir=data_path, beam_runner='DirectRunner')
#dataset = load_dataset('wikipedia', '20200501.sv', cache_dir=data_path, beam_runner='DirectRunner')
```
This fails in the apache beam runner.
```
Traceback (most recent call last):
File "D:/ML/wikiembedding/gpt2_sv.py", line 36, in <module>
dataset = load_dataset("wikipedia", "20200501.en", split="train", cache_dir=my_cache_dir, beam_runner='DirectRunner')
File "C:\Users\seto\AppData\Local\Programs\Python\Python38\lib\site-packages\nlp\load.py", line 548, in load_dataset
builder_instance.download_and_prepare(
File "C:\Users\seto\AppData\Local\Programs\Python\Python38\lib\site-packages\nlp\builder.py", line 462, in download_and_prepare
self._download_and_prepare(
File "C:\Users\seto\AppData\Local\Programs\Python\Python38\lib\site-packages\nlp\builder.py", line 969, in _download_and_prepare
pipeline_results = pipeline.run()
File "C:\Users\seto\AppData\Local\Programs\Python\Python38\lib\site-packages\apache_beam\pipeline.py", line 534, in run
return self.runner.run_pipeline(self, self._options)
....
File "C:\Users\seto\AppData\Local\Programs\Python\Python38\lib\site-packages\apache_beam\runners\worker\bundle_processor.py", line 218, in process_encoded
self.output(decoded_value)
File "C:\Users\seto\AppData\Local\Programs\Python\Python38\lib\site-packages\apache_beam\runners\worker\operations.py", line 332, in output
cython.cast(Receiver, self.receivers[output_index]).receive(windowed_value)
File "C:\Users\seto\AppData\Local\Programs\Python\Python38\lib\site-packages\Cython\Shadow.py", line 167, in cast
return type(*args)
TypeError: Receiver() takes no arguments
```
This is run on a Windows 10 machine with python 3.8. I get the same error loading the swedish wikipedia dump.
Which version of Apache Beam do you have (can you copy your full environment info here)? |
https://github.com/huggingface/datasets/issues/508 | TypeError: Receiver() takes no arguments | apache-beam==2.23.0
nlp==0.4.0
For me this was resolved by running the same python script on Linux (or really WSL). | I am trying to load a wikipedia data set
```
import nlp
from nlp import load_dataset
dataset = load_dataset("wikipedia", "20200501.en", split="train", cache_dir=data_path, beam_runner='DirectRunner')
#dataset = load_dataset('wikipedia', '20200501.sv', cache_dir=data_path, beam_runner='DirectRunner')
```
This fails in the apache beam runner.
```
Traceback (most recent call last):
File "D:/ML/wikiembedding/gpt2_sv.py", line 36, in <module>
dataset = load_dataset("wikipedia", "20200501.en", split="train", cache_dir=my_cache_dir, beam_runner='DirectRunner')
File "C:\Users\seto\AppData\Local\Programs\Python\Python38\lib\site-packages\nlp\load.py", line 548, in load_dataset
builder_instance.download_and_prepare(
File "C:\Users\seto\AppData\Local\Programs\Python\Python38\lib\site-packages\nlp\builder.py", line 462, in download_and_prepare
self._download_and_prepare(
File "C:\Users\seto\AppData\Local\Programs\Python\Python38\lib\site-packages\nlp\builder.py", line 969, in _download_and_prepare
pipeline_results = pipeline.run()
File "C:\Users\seto\AppData\Local\Programs\Python\Python38\lib\site-packages\apache_beam\pipeline.py", line 534, in run
return self.runner.run_pipeline(self, self._options)
....
File "C:\Users\seto\AppData\Local\Programs\Python\Python38\lib\site-packages\apache_beam\runners\worker\bundle_processor.py", line 218, in process_encoded
self.output(decoded_value)
File "C:\Users\seto\AppData\Local\Programs\Python\Python38\lib\site-packages\apache_beam\runners\worker\operations.py", line 332, in output
cython.cast(Receiver, self.receivers[output_index]).receive(windowed_value)
File "C:\Users\seto\AppData\Local\Programs\Python\Python38\lib\site-packages\Cython\Shadow.py", line 167, in cast
return type(*args)
TypeError: Receiver() takes no arguments
```
This is run on a Windows 10 machine with python 3.8. I get the same error loading the swedish wikipedia dump. | 18 | TypeError: Receiver() takes no arguments
I am trying to load a wikipedia data set
```
import nlp
from nlp import load_dataset
dataset = load_dataset("wikipedia", "20200501.en", split="train", cache_dir=data_path, beam_runner='DirectRunner')
#dataset = load_dataset('wikipedia', '20200501.sv', cache_dir=data_path, beam_runner='DirectRunner')
```
This fails in the apache beam runner.
```
Traceback (most recent call last):
File "D:/ML/wikiembedding/gpt2_sv.py", line 36, in <module>
dataset = load_dataset("wikipedia", "20200501.en", split="train", cache_dir=my_cache_dir, beam_runner='DirectRunner')
File "C:\Users\seto\AppData\Local\Programs\Python\Python38\lib\site-packages\nlp\load.py", line 548, in load_dataset
builder_instance.download_and_prepare(
File "C:\Users\seto\AppData\Local\Programs\Python\Python38\lib\site-packages\nlp\builder.py", line 462, in download_and_prepare
self._download_and_prepare(
File "C:\Users\seto\AppData\Local\Programs\Python\Python38\lib\site-packages\nlp\builder.py", line 969, in _download_and_prepare
pipeline_results = pipeline.run()
File "C:\Users\seto\AppData\Local\Programs\Python\Python38\lib\site-packages\apache_beam\pipeline.py", line 534, in run
return self.runner.run_pipeline(self, self._options)
....
File "C:\Users\seto\AppData\Local\Programs\Python\Python38\lib\site-packages\apache_beam\runners\worker\bundle_processor.py", line 218, in process_encoded
self.output(decoded_value)
File "C:\Users\seto\AppData\Local\Programs\Python\Python38\lib\site-packages\apache_beam\runners\worker\operations.py", line 332, in output
cython.cast(Receiver, self.receivers[output_index]).receive(windowed_value)
File "C:\Users\seto\AppData\Local\Programs\Python\Python38\lib\site-packages\Cython\Shadow.py", line 167, in cast
return type(*args)
TypeError: Receiver() takes no arguments
```
This is run on a Windows 10 machine with python 3.8. I get the same error loading the swedish wikipedia dump.
apache-beam==2.23.0
nlp==0.4.0
For me this was resolved by running the same python script on Linux (or really WSL). |
https://github.com/huggingface/datasets/issues/508 | TypeError: Receiver() takes no arguments | Do you manage to run a dummy beam pipeline with python on windows ?
You can test a dummy pipeline with [this code](https://github.com/apache/beam/blob/master/sdks/python/apache_beam/examples/wordcount_minimal.py)
If you get the same error, it means that the issue comes from apache beam.
Otherwise we'll investigate what went wrong here | I am trying to load a wikipedia data set
```
import nlp
from nlp import load_dataset
dataset = load_dataset("wikipedia", "20200501.en", split="train", cache_dir=data_path, beam_runner='DirectRunner')
#dataset = load_dataset('wikipedia', '20200501.sv', cache_dir=data_path, beam_runner='DirectRunner')
```
This fails in the apache beam runner.
```
Traceback (most recent call last):
File "D:/ML/wikiembedding/gpt2_sv.py", line 36, in <module>
dataset = load_dataset("wikipedia", "20200501.en", split="train", cache_dir=my_cache_dir, beam_runner='DirectRunner')
File "C:\Users\seto\AppData\Local\Programs\Python\Python38\lib\site-packages\nlp\load.py", line 548, in load_dataset
builder_instance.download_and_prepare(
File "C:\Users\seto\AppData\Local\Programs\Python\Python38\lib\site-packages\nlp\builder.py", line 462, in download_and_prepare
self._download_and_prepare(
File "C:\Users\seto\AppData\Local\Programs\Python\Python38\lib\site-packages\nlp\builder.py", line 969, in _download_and_prepare
pipeline_results = pipeline.run()
File "C:\Users\seto\AppData\Local\Programs\Python\Python38\lib\site-packages\apache_beam\pipeline.py", line 534, in run
return self.runner.run_pipeline(self, self._options)
....
File "C:\Users\seto\AppData\Local\Programs\Python\Python38\lib\site-packages\apache_beam\runners\worker\bundle_processor.py", line 218, in process_encoded
self.output(decoded_value)
File "C:\Users\seto\AppData\Local\Programs\Python\Python38\lib\site-packages\apache_beam\runners\worker\operations.py", line 332, in output
cython.cast(Receiver, self.receivers[output_index]).receive(windowed_value)
File "C:\Users\seto\AppData\Local\Programs\Python\Python38\lib\site-packages\Cython\Shadow.py", line 167, in cast
return type(*args)
TypeError: Receiver() takes no arguments
```
This is run on a Windows 10 machine with python 3.8. I get the same error loading the swedish wikipedia dump. | 45 | TypeError: Receiver() takes no arguments
I am trying to load a wikipedia data set
```
import nlp
from nlp import load_dataset
dataset = load_dataset("wikipedia", "20200501.en", split="train", cache_dir=data_path, beam_runner='DirectRunner')
#dataset = load_dataset('wikipedia', '20200501.sv', cache_dir=data_path, beam_runner='DirectRunner')
```
This fails in the apache beam runner.
```
Traceback (most recent call last):
File "D:/ML/wikiembedding/gpt2_sv.py", line 36, in <module>
dataset = load_dataset("wikipedia", "20200501.en", split="train", cache_dir=my_cache_dir, beam_runner='DirectRunner')
File "C:\Users\seto\AppData\Local\Programs\Python\Python38\lib\site-packages\nlp\load.py", line 548, in load_dataset
builder_instance.download_and_prepare(
File "C:\Users\seto\AppData\Local\Programs\Python\Python38\lib\site-packages\nlp\builder.py", line 462, in download_and_prepare
self._download_and_prepare(
File "C:\Users\seto\AppData\Local\Programs\Python\Python38\lib\site-packages\nlp\builder.py", line 969, in _download_and_prepare
pipeline_results = pipeline.run()
File "C:\Users\seto\AppData\Local\Programs\Python\Python38\lib\site-packages\apache_beam\pipeline.py", line 534, in run
return self.runner.run_pipeline(self, self._options)
....
File "C:\Users\seto\AppData\Local\Programs\Python\Python38\lib\site-packages\apache_beam\runners\worker\bundle_processor.py", line 218, in process_encoded
self.output(decoded_value)
File "C:\Users\seto\AppData\Local\Programs\Python\Python38\lib\site-packages\apache_beam\runners\worker\operations.py", line 332, in output
cython.cast(Receiver, self.receivers[output_index]).receive(windowed_value)
File "C:\Users\seto\AppData\Local\Programs\Python\Python38\lib\site-packages\Cython\Shadow.py", line 167, in cast
return type(*args)
TypeError: Receiver() takes no arguments
```
This is run on a Windows 10 machine with python 3.8. I get the same error loading the swedish wikipedia dump.
Do you manage to run a dummy beam pipeline with python on windows ?
You can test a dummy pipeline with [this code](https://github.com/apache/beam/blob/master/sdks/python/apache_beam/examples/wordcount_minimal.py)
If you get the same error, it means that the issue comes from apache beam.
Otherwise we'll investigate what went wrong here |
https://github.com/huggingface/datasets/issues/508 | TypeError: Receiver() takes no arguments | Still, same error, so I guess it is on apache beam then.
Thanks for the investigation. | I am trying to load a wikipedia data set
```
import nlp
from nlp import load_dataset
dataset = load_dataset("wikipedia", "20200501.en", split="train", cache_dir=data_path, beam_runner='DirectRunner')
#dataset = load_dataset('wikipedia', '20200501.sv', cache_dir=data_path, beam_runner='DirectRunner')
```
This fails in the apache beam runner.
```
Traceback (most recent call last):
File "D:/ML/wikiembedding/gpt2_sv.py", line 36, in <module>
dataset = load_dataset("wikipedia", "20200501.en", split="train", cache_dir=my_cache_dir, beam_runner='DirectRunner')
File "C:\Users\seto\AppData\Local\Programs\Python\Python38\lib\site-packages\nlp\load.py", line 548, in load_dataset
builder_instance.download_and_prepare(
File "C:\Users\seto\AppData\Local\Programs\Python\Python38\lib\site-packages\nlp\builder.py", line 462, in download_and_prepare
self._download_and_prepare(
File "C:\Users\seto\AppData\Local\Programs\Python\Python38\lib\site-packages\nlp\builder.py", line 969, in _download_and_prepare
pipeline_results = pipeline.run()
File "C:\Users\seto\AppData\Local\Programs\Python\Python38\lib\site-packages\apache_beam\pipeline.py", line 534, in run
return self.runner.run_pipeline(self, self._options)
....
File "C:\Users\seto\AppData\Local\Programs\Python\Python38\lib\site-packages\apache_beam\runners\worker\bundle_processor.py", line 218, in process_encoded
self.output(decoded_value)
File "C:\Users\seto\AppData\Local\Programs\Python\Python38\lib\site-packages\apache_beam\runners\worker\operations.py", line 332, in output
cython.cast(Receiver, self.receivers[output_index]).receive(windowed_value)
File "C:\Users\seto\AppData\Local\Programs\Python\Python38\lib\site-packages\Cython\Shadow.py", line 167, in cast
return type(*args)
TypeError: Receiver() takes no arguments
```
This is run on a Windows 10 machine with python 3.8. I get the same error loading the swedish wikipedia dump. | 16 | TypeError: Receiver() takes no arguments
I am trying to load a wikipedia data set
```
import nlp
from nlp import load_dataset
dataset = load_dataset("wikipedia", "20200501.en", split="train", cache_dir=data_path, beam_runner='DirectRunner')
#dataset = load_dataset('wikipedia', '20200501.sv', cache_dir=data_path, beam_runner='DirectRunner')
```
This fails in the apache beam runner.
```
Traceback (most recent call last):
File "D:/ML/wikiembedding/gpt2_sv.py", line 36, in <module>
dataset = load_dataset("wikipedia", "20200501.en", split="train", cache_dir=my_cache_dir, beam_runner='DirectRunner')
File "C:\Users\seto\AppData\Local\Programs\Python\Python38\lib\site-packages\nlp\load.py", line 548, in load_dataset
builder_instance.download_and_prepare(
File "C:\Users\seto\AppData\Local\Programs\Python\Python38\lib\site-packages\nlp\builder.py", line 462, in download_and_prepare
self._download_and_prepare(
File "C:\Users\seto\AppData\Local\Programs\Python\Python38\lib\site-packages\nlp\builder.py", line 969, in _download_and_prepare
pipeline_results = pipeline.run()
File "C:\Users\seto\AppData\Local\Programs\Python\Python38\lib\site-packages\apache_beam\pipeline.py", line 534, in run
return self.runner.run_pipeline(self, self._options)
....
File "C:\Users\seto\AppData\Local\Programs\Python\Python38\lib\site-packages\apache_beam\runners\worker\bundle_processor.py", line 218, in process_encoded
self.output(decoded_value)
File "C:\Users\seto\AppData\Local\Programs\Python\Python38\lib\site-packages\apache_beam\runners\worker\operations.py", line 332, in output
cython.cast(Receiver, self.receivers[output_index]).receive(windowed_value)
File "C:\Users\seto\AppData\Local\Programs\Python\Python38\lib\site-packages\Cython\Shadow.py", line 167, in cast
return type(*args)
TypeError: Receiver() takes no arguments
```
This is run on a Windows 10 machine with python 3.8. I get the same error loading the swedish wikipedia dump.
Still, same error, so I guess it is on apache beam then.
Thanks for the investigation. |
https://github.com/huggingface/datasets/issues/508 | TypeError: Receiver() takes no arguments | Thanks for trying
Let us know if you find clues of what caused this issue, or if you find a fix | I am trying to load a wikipedia data set
```
import nlp
from nlp import load_dataset
dataset = load_dataset("wikipedia", "20200501.en", split="train", cache_dir=data_path, beam_runner='DirectRunner')
#dataset = load_dataset('wikipedia', '20200501.sv', cache_dir=data_path, beam_runner='DirectRunner')
```
This fails in the apache beam runner.
```
Traceback (most recent call last):
File "D:/ML/wikiembedding/gpt2_sv.py", line 36, in <module>
dataset = load_dataset("wikipedia", "20200501.en", split="train", cache_dir=my_cache_dir, beam_runner='DirectRunner')
File "C:\Users\seto\AppData\Local\Programs\Python\Python38\lib\site-packages\nlp\load.py", line 548, in load_dataset
builder_instance.download_and_prepare(
File "C:\Users\seto\AppData\Local\Programs\Python\Python38\lib\site-packages\nlp\builder.py", line 462, in download_and_prepare
self._download_and_prepare(
File "C:\Users\seto\AppData\Local\Programs\Python\Python38\lib\site-packages\nlp\builder.py", line 969, in _download_and_prepare
pipeline_results = pipeline.run()
File "C:\Users\seto\AppData\Local\Programs\Python\Python38\lib\site-packages\apache_beam\pipeline.py", line 534, in run
return self.runner.run_pipeline(self, self._options)
....
File "C:\Users\seto\AppData\Local\Programs\Python\Python38\lib\site-packages\apache_beam\runners\worker\bundle_processor.py", line 218, in process_encoded
self.output(decoded_value)
File "C:\Users\seto\AppData\Local\Programs\Python\Python38\lib\site-packages\apache_beam\runners\worker\operations.py", line 332, in output
cython.cast(Receiver, self.receivers[output_index]).receive(windowed_value)
File "C:\Users\seto\AppData\Local\Programs\Python\Python38\lib\site-packages\Cython\Shadow.py", line 167, in cast
return type(*args)
TypeError: Receiver() takes no arguments
```
This is run on a Windows 10 machine with python 3.8. I get the same error loading the swedish wikipedia dump. | 21 | TypeError: Receiver() takes no arguments
I am trying to load a wikipedia data set
```
import nlp
from nlp import load_dataset
dataset = load_dataset("wikipedia", "20200501.en", split="train", cache_dir=data_path, beam_runner='DirectRunner')
#dataset = load_dataset('wikipedia', '20200501.sv', cache_dir=data_path, beam_runner='DirectRunner')
```
This fails in the apache beam runner.
```
Traceback (most recent call last):
File "D:/ML/wikiembedding/gpt2_sv.py", line 36, in <module>
dataset = load_dataset("wikipedia", "20200501.en", split="train", cache_dir=my_cache_dir, beam_runner='DirectRunner')
File "C:\Users\seto\AppData\Local\Programs\Python\Python38\lib\site-packages\nlp\load.py", line 548, in load_dataset
builder_instance.download_and_prepare(
File "C:\Users\seto\AppData\Local\Programs\Python\Python38\lib\site-packages\nlp\builder.py", line 462, in download_and_prepare
self._download_and_prepare(
File "C:\Users\seto\AppData\Local\Programs\Python\Python38\lib\site-packages\nlp\builder.py", line 969, in _download_and_prepare
pipeline_results = pipeline.run()
File "C:\Users\seto\AppData\Local\Programs\Python\Python38\lib\site-packages\apache_beam\pipeline.py", line 534, in run
return self.runner.run_pipeline(self, self._options)
....
File "C:\Users\seto\AppData\Local\Programs\Python\Python38\lib\site-packages\apache_beam\runners\worker\bundle_processor.py", line 218, in process_encoded
self.output(decoded_value)
File "C:\Users\seto\AppData\Local\Programs\Python\Python38\lib\site-packages\apache_beam\runners\worker\operations.py", line 332, in output
cython.cast(Receiver, self.receivers[output_index]).receive(windowed_value)
File "C:\Users\seto\AppData\Local\Programs\Python\Python38\lib\site-packages\Cython\Shadow.py", line 167, in cast
return type(*args)
TypeError: Receiver() takes no arguments
```
This is run on a Windows 10 machine with python 3.8. I get the same error loading the swedish wikipedia dump.
Thanks for trying
Let us know if you find clues of what caused this issue, or if you find a fix |
https://github.com/huggingface/datasets/issues/507 | Errors when I use | Looks like an issue with 3.0.2 transformers version. Works fine when I use "master" version of transformers. | I tried the following example code from https://huggingface.co/deepset/roberta-base-squad2 and got errors
I am using **transformers 3.0.2** code .
from transformers.pipelines import pipeline
from transformers.modeling_auto import AutoModelForQuestionAnswering
from transformers.tokenization_auto import AutoTokenizer
model_name = "deepset/roberta-base-squad2"
nlp = pipeline('question-answering', model=model_name, tokenizer=model_name)
QA_input = {
'question': 'Why is model conversion important?',
'context': 'The option to convert models between FARM and transformers gives freedom to the user and let people easily switch between frameworks.'
}
res = nlp(QA_input)
The errors are :
res = nlp(QA_input)
File ".local/lib/python3.6/site-packages/transformers/pipelines.py", line 1316, in __call__
for s, e, score in zip(starts, ends, scores)
File ".local/lib/python3.6/site-packages/transformers/pipelines.py", line 1316, in <listcomp>
for s, e, score in zip(starts, ends, scores)
KeyError: 0
| 17 | Errors when I use
I tried the following example code from https://huggingface.co/deepset/roberta-base-squad2 and got errors
I am using **transformers 3.0.2** code .
from transformers.pipelines import pipeline
from transformers.modeling_auto import AutoModelForQuestionAnswering
from transformers.tokenization_auto import AutoTokenizer
model_name = "deepset/roberta-base-squad2"
nlp = pipeline('question-answering', model=model_name, tokenizer=model_name)
QA_input = {
'question': 'Why is model conversion important?',
'context': 'The option to convert models between FARM and transformers gives freedom to the user and let people easily switch between frameworks.'
}
res = nlp(QA_input)
The errors are :
res = nlp(QA_input)
File ".local/lib/python3.6/site-packages/transformers/pipelines.py", line 1316, in __call__
for s, e, score in zip(starts, ends, scores)
File ".local/lib/python3.6/site-packages/transformers/pipelines.py", line 1316, in <listcomp>
for s, e, score in zip(starts, ends, scores)
KeyError: 0
Looks like an issue with 3.0.2 transformers version. Works fine when I use "master" version of transformers. |
https://github.com/huggingface/datasets/issues/501 | Caching doesn't work for map (non-deterministic) | Thanks for reporting !
To store the cache file, we compute a hash of the function given in `.map`, using our own hashing function.
The hash doesn't seem to stay the same over sessions for the tokenizer.
Apparently this is because of the regex at `tokenizer.pat` is not well supported by our hashing function.
I'm working on a fix | The caching functionality doesn't work reliably when tokenizing a dataset. Here's a small example to reproduce it.
```python
import nlp
import transformers
def main():
ds = nlp.load_dataset("reddit", split="train[:500]")
tokenizer = transformers.AutoTokenizer.from_pretrained("gpt2")
def convert_to_features(example_batch):
input_str = example_batch["body"]
encodings = tokenizer(input_str, add_special_tokens=True, truncation=True)
return encodings
ds = ds.map(convert_to_features, batched=True)
if __name__ == "__main__":
main()
```
Roughly 3/10 times, this example recomputes the tokenization.
Is this expected behaviour? | 59 | Caching doesn't work for map (non-deterministic)
The caching functionality doesn't work reliably when tokenizing a dataset. Here's a small example to reproduce it.
```python
import nlp
import transformers
def main():
ds = nlp.load_dataset("reddit", split="train[:500]")
tokenizer = transformers.AutoTokenizer.from_pretrained("gpt2")
def convert_to_features(example_batch):
input_str = example_batch["body"]
encodings = tokenizer(input_str, add_special_tokens=True, truncation=True)
return encodings
ds = ds.map(convert_to_features, batched=True)
if __name__ == "__main__":
main()
```
Roughly 3/10 times, this example recomputes the tokenization.
Is this expected behaviour?
Thanks for reporting !
To store the cache file, we compute a hash of the function given in `.map`, using our own hashing function.
The hash doesn't seem to stay the same over sessions for the tokenizer.
Apparently this is because of the regex at `tokenizer.pat` is not well supported by our hashing function.
I'm working on a fix |
https://github.com/huggingface/datasets/issues/501 | Caching doesn't work for map (non-deterministic) | Hi. I believe the fix was for the nlp library. Is there a solution to handle compiled regex expressions in .map() with the caching. I want to run a simple regex pattern on a big dataset, but I am running into the issue of compiled expression not being cached.
Instead of opening a new issue, I thought I would put my query here. Let me know if a new issue would be more suitable. Thanks | The caching functionality doesn't work reliably when tokenizing a dataset. Here's a small example to reproduce it.
```python
import nlp
import transformers
def main():
ds = nlp.load_dataset("reddit", split="train[:500]")
tokenizer = transformers.AutoTokenizer.from_pretrained("gpt2")
def convert_to_features(example_batch):
input_str = example_batch["body"]
encodings = tokenizer(input_str, add_special_tokens=True, truncation=True)
return encodings
ds = ds.map(convert_to_features, batched=True)
if __name__ == "__main__":
main()
```
Roughly 3/10 times, this example recomputes the tokenization.
Is this expected behaviour? | 75 | Caching doesn't work for map (non-deterministic)
The caching functionality doesn't work reliably when tokenizing a dataset. Here's a small example to reproduce it.
```python
import nlp
import transformers
def main():
ds = nlp.load_dataset("reddit", split="train[:500]")
tokenizer = transformers.AutoTokenizer.from_pretrained("gpt2")
def convert_to_features(example_batch):
input_str = example_batch["body"]
encodings = tokenizer(input_str, add_special_tokens=True, truncation=True)
return encodings
ds = ds.map(convert_to_features, batched=True)
if __name__ == "__main__":
main()
```
Roughly 3/10 times, this example recomputes the tokenization.
Is this expected behaviour?
Hi. I believe the fix was for the nlp library. Is there a solution to handle compiled regex expressions in .map() with the caching. I want to run a simple regex pattern on a big dataset, but I am running into the issue of compiled expression not being cached.
Instead of opening a new issue, I thought I would put my query here. Let me know if a new issue would be more suitable. Thanks |
https://github.com/huggingface/datasets/issues/501 | Caching doesn't work for map (non-deterministic) | Hi @MaveriQ! This fix is also included in the `datasets` library. Can you provide a reproducer? | The caching functionality doesn't work reliably when tokenizing a dataset. Here's a small example to reproduce it.
```python
import nlp
import transformers
def main():
ds = nlp.load_dataset("reddit", split="train[:500]")
tokenizer = transformers.AutoTokenizer.from_pretrained("gpt2")
def convert_to_features(example_batch):
input_str = example_batch["body"]
encodings = tokenizer(input_str, add_special_tokens=True, truncation=True)
return encodings
ds = ds.map(convert_to_features, batched=True)
if __name__ == "__main__":
main()
```
Roughly 3/10 times, this example recomputes the tokenization.
Is this expected behaviour? | 16 | Caching doesn't work for map (non-deterministic)
The caching functionality doesn't work reliably when tokenizing a dataset. Here's a small example to reproduce it.
```python
import nlp
import transformers
def main():
ds = nlp.load_dataset("reddit", split="train[:500]")
tokenizer = transformers.AutoTokenizer.from_pretrained("gpt2")
def convert_to_features(example_batch):
input_str = example_batch["body"]
encodings = tokenizer(input_str, add_special_tokens=True, truncation=True)
return encodings
ds = ds.map(convert_to_features, batched=True)
if __name__ == "__main__":
main()
```
Roughly 3/10 times, this example recomputes the tokenization.
Is this expected behaviour?
Hi @MaveriQ! This fix is also included in the `datasets` library. Can you provide a reproducer? |
https://github.com/huggingface/datasets/issues/492 | nlp.Features does not distinguish between nullable and non-nullable types in PyArrow schema | In 0.4.0, the assertion in `concatenate_datasets ` is on the features, and not the schema.
Could you try to update `nlp` ?
Also, since 0.4.0, you can use `dset_wikipedia.cast_(dset_books.features)` to avoid the schema cast hack. | Here's the code I'm trying to run:
```python
dset_wikipedia = nlp.load_dataset("wikipedia", "20200501.en", split="train", cache_dir=args.cache_dir)
dset_wikipedia.drop(columns=["title"])
dset_wikipedia.features.pop("title")
dset_books = nlp.load_dataset("bookcorpus", split="train", cache_dir=args.cache_dir)
dset = nlp.concatenate_datasets([dset_wikipedia, dset_books])
```
This fails because they have different schemas, despite having identical features.
```python
assert dset_wikipedia.features == dset_books.features # True
assert dset_wikipedia._data.schema == dset_books._data.schema # False
```
The Wikipedia dataset has 'text: string', while the BookCorpus dataset has 'text: string not null'. Currently I hack together a working schema match with the following line, but it would be better if this was handled in Features themselves.
```python
dset_wikipedia._data = dset_wikipedia.data.cast(dset_books._data.schema)
```
| 35 | nlp.Features does not distinguish between nullable and non-nullable types in PyArrow schema
Here's the code I'm trying to run:
```python
dset_wikipedia = nlp.load_dataset("wikipedia", "20200501.en", split="train", cache_dir=args.cache_dir)
dset_wikipedia.drop(columns=["title"])
dset_wikipedia.features.pop("title")
dset_books = nlp.load_dataset("bookcorpus", split="train", cache_dir=args.cache_dir)
dset = nlp.concatenate_datasets([dset_wikipedia, dset_books])
```
This fails because they have different schemas, despite having identical features.
```python
assert dset_wikipedia.features == dset_books.features # True
assert dset_wikipedia._data.schema == dset_books._data.schema # False
```
The Wikipedia dataset has 'text: string', while the BookCorpus dataset has 'text: string not null'. Currently I hack together a working schema match with the following line, but it would be better if this was handled in Features themselves.
```python
dset_wikipedia._data = dset_wikipedia.data.cast(dset_books._data.schema)
```
In 0.4.0, the assertion in `concatenate_datasets ` is on the features, and not the schema.
Could you try to update `nlp` ?
Also, since 0.4.0, you can use `dset_wikipedia.cast_(dset_books.features)` to avoid the schema cast hack. |
https://github.com/huggingface/datasets/issues/492 | nlp.Features does not distinguish between nullable and non-nullable types in PyArrow schema | I'm using the master branch. The assertion failure comes from the underlying `pa.concat_tables()`, which is in the pyarrow package. That method does check schemas.
Since `features.type` does not contain information about nullable vs non-nullable features, the `cast_()` method won't resolve the schema mismatch. There is information in a schema which is not stored in features. | Here's the code I'm trying to run:
```python
dset_wikipedia = nlp.load_dataset("wikipedia", "20200501.en", split="train", cache_dir=args.cache_dir)
dset_wikipedia.drop(columns=["title"])
dset_wikipedia.features.pop("title")
dset_books = nlp.load_dataset("bookcorpus", split="train", cache_dir=args.cache_dir)
dset = nlp.concatenate_datasets([dset_wikipedia, dset_books])
```
This fails because they have different schemas, despite having identical features.
```python
assert dset_wikipedia.features == dset_books.features # True
assert dset_wikipedia._data.schema == dset_books._data.schema # False
```
The Wikipedia dataset has 'text: string', while the BookCorpus dataset has 'text: string not null'. Currently I hack together a working schema match with the following line, but it would be better if this was handled in Features themselves.
```python
dset_wikipedia._data = dset_wikipedia.data.cast(dset_books._data.schema)
```
| 55 | nlp.Features does not distinguish between nullable and non-nullable types in PyArrow schema
Here's the code I'm trying to run:
```python
dset_wikipedia = nlp.load_dataset("wikipedia", "20200501.en", split="train", cache_dir=args.cache_dir)
dset_wikipedia.drop(columns=["title"])
dset_wikipedia.features.pop("title")
dset_books = nlp.load_dataset("bookcorpus", split="train", cache_dir=args.cache_dir)
dset = nlp.concatenate_datasets([dset_wikipedia, dset_books])
```
This fails because they have different schemas, despite having identical features.
```python
assert dset_wikipedia.features == dset_books.features # True
assert dset_wikipedia._data.schema == dset_books._data.schema # False
```
The Wikipedia dataset has 'text: string', while the BookCorpus dataset has 'text: string not null'. Currently I hack together a working schema match with the following line, but it would be better if this was handled in Features themselves.
```python
dset_wikipedia._data = dset_wikipedia.data.cast(dset_books._data.schema)
```
I'm using the master branch. The assertion failure comes from the underlying `pa.concat_tables()`, which is in the pyarrow package. That method does check schemas.
Since `features.type` does not contain information about nullable vs non-nullable features, the `cast_()` method won't resolve the schema mismatch. There is information in a schema which is not stored in features. |
https://github.com/huggingface/datasets/issues/492 | nlp.Features does not distinguish between nullable and non-nullable types in PyArrow schema | I'm doing a refactor of type inference in #363 . Both text fields should match after that | Here's the code I'm trying to run:
```python
dset_wikipedia = nlp.load_dataset("wikipedia", "20200501.en", split="train", cache_dir=args.cache_dir)
dset_wikipedia.drop(columns=["title"])
dset_wikipedia.features.pop("title")
dset_books = nlp.load_dataset("bookcorpus", split="train", cache_dir=args.cache_dir)
dset = nlp.concatenate_datasets([dset_wikipedia, dset_books])
```
This fails because they have different schemas, despite having identical features.
```python
assert dset_wikipedia.features == dset_books.features # True
assert dset_wikipedia._data.schema == dset_books._data.schema # False
```
The Wikipedia dataset has 'text: string', while the BookCorpus dataset has 'text: string not null'. Currently I hack together a working schema match with the following line, but it would be better if this was handled in Features themselves.
```python
dset_wikipedia._data = dset_wikipedia.data.cast(dset_books._data.schema)
```
| 17 | nlp.Features does not distinguish between nullable and non-nullable types in PyArrow schema
Here's the code I'm trying to run:
```python
dset_wikipedia = nlp.load_dataset("wikipedia", "20200501.en", split="train", cache_dir=args.cache_dir)
dset_wikipedia.drop(columns=["title"])
dset_wikipedia.features.pop("title")
dset_books = nlp.load_dataset("bookcorpus", split="train", cache_dir=args.cache_dir)
dset = nlp.concatenate_datasets([dset_wikipedia, dset_books])
```
This fails because they have different schemas, despite having identical features.
```python
assert dset_wikipedia.features == dset_books.features # True
assert dset_wikipedia._data.schema == dset_books._data.schema # False
```
The Wikipedia dataset has 'text: string', while the BookCorpus dataset has 'text: string not null'. Currently I hack together a working schema match with the following line, but it would be better if this was handled in Features themselves.
```python
dset_wikipedia._data = dset_wikipedia.data.cast(dset_books._data.schema)
```
I'm doing a refactor of type inference in #363 . Both text fields should match after that |
https://github.com/huggingface/datasets/issues/492 | nlp.Features does not distinguish between nullable and non-nullable types in PyArrow schema | It should be good now. I was able to run
```python
>>> from nlp import concatenate_datasets, load_dataset
>>>
>>> bookcorpus = load_dataset("bookcorpus", split="train")
>>> wiki = load_dataset("wikipedia", "20200501.en", split="train")
>>> wiki.remove_columns_("title") # only keep the text
>>>
>>> assert bookcorpus.features.type == wiki.features.type
>>> bert_dataset = concatenate_datasets([bookcorpus, wiki])
``` | Here's the code I'm trying to run:
```python
dset_wikipedia = nlp.load_dataset("wikipedia", "20200501.en", split="train", cache_dir=args.cache_dir)
dset_wikipedia.drop(columns=["title"])
dset_wikipedia.features.pop("title")
dset_books = nlp.load_dataset("bookcorpus", split="train", cache_dir=args.cache_dir)
dset = nlp.concatenate_datasets([dset_wikipedia, dset_books])
```
This fails because they have different schemas, despite having identical features.
```python
assert dset_wikipedia.features == dset_books.features # True
assert dset_wikipedia._data.schema == dset_books._data.schema # False
```
The Wikipedia dataset has 'text: string', while the BookCorpus dataset has 'text: string not null'. Currently I hack together a working schema match with the following line, but it would be better if this was handled in Features themselves.
```python
dset_wikipedia._data = dset_wikipedia.data.cast(dset_books._data.schema)
```
| 48 | nlp.Features does not distinguish between nullable and non-nullable types in PyArrow schema
Here's the code I'm trying to run:
```python
dset_wikipedia = nlp.load_dataset("wikipedia", "20200501.en", split="train", cache_dir=args.cache_dir)
dset_wikipedia.drop(columns=["title"])
dset_wikipedia.features.pop("title")
dset_books = nlp.load_dataset("bookcorpus", split="train", cache_dir=args.cache_dir)
dset = nlp.concatenate_datasets([dset_wikipedia, dset_books])
```
This fails because they have different schemas, despite having identical features.
```python
assert dset_wikipedia.features == dset_books.features # True
assert dset_wikipedia._data.schema == dset_books._data.schema # False
```
The Wikipedia dataset has 'text: string', while the BookCorpus dataset has 'text: string not null'. Currently I hack together a working schema match with the following line, but it would be better if this was handled in Features themselves.
```python
dset_wikipedia._data = dset_wikipedia.data.cast(dset_books._data.schema)
```
It should be good now. I was able to run
```python
>>> from nlp import concatenate_datasets, load_dataset
>>>
>>> bookcorpus = load_dataset("bookcorpus", split="train")
>>> wiki = load_dataset("wikipedia", "20200501.en", split="train")
>>> wiki.remove_columns_("title") # only keep the text
>>>
>>> assert bookcorpus.features.type == wiki.features.type
>>> bert_dataset = concatenate_datasets([bookcorpus, wiki])
``` |
https://github.com/huggingface/datasets/issues/488 | issues with downloading datasets for wmt16 and wmt19 | I found `UNv1.0.en-ru.tar.gz` here: https://conferences.unite.un.org/uncorpus/en/downloadoverview, so it can be reconstructed with:
```
wget -c https://stuncorpusprod.blob.core.windows.net/corpusfiles/UNv1.0.en-ru.tar.gz.00
wget -c https://stuncorpusprod.blob.core.windows.net/corpusfiles/UNv1.0.en-ru.tar.gz.01
wget -c https://stuncorpusprod.blob.core.windows.net/corpusfiles/UNv1.0.en-ru.tar.gz.02
cat UNv1.0.en-ru.tar.gz.0* > UNv1.0.en-ru.tar.gz
```
it has other languages as well, in case https://storage.googleapis.com/tfdataset-data/downloadataset/uncorpus/ is gone | I have encountered multiple issues while trying to:
```
import nlp
dataset = nlp.load_dataset('wmt16', 'ru-en')
metric = nlp.load_metric('wmt16')
```
1. I had to do `pip install -e ".[dev]" ` on master, currently released nlp didn't work (sorry, didn't save the error) - I went back to the released version and now it worked. So it must have been some outdated dependencies that `pip install -e ".[dev]" ` fixed.
2. it was downloading at 60kbs - almost 5 hours to get the dataset. It was downloading all pairs and not just the one I asked for.
I tried the same code with `wmt19` in parallel and it took a few secs to download and it only fetched data for the requested pair. (but it failed too, see below)
3. my machine has crushed and when I retried I got:
```
Traceback (most recent call last):
File "./download.py", line 9, in <module>
dataset = nlp.load_dataset('wmt16', 'ru-en')
File "/mnt/nvme1/code/huggingface/nlp-master/src/nlp/load.py", line 549, in load_dataset
download_config=download_config, download_mode=download_mode, ignore_verifications=ignore_verifications,
File "/mnt/nvme1/code/huggingface/nlp-master/src/nlp/builder.py", line 449, in download_and_prepare
with incomplete_dir(self._cache_dir) as tmp_data_dir:
File "/home/stas/anaconda3/envs/main/lib/python3.7/contextlib.py", line 112, in __enter__
return next(self.gen)
File "/mnt/nvme1/code/huggingface/nlp-master/src/nlp/builder.py", line 422, in incomplete_dir
os.makedirs(tmp_dir)
File "/home/stas/anaconda3/envs/main/lib/python3.7/os.py", line 221, in makedirs
mkdir(name, mode)
FileExistsError: [Errno 17] File exists: '/home/stas/.cache/huggingface/datasets/wmt16/ru-en/1.0.0/4d8269cdd971ed26984a9c0e4a158e0c7afc8135fac8fb8ee43ceecf38fd422d.incomplete'
```
it can't handle resumes. but neither allows a new start. Had to delete it manually.
4. and finally when it downloaded the dataset, it then failed to fetch the metrics:
```
Traceback (most recent call last):
File "./download.py", line 15, in <module>
metric = nlp.load_metric('wmt16')
File "/mnt/nvme1/code/huggingface/nlp-master/src/nlp/load.py", line 442, in load_metric
module_path, hash = prepare_module(path, download_config=download_config, dataset=False)
File "/mnt/nvme1/code/huggingface/nlp-master/src/nlp/load.py", line 258, in prepare_module
local_path = cached_path(file_path, download_config=download_config)
File "/mnt/nvme1/code/huggingface/nlp-master/src/nlp/utils/file_utils.py", line 198, in cached_path
local_files_only=download_config.local_files_only,
File "/mnt/nvme1/code/huggingface/nlp-master/src/nlp/utils/file_utils.py", line 356, in get_from_cache
raise ConnectionError("Couldn't reach {}".format(url))
ConnectionError: Couldn't reach https://s3.amazonaws.com/datasets.huggingface.co/nlp/metrics/wmt16/wmt16.py
```
5. If I run the same code with `wmt19`, it fails too:
```
ConnectionError: Couldn't reach https://storage.googleapis.com/tfdataset-data/downloadataset/uncorpus/UNv1.0.en-ru.tar.gz
``` | 37 | issues with downloading datasets for wmt16 and wmt19
I have encountered multiple issues while trying to:
```
import nlp
dataset = nlp.load_dataset('wmt16', 'ru-en')
metric = nlp.load_metric('wmt16')
```
1. I had to do `pip install -e ".[dev]" ` on master, currently released nlp didn't work (sorry, didn't save the error) - I went back to the released version and now it worked. So it must have been some outdated dependencies that `pip install -e ".[dev]" ` fixed.
2. it was downloading at 60kbs - almost 5 hours to get the dataset. It was downloading all pairs and not just the one I asked for.
I tried the same code with `wmt19` in parallel and it took a few secs to download and it only fetched data for the requested pair. (but it failed too, see below)
3. my machine has crushed and when I retried I got:
```
Traceback (most recent call last):
File "./download.py", line 9, in <module>
dataset = nlp.load_dataset('wmt16', 'ru-en')
File "/mnt/nvme1/code/huggingface/nlp-master/src/nlp/load.py", line 549, in load_dataset
download_config=download_config, download_mode=download_mode, ignore_verifications=ignore_verifications,
File "/mnt/nvme1/code/huggingface/nlp-master/src/nlp/builder.py", line 449, in download_and_prepare
with incomplete_dir(self._cache_dir) as tmp_data_dir:
File "/home/stas/anaconda3/envs/main/lib/python3.7/contextlib.py", line 112, in __enter__
return next(self.gen)
File "/mnt/nvme1/code/huggingface/nlp-master/src/nlp/builder.py", line 422, in incomplete_dir
os.makedirs(tmp_dir)
File "/home/stas/anaconda3/envs/main/lib/python3.7/os.py", line 221, in makedirs
mkdir(name, mode)
FileExistsError: [Errno 17] File exists: '/home/stas/.cache/huggingface/datasets/wmt16/ru-en/1.0.0/4d8269cdd971ed26984a9c0e4a158e0c7afc8135fac8fb8ee43ceecf38fd422d.incomplete'
```
it can't handle resumes. but neither allows a new start. Had to delete it manually.
4. and finally when it downloaded the dataset, it then failed to fetch the metrics:
```
Traceback (most recent call last):
File "./download.py", line 15, in <module>
metric = nlp.load_metric('wmt16')
File "/mnt/nvme1/code/huggingface/nlp-master/src/nlp/load.py", line 442, in load_metric
module_path, hash = prepare_module(path, download_config=download_config, dataset=False)
File "/mnt/nvme1/code/huggingface/nlp-master/src/nlp/load.py", line 258, in prepare_module
local_path = cached_path(file_path, download_config=download_config)
File "/mnt/nvme1/code/huggingface/nlp-master/src/nlp/utils/file_utils.py", line 198, in cached_path
local_files_only=download_config.local_files_only,
File "/mnt/nvme1/code/huggingface/nlp-master/src/nlp/utils/file_utils.py", line 356, in get_from_cache
raise ConnectionError("Couldn't reach {}".format(url))
ConnectionError: Couldn't reach https://s3.amazonaws.com/datasets.huggingface.co/nlp/metrics/wmt16/wmt16.py
```
5. If I run the same code with `wmt19`, it fails too:
```
ConnectionError: Couldn't reach https://storage.googleapis.com/tfdataset-data/downloadataset/uncorpus/UNv1.0.en-ru.tar.gz
```
I found `UNv1.0.en-ru.tar.gz` here: https://conferences.unite.un.org/uncorpus/en/downloadoverview, so it can be reconstructed with:
```
wget -c https://stuncorpusprod.blob.core.windows.net/corpusfiles/UNv1.0.en-ru.tar.gz.00
wget -c https://stuncorpusprod.blob.core.windows.net/corpusfiles/UNv1.0.en-ru.tar.gz.01
wget -c https://stuncorpusprod.blob.core.windows.net/corpusfiles/UNv1.0.en-ru.tar.gz.02
cat UNv1.0.en-ru.tar.gz.0* > UNv1.0.en-ru.tar.gz
```
it has other languages as well, in case https://storage.googleapis.com/tfdataset-data/downloadataset/uncorpus/ is gone |
https://github.com/huggingface/datasets/issues/488 | issues with downloading datasets for wmt16 and wmt19 | Further, `nlp.load_dataset('wmt19', 'ru-en')` has only the `train` and `val` datasets. `test` is missing.
Fixed locally for summarization needs, by running:
```
pip install sacrebleu
sacrebleu -t wmt19 -l ru-en --echo src > test.source
sacrebleu -t wmt19 -l ru-en --echo ref > test.target
```
h/t @sshleifer | I have encountered multiple issues while trying to:
```
import nlp
dataset = nlp.load_dataset('wmt16', 'ru-en')
metric = nlp.load_metric('wmt16')
```
1. I had to do `pip install -e ".[dev]" ` on master, currently released nlp didn't work (sorry, didn't save the error) - I went back to the released version and now it worked. So it must have been some outdated dependencies that `pip install -e ".[dev]" ` fixed.
2. it was downloading at 60kbs - almost 5 hours to get the dataset. It was downloading all pairs and not just the one I asked for.
I tried the same code with `wmt19` in parallel and it took a few secs to download and it only fetched data for the requested pair. (but it failed too, see below)
3. my machine has crushed and when I retried I got:
```
Traceback (most recent call last):
File "./download.py", line 9, in <module>
dataset = nlp.load_dataset('wmt16', 'ru-en')
File "/mnt/nvme1/code/huggingface/nlp-master/src/nlp/load.py", line 549, in load_dataset
download_config=download_config, download_mode=download_mode, ignore_verifications=ignore_verifications,
File "/mnt/nvme1/code/huggingface/nlp-master/src/nlp/builder.py", line 449, in download_and_prepare
with incomplete_dir(self._cache_dir) as tmp_data_dir:
File "/home/stas/anaconda3/envs/main/lib/python3.7/contextlib.py", line 112, in __enter__
return next(self.gen)
File "/mnt/nvme1/code/huggingface/nlp-master/src/nlp/builder.py", line 422, in incomplete_dir
os.makedirs(tmp_dir)
File "/home/stas/anaconda3/envs/main/lib/python3.7/os.py", line 221, in makedirs
mkdir(name, mode)
FileExistsError: [Errno 17] File exists: '/home/stas/.cache/huggingface/datasets/wmt16/ru-en/1.0.0/4d8269cdd971ed26984a9c0e4a158e0c7afc8135fac8fb8ee43ceecf38fd422d.incomplete'
```
it can't handle resumes. but neither allows a new start. Had to delete it manually.
4. and finally when it downloaded the dataset, it then failed to fetch the metrics:
```
Traceback (most recent call last):
File "./download.py", line 15, in <module>
metric = nlp.load_metric('wmt16')
File "/mnt/nvme1/code/huggingface/nlp-master/src/nlp/load.py", line 442, in load_metric
module_path, hash = prepare_module(path, download_config=download_config, dataset=False)
File "/mnt/nvme1/code/huggingface/nlp-master/src/nlp/load.py", line 258, in prepare_module
local_path = cached_path(file_path, download_config=download_config)
File "/mnt/nvme1/code/huggingface/nlp-master/src/nlp/utils/file_utils.py", line 198, in cached_path
local_files_only=download_config.local_files_only,
File "/mnt/nvme1/code/huggingface/nlp-master/src/nlp/utils/file_utils.py", line 356, in get_from_cache
raise ConnectionError("Couldn't reach {}".format(url))
ConnectionError: Couldn't reach https://s3.amazonaws.com/datasets.huggingface.co/nlp/metrics/wmt16/wmt16.py
```
5. If I run the same code with `wmt19`, it fails too:
```
ConnectionError: Couldn't reach https://storage.googleapis.com/tfdataset-data/downloadataset/uncorpus/UNv1.0.en-ru.tar.gz
``` | 45 | issues with downloading datasets for wmt16 and wmt19
I have encountered multiple issues while trying to:
```
import nlp
dataset = nlp.load_dataset('wmt16', 'ru-en')
metric = nlp.load_metric('wmt16')
```
1. I had to do `pip install -e ".[dev]" ` on master, currently released nlp didn't work (sorry, didn't save the error) - I went back to the released version and now it worked. So it must have been some outdated dependencies that `pip install -e ".[dev]" ` fixed.
2. it was downloading at 60kbs - almost 5 hours to get the dataset. It was downloading all pairs and not just the one I asked for.
I tried the same code with `wmt19` in parallel and it took a few secs to download and it only fetched data for the requested pair. (but it failed too, see below)
3. my machine has crushed and when I retried I got:
```
Traceback (most recent call last):
File "./download.py", line 9, in <module>
dataset = nlp.load_dataset('wmt16', 'ru-en')
File "/mnt/nvme1/code/huggingface/nlp-master/src/nlp/load.py", line 549, in load_dataset
download_config=download_config, download_mode=download_mode, ignore_verifications=ignore_verifications,
File "/mnt/nvme1/code/huggingface/nlp-master/src/nlp/builder.py", line 449, in download_and_prepare
with incomplete_dir(self._cache_dir) as tmp_data_dir:
File "/home/stas/anaconda3/envs/main/lib/python3.7/contextlib.py", line 112, in __enter__
return next(self.gen)
File "/mnt/nvme1/code/huggingface/nlp-master/src/nlp/builder.py", line 422, in incomplete_dir
os.makedirs(tmp_dir)
File "/home/stas/anaconda3/envs/main/lib/python3.7/os.py", line 221, in makedirs
mkdir(name, mode)
FileExistsError: [Errno 17] File exists: '/home/stas/.cache/huggingface/datasets/wmt16/ru-en/1.0.0/4d8269cdd971ed26984a9c0e4a158e0c7afc8135fac8fb8ee43ceecf38fd422d.incomplete'
```
it can't handle resumes. but neither allows a new start. Had to delete it manually.
4. and finally when it downloaded the dataset, it then failed to fetch the metrics:
```
Traceback (most recent call last):
File "./download.py", line 15, in <module>
metric = nlp.load_metric('wmt16')
File "/mnt/nvme1/code/huggingface/nlp-master/src/nlp/load.py", line 442, in load_metric
module_path, hash = prepare_module(path, download_config=download_config, dataset=False)
File "/mnt/nvme1/code/huggingface/nlp-master/src/nlp/load.py", line 258, in prepare_module
local_path = cached_path(file_path, download_config=download_config)
File "/mnt/nvme1/code/huggingface/nlp-master/src/nlp/utils/file_utils.py", line 198, in cached_path
local_files_only=download_config.local_files_only,
File "/mnt/nvme1/code/huggingface/nlp-master/src/nlp/utils/file_utils.py", line 356, in get_from_cache
raise ConnectionError("Couldn't reach {}".format(url))
ConnectionError: Couldn't reach https://s3.amazonaws.com/datasets.huggingface.co/nlp/metrics/wmt16/wmt16.py
```
5. If I run the same code with `wmt19`, it fails too:
```
ConnectionError: Couldn't reach https://storage.googleapis.com/tfdataset-data/downloadataset/uncorpus/UNv1.0.en-ru.tar.gz
```
Further, `nlp.load_dataset('wmt19', 'ru-en')` has only the `train` and `val` datasets. `test` is missing.
Fixed locally for summarization needs, by running:
```
pip install sacrebleu
sacrebleu -t wmt19 -l ru-en --echo src > test.source
sacrebleu -t wmt19 -l ru-en --echo ref > test.target
```
h/t @sshleifer |
https://github.com/huggingface/datasets/issues/486 | Bookcorpus data contains pretokenized text | Yes indeed it looks like some `'` and spaces are missing (for example in `dont` or `didnt`).
Do you know if there exist some copies without this issue ?
How would you fix this issue on the current data exactly ? I can see that the data is raw text (not tokenized) so I'm not sure I understand how you would do it. Could you provide more details ? | It seem that the bookcoprus data downloaded through the library was pretokenized with NLTK's Treebank tokenizer, which changes the text in incompatible ways to how, for instance, BERT's wordpiece tokenizer works. For example, "didn't" becomes "did" + "n't", and double quotes are changed to `` and '' for start and end quotes, respectively.
On my own projects, I just run the data through NLTK's TreebankWordDetokenizer to reverse the tokenization (as best as possible). I think it would be beneficial to apply this transformation directly on your remote cached copy of the dataset. If you choose to do so, I would also suggest to use my fork of NLTK that fixes several bugs in their detokenizer (I've opened a pull-request, but they've yet to respond): https://github.com/nltk/nltk/pull/2575 | 69 | Bookcorpus data contains pretokenized text
It seem that the bookcoprus data downloaded through the library was pretokenized with NLTK's Treebank tokenizer, which changes the text in incompatible ways to how, for instance, BERT's wordpiece tokenizer works. For example, "didn't" becomes "did" + "n't", and double quotes are changed to `` and '' for start and end quotes, respectively.
On my own projects, I just run the data through NLTK's TreebankWordDetokenizer to reverse the tokenization (as best as possible). I think it would be beneficial to apply this transformation directly on your remote cached copy of the dataset. If you choose to do so, I would also suggest to use my fork of NLTK that fixes several bugs in their detokenizer (I've opened a pull-request, but they've yet to respond): https://github.com/nltk/nltk/pull/2575
Yes indeed it looks like some `'` and spaces are missing (for example in `dont` or `didnt`).
Do you know if there exist some copies without this issue ?
How would you fix this issue on the current data exactly ? I can see that the data is raw text (not tokenized) so I'm not sure I understand how you would do it. Could you provide more details ? |
https://github.com/huggingface/datasets/issues/486 | Bookcorpus data contains pretokenized text | I'm afraid that I don't know how to obtain the original BookCorpus data. I believe this version came from an anonymous Google Drive link posted in another issue.
Going through the raw text in this version, it's apparent that NLTK's TreebankWordTokenizer was applied on it (I gave some examples in my original post), followed by:
`' '.join(tokens)`
You can retrieve the tokenization by splitting on whitespace. You can then "detokenize" it with TreebankWordDetokenizer class of NLTK (though, as I suggested, use the fixed version in my repo). This will bring the text closer to its original form, but some steps of TreebankWordTokenizer are destructive, so it wouldn't be one-to-one. Something along the lines of the following should work:
```
treebank_detokenizer = nltk.tokenize.treebank.TreebankWordDetokenizer()
db = nlp.load_dataset('bookcorpus', split=nlp.Split.TRAIN)
db = db.map(lambda x: treebank_detokenizer.detokenize(x['text'].split()))
```
Regarding other issues beyond the above, I'm afraid that I can't help with that. | It seem that the bookcoprus data downloaded through the library was pretokenized with NLTK's Treebank tokenizer, which changes the text in incompatible ways to how, for instance, BERT's wordpiece tokenizer works. For example, "didn't" becomes "did" + "n't", and double quotes are changed to `` and '' for start and end quotes, respectively.
On my own projects, I just run the data through NLTK's TreebankWordDetokenizer to reverse the tokenization (as best as possible). I think it would be beneficial to apply this transformation directly on your remote cached copy of the dataset. If you choose to do so, I would also suggest to use my fork of NLTK that fixes several bugs in their detokenizer (I've opened a pull-request, but they've yet to respond): https://github.com/nltk/nltk/pull/2575 | 146 | Bookcorpus data contains pretokenized text
It seem that the bookcoprus data downloaded through the library was pretokenized with NLTK's Treebank tokenizer, which changes the text in incompatible ways to how, for instance, BERT's wordpiece tokenizer works. For example, "didn't" becomes "did" + "n't", and double quotes are changed to `` and '' for start and end quotes, respectively.
On my own projects, I just run the data through NLTK's TreebankWordDetokenizer to reverse the tokenization (as best as possible). I think it would be beneficial to apply this transformation directly on your remote cached copy of the dataset. If you choose to do so, I would also suggest to use my fork of NLTK that fixes several bugs in their detokenizer (I've opened a pull-request, but they've yet to respond): https://github.com/nltk/nltk/pull/2575
I'm afraid that I don't know how to obtain the original BookCorpus data. I believe this version came from an anonymous Google Drive link posted in another issue.
Going through the raw text in this version, it's apparent that NLTK's TreebankWordTokenizer was applied on it (I gave some examples in my original post), followed by:
`' '.join(tokens)`
You can retrieve the tokenization by splitting on whitespace. You can then "detokenize" it with TreebankWordDetokenizer class of NLTK (though, as I suggested, use the fixed version in my repo). This will bring the text closer to its original form, but some steps of TreebankWordTokenizer are destructive, so it wouldn't be one-to-one. Something along the lines of the following should work:
```
treebank_detokenizer = nltk.tokenize.treebank.TreebankWordDetokenizer()
db = nlp.load_dataset('bookcorpus', split=nlp.Split.TRAIN)
db = db.map(lambda x: treebank_detokenizer.detokenize(x['text'].split()))
```
Regarding other issues beyond the above, I'm afraid that I can't help with that. |
https://github.com/huggingface/datasets/issues/486 | Bookcorpus data contains pretokenized text | Ok I get it, that would be very cool indeed
What kinds of patterns the detokenizer can't retrieve ? | It seem that the bookcoprus data downloaded through the library was pretokenized with NLTK's Treebank tokenizer, which changes the text in incompatible ways to how, for instance, BERT's wordpiece tokenizer works. For example, "didn't" becomes "did" + "n't", and double quotes are changed to `` and '' for start and end quotes, respectively.
On my own projects, I just run the data through NLTK's TreebankWordDetokenizer to reverse the tokenization (as best as possible). I think it would be beneficial to apply this transformation directly on your remote cached copy of the dataset. If you choose to do so, I would also suggest to use my fork of NLTK that fixes several bugs in their detokenizer (I've opened a pull-request, but they've yet to respond): https://github.com/nltk/nltk/pull/2575 | 19 | Bookcorpus data contains pretokenized text
It seem that the bookcoprus data downloaded through the library was pretokenized with NLTK's Treebank tokenizer, which changes the text in incompatible ways to how, for instance, BERT's wordpiece tokenizer works. For example, "didn't" becomes "did" + "n't", and double quotes are changed to `` and '' for start and end quotes, respectively.
On my own projects, I just run the data through NLTK's TreebankWordDetokenizer to reverse the tokenization (as best as possible). I think it would be beneficial to apply this transformation directly on your remote cached copy of the dataset. If you choose to do so, I would also suggest to use my fork of NLTK that fixes several bugs in their detokenizer (I've opened a pull-request, but they've yet to respond): https://github.com/nltk/nltk/pull/2575
Ok I get it, that would be very cool indeed
What kinds of patterns the detokenizer can't retrieve ? |
https://github.com/huggingface/datasets/issues/486 | Bookcorpus data contains pretokenized text | The TreebankTokenizer makes some assumptions about whitespace, parentheses, quotation marks, etc. For instance, while tokenizing the following text:
```
Dwayne "The Rock" Johnson
```
will result in:
```
Dwayne `` The Rock '' Johnson
```
where the left and right quotation marks are turned into distinct symbols. Upon reconstruction, we can attach the left part to its token on the right, and respectively for the right part. However, the following texts would be tokenized exactly the same:
```
Dwayne " The Rock " Johnson
Dwayne " The Rock" Johnson
Dwayne " The Rock" Johnson
...
```
In the above examples, the detokenizer would correct these inputs into the canonical text
```
Dwayne "The Rock" Johnson
```
However, there are cases where there the solution cannot easily be inferred (at least without a true LM - this tokenizer is just a bunch of regexes). For instance, in cases where you have a fragment that contains the end of quote, but not its beginning, plus an accidental space:
```
... and it sounds fantastic, " he said.
```
In the above case, the tokenizer would assume that the quotes refer to the next token, and so upon detokenization it will result in the following mistake:
```
... and it sounds fantastic, "he said.
```
While these are all odd edge cases (the basic assumptions do make sense), in noisy data they can occur, which is why I mentioned that the detokenizer cannot restore the original perfectly.
| It seem that the bookcoprus data downloaded through the library was pretokenized with NLTK's Treebank tokenizer, which changes the text in incompatible ways to how, for instance, BERT's wordpiece tokenizer works. For example, "didn't" becomes "did" + "n't", and double quotes are changed to `` and '' for start and end quotes, respectively.
On my own projects, I just run the data through NLTK's TreebankWordDetokenizer to reverse the tokenization (as best as possible). I think it would be beneficial to apply this transformation directly on your remote cached copy of the dataset. If you choose to do so, I would also suggest to use my fork of NLTK that fixes several bugs in their detokenizer (I've opened a pull-request, but they've yet to respond): https://github.com/nltk/nltk/pull/2575 | 244 | Bookcorpus data contains pretokenized text
It seem that the bookcoprus data downloaded through the library was pretokenized with NLTK's Treebank tokenizer, which changes the text in incompatible ways to how, for instance, BERT's wordpiece tokenizer works. For example, "didn't" becomes "did" + "n't", and double quotes are changed to `` and '' for start and end quotes, respectively.
On my own projects, I just run the data through NLTK's TreebankWordDetokenizer to reverse the tokenization (as best as possible). I think it would be beneficial to apply this transformation directly on your remote cached copy of the dataset. If you choose to do so, I would also suggest to use my fork of NLTK that fixes several bugs in their detokenizer (I've opened a pull-request, but they've yet to respond): https://github.com/nltk/nltk/pull/2575
The TreebankTokenizer makes some assumptions about whitespace, parentheses, quotation marks, etc. For instance, while tokenizing the following text:
```
Dwayne "The Rock" Johnson
```
will result in:
```
Dwayne `` The Rock '' Johnson
```
where the left and right quotation marks are turned into distinct symbols. Upon reconstruction, we can attach the left part to its token on the right, and respectively for the right part. However, the following texts would be tokenized exactly the same:
```
Dwayne " The Rock " Johnson
Dwayne " The Rock" Johnson
Dwayne " The Rock" Johnson
...
```
In the above examples, the detokenizer would correct these inputs into the canonical text
```
Dwayne "The Rock" Johnson
```
However, there are cases where there the solution cannot easily be inferred (at least without a true LM - this tokenizer is just a bunch of regexes). For instance, in cases where you have a fragment that contains the end of quote, but not its beginning, plus an accidental space:
```
... and it sounds fantastic, " he said.
```
In the above case, the tokenizer would assume that the quotes refer to the next token, and so upon detokenization it will result in the following mistake:
```
... and it sounds fantastic, "he said.
```
While these are all odd edge cases (the basic assumptions do make sense), in noisy data they can occur, which is why I mentioned that the detokenizer cannot restore the original perfectly.
|
https://github.com/huggingface/datasets/issues/486 | Bookcorpus data contains pretokenized text | To confirm, since this is preprocessed, this was not the exact version of the Book Corpus used to actually train the models described here (particularly Distilbert)? https://huggingface.co/datasets/bookcorpus
Or does this preprocessing exactly match that of the papers? | It seem that the bookcoprus data downloaded through the library was pretokenized with NLTK's Treebank tokenizer, which changes the text in incompatible ways to how, for instance, BERT's wordpiece tokenizer works. For example, "didn't" becomes "did" + "n't", and double quotes are changed to `` and '' for start and end quotes, respectively.
On my own projects, I just run the data through NLTK's TreebankWordDetokenizer to reverse the tokenization (as best as possible). I think it would be beneficial to apply this transformation directly on your remote cached copy of the dataset. If you choose to do so, I would also suggest to use my fork of NLTK that fixes several bugs in their detokenizer (I've opened a pull-request, but they've yet to respond): https://github.com/nltk/nltk/pull/2575 | 37 | Bookcorpus data contains pretokenized text
It seem that the bookcoprus data downloaded through the library was pretokenized with NLTK's Treebank tokenizer, which changes the text in incompatible ways to how, for instance, BERT's wordpiece tokenizer works. For example, "didn't" becomes "did" + "n't", and double quotes are changed to `` and '' for start and end quotes, respectively.
On my own projects, I just run the data through NLTK's TreebankWordDetokenizer to reverse the tokenization (as best as possible). I think it would be beneficial to apply this transformation directly on your remote cached copy of the dataset. If you choose to do so, I would also suggest to use my fork of NLTK that fixes several bugs in their detokenizer (I've opened a pull-request, but they've yet to respond): https://github.com/nltk/nltk/pull/2575
To confirm, since this is preprocessed, this was not the exact version of the Book Corpus used to actually train the models described here (particularly Distilbert)? https://huggingface.co/datasets/bookcorpus
Or does this preprocessing exactly match that of the papers? |
https://github.com/huggingface/datasets/issues/486 | Bookcorpus data contains pretokenized text | I believe these are just artifacts of this particular source. It might be better to crawl it again, or use another preprocessed source, as found here: https://github.com/soskek/bookcorpus | It seem that the bookcoprus data downloaded through the library was pretokenized with NLTK's Treebank tokenizer, which changes the text in incompatible ways to how, for instance, BERT's wordpiece tokenizer works. For example, "didn't" becomes "did" + "n't", and double quotes are changed to `` and '' for start and end quotes, respectively.
On my own projects, I just run the data through NLTK's TreebankWordDetokenizer to reverse the tokenization (as best as possible). I think it would be beneficial to apply this transformation directly on your remote cached copy of the dataset. If you choose to do so, I would also suggest to use my fork of NLTK that fixes several bugs in their detokenizer (I've opened a pull-request, but they've yet to respond): https://github.com/nltk/nltk/pull/2575 | 27 | Bookcorpus data contains pretokenized text
It seem that the bookcoprus data downloaded through the library was pretokenized with NLTK's Treebank tokenizer, which changes the text in incompatible ways to how, for instance, BERT's wordpiece tokenizer works. For example, "didn't" becomes "did" + "n't", and double quotes are changed to `` and '' for start and end quotes, respectively.
On my own projects, I just run the data through NLTK's TreebankWordDetokenizer to reverse the tokenization (as best as possible). I think it would be beneficial to apply this transformation directly on your remote cached copy of the dataset. If you choose to do so, I would also suggest to use my fork of NLTK that fixes several bugs in their detokenizer (I've opened a pull-request, but they've yet to respond): https://github.com/nltk/nltk/pull/2575
I believe these are just artifacts of this particular source. It might be better to crawl it again, or use another preprocessed source, as found here: https://github.com/soskek/bookcorpus |
https://github.com/huggingface/datasets/issues/486 | Bookcorpus data contains pretokenized text | Yes actually the BookCorpus on hugginface is based on [this](https://github.com/soskek/bookcorpus/issues/24#issuecomment-643933352). And I kind of regret naming it as "BookCorpus" instead of something like "BookCorpusLike".
But there is a good news ! @shawwn has replicated BookCorpus in his way, and also provided a link to download the plain text files. see [here](https://github.com/soskek/bookcorpus/issues/27). There is chance we can have a "OpenBookCorpus" ! | It seem that the bookcoprus data downloaded through the library was pretokenized with NLTK's Treebank tokenizer, which changes the text in incompatible ways to how, for instance, BERT's wordpiece tokenizer works. For example, "didn't" becomes "did" + "n't", and double quotes are changed to `` and '' for start and end quotes, respectively.
On my own projects, I just run the data through NLTK's TreebankWordDetokenizer to reverse the tokenization (as best as possible). I think it would be beneficial to apply this transformation directly on your remote cached copy of the dataset. If you choose to do so, I would also suggest to use my fork of NLTK that fixes several bugs in their detokenizer (I've opened a pull-request, but they've yet to respond): https://github.com/nltk/nltk/pull/2575 | 60 | Bookcorpus data contains pretokenized text
It seem that the bookcoprus data downloaded through the library was pretokenized with NLTK's Treebank tokenizer, which changes the text in incompatible ways to how, for instance, BERT's wordpiece tokenizer works. For example, "didn't" becomes "did" + "n't", and double quotes are changed to `` and '' for start and end quotes, respectively.
On my own projects, I just run the data through NLTK's TreebankWordDetokenizer to reverse the tokenization (as best as possible). I think it would be beneficial to apply this transformation directly on your remote cached copy of the dataset. If you choose to do so, I would also suggest to use my fork of NLTK that fixes several bugs in their detokenizer (I've opened a pull-request, but they've yet to respond): https://github.com/nltk/nltk/pull/2575
Yes actually the BookCorpus on hugginface is based on [this](https://github.com/soskek/bookcorpus/issues/24#issuecomment-643933352). And I kind of regret naming it as "BookCorpus" instead of something like "BookCorpusLike".
But there is a good news ! @shawwn has replicated BookCorpus in his way, and also provided a link to download the plain text files. see [here](https://github.com/soskek/bookcorpus/issues/27). There is chance we can have a "OpenBookCorpus" ! |
https://github.com/huggingface/datasets/issues/482 | Bugs : dataset.map() is frozen on ELI5 | This comes from an overflow in pyarrow's array.
It is stuck inside the loop that reduces the batch size to avoid the overflow.
I'll take a look | Hi Huggingface Team!
Thank you guys once again for this amazing repo.
I have tried to prepare ELI5 to train with T5, based on [this wonderful notebook of Suraj Patil](https://github.com/patil-suraj/exploring-T5/blob/master/T5_on_TPU.ipynb)
However, when I run `dataset.map()` on ELI5 to prepare `input_text, target_text`, `dataset.map` is **frozen** in the first hundreds examples. On the contrary, this works totally fine on SQUAD (80,000 examples). Both `nlp` version 0.3.0 and 0.4.0 cause frozen process . Also try various `pyarrow` versions from 0.16.0 / 0.17.0 / 1.0.0 also have the same frozen process.
Reproducible code can be found on [this colab notebook ](https://colab.research.google.com/drive/14wttOTv3ky74B_c0kv5WrbgQjCF2fYQk?usp=sharing), where I also show that the same mapping function works fine on SQUAD, so the problem is likely due to ELI5 somehow.
----------------------------------------
**More Info :** instead of `map`, if I run `for` loop and apply function by myself, there's no error and can finish within 10 seconds. However, `nlp dataset` is immutable (I couldn't manually assign a new key-value to `dataset `object)
I also notice that SQUAD texts are quite clean while ELI5 texts contain many special characters, not sure if this is the cause ? | 27 | Bugs : dataset.map() is frozen on ELI5
Hi Huggingface Team!
Thank you guys once again for this amazing repo.
I have tried to prepare ELI5 to train with T5, based on [this wonderful notebook of Suraj Patil](https://github.com/patil-suraj/exploring-T5/blob/master/T5_on_TPU.ipynb)
However, when I run `dataset.map()` on ELI5 to prepare `input_text, target_text`, `dataset.map` is **frozen** in the first hundreds examples. On the contrary, this works totally fine on SQUAD (80,000 examples). Both `nlp` version 0.3.0 and 0.4.0 cause frozen process . Also try various `pyarrow` versions from 0.16.0 / 0.17.0 / 1.0.0 also have the same frozen process.
Reproducible code can be found on [this colab notebook ](https://colab.research.google.com/drive/14wttOTv3ky74B_c0kv5WrbgQjCF2fYQk?usp=sharing), where I also show that the same mapping function works fine on SQUAD, so the problem is likely due to ELI5 somehow.
----------------------------------------
**More Info :** instead of `map`, if I run `for` loop and apply function by myself, there's no error and can finish within 10 seconds. However, `nlp dataset` is immutable (I couldn't manually assign a new key-value to `dataset `object)
I also notice that SQUAD texts are quite clean while ELI5 texts contain many special characters, not sure if this is the cause ?
This comes from an overflow in pyarrow's array.
It is stuck inside the loop that reduces the batch size to avoid the overflow.
I'll take a look |
https://github.com/huggingface/datasets/issues/482 | Bugs : dataset.map() is frozen on ELI5 | I created a PR to fix the issue.
It was due to an overflow check that handled badly an empty list.
You can try the changes by using
```
!pip install git+https://github.com/huggingface/nlp.git@fix-bad-type-in-overflow-check
```
Also I noticed that the first 1000 examples have an empty list in the `title_urls` field. The feature type inference in `.map` will consider it `null` because of that, and it will crash when it encounter the next example with a `title_urls` that is not empty.
Therefore to fix that, what you can do for now is increase the writer batch size so that the feature inference will take into account at least one example with a non-empty `title_urls`:
```python
# default batch size is 1_000 and it's not enough for feature type inference because of empty lists
valid_dataset = valid_dataset.map(make_input_target, writer_batch_size=3_000)
```
I was able to run the frozen cell with these changes. | Hi Huggingface Team!
Thank you guys once again for this amazing repo.
I have tried to prepare ELI5 to train with T5, based on [this wonderful notebook of Suraj Patil](https://github.com/patil-suraj/exploring-T5/blob/master/T5_on_TPU.ipynb)
However, when I run `dataset.map()` on ELI5 to prepare `input_text, target_text`, `dataset.map` is **frozen** in the first hundreds examples. On the contrary, this works totally fine on SQUAD (80,000 examples). Both `nlp` version 0.3.0 and 0.4.0 cause frozen process . Also try various `pyarrow` versions from 0.16.0 / 0.17.0 / 1.0.0 also have the same frozen process.
Reproducible code can be found on [this colab notebook ](https://colab.research.google.com/drive/14wttOTv3ky74B_c0kv5WrbgQjCF2fYQk?usp=sharing), where I also show that the same mapping function works fine on SQUAD, so the problem is likely due to ELI5 somehow.
----------------------------------------
**More Info :** instead of `map`, if I run `for` loop and apply function by myself, there's no error and can finish within 10 seconds. However, `nlp dataset` is immutable (I couldn't manually assign a new key-value to `dataset `object)
I also notice that SQUAD texts are quite clean while ELI5 texts contain many special characters, not sure if this is the cause ? | 147 | Bugs : dataset.map() is frozen on ELI5
Hi Huggingface Team!
Thank you guys once again for this amazing repo.
I have tried to prepare ELI5 to train with T5, based on [this wonderful notebook of Suraj Patil](https://github.com/patil-suraj/exploring-T5/blob/master/T5_on_TPU.ipynb)
However, when I run `dataset.map()` on ELI5 to prepare `input_text, target_text`, `dataset.map` is **frozen** in the first hundreds examples. On the contrary, this works totally fine on SQUAD (80,000 examples). Both `nlp` version 0.3.0 and 0.4.0 cause frozen process . Also try various `pyarrow` versions from 0.16.0 / 0.17.0 / 1.0.0 also have the same frozen process.
Reproducible code can be found on [this colab notebook ](https://colab.research.google.com/drive/14wttOTv3ky74B_c0kv5WrbgQjCF2fYQk?usp=sharing), where I also show that the same mapping function works fine on SQUAD, so the problem is likely due to ELI5 somehow.
----------------------------------------
**More Info :** instead of `map`, if I run `for` loop and apply function by myself, there's no error and can finish within 10 seconds. However, `nlp dataset` is immutable (I couldn't manually assign a new key-value to `dataset `object)
I also notice that SQUAD texts are quite clean while ELI5 texts contain many special characters, not sure if this is the cause ?
I created a PR to fix the issue.
It was due to an overflow check that handled badly an empty list.
You can try the changes by using
```
!pip install git+https://github.com/huggingface/nlp.git@fix-bad-type-in-overflow-check
```
Also I noticed that the first 1000 examples have an empty list in the `title_urls` field. The feature type inference in `.map` will consider it `null` because of that, and it will crash when it encounter the next example with a `title_urls` that is not empty.
Therefore to fix that, what you can do for now is increase the writer batch size so that the feature inference will take into account at least one example with a non-empty `title_urls`:
```python
# default batch size is 1_000 and it's not enough for feature type inference because of empty lists
valid_dataset = valid_dataset.map(make_input_target, writer_batch_size=3_000)
```
I was able to run the frozen cell with these changes. |
https://github.com/huggingface/datasets/issues/482 | Bugs : dataset.map() is frozen on ELI5 | @lhoestq mapping the function `make_input_target` was passed by your fixing.
However, there is another error in the final step of `valid_dataset.map(convert_to_features, batched=True)`
`ArrowInvalid: Could not convert Thepiratebay.vg with type str: converting to null type`
(The [same colab notebook above with new error message](https://colab.research.google.com/drive/14wttOTv3ky74B_c0kv5WrbgQjCF2fYQk?usp=sharing#scrollTo=5sRrJ3_C8rLt))
Do you have some ideas? (I am really sorry I could not debug it by myself since I never used `pyarrow` before)
Note that `train_dataset.map(convert_to_features, batched=True)` can be run successfully even though train_dataset is 27x bigger than `valid_dataset` so I believe the problem lies in some field of `valid_dataset` again . | Hi Huggingface Team!
Thank you guys once again for this amazing repo.
I have tried to prepare ELI5 to train with T5, based on [this wonderful notebook of Suraj Patil](https://github.com/patil-suraj/exploring-T5/blob/master/T5_on_TPU.ipynb)
However, when I run `dataset.map()` on ELI5 to prepare `input_text, target_text`, `dataset.map` is **frozen** in the first hundreds examples. On the contrary, this works totally fine on SQUAD (80,000 examples). Both `nlp` version 0.3.0 and 0.4.0 cause frozen process . Also try various `pyarrow` versions from 0.16.0 / 0.17.0 / 1.0.0 also have the same frozen process.
Reproducible code can be found on [this colab notebook ](https://colab.research.google.com/drive/14wttOTv3ky74B_c0kv5WrbgQjCF2fYQk?usp=sharing), where I also show that the same mapping function works fine on SQUAD, so the problem is likely due to ELI5 somehow.
----------------------------------------
**More Info :** instead of `map`, if I run `for` loop and apply function by myself, there's no error and can finish within 10 seconds. However, `nlp dataset` is immutable (I couldn't manually assign a new key-value to `dataset `object)
I also notice that SQUAD texts are quite clean while ELI5 texts contain many special characters, not sure if this is the cause ? | 94 | Bugs : dataset.map() is frozen on ELI5
Hi Huggingface Team!
Thank you guys once again for this amazing repo.
I have tried to prepare ELI5 to train with T5, based on [this wonderful notebook of Suraj Patil](https://github.com/patil-suraj/exploring-T5/blob/master/T5_on_TPU.ipynb)
However, when I run `dataset.map()` on ELI5 to prepare `input_text, target_text`, `dataset.map` is **frozen** in the first hundreds examples. On the contrary, this works totally fine on SQUAD (80,000 examples). Both `nlp` version 0.3.0 and 0.4.0 cause frozen process . Also try various `pyarrow` versions from 0.16.0 / 0.17.0 / 1.0.0 also have the same frozen process.
Reproducible code can be found on [this colab notebook ](https://colab.research.google.com/drive/14wttOTv3ky74B_c0kv5WrbgQjCF2fYQk?usp=sharing), where I also show that the same mapping function works fine on SQUAD, so the problem is likely due to ELI5 somehow.
----------------------------------------
**More Info :** instead of `map`, if I run `for` loop and apply function by myself, there's no error and can finish within 10 seconds. However, `nlp dataset` is immutable (I couldn't manually assign a new key-value to `dataset `object)
I also notice that SQUAD texts are quite clean while ELI5 texts contain many special characters, not sure if this is the cause ?
@lhoestq mapping the function `make_input_target` was passed by your fixing.
However, there is another error in the final step of `valid_dataset.map(convert_to_features, batched=True)`
`ArrowInvalid: Could not convert Thepiratebay.vg with type str: converting to null type`
(The [same colab notebook above with new error message](https://colab.research.google.com/drive/14wttOTv3ky74B_c0kv5WrbgQjCF2fYQk?usp=sharing#scrollTo=5sRrJ3_C8rLt))
Do you have some ideas? (I am really sorry I could not debug it by myself since I never used `pyarrow` before)
Note that `train_dataset.map(convert_to_features, batched=True)` can be run successfully even though train_dataset is 27x bigger than `valid_dataset` so I believe the problem lies in some field of `valid_dataset` again . |
https://github.com/huggingface/datasets/issues/482 | Bugs : dataset.map() is frozen on ELI5 | I got this issue too and fixed it by specifying `writer_batch_size=3_000` in `.map`.
This is because Arrow didn't expect `Thepiratebay.vg` in `title_urls `, as all previous examples have empty lists in `title_urls ` | Hi Huggingface Team!
Thank you guys once again for this amazing repo.
I have tried to prepare ELI5 to train with T5, based on [this wonderful notebook of Suraj Patil](https://github.com/patil-suraj/exploring-T5/blob/master/T5_on_TPU.ipynb)
However, when I run `dataset.map()` on ELI5 to prepare `input_text, target_text`, `dataset.map` is **frozen** in the first hundreds examples. On the contrary, this works totally fine on SQUAD (80,000 examples). Both `nlp` version 0.3.0 and 0.4.0 cause frozen process . Also try various `pyarrow` versions from 0.16.0 / 0.17.0 / 1.0.0 also have the same frozen process.
Reproducible code can be found on [this colab notebook ](https://colab.research.google.com/drive/14wttOTv3ky74B_c0kv5WrbgQjCF2fYQk?usp=sharing), where I also show that the same mapping function works fine on SQUAD, so the problem is likely due to ELI5 somehow.
----------------------------------------
**More Info :** instead of `map`, if I run `for` loop and apply function by myself, there's no error and can finish within 10 seconds. However, `nlp dataset` is immutable (I couldn't manually assign a new key-value to `dataset `object)
I also notice that SQUAD texts are quite clean while ELI5 texts contain many special characters, not sure if this is the cause ? | 33 | Bugs : dataset.map() is frozen on ELI5
Hi Huggingface Team!
Thank you guys once again for this amazing repo.
I have tried to prepare ELI5 to train with T5, based on [this wonderful notebook of Suraj Patil](https://github.com/patil-suraj/exploring-T5/blob/master/T5_on_TPU.ipynb)
However, when I run `dataset.map()` on ELI5 to prepare `input_text, target_text`, `dataset.map` is **frozen** in the first hundreds examples. On the contrary, this works totally fine on SQUAD (80,000 examples). Both `nlp` version 0.3.0 and 0.4.0 cause frozen process . Also try various `pyarrow` versions from 0.16.0 / 0.17.0 / 1.0.0 also have the same frozen process.
Reproducible code can be found on [this colab notebook ](https://colab.research.google.com/drive/14wttOTv3ky74B_c0kv5WrbgQjCF2fYQk?usp=sharing), where I also show that the same mapping function works fine on SQUAD, so the problem is likely due to ELI5 somehow.
----------------------------------------
**More Info :** instead of `map`, if I run `for` loop and apply function by myself, there's no error and can finish within 10 seconds. However, `nlp dataset` is immutable (I couldn't manually assign a new key-value to `dataset `object)
I also notice that SQUAD texts are quite clean while ELI5 texts contain many special characters, not sure if this is the cause ?
I got this issue too and fixed it by specifying `writer_batch_size=3_000` in `.map`.
This is because Arrow didn't expect `Thepiratebay.vg` in `title_urls `, as all previous examples have empty lists in `title_urls ` |
https://github.com/huggingface/datasets/issues/482 | Bugs : dataset.map() is frozen on ELI5 | I'm getting a hanging `dataset.map()` when running a gradio app with `gradio` for auto-reloading instead of `python` | Hi Huggingface Team!
Thank you guys once again for this amazing repo.
I have tried to prepare ELI5 to train with T5, based on [this wonderful notebook of Suraj Patil](https://github.com/patil-suraj/exploring-T5/blob/master/T5_on_TPU.ipynb)
However, when I run `dataset.map()` on ELI5 to prepare `input_text, target_text`, `dataset.map` is **frozen** in the first hundreds examples. On the contrary, this works totally fine on SQUAD (80,000 examples). Both `nlp` version 0.3.0 and 0.4.0 cause frozen process . Also try various `pyarrow` versions from 0.16.0 / 0.17.0 / 1.0.0 also have the same frozen process.
Reproducible code can be found on [this colab notebook ](https://colab.research.google.com/drive/14wttOTv3ky74B_c0kv5WrbgQjCF2fYQk?usp=sharing), where I also show that the same mapping function works fine on SQUAD, so the problem is likely due to ELI5 somehow.
----------------------------------------
**More Info :** instead of `map`, if I run `for` loop and apply function by myself, there's no error and can finish within 10 seconds. However, `nlp dataset` is immutable (I couldn't manually assign a new key-value to `dataset `object)
I also notice that SQUAD texts are quite clean while ELI5 texts contain many special characters, not sure if this is the cause ? | 17 | Bugs : dataset.map() is frozen on ELI5
Hi Huggingface Team!
Thank you guys once again for this amazing repo.
I have tried to prepare ELI5 to train with T5, based on [this wonderful notebook of Suraj Patil](https://github.com/patil-suraj/exploring-T5/blob/master/T5_on_TPU.ipynb)
However, when I run `dataset.map()` on ELI5 to prepare `input_text, target_text`, `dataset.map` is **frozen** in the first hundreds examples. On the contrary, this works totally fine on SQUAD (80,000 examples). Both `nlp` version 0.3.0 and 0.4.0 cause frozen process . Also try various `pyarrow` versions from 0.16.0 / 0.17.0 / 1.0.0 also have the same frozen process.
Reproducible code can be found on [this colab notebook ](https://colab.research.google.com/drive/14wttOTv3ky74B_c0kv5WrbgQjCF2fYQk?usp=sharing), where I also show that the same mapping function works fine on SQUAD, so the problem is likely due to ELI5 somehow.
----------------------------------------
**More Info :** instead of `map`, if I run `for` loop and apply function by myself, there's no error and can finish within 10 seconds. However, `nlp dataset` is immutable (I couldn't manually assign a new key-value to `dataset `object)
I also notice that SQUAD texts are quite clean while ELI5 texts contain many special characters, not sure if this is the cause ?
I'm getting a hanging `dataset.map()` when running a gradio app with `gradio` for auto-reloading instead of `python` |
https://github.com/huggingface/datasets/issues/482 | Bugs : dataset.map() is frozen on ELI5 | Maybe this is an issue with gradio, could you open an issue on their repo ? `Dataset.map` simply uses `multiprocess.Pool` for multiprocessing
If you interrupt the program mayeb the stack trace would give some information of where it was hanging in the code (maybe a lock somewhere ?) | Hi Huggingface Team!
Thank you guys once again for this amazing repo.
I have tried to prepare ELI5 to train with T5, based on [this wonderful notebook of Suraj Patil](https://github.com/patil-suraj/exploring-T5/blob/master/T5_on_TPU.ipynb)
However, when I run `dataset.map()` on ELI5 to prepare `input_text, target_text`, `dataset.map` is **frozen** in the first hundreds examples. On the contrary, this works totally fine on SQUAD (80,000 examples). Both `nlp` version 0.3.0 and 0.4.0 cause frozen process . Also try various `pyarrow` versions from 0.16.0 / 0.17.0 / 1.0.0 also have the same frozen process.
Reproducible code can be found on [this colab notebook ](https://colab.research.google.com/drive/14wttOTv3ky74B_c0kv5WrbgQjCF2fYQk?usp=sharing), where I also show that the same mapping function works fine on SQUAD, so the problem is likely due to ELI5 somehow.
----------------------------------------
**More Info :** instead of `map`, if I run `for` loop and apply function by myself, there's no error and can finish within 10 seconds. However, `nlp dataset` is immutable (I couldn't manually assign a new key-value to `dataset `object)
I also notice that SQUAD texts are quite clean while ELI5 texts contain many special characters, not sure if this is the cause ? | 48 | Bugs : dataset.map() is frozen on ELI5
Hi Huggingface Team!
Thank you guys once again for this amazing repo.
I have tried to prepare ELI5 to train with T5, based on [this wonderful notebook of Suraj Patil](https://github.com/patil-suraj/exploring-T5/blob/master/T5_on_TPU.ipynb)
However, when I run `dataset.map()` on ELI5 to prepare `input_text, target_text`, `dataset.map` is **frozen** in the first hundreds examples. On the contrary, this works totally fine on SQUAD (80,000 examples). Both `nlp` version 0.3.0 and 0.4.0 cause frozen process . Also try various `pyarrow` versions from 0.16.0 / 0.17.0 / 1.0.0 also have the same frozen process.
Reproducible code can be found on [this colab notebook ](https://colab.research.google.com/drive/14wttOTv3ky74B_c0kv5WrbgQjCF2fYQk?usp=sharing), where I also show that the same mapping function works fine on SQUAD, so the problem is likely due to ELI5 somehow.
----------------------------------------
**More Info :** instead of `map`, if I run `for` loop and apply function by myself, there's no error and can finish within 10 seconds. However, `nlp dataset` is immutable (I couldn't manually assign a new key-value to `dataset `object)
I also notice that SQUAD texts are quite clean while ELI5 texts contain many special characters, not sure if this is the cause ?
Maybe this is an issue with gradio, could you open an issue on their repo ? `Dataset.map` simply uses `multiprocess.Pool` for multiprocessing
If you interrupt the program mayeb the stack trace would give some information of where it was hanging in the code (maybe a lock somewhere ?) |
https://github.com/huggingface/datasets/issues/478 | Export TFRecord to GCP bucket | Nevermind, I restarted my python session and it worked fine...
---
I had an authentification error, and I authenticated from another terminal. After that, no more error but it was not working. Restarting the sessions makes it work :) | Previously, I was writing TFRecords manually to GCP bucket with : `with tf.io.TFRecordWriter('gs://my_bucket/x.tfrecord')`
Since `0.4.0` is out with the `export()` function, I tried it. But it seems TFRecords cannot be directly written to GCP bucket.
`dataset.export('local.tfrecord')` works fine,
but `dataset.export('gs://my_bucket/x.tfrecord')` does not work.
There is no error message, I just can't find the file on my bucket...
---
Looking at the code, `nlp` is using `tf.data.experimental.TFRecordWriter`, while I was using `tf.io.TFRecordWriter`.
**What's the difference between those 2 ? How can I write TFRecords files directly to GCP bucket ?**
@jarednielsen @lhoestq | 39 | Export TFRecord to GCP bucket
Previously, I was writing TFRecords manually to GCP bucket with : `with tf.io.TFRecordWriter('gs://my_bucket/x.tfrecord')`
Since `0.4.0` is out with the `export()` function, I tried it. But it seems TFRecords cannot be directly written to GCP bucket.
`dataset.export('local.tfrecord')` works fine,
but `dataset.export('gs://my_bucket/x.tfrecord')` does not work.
There is no error message, I just can't find the file on my bucket...
---
Looking at the code, `nlp` is using `tf.data.experimental.TFRecordWriter`, while I was using `tf.io.TFRecordWriter`.
**What's the difference between those 2 ? How can I write TFRecords files directly to GCP bucket ?**
@jarednielsen @lhoestq
Nevermind, I restarted my python session and it worked fine...
---
I had an authentification error, and I authenticated from another terminal. After that, no more error but it was not working. Restarting the sessions makes it work :) |
https://github.com/huggingface/datasets/issues/477 | Overview.ipynb throws exceptions with nlp 0.4.0 | Thanks for reporting this issue
There was a bug where numpy arrays would get returned instead of tensorflow tensors.
This is fixed on master.
I tried to re-run the colab and encountered this error instead:
```
AttributeError: 'tensorflow.python.framework.ops.EagerTensor' object has no attribute 'to_tensor'
```
This is because the dataset returns a Tensor and not a RaggedTensor.
But I think we should always return a RaggedTensor unless the length of the sequence is fixed (it that case they can be stack into a Tensor). | with nlp 0.4.0, the TensorFlow example in Overview.ipynb throws the following exceptions:
---------------------------------------------------------------------------
AttributeError Traceback (most recent call last)
<ipython-input-5-48907f2ad433> in <module>
----> 1 features = {x: train_tf_dataset[x].to_tensor(default_value=0, shape=[None, tokenizer.max_len]) for x in columns[:3]}
2 labels = {"output_1": train_tf_dataset["start_positions"].to_tensor(default_value=0, shape=[None, 1])}
3 labels["output_2"] = train_tf_dataset["end_positions"].to_tensor(default_value=0, shape=[None, 1])
4 tfdataset = tf.data.Dataset.from_tensor_slices((features, labels)).batch(8)
<ipython-input-5-48907f2ad433> in <dictcomp>(.0)
----> 1 features = {x: train_tf_dataset[x].to_tensor(default_value=0, shape=[None, tokenizer.max_len]) for x in columns[:3]}
2 labels = {"output_1": train_tf_dataset["start_positions"].to_tensor(default_value=0, shape=[None, 1])}
3 labels["output_2"] = train_tf_dataset["end_positions"].to_tensor(default_value=0, shape=[None, 1])
4 tfdataset = tf.data.Dataset.from_tensor_slices((features, labels)).batch(8)
AttributeError: 'numpy.ndarray' object has no attribute 'to_tensor' | 83 | Overview.ipynb throws exceptions with nlp 0.4.0
with nlp 0.4.0, the TensorFlow example in Overview.ipynb throws the following exceptions:
---------------------------------------------------------------------------
AttributeError Traceback (most recent call last)
<ipython-input-5-48907f2ad433> in <module>
----> 1 features = {x: train_tf_dataset[x].to_tensor(default_value=0, shape=[None, tokenizer.max_len]) for x in columns[:3]}
2 labels = {"output_1": train_tf_dataset["start_positions"].to_tensor(default_value=0, shape=[None, 1])}
3 labels["output_2"] = train_tf_dataset["end_positions"].to_tensor(default_value=0, shape=[None, 1])
4 tfdataset = tf.data.Dataset.from_tensor_slices((features, labels)).batch(8)
<ipython-input-5-48907f2ad433> in <dictcomp>(.0)
----> 1 features = {x: train_tf_dataset[x].to_tensor(default_value=0, shape=[None, tokenizer.max_len]) for x in columns[:3]}
2 labels = {"output_1": train_tf_dataset["start_positions"].to_tensor(default_value=0, shape=[None, 1])}
3 labels["output_2"] = train_tf_dataset["end_positions"].to_tensor(default_value=0, shape=[None, 1])
4 tfdataset = tf.data.Dataset.from_tensor_slices((features, labels)).batch(8)
AttributeError: 'numpy.ndarray' object has no attribute 'to_tensor'
Thanks for reporting this issue
There was a bug where numpy arrays would get returned instead of tensorflow tensors.
This is fixed on master.
I tried to re-run the colab and encountered this error instead:
```
AttributeError: 'tensorflow.python.framework.ops.EagerTensor' object has no attribute 'to_tensor'
```
This is because the dataset returns a Tensor and not a RaggedTensor.
But I think we should always return a RaggedTensor unless the length of the sequence is fixed (it that case they can be stack into a Tensor). |
https://github.com/huggingface/datasets/issues/477 | Overview.ipynb throws exceptions with nlp 0.4.0 | Hi, I got another error (on Colab):
```python
# You can read a few attributes of the datasets before loading them (they are python dataclasses)
from dataclasses import asdict
for key, value in asdict(datasets[6]).items():
print('π ' + key + ': ' + str(value))
---------------------------------------------------------------------------
TypeError Traceback (most recent call last)
<ipython-input-6-b8ace6c227a2> in <module>()
2 from dataclasses import asdict
3
----> 4 for key, value in asdict(datasets[6]).items():
5 print('π ' + key + ': ' + str(value))
/usr/local/lib/python3.6/dist-packages/dataclasses.py in asdict(obj, dict_factory)
1008 """
1009 if not _is_dataclass_instance(obj):
-> 1010 raise TypeError("asdict() should be called on dataclass instances")
1011 return _asdict_inner(obj, dict_factory)
1012
TypeError: asdict() should be called on dataclass instances
``` | with nlp 0.4.0, the TensorFlow example in Overview.ipynb throws the following exceptions:
---------------------------------------------------------------------------
AttributeError Traceback (most recent call last)
<ipython-input-5-48907f2ad433> in <module>
----> 1 features = {x: train_tf_dataset[x].to_tensor(default_value=0, shape=[None, tokenizer.max_len]) for x in columns[:3]}
2 labels = {"output_1": train_tf_dataset["start_positions"].to_tensor(default_value=0, shape=[None, 1])}
3 labels["output_2"] = train_tf_dataset["end_positions"].to_tensor(default_value=0, shape=[None, 1])
4 tfdataset = tf.data.Dataset.from_tensor_slices((features, labels)).batch(8)
<ipython-input-5-48907f2ad433> in <dictcomp>(.0)
----> 1 features = {x: train_tf_dataset[x].to_tensor(default_value=0, shape=[None, tokenizer.max_len]) for x in columns[:3]}
2 labels = {"output_1": train_tf_dataset["start_positions"].to_tensor(default_value=0, shape=[None, 1])}
3 labels["output_2"] = train_tf_dataset["end_positions"].to_tensor(default_value=0, shape=[None, 1])
4 tfdataset = tf.data.Dataset.from_tensor_slices((features, labels)).batch(8)
AttributeError: 'numpy.ndarray' object has no attribute 'to_tensor' | 110 | Overview.ipynb throws exceptions with nlp 0.4.0
with nlp 0.4.0, the TensorFlow example in Overview.ipynb throws the following exceptions:
---------------------------------------------------------------------------
AttributeError Traceback (most recent call last)
<ipython-input-5-48907f2ad433> in <module>
----> 1 features = {x: train_tf_dataset[x].to_tensor(default_value=0, shape=[None, tokenizer.max_len]) for x in columns[:3]}
2 labels = {"output_1": train_tf_dataset["start_positions"].to_tensor(default_value=0, shape=[None, 1])}
3 labels["output_2"] = train_tf_dataset["end_positions"].to_tensor(default_value=0, shape=[None, 1])
4 tfdataset = tf.data.Dataset.from_tensor_slices((features, labels)).batch(8)
<ipython-input-5-48907f2ad433> in <dictcomp>(.0)
----> 1 features = {x: train_tf_dataset[x].to_tensor(default_value=0, shape=[None, tokenizer.max_len]) for x in columns[:3]}
2 labels = {"output_1": train_tf_dataset["start_positions"].to_tensor(default_value=0, shape=[None, 1])}
3 labels["output_2"] = train_tf_dataset["end_positions"].to_tensor(default_value=0, shape=[None, 1])
4 tfdataset = tf.data.Dataset.from_tensor_slices((features, labels)).batch(8)
AttributeError: 'numpy.ndarray' object has no attribute 'to_tensor'
Hi, I got another error (on Colab):
```python
# You can read a few attributes of the datasets before loading them (they are python dataclasses)
from dataclasses import asdict
for key, value in asdict(datasets[6]).items():
print('π ' + key + ': ' + str(value))
---------------------------------------------------------------------------
TypeError Traceback (most recent call last)
<ipython-input-6-b8ace6c227a2> in <module>()
2 from dataclasses import asdict
3
----> 4 for key, value in asdict(datasets[6]).items():
5 print('π ' + key + ': ' + str(value))
/usr/local/lib/python3.6/dist-packages/dataclasses.py in asdict(obj, dict_factory)
1008 """
1009 if not _is_dataclass_instance(obj):
-> 1010 raise TypeError("asdict() should be called on dataclass instances")
1011 return _asdict_inner(obj, dict_factory)
1012
TypeError: asdict() should be called on dataclass instances
``` |
https://github.com/huggingface/datasets/issues/474 | test_load_real_dataset when config has BUILDER_CONFIGS that matter | The `data_dir` parameter has been removed. Now the error is `ValueError: Config name is missing`
As mentioned in #470 I think we can have one test with the first config of BUILDER_CONFIGS, and another test that runs all of the configs in BUILDER_CONFIGS | It a dataset has custom `BUILDER_CONFIGS` with non-keyword arguments (or keyword arguments with non default values), the config is not loaded during the test and causes an error.
I think the problem is that `test_load_real_dataset` calls `load_dataset` with `data_dir=temp_data_dir` ([here](https://github.com/huggingface/nlp/blob/master/tests/test_dataset_common.py#L200)). This causes [this line](https://github.com/huggingface/nlp/blob/master/src/nlp/builder.py#L201) to always be false because `config_kwargs` is not `None`. [This line](https://github.com/huggingface/nlp/blob/master/src/nlp/builder.py#L222) will be run instead, which doesn't use `BUILDER_CONFIGS`.
For an example, you can try running the test for lince:
` RUN_SLOW=1 pytest tests/test_dataset_common.py::LocalDatasetTest::test_load_real_dataset_lince`
which yields
> E TypeError: __init__() missing 3 required positional arguments: 'colnames', 'classes', and 'label_column' | 43 | test_load_real_dataset when config has BUILDER_CONFIGS that matter
It a dataset has custom `BUILDER_CONFIGS` with non-keyword arguments (or keyword arguments with non default values), the config is not loaded during the test and causes an error.
I think the problem is that `test_load_real_dataset` calls `load_dataset` with `data_dir=temp_data_dir` ([here](https://github.com/huggingface/nlp/blob/master/tests/test_dataset_common.py#L200)). This causes [this line](https://github.com/huggingface/nlp/blob/master/src/nlp/builder.py#L201) to always be false because `config_kwargs` is not `None`. [This line](https://github.com/huggingface/nlp/blob/master/src/nlp/builder.py#L222) will be run instead, which doesn't use `BUILDER_CONFIGS`.
For an example, you can try running the test for lince:
` RUN_SLOW=1 pytest tests/test_dataset_common.py::LocalDatasetTest::test_load_real_dataset_lince`
which yields
> E TypeError: __init__() missing 3 required positional arguments: 'colnames', 'classes', and 'label_column'
The `data_dir` parameter has been removed. Now the error is `ValueError: Config name is missing`
As mentioned in #470 I think we can have one test with the first config of BUILDER_CONFIGS, and another test that runs all of the configs in BUILDER_CONFIGS |
https://github.com/huggingface/datasets/issues/474 | test_load_real_dataset when config has BUILDER_CONFIGS that matter | This was fixed in #527
Closing this one, but feel free to re-open if you have other questions | It a dataset has custom `BUILDER_CONFIGS` with non-keyword arguments (or keyword arguments with non default values), the config is not loaded during the test and causes an error.
I think the problem is that `test_load_real_dataset` calls `load_dataset` with `data_dir=temp_data_dir` ([here](https://github.com/huggingface/nlp/blob/master/tests/test_dataset_common.py#L200)). This causes [this line](https://github.com/huggingface/nlp/blob/master/src/nlp/builder.py#L201) to always be false because `config_kwargs` is not `None`. [This line](https://github.com/huggingface/nlp/blob/master/src/nlp/builder.py#L222) will be run instead, which doesn't use `BUILDER_CONFIGS`.
For an example, you can try running the test for lince:
` RUN_SLOW=1 pytest tests/test_dataset_common.py::LocalDatasetTest::test_load_real_dataset_lince`
which yields
> E TypeError: __init__() missing 3 required positional arguments: 'colnames', 'classes', and 'label_column' | 18 | test_load_real_dataset when config has BUILDER_CONFIGS that matter
It a dataset has custom `BUILDER_CONFIGS` with non-keyword arguments (or keyword arguments with non default values), the config is not loaded during the test and causes an error.
I think the problem is that `test_load_real_dataset` calls `load_dataset` with `data_dir=temp_data_dir` ([here](https://github.com/huggingface/nlp/blob/master/tests/test_dataset_common.py#L200)). This causes [this line](https://github.com/huggingface/nlp/blob/master/src/nlp/builder.py#L201) to always be false because `config_kwargs` is not `None`. [This line](https://github.com/huggingface/nlp/blob/master/src/nlp/builder.py#L222) will be run instead, which doesn't use `BUILDER_CONFIGS`.
For an example, you can try running the test for lince:
` RUN_SLOW=1 pytest tests/test_dataset_common.py::LocalDatasetTest::test_load_real_dataset_lince`
which yields
> E TypeError: __init__() missing 3 required positional arguments: 'colnames', 'classes', and 'label_column'
This was fixed in #527
Closing this one, but feel free to re-open if you have other questions |
https://github.com/huggingface/datasets/issues/469 | invalid data type 'str' at _convert_outputs in arrow_dataset.py | Hi ! Did you try to set the output format to pytorch ? (or tensorflow if you're using tensorflow)
It can be done with `dataset.set_format("torch", columns=columns)` (or "tensorflow").
Note that for pytorch, string columns can't be converted to `torch.Tensor`, so you have to specify in `columns=` the list of columns you want to keep (`input_ids` for example) | I trying to build multi label text classifier model using Transformers lib.
I'm using Transformers NLP to load the data set, while calling trainer.train() method. It throws the following error
File "C:\***\arrow_dataset.py", line 343, in _convert_outputs
v = command(v)
TypeError: new(): invalid data type 'str'
I'm using pyarrow 1.0.0. And I have simple custom data set with Text and Integer Label.
Ex: Data
Text , Label #Column Header
I'm facing an Network issue, 1
I forgot my password, 2
Error StackTrace:
File "C:\**\transformers\trainer.py", line 492, in train
for step, inputs in enumerate(epoch_iterator):
File "C:\**\tqdm\std.py", line 1104, in __iter__
for obj in iterable:
File "C:\**\torch\utils\data\dataloader.py", line 345, in __next__
data = self._next_data()
File "C:\**\torch\utils\data\dataloader.py", line 385, in _next_data
data = self._dataset_fetcher.fetch(index) # may raise StopIteration
File "C:\**\torch\utils\data\_utils\fetch.py", line 44, in fetch
data = [self.dataset[idx] for idx in possibly_batched_index]
File "C:\**\torch\utils\data\_utils\fetch.py", line 44, in <listcomp>
data = [self.dataset[idx] for idx in possibly_batched_index]
File "C:\**\nlp\arrow_dataset.py", line 414, in __getitem__
output_all_columns=self._output_all_columns,
File "C:\**\nlp\arrow_dataset.py", line 403, in _getitem
outputs, format_type=format_type, format_columns=format_columns, output_all_columns=output_all_columns
File "C:\**\nlp\arrow_dataset.py", line 343, in _convert_outputs
v = command(v)
TypeError: new(): invalid data type 'str'
| 57 | invalid data type 'str' at _convert_outputs in arrow_dataset.py
I trying to build multi label text classifier model using Transformers lib.
I'm using Transformers NLP to load the data set, while calling trainer.train() method. It throws the following error
File "C:\***\arrow_dataset.py", line 343, in _convert_outputs
v = command(v)
TypeError: new(): invalid data type 'str'
I'm using pyarrow 1.0.0. And I have simple custom data set with Text and Integer Label.
Ex: Data
Text , Label #Column Header
I'm facing an Network issue, 1
I forgot my password, 2
Error StackTrace:
File "C:\**\transformers\trainer.py", line 492, in train
for step, inputs in enumerate(epoch_iterator):
File "C:\**\tqdm\std.py", line 1104, in __iter__
for obj in iterable:
File "C:\**\torch\utils\data\dataloader.py", line 345, in __next__
data = self._next_data()
File "C:\**\torch\utils\data\dataloader.py", line 385, in _next_data
data = self._dataset_fetcher.fetch(index) # may raise StopIteration
File "C:\**\torch\utils\data\_utils\fetch.py", line 44, in fetch
data = [self.dataset[idx] for idx in possibly_batched_index]
File "C:\**\torch\utils\data\_utils\fetch.py", line 44, in <listcomp>
data = [self.dataset[idx] for idx in possibly_batched_index]
File "C:\**\nlp\arrow_dataset.py", line 414, in __getitem__
output_all_columns=self._output_all_columns,
File "C:\**\nlp\arrow_dataset.py", line 403, in _getitem
outputs, format_type=format_type, format_columns=format_columns, output_all_columns=output_all_columns
File "C:\**\nlp\arrow_dataset.py", line 343, in _convert_outputs
v = command(v)
TypeError: new(): invalid data type 'str'
Hi ! Did you try to set the output format to pytorch ? (or tensorflow if you're using tensorflow)
It can be done with `dataset.set_format("torch", columns=columns)` (or "tensorflow").
Note that for pytorch, string columns can't be converted to `torch.Tensor`, so you have to specify in `columns=` the list of columns you want to keep (`input_ids` for example) |
https://github.com/huggingface/datasets/issues/469 | invalid data type 'str' at _convert_outputs in arrow_dataset.py | Hello . Yes, I did set the output format as below for the two columns
`train_dataset.set_format('torch',columns=['Text','Label'])`
| I trying to build multi label text classifier model using Transformers lib.
I'm using Transformers NLP to load the data set, while calling trainer.train() method. It throws the following error
File "C:\***\arrow_dataset.py", line 343, in _convert_outputs
v = command(v)
TypeError: new(): invalid data type 'str'
I'm using pyarrow 1.0.0. And I have simple custom data set with Text and Integer Label.
Ex: Data
Text , Label #Column Header
I'm facing an Network issue, 1
I forgot my password, 2
Error StackTrace:
File "C:\**\transformers\trainer.py", line 492, in train
for step, inputs in enumerate(epoch_iterator):
File "C:\**\tqdm\std.py", line 1104, in __iter__
for obj in iterable:
File "C:\**\torch\utils\data\dataloader.py", line 345, in __next__
data = self._next_data()
File "C:\**\torch\utils\data\dataloader.py", line 385, in _next_data
data = self._dataset_fetcher.fetch(index) # may raise StopIteration
File "C:\**\torch\utils\data\_utils\fetch.py", line 44, in fetch
data = [self.dataset[idx] for idx in possibly_batched_index]
File "C:\**\torch\utils\data\_utils\fetch.py", line 44, in <listcomp>
data = [self.dataset[idx] for idx in possibly_batched_index]
File "C:\**\nlp\arrow_dataset.py", line 414, in __getitem__
output_all_columns=self._output_all_columns,
File "C:\**\nlp\arrow_dataset.py", line 403, in _getitem
outputs, format_type=format_type, format_columns=format_columns, output_all_columns=output_all_columns
File "C:\**\nlp\arrow_dataset.py", line 343, in _convert_outputs
v = command(v)
TypeError: new(): invalid data type 'str'
| 16 | invalid data type 'str' at _convert_outputs in arrow_dataset.py
I trying to build multi label text classifier model using Transformers lib.
I'm using Transformers NLP to load the data set, while calling trainer.train() method. It throws the following error
File "C:\***\arrow_dataset.py", line 343, in _convert_outputs
v = command(v)
TypeError: new(): invalid data type 'str'
I'm using pyarrow 1.0.0. And I have simple custom data set with Text and Integer Label.
Ex: Data
Text , Label #Column Header
I'm facing an Network issue, 1
I forgot my password, 2
Error StackTrace:
File "C:\**\transformers\trainer.py", line 492, in train
for step, inputs in enumerate(epoch_iterator):
File "C:\**\tqdm\std.py", line 1104, in __iter__
for obj in iterable:
File "C:\**\torch\utils\data\dataloader.py", line 345, in __next__
data = self._next_data()
File "C:\**\torch\utils\data\dataloader.py", line 385, in _next_data
data = self._dataset_fetcher.fetch(index) # may raise StopIteration
File "C:\**\torch\utils\data\_utils\fetch.py", line 44, in fetch
data = [self.dataset[idx] for idx in possibly_batched_index]
File "C:\**\torch\utils\data\_utils\fetch.py", line 44, in <listcomp>
data = [self.dataset[idx] for idx in possibly_batched_index]
File "C:\**\nlp\arrow_dataset.py", line 414, in __getitem__
output_all_columns=self._output_all_columns,
File "C:\**\nlp\arrow_dataset.py", line 403, in _getitem
outputs, format_type=format_type, format_columns=format_columns, output_all_columns=output_all_columns
File "C:\**\nlp\arrow_dataset.py", line 343, in _convert_outputs
v = command(v)
TypeError: new(): invalid data type 'str'
Hello . Yes, I did set the output format as below for the two columns
`train_dataset.set_format('torch',columns=['Text','Label'])`
|
https://github.com/huggingface/datasets/issues/469 | invalid data type 'str' at _convert_outputs in arrow_dataset.py | I think you're having this issue because you try to format strings as pytorch tensors, which is not possible.
Indeed by having "Text" in `columns=['Text','Label']`, you try to convert the text values to pytorch tensors.
Instead I recommend you to first tokenize your dataset using a tokenizer from transformers. For example
```python
from transformers import BertTokenizer
tokenizer = BertTokenizer.from_pretrained("bert-base-uncased")
train_dataset.map(lambda x: tokenizer(x["Text"]), batched=True)
train_dataset.set_format("torch", column=["input_ids"])
```
Another way to fix your issue would be to not set the format to pytorch, and leave the dataset as it is by default. In that case, the strings are returned normally when you get examples from your dataloader. It means that you would have to tokenize the examples in the training loop (or using a data collator) though.
Let me know if you have other questions | I trying to build multi label text classifier model using Transformers lib.
I'm using Transformers NLP to load the data set, while calling trainer.train() method. It throws the following error
File "C:\***\arrow_dataset.py", line 343, in _convert_outputs
v = command(v)
TypeError: new(): invalid data type 'str'
I'm using pyarrow 1.0.0. And I have simple custom data set with Text and Integer Label.
Ex: Data
Text , Label #Column Header
I'm facing an Network issue, 1
I forgot my password, 2
Error StackTrace:
File "C:\**\transformers\trainer.py", line 492, in train
for step, inputs in enumerate(epoch_iterator):
File "C:\**\tqdm\std.py", line 1104, in __iter__
for obj in iterable:
File "C:\**\torch\utils\data\dataloader.py", line 345, in __next__
data = self._next_data()
File "C:\**\torch\utils\data\dataloader.py", line 385, in _next_data
data = self._dataset_fetcher.fetch(index) # may raise StopIteration
File "C:\**\torch\utils\data\_utils\fetch.py", line 44, in fetch
data = [self.dataset[idx] for idx in possibly_batched_index]
File "C:\**\torch\utils\data\_utils\fetch.py", line 44, in <listcomp>
data = [self.dataset[idx] for idx in possibly_batched_index]
File "C:\**\nlp\arrow_dataset.py", line 414, in __getitem__
output_all_columns=self._output_all_columns,
File "C:\**\nlp\arrow_dataset.py", line 403, in _getitem
outputs, format_type=format_type, format_columns=format_columns, output_all_columns=output_all_columns
File "C:\**\nlp\arrow_dataset.py", line 343, in _convert_outputs
v = command(v)
TypeError: new(): invalid data type 'str'
| 133 | invalid data type 'str' at _convert_outputs in arrow_dataset.py
I trying to build multi label text classifier model using Transformers lib.
I'm using Transformers NLP to load the data set, while calling trainer.train() method. It throws the following error
File "C:\***\arrow_dataset.py", line 343, in _convert_outputs
v = command(v)
TypeError: new(): invalid data type 'str'
I'm using pyarrow 1.0.0. And I have simple custom data set with Text and Integer Label.
Ex: Data
Text , Label #Column Header
I'm facing an Network issue, 1
I forgot my password, 2
Error StackTrace:
File "C:\**\transformers\trainer.py", line 492, in train
for step, inputs in enumerate(epoch_iterator):
File "C:\**\tqdm\std.py", line 1104, in __iter__
for obj in iterable:
File "C:\**\torch\utils\data\dataloader.py", line 345, in __next__
data = self._next_data()
File "C:\**\torch\utils\data\dataloader.py", line 385, in _next_data
data = self._dataset_fetcher.fetch(index) # may raise StopIteration
File "C:\**\torch\utils\data\_utils\fetch.py", line 44, in fetch
data = [self.dataset[idx] for idx in possibly_batched_index]
File "C:\**\torch\utils\data\_utils\fetch.py", line 44, in <listcomp>
data = [self.dataset[idx] for idx in possibly_batched_index]
File "C:\**\nlp\arrow_dataset.py", line 414, in __getitem__
output_all_columns=self._output_all_columns,
File "C:\**\nlp\arrow_dataset.py", line 403, in _getitem
outputs, format_type=format_type, format_columns=format_columns, output_all_columns=output_all_columns
File "C:\**\nlp\arrow_dataset.py", line 343, in _convert_outputs
v = command(v)
TypeError: new(): invalid data type 'str'
I think you're having this issue because you try to format strings as pytorch tensors, which is not possible.
Indeed by having "Text" in `columns=['Text','Label']`, you try to convert the text values to pytorch tensors.
Instead I recommend you to first tokenize your dataset using a tokenizer from transformers. For example
```python
from transformers import BertTokenizer
tokenizer = BertTokenizer.from_pretrained("bert-base-uncased")
train_dataset.map(lambda x: tokenizer(x["Text"]), batched=True)
train_dataset.set_format("torch", column=["input_ids"])
```
Another way to fix your issue would be to not set the format to pytorch, and leave the dataset as it is by default. In that case, the strings are returned normally when you get examples from your dataloader. It means that you would have to tokenize the examples in the training loop (or using a data collator) though.
Let me know if you have other questions |
https://github.com/huggingface/datasets/issues/469 | invalid data type 'str' at _convert_outputs in arrow_dataset.py | Hi, actually the thing is I am getting the same error and even after tokenizing them I am passing them through batch_encode_plus.
I dont know what seems to be the problem is. I even converted it into 'pt' while passing them through batch_encode_plus but when I am evaluating my model , i am getting this error
---------------------------------------------------------------------------
TypeError Traceback (most recent call last)
<ipython-input-145-ca218223c9fc> in <module>()
----> 1 val_loss, predictions, true_val = evaluate(dataloader_validation)
2 val_f1 = f1_score_func(predictions, true_val)
3 tqdm.write(f'Validation loss: {val_loss}')
4 tqdm.write(f'F1 Score (Weighted): {val_f1}')
6 frames
/usr/local/lib/python3.6/dist-packages/torch/utils/data/dataset.py in <genexpr>(.0)
160
161 def __getitem__(self, index):
--> 162 return tuple(tensor[index] for tensor in self.tensors)
163
164 def __len__(self):
TypeError: new(): invalid data type 'str' | I trying to build multi label text classifier model using Transformers lib.
I'm using Transformers NLP to load the data set, while calling trainer.train() method. It throws the following error
File "C:\***\arrow_dataset.py", line 343, in _convert_outputs
v = command(v)
TypeError: new(): invalid data type 'str'
I'm using pyarrow 1.0.0. And I have simple custom data set with Text and Integer Label.
Ex: Data
Text , Label #Column Header
I'm facing an Network issue, 1
I forgot my password, 2
Error StackTrace:
File "C:\**\transformers\trainer.py", line 492, in train
for step, inputs in enumerate(epoch_iterator):
File "C:\**\tqdm\std.py", line 1104, in __iter__
for obj in iterable:
File "C:\**\torch\utils\data\dataloader.py", line 345, in __next__
data = self._next_data()
File "C:\**\torch\utils\data\dataloader.py", line 385, in _next_data
data = self._dataset_fetcher.fetch(index) # may raise StopIteration
File "C:\**\torch\utils\data\_utils\fetch.py", line 44, in fetch
data = [self.dataset[idx] for idx in possibly_batched_index]
File "C:\**\torch\utils\data\_utils\fetch.py", line 44, in <listcomp>
data = [self.dataset[idx] for idx in possibly_batched_index]
File "C:\**\nlp\arrow_dataset.py", line 414, in __getitem__
output_all_columns=self._output_all_columns,
File "C:\**\nlp\arrow_dataset.py", line 403, in _getitem
outputs, format_type=format_type, format_columns=format_columns, output_all_columns=output_all_columns
File "C:\**\nlp\arrow_dataset.py", line 343, in _convert_outputs
v = command(v)
TypeError: new(): invalid data type 'str'
| 115 | invalid data type 'str' at _convert_outputs in arrow_dataset.py
I trying to build multi label text classifier model using Transformers lib.
I'm using Transformers NLP to load the data set, while calling trainer.train() method. It throws the following error
File "C:\***\arrow_dataset.py", line 343, in _convert_outputs
v = command(v)
TypeError: new(): invalid data type 'str'
I'm using pyarrow 1.0.0. And I have simple custom data set with Text and Integer Label.
Ex: Data
Text , Label #Column Header
I'm facing an Network issue, 1
I forgot my password, 2
Error StackTrace:
File "C:\**\transformers\trainer.py", line 492, in train
for step, inputs in enumerate(epoch_iterator):
File "C:\**\tqdm\std.py", line 1104, in __iter__
for obj in iterable:
File "C:\**\torch\utils\data\dataloader.py", line 345, in __next__
data = self._next_data()
File "C:\**\torch\utils\data\dataloader.py", line 385, in _next_data
data = self._dataset_fetcher.fetch(index) # may raise StopIteration
File "C:\**\torch\utils\data\_utils\fetch.py", line 44, in fetch
data = [self.dataset[idx] for idx in possibly_batched_index]
File "C:\**\torch\utils\data\_utils\fetch.py", line 44, in <listcomp>
data = [self.dataset[idx] for idx in possibly_batched_index]
File "C:\**\nlp\arrow_dataset.py", line 414, in __getitem__
output_all_columns=self._output_all_columns,
File "C:\**\nlp\arrow_dataset.py", line 403, in _getitem
outputs, format_type=format_type, format_columns=format_columns, output_all_columns=output_all_columns
File "C:\**\nlp\arrow_dataset.py", line 343, in _convert_outputs
v = command(v)
TypeError: new(): invalid data type 'str'
Hi, actually the thing is I am getting the same error and even after tokenizing them I am passing them through batch_encode_plus.
I dont know what seems to be the problem is. I even converted it into 'pt' while passing them through batch_encode_plus but when I am evaluating my model , i am getting this error
---------------------------------------------------------------------------
TypeError Traceback (most recent call last)
<ipython-input-145-ca218223c9fc> in <module>()
----> 1 val_loss, predictions, true_val = evaluate(dataloader_validation)
2 val_f1 = f1_score_func(predictions, true_val)
3 tqdm.write(f'Validation loss: {val_loss}')
4 tqdm.write(f'F1 Score (Weighted): {val_f1}')
6 frames
/usr/local/lib/python3.6/dist-packages/torch/utils/data/dataset.py in <genexpr>(.0)
160
161 def __getitem__(self, index):
--> 162 return tuple(tensor[index] for tensor in self.tensors)
163
164 def __len__(self):
TypeError: new(): invalid data type 'str' |
https://github.com/huggingface/datasets/issues/469 | invalid data type 'str' at _convert_outputs in arrow_dataset.py | > Hi, actually the thing is I am getting the same error and even after tokenizing them I am passing them through batch_encode_plus.
> I dont know what seems to be the problem is. I even converted it into 'pt' while passing them through batch_encode_plus but when I am evaluating my model , i am getting this error
>
> TypeError Traceback (most recent call last)
> in ()
> ----> 1 val_loss, predictions, true_val = evaluate(dataloader_validation)
> 2 val_f1 = f1_score_func(predictions, true_val)
> 3 tqdm.write(f'Validation loss: {val_loss}')
> 4 tqdm.write(f'F1 Score (Weighted): {val_f1}')
>
> 6 frames
> /usr/local/lib/python3.6/dist-packages/torch/utils/data/dataset.py in (.0)
> 160
> 161 def **getitem**(self, index):
> --> 162 return tuple(tensor[index] for tensor in self.tensors)
> 163
> 164 def **len**(self):
>
> TypeError: new(): invalid data type 'str'
I got the same error and fix it .
you can check your input where there may be string contained.
such as
```
a = [1,2,3,4,'<unk>']
torch.tensor(a)
``` | I trying to build multi label text classifier model using Transformers lib.
I'm using Transformers NLP to load the data set, while calling trainer.train() method. It throws the following error
File "C:\***\arrow_dataset.py", line 343, in _convert_outputs
v = command(v)
TypeError: new(): invalid data type 'str'
I'm using pyarrow 1.0.0. And I have simple custom data set with Text and Integer Label.
Ex: Data
Text , Label #Column Header
I'm facing an Network issue, 1
I forgot my password, 2
Error StackTrace:
File "C:\**\transformers\trainer.py", line 492, in train
for step, inputs in enumerate(epoch_iterator):
File "C:\**\tqdm\std.py", line 1104, in __iter__
for obj in iterable:
File "C:\**\torch\utils\data\dataloader.py", line 345, in __next__
data = self._next_data()
File "C:\**\torch\utils\data\dataloader.py", line 385, in _next_data
data = self._dataset_fetcher.fetch(index) # may raise StopIteration
File "C:\**\torch\utils\data\_utils\fetch.py", line 44, in fetch
data = [self.dataset[idx] for idx in possibly_batched_index]
File "C:\**\torch\utils\data\_utils\fetch.py", line 44, in <listcomp>
data = [self.dataset[idx] for idx in possibly_batched_index]
File "C:\**\nlp\arrow_dataset.py", line 414, in __getitem__
output_all_columns=self._output_all_columns,
File "C:\**\nlp\arrow_dataset.py", line 403, in _getitem
outputs, format_type=format_type, format_columns=format_columns, output_all_columns=output_all_columns
File "C:\**\nlp\arrow_dataset.py", line 343, in _convert_outputs
v = command(v)
TypeError: new(): invalid data type 'str'
| 160 | invalid data type 'str' at _convert_outputs in arrow_dataset.py
I trying to build multi label text classifier model using Transformers lib.
I'm using Transformers NLP to load the data set, while calling trainer.train() method. It throws the following error
File "C:\***\arrow_dataset.py", line 343, in _convert_outputs
v = command(v)
TypeError: new(): invalid data type 'str'
I'm using pyarrow 1.0.0. And I have simple custom data set with Text and Integer Label.
Ex: Data
Text , Label #Column Header
I'm facing an Network issue, 1
I forgot my password, 2
Error StackTrace:
File "C:\**\transformers\trainer.py", line 492, in train
for step, inputs in enumerate(epoch_iterator):
File "C:\**\tqdm\std.py", line 1104, in __iter__
for obj in iterable:
File "C:\**\torch\utils\data\dataloader.py", line 345, in __next__
data = self._next_data()
File "C:\**\torch\utils\data\dataloader.py", line 385, in _next_data
data = self._dataset_fetcher.fetch(index) # may raise StopIteration
File "C:\**\torch\utils\data\_utils\fetch.py", line 44, in fetch
data = [self.dataset[idx] for idx in possibly_batched_index]
File "C:\**\torch\utils\data\_utils\fetch.py", line 44, in <listcomp>
data = [self.dataset[idx] for idx in possibly_batched_index]
File "C:\**\nlp\arrow_dataset.py", line 414, in __getitem__
output_all_columns=self._output_all_columns,
File "C:\**\nlp\arrow_dataset.py", line 403, in _getitem
outputs, format_type=format_type, format_columns=format_columns, output_all_columns=output_all_columns
File "C:\**\nlp\arrow_dataset.py", line 343, in _convert_outputs
v = command(v)
TypeError: new(): invalid data type 'str'
> Hi, actually the thing is I am getting the same error and even after tokenizing them I am passing them through batch_encode_plus.
> I dont know what seems to be the problem is. I even converted it into 'pt' while passing them through batch_encode_plus but when I am evaluating my model , i am getting this error
>
> TypeError Traceback (most recent call last)
> in ()
> ----> 1 val_loss, predictions, true_val = evaluate(dataloader_validation)
> 2 val_f1 = f1_score_func(predictions, true_val)
> 3 tqdm.write(f'Validation loss: {val_loss}')
> 4 tqdm.write(f'F1 Score (Weighted): {val_f1}')
>
> 6 frames
> /usr/local/lib/python3.6/dist-packages/torch/utils/data/dataset.py in (.0)
> 160
> 161 def **getitem**(self, index):
> --> 162 return tuple(tensor[index] for tensor in self.tensors)
> 163
> 164 def **len**(self):
>
> TypeError: new(): invalid data type 'str'
I got the same error and fix it .
you can check your input where there may be string contained.
such as
```
a = [1,2,3,4,'<unk>']
torch.tensor(a)
``` |
https://github.com/huggingface/datasets/issues/469 | invalid data type 'str' at _convert_outputs in arrow_dataset.py | I didn't know tokenizers could return strings in the token ids. Which tokenizer are you using to get this @Doragd ? | I trying to build multi label text classifier model using Transformers lib.
I'm using Transformers NLP to load the data set, while calling trainer.train() method. It throws the following error
File "C:\***\arrow_dataset.py", line 343, in _convert_outputs
v = command(v)
TypeError: new(): invalid data type 'str'
I'm using pyarrow 1.0.0. And I have simple custom data set with Text and Integer Label.
Ex: Data
Text , Label #Column Header
I'm facing an Network issue, 1
I forgot my password, 2
Error StackTrace:
File "C:\**\transformers\trainer.py", line 492, in train
for step, inputs in enumerate(epoch_iterator):
File "C:\**\tqdm\std.py", line 1104, in __iter__
for obj in iterable:
File "C:\**\torch\utils\data\dataloader.py", line 345, in __next__
data = self._next_data()
File "C:\**\torch\utils\data\dataloader.py", line 385, in _next_data
data = self._dataset_fetcher.fetch(index) # may raise StopIteration
File "C:\**\torch\utils\data\_utils\fetch.py", line 44, in fetch
data = [self.dataset[idx] for idx in possibly_batched_index]
File "C:\**\torch\utils\data\_utils\fetch.py", line 44, in <listcomp>
data = [self.dataset[idx] for idx in possibly_batched_index]
File "C:\**\nlp\arrow_dataset.py", line 414, in __getitem__
output_all_columns=self._output_all_columns,
File "C:\**\nlp\arrow_dataset.py", line 403, in _getitem
outputs, format_type=format_type, format_columns=format_columns, output_all_columns=output_all_columns
File "C:\**\nlp\arrow_dataset.py", line 343, in _convert_outputs
v = command(v)
TypeError: new(): invalid data type 'str'
| 21 | invalid data type 'str' at _convert_outputs in arrow_dataset.py
I trying to build multi label text classifier model using Transformers lib.
I'm using Transformers NLP to load the data set, while calling trainer.train() method. It throws the following error
File "C:\***\arrow_dataset.py", line 343, in _convert_outputs
v = command(v)
TypeError: new(): invalid data type 'str'
I'm using pyarrow 1.0.0. And I have simple custom data set with Text and Integer Label.
Ex: Data
Text , Label #Column Header
I'm facing an Network issue, 1
I forgot my password, 2
Error StackTrace:
File "C:\**\transformers\trainer.py", line 492, in train
for step, inputs in enumerate(epoch_iterator):
File "C:\**\tqdm\std.py", line 1104, in __iter__
for obj in iterable:
File "C:\**\torch\utils\data\dataloader.py", line 345, in __next__
data = self._next_data()
File "C:\**\torch\utils\data\dataloader.py", line 385, in _next_data
data = self._dataset_fetcher.fetch(index) # may raise StopIteration
File "C:\**\torch\utils\data\_utils\fetch.py", line 44, in fetch
data = [self.dataset[idx] for idx in possibly_batched_index]
File "C:\**\torch\utils\data\_utils\fetch.py", line 44, in <listcomp>
data = [self.dataset[idx] for idx in possibly_batched_index]
File "C:\**\nlp\arrow_dataset.py", line 414, in __getitem__
output_all_columns=self._output_all_columns,
File "C:\**\nlp\arrow_dataset.py", line 403, in _getitem
outputs, format_type=format_type, format_columns=format_columns, output_all_columns=output_all_columns
File "C:\**\nlp\arrow_dataset.py", line 343, in _convert_outputs
v = command(v)
TypeError: new(): invalid data type 'str'
I didn't know tokenizers could return strings in the token ids. Which tokenizer are you using to get this @Doragd ? |
https://github.com/huggingface/datasets/issues/469 | invalid data type 'str' at _convert_outputs in arrow_dataset.py | > I didn't know tokenizers could return strings in the token ids. Which tokenizer are you using to get this @Doragd ?
i'm sorry that i met this issue in another place (not in huggingface repo). | I trying to build multi label text classifier model using Transformers lib.
I'm using Transformers NLP to load the data set, while calling trainer.train() method. It throws the following error
File "C:\***\arrow_dataset.py", line 343, in _convert_outputs
v = command(v)
TypeError: new(): invalid data type 'str'
I'm using pyarrow 1.0.0. And I have simple custom data set with Text and Integer Label.
Ex: Data
Text , Label #Column Header
I'm facing an Network issue, 1
I forgot my password, 2
Error StackTrace:
File "C:\**\transformers\trainer.py", line 492, in train
for step, inputs in enumerate(epoch_iterator):
File "C:\**\tqdm\std.py", line 1104, in __iter__
for obj in iterable:
File "C:\**\torch\utils\data\dataloader.py", line 345, in __next__
data = self._next_data()
File "C:\**\torch\utils\data\dataloader.py", line 385, in _next_data
data = self._dataset_fetcher.fetch(index) # may raise StopIteration
File "C:\**\torch\utils\data\_utils\fetch.py", line 44, in fetch
data = [self.dataset[idx] for idx in possibly_batched_index]
File "C:\**\torch\utils\data\_utils\fetch.py", line 44, in <listcomp>
data = [self.dataset[idx] for idx in possibly_batched_index]
File "C:\**\nlp\arrow_dataset.py", line 414, in __getitem__
output_all_columns=self._output_all_columns,
File "C:\**\nlp\arrow_dataset.py", line 403, in _getitem
outputs, format_type=format_type, format_columns=format_columns, output_all_columns=output_all_columns
File "C:\**\nlp\arrow_dataset.py", line 343, in _convert_outputs
v = command(v)
TypeError: new(): invalid data type 'str'
| 36 | invalid data type 'str' at _convert_outputs in arrow_dataset.py
I trying to build multi label text classifier model using Transformers lib.
I'm using Transformers NLP to load the data set, while calling trainer.train() method. It throws the following error
File "C:\***\arrow_dataset.py", line 343, in _convert_outputs
v = command(v)
TypeError: new(): invalid data type 'str'
I'm using pyarrow 1.0.0. And I have simple custom data set with Text and Integer Label.
Ex: Data
Text , Label #Column Header
I'm facing an Network issue, 1
I forgot my password, 2
Error StackTrace:
File "C:\**\transformers\trainer.py", line 492, in train
for step, inputs in enumerate(epoch_iterator):
File "C:\**\tqdm\std.py", line 1104, in __iter__
for obj in iterable:
File "C:\**\torch\utils\data\dataloader.py", line 345, in __next__
data = self._next_data()
File "C:\**\torch\utils\data\dataloader.py", line 385, in _next_data
data = self._dataset_fetcher.fetch(index) # may raise StopIteration
File "C:\**\torch\utils\data\_utils\fetch.py", line 44, in fetch
data = [self.dataset[idx] for idx in possibly_batched_index]
File "C:\**\torch\utils\data\_utils\fetch.py", line 44, in <listcomp>
data = [self.dataset[idx] for idx in possibly_batched_index]
File "C:\**\nlp\arrow_dataset.py", line 414, in __getitem__
output_all_columns=self._output_all_columns,
File "C:\**\nlp\arrow_dataset.py", line 403, in _getitem
outputs, format_type=format_type, format_columns=format_columns, output_all_columns=output_all_columns
File "C:\**\nlp\arrow_dataset.py", line 343, in _convert_outputs
v = command(v)
TypeError: new(): invalid data type 'str'
> I didn't know tokenizers could return strings in the token ids. Which tokenizer are you using to get this @Doragd ?
i'm sorry that i met this issue in another place (not in huggingface repo). |
https://github.com/huggingface/datasets/issues/469 | invalid data type 'str' at _convert_outputs in arrow_dataset.py | @akhilkapil do you have strings in your dataset ? When you set the dataset format to "pytorch" you should exclude columns with strings as pytorch can't make tensors out of strings | I trying to build multi label text classifier model using Transformers lib.
I'm using Transformers NLP to load the data set, while calling trainer.train() method. It throws the following error
File "C:\***\arrow_dataset.py", line 343, in _convert_outputs
v = command(v)
TypeError: new(): invalid data type 'str'
I'm using pyarrow 1.0.0. And I have simple custom data set with Text and Integer Label.
Ex: Data
Text , Label #Column Header
I'm facing an Network issue, 1
I forgot my password, 2
Error StackTrace:
File "C:\**\transformers\trainer.py", line 492, in train
for step, inputs in enumerate(epoch_iterator):
File "C:\**\tqdm\std.py", line 1104, in __iter__
for obj in iterable:
File "C:\**\torch\utils\data\dataloader.py", line 345, in __next__
data = self._next_data()
File "C:\**\torch\utils\data\dataloader.py", line 385, in _next_data
data = self._dataset_fetcher.fetch(index) # may raise StopIteration
File "C:\**\torch\utils\data\_utils\fetch.py", line 44, in fetch
data = [self.dataset[idx] for idx in possibly_batched_index]
File "C:\**\torch\utils\data\_utils\fetch.py", line 44, in <listcomp>
data = [self.dataset[idx] for idx in possibly_batched_index]
File "C:\**\nlp\arrow_dataset.py", line 414, in __getitem__
output_all_columns=self._output_all_columns,
File "C:\**\nlp\arrow_dataset.py", line 403, in _getitem
outputs, format_type=format_type, format_columns=format_columns, output_all_columns=output_all_columns
File "C:\**\nlp\arrow_dataset.py", line 343, in _convert_outputs
v = command(v)
TypeError: new(): invalid data type 'str'
| 31 | invalid data type 'str' at _convert_outputs in arrow_dataset.py
I trying to build multi label text classifier model using Transformers lib.
I'm using Transformers NLP to load the data set, while calling trainer.train() method. It throws the following error
File "C:\***\arrow_dataset.py", line 343, in _convert_outputs
v = command(v)
TypeError: new(): invalid data type 'str'
I'm using pyarrow 1.0.0. And I have simple custom data set with Text and Integer Label.
Ex: Data
Text , Label #Column Header
I'm facing an Network issue, 1
I forgot my password, 2
Error StackTrace:
File "C:\**\transformers\trainer.py", line 492, in train
for step, inputs in enumerate(epoch_iterator):
File "C:\**\tqdm\std.py", line 1104, in __iter__
for obj in iterable:
File "C:\**\torch\utils\data\dataloader.py", line 345, in __next__
data = self._next_data()
File "C:\**\torch\utils\data\dataloader.py", line 385, in _next_data
data = self._dataset_fetcher.fetch(index) # may raise StopIteration
File "C:\**\torch\utils\data\_utils\fetch.py", line 44, in fetch
data = [self.dataset[idx] for idx in possibly_batched_index]
File "C:\**\torch\utils\data\_utils\fetch.py", line 44, in <listcomp>
data = [self.dataset[idx] for idx in possibly_batched_index]
File "C:\**\nlp\arrow_dataset.py", line 414, in __getitem__
output_all_columns=self._output_all_columns,
File "C:\**\nlp\arrow_dataset.py", line 403, in _getitem
outputs, format_type=format_type, format_columns=format_columns, output_all_columns=output_all_columns
File "C:\**\nlp\arrow_dataset.py", line 343, in _convert_outputs
v = command(v)
TypeError: new(): invalid data type 'str'
@akhilkapil do you have strings in your dataset ? When you set the dataset format to "pytorch" you should exclude columns with strings as pytorch can't make tensors out of strings |
https://github.com/huggingface/datasets/issues/468 | UnicodeDecodeError while loading PAN-X task of XTREME dataset | Indeed. Solution 1 is the simplest.
This is actually a recurring problem.
I think we should scan all the datasets with regexpr to fix the use of `open()` without encodings.
And probably add a test in the CI to forbid using this in the future. | Hi π€ team!
## Description of the problem
I'm running into a `UnicodeDecodeError` while trying to load the PAN-X subset the XTREME dataset:
```
---------------------------------------------------------------------------
UnicodeDecodeError Traceback (most recent call last)
<ipython-input-5-1d61f439b843> in <module>
----> 1 dataset = load_dataset("xtreme", "PAN-X.en", data_dir='./data')
/usr/local/lib/python3.6/dist-packages/nlp/load.py in load_dataset(path, name, version, data_dir, data_files, split, cache_dir, features, download_config, download_mode, ignore_verifications, save_infos, **config_kwargs)
528 ignore_verifications = ignore_verifications or save_infos
529 # Download/copy dataset processing script
--> 530 module_path, hash = prepare_module(path, download_config=download_config, dataset=True)
531
532 # Get dataset builder class from the processing script
/usr/local/lib/python3.6/dist-packages/nlp/load.py in prepare_module(path, download_config, dataset, force_local_path, **download_kwargs)
265
266 # Download external imports if needed
--> 267 imports = get_imports(local_path)
268 local_imports = []
269 library_imports = []
/usr/local/lib/python3.6/dist-packages/nlp/load.py in get_imports(file_path)
156 lines = []
157 with open(file_path, mode="r") as f:
--> 158 lines.extend(f.readlines())
159
160 logger.info("Checking %s for additional imports.", file_path)
/usr/lib/python3.6/encodings/ascii.py in decode(self, input, final)
24 class IncrementalDecoder(codecs.IncrementalDecoder):
25 def decode(self, input, final=False):
---> 26 return codecs.ascii_decode(input, self.errors)[0]
27
28 class StreamWriter(Codec,codecs.StreamWriter):
UnicodeDecodeError: 'ascii' codec can't decode byte 0xe2 in position 111: ordinal not in range(128)
```
## Steps to reproduce
Install from nlp's master branch
```python
pip install git+https://github.com/huggingface/nlp.git
```
then run
```python
from nlp import load_dataset
# AmazonPhotos.zip is located in data/
dataset = load_dataset("xtreme", "PAN-X.en", data_dir='./data')
```
## OS / platform details
- `nlp` version: latest from master
- Platform: Linux-4.15.0-72-generic-x86_64-with-Ubuntu-18.04-bionic
- Python version: 3.6.9
- PyTorch version (GPU?): 1.4.0 (True)
- Tensorflow version (GPU?): 2.1.0 (True)
- Using GPU in script?: True
- Using distributed or parallel set-up in script?: False
## Proposed solution
Either change [line 762](https://github.com/huggingface/nlp/blob/7ada00b1d62f94eee22a7df38c6b01e3f27194b7/datasets/xtreme/xtreme.py#L762) in `xtreme.py` to include UTF-8 encoding:
```
# old
with open(filepath) as f
# new
with open(filepath, encoding='utf-8') as f
```
or raise a warning that suggests setting the locale explicitly, e.g.
```python
import locale
locale.setlocale(locale.LC_ALL, 'C.UTF-8')
```
I have a preference for the first solution. Let me know if you agree and I'll be happy to implement the simple fix! | 45 | UnicodeDecodeError while loading PAN-X task of XTREME dataset
Hi π€ team!
## Description of the problem
I'm running into a `UnicodeDecodeError` while trying to load the PAN-X subset the XTREME dataset:
```
---------------------------------------------------------------------------
UnicodeDecodeError Traceback (most recent call last)
<ipython-input-5-1d61f439b843> in <module>
----> 1 dataset = load_dataset("xtreme", "PAN-X.en", data_dir='./data')
/usr/local/lib/python3.6/dist-packages/nlp/load.py in load_dataset(path, name, version, data_dir, data_files, split, cache_dir, features, download_config, download_mode, ignore_verifications, save_infos, **config_kwargs)
528 ignore_verifications = ignore_verifications or save_infos
529 # Download/copy dataset processing script
--> 530 module_path, hash = prepare_module(path, download_config=download_config, dataset=True)
531
532 # Get dataset builder class from the processing script
/usr/local/lib/python3.6/dist-packages/nlp/load.py in prepare_module(path, download_config, dataset, force_local_path, **download_kwargs)
265
266 # Download external imports if needed
--> 267 imports = get_imports(local_path)
268 local_imports = []
269 library_imports = []
/usr/local/lib/python3.6/dist-packages/nlp/load.py in get_imports(file_path)
156 lines = []
157 with open(file_path, mode="r") as f:
--> 158 lines.extend(f.readlines())
159
160 logger.info("Checking %s for additional imports.", file_path)
/usr/lib/python3.6/encodings/ascii.py in decode(self, input, final)
24 class IncrementalDecoder(codecs.IncrementalDecoder):
25 def decode(self, input, final=False):
---> 26 return codecs.ascii_decode(input, self.errors)[0]
27
28 class StreamWriter(Codec,codecs.StreamWriter):
UnicodeDecodeError: 'ascii' codec can't decode byte 0xe2 in position 111: ordinal not in range(128)
```
## Steps to reproduce
Install from nlp's master branch
```python
pip install git+https://github.com/huggingface/nlp.git
```
then run
```python
from nlp import load_dataset
# AmazonPhotos.zip is located in data/
dataset = load_dataset("xtreme", "PAN-X.en", data_dir='./data')
```
## OS / platform details
- `nlp` version: latest from master
- Platform: Linux-4.15.0-72-generic-x86_64-with-Ubuntu-18.04-bionic
- Python version: 3.6.9
- PyTorch version (GPU?): 1.4.0 (True)
- Tensorflow version (GPU?): 2.1.0 (True)
- Using GPU in script?: True
- Using distributed or parallel set-up in script?: False
## Proposed solution
Either change [line 762](https://github.com/huggingface/nlp/blob/7ada00b1d62f94eee22a7df38c6b01e3f27194b7/datasets/xtreme/xtreme.py#L762) in `xtreme.py` to include UTF-8 encoding:
```
# old
with open(filepath) as f
# new
with open(filepath, encoding='utf-8') as f
```
or raise a warning that suggests setting the locale explicitly, e.g.
```python
import locale
locale.setlocale(locale.LC_ALL, 'C.UTF-8')
```
I have a preference for the first solution. Let me know if you agree and I'll be happy to implement the simple fix!
Indeed. Solution 1 is the simplest.
This is actually a recurring problem.
I think we should scan all the datasets with regexpr to fix the use of `open()` without encodings.
And probably add a test in the CI to forbid using this in the future. |
https://github.com/huggingface/datasets/issues/468 | UnicodeDecodeError while loading PAN-X task of XTREME dataset | I've created a simple function that seems to do the trick:
```python
def apply_encoding_on_file_open(filepath: str):
"""Apply UTF-8 encoding for all instances where a non-binary file is opened."""
with open(filepath, 'r', encoding='utf-8') as input_file:
regexp = re.compile(r"""
(?!.*\b(?:encoding|rb|wb|wb+|ab|ab+)\b)
(open)
\((.*)\)
""")
input_text = input_file.read()
match = regexp.search(input_text)
if match:
print('Found match!', match.group())
# append utf-8 encoding to matching groups in-place
output = regexp.sub(lambda m: m.group()[:-1]+', encoding="utf-8")', input_text)
with open(filepath, 'w', encoding='utf-8') as output_file:
output_file.write(output)
else:
print("No match found!")
```
The regexp does a negative lookahead to avoid matching on cases where the encoding is already specified or when binary files are involved.
From an implementation perspective:
* Would it make sense to include this function in `nlp-cli` so that we can run something like
```
nlp-cli fix_encoding path/to/folder
```
and the command recursively fixes all files in the target?
* What is the desired behaviour in the CI test? Here we could either have a simple script that we run as a `job` in the CI and raises an error if a missing encoding is detected. Alternatively we could incorporate this behaviour into the CLI and run that in the CI.
Please let me know what you prefer among the alternatives.
| Hi π€ team!
## Description of the problem
I'm running into a `UnicodeDecodeError` while trying to load the PAN-X subset the XTREME dataset:
```
---------------------------------------------------------------------------
UnicodeDecodeError Traceback (most recent call last)
<ipython-input-5-1d61f439b843> in <module>
----> 1 dataset = load_dataset("xtreme", "PAN-X.en", data_dir='./data')
/usr/local/lib/python3.6/dist-packages/nlp/load.py in load_dataset(path, name, version, data_dir, data_files, split, cache_dir, features, download_config, download_mode, ignore_verifications, save_infos, **config_kwargs)
528 ignore_verifications = ignore_verifications or save_infos
529 # Download/copy dataset processing script
--> 530 module_path, hash = prepare_module(path, download_config=download_config, dataset=True)
531
532 # Get dataset builder class from the processing script
/usr/local/lib/python3.6/dist-packages/nlp/load.py in prepare_module(path, download_config, dataset, force_local_path, **download_kwargs)
265
266 # Download external imports if needed
--> 267 imports = get_imports(local_path)
268 local_imports = []
269 library_imports = []
/usr/local/lib/python3.6/dist-packages/nlp/load.py in get_imports(file_path)
156 lines = []
157 with open(file_path, mode="r") as f:
--> 158 lines.extend(f.readlines())
159
160 logger.info("Checking %s for additional imports.", file_path)
/usr/lib/python3.6/encodings/ascii.py in decode(self, input, final)
24 class IncrementalDecoder(codecs.IncrementalDecoder):
25 def decode(self, input, final=False):
---> 26 return codecs.ascii_decode(input, self.errors)[0]
27
28 class StreamWriter(Codec,codecs.StreamWriter):
UnicodeDecodeError: 'ascii' codec can't decode byte 0xe2 in position 111: ordinal not in range(128)
```
## Steps to reproduce
Install from nlp's master branch
```python
pip install git+https://github.com/huggingface/nlp.git
```
then run
```python
from nlp import load_dataset
# AmazonPhotos.zip is located in data/
dataset = load_dataset("xtreme", "PAN-X.en", data_dir='./data')
```
## OS / platform details
- `nlp` version: latest from master
- Platform: Linux-4.15.0-72-generic-x86_64-with-Ubuntu-18.04-bionic
- Python version: 3.6.9
- PyTorch version (GPU?): 1.4.0 (True)
- Tensorflow version (GPU?): 2.1.0 (True)
- Using GPU in script?: True
- Using distributed or parallel set-up in script?: False
## Proposed solution
Either change [line 762](https://github.com/huggingface/nlp/blob/7ada00b1d62f94eee22a7df38c6b01e3f27194b7/datasets/xtreme/xtreme.py#L762) in `xtreme.py` to include UTF-8 encoding:
```
# old
with open(filepath) as f
# new
with open(filepath, encoding='utf-8') as f
```
or raise a warning that suggests setting the locale explicitly, e.g.
```python
import locale
locale.setlocale(locale.LC_ALL, 'C.UTF-8')
```
I have a preference for the first solution. Let me know if you agree and I'll be happy to implement the simple fix! | 200 | UnicodeDecodeError while loading PAN-X task of XTREME dataset
Hi π€ team!
## Description of the problem
I'm running into a `UnicodeDecodeError` while trying to load the PAN-X subset the XTREME dataset:
```
---------------------------------------------------------------------------
UnicodeDecodeError Traceback (most recent call last)
<ipython-input-5-1d61f439b843> in <module>
----> 1 dataset = load_dataset("xtreme", "PAN-X.en", data_dir='./data')
/usr/local/lib/python3.6/dist-packages/nlp/load.py in load_dataset(path, name, version, data_dir, data_files, split, cache_dir, features, download_config, download_mode, ignore_verifications, save_infos, **config_kwargs)
528 ignore_verifications = ignore_verifications or save_infos
529 # Download/copy dataset processing script
--> 530 module_path, hash = prepare_module(path, download_config=download_config, dataset=True)
531
532 # Get dataset builder class from the processing script
/usr/local/lib/python3.6/dist-packages/nlp/load.py in prepare_module(path, download_config, dataset, force_local_path, **download_kwargs)
265
266 # Download external imports if needed
--> 267 imports = get_imports(local_path)
268 local_imports = []
269 library_imports = []
/usr/local/lib/python3.6/dist-packages/nlp/load.py in get_imports(file_path)
156 lines = []
157 with open(file_path, mode="r") as f:
--> 158 lines.extend(f.readlines())
159
160 logger.info("Checking %s for additional imports.", file_path)
/usr/lib/python3.6/encodings/ascii.py in decode(self, input, final)
24 class IncrementalDecoder(codecs.IncrementalDecoder):
25 def decode(self, input, final=False):
---> 26 return codecs.ascii_decode(input, self.errors)[0]
27
28 class StreamWriter(Codec,codecs.StreamWriter):
UnicodeDecodeError: 'ascii' codec can't decode byte 0xe2 in position 111: ordinal not in range(128)
```
## Steps to reproduce
Install from nlp's master branch
```python
pip install git+https://github.com/huggingface/nlp.git
```
then run
```python
from nlp import load_dataset
# AmazonPhotos.zip is located in data/
dataset = load_dataset("xtreme", "PAN-X.en", data_dir='./data')
```
## OS / platform details
- `nlp` version: latest from master
- Platform: Linux-4.15.0-72-generic-x86_64-with-Ubuntu-18.04-bionic
- Python version: 3.6.9
- PyTorch version (GPU?): 1.4.0 (True)
- Tensorflow version (GPU?): 2.1.0 (True)
- Using GPU in script?: True
- Using distributed or parallel set-up in script?: False
## Proposed solution
Either change [line 762](https://github.com/huggingface/nlp/blob/7ada00b1d62f94eee22a7df38c6b01e3f27194b7/datasets/xtreme/xtreme.py#L762) in `xtreme.py` to include UTF-8 encoding:
```
# old
with open(filepath) as f
# new
with open(filepath, encoding='utf-8') as f
```
or raise a warning that suggests setting the locale explicitly, e.g.
```python
import locale
locale.setlocale(locale.LC_ALL, 'C.UTF-8')
```
I have a preference for the first solution. Let me know if you agree and I'll be happy to implement the simple fix!
I've created a simple function that seems to do the trick:
```python
def apply_encoding_on_file_open(filepath: str):
"""Apply UTF-8 encoding for all instances where a non-binary file is opened."""
with open(filepath, 'r', encoding='utf-8') as input_file:
regexp = re.compile(r"""
(?!.*\b(?:encoding|rb|wb|wb+|ab|ab+)\b)
(open)
\((.*)\)
""")
input_text = input_file.read()
match = regexp.search(input_text)
if match:
print('Found match!', match.group())
# append utf-8 encoding to matching groups in-place
output = regexp.sub(lambda m: m.group()[:-1]+', encoding="utf-8")', input_text)
with open(filepath, 'w', encoding='utf-8') as output_file:
output_file.write(output)
else:
print("No match found!")
```
The regexp does a negative lookahead to avoid matching on cases where the encoding is already specified or when binary files are involved.
From an implementation perspective:
* Would it make sense to include this function in `nlp-cli` so that we can run something like
```
nlp-cli fix_encoding path/to/folder
```
and the command recursively fixes all files in the target?
* What is the desired behaviour in the CI test? Here we could either have a simple script that we run as a `job` in the CI and raises an error if a missing encoding is detected. Alternatively we could incorporate this behaviour into the CLI and run that in the CI.
Please let me know what you prefer among the alternatives.
|
https://github.com/huggingface/datasets/issues/468 | UnicodeDecodeError while loading PAN-X task of XTREME dataset | I realised I was overthinking the problem, so decided to just run the regexp over the codebase and make the PR. In other words, we can ignore my comments about using the CLI πΈ | Hi π€ team!
## Description of the problem
I'm running into a `UnicodeDecodeError` while trying to load the PAN-X subset the XTREME dataset:
```
---------------------------------------------------------------------------
UnicodeDecodeError Traceback (most recent call last)
<ipython-input-5-1d61f439b843> in <module>
----> 1 dataset = load_dataset("xtreme", "PAN-X.en", data_dir='./data')
/usr/local/lib/python3.6/dist-packages/nlp/load.py in load_dataset(path, name, version, data_dir, data_files, split, cache_dir, features, download_config, download_mode, ignore_verifications, save_infos, **config_kwargs)
528 ignore_verifications = ignore_verifications or save_infos
529 # Download/copy dataset processing script
--> 530 module_path, hash = prepare_module(path, download_config=download_config, dataset=True)
531
532 # Get dataset builder class from the processing script
/usr/local/lib/python3.6/dist-packages/nlp/load.py in prepare_module(path, download_config, dataset, force_local_path, **download_kwargs)
265
266 # Download external imports if needed
--> 267 imports = get_imports(local_path)
268 local_imports = []
269 library_imports = []
/usr/local/lib/python3.6/dist-packages/nlp/load.py in get_imports(file_path)
156 lines = []
157 with open(file_path, mode="r") as f:
--> 158 lines.extend(f.readlines())
159
160 logger.info("Checking %s for additional imports.", file_path)
/usr/lib/python3.6/encodings/ascii.py in decode(self, input, final)
24 class IncrementalDecoder(codecs.IncrementalDecoder):
25 def decode(self, input, final=False):
---> 26 return codecs.ascii_decode(input, self.errors)[0]
27
28 class StreamWriter(Codec,codecs.StreamWriter):
UnicodeDecodeError: 'ascii' codec can't decode byte 0xe2 in position 111: ordinal not in range(128)
```
## Steps to reproduce
Install from nlp's master branch
```python
pip install git+https://github.com/huggingface/nlp.git
```
then run
```python
from nlp import load_dataset
# AmazonPhotos.zip is located in data/
dataset = load_dataset("xtreme", "PAN-X.en", data_dir='./data')
```
## OS / platform details
- `nlp` version: latest from master
- Platform: Linux-4.15.0-72-generic-x86_64-with-Ubuntu-18.04-bionic
- Python version: 3.6.9
- PyTorch version (GPU?): 1.4.0 (True)
- Tensorflow version (GPU?): 2.1.0 (True)
- Using GPU in script?: True
- Using distributed or parallel set-up in script?: False
## Proposed solution
Either change [line 762](https://github.com/huggingface/nlp/blob/7ada00b1d62f94eee22a7df38c6b01e3f27194b7/datasets/xtreme/xtreme.py#L762) in `xtreme.py` to include UTF-8 encoding:
```
# old
with open(filepath) as f
# new
with open(filepath, encoding='utf-8') as f
```
or raise a warning that suggests setting the locale explicitly, e.g.
```python
import locale
locale.setlocale(locale.LC_ALL, 'C.UTF-8')
```
I have a preference for the first solution. Let me know if you agree and I'll be happy to implement the simple fix! | 34 | UnicodeDecodeError while loading PAN-X task of XTREME dataset
Hi π€ team!
## Description of the problem
I'm running into a `UnicodeDecodeError` while trying to load the PAN-X subset the XTREME dataset:
```
---------------------------------------------------------------------------
UnicodeDecodeError Traceback (most recent call last)
<ipython-input-5-1d61f439b843> in <module>
----> 1 dataset = load_dataset("xtreme", "PAN-X.en", data_dir='./data')
/usr/local/lib/python3.6/dist-packages/nlp/load.py in load_dataset(path, name, version, data_dir, data_files, split, cache_dir, features, download_config, download_mode, ignore_verifications, save_infos, **config_kwargs)
528 ignore_verifications = ignore_verifications or save_infos
529 # Download/copy dataset processing script
--> 530 module_path, hash = prepare_module(path, download_config=download_config, dataset=True)
531
532 # Get dataset builder class from the processing script
/usr/local/lib/python3.6/dist-packages/nlp/load.py in prepare_module(path, download_config, dataset, force_local_path, **download_kwargs)
265
266 # Download external imports if needed
--> 267 imports = get_imports(local_path)
268 local_imports = []
269 library_imports = []
/usr/local/lib/python3.6/dist-packages/nlp/load.py in get_imports(file_path)
156 lines = []
157 with open(file_path, mode="r") as f:
--> 158 lines.extend(f.readlines())
159
160 logger.info("Checking %s for additional imports.", file_path)
/usr/lib/python3.6/encodings/ascii.py in decode(self, input, final)
24 class IncrementalDecoder(codecs.IncrementalDecoder):
25 def decode(self, input, final=False):
---> 26 return codecs.ascii_decode(input, self.errors)[0]
27
28 class StreamWriter(Codec,codecs.StreamWriter):
UnicodeDecodeError: 'ascii' codec can't decode byte 0xe2 in position 111: ordinal not in range(128)
```
## Steps to reproduce
Install from nlp's master branch
```python
pip install git+https://github.com/huggingface/nlp.git
```
then run
```python
from nlp import load_dataset
# AmazonPhotos.zip is located in data/
dataset = load_dataset("xtreme", "PAN-X.en", data_dir='./data')
```
## OS / platform details
- `nlp` version: latest from master
- Platform: Linux-4.15.0-72-generic-x86_64-with-Ubuntu-18.04-bionic
- Python version: 3.6.9
- PyTorch version (GPU?): 1.4.0 (True)
- Tensorflow version (GPU?): 2.1.0 (True)
- Using GPU in script?: True
- Using distributed or parallel set-up in script?: False
## Proposed solution
Either change [line 762](https://github.com/huggingface/nlp/blob/7ada00b1d62f94eee22a7df38c6b01e3f27194b7/datasets/xtreme/xtreme.py#L762) in `xtreme.py` to include UTF-8 encoding:
```
# old
with open(filepath) as f
# new
with open(filepath, encoding='utf-8') as f
```
or raise a warning that suggests setting the locale explicitly, e.g.
```python
import locale
locale.setlocale(locale.LC_ALL, 'C.UTF-8')
```
I have a preference for the first solution. Let me know if you agree and I'll be happy to implement the simple fix!
I realised I was overthinking the problem, so decided to just run the regexp over the codebase and make the PR. In other words, we can ignore my comments about using the CLI πΈ |
https://github.com/huggingface/datasets/issues/444 | Keep loading old file even I specify a new file in load_dataset | This is the only fix I could come up with without touching the repo's code.
```python
from nlp.builder import FORCE_REDOWNLOAD
dataset = load_dataset('csv', data_file='./a.csv', download_mode=FORCE_REDOWNLOAD, version='0.0.1')
```
You'll have to change the version each time you want to load a different csv file.
If you're willing to add a ```print```, you can go to ```nlp.load``` and add ```print(builder_instance.cache_dir)``` right before the ```return ds``` in the ```load_dataset``` method. It'll print the cache folder, and you'll just have to erase it (and then you won't need the change here above). | I used load a file called 'a.csv' by
```
dataset = load_dataset('csv', data_file='./a.csv')
```
And after a while, I tried to load another csv called 'b.csv'
```
dataset = load_dataset('csv', data_file='./b.csv')
```
However, the new dataset seems to remain the old 'a.csv' and not loading new csv file.
Even worse, after I load a.csv, the load_dataset function keeps loading the 'a.csv' afterward.
Is this a cache problem?
| 88 | Keep loading old file even I specify a new file in load_dataset
I used load a file called 'a.csv' by
```
dataset = load_dataset('csv', data_file='./a.csv')
```
And after a while, I tried to load another csv called 'b.csv'
```
dataset = load_dataset('csv', data_file='./b.csv')
```
However, the new dataset seems to remain the old 'a.csv' and not loading new csv file.
Even worse, after I load a.csv, the load_dataset function keeps loading the 'a.csv' afterward.
Is this a cache problem?
This is the only fix I could come up with without touching the repo's code.
```python
from nlp.builder import FORCE_REDOWNLOAD
dataset = load_dataset('csv', data_file='./a.csv', download_mode=FORCE_REDOWNLOAD, version='0.0.1')
```
You'll have to change the version each time you want to load a different csv file.
If you're willing to add a ```print```, you can go to ```nlp.load``` and add ```print(builder_instance.cache_dir)``` right before the ```return ds``` in the ```load_dataset``` method. It'll print the cache folder, and you'll just have to erase it (and then you won't need the change here above). |
https://github.com/huggingface/datasets/issues/443 | Cannot unpickle saved .pt dataset with torch.save()/load() | This seems to be fixed in a non-released version.
Installing nlp from source
```
git clone https://github.com/huggingface/nlp
cd nlp
pip install .
```
solves the issue. | Saving a formatted torch dataset to file using `torch.save()`. Loading the same file fails during unpickling:
```python
>>> import torch
>>> import nlp
>>> squad = nlp.load_dataset("squad.py", split="train")
>>> squad
Dataset(features: {'source_text': Value(dtype='string', id=None), 'target_text': Value(dtype='string', id=None)}, num_rows: 87599)
>>> squad = squad.map(create_features, batched=True)
>>> squad.set_format(type="torch", columns=["source_ids", "target_ids", "attention_mask"])
>>> torch.save(squad, "squad.pt")
>>> squad_pt = torch.load("squad.pt")
Traceback (most recent call last):
File "<stdin>", line 1, in <module>
File "/home/vegarab/.conda/envs/torch/lib/python3.7/site-packages/torch/serialization.py", line 593, in load
return _legacy_load(opened_file, map_location, pickle_module, **pickle_load_args)
File "/home/vegarab/.conda/envs/torch/lib/python3.7/site-packages/torch/serialization.py", line 773, in _legacy_load
result = unpickler.load()
File "/home/vegarab/.conda/envs/torch/lib/python3.7/site-packages/nlp/splits.py", line 493, in __setitem__
raise ValueError("Cannot add elem. Use .add() instead.")
ValueError: Cannot add elem. Use .add() instead.
```
where `create_features` is a function that tokenizes the data using `batch_encode_plus` and returns a Dict with `input_ids`, `target_ids` and `attention_mask`.
```python
def create_features(batch):
source_text_encoding = tokenizer.batch_encode_plus(
batch["source_text"],
max_length=max_source_length,
pad_to_max_length=True,
truncation=True)
target_text_encoding = tokenizer.batch_encode_plus(
batch["target_text"],
max_length=max_target_length,
pad_to_max_length=True,
truncation=True)
features = {
"source_ids": source_text_encoding["input_ids"],
"target_ids": target_text_encoding["input_ids"],
"attention_mask": source_text_encoding["attention_mask"]
}
return features
```
I found a similar issue in [issue 5267 in the huggingface/transformers repo](https://github.com/huggingface/transformers/issues/5267) which was solved by downgrading to `nlp==0.2.0`. That did not solve this problem, however. | 26 | Cannot unpickle saved .pt dataset with torch.save()/load()
Saving a formatted torch dataset to file using `torch.save()`. Loading the same file fails during unpickling:
```python
>>> import torch
>>> import nlp
>>> squad = nlp.load_dataset("squad.py", split="train")
>>> squad
Dataset(features: {'source_text': Value(dtype='string', id=None), 'target_text': Value(dtype='string', id=None)}, num_rows: 87599)
>>> squad = squad.map(create_features, batched=True)
>>> squad.set_format(type="torch", columns=["source_ids", "target_ids", "attention_mask"])
>>> torch.save(squad, "squad.pt")
>>> squad_pt = torch.load("squad.pt")
Traceback (most recent call last):
File "<stdin>", line 1, in <module>
File "/home/vegarab/.conda/envs/torch/lib/python3.7/site-packages/torch/serialization.py", line 593, in load
return _legacy_load(opened_file, map_location, pickle_module, **pickle_load_args)
File "/home/vegarab/.conda/envs/torch/lib/python3.7/site-packages/torch/serialization.py", line 773, in _legacy_load
result = unpickler.load()
File "/home/vegarab/.conda/envs/torch/lib/python3.7/site-packages/nlp/splits.py", line 493, in __setitem__
raise ValueError("Cannot add elem. Use .add() instead.")
ValueError: Cannot add elem. Use .add() instead.
```
where `create_features` is a function that tokenizes the data using `batch_encode_plus` and returns a Dict with `input_ids`, `target_ids` and `attention_mask`.
```python
def create_features(batch):
source_text_encoding = tokenizer.batch_encode_plus(
batch["source_text"],
max_length=max_source_length,
pad_to_max_length=True,
truncation=True)
target_text_encoding = tokenizer.batch_encode_plus(
batch["target_text"],
max_length=max_target_length,
pad_to_max_length=True,
truncation=True)
features = {
"source_ids": source_text_encoding["input_ids"],
"target_ids": target_text_encoding["input_ids"],
"attention_mask": source_text_encoding["attention_mask"]
}
return features
```
I found a similar issue in [issue 5267 in the huggingface/transformers repo](https://github.com/huggingface/transformers/issues/5267) which was solved by downgrading to `nlp==0.2.0`. That did not solve this problem, however.
This seems to be fixed in a non-released version.
Installing nlp from source
```
git clone https://github.com/huggingface/nlp
cd nlp
pip install .
```
solves the issue. |
https://github.com/huggingface/datasets/issues/439 | Issues: Adding a FAISS or Elastic Search index to a Dataset | `DPRContextEncoder` and `DPRContextEncoderTokenizer` will be available in the next release of `transformers`.
Right now you can experiment with it by installing `transformers` from the master branch.
You can also check the docs of DPR [here](https://huggingface.co/transformers/master/model_doc/dpr.html).
Moreover all the indexing features will also be available in the next release of `nlp`. | It seems the DPRContextEncoder, DPRContextEncoderTokenizer cited[ in this documentation](https://huggingface.co/nlp/faiss_and_ea.html) is not implemented ? It didnot work with the standard nlp installation . Also, I couldn't find or use it with the latest nlp install from github in Colab. Is there any dependency on the latest PyArrow 1.0.0 ? Is it yet to be made generally available ? | 50 | Issues: Adding a FAISS or Elastic Search index to a Dataset
It seems the DPRContextEncoder, DPRContextEncoderTokenizer cited[ in this documentation](https://huggingface.co/nlp/faiss_and_ea.html) is not implemented ? It didnot work with the standard nlp installation . Also, I couldn't find or use it with the latest nlp install from github in Colab. Is there any dependency on the latest PyArrow 1.0.0 ? Is it yet to be made generally available ?
`DPRContextEncoder` and `DPRContextEncoderTokenizer` will be available in the next release of `transformers`.
Right now you can experiment with it by installing `transformers` from the master branch.
You can also check the docs of DPR [here](https://huggingface.co/transformers/master/model_doc/dpr.html).
Moreover all the indexing features will also be available in the next release of `nlp`. |
https://github.com/huggingface/datasets/issues/439 | Issues: Adding a FAISS or Elastic Search index to a Dataset | @lhoestq I tried installing transformer from the master branch. Python imports for DPR again didnt' work. Anyways, Looking forward to trying it in the next release of nlp | It seems the DPRContextEncoder, DPRContextEncoderTokenizer cited[ in this documentation](https://huggingface.co/nlp/faiss_and_ea.html) is not implemented ? It didnot work with the standard nlp installation . Also, I couldn't find or use it with the latest nlp install from github in Colab. Is there any dependency on the latest PyArrow 1.0.0 ? Is it yet to be made generally available ? | 28 | Issues: Adding a FAISS or Elastic Search index to a Dataset
It seems the DPRContextEncoder, DPRContextEncoderTokenizer cited[ in this documentation](https://huggingface.co/nlp/faiss_and_ea.html) is not implemented ? It didnot work with the standard nlp installation . Also, I couldn't find or use it with the latest nlp install from github in Colab. Is there any dependency on the latest PyArrow 1.0.0 ? Is it yet to be made generally available ?
@lhoestq I tried installing transformer from the master branch. Python imports for DPR again didnt' work. Anyways, Looking forward to trying it in the next release of nlp |
https://github.com/huggingface/datasets/issues/438 | New Datasets: IWSLT15+, ITTB | Thanks Sam, we now have a very detailed tutorial and template on how to add a new dataset to the library. It typically take 1-2 hours to add one. Do you want to give it a try ?
The tutorial on writing a new dataset loading script is here: https://huggingface.co/nlp/add_dataset.html
And the part on how to share a new dataset is here: https://huggingface.co/nlp/share_dataset.html | **Links:**
[iwslt](https://pytorchnlp.readthedocs.io/en/latest/_modules/torchnlp/datasets/iwslt.html)
Don't know if that link is up to date.
[ittb](http://www.cfilt.iitb.ac.in/iitb_parallel/)
**Motivation**: replicate mbart finetuning results (table below)
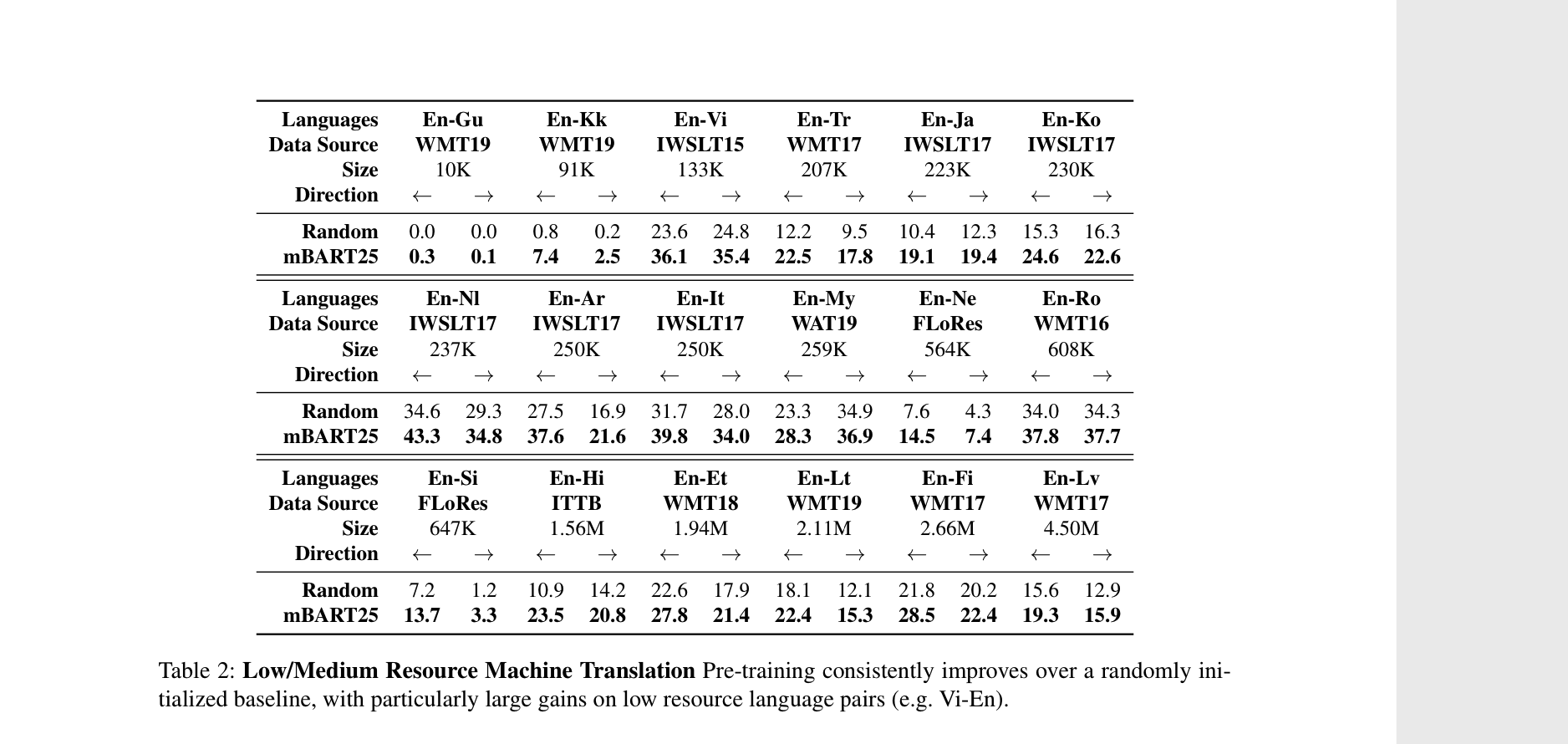
For future readers, we already have the following language pairs in the wmt namespaces:
```
wmt14: ['cs-en', 'de-en', 'fr-en', 'hi-en', 'ru-en']
wmt15: ['cs-en', 'de-en', 'fi-en', 'fr-en', 'ru-en']
wmt16: ['cs-en', 'de-en', 'fi-en', 'ro-en', 'ru-en', 'tr-en']
wmt17: ['cs-en', 'de-en', 'fi-en', 'lv-en', 'ru-en', 'tr-en', 'zh-en']
wmt18: ['cs-en', 'de-en', 'et-en', 'fi-en', 'kk-en', 'ru-en', 'tr-en', 'zh-en']
wmt19: ['cs-en', 'de-en', 'fi-en', 'gu-en', 'kk-en', 'lt-en', 'ru-en', 'zh-en', 'fr-de']
``` | 63 | New Datasets: IWSLT15+, ITTB
**Links:**
[iwslt](https://pytorchnlp.readthedocs.io/en/latest/_modules/torchnlp/datasets/iwslt.html)
Don't know if that link is up to date.
[ittb](http://www.cfilt.iitb.ac.in/iitb_parallel/)
**Motivation**: replicate mbart finetuning results (table below)
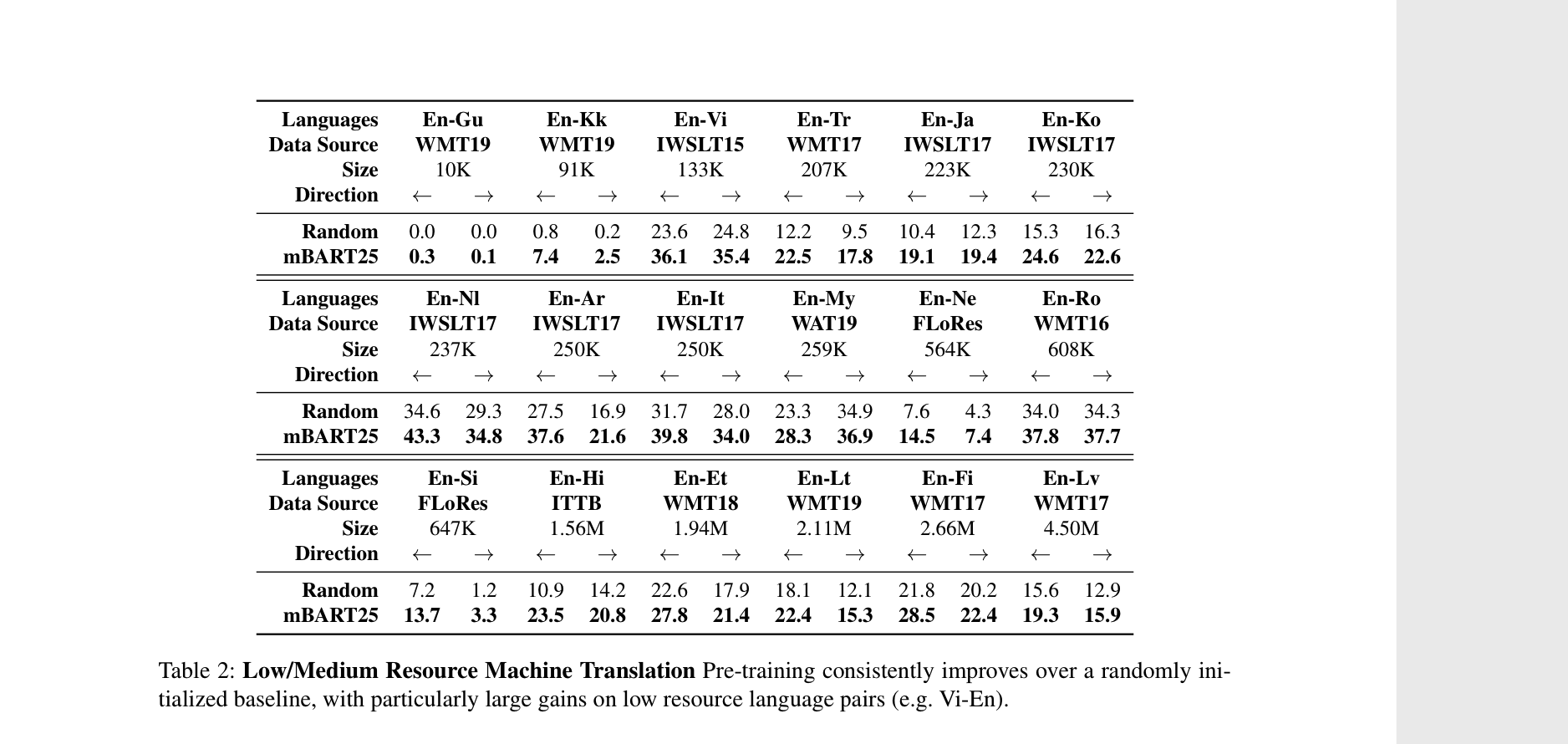
For future readers, we already have the following language pairs in the wmt namespaces:
```
wmt14: ['cs-en', 'de-en', 'fr-en', 'hi-en', 'ru-en']
wmt15: ['cs-en', 'de-en', 'fi-en', 'fr-en', 'ru-en']
wmt16: ['cs-en', 'de-en', 'fi-en', 'ro-en', 'ru-en', 'tr-en']
wmt17: ['cs-en', 'de-en', 'fi-en', 'lv-en', 'ru-en', 'tr-en', 'zh-en']
wmt18: ['cs-en', 'de-en', 'et-en', 'fi-en', 'kk-en', 'ru-en', 'tr-en', 'zh-en']
wmt19: ['cs-en', 'de-en', 'fi-en', 'gu-en', 'kk-en', 'lt-en', 'ru-en', 'zh-en', 'fr-de']
```
Thanks Sam, we now have a very detailed tutorial and template on how to add a new dataset to the library. It typically take 1-2 hours to add one. Do you want to give it a try ?
The tutorial on writing a new dataset loading script is here: https://huggingface.co/nlp/add_dataset.html
And the part on how to share a new dataset is here: https://huggingface.co/nlp/share_dataset.html |
https://github.com/huggingface/datasets/issues/438 | New Datasets: IWSLT15+, ITTB | Hi @sshleifer, I'm trying to add IWSLT using the link you provided but the download urls are not working. Only `[en, de]` pair is working. For others language pairs it throws a `404` error.
| **Links:**
[iwslt](https://pytorchnlp.readthedocs.io/en/latest/_modules/torchnlp/datasets/iwslt.html)
Don't know if that link is up to date.
[ittb](http://www.cfilt.iitb.ac.in/iitb_parallel/)
**Motivation**: replicate mbart finetuning results (table below)
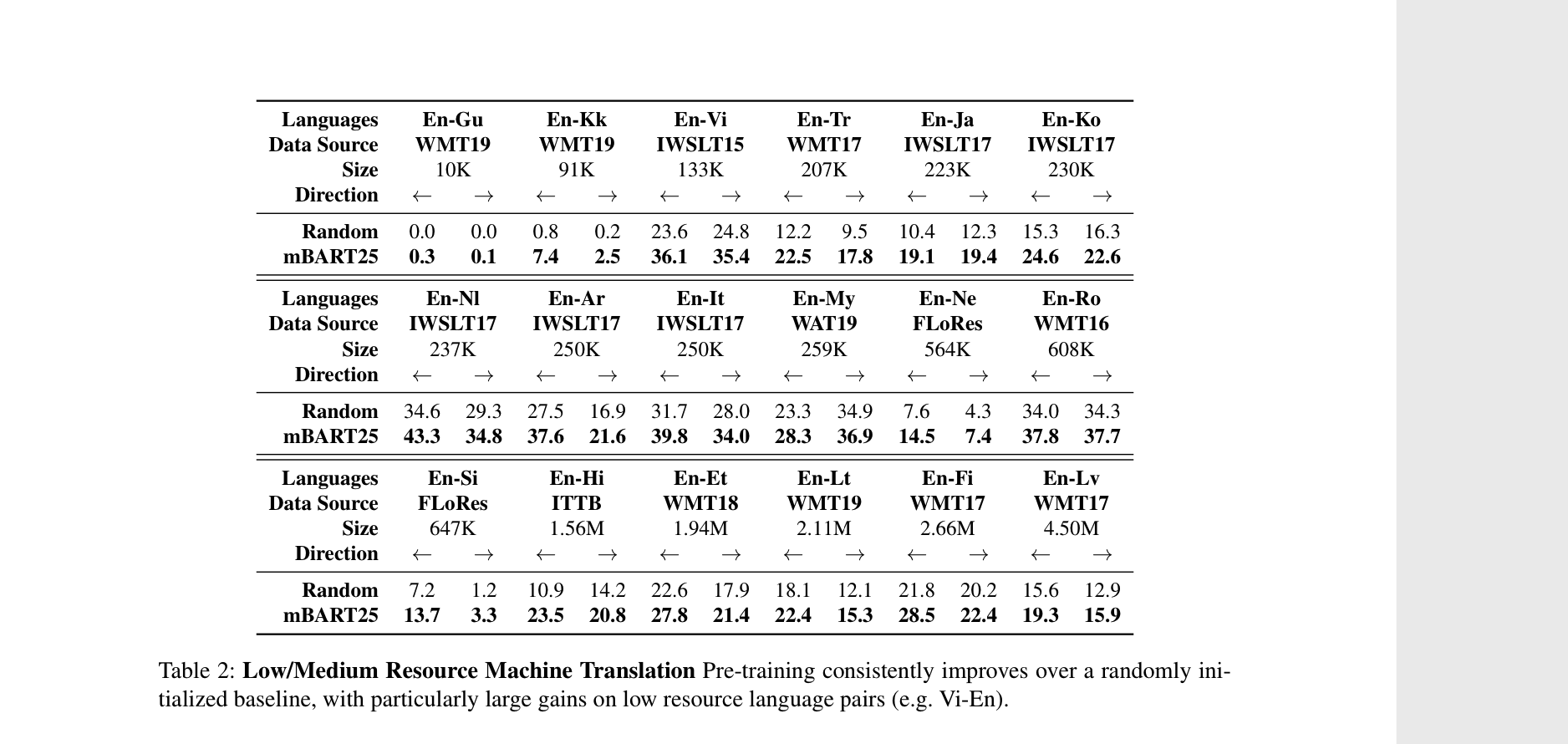
For future readers, we already have the following language pairs in the wmt namespaces:
```
wmt14: ['cs-en', 'de-en', 'fr-en', 'hi-en', 'ru-en']
wmt15: ['cs-en', 'de-en', 'fi-en', 'fr-en', 'ru-en']
wmt16: ['cs-en', 'de-en', 'fi-en', 'ro-en', 'ru-en', 'tr-en']
wmt17: ['cs-en', 'de-en', 'fi-en', 'lv-en', 'ru-en', 'tr-en', 'zh-en']
wmt18: ['cs-en', 'de-en', 'et-en', 'fi-en', 'kk-en', 'ru-en', 'tr-en', 'zh-en']
wmt19: ['cs-en', 'de-en', 'fi-en', 'gu-en', 'kk-en', 'lt-en', 'ru-en', 'zh-en', 'fr-de']
``` | 34 | New Datasets: IWSLT15+, ITTB
**Links:**
[iwslt](https://pytorchnlp.readthedocs.io/en/latest/_modules/torchnlp/datasets/iwslt.html)
Don't know if that link is up to date.
[ittb](http://www.cfilt.iitb.ac.in/iitb_parallel/)
**Motivation**: replicate mbart finetuning results (table below)
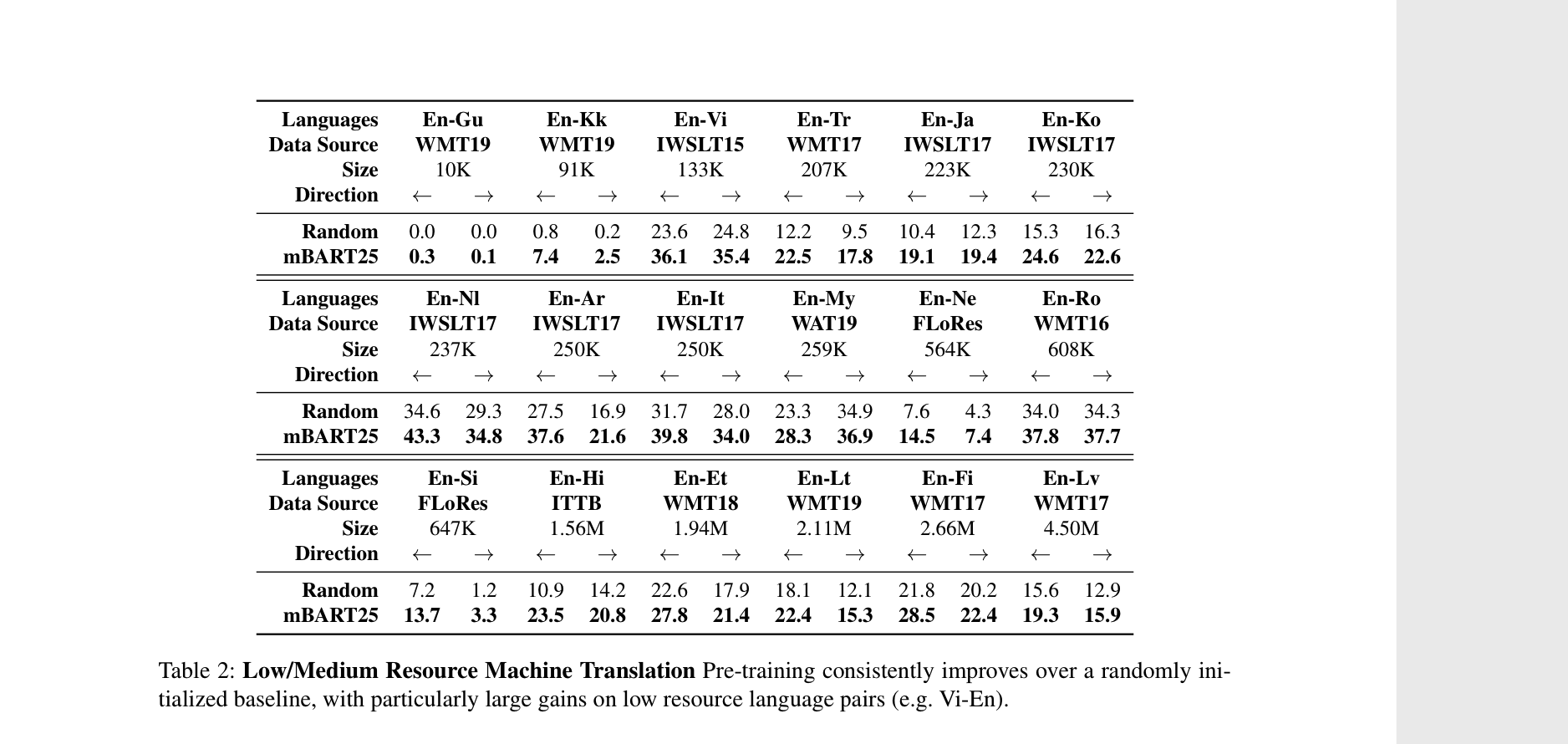
For future readers, we already have the following language pairs in the wmt namespaces:
```
wmt14: ['cs-en', 'de-en', 'fr-en', 'hi-en', 'ru-en']
wmt15: ['cs-en', 'de-en', 'fi-en', 'fr-en', 'ru-en']
wmt16: ['cs-en', 'de-en', 'fi-en', 'ro-en', 'ru-en', 'tr-en']
wmt17: ['cs-en', 'de-en', 'fi-en', 'lv-en', 'ru-en', 'tr-en', 'zh-en']
wmt18: ['cs-en', 'de-en', 'et-en', 'fi-en', 'kk-en', 'ru-en', 'tr-en', 'zh-en']
wmt19: ['cs-en', 'de-en', 'fi-en', 'gu-en', 'kk-en', 'lt-en', 'ru-en', 'zh-en', 'fr-de']
```
Hi @sshleifer, I'm trying to add IWSLT using the link you provided but the download urls are not working. Only `[en, de]` pair is working. For others language pairs it throws a `404` error.
|
https://github.com/huggingface/datasets/issues/436 | Google Colab - load_dataset - PyArrow exception | +1! this is the reason our tests are failing at [TextAttack](https://github.com/QData/TextAttack)
(Though it's worth noting if we fixed the version number of pyarrow to 0.16.0 that would fix our problem too. But in this case we'll just wait for you all to update) | With latest PyArrow 1.0.0 installed, I get the following exception . Restarting colab has the same issue
ImportWarning: To use `nlp`, the module `pyarrow>=0.16.0` is required, and the current version of `pyarrow` doesn't match this condition. If you are running this in a Google Colab, you should probably just restart the runtime to use the right version of `pyarrow`.
The error goes only when I install version 0.16.0
i.e. !pip install pyarrow==0.16.0 | 43 | Google Colab - load_dataset - PyArrow exception
With latest PyArrow 1.0.0 installed, I get the following exception . Restarting colab has the same issue
ImportWarning: To use `nlp`, the module `pyarrow>=0.16.0` is required, and the current version of `pyarrow` doesn't match this condition. If you are running this in a Google Colab, you should probably just restart the runtime to use the right version of `pyarrow`.
The error goes only when I install version 0.16.0
i.e. !pip install pyarrow==0.16.0
+1! this is the reason our tests are failing at [TextAttack](https://github.com/QData/TextAttack)
(Though it's worth noting if we fixed the version number of pyarrow to 0.16.0 that would fix our problem too. But in this case we'll just wait for you all to update) |
https://github.com/huggingface/datasets/issues/436 | Google Colab - load_dataset - PyArrow exception | Came to raise this issue, great to see other already have and it's being fixed so soon!
As an aside, since no one wrote this already, it seems like the version check only looks at the second part of the version number making sure it is >16, but pyarrow newest version is 1.0.0 so the second past is 0! | With latest PyArrow 1.0.0 installed, I get the following exception . Restarting colab has the same issue
ImportWarning: To use `nlp`, the module `pyarrow>=0.16.0` is required, and the current version of `pyarrow` doesn't match this condition. If you are running this in a Google Colab, you should probably just restart the runtime to use the right version of `pyarrow`.
The error goes only when I install version 0.16.0
i.e. !pip install pyarrow==0.16.0 | 59 | Google Colab - load_dataset - PyArrow exception
With latest PyArrow 1.0.0 installed, I get the following exception . Restarting colab has the same issue
ImportWarning: To use `nlp`, the module `pyarrow>=0.16.0` is required, and the current version of `pyarrow` doesn't match this condition. If you are running this in a Google Colab, you should probably just restart the runtime to use the right version of `pyarrow`.
The error goes only when I install version 0.16.0
i.e. !pip install pyarrow==0.16.0
Came to raise this issue, great to see other already have and it's being fixed so soon!
As an aside, since no one wrote this already, it seems like the version check only looks at the second part of the version number making sure it is >16, but pyarrow newest version is 1.0.0 so the second past is 0! |
https://github.com/huggingface/datasets/issues/436 | Google Colab - load_dataset - PyArrow exception | > Indeed, weβll make a new PyPi release next week to solve this. Cc @lhoestq
Yes definitely | With latest PyArrow 1.0.0 installed, I get the following exception . Restarting colab has the same issue
ImportWarning: To use `nlp`, the module `pyarrow>=0.16.0` is required, and the current version of `pyarrow` doesn't match this condition. If you are running this in a Google Colab, you should probably just restart the runtime to use the right version of `pyarrow`.
The error goes only when I install version 0.16.0
i.e. !pip install pyarrow==0.16.0 | 17 | Google Colab - load_dataset - PyArrow exception
With latest PyArrow 1.0.0 installed, I get the following exception . Restarting colab has the same issue
ImportWarning: To use `nlp`, the module `pyarrow>=0.16.0` is required, and the current version of `pyarrow` doesn't match this condition. If you are running this in a Google Colab, you should probably just restart the runtime to use the right version of `pyarrow`.
The error goes only when I install version 0.16.0
i.e. !pip install pyarrow==0.16.0
> Indeed, weβll make a new PyPi release next week to solve this. Cc @lhoestq
Yes definitely |
https://github.com/huggingface/datasets/issues/435 | ImportWarning for pyarrow 1.0.0 | This was fixed in #434
We'll do a release later this week to include this fix.
Thanks for reporting | The following PR raised ImportWarning at `pyarrow ==1.0.0` https://github.com/huggingface/nlp/pull/265/files | 19 | ImportWarning for pyarrow 1.0.0
The following PR raised ImportWarning at `pyarrow ==1.0.0` https://github.com/huggingface/nlp/pull/265/files
This was fixed in #434
We'll do a release later this week to include this fix.
Thanks for reporting |
https://github.com/huggingface/datasets/issues/435 | ImportWarning for pyarrow 1.0.0 | I dont know if the fix was made but the problem is still present :
Instaled with pip : NLP 0.3.0 // pyarrow 1.0.0
OS : archlinux with kernel zen 5.8.5 | The following PR raised ImportWarning at `pyarrow ==1.0.0` https://github.com/huggingface/nlp/pull/265/files | 31 | ImportWarning for pyarrow 1.0.0
The following PR raised ImportWarning at `pyarrow ==1.0.0` https://github.com/huggingface/nlp/pull/265/files
I dont know if the fix was made but the problem is still present :
Instaled with pip : NLP 0.3.0 // pyarrow 1.0.0
OS : archlinux with kernel zen 5.8.5 |
https://github.com/huggingface/datasets/issues/433 | How to reuse functionality of a (generic) dataset? | Hi @ArneBinder, we have a few "generic" datasets which are intended to load data files with a predefined format:
- csv: https://github.com/huggingface/nlp/tree/master/datasets/csv
- json: https://github.com/huggingface/nlp/tree/master/datasets/json
- text: https://github.com/huggingface/nlp/tree/master/datasets/text
You can find more details about this way to load datasets here in the documentation: https://huggingface.co/nlp/loading_datasets.html#from-local-files
Maybe your brat loading script could be shared in a similar fashion? | I have written a generic dataset for corpora created with the Brat annotation tool ([specification](https://brat.nlplab.org/standoff.html), [dataset code](https://github.com/ArneBinder/nlp/blob/brat/datasets/brat/brat.py)). Now I wonder how to use that to create specific dataset instances. What's the recommended way to reuse formats and loading functionality for datasets with a common format?
In my case, it took a bit of time to create the Brat dataset and I think others would appreciate to not have to think about that again. Also, I assume there are other formats (e.g. conll) that are widely used, so having this would really ease dataset onboarding and adoption of the library. | 56 | How to reuse functionality of a (generic) dataset?
I have written a generic dataset for corpora created with the Brat annotation tool ([specification](https://brat.nlplab.org/standoff.html), [dataset code](https://github.com/ArneBinder/nlp/blob/brat/datasets/brat/brat.py)). Now I wonder how to use that to create specific dataset instances. What's the recommended way to reuse formats and loading functionality for datasets with a common format?
In my case, it took a bit of time to create the Brat dataset and I think others would appreciate to not have to think about that again. Also, I assume there are other formats (e.g. conll) that are widely used, so having this would really ease dataset onboarding and adoption of the library.
Hi @ArneBinder, we have a few "generic" datasets which are intended to load data files with a predefined format:
- csv: https://github.com/huggingface/nlp/tree/master/datasets/csv
- json: https://github.com/huggingface/nlp/tree/master/datasets/json
- text: https://github.com/huggingface/nlp/tree/master/datasets/text
You can find more details about this way to load datasets here in the documentation: https://huggingface.co/nlp/loading_datasets.html#from-local-files
Maybe your brat loading script could be shared in a similar fashion? |
https://github.com/huggingface/datasets/issues/433 | How to reuse functionality of a (generic) dataset? | > Maybe your brat loading script could be shared in a similar fashion?
@thomwolf that was also my first idea and I think I will tackle that in the next days. I separated the code and created a real abstract class `AbstractBrat` to allow to inherit from that (I've just seen that the dataset_loader loads the first non abstract class), now `Brat` is very similar in its functionality to https://github.com/huggingface/nlp/tree/master/datasets/text but inherits from `AbstractBrat`.
However, it is still not clear to me how to add a specific dataset (as explained in https://huggingface.co/nlp/add_dataset.html) to your repo that uses this format/abstract class, i.e. re-using the `features` entry of the `DatasetInfo` object and `_generate_examples()`. Again, by doing so, the only remaining entries/functions to define would be `_DESCRIPTION`, `_CITATION`, `homepage` and `_URL` (which is all copy-paste stuff) and `_split_generators()`.
In a lack of better ideas, I tried sth like below, but of course it does not work outside `nlp` (`AbstractBrat` is currently defined in [datasets/brat.py](https://github.com/ArneBinder/nlp/blob/5e81fb8710546ee7be3353a7f02a3045e9a8351e/datasets/brat/brat.py)):
```python
from __future__ import absolute_import, division, print_function
import os
import nlp
from datasets.brat.brat import AbstractBrat
_CITATION = """
@inproceedings{lauscher2018b,
title = {An argument-annotated corpus of scientific publications},
booktitle = {Proceedings of the 5th Workshop on Mining Argumentation},
publisher = {Association for Computational Linguistics},
author = {Lauscher, Anne and Glava\v{s}, Goran and Ponzetto, Simone Paolo},
address = {Brussels, Belgium},
year = {2018},
pages = {40β46}
}
"""
_DESCRIPTION = """\
This dataset is an extension of the Dr. Inventor corpus (Fisas et al., 2015, 2016) with an annotation layer containing
fine-grained argumentative components and relations. It is the first argument-annotated corpus of scientific
publications (in English), which allows for joint analyses of argumentation and other rhetorical dimensions of
scientific writing.
"""
_URL = "http://data.dws.informatik.uni-mannheim.de/sci-arg/compiled_corpus.zip"
class Sciarg(AbstractBrat):
VERSION = nlp.Version("1.0.0")
def _info(self):
brat_features = super()._info().features
return nlp.DatasetInfo(
# This is the description that will appear on the datasets page.
description=_DESCRIPTION,
# nlp.features.FeatureConnectors
features=brat_features,
# If there's a common (input, target) tuple from the features,
# specify them here. They'll be used if as_supervised=True in
# builder.as_dataset.
#supervised_keys=None,
# Homepage of the dataset for documentation
homepage="https://github.com/anlausch/ArguminSci",
citation=_CITATION,
)
def _split_generators(self, dl_manager):
"""Returns SplitGenerators."""
# TODO: Downloads the data and defines the splits
# dl_manager is a nlp.download.DownloadManager that can be used to
# download and extract URLs
dl_dir = dl_manager.download_and_extract(_URL)
data_dir = os.path.join(dl_dir, "compiled_corpus")
print(f'data_dir: {data_dir}')
return [
nlp.SplitGenerator(
name=nlp.Split.TRAIN,
# These kwargs will be passed to _generate_examples
gen_kwargs={
"directory": data_dir,
},
),
]
```
Nevertheless, many thanks for tackling the dataset accessibility problem with this great library! | I have written a generic dataset for corpora created with the Brat annotation tool ([specification](https://brat.nlplab.org/standoff.html), [dataset code](https://github.com/ArneBinder/nlp/blob/brat/datasets/brat/brat.py)). Now I wonder how to use that to create specific dataset instances. What's the recommended way to reuse formats and loading functionality for datasets with a common format?
In my case, it took a bit of time to create the Brat dataset and I think others would appreciate to not have to think about that again. Also, I assume there are other formats (e.g. conll) that are widely used, so having this would really ease dataset onboarding and adoption of the library. | 416 | How to reuse functionality of a (generic) dataset?
I have written a generic dataset for corpora created with the Brat annotation tool ([specification](https://brat.nlplab.org/standoff.html), [dataset code](https://github.com/ArneBinder/nlp/blob/brat/datasets/brat/brat.py)). Now I wonder how to use that to create specific dataset instances. What's the recommended way to reuse formats and loading functionality for datasets with a common format?
In my case, it took a bit of time to create the Brat dataset and I think others would appreciate to not have to think about that again. Also, I assume there are other formats (e.g. conll) that are widely used, so having this would really ease dataset onboarding and adoption of the library.
> Maybe your brat loading script could be shared in a similar fashion?
@thomwolf that was also my first idea and I think I will tackle that in the next days. I separated the code and created a real abstract class `AbstractBrat` to allow to inherit from that (I've just seen that the dataset_loader loads the first non abstract class), now `Brat` is very similar in its functionality to https://github.com/huggingface/nlp/tree/master/datasets/text but inherits from `AbstractBrat`.
However, it is still not clear to me how to add a specific dataset (as explained in https://huggingface.co/nlp/add_dataset.html) to your repo that uses this format/abstract class, i.e. re-using the `features` entry of the `DatasetInfo` object and `_generate_examples()`. Again, by doing so, the only remaining entries/functions to define would be `_DESCRIPTION`, `_CITATION`, `homepage` and `_URL` (which is all copy-paste stuff) and `_split_generators()`.
In a lack of better ideas, I tried sth like below, but of course it does not work outside `nlp` (`AbstractBrat` is currently defined in [datasets/brat.py](https://github.com/ArneBinder/nlp/blob/5e81fb8710546ee7be3353a7f02a3045e9a8351e/datasets/brat/brat.py)):
```python
from __future__ import absolute_import, division, print_function
import os
import nlp
from datasets.brat.brat import AbstractBrat
_CITATION = """
@inproceedings{lauscher2018b,
title = {An argument-annotated corpus of scientific publications},
booktitle = {Proceedings of the 5th Workshop on Mining Argumentation},
publisher = {Association for Computational Linguistics},
author = {Lauscher, Anne and Glava\v{s}, Goran and Ponzetto, Simone Paolo},
address = {Brussels, Belgium},
year = {2018},
pages = {40β46}
}
"""
_DESCRIPTION = """\
This dataset is an extension of the Dr. Inventor corpus (Fisas et al., 2015, 2016) with an annotation layer containing
fine-grained argumentative components and relations. It is the first argument-annotated corpus of scientific
publications (in English), which allows for joint analyses of argumentation and other rhetorical dimensions of
scientific writing.
"""
_URL = "http://data.dws.informatik.uni-mannheim.de/sci-arg/compiled_corpus.zip"
class Sciarg(AbstractBrat):
VERSION = nlp.Version("1.0.0")
def _info(self):
brat_features = super()._info().features
return nlp.DatasetInfo(
# This is the description that will appear on the datasets page.
description=_DESCRIPTION,
# nlp.features.FeatureConnectors
features=brat_features,
# If there's a common (input, target) tuple from the features,
# specify them here. They'll be used if as_supervised=True in
# builder.as_dataset.
#supervised_keys=None,
# Homepage of the dataset for documentation
homepage="https://github.com/anlausch/ArguminSci",
citation=_CITATION,
)
def _split_generators(self, dl_manager):
"""Returns SplitGenerators."""
# TODO: Downloads the data and defines the splits
# dl_manager is a nlp.download.DownloadManager that can be used to
# download and extract URLs
dl_dir = dl_manager.download_and_extract(_URL)
data_dir = os.path.join(dl_dir, "compiled_corpus")
print(f'data_dir: {data_dir}')
return [
nlp.SplitGenerator(
name=nlp.Split.TRAIN,
# These kwargs will be passed to _generate_examples
gen_kwargs={
"directory": data_dir,
},
),
]
```
Nevertheless, many thanks for tackling the dataset accessibility problem with this great library! |
https://github.com/huggingface/datasets/issues/433 | How to reuse functionality of a (generic) dataset? | Hi! You can either copy&paste the builder script and import the builder from there or use `datasets.load_dataset_builder` inside the script and call the methods of the returned builder object. | I have written a generic dataset for corpora created with the Brat annotation tool ([specification](https://brat.nlplab.org/standoff.html), [dataset code](https://github.com/ArneBinder/nlp/blob/brat/datasets/brat/brat.py)). Now I wonder how to use that to create specific dataset instances. What's the recommended way to reuse formats and loading functionality for datasets with a common format?
In my case, it took a bit of time to create the Brat dataset and I think others would appreciate to not have to think about that again. Also, I assume there are other formats (e.g. conll) that are widely used, so having this would really ease dataset onboarding and adoption of the library. | 29 | How to reuse functionality of a (generic) dataset?
I have written a generic dataset for corpora created with the Brat annotation tool ([specification](https://brat.nlplab.org/standoff.html), [dataset code](https://github.com/ArneBinder/nlp/blob/brat/datasets/brat/brat.py)). Now I wonder how to use that to create specific dataset instances. What's the recommended way to reuse formats and loading functionality for datasets with a common format?
In my case, it took a bit of time to create the Brat dataset and I think others would appreciate to not have to think about that again. Also, I assume there are other formats (e.g. conll) that are widely used, so having this would really ease dataset onboarding and adoption of the library.
Hi! You can either copy&paste the builder script and import the builder from there or use `datasets.load_dataset_builder` inside the script and call the methods of the returned builder object. |
https://github.com/huggingface/datasets/issues/426 | [FEATURE REQUEST] Multiprocessing with for dataset.map, dataset.filter | Yes, that would be nice. We could take a look at what tensorflow `tf.data` does under the hood for instance. | It would be nice to be able to speed up `dataset.map` or `dataset.filter`. Perhaps this is as easy as sharding the dataset sending each shard to a process/thread/dask pool and using the new `nlp.concatenate_dataset()` function to join them all together? | 20 | [FEATURE REQUEST] Multiprocessing with for dataset.map, dataset.filter
It would be nice to be able to speed up `dataset.map` or `dataset.filter`. Perhaps this is as easy as sharding the dataset sending each shard to a process/thread/dask pool and using the new `nlp.concatenate_dataset()` function to join them all together?
Yes, that would be nice. We could take a look at what tensorflow `tf.data` does under the hood for instance. |
https://github.com/huggingface/datasets/issues/426 | [FEATURE REQUEST] Multiprocessing with for dataset.map, dataset.filter | So `tf.data.Dataset.map()` returns a `ParallelMapDataset` if `num_parallel_calls is not None` [link](https://github.com/tensorflow/tensorflow/blob/2b96f3662bd776e277f86997659e61046b56c315/tensorflow/python/data/ops/dataset_ops.py#L1623).
There, `num_parallel_calls` is turned into a tensor and and fed to `gen_dataset_ops.parallel_map_dataset` where it looks like tensorflow takes over.
We could start with something simple like a thread or process pool that `imap`s over some shards.
| It would be nice to be able to speed up `dataset.map` or `dataset.filter`. Perhaps this is as easy as sharding the dataset sending each shard to a process/thread/dask pool and using the new `nlp.concatenate_dataset()` function to join them all together? | 47 | [FEATURE REQUEST] Multiprocessing with for dataset.map, dataset.filter
It would be nice to be able to speed up `dataset.map` or `dataset.filter`. Perhaps this is as easy as sharding the dataset sending each shard to a process/thread/dask pool and using the new `nlp.concatenate_dataset()` function to join them all together?
So `tf.data.Dataset.map()` returns a `ParallelMapDataset` if `num_parallel_calls is not None` [link](https://github.com/tensorflow/tensorflow/blob/2b96f3662bd776e277f86997659e61046b56c315/tensorflow/python/data/ops/dataset_ops.py#L1623).
There, `num_parallel_calls` is turned into a tensor and and fed to `gen_dataset_ops.parallel_map_dataset` where it looks like tensorflow takes over.
We could start with something simple like a thread or process pool that `imap`s over some shards.
|
https://github.com/huggingface/datasets/issues/426 | [FEATURE REQUEST] Multiprocessing with for dataset.map, dataset.filter | Multiprocessing was added in #552 . You can set the number of processes with `.map(..., num_proc=...)`. It also works for `filter`
Closing this one, but feel free to reo-open if you have other questions | It would be nice to be able to speed up `dataset.map` or `dataset.filter`. Perhaps this is as easy as sharding the dataset sending each shard to a process/thread/dask pool and using the new `nlp.concatenate_dataset()` function to join them all together? | 34 | [FEATURE REQUEST] Multiprocessing with for dataset.map, dataset.filter
It would be nice to be able to speed up `dataset.map` or `dataset.filter`. Perhaps this is as easy as sharding the dataset sending each shard to a process/thread/dask pool and using the new `nlp.concatenate_dataset()` function to join them all together?
Multiprocessing was added in #552 . You can set the number of processes with `.map(..., num_proc=...)`. It also works for `filter`
Closing this one, but feel free to reo-open if you have other questions |
https://github.com/huggingface/datasets/issues/426 | [FEATURE REQUEST] Multiprocessing with for dataset.map, dataset.filter | @lhoestq Great feature implemented! Do you have plans to add it to official tutorials [Processing data in a Dataset](https://huggingface.co/docs/datasets/processing.html?highlight=save#augmenting-the-dataset)? It took me sometime to find this parallel processing api. | It would be nice to be able to speed up `dataset.map` or `dataset.filter`. Perhaps this is as easy as sharding the dataset sending each shard to a process/thread/dask pool and using the new `nlp.concatenate_dataset()` function to join them all together? | 29 | [FEATURE REQUEST] Multiprocessing with for dataset.map, dataset.filter
It would be nice to be able to speed up `dataset.map` or `dataset.filter`. Perhaps this is as easy as sharding the dataset sending each shard to a process/thread/dask pool and using the new `nlp.concatenate_dataset()` function to join them all together?
@lhoestq Great feature implemented! Do you have plans to add it to official tutorials [Processing data in a Dataset](https://huggingface.co/docs/datasets/processing.html?highlight=save#augmenting-the-dataset)? It took me sometime to find this parallel processing api. |
https://github.com/huggingface/datasets/issues/425 | Correct data structure for PAN-X task in XTREME dataset? | Hi @lhoestq
I made the proposed changes to the `xtreme.py` script. I noticed that I also need to change the schema in the `dataset_infos.json` file. More specifically the `"features"` part of the PAN-X.LANG dataset:
```json
"features":{
"word":{
"dtype":"string",
"id":null,
"_type":"Value"
},
"ner_tag":{
"dtype":"string",
"id":null,
"_type":"Value"
},
"lang":{
"dtype":"string",
"id":null,
"_type":"Value"
}
}
```
To fit the code above the fields `"word"`, `"ner_tag"`, and `"lang"` would become `"words"`, `ner_tags"` and `"langs"`. In addition the `dtype` should be changed from `"string"` to `"list"`.
I made this changes but when trying to test this locally with `dataset = load_dataset("xtreme", "PAN-X.en", data_dir='./data')` I face the issue that the `dataset_info.json` file is always overwritten by a downloaded version with the old settings, which then throws an error because the schema does not match. This makes it hard to test the changes locally. Do you have any suggestions on how to deal with that?
| Hi π€ team!
## Description of the problem
Thanks to the fix from #416 I am now able to load the NER task in the XTREME dataset as follows:
```python
from nlp import load_dataset
# AmazonPhotos.zip is located in data/
dataset = load_dataset("xtreme", "PAN-X.en", data_dir='./data')
dataset_train = dataset['train']
```
However, I am not sure that `load_dataset()` is returning the correct data structure for NER.
Currently, every row in `dataset_train` is of the form
```python
{'word': str, 'ner_tag': str, 'lang': str}
```
but I think we actually want something like
```python
{'words': List[str], 'ner_tags': List[str], 'langs': List[str]}
```
so that each row corresponds to a _sequence_ of words associated with each example. With the current data structure I do not think it is possible to transform `dataset_train` into a form suitable for training because we do not know the boundaries between examples.
Indeed, [this line](https://github.com/google-research/xtreme/blob/522434d1aece34131d997a97ce7e9242a51a688a/third_party/utils_tag.py#L58) in the XTREME repo, processes the texts as lists of sentences, tags, and languages.
## Proposed solution
Replace
```python
with open(filepath) as f:
data = csv.reader(f, delimiter="\t", quoting=csv.QUOTE_NONE)
for id_, row in enumerate(data):
if row:
lang, word = row[0].split(":")[0], row[0].split(":")[1]
tag = row[1]
yield id_, {"word": word, "ner_tag": tag, "lang": lang}
```
from [these lines](https://github.com/huggingface/nlp/blob/ce7d3a1d630b78fe27188d1706f3ea980e8eec43/datasets/xtreme/xtreme.py#L881-L887) of the `_generate_examples()` function with something like
```python
guid_index = 1
with open(filepath, encoding="utf-8") as f:
words = []
ner_tags = []
langs = []
for line in f:
if line.startswith("-DOCSTART-") or line == "" or line == "\n":
if words:
yield guid_index, {"words": words, "ner_tags": ner_tags, "langs": langs}
guid_index += 1
words = []
ner_tags = []
else:
# pan-x data is tab separated
splits = line.split("\t")
# strip out en: prefix
langs.append(splits[0][:2])
words.append(splits[0][3:])
if len(splits) > 1:
labels.append(splits[-1].replace("\n", ""))
else:
# examples have no label in test set
labels.append("O")
```
If you agree, me or @lvwerra would be happy to implement this and create a PR. | 148 | Correct data structure for PAN-X task in XTREME dataset?
Hi π€ team!
## Description of the problem
Thanks to the fix from #416 I am now able to load the NER task in the XTREME dataset as follows:
```python
from nlp import load_dataset
# AmazonPhotos.zip is located in data/
dataset = load_dataset("xtreme", "PAN-X.en", data_dir='./data')
dataset_train = dataset['train']
```
However, I am not sure that `load_dataset()` is returning the correct data structure for NER.
Currently, every row in `dataset_train` is of the form
```python
{'word': str, 'ner_tag': str, 'lang': str}
```
but I think we actually want something like
```python
{'words': List[str], 'ner_tags': List[str], 'langs': List[str]}
```
so that each row corresponds to a _sequence_ of words associated with each example. With the current data structure I do not think it is possible to transform `dataset_train` into a form suitable for training because we do not know the boundaries between examples.
Indeed, [this line](https://github.com/google-research/xtreme/blob/522434d1aece34131d997a97ce7e9242a51a688a/third_party/utils_tag.py#L58) in the XTREME repo, processes the texts as lists of sentences, tags, and languages.
## Proposed solution
Replace
```python
with open(filepath) as f:
data = csv.reader(f, delimiter="\t", quoting=csv.QUOTE_NONE)
for id_, row in enumerate(data):
if row:
lang, word = row[0].split(":")[0], row[0].split(":")[1]
tag = row[1]
yield id_, {"word": word, "ner_tag": tag, "lang": lang}
```
from [these lines](https://github.com/huggingface/nlp/blob/ce7d3a1d630b78fe27188d1706f3ea980e8eec43/datasets/xtreme/xtreme.py#L881-L887) of the `_generate_examples()` function with something like
```python
guid_index = 1
with open(filepath, encoding="utf-8") as f:
words = []
ner_tags = []
langs = []
for line in f:
if line.startswith("-DOCSTART-") or line == "" or line == "\n":
if words:
yield guid_index, {"words": words, "ner_tags": ner_tags, "langs": langs}
guid_index += 1
words = []
ner_tags = []
else:
# pan-x data is tab separated
splits = line.split("\t")
# strip out en: prefix
langs.append(splits[0][:2])
words.append(splits[0][3:])
if len(splits) > 1:
labels.append(splits[-1].replace("\n", ""))
else:
# examples have no label in test set
labels.append("O")
```
If you agree, me or @lvwerra would be happy to implement this and create a PR.
Hi @lhoestq
I made the proposed changes to the `xtreme.py` script. I noticed that I also need to change the schema in the `dataset_infos.json` file. More specifically the `"features"` part of the PAN-X.LANG dataset:
```json
"features":{
"word":{
"dtype":"string",
"id":null,
"_type":"Value"
},
"ner_tag":{
"dtype":"string",
"id":null,
"_type":"Value"
},
"lang":{
"dtype":"string",
"id":null,
"_type":"Value"
}
}
```
To fit the code above the fields `"word"`, `"ner_tag"`, and `"lang"` would become `"words"`, `ner_tags"` and `"langs"`. In addition the `dtype` should be changed from `"string"` to `"list"`.
I made this changes but when trying to test this locally with `dataset = load_dataset("xtreme", "PAN-X.en", data_dir='./data')` I face the issue that the `dataset_info.json` file is always overwritten by a downloaded version with the old settings, which then throws an error because the schema does not match. This makes it hard to test the changes locally. Do you have any suggestions on how to deal with that?
|
https://github.com/huggingface/datasets/issues/425 | Correct data structure for PAN-X task in XTREME dataset? | Hi !
You have to point to your local script.
First clone the repo and then:
```python
dataset = load_dataset("./datasets/xtreme", "PAN-X.en")
```
The "xtreme" directory contains "xtreme.py".
You also have to change the features definition in the `_info` method. You could use:
```python
features = nlp.Features({
"words": [nlp.Value("string")],
"ner_tags": [nlp.Value("string")],
"langs": [nlp.Value("string")],
})
```
Hope this helps !
Let me know if you have other questions. | Hi π€ team!
## Description of the problem
Thanks to the fix from #416 I am now able to load the NER task in the XTREME dataset as follows:
```python
from nlp import load_dataset
# AmazonPhotos.zip is located in data/
dataset = load_dataset("xtreme", "PAN-X.en", data_dir='./data')
dataset_train = dataset['train']
```
However, I am not sure that `load_dataset()` is returning the correct data structure for NER.
Currently, every row in `dataset_train` is of the form
```python
{'word': str, 'ner_tag': str, 'lang': str}
```
but I think we actually want something like
```python
{'words': List[str], 'ner_tags': List[str], 'langs': List[str]}
```
so that each row corresponds to a _sequence_ of words associated with each example. With the current data structure I do not think it is possible to transform `dataset_train` into a form suitable for training because we do not know the boundaries between examples.
Indeed, [this line](https://github.com/google-research/xtreme/blob/522434d1aece34131d997a97ce7e9242a51a688a/third_party/utils_tag.py#L58) in the XTREME repo, processes the texts as lists of sentences, tags, and languages.
## Proposed solution
Replace
```python
with open(filepath) as f:
data = csv.reader(f, delimiter="\t", quoting=csv.QUOTE_NONE)
for id_, row in enumerate(data):
if row:
lang, word = row[0].split(":")[0], row[0].split(":")[1]
tag = row[1]
yield id_, {"word": word, "ner_tag": tag, "lang": lang}
```
from [these lines](https://github.com/huggingface/nlp/blob/ce7d3a1d630b78fe27188d1706f3ea980e8eec43/datasets/xtreme/xtreme.py#L881-L887) of the `_generate_examples()` function with something like
```python
guid_index = 1
with open(filepath, encoding="utf-8") as f:
words = []
ner_tags = []
langs = []
for line in f:
if line.startswith("-DOCSTART-") or line == "" or line == "\n":
if words:
yield guid_index, {"words": words, "ner_tags": ner_tags, "langs": langs}
guid_index += 1
words = []
ner_tags = []
else:
# pan-x data is tab separated
splits = line.split("\t")
# strip out en: prefix
langs.append(splits[0][:2])
words.append(splits[0][3:])
if len(splits) > 1:
labels.append(splits[-1].replace("\n", ""))
else:
# examples have no label in test set
labels.append("O")
```
If you agree, me or @lvwerra would be happy to implement this and create a PR. | 66 | Correct data structure for PAN-X task in XTREME dataset?
Hi π€ team!
## Description of the problem
Thanks to the fix from #416 I am now able to load the NER task in the XTREME dataset as follows:
```python
from nlp import load_dataset
# AmazonPhotos.zip is located in data/
dataset = load_dataset("xtreme", "PAN-X.en", data_dir='./data')
dataset_train = dataset['train']
```
However, I am not sure that `load_dataset()` is returning the correct data structure for NER.
Currently, every row in `dataset_train` is of the form
```python
{'word': str, 'ner_tag': str, 'lang': str}
```
but I think we actually want something like
```python
{'words': List[str], 'ner_tags': List[str], 'langs': List[str]}
```
so that each row corresponds to a _sequence_ of words associated with each example. With the current data structure I do not think it is possible to transform `dataset_train` into a form suitable for training because we do not know the boundaries between examples.
Indeed, [this line](https://github.com/google-research/xtreme/blob/522434d1aece34131d997a97ce7e9242a51a688a/third_party/utils_tag.py#L58) in the XTREME repo, processes the texts as lists of sentences, tags, and languages.
## Proposed solution
Replace
```python
with open(filepath) as f:
data = csv.reader(f, delimiter="\t", quoting=csv.QUOTE_NONE)
for id_, row in enumerate(data):
if row:
lang, word = row[0].split(":")[0], row[0].split(":")[1]
tag = row[1]
yield id_, {"word": word, "ner_tag": tag, "lang": lang}
```
from [these lines](https://github.com/huggingface/nlp/blob/ce7d3a1d630b78fe27188d1706f3ea980e8eec43/datasets/xtreme/xtreme.py#L881-L887) of the `_generate_examples()` function with something like
```python
guid_index = 1
with open(filepath, encoding="utf-8") as f:
words = []
ner_tags = []
langs = []
for line in f:
if line.startswith("-DOCSTART-") or line == "" or line == "\n":
if words:
yield guid_index, {"words": words, "ner_tags": ner_tags, "langs": langs}
guid_index += 1
words = []
ner_tags = []
else:
# pan-x data is tab separated
splits = line.split("\t")
# strip out en: prefix
langs.append(splits[0][:2])
words.append(splits[0][3:])
if len(splits) > 1:
labels.append(splits[-1].replace("\n", ""))
else:
# examples have no label in test set
labels.append("O")
```
If you agree, me or @lvwerra would be happy to implement this and create a PR.
Hi !
You have to point to your local script.
First clone the repo and then:
```python
dataset = load_dataset("./datasets/xtreme", "PAN-X.en")
```
The "xtreme" directory contains "xtreme.py".
You also have to change the features definition in the `_info` method. You could use:
```python
features = nlp.Features({
"words": [nlp.Value("string")],
"ner_tags": [nlp.Value("string")],
"langs": [nlp.Value("string")],
})
```
Hope this helps !
Let me know if you have other questions. |
https://github.com/huggingface/datasets/issues/425 | Correct data structure for PAN-X task in XTREME dataset? | Thanks, I am making progress. I got a new error `NonMatchingSplitsSizesError ` (see traceback below), which I suspect is due to the fact that number of rows in the dataset changed (one row per word --> one row per sentence) as well as the number of bytes due to the slightly updated data structure.
```python
NonMatchingSplitsSizesError: [{'expected': SplitInfo(name='validation', num_bytes=1756492, num_examples=80536, dataset_name='xtreme'), 'recorded': SplitInfo(name='validation', num_bytes=1837109, num_examples=10000, dataset_name='xtreme')}, {'expected': SplitInfo(name='test', num_bytes=1752572, num_examples=80326, dataset_name='xtreme'), 'recorded': SplitInfo(name='test', num_bytes=1833214, num_examples=10000, dataset_name='xtreme')}, {'expected': SplitInfo(name='train', num_bytes=3496832, num_examples=160394, dataset_name='xtreme'), 'recorded': SplitInfo(name='train', num_bytes=3658428, num_examples=20000, dataset_name='xtreme')}]
```
I can fix the error by replacing the values in the `datasets_infos.json` file, which I tested for English. However, to update this for all 40 datasets manually is slightly painful. Is there a better way to update the expected values for all datasets? | Hi π€ team!
## Description of the problem
Thanks to the fix from #416 I am now able to load the NER task in the XTREME dataset as follows:
```python
from nlp import load_dataset
# AmazonPhotos.zip is located in data/
dataset = load_dataset("xtreme", "PAN-X.en", data_dir='./data')
dataset_train = dataset['train']
```
However, I am not sure that `load_dataset()` is returning the correct data structure for NER.
Currently, every row in `dataset_train` is of the form
```python
{'word': str, 'ner_tag': str, 'lang': str}
```
but I think we actually want something like
```python
{'words': List[str], 'ner_tags': List[str], 'langs': List[str]}
```
so that each row corresponds to a _sequence_ of words associated with each example. With the current data structure I do not think it is possible to transform `dataset_train` into a form suitable for training because we do not know the boundaries between examples.
Indeed, [this line](https://github.com/google-research/xtreme/blob/522434d1aece34131d997a97ce7e9242a51a688a/third_party/utils_tag.py#L58) in the XTREME repo, processes the texts as lists of sentences, tags, and languages.
## Proposed solution
Replace
```python
with open(filepath) as f:
data = csv.reader(f, delimiter="\t", quoting=csv.QUOTE_NONE)
for id_, row in enumerate(data):
if row:
lang, word = row[0].split(":")[0], row[0].split(":")[1]
tag = row[1]
yield id_, {"word": word, "ner_tag": tag, "lang": lang}
```
from [these lines](https://github.com/huggingface/nlp/blob/ce7d3a1d630b78fe27188d1706f3ea980e8eec43/datasets/xtreme/xtreme.py#L881-L887) of the `_generate_examples()` function with something like
```python
guid_index = 1
with open(filepath, encoding="utf-8") as f:
words = []
ner_tags = []
langs = []
for line in f:
if line.startswith("-DOCSTART-") or line == "" or line == "\n":
if words:
yield guid_index, {"words": words, "ner_tags": ner_tags, "langs": langs}
guid_index += 1
words = []
ner_tags = []
else:
# pan-x data is tab separated
splits = line.split("\t")
# strip out en: prefix
langs.append(splits[0][:2])
words.append(splits[0][3:])
if len(splits) > 1:
labels.append(splits[-1].replace("\n", ""))
else:
# examples have no label in test set
labels.append("O")
```
If you agree, me or @lvwerra would be happy to implement this and create a PR. | 130 | Correct data structure for PAN-X task in XTREME dataset?
Hi π€ team!
## Description of the problem
Thanks to the fix from #416 I am now able to load the NER task in the XTREME dataset as follows:
```python
from nlp import load_dataset
# AmazonPhotos.zip is located in data/
dataset = load_dataset("xtreme", "PAN-X.en", data_dir='./data')
dataset_train = dataset['train']
```
However, I am not sure that `load_dataset()` is returning the correct data structure for NER.
Currently, every row in `dataset_train` is of the form
```python
{'word': str, 'ner_tag': str, 'lang': str}
```
but I think we actually want something like
```python
{'words': List[str], 'ner_tags': List[str], 'langs': List[str]}
```
so that each row corresponds to a _sequence_ of words associated with each example. With the current data structure I do not think it is possible to transform `dataset_train` into a form suitable for training because we do not know the boundaries between examples.
Indeed, [this line](https://github.com/google-research/xtreme/blob/522434d1aece34131d997a97ce7e9242a51a688a/third_party/utils_tag.py#L58) in the XTREME repo, processes the texts as lists of sentences, tags, and languages.
## Proposed solution
Replace
```python
with open(filepath) as f:
data = csv.reader(f, delimiter="\t", quoting=csv.QUOTE_NONE)
for id_, row in enumerate(data):
if row:
lang, word = row[0].split(":")[0], row[0].split(":")[1]
tag = row[1]
yield id_, {"word": word, "ner_tag": tag, "lang": lang}
```
from [these lines](https://github.com/huggingface/nlp/blob/ce7d3a1d630b78fe27188d1706f3ea980e8eec43/datasets/xtreme/xtreme.py#L881-L887) of the `_generate_examples()` function with something like
```python
guid_index = 1
with open(filepath, encoding="utf-8") as f:
words = []
ner_tags = []
langs = []
for line in f:
if line.startswith("-DOCSTART-") or line == "" or line == "\n":
if words:
yield guid_index, {"words": words, "ner_tags": ner_tags, "langs": langs}
guid_index += 1
words = []
ner_tags = []
else:
# pan-x data is tab separated
splits = line.split("\t")
# strip out en: prefix
langs.append(splits[0][:2])
words.append(splits[0][3:])
if len(splits) > 1:
labels.append(splits[-1].replace("\n", ""))
else:
# examples have no label in test set
labels.append("O")
```
If you agree, me or @lvwerra would be happy to implement this and create a PR.
Thanks, I am making progress. I got a new error `NonMatchingSplitsSizesError ` (see traceback below), which I suspect is due to the fact that number of rows in the dataset changed (one row per word --> one row per sentence) as well as the number of bytes due to the slightly updated data structure.
```python
NonMatchingSplitsSizesError: [{'expected': SplitInfo(name='validation', num_bytes=1756492, num_examples=80536, dataset_name='xtreme'), 'recorded': SplitInfo(name='validation', num_bytes=1837109, num_examples=10000, dataset_name='xtreme')}, {'expected': SplitInfo(name='test', num_bytes=1752572, num_examples=80326, dataset_name='xtreme'), 'recorded': SplitInfo(name='test', num_bytes=1833214, num_examples=10000, dataset_name='xtreme')}, {'expected': SplitInfo(name='train', num_bytes=3496832, num_examples=160394, dataset_name='xtreme'), 'recorded': SplitInfo(name='train', num_bytes=3658428, num_examples=20000, dataset_name='xtreme')}]
```
I can fix the error by replacing the values in the `datasets_infos.json` file, which I tested for English. However, to update this for all 40 datasets manually is slightly painful. Is there a better way to update the expected values for all datasets? |
https://github.com/huggingface/datasets/issues/425 | Correct data structure for PAN-X task in XTREME dataset? | One more thing about features. I mentioned
```python
features = nlp.Features({
"words": [nlp.Value("string")],
"ner_tags": [nlp.Value("string")],
"langs": [nlp.Value("string")],
})
```
but it's actually not consistent with the way we write datasets. Something like this is simpler to read and more consistent with the way we define datasets:
```python
features = nlp.Features({
"words": nlp.Sequence(nlp.Value("string")),
"ner_tags": nlp.Sequence(nlp.Value("string")),
"langs": nlp.Sequence(nlp.Value("string")),
})
```
Sorry about that | Hi π€ team!
## Description of the problem
Thanks to the fix from #416 I am now able to load the NER task in the XTREME dataset as follows:
```python
from nlp import load_dataset
# AmazonPhotos.zip is located in data/
dataset = load_dataset("xtreme", "PAN-X.en", data_dir='./data')
dataset_train = dataset['train']
```
However, I am not sure that `load_dataset()` is returning the correct data structure for NER.
Currently, every row in `dataset_train` is of the form
```python
{'word': str, 'ner_tag': str, 'lang': str}
```
but I think we actually want something like
```python
{'words': List[str], 'ner_tags': List[str], 'langs': List[str]}
```
so that each row corresponds to a _sequence_ of words associated with each example. With the current data structure I do not think it is possible to transform `dataset_train` into a form suitable for training because we do not know the boundaries between examples.
Indeed, [this line](https://github.com/google-research/xtreme/blob/522434d1aece34131d997a97ce7e9242a51a688a/third_party/utils_tag.py#L58) in the XTREME repo, processes the texts as lists of sentences, tags, and languages.
## Proposed solution
Replace
```python
with open(filepath) as f:
data = csv.reader(f, delimiter="\t", quoting=csv.QUOTE_NONE)
for id_, row in enumerate(data):
if row:
lang, word = row[0].split(":")[0], row[0].split(":")[1]
tag = row[1]
yield id_, {"word": word, "ner_tag": tag, "lang": lang}
```
from [these lines](https://github.com/huggingface/nlp/blob/ce7d3a1d630b78fe27188d1706f3ea980e8eec43/datasets/xtreme/xtreme.py#L881-L887) of the `_generate_examples()` function with something like
```python
guid_index = 1
with open(filepath, encoding="utf-8") as f:
words = []
ner_tags = []
langs = []
for line in f:
if line.startswith("-DOCSTART-") or line == "" or line == "\n":
if words:
yield guid_index, {"words": words, "ner_tags": ner_tags, "langs": langs}
guid_index += 1
words = []
ner_tags = []
else:
# pan-x data is tab separated
splits = line.split("\t")
# strip out en: prefix
langs.append(splits[0][:2])
words.append(splits[0][3:])
if len(splits) > 1:
labels.append(splits[-1].replace("\n", ""))
else:
# examples have no label in test set
labels.append("O")
```
If you agree, me or @lvwerra would be happy to implement this and create a PR. | 61 | Correct data structure for PAN-X task in XTREME dataset?
Hi π€ team!
## Description of the problem
Thanks to the fix from #416 I am now able to load the NER task in the XTREME dataset as follows:
```python
from nlp import load_dataset
# AmazonPhotos.zip is located in data/
dataset = load_dataset("xtreme", "PAN-X.en", data_dir='./data')
dataset_train = dataset['train']
```
However, I am not sure that `load_dataset()` is returning the correct data structure for NER.
Currently, every row in `dataset_train` is of the form
```python
{'word': str, 'ner_tag': str, 'lang': str}
```
but I think we actually want something like
```python
{'words': List[str], 'ner_tags': List[str], 'langs': List[str]}
```
so that each row corresponds to a _sequence_ of words associated with each example. With the current data structure I do not think it is possible to transform `dataset_train` into a form suitable for training because we do not know the boundaries between examples.
Indeed, [this line](https://github.com/google-research/xtreme/blob/522434d1aece34131d997a97ce7e9242a51a688a/third_party/utils_tag.py#L58) in the XTREME repo, processes the texts as lists of sentences, tags, and languages.
## Proposed solution
Replace
```python
with open(filepath) as f:
data = csv.reader(f, delimiter="\t", quoting=csv.QUOTE_NONE)
for id_, row in enumerate(data):
if row:
lang, word = row[0].split(":")[0], row[0].split(":")[1]
tag = row[1]
yield id_, {"word": word, "ner_tag": tag, "lang": lang}
```
from [these lines](https://github.com/huggingface/nlp/blob/ce7d3a1d630b78fe27188d1706f3ea980e8eec43/datasets/xtreme/xtreme.py#L881-L887) of the `_generate_examples()` function with something like
```python
guid_index = 1
with open(filepath, encoding="utf-8") as f:
words = []
ner_tags = []
langs = []
for line in f:
if line.startswith("-DOCSTART-") or line == "" or line == "\n":
if words:
yield guid_index, {"words": words, "ner_tags": ner_tags, "langs": langs}
guid_index += 1
words = []
ner_tags = []
else:
# pan-x data is tab separated
splits = line.split("\t")
# strip out en: prefix
langs.append(splits[0][:2])
words.append(splits[0][3:])
if len(splits) > 1:
labels.append(splits[-1].replace("\n", ""))
else:
# examples have no label in test set
labels.append("O")
```
If you agree, me or @lvwerra would be happy to implement this and create a PR.
One more thing about features. I mentioned
```python
features = nlp.Features({
"words": [nlp.Value("string")],
"ner_tags": [nlp.Value("string")],
"langs": [nlp.Value("string")],
})
```
but it's actually not consistent with the way we write datasets. Something like this is simpler to read and more consistent with the way we define datasets:
```python
features = nlp.Features({
"words": nlp.Sequence(nlp.Value("string")),
"ner_tags": nlp.Sequence(nlp.Value("string")),
"langs": nlp.Sequence(nlp.Value("string")),
})
```
Sorry about that |
https://github.com/huggingface/datasets/issues/418 | Addition of google drive links to dl_manager | I think the problem is the way you wrote your urls. Try the following structure to see `https://drive.google.com/uc?export=download&id=your_file_id` .
@lhoestq | Hello there, I followed the template to create a download script of my own, which works fine for me, although I had to shun the dl_manager because it was downloading nothing from the drive links and instead use gdown.
This is the script for me:
```python
class EmoConfig(nlp.BuilderConfig):
"""BuilderConfig for SQUAD."""
def __init__(self, **kwargs):
"""BuilderConfig for EmoContext.
Args:
**kwargs: keyword arguments forwarded to super.
"""
super(EmoConfig, self).__init__(**kwargs)
_TEST_URL = "https://drive.google.com/file/d/1Hn5ytHSSoGOC4sjm3wYy0Dh0oY_oXBbb/view?usp=sharing"
_TRAIN_URL = "https://drive.google.com/file/d/12Uz59TYg_NtxOy7SXraYeXPMRT7oaO7X/view?usp=sharing"
class EmoDataset(nlp.GeneratorBasedBuilder):
""" SemEval-2019 Task 3: EmoContext Contextual Emotion Detection in Text. Version 1.0.0 """
VERSION = nlp.Version("1.0.0")
force = False
def _info(self):
return nlp.DatasetInfo(
description=_DESCRIPTION,
features=nlp.Features(
{
"text": nlp.Value("string"),
"label": nlp.features.ClassLabel(names=["others", "happy", "sad", "angry"]),
}
),
supervised_keys=None,
homepage="https://www.aclweb.org/anthology/S19-2005/",
citation=_CITATION,
)
def _get_drive_url(self, url):
base_url = 'https://drive.google.com/uc?id='
split_url = url.split('/')
return base_url + split_url[5]
def _split_generators(self, dl_manager):
"""Returns SplitGenerators."""
if(not os.path.exists("emo-train.json") or self.force):
gdown.download(self._get_drive_url(_TRAIN_URL), "emo-train.json", quiet = True)
if(not os.path.exists("emo-test.json") or self.force):
gdown.download(self._get_drive_url(_TEST_URL), "emo-test.json", quiet = True)
return [
nlp.SplitGenerator(
name=nlp.Split.TRAIN,
gen_kwargs={
"filepath": "emo-train.json",
"split": "train",
},
),
nlp.SplitGenerator(
name=nlp.Split.TEST,
gen_kwargs={"filepath": "emo-test.json", "split": "test"},
),
]
def _generate_examples(self, filepath, split):
""" Yields examples. """
with open(filepath, 'rb') as f:
data = json.load(f)
for id_, text, label in zip(data["text"].keys(), data["text"].values(), data["Label"].values()):
yield id_, {
"text": text,
"label": label,
}
```
Can someone help me in adding gdrive links to be used with default dl_manager or adding gdown as another dl_manager, because I'd like to add this dataset to nlp's official database. | 20 | Addition of google drive links to dl_manager
Hello there, I followed the template to create a download script of my own, which works fine for me, although I had to shun the dl_manager because it was downloading nothing from the drive links and instead use gdown.
This is the script for me:
```python
class EmoConfig(nlp.BuilderConfig):
"""BuilderConfig for SQUAD."""
def __init__(self, **kwargs):
"""BuilderConfig for EmoContext.
Args:
**kwargs: keyword arguments forwarded to super.
"""
super(EmoConfig, self).__init__(**kwargs)
_TEST_URL = "https://drive.google.com/file/d/1Hn5ytHSSoGOC4sjm3wYy0Dh0oY_oXBbb/view?usp=sharing"
_TRAIN_URL = "https://drive.google.com/file/d/12Uz59TYg_NtxOy7SXraYeXPMRT7oaO7X/view?usp=sharing"
class EmoDataset(nlp.GeneratorBasedBuilder):
""" SemEval-2019 Task 3: EmoContext Contextual Emotion Detection in Text. Version 1.0.0 """
VERSION = nlp.Version("1.0.0")
force = False
def _info(self):
return nlp.DatasetInfo(
description=_DESCRIPTION,
features=nlp.Features(
{
"text": nlp.Value("string"),
"label": nlp.features.ClassLabel(names=["others", "happy", "sad", "angry"]),
}
),
supervised_keys=None,
homepage="https://www.aclweb.org/anthology/S19-2005/",
citation=_CITATION,
)
def _get_drive_url(self, url):
base_url = 'https://drive.google.com/uc?id='
split_url = url.split('/')
return base_url + split_url[5]
def _split_generators(self, dl_manager):
"""Returns SplitGenerators."""
if(not os.path.exists("emo-train.json") or self.force):
gdown.download(self._get_drive_url(_TRAIN_URL), "emo-train.json", quiet = True)
if(not os.path.exists("emo-test.json") or self.force):
gdown.download(self._get_drive_url(_TEST_URL), "emo-test.json", quiet = True)
return [
nlp.SplitGenerator(
name=nlp.Split.TRAIN,
gen_kwargs={
"filepath": "emo-train.json",
"split": "train",
},
),
nlp.SplitGenerator(
name=nlp.Split.TEST,
gen_kwargs={"filepath": "emo-test.json", "split": "test"},
),
]
def _generate_examples(self, filepath, split):
""" Yields examples. """
with open(filepath, 'rb') as f:
data = json.load(f)
for id_, text, label in zip(data["text"].keys(), data["text"].values(), data["Label"].values()):
yield id_, {
"text": text,
"label": label,
}
```
Can someone help me in adding gdrive links to be used with default dl_manager or adding gdown as another dl_manager, because I'd like to add this dataset to nlp's official database.
I think the problem is the way you wrote your urls. Try the following structure to see `https://drive.google.com/uc?export=download&id=your_file_id` .
@lhoestq |
https://github.com/huggingface/datasets/issues/418 | Addition of google drive links to dl_manager | Oh sorry, I think `_get_drive_url` is doing that.
Have you tried to use `dl_manager.download_and_extract(_get_drive_url(_TRAIN_URL)`? it should work with google drive links.
| Hello there, I followed the template to create a download script of my own, which works fine for me, although I had to shun the dl_manager because it was downloading nothing from the drive links and instead use gdown.
This is the script for me:
```python
class EmoConfig(nlp.BuilderConfig):
"""BuilderConfig for SQUAD."""
def __init__(self, **kwargs):
"""BuilderConfig for EmoContext.
Args:
**kwargs: keyword arguments forwarded to super.
"""
super(EmoConfig, self).__init__(**kwargs)
_TEST_URL = "https://drive.google.com/file/d/1Hn5ytHSSoGOC4sjm3wYy0Dh0oY_oXBbb/view?usp=sharing"
_TRAIN_URL = "https://drive.google.com/file/d/12Uz59TYg_NtxOy7SXraYeXPMRT7oaO7X/view?usp=sharing"
class EmoDataset(nlp.GeneratorBasedBuilder):
""" SemEval-2019 Task 3: EmoContext Contextual Emotion Detection in Text. Version 1.0.0 """
VERSION = nlp.Version("1.0.0")
force = False
def _info(self):
return nlp.DatasetInfo(
description=_DESCRIPTION,
features=nlp.Features(
{
"text": nlp.Value("string"),
"label": nlp.features.ClassLabel(names=["others", "happy", "sad", "angry"]),
}
),
supervised_keys=None,
homepage="https://www.aclweb.org/anthology/S19-2005/",
citation=_CITATION,
)
def _get_drive_url(self, url):
base_url = 'https://drive.google.com/uc?id='
split_url = url.split('/')
return base_url + split_url[5]
def _split_generators(self, dl_manager):
"""Returns SplitGenerators."""
if(not os.path.exists("emo-train.json") or self.force):
gdown.download(self._get_drive_url(_TRAIN_URL), "emo-train.json", quiet = True)
if(not os.path.exists("emo-test.json") or self.force):
gdown.download(self._get_drive_url(_TEST_URL), "emo-test.json", quiet = True)
return [
nlp.SplitGenerator(
name=nlp.Split.TRAIN,
gen_kwargs={
"filepath": "emo-train.json",
"split": "train",
},
),
nlp.SplitGenerator(
name=nlp.Split.TEST,
gen_kwargs={"filepath": "emo-test.json", "split": "test"},
),
]
def _generate_examples(self, filepath, split):
""" Yields examples. """
with open(filepath, 'rb') as f:
data = json.load(f)
for id_, text, label in zip(data["text"].keys(), data["text"].values(), data["Label"].values()):
yield id_, {
"text": text,
"label": label,
}
```
Can someone help me in adding gdrive links to be used with default dl_manager or adding gdown as another dl_manager, because I'd like to add this dataset to nlp's official database. | 21 | Addition of google drive links to dl_manager
Hello there, I followed the template to create a download script of my own, which works fine for me, although I had to shun the dl_manager because it was downloading nothing from the drive links and instead use gdown.
This is the script for me:
```python
class EmoConfig(nlp.BuilderConfig):
"""BuilderConfig for SQUAD."""
def __init__(self, **kwargs):
"""BuilderConfig for EmoContext.
Args:
**kwargs: keyword arguments forwarded to super.
"""
super(EmoConfig, self).__init__(**kwargs)
_TEST_URL = "https://drive.google.com/file/d/1Hn5ytHSSoGOC4sjm3wYy0Dh0oY_oXBbb/view?usp=sharing"
_TRAIN_URL = "https://drive.google.com/file/d/12Uz59TYg_NtxOy7SXraYeXPMRT7oaO7X/view?usp=sharing"
class EmoDataset(nlp.GeneratorBasedBuilder):
""" SemEval-2019 Task 3: EmoContext Contextual Emotion Detection in Text. Version 1.0.0 """
VERSION = nlp.Version("1.0.0")
force = False
def _info(self):
return nlp.DatasetInfo(
description=_DESCRIPTION,
features=nlp.Features(
{
"text": nlp.Value("string"),
"label": nlp.features.ClassLabel(names=["others", "happy", "sad", "angry"]),
}
),
supervised_keys=None,
homepage="https://www.aclweb.org/anthology/S19-2005/",
citation=_CITATION,
)
def _get_drive_url(self, url):
base_url = 'https://drive.google.com/uc?id='
split_url = url.split('/')
return base_url + split_url[5]
def _split_generators(self, dl_manager):
"""Returns SplitGenerators."""
if(not os.path.exists("emo-train.json") or self.force):
gdown.download(self._get_drive_url(_TRAIN_URL), "emo-train.json", quiet = True)
if(not os.path.exists("emo-test.json") or self.force):
gdown.download(self._get_drive_url(_TEST_URL), "emo-test.json", quiet = True)
return [
nlp.SplitGenerator(
name=nlp.Split.TRAIN,
gen_kwargs={
"filepath": "emo-train.json",
"split": "train",
},
),
nlp.SplitGenerator(
name=nlp.Split.TEST,
gen_kwargs={"filepath": "emo-test.json", "split": "test"},
),
]
def _generate_examples(self, filepath, split):
""" Yields examples. """
with open(filepath, 'rb') as f:
data = json.load(f)
for id_, text, label in zip(data["text"].keys(), data["text"].values(), data["Label"].values()):
yield id_, {
"text": text,
"label": label,
}
```
Can someone help me in adding gdrive links to be used with default dl_manager or adding gdown as another dl_manager, because I'd like to add this dataset to nlp's official database.
Oh sorry, I think `_get_drive_url` is doing that.
Have you tried to use `dl_manager.download_and_extract(_get_drive_url(_TRAIN_URL)`? it should work with google drive links.
|
https://github.com/huggingface/datasets/issues/414 | from_dict delete? | `from_dict` was added in #350 that was unfortunately not included in the 0.3.0 release. It's going to be included in the next release that will be out pretty soon though.
Right now if you want to use `from_dict` you have to install the package from the master branch
```
pip install git+https://github.com/huggingface/nlp.git
``` | AttributeError: type object 'Dataset' has no attribute 'from_dict' | 53 | from_dict delete?
AttributeError: type object 'Dataset' has no attribute 'from_dict'
`from_dict` was added in #350 that was unfortunately not included in the 0.3.0 release. It's going to be included in the next release that will be out pretty soon though.
Right now if you want to use `from_dict` you have to install the package from the master branch
```
pip install git+https://github.com/huggingface/nlp.git
``` |
https://github.com/huggingface/datasets/issues/414 | from_dict delete? | > `from_dict` was added in #350 that was unfortunately not included in the 0.3.0 release. It's going to be included in the next release that will be out pretty soon though.
> Right now if you want to use `from_dict` you have to install the package from the master branch
>
> ```
> pip install git+https://github.com/huggingface/nlp.git
> ```
OK, thank you.
| AttributeError: type object 'Dataset' has no attribute 'from_dict' | 62 | from_dict delete?
AttributeError: type object 'Dataset' has no attribute 'from_dict'
> `from_dict` was added in #350 that was unfortunately not included in the 0.3.0 release. It's going to be included in the next release that will be out pretty soon though.
> Right now if you want to use `from_dict` you have to install the package from the master branch
>
> ```
> pip install git+https://github.com/huggingface/nlp.git
> ```
OK, thank you.
|
https://github.com/huggingface/datasets/issues/413 | Is there a way to download only NQ dev? | Unfortunately it's not possible to download only the dev set of NQ.
I think we could add a way to download only the test set by adding a custom configuration to the processing script though. | Maybe I missed that in the docs, but is there a way to only download the dev set of natural questions (~1 GB)?
As we want to benchmark QA models on different datasets, I would like to avoid downloading the 41GB of training data.
I tried
```
dataset = nlp.load_dataset('natural_questions', split="validation", beam_runner="DirectRunner")
```
But this still triggered a big download of presumably the whole dataset. Is there any way of doing this or are splits / slicing options only available after downloading?
Thanks! | 35 | Is there a way to download only NQ dev?
Maybe I missed that in the docs, but is there a way to only download the dev set of natural questions (~1 GB)?
As we want to benchmark QA models on different datasets, I would like to avoid downloading the 41GB of training data.
I tried
```
dataset = nlp.load_dataset('natural_questions', split="validation", beam_runner="DirectRunner")
```
But this still triggered a big download of presumably the whole dataset. Is there any way of doing this or are splits / slicing options only available after downloading?
Thanks!
Unfortunately it's not possible to download only the dev set of NQ.
I think we could add a way to download only the test set by adding a custom configuration to the processing script though. |
https://github.com/huggingface/datasets/issues/413 | Is there a way to download only NQ dev? | Ok, got it. I think this could be a valuable feature - especially for large datasets like NQ, but potentially also others.
For us, it will in this case make the difference of using the library or keeping the old downloads of the raw dev datasets.
However, I don't know if that fits into your plans with the library and can also understand if you don't want to support this. | Maybe I missed that in the docs, but is there a way to only download the dev set of natural questions (~1 GB)?
As we want to benchmark QA models on different datasets, I would like to avoid downloading the 41GB of training data.
I tried
```
dataset = nlp.load_dataset('natural_questions', split="validation", beam_runner="DirectRunner")
```
But this still triggered a big download of presumably the whole dataset. Is there any way of doing this or are splits / slicing options only available after downloading?
Thanks! | 70 | Is there a way to download only NQ dev?
Maybe I missed that in the docs, but is there a way to only download the dev set of natural questions (~1 GB)?
As we want to benchmark QA models on different datasets, I would like to avoid downloading the 41GB of training data.
I tried
```
dataset = nlp.load_dataset('natural_questions', split="validation", beam_runner="DirectRunner")
```
But this still triggered a big download of presumably the whole dataset. Is there any way of doing this or are splits / slicing options only available after downloading?
Thanks!
Ok, got it. I think this could be a valuable feature - especially for large datasets like NQ, but potentially also others.
For us, it will in this case make the difference of using the library or keeping the old downloads of the raw dev datasets.
However, I don't know if that fits into your plans with the library and can also understand if you don't want to support this. |
https://github.com/huggingface/datasets/issues/413 | Is there a way to download only NQ dev? | I don't think we could force this behavior generally since the dataset script authors are free to organize the file download as they want (sometimes the mapping between split and files can be very much nontrivial) but we can add an additional configuration for Natural Question indeed as @lhoestq indicate. | Maybe I missed that in the docs, but is there a way to only download the dev set of natural questions (~1 GB)?
As we want to benchmark QA models on different datasets, I would like to avoid downloading the 41GB of training data.
I tried
```
dataset = nlp.load_dataset('natural_questions', split="validation", beam_runner="DirectRunner")
```
But this still triggered a big download of presumably the whole dataset. Is there any way of doing this or are splits / slicing options only available after downloading?
Thanks! | 50 | Is there a way to download only NQ dev?
Maybe I missed that in the docs, but is there a way to only download the dev set of natural questions (~1 GB)?
As we want to benchmark QA models on different datasets, I would like to avoid downloading the 41GB of training data.
I tried
```
dataset = nlp.load_dataset('natural_questions', split="validation", beam_runner="DirectRunner")
```
But this still triggered a big download of presumably the whole dataset. Is there any way of doing this or are splits / slicing options only available after downloading?
Thanks!
I don't think we could force this behavior generally since the dataset script authors are free to organize the file download as they want (sometimes the mapping between split and files can be very much nontrivial) but we can add an additional configuration for Natural Question indeed as @lhoestq indicate. |
https://github.com/huggingface/datasets/issues/412 | Unable to load XTREME dataset from disk | Hi @lewtun, you have to provide the full path to the downloaded file for example `/home/lewtum/..` | Hi π€ team!
## Description of the problem
Following the [docs](https://huggingface.co/nlp/loading_datasets.html?highlight=xtreme#manually-downloading-files) I'm trying to load the `PAN-X.fr` dataset from the [XTREME](https://github.com/google-research/xtreme) benchmark.
I have manually downloaded the `AmazonPhotos.zip` file from [here](https://www.amazon.com/clouddrive/share/d3KGCRCIYwhKJF0H3eWA26hjg2ZCRhjpEQtDL70FSBN?_encoding=UTF8&%2AVersion%2A=1&%2Aentries%2A=0&mgh=1) and am running into a `FileNotFoundError` when I point to the location of the dataset.
As far as I can tell, the problem is that `AmazonPhotos.zip` decompresses to `panx_dataset` and `load_dataset()` is not looking in the correct path:
```
# path where load_dataset is looking for fr.tar.gz
/root/.cache/huggingface/datasets/9b8c4f1578e45cb2539332c79738beb3b54afbcd842b079cabfd79e3ed6704f6/
# path where it actually exists
/root/.cache/huggingface/datasets/9b8c4f1578e45cb2539332c79738beb3b54afbcd842b079cabfd79e3ed6704f6/panx_dataset/
```
## Steps to reproduce the problem
1. Manually download the XTREME benchmark from [here](https://www.amazon.com/clouddrive/share/d3KGCRCIYwhKJF0H3eWA26hjg2ZCRhjpEQtDL70FSBN?_encoding=UTF8&%2AVersion%2A=1&%2Aentries%2A=0&mgh=1)
2. Run the following code snippet
```python
from nlp import load_dataset
# AmazonPhotos.zip is in the root of the folder
dataset = load_dataset("xtreme", "PAN-X.fr", data_dir='./')
```
3. Here is the stack trace
```
---------------------------------------------------------------------------
FileNotFoundError Traceback (most recent call last)
<ipython-input-4-26786bb5fa93> in <module>
----> 1 dataset = load_dataset("xtreme", "PAN-X.fr", data_dir='./')
/usr/local/lib/python3.6/dist-packages/nlp/load.py in load_dataset(path, name, version, data_dir, data_files, split, cache_dir, download_config, download_mode, ignore_verifications, save_infos, **config_kwargs)
522 download_mode=download_mode,
523 ignore_verifications=ignore_verifications,
--> 524 save_infos=save_infos,
525 )
526
/usr/local/lib/python3.6/dist-packages/nlp/builder.py in download_and_prepare(self, download_config, download_mode, ignore_verifications, save_infos, try_from_hf_gcs, dl_manager, **download_and_prepare_kwargs)
430 verify_infos = not save_infos and not ignore_verifications
431 self._download_and_prepare(
--> 432 dl_manager=dl_manager, verify_infos=verify_infos, **download_and_prepare_kwargs
433 )
434 # Sync info
/usr/local/lib/python3.6/dist-packages/nlp/builder.py in _download_and_prepare(self, dl_manager, verify_infos, **prepare_split_kwargs)
464 split_dict = SplitDict(dataset_name=self.name)
465 split_generators_kwargs = self._make_split_generators_kwargs(prepare_split_kwargs)
--> 466 split_generators = self._split_generators(dl_manager, **split_generators_kwargs)
467 # Checksums verification
468 if verify_infos:
/usr/local/lib/python3.6/dist-packages/nlp/datasets/xtreme/b8c2ed3583a7a7ac60b503576dfed3271ac86757628897e945bd329c43b8a746/xtreme.py in _split_generators(self, dl_manager)
725 panx_dl_dir = dl_manager.extract(panx_path)
726 lang = self.config.name.split(".")[1]
--> 727 lang_folder = dl_manager.extract(os.path.join(panx_dl_dir, lang + ".tar.gz"))
728 return [
729 nlp.SplitGenerator(
/usr/local/lib/python3.6/dist-packages/nlp/utils/download_manager.py in extract(self, path_or_paths)
196 """
197 return map_nested(
--> 198 lambda path: cached_path(path, extract_compressed_file=True, force_extract=False), path_or_paths,
199 )
200
/usr/local/lib/python3.6/dist-packages/nlp/utils/py_utils.py in map_nested(function, data_struct, dict_only, map_tuple)
170 return tuple(mapped)
171 # Singleton
--> 172 return function(data_struct)
173
174
/usr/local/lib/python3.6/dist-packages/nlp/utils/download_manager.py in <lambda>(path)
196 """
197 return map_nested(
--> 198 lambda path: cached_path(path, extract_compressed_file=True, force_extract=False), path_or_paths,
199 )
200
/usr/local/lib/python3.6/dist-packages/nlp/utils/file_utils.py in cached_path(url_or_filename, download_config, **download_kwargs)
203 elif urlparse(url_or_filename).scheme == "":
204 # File, but it doesn't exist.
--> 205 raise FileNotFoundError("Local file {} doesn't exist".format(url_or_filename))
206 else:
207 # Something unknown
FileNotFoundError: Local file /root/.cache/huggingface/datasets/9b8c4f1578e45cb2539332c79738beb3b54afbcd842b079cabfd79e3ed6704f6/fr.tar.gz doesn't exist
```
## OS and hardware
```
- `nlp` version: 0.3.0
- Platform: Linux-4.15.0-72-generic-x86_64-with-Ubuntu-18.04-bionic
- Python version: 3.6.9
- PyTorch version (GPU?): 1.4.0 (True)
- Tensorflow version (GPU?): 2.1.0 (True)
- Using GPU in script?: <fill in>
- Using distributed or parallel set-up in script?: <fill in>
``` | 16 | Unable to load XTREME dataset from disk
Hi π€ team!
## Description of the problem
Following the [docs](https://huggingface.co/nlp/loading_datasets.html?highlight=xtreme#manually-downloading-files) I'm trying to load the `PAN-X.fr` dataset from the [XTREME](https://github.com/google-research/xtreme) benchmark.
I have manually downloaded the `AmazonPhotos.zip` file from [here](https://www.amazon.com/clouddrive/share/d3KGCRCIYwhKJF0H3eWA26hjg2ZCRhjpEQtDL70FSBN?_encoding=UTF8&%2AVersion%2A=1&%2Aentries%2A=0&mgh=1) and am running into a `FileNotFoundError` when I point to the location of the dataset.
As far as I can tell, the problem is that `AmazonPhotos.zip` decompresses to `panx_dataset` and `load_dataset()` is not looking in the correct path:
```
# path where load_dataset is looking for fr.tar.gz
/root/.cache/huggingface/datasets/9b8c4f1578e45cb2539332c79738beb3b54afbcd842b079cabfd79e3ed6704f6/
# path where it actually exists
/root/.cache/huggingface/datasets/9b8c4f1578e45cb2539332c79738beb3b54afbcd842b079cabfd79e3ed6704f6/panx_dataset/
```
## Steps to reproduce the problem
1. Manually download the XTREME benchmark from [here](https://www.amazon.com/clouddrive/share/d3KGCRCIYwhKJF0H3eWA26hjg2ZCRhjpEQtDL70FSBN?_encoding=UTF8&%2AVersion%2A=1&%2Aentries%2A=0&mgh=1)
2. Run the following code snippet
```python
from nlp import load_dataset
# AmazonPhotos.zip is in the root of the folder
dataset = load_dataset("xtreme", "PAN-X.fr", data_dir='./')
```
3. Here is the stack trace
```
---------------------------------------------------------------------------
FileNotFoundError Traceback (most recent call last)
<ipython-input-4-26786bb5fa93> in <module>
----> 1 dataset = load_dataset("xtreme", "PAN-X.fr", data_dir='./')
/usr/local/lib/python3.6/dist-packages/nlp/load.py in load_dataset(path, name, version, data_dir, data_files, split, cache_dir, download_config, download_mode, ignore_verifications, save_infos, **config_kwargs)
522 download_mode=download_mode,
523 ignore_verifications=ignore_verifications,
--> 524 save_infos=save_infos,
525 )
526
/usr/local/lib/python3.6/dist-packages/nlp/builder.py in download_and_prepare(self, download_config, download_mode, ignore_verifications, save_infos, try_from_hf_gcs, dl_manager, **download_and_prepare_kwargs)
430 verify_infos = not save_infos and not ignore_verifications
431 self._download_and_prepare(
--> 432 dl_manager=dl_manager, verify_infos=verify_infos, **download_and_prepare_kwargs
433 )
434 # Sync info
/usr/local/lib/python3.6/dist-packages/nlp/builder.py in _download_and_prepare(self, dl_manager, verify_infos, **prepare_split_kwargs)
464 split_dict = SplitDict(dataset_name=self.name)
465 split_generators_kwargs = self._make_split_generators_kwargs(prepare_split_kwargs)
--> 466 split_generators = self._split_generators(dl_manager, **split_generators_kwargs)
467 # Checksums verification
468 if verify_infos:
/usr/local/lib/python3.6/dist-packages/nlp/datasets/xtreme/b8c2ed3583a7a7ac60b503576dfed3271ac86757628897e945bd329c43b8a746/xtreme.py in _split_generators(self, dl_manager)
725 panx_dl_dir = dl_manager.extract(panx_path)
726 lang = self.config.name.split(".")[1]
--> 727 lang_folder = dl_manager.extract(os.path.join(panx_dl_dir, lang + ".tar.gz"))
728 return [
729 nlp.SplitGenerator(
/usr/local/lib/python3.6/dist-packages/nlp/utils/download_manager.py in extract(self, path_or_paths)
196 """
197 return map_nested(
--> 198 lambda path: cached_path(path, extract_compressed_file=True, force_extract=False), path_or_paths,
199 )
200
/usr/local/lib/python3.6/dist-packages/nlp/utils/py_utils.py in map_nested(function, data_struct, dict_only, map_tuple)
170 return tuple(mapped)
171 # Singleton
--> 172 return function(data_struct)
173
174
/usr/local/lib/python3.6/dist-packages/nlp/utils/download_manager.py in <lambda>(path)
196 """
197 return map_nested(
--> 198 lambda path: cached_path(path, extract_compressed_file=True, force_extract=False), path_or_paths,
199 )
200
/usr/local/lib/python3.6/dist-packages/nlp/utils/file_utils.py in cached_path(url_or_filename, download_config, **download_kwargs)
203 elif urlparse(url_or_filename).scheme == "":
204 # File, but it doesn't exist.
--> 205 raise FileNotFoundError("Local file {} doesn't exist".format(url_or_filename))
206 else:
207 # Something unknown
FileNotFoundError: Local file /root/.cache/huggingface/datasets/9b8c4f1578e45cb2539332c79738beb3b54afbcd842b079cabfd79e3ed6704f6/fr.tar.gz doesn't exist
```
## OS and hardware
```
- `nlp` version: 0.3.0
- Platform: Linux-4.15.0-72-generic-x86_64-with-Ubuntu-18.04-bionic
- Python version: 3.6.9
- PyTorch version (GPU?): 1.4.0 (True)
- Tensorflow version (GPU?): 2.1.0 (True)
- Using GPU in script?: <fill in>
- Using distributed or parallel set-up in script?: <fill in>
```
Hi @lewtun, you have to provide the full path to the downloaded file for example `/home/lewtum/..` |
https://github.com/huggingface/datasets/issues/412 | Unable to load XTREME dataset from disk | I was able to repro. Opening a PR to fix that.
Thanks for reporting this issue ! | Hi π€ team!
## Description of the problem
Following the [docs](https://huggingface.co/nlp/loading_datasets.html?highlight=xtreme#manually-downloading-files) I'm trying to load the `PAN-X.fr` dataset from the [XTREME](https://github.com/google-research/xtreme) benchmark.
I have manually downloaded the `AmazonPhotos.zip` file from [here](https://www.amazon.com/clouddrive/share/d3KGCRCIYwhKJF0H3eWA26hjg2ZCRhjpEQtDL70FSBN?_encoding=UTF8&%2AVersion%2A=1&%2Aentries%2A=0&mgh=1) and am running into a `FileNotFoundError` when I point to the location of the dataset.
As far as I can tell, the problem is that `AmazonPhotos.zip` decompresses to `panx_dataset` and `load_dataset()` is not looking in the correct path:
```
# path where load_dataset is looking for fr.tar.gz
/root/.cache/huggingface/datasets/9b8c4f1578e45cb2539332c79738beb3b54afbcd842b079cabfd79e3ed6704f6/
# path where it actually exists
/root/.cache/huggingface/datasets/9b8c4f1578e45cb2539332c79738beb3b54afbcd842b079cabfd79e3ed6704f6/panx_dataset/
```
## Steps to reproduce the problem
1. Manually download the XTREME benchmark from [here](https://www.amazon.com/clouddrive/share/d3KGCRCIYwhKJF0H3eWA26hjg2ZCRhjpEQtDL70FSBN?_encoding=UTF8&%2AVersion%2A=1&%2Aentries%2A=0&mgh=1)
2. Run the following code snippet
```python
from nlp import load_dataset
# AmazonPhotos.zip is in the root of the folder
dataset = load_dataset("xtreme", "PAN-X.fr", data_dir='./')
```
3. Here is the stack trace
```
---------------------------------------------------------------------------
FileNotFoundError Traceback (most recent call last)
<ipython-input-4-26786bb5fa93> in <module>
----> 1 dataset = load_dataset("xtreme", "PAN-X.fr", data_dir='./')
/usr/local/lib/python3.6/dist-packages/nlp/load.py in load_dataset(path, name, version, data_dir, data_files, split, cache_dir, download_config, download_mode, ignore_verifications, save_infos, **config_kwargs)
522 download_mode=download_mode,
523 ignore_verifications=ignore_verifications,
--> 524 save_infos=save_infos,
525 )
526
/usr/local/lib/python3.6/dist-packages/nlp/builder.py in download_and_prepare(self, download_config, download_mode, ignore_verifications, save_infos, try_from_hf_gcs, dl_manager, **download_and_prepare_kwargs)
430 verify_infos = not save_infos and not ignore_verifications
431 self._download_and_prepare(
--> 432 dl_manager=dl_manager, verify_infos=verify_infos, **download_and_prepare_kwargs
433 )
434 # Sync info
/usr/local/lib/python3.6/dist-packages/nlp/builder.py in _download_and_prepare(self, dl_manager, verify_infos, **prepare_split_kwargs)
464 split_dict = SplitDict(dataset_name=self.name)
465 split_generators_kwargs = self._make_split_generators_kwargs(prepare_split_kwargs)
--> 466 split_generators = self._split_generators(dl_manager, **split_generators_kwargs)
467 # Checksums verification
468 if verify_infos:
/usr/local/lib/python3.6/dist-packages/nlp/datasets/xtreme/b8c2ed3583a7a7ac60b503576dfed3271ac86757628897e945bd329c43b8a746/xtreme.py in _split_generators(self, dl_manager)
725 panx_dl_dir = dl_manager.extract(panx_path)
726 lang = self.config.name.split(".")[1]
--> 727 lang_folder = dl_manager.extract(os.path.join(panx_dl_dir, lang + ".tar.gz"))
728 return [
729 nlp.SplitGenerator(
/usr/local/lib/python3.6/dist-packages/nlp/utils/download_manager.py in extract(self, path_or_paths)
196 """
197 return map_nested(
--> 198 lambda path: cached_path(path, extract_compressed_file=True, force_extract=False), path_or_paths,
199 )
200
/usr/local/lib/python3.6/dist-packages/nlp/utils/py_utils.py in map_nested(function, data_struct, dict_only, map_tuple)
170 return tuple(mapped)
171 # Singleton
--> 172 return function(data_struct)
173
174
/usr/local/lib/python3.6/dist-packages/nlp/utils/download_manager.py in <lambda>(path)
196 """
197 return map_nested(
--> 198 lambda path: cached_path(path, extract_compressed_file=True, force_extract=False), path_or_paths,
199 )
200
/usr/local/lib/python3.6/dist-packages/nlp/utils/file_utils.py in cached_path(url_or_filename, download_config, **download_kwargs)
203 elif urlparse(url_or_filename).scheme == "":
204 # File, but it doesn't exist.
--> 205 raise FileNotFoundError("Local file {} doesn't exist".format(url_or_filename))
206 else:
207 # Something unknown
FileNotFoundError: Local file /root/.cache/huggingface/datasets/9b8c4f1578e45cb2539332c79738beb3b54afbcd842b079cabfd79e3ed6704f6/fr.tar.gz doesn't exist
```
## OS and hardware
```
- `nlp` version: 0.3.0
- Platform: Linux-4.15.0-72-generic-x86_64-with-Ubuntu-18.04-bionic
- Python version: 3.6.9
- PyTorch version (GPU?): 1.4.0 (True)
- Tensorflow version (GPU?): 2.1.0 (True)
- Using GPU in script?: <fill in>
- Using distributed or parallel set-up in script?: <fill in>
``` | 17 | Unable to load XTREME dataset from disk
Hi π€ team!
## Description of the problem
Following the [docs](https://huggingface.co/nlp/loading_datasets.html?highlight=xtreme#manually-downloading-files) I'm trying to load the `PAN-X.fr` dataset from the [XTREME](https://github.com/google-research/xtreme) benchmark.
I have manually downloaded the `AmazonPhotos.zip` file from [here](https://www.amazon.com/clouddrive/share/d3KGCRCIYwhKJF0H3eWA26hjg2ZCRhjpEQtDL70FSBN?_encoding=UTF8&%2AVersion%2A=1&%2Aentries%2A=0&mgh=1) and am running into a `FileNotFoundError` when I point to the location of the dataset.
As far as I can tell, the problem is that `AmazonPhotos.zip` decompresses to `panx_dataset` and `load_dataset()` is not looking in the correct path:
```
# path where load_dataset is looking for fr.tar.gz
/root/.cache/huggingface/datasets/9b8c4f1578e45cb2539332c79738beb3b54afbcd842b079cabfd79e3ed6704f6/
# path where it actually exists
/root/.cache/huggingface/datasets/9b8c4f1578e45cb2539332c79738beb3b54afbcd842b079cabfd79e3ed6704f6/panx_dataset/
```
## Steps to reproduce the problem
1. Manually download the XTREME benchmark from [here](https://www.amazon.com/clouddrive/share/d3KGCRCIYwhKJF0H3eWA26hjg2ZCRhjpEQtDL70FSBN?_encoding=UTF8&%2AVersion%2A=1&%2Aentries%2A=0&mgh=1)
2. Run the following code snippet
```python
from nlp import load_dataset
# AmazonPhotos.zip is in the root of the folder
dataset = load_dataset("xtreme", "PAN-X.fr", data_dir='./')
```
3. Here is the stack trace
```
---------------------------------------------------------------------------
FileNotFoundError Traceback (most recent call last)
<ipython-input-4-26786bb5fa93> in <module>
----> 1 dataset = load_dataset("xtreme", "PAN-X.fr", data_dir='./')
/usr/local/lib/python3.6/dist-packages/nlp/load.py in load_dataset(path, name, version, data_dir, data_files, split, cache_dir, download_config, download_mode, ignore_verifications, save_infos, **config_kwargs)
522 download_mode=download_mode,
523 ignore_verifications=ignore_verifications,
--> 524 save_infos=save_infos,
525 )
526
/usr/local/lib/python3.6/dist-packages/nlp/builder.py in download_and_prepare(self, download_config, download_mode, ignore_verifications, save_infos, try_from_hf_gcs, dl_manager, **download_and_prepare_kwargs)
430 verify_infos = not save_infos and not ignore_verifications
431 self._download_and_prepare(
--> 432 dl_manager=dl_manager, verify_infos=verify_infos, **download_and_prepare_kwargs
433 )
434 # Sync info
/usr/local/lib/python3.6/dist-packages/nlp/builder.py in _download_and_prepare(self, dl_manager, verify_infos, **prepare_split_kwargs)
464 split_dict = SplitDict(dataset_name=self.name)
465 split_generators_kwargs = self._make_split_generators_kwargs(prepare_split_kwargs)
--> 466 split_generators = self._split_generators(dl_manager, **split_generators_kwargs)
467 # Checksums verification
468 if verify_infos:
/usr/local/lib/python3.6/dist-packages/nlp/datasets/xtreme/b8c2ed3583a7a7ac60b503576dfed3271ac86757628897e945bd329c43b8a746/xtreme.py in _split_generators(self, dl_manager)
725 panx_dl_dir = dl_manager.extract(panx_path)
726 lang = self.config.name.split(".")[1]
--> 727 lang_folder = dl_manager.extract(os.path.join(panx_dl_dir, lang + ".tar.gz"))
728 return [
729 nlp.SplitGenerator(
/usr/local/lib/python3.6/dist-packages/nlp/utils/download_manager.py in extract(self, path_or_paths)
196 """
197 return map_nested(
--> 198 lambda path: cached_path(path, extract_compressed_file=True, force_extract=False), path_or_paths,
199 )
200
/usr/local/lib/python3.6/dist-packages/nlp/utils/py_utils.py in map_nested(function, data_struct, dict_only, map_tuple)
170 return tuple(mapped)
171 # Singleton
--> 172 return function(data_struct)
173
174
/usr/local/lib/python3.6/dist-packages/nlp/utils/download_manager.py in <lambda>(path)
196 """
197 return map_nested(
--> 198 lambda path: cached_path(path, extract_compressed_file=True, force_extract=False), path_or_paths,
199 )
200
/usr/local/lib/python3.6/dist-packages/nlp/utils/file_utils.py in cached_path(url_or_filename, download_config, **download_kwargs)
203 elif urlparse(url_or_filename).scheme == "":
204 # File, but it doesn't exist.
--> 205 raise FileNotFoundError("Local file {} doesn't exist".format(url_or_filename))
206 else:
207 # Something unknown
FileNotFoundError: Local file /root/.cache/huggingface/datasets/9b8c4f1578e45cb2539332c79738beb3b54afbcd842b079cabfd79e3ed6704f6/fr.tar.gz doesn't exist
```
## OS and hardware
```
- `nlp` version: 0.3.0
- Platform: Linux-4.15.0-72-generic-x86_64-with-Ubuntu-18.04-bionic
- Python version: 3.6.9
- PyTorch version (GPU?): 1.4.0 (True)
- Tensorflow version (GPU?): 2.1.0 (True)
- Using GPU in script?: <fill in>
- Using distributed or parallel set-up in script?: <fill in>
```
I was able to repro. Opening a PR to fix that.
Thanks for reporting this issue ! |
https://github.com/huggingface/datasets/issues/407 | MissingBeamOptions for Wikipedia 20200501.en | Fixed. Could you try again @mitchellgordon95 ?
It was due a file not being updated on S3.
We need to make sure all the datasets scripts get updated properly @julien-c | There may or may not be a regression for the pre-processed Wikipedia dataset. This was working fine 10 commits ago (without having Apache Beam available):
```
nlp.load_dataset('wikipedia', "20200501.en", split='train')
```
And now, having pulled master, I get:
```
Downloading and preparing dataset wikipedia/20200501.en (download: 16.99 GiB, generated: 17.07 GiB, total: 34.06 GiB) to /home/hltcoe/mgordon/.cache/huggingface/datasets/wikipedia/20200501.en/1.0.0/76b0b2747b679bb0ee7a1621e50e5a6378477add0c662668a324a5bc07d516dd...
Traceback (most recent call last):
File "scripts/download.py", line 11, in <module>
fire.Fire(download_pretrain)
File "/home/hltcoe/mgordon/.conda/envs/huggingface/lib/python3.6/site-packages/fire/core.py", line 138, in Fire
component_trace = _Fire(component, args, parsed_flag_args, context, name)
File "/home/hltcoe/mgordon/.conda/envs/huggingface/lib/python3.6/site-packages/fire/core.py", line 468, in _Fire
target=component.__name__)
File "/home/hltcoe/mgordon/.conda/envs/huggingface/lib/python3.6/site-packages/fire/core.py", line 672, in _CallAndUpdateTrace
component = fn(*varargs, **kwargs)
File "scripts/download.py", line 6, in download_pretrain
nlp.load_dataset('wikipedia', "20200501.en", split='train')
File "/exp/mgordon/nlp/src/nlp/load.py", line 534, in load_dataset
save_infos=save_infos,
File "/exp/mgordon/nlp/src/nlp/builder.py", line 460, in download_and_prepare
dl_manager=dl_manager, verify_infos=verify_infos, **download_and_prepare_kwargs
File "/exp/mgordon/nlp/src/nlp/builder.py", line 870, in _download_and_prepare
"\n\t`{}`".format(usage_example)
nlp.builder.MissingBeamOptions: Trying to generate a dataset using Apache Beam, yet no Beam Runner or PipelineOptions() has been provided in `load_dataset` or in the builder arguments. For big datasets it has to run on large-scale data processing tools like Dataflow, S
park, etc. More information about Apache Beam runners at https://beam.apache.org/documentation/runners/capability-matrix/
If you really want to run it locally because you feel like the Dataset is small enough, you can use the local beam runner called `DirectRunner` (you may run out of memory).
Example of usage:
`load_dataset('wikipedia', '20200501.en', beam_runner='DirectRunner')`
``` | 30 | MissingBeamOptions for Wikipedia 20200501.en
There may or may not be a regression for the pre-processed Wikipedia dataset. This was working fine 10 commits ago (without having Apache Beam available):
```
nlp.load_dataset('wikipedia', "20200501.en", split='train')
```
And now, having pulled master, I get:
```
Downloading and preparing dataset wikipedia/20200501.en (download: 16.99 GiB, generated: 17.07 GiB, total: 34.06 GiB) to /home/hltcoe/mgordon/.cache/huggingface/datasets/wikipedia/20200501.en/1.0.0/76b0b2747b679bb0ee7a1621e50e5a6378477add0c662668a324a5bc07d516dd...
Traceback (most recent call last):
File "scripts/download.py", line 11, in <module>
fire.Fire(download_pretrain)
File "/home/hltcoe/mgordon/.conda/envs/huggingface/lib/python3.6/site-packages/fire/core.py", line 138, in Fire
component_trace = _Fire(component, args, parsed_flag_args, context, name)
File "/home/hltcoe/mgordon/.conda/envs/huggingface/lib/python3.6/site-packages/fire/core.py", line 468, in _Fire
target=component.__name__)
File "/home/hltcoe/mgordon/.conda/envs/huggingface/lib/python3.6/site-packages/fire/core.py", line 672, in _CallAndUpdateTrace
component = fn(*varargs, **kwargs)
File "scripts/download.py", line 6, in download_pretrain
nlp.load_dataset('wikipedia', "20200501.en", split='train')
File "/exp/mgordon/nlp/src/nlp/load.py", line 534, in load_dataset
save_infos=save_infos,
File "/exp/mgordon/nlp/src/nlp/builder.py", line 460, in download_and_prepare
dl_manager=dl_manager, verify_infos=verify_infos, **download_and_prepare_kwargs
File "/exp/mgordon/nlp/src/nlp/builder.py", line 870, in _download_and_prepare
"\n\t`{}`".format(usage_example)
nlp.builder.MissingBeamOptions: Trying to generate a dataset using Apache Beam, yet no Beam Runner or PipelineOptions() has been provided in `load_dataset` or in the builder arguments. For big datasets it has to run on large-scale data processing tools like Dataflow, S
park, etc. More information about Apache Beam runners at https://beam.apache.org/documentation/runners/capability-matrix/
If you really want to run it locally because you feel like the Dataset is small enough, you can use the local beam runner called `DirectRunner` (you may run out of memory).
Example of usage:
`load_dataset('wikipedia', '20200501.en', beam_runner='DirectRunner')`
```
Fixed. Could you try again @mitchellgordon95 ?
It was due a file not being updated on S3.
We need to make sure all the datasets scripts get updated properly @julien-c |
https://github.com/huggingface/datasets/issues/407 | MissingBeamOptions for Wikipedia 20200501.en | I found the same issue with almost any language other than English. (For English, it works). Will someone need to update the file on S3 again? | There may or may not be a regression for the pre-processed Wikipedia dataset. This was working fine 10 commits ago (without having Apache Beam available):
```
nlp.load_dataset('wikipedia', "20200501.en", split='train')
```
And now, having pulled master, I get:
```
Downloading and preparing dataset wikipedia/20200501.en (download: 16.99 GiB, generated: 17.07 GiB, total: 34.06 GiB) to /home/hltcoe/mgordon/.cache/huggingface/datasets/wikipedia/20200501.en/1.0.0/76b0b2747b679bb0ee7a1621e50e5a6378477add0c662668a324a5bc07d516dd...
Traceback (most recent call last):
File "scripts/download.py", line 11, in <module>
fire.Fire(download_pretrain)
File "/home/hltcoe/mgordon/.conda/envs/huggingface/lib/python3.6/site-packages/fire/core.py", line 138, in Fire
component_trace = _Fire(component, args, parsed_flag_args, context, name)
File "/home/hltcoe/mgordon/.conda/envs/huggingface/lib/python3.6/site-packages/fire/core.py", line 468, in _Fire
target=component.__name__)
File "/home/hltcoe/mgordon/.conda/envs/huggingface/lib/python3.6/site-packages/fire/core.py", line 672, in _CallAndUpdateTrace
component = fn(*varargs, **kwargs)
File "scripts/download.py", line 6, in download_pretrain
nlp.load_dataset('wikipedia', "20200501.en", split='train')
File "/exp/mgordon/nlp/src/nlp/load.py", line 534, in load_dataset
save_infos=save_infos,
File "/exp/mgordon/nlp/src/nlp/builder.py", line 460, in download_and_prepare
dl_manager=dl_manager, verify_infos=verify_infos, **download_and_prepare_kwargs
File "/exp/mgordon/nlp/src/nlp/builder.py", line 870, in _download_and_prepare
"\n\t`{}`".format(usage_example)
nlp.builder.MissingBeamOptions: Trying to generate a dataset using Apache Beam, yet no Beam Runner or PipelineOptions() has been provided in `load_dataset` or in the builder arguments. For big datasets it has to run on large-scale data processing tools like Dataflow, S
park, etc. More information about Apache Beam runners at https://beam.apache.org/documentation/runners/capability-matrix/
If you really want to run it locally because you feel like the Dataset is small enough, you can use the local beam runner called `DirectRunner` (you may run out of memory).
Example of usage:
`load_dataset('wikipedia', '20200501.en', beam_runner='DirectRunner')`
``` | 26 | MissingBeamOptions for Wikipedia 20200501.en
There may or may not be a regression for the pre-processed Wikipedia dataset. This was working fine 10 commits ago (without having Apache Beam available):
```
nlp.load_dataset('wikipedia', "20200501.en", split='train')
```
And now, having pulled master, I get:
```
Downloading and preparing dataset wikipedia/20200501.en (download: 16.99 GiB, generated: 17.07 GiB, total: 34.06 GiB) to /home/hltcoe/mgordon/.cache/huggingface/datasets/wikipedia/20200501.en/1.0.0/76b0b2747b679bb0ee7a1621e50e5a6378477add0c662668a324a5bc07d516dd...
Traceback (most recent call last):
File "scripts/download.py", line 11, in <module>
fire.Fire(download_pretrain)
File "/home/hltcoe/mgordon/.conda/envs/huggingface/lib/python3.6/site-packages/fire/core.py", line 138, in Fire
component_trace = _Fire(component, args, parsed_flag_args, context, name)
File "/home/hltcoe/mgordon/.conda/envs/huggingface/lib/python3.6/site-packages/fire/core.py", line 468, in _Fire
target=component.__name__)
File "/home/hltcoe/mgordon/.conda/envs/huggingface/lib/python3.6/site-packages/fire/core.py", line 672, in _CallAndUpdateTrace
component = fn(*varargs, **kwargs)
File "scripts/download.py", line 6, in download_pretrain
nlp.load_dataset('wikipedia', "20200501.en", split='train')
File "/exp/mgordon/nlp/src/nlp/load.py", line 534, in load_dataset
save_infos=save_infos,
File "/exp/mgordon/nlp/src/nlp/builder.py", line 460, in download_and_prepare
dl_manager=dl_manager, verify_infos=verify_infos, **download_and_prepare_kwargs
File "/exp/mgordon/nlp/src/nlp/builder.py", line 870, in _download_and_prepare
"\n\t`{}`".format(usage_example)
nlp.builder.MissingBeamOptions: Trying to generate a dataset using Apache Beam, yet no Beam Runner or PipelineOptions() has been provided in `load_dataset` or in the builder arguments. For big datasets it has to run on large-scale data processing tools like Dataflow, S
park, etc. More information about Apache Beam runners at https://beam.apache.org/documentation/runners/capability-matrix/
If you really want to run it locally because you feel like the Dataset is small enough, you can use the local beam runner called `DirectRunner` (you may run out of memory).
Example of usage:
`load_dataset('wikipedia', '20200501.en', beam_runner='DirectRunner')`
```
I found the same issue with almost any language other than English. (For English, it works). Will someone need to update the file on S3 again? |
https://github.com/huggingface/datasets/issues/407 | MissingBeamOptions for Wikipedia 20200501.en | This is because only some languages are already preprocessed (en, de, fr, it) and stored on our google storage.
We plan to have a systematic way to preprocess more wikipedia languages in the future.
For the other languages you have to process them on your side using apache beam. That's why the lib asks for a Beam runner. | There may or may not be a regression for the pre-processed Wikipedia dataset. This was working fine 10 commits ago (without having Apache Beam available):
```
nlp.load_dataset('wikipedia', "20200501.en", split='train')
```
And now, having pulled master, I get:
```
Downloading and preparing dataset wikipedia/20200501.en (download: 16.99 GiB, generated: 17.07 GiB, total: 34.06 GiB) to /home/hltcoe/mgordon/.cache/huggingface/datasets/wikipedia/20200501.en/1.0.0/76b0b2747b679bb0ee7a1621e50e5a6378477add0c662668a324a5bc07d516dd...
Traceback (most recent call last):
File "scripts/download.py", line 11, in <module>
fire.Fire(download_pretrain)
File "/home/hltcoe/mgordon/.conda/envs/huggingface/lib/python3.6/site-packages/fire/core.py", line 138, in Fire
component_trace = _Fire(component, args, parsed_flag_args, context, name)
File "/home/hltcoe/mgordon/.conda/envs/huggingface/lib/python3.6/site-packages/fire/core.py", line 468, in _Fire
target=component.__name__)
File "/home/hltcoe/mgordon/.conda/envs/huggingface/lib/python3.6/site-packages/fire/core.py", line 672, in _CallAndUpdateTrace
component = fn(*varargs, **kwargs)
File "scripts/download.py", line 6, in download_pretrain
nlp.load_dataset('wikipedia', "20200501.en", split='train')
File "/exp/mgordon/nlp/src/nlp/load.py", line 534, in load_dataset
save_infos=save_infos,
File "/exp/mgordon/nlp/src/nlp/builder.py", line 460, in download_and_prepare
dl_manager=dl_manager, verify_infos=verify_infos, **download_and_prepare_kwargs
File "/exp/mgordon/nlp/src/nlp/builder.py", line 870, in _download_and_prepare
"\n\t`{}`".format(usage_example)
nlp.builder.MissingBeamOptions: Trying to generate a dataset using Apache Beam, yet no Beam Runner or PipelineOptions() has been provided in `load_dataset` or in the builder arguments. For big datasets it has to run on large-scale data processing tools like Dataflow, S
park, etc. More information about Apache Beam runners at https://beam.apache.org/documentation/runners/capability-matrix/
If you really want to run it locally because you feel like the Dataset is small enough, you can use the local beam runner called `DirectRunner` (you may run out of memory).
Example of usage:
`load_dataset('wikipedia', '20200501.en', beam_runner='DirectRunner')`
``` | 58 | MissingBeamOptions for Wikipedia 20200501.en
There may or may not be a regression for the pre-processed Wikipedia dataset. This was working fine 10 commits ago (without having Apache Beam available):
```
nlp.load_dataset('wikipedia', "20200501.en", split='train')
```
And now, having pulled master, I get:
```
Downloading and preparing dataset wikipedia/20200501.en (download: 16.99 GiB, generated: 17.07 GiB, total: 34.06 GiB) to /home/hltcoe/mgordon/.cache/huggingface/datasets/wikipedia/20200501.en/1.0.0/76b0b2747b679bb0ee7a1621e50e5a6378477add0c662668a324a5bc07d516dd...
Traceback (most recent call last):
File "scripts/download.py", line 11, in <module>
fire.Fire(download_pretrain)
File "/home/hltcoe/mgordon/.conda/envs/huggingface/lib/python3.6/site-packages/fire/core.py", line 138, in Fire
component_trace = _Fire(component, args, parsed_flag_args, context, name)
File "/home/hltcoe/mgordon/.conda/envs/huggingface/lib/python3.6/site-packages/fire/core.py", line 468, in _Fire
target=component.__name__)
File "/home/hltcoe/mgordon/.conda/envs/huggingface/lib/python3.6/site-packages/fire/core.py", line 672, in _CallAndUpdateTrace
component = fn(*varargs, **kwargs)
File "scripts/download.py", line 6, in download_pretrain
nlp.load_dataset('wikipedia', "20200501.en", split='train')
File "/exp/mgordon/nlp/src/nlp/load.py", line 534, in load_dataset
save_infos=save_infos,
File "/exp/mgordon/nlp/src/nlp/builder.py", line 460, in download_and_prepare
dl_manager=dl_manager, verify_infos=verify_infos, **download_and_prepare_kwargs
File "/exp/mgordon/nlp/src/nlp/builder.py", line 870, in _download_and_prepare
"\n\t`{}`".format(usage_example)
nlp.builder.MissingBeamOptions: Trying to generate a dataset using Apache Beam, yet no Beam Runner or PipelineOptions() has been provided in `load_dataset` or in the builder arguments. For big datasets it has to run on large-scale data processing tools like Dataflow, S
park, etc. More information about Apache Beam runners at https://beam.apache.org/documentation/runners/capability-matrix/
If you really want to run it locally because you feel like the Dataset is small enough, you can use the local beam runner called `DirectRunner` (you may run out of memory).
Example of usage:
`load_dataset('wikipedia', '20200501.en', beam_runner='DirectRunner')`
```
This is because only some languages are already preprocessed (en, de, fr, it) and stored on our google storage.
We plan to have a systematic way to preprocess more wikipedia languages in the future.
For the other languages you have to process them on your side using apache beam. That's why the lib asks for a Beam runner. |
https://github.com/huggingface/datasets/issues/406 | Faster Shuffling? | I think the slowness here probably come from the fact that we are copying from and to python.
@lhoestq for all the `select`-based methods I think we should stay in Arrow format and update the writer so that it can accept Arrow tables or batches as well. What do you think? | Consider shuffling bookcorpus:
```
dataset = nlp.load_dataset('bookcorpus', split='train')
dataset.shuffle()
```
According to tqdm, this will take around 2.5 hours on my machine to complete (even with the faster version of select from #405). I've also tried with `keep_in_memory=True` and `writer_batch_size=1000`.
But I can also just write the lines to a text file:
```
batch_size = 100000
with open('tmp.txt', 'w+') as out_f:
for i in tqdm(range(0, len(dataset), batch_size)):
batch = dataset[i:i+batch_size]['text']
print("\n".join(batch), file=out_f)
```
Which completes in a couple minutes, followed by `shuf tmp.txt > tmp2.txt` which completes in under a minute. And finally,
```
dataset = nlp.load_dataset('text', data_files='tmp2.txt')
```
Which completes in under 10 minutes. I read up on Apache Arrow this morning, and it seems like the columnar data format is not especially well-suited to shuffling rows, since moving items around requires a lot of book-keeping.
Is shuffle inherently slow, or am I just using it wrong? And if it is slow, would it make sense to try converting the data to a row-based format on disk and then shuffling? (Instead of calling select with a random permutation, as is currently done.) | 51 | Faster Shuffling?
Consider shuffling bookcorpus:
```
dataset = nlp.load_dataset('bookcorpus', split='train')
dataset.shuffle()
```
According to tqdm, this will take around 2.5 hours on my machine to complete (even with the faster version of select from #405). I've also tried with `keep_in_memory=True` and `writer_batch_size=1000`.
But I can also just write the lines to a text file:
```
batch_size = 100000
with open('tmp.txt', 'w+') as out_f:
for i in tqdm(range(0, len(dataset), batch_size)):
batch = dataset[i:i+batch_size]['text']
print("\n".join(batch), file=out_f)
```
Which completes in a couple minutes, followed by `shuf tmp.txt > tmp2.txt` which completes in under a minute. And finally,
```
dataset = nlp.load_dataset('text', data_files='tmp2.txt')
```
Which completes in under 10 minutes. I read up on Apache Arrow this morning, and it seems like the columnar data format is not especially well-suited to shuffling rows, since moving items around requires a lot of book-keeping.
Is shuffle inherently slow, or am I just using it wrong? And if it is slow, would it make sense to try converting the data to a row-based format on disk and then shuffling? (Instead of calling select with a random permutation, as is currently done.)
I think the slowness here probably come from the fact that we are copying from and to python.
@lhoestq for all the `select`-based methods I think we should stay in Arrow format and update the writer so that it can accept Arrow tables or batches as well. What do you think? |
https://github.com/huggingface/datasets/issues/406 | Faster Shuffling? | > @lhoestq for all the `select`-based methods I think we should stay in Arrow format and update the writer so that it can accept Arrow tables or batches as well. What do you think?
I just tried with `writer.write_table` with tables of 1000 elements and it's slower that the solution in #405
On my side (select 10 000 examples):
- Original implementation: 12s
- Batched solution: 100ms
- solution using arrow tables: 350ms
I'll try with arrays and record batches to see if we can make it work. | Consider shuffling bookcorpus:
```
dataset = nlp.load_dataset('bookcorpus', split='train')
dataset.shuffle()
```
According to tqdm, this will take around 2.5 hours on my machine to complete (even with the faster version of select from #405). I've also tried with `keep_in_memory=True` and `writer_batch_size=1000`.
But I can also just write the lines to a text file:
```
batch_size = 100000
with open('tmp.txt', 'w+') as out_f:
for i in tqdm(range(0, len(dataset), batch_size)):
batch = dataset[i:i+batch_size]['text']
print("\n".join(batch), file=out_f)
```
Which completes in a couple minutes, followed by `shuf tmp.txt > tmp2.txt` which completes in under a minute. And finally,
```
dataset = nlp.load_dataset('text', data_files='tmp2.txt')
```
Which completes in under 10 minutes. I read up on Apache Arrow this morning, and it seems like the columnar data format is not especially well-suited to shuffling rows, since moving items around requires a lot of book-keeping.
Is shuffle inherently slow, or am I just using it wrong? And if it is slow, would it make sense to try converting the data to a row-based format on disk and then shuffling? (Instead of calling select with a random permutation, as is currently done.) | 88 | Faster Shuffling?
Consider shuffling bookcorpus:
```
dataset = nlp.load_dataset('bookcorpus', split='train')
dataset.shuffle()
```
According to tqdm, this will take around 2.5 hours on my machine to complete (even with the faster version of select from #405). I've also tried with `keep_in_memory=True` and `writer_batch_size=1000`.
But I can also just write the lines to a text file:
```
batch_size = 100000
with open('tmp.txt', 'w+') as out_f:
for i in tqdm(range(0, len(dataset), batch_size)):
batch = dataset[i:i+batch_size]['text']
print("\n".join(batch), file=out_f)
```
Which completes in a couple minutes, followed by `shuf tmp.txt > tmp2.txt` which completes in under a minute. And finally,
```
dataset = nlp.load_dataset('text', data_files='tmp2.txt')
```
Which completes in under 10 minutes. I read up on Apache Arrow this morning, and it seems like the columnar data format is not especially well-suited to shuffling rows, since moving items around requires a lot of book-keeping.
Is shuffle inherently slow, or am I just using it wrong? And if it is slow, would it make sense to try converting the data to a row-based format on disk and then shuffling? (Instead of calling select with a random permutation, as is currently done.)
> @lhoestq for all the `select`-based methods I think we should stay in Arrow format and update the writer so that it can accept Arrow tables or batches as well. What do you think?
I just tried with `writer.write_table` with tables of 1000 elements and it's slower that the solution in #405
On my side (select 10 000 examples):
- Original implementation: 12s
- Batched solution: 100ms
- solution using arrow tables: 350ms
I'll try with arrays and record batches to see if we can make it work. |
https://github.com/huggingface/datasets/issues/406 | Faster Shuffling? | I tried using `.take` from pyarrow recordbatches but it doesn't improve the speed that much:
```python
import nlp
import numpy as np
dset = nlp.Dataset.from_file("dummy_test_select.arrow") # dummy dataset with 100000 examples like {"a": "h"*512}
indices = np.random.randint(0, 100_000, 1000_000)
```
```python
%%time
batch_size = 10_000
writer = ArrowWriter(schema=dset.schema, path="dummy_path",
writer_batch_size=1000, disable_nullable=False)
for i in tqdm(range(0, len(indices), batch_size)):
table = pa.concat_tables(dset._data.slice(int(i), 1) for i in indices[i : min(len(indices), i + batch_size)])
batch = table.to_pydict()
writer.write_batch(batch)
writer.finalize()
# 9.12s
```
```python
%%time
batch_size = 10_000
writer = ArrowWriter(schema=dset.schema, path="dummy_path",
writer_batch_size=1000, disable_nullable=False)
for i in tqdm(range(0, len(indices), batch_size)):
batch_indices = indices[i : min(len(indices), i + batch_size)]
# First, extract only the indices that we need with a mask
mask = [False] * len(dset)
for k in batch_indices:
mask[k] = True
t_batch = dset._data.filter(pa.array(mask))
# Second, build the list of indices for the filtered table, and taking care of duplicates
rev_positions = {}
duplicates = 0
for i, j in enumerate(sorted(batch_indices)):
if j in rev_positions:
duplicates += 1
else:
rev_positions[j] = i - duplicates
rev_map = [rev_positions[j] for j in batch_indices]
# Third, use `.take` from the combined recordbatch
t_combined = t_batch.combine_chunks() # load in memory
recordbatch = t_combined.to_batches()[0]
table = pa.Table.from_arrays(
[recordbatch[c].take(pa.array(rev_map)) for c in range(len(dset._data.column_names))],
schema=writer.schema
)
writer.write_table(table)
writer.finalize()
# 3.2s
```
| Consider shuffling bookcorpus:
```
dataset = nlp.load_dataset('bookcorpus', split='train')
dataset.shuffle()
```
According to tqdm, this will take around 2.5 hours on my machine to complete (even with the faster version of select from #405). I've also tried with `keep_in_memory=True` and `writer_batch_size=1000`.
But I can also just write the lines to a text file:
```
batch_size = 100000
with open('tmp.txt', 'w+') as out_f:
for i in tqdm(range(0, len(dataset), batch_size)):
batch = dataset[i:i+batch_size]['text']
print("\n".join(batch), file=out_f)
```
Which completes in a couple minutes, followed by `shuf tmp.txt > tmp2.txt` which completes in under a minute. And finally,
```
dataset = nlp.load_dataset('text', data_files='tmp2.txt')
```
Which completes in under 10 minutes. I read up on Apache Arrow this morning, and it seems like the columnar data format is not especially well-suited to shuffling rows, since moving items around requires a lot of book-keeping.
Is shuffle inherently slow, or am I just using it wrong? And if it is slow, would it make sense to try converting the data to a row-based format on disk and then shuffling? (Instead of calling select with a random permutation, as is currently done.) | 210 | Faster Shuffling?
Consider shuffling bookcorpus:
```
dataset = nlp.load_dataset('bookcorpus', split='train')
dataset.shuffle()
```
According to tqdm, this will take around 2.5 hours on my machine to complete (even with the faster version of select from #405). I've also tried with `keep_in_memory=True` and `writer_batch_size=1000`.
But I can also just write the lines to a text file:
```
batch_size = 100000
with open('tmp.txt', 'w+') as out_f:
for i in tqdm(range(0, len(dataset), batch_size)):
batch = dataset[i:i+batch_size]['text']
print("\n".join(batch), file=out_f)
```
Which completes in a couple minutes, followed by `shuf tmp.txt > tmp2.txt` which completes in under a minute. And finally,
```
dataset = nlp.load_dataset('text', data_files='tmp2.txt')
```
Which completes in under 10 minutes. I read up on Apache Arrow this morning, and it seems like the columnar data format is not especially well-suited to shuffling rows, since moving items around requires a lot of book-keeping.
Is shuffle inherently slow, or am I just using it wrong? And if it is slow, would it make sense to try converting the data to a row-based format on disk and then shuffling? (Instead of calling select with a random permutation, as is currently done.)
I tried using `.take` from pyarrow recordbatches but it doesn't improve the speed that much:
```python
import nlp
import numpy as np
dset = nlp.Dataset.from_file("dummy_test_select.arrow") # dummy dataset with 100000 examples like {"a": "h"*512}
indices = np.random.randint(0, 100_000, 1000_000)
```
```python
%%time
batch_size = 10_000
writer = ArrowWriter(schema=dset.schema, path="dummy_path",
writer_batch_size=1000, disable_nullable=False)
for i in tqdm(range(0, len(indices), batch_size)):
table = pa.concat_tables(dset._data.slice(int(i), 1) for i in indices[i : min(len(indices), i + batch_size)])
batch = table.to_pydict()
writer.write_batch(batch)
writer.finalize()
# 9.12s
```
```python
%%time
batch_size = 10_000
writer = ArrowWriter(schema=dset.schema, path="dummy_path",
writer_batch_size=1000, disable_nullable=False)
for i in tqdm(range(0, len(indices), batch_size)):
batch_indices = indices[i : min(len(indices), i + batch_size)]
# First, extract only the indices that we need with a mask
mask = [False] * len(dset)
for k in batch_indices:
mask[k] = True
t_batch = dset._data.filter(pa.array(mask))
# Second, build the list of indices for the filtered table, and taking care of duplicates
rev_positions = {}
duplicates = 0
for i, j in enumerate(sorted(batch_indices)):
if j in rev_positions:
duplicates += 1
else:
rev_positions[j] = i - duplicates
rev_map = [rev_positions[j] for j in batch_indices]
# Third, use `.take` from the combined recordbatch
t_combined = t_batch.combine_chunks() # load in memory
recordbatch = t_combined.to_batches()[0]
table = pa.Table.from_arrays(
[recordbatch[c].take(pa.array(rev_map)) for c in range(len(dset._data.column_names))],
schema=writer.schema
)
writer.write_table(table)
writer.finalize()
# 3.2s
```
|
https://github.com/huggingface/datasets/issues/406 | Faster Shuffling? | Shuffling is now significantly faster thanks to #513
Feel free to play with it now :)
Closing this one, but feel free to re-open if you have other questions | Consider shuffling bookcorpus:
```
dataset = nlp.load_dataset('bookcorpus', split='train')
dataset.shuffle()
```
According to tqdm, this will take around 2.5 hours on my machine to complete (even with the faster version of select from #405). I've also tried with `keep_in_memory=True` and `writer_batch_size=1000`.
But I can also just write the lines to a text file:
```
batch_size = 100000
with open('tmp.txt', 'w+') as out_f:
for i in tqdm(range(0, len(dataset), batch_size)):
batch = dataset[i:i+batch_size]['text']
print("\n".join(batch), file=out_f)
```
Which completes in a couple minutes, followed by `shuf tmp.txt > tmp2.txt` which completes in under a minute. And finally,
```
dataset = nlp.load_dataset('text', data_files='tmp2.txt')
```
Which completes in under 10 minutes. I read up on Apache Arrow this morning, and it seems like the columnar data format is not especially well-suited to shuffling rows, since moving items around requires a lot of book-keeping.
Is shuffle inherently slow, or am I just using it wrong? And if it is slow, would it make sense to try converting the data to a row-based format on disk and then shuffling? (Instead of calling select with a random permutation, as is currently done.) | 29 | Faster Shuffling?
Consider shuffling bookcorpus:
```
dataset = nlp.load_dataset('bookcorpus', split='train')
dataset.shuffle()
```
According to tqdm, this will take around 2.5 hours on my machine to complete (even with the faster version of select from #405). I've also tried with `keep_in_memory=True` and `writer_batch_size=1000`.
But I can also just write the lines to a text file:
```
batch_size = 100000
with open('tmp.txt', 'w+') as out_f:
for i in tqdm(range(0, len(dataset), batch_size)):
batch = dataset[i:i+batch_size]['text']
print("\n".join(batch), file=out_f)
```
Which completes in a couple minutes, followed by `shuf tmp.txt > tmp2.txt` which completes in under a minute. And finally,
```
dataset = nlp.load_dataset('text', data_files='tmp2.txt')
```
Which completes in under 10 minutes. I read up on Apache Arrow this morning, and it seems like the columnar data format is not especially well-suited to shuffling rows, since moving items around requires a lot of book-keeping.
Is shuffle inherently slow, or am I just using it wrong? And if it is slow, would it make sense to try converting the data to a row-based format on disk and then shuffling? (Instead of calling select with a random permutation, as is currently done.)
Shuffling is now significantly faster thanks to #513
Feel free to play with it now :)
Closing this one, but feel free to re-open if you have other questions |
https://github.com/huggingface/datasets/issues/406 | Faster Shuffling? | > Shuffling is now significantly faster thanks to #513 Feel free to play with it now :)
>
> Closing this one, but feel free to re-open if you have other questions
I have a similar issue. My code is
```
for batch_num in range(num_batches):
print(f'--> {batch_num=}\n') if verbose else None
# - Get batch
shuffled_dataset = dataset.shuffle(buffer_size=buffer_size, seed=seed)
raw_text_batch = shuffled_dataset.take(batch_size)
tokenized_batch = map(raw_text_batch)
if verbose:
time_start = time.time()
print(f'{raw_text_batch=}')
print(f'{tokenized_batch=}')
print(f'{next(iter(raw_text_batch))=}')
print(f'{next(iter(tokenized_batch))=}')
print(f'Time it took: {time.time() - time_start} seconds \a\n')
```
without the suffle it takes 4.5 secs with it takes 87.1 secs. Is this difference expected? my dataset version is:
```
(beyond_scale) brando9@ampere1:~/beyond-scale-language-data-diversity$ pip list | grep dataset
datasets 2.14.3
```
@lhoestq thoughts?
| Consider shuffling bookcorpus:
```
dataset = nlp.load_dataset('bookcorpus', split='train')
dataset.shuffle()
```
According to tqdm, this will take around 2.5 hours on my machine to complete (even with the faster version of select from #405). I've also tried with `keep_in_memory=True` and `writer_batch_size=1000`.
But I can also just write the lines to a text file:
```
batch_size = 100000
with open('tmp.txt', 'w+') as out_f:
for i in tqdm(range(0, len(dataset), batch_size)):
batch = dataset[i:i+batch_size]['text']
print("\n".join(batch), file=out_f)
```
Which completes in a couple minutes, followed by `shuf tmp.txt > tmp2.txt` which completes in under a minute. And finally,
```
dataset = nlp.load_dataset('text', data_files='tmp2.txt')
```
Which completes in under 10 minutes. I read up on Apache Arrow this morning, and it seems like the columnar data format is not especially well-suited to shuffling rows, since moving items around requires a lot of book-keeping.
Is shuffle inherently slow, or am I just using it wrong? And if it is slow, would it make sense to try converting the data to a row-based format on disk and then shuffling? (Instead of calling select with a random permutation, as is currently done.) | 116 | Faster Shuffling?
Consider shuffling bookcorpus:
```
dataset = nlp.load_dataset('bookcorpus', split='train')
dataset.shuffle()
```
According to tqdm, this will take around 2.5 hours on my machine to complete (even with the faster version of select from #405). I've also tried with `keep_in_memory=True` and `writer_batch_size=1000`.
But I can also just write the lines to a text file:
```
batch_size = 100000
with open('tmp.txt', 'w+') as out_f:
for i in tqdm(range(0, len(dataset), batch_size)):
batch = dataset[i:i+batch_size]['text']
print("\n".join(batch), file=out_f)
```
Which completes in a couple minutes, followed by `shuf tmp.txt > tmp2.txt` which completes in under a minute. And finally,
```
dataset = nlp.load_dataset('text', data_files='tmp2.txt')
```
Which completes in under 10 minutes. I read up on Apache Arrow this morning, and it seems like the columnar data format is not especially well-suited to shuffling rows, since moving items around requires a lot of book-keeping.
Is shuffle inherently slow, or am I just using it wrong? And if it is slow, would it make sense to try converting the data to a row-based format on disk and then shuffling? (Instead of calling select with a random permutation, as is currently done.)
> Shuffling is now significantly faster thanks to #513 Feel free to play with it now :)
>
> Closing this one, but feel free to re-open if you have other questions
I have a similar issue. My code is
```
for batch_num in range(num_batches):
print(f'--> {batch_num=}\n') if verbose else None
# - Get batch
shuffled_dataset = dataset.shuffle(buffer_size=buffer_size, seed=seed)
raw_text_batch = shuffled_dataset.take(batch_size)
tokenized_batch = map(raw_text_batch)
if verbose:
time_start = time.time()
print(f'{raw_text_batch=}')
print(f'{tokenized_batch=}')
print(f'{next(iter(raw_text_batch))=}')
print(f'{next(iter(tokenized_batch))=}')
print(f'Time it took: {time.time() - time_start} seconds \a\n')
```
without the suffle it takes 4.5 secs with it takes 87.1 secs. Is this difference expected? my dataset version is:
```
(beyond_scale) brando9@ampere1:~/beyond-scale-language-data-diversity$ pip list | grep dataset
datasets 2.14.3
```
@lhoestq thoughts?
|
https://github.com/huggingface/datasets/issues/406 | Faster Shuffling? | still slow even with update to `2.14.4` most recent as of this writing
```
Time it took: 4.301205635070801 seconds
--> batch_num=0
raw_text_batch=<datasets.iterable_dataset.IterableDataset object at 0x7f1fea7c2a40>
tokenized_batch=<datasets.iterable_dataset.IterableDataset object at 0x7f1fea7c2f20>
next(iter(raw_text_batch))={'text': "No matter which style you choose, you can be sure of one thing: our quality and craftsmanship are the best in the business. It's who we are and what we believe in. And it's evident every day on the factory floor where our dedicated teams take great pride in every stitch.", 'timestamp': '2019-04-19T06:43:50Z', 'url': 'https://institchescustoms.com/katzkin.html'}
next(iter(tokenized_batch))={'input_ids': tensor([ 2949, 2300, 543, 3918, 345, 3853, 11, 345, 460, 307,
1654, 286, 530, 1517, 25, 674, 3081, 290, 5977, 49820,
389, 262, 1266, 287, 262, 1597, 13, 632, 338, 508,
356, 389, 290, 644, 356, 1975, 287, 13, 843, 340,
338, 10678, 790, 1110, 319, 262, 8860, 4314, 810, 674,
7256, 3466, 1011, 1049, 11293, 287, 790, 24695, 13, 50256,
50256, 50256, 50256, 50256, 50256, 50256, 50256, 50256, 50256, 50256,
50256, 50256, 50256, 50256, 50256, 50256, 50256, 50256, 50256, 50256,
50256, 50256, 50256, 50256, 50256, 50256, 50256, 50256, 50256, 50256,
50256, 50256, 50256, 50256, 50256, 50256, 50256, 50256, 50256, 50256,
50256, 50256, 50256, 50256, 50256, 50256, 50256, 50256, 50256, 50256,
50256, 50256, 50256, 50256, 50256, 50256, 50256, 50256, 50256, 50256,
50256, 50256, 50256, 50256, 50256, 50256, 50256, 50256]), 'attention_mask': tensor([1, 1, 1, 1, 1, 1, 1, 1, 1, 1, 1, 1, 1, 1, 1, 1, 1, 1, 1, 1, 1, 1, 1, 1,
1, 1, 1, 1, 1, 1, 1, 1, 1, 1, 1, 1, 1, 1, 1, 1, 1, 1, 1, 1, 1, 1, 1, 1,
1, 1, 1, 1, 1, 1, 1, 1, 1, 1, 1, 0, 0, 0, 0, 0, 0, 0, 0, 0, 0, 0, 0, 0,
0, 0, 0, 0, 0, 0, 0, 0, 0, 0, 0, 0, 0, 0, 0, 0, 0, 0, 0, 0, 0, 0, 0, 0,
0, 0, 0, 0, 0, 0, 0, 0, 0, 0, 0, 0, 0, 0, 0, 0, 0, 0, 0, 0, 0, 0, 0, 0,
0, 0, 0, 0, 0, 0, 0, 0])}
Time it took: 102.99258613586426 seconds
``` | Consider shuffling bookcorpus:
```
dataset = nlp.load_dataset('bookcorpus', split='train')
dataset.shuffle()
```
According to tqdm, this will take around 2.5 hours on my machine to complete (even with the faster version of select from #405). I've also tried with `keep_in_memory=True` and `writer_batch_size=1000`.
But I can also just write the lines to a text file:
```
batch_size = 100000
with open('tmp.txt', 'w+') as out_f:
for i in tqdm(range(0, len(dataset), batch_size)):
batch = dataset[i:i+batch_size]['text']
print("\n".join(batch), file=out_f)
```
Which completes in a couple minutes, followed by `shuf tmp.txt > tmp2.txt` which completes in under a minute. And finally,
```
dataset = nlp.load_dataset('text', data_files='tmp2.txt')
```
Which completes in under 10 minutes. I read up on Apache Arrow this morning, and it seems like the columnar data format is not especially well-suited to shuffling rows, since moving items around requires a lot of book-keeping.
Is shuffle inherently slow, or am I just using it wrong? And if it is slow, would it make sense to try converting the data to a row-based format on disk and then shuffling? (Instead of calling select with a random permutation, as is currently done.) | 350 | Faster Shuffling?
Consider shuffling bookcorpus:
```
dataset = nlp.load_dataset('bookcorpus', split='train')
dataset.shuffle()
```
According to tqdm, this will take around 2.5 hours on my machine to complete (even with the faster version of select from #405). I've also tried with `keep_in_memory=True` and `writer_batch_size=1000`.
But I can also just write the lines to a text file:
```
batch_size = 100000
with open('tmp.txt', 'w+') as out_f:
for i in tqdm(range(0, len(dataset), batch_size)):
batch = dataset[i:i+batch_size]['text']
print("\n".join(batch), file=out_f)
```
Which completes in a couple minutes, followed by `shuf tmp.txt > tmp2.txt` which completes in under a minute. And finally,
```
dataset = nlp.load_dataset('text', data_files='tmp2.txt')
```
Which completes in under 10 minutes. I read up on Apache Arrow this morning, and it seems like the columnar data format is not especially well-suited to shuffling rows, since moving items around requires a lot of book-keeping.
Is shuffle inherently slow, or am I just using it wrong? And if it is slow, would it make sense to try converting the data to a row-based format on disk and then shuffling? (Instead of calling select with a random permutation, as is currently done.)
still slow even with update to `2.14.4` most recent as of this writing
```
Time it took: 4.301205635070801 seconds
--> batch_num=0
raw_text_batch=<datasets.iterable_dataset.IterableDataset object at 0x7f1fea7c2a40>
tokenized_batch=<datasets.iterable_dataset.IterableDataset object at 0x7f1fea7c2f20>
next(iter(raw_text_batch))={'text': "No matter which style you choose, you can be sure of one thing: our quality and craftsmanship are the best in the business. It's who we are and what we believe in. And it's evident every day on the factory floor where our dedicated teams take great pride in every stitch.", 'timestamp': '2019-04-19T06:43:50Z', 'url': 'https://institchescustoms.com/katzkin.html'}
next(iter(tokenized_batch))={'input_ids': tensor([ 2949, 2300, 543, 3918, 345, 3853, 11, 345, 460, 307,
1654, 286, 530, 1517, 25, 674, 3081, 290, 5977, 49820,
389, 262, 1266, 287, 262, 1597, 13, 632, 338, 508,
356, 389, 290, 644, 356, 1975, 287, 13, 843, 340,
338, 10678, 790, 1110, 319, 262, 8860, 4314, 810, 674,
7256, 3466, 1011, 1049, 11293, 287, 790, 24695, 13, 50256,
50256, 50256, 50256, 50256, 50256, 50256, 50256, 50256, 50256, 50256,
50256, 50256, 50256, 50256, 50256, 50256, 50256, 50256, 50256, 50256,
50256, 50256, 50256, 50256, 50256, 50256, 50256, 50256, 50256, 50256,
50256, 50256, 50256, 50256, 50256, 50256, 50256, 50256, 50256, 50256,
50256, 50256, 50256, 50256, 50256, 50256, 50256, 50256, 50256, 50256,
50256, 50256, 50256, 50256, 50256, 50256, 50256, 50256, 50256, 50256,
50256, 50256, 50256, 50256, 50256, 50256, 50256, 50256]), 'attention_mask': tensor([1, 1, 1, 1, 1, 1, 1, 1, 1, 1, 1, 1, 1, 1, 1, 1, 1, 1, 1, 1, 1, 1, 1, 1,
1, 1, 1, 1, 1, 1, 1, 1, 1, 1, 1, 1, 1, 1, 1, 1, 1, 1, 1, 1, 1, 1, 1, 1,
1, 1, 1, 1, 1, 1, 1, 1, 1, 1, 1, 0, 0, 0, 0, 0, 0, 0, 0, 0, 0, 0, 0, 0,
0, 0, 0, 0, 0, 0, 0, 0, 0, 0, 0, 0, 0, 0, 0, 0, 0, 0, 0, 0, 0, 0, 0, 0,
0, 0, 0, 0, 0, 0, 0, 0, 0, 0, 0, 0, 0, 0, 0, 0, 0, 0, 0, 0, 0, 0, 0, 0,
0, 0, 0, 0, 0, 0, 0, 0])}
Time it took: 102.99258613586426 seconds
``` |
https://github.com/huggingface/datasets/issues/406 | Faster Shuffling? | Shuffling leads to doing random access in many different locations on disk which is slower than reading contiguous data.
There is a super fast approximate shuffling algorithm implemented for iterable datasets though:
```python
iterable_dataset = dataset.to_iterable_dataset(num_shards=1024)
shuffled_dataset = iterable_dataset.shuffle(buffer_size=1000)
```
(the first batch might be a bit slow to get because the algorithm first fills a buffer before returning the first batch, see the [docs](https://huggingface.co/docs/datasets/v2.14.4/en/stream#shuffle) for more info) | Consider shuffling bookcorpus:
```
dataset = nlp.load_dataset('bookcorpus', split='train')
dataset.shuffle()
```
According to tqdm, this will take around 2.5 hours on my machine to complete (even with the faster version of select from #405). I've also tried with `keep_in_memory=True` and `writer_batch_size=1000`.
But I can also just write the lines to a text file:
```
batch_size = 100000
with open('tmp.txt', 'w+') as out_f:
for i in tqdm(range(0, len(dataset), batch_size)):
batch = dataset[i:i+batch_size]['text']
print("\n".join(batch), file=out_f)
```
Which completes in a couple minutes, followed by `shuf tmp.txt > tmp2.txt` which completes in under a minute. And finally,
```
dataset = nlp.load_dataset('text', data_files='tmp2.txt')
```
Which completes in under 10 minutes. I read up on Apache Arrow this morning, and it seems like the columnar data format is not especially well-suited to shuffling rows, since moving items around requires a lot of book-keeping.
Is shuffle inherently slow, or am I just using it wrong? And if it is slow, would it make sense to try converting the data to a row-based format on disk and then shuffling? (Instead of calling select with a random permutation, as is currently done.) | 68 | Faster Shuffling?
Consider shuffling bookcorpus:
```
dataset = nlp.load_dataset('bookcorpus', split='train')
dataset.shuffle()
```
According to tqdm, this will take around 2.5 hours on my machine to complete (even with the faster version of select from #405). I've also tried with `keep_in_memory=True` and `writer_batch_size=1000`.
But I can also just write the lines to a text file:
```
batch_size = 100000
with open('tmp.txt', 'w+') as out_f:
for i in tqdm(range(0, len(dataset), batch_size)):
batch = dataset[i:i+batch_size]['text']
print("\n".join(batch), file=out_f)
```
Which completes in a couple minutes, followed by `shuf tmp.txt > tmp2.txt` which completes in under a minute. And finally,
```
dataset = nlp.load_dataset('text', data_files='tmp2.txt')
```
Which completes in under 10 minutes. I read up on Apache Arrow this morning, and it seems like the columnar data format is not especially well-suited to shuffling rows, since moving items around requires a lot of book-keeping.
Is shuffle inherently slow, or am I just using it wrong? And if it is slow, would it make sense to try converting the data to a row-based format on disk and then shuffling? (Instead of calling select with a random permutation, as is currently done.)
Shuffling leads to doing random access in many different locations on disk which is slower than reading contiguous data.
There is a super fast approximate shuffling algorithm implemented for iterable datasets though:
```python
iterable_dataset = dataset.to_iterable_dataset(num_shards=1024)
shuffled_dataset = iterable_dataset.shuffle(buffer_size=1000)
```
(the first batch might be a bit slow to get because the algorithm first fills a buffer before returning the first batch, see the [docs](https://huggingface.co/docs/datasets/v2.14.4/en/stream#shuffle) for more info) |
https://github.com/huggingface/datasets/issues/388 | π [Dataset] Cannot download wmt14, wmt15 and wmt17 | similar slow download speed here for nlp.load_dataset('wmt14', 'fr-en')
`
Downloading: 100%|ββββββββββββββββββββββββββββββββββββββββββββββββββββββββββ| 658M/658M [1:00:42<00:00, 181kB/s]
Downloading: 100%|ββββββββββββββββββββββββββββββββββββββββββββββββββββββββββ| 918M/918M [1:39:38<00:00, 154kB/s]
Downloading: 2%|β | 40.9M/2.37G [04:48<5:03:06, 128kB/s]
`
Could we just download a specific subdataset in 'wmt14', such as 'newstest14'? | 1. I try downloading `wmt14`, `wmt15`, `wmt17`, `wmt19` with the following code:
```
nlp.load_dataset('wmt14','de-en')
nlp.load_dataset('wmt15','de-en')
nlp.load_dataset('wmt17','de-en')
nlp.load_dataset('wmt19','de-en')
```
The code runs but the download speed is **extremely slow**, the same behaviour is not observed on `wmt16` and `wmt18`
2. When trying to download `wmt17 zh-en`, I got the following error:
> ConnectionError: Couldn't reach https://storage.googleapis.com/tfdataset-data/downloadataset/uncorpus/UNv1.0.en-zh.tar.gz | 38 | π [Dataset] Cannot download wmt14, wmt15 and wmt17
1. I try downloading `wmt14`, `wmt15`, `wmt17`, `wmt19` with the following code:
```
nlp.load_dataset('wmt14','de-en')
nlp.load_dataset('wmt15','de-en')
nlp.load_dataset('wmt17','de-en')
nlp.load_dataset('wmt19','de-en')
```
The code runs but the download speed is **extremely slow**, the same behaviour is not observed on `wmt16` and `wmt18`
2. When trying to download `wmt17 zh-en`, I got the following error:
> ConnectionError: Couldn't reach https://storage.googleapis.com/tfdataset-data/downloadataset/uncorpus/UNv1.0.en-zh.tar.gz
similar slow download speed here for nlp.load_dataset('wmt14', 'fr-en')
`
Downloading: 100%|ββββββββββββββββββββββββββββββββββββββββββββββββββββββββββ| 658M/658M [1:00:42<00:00, 181kB/s]
Downloading: 100%|ββββββββββββββββββββββββββββββββββββββββββββββββββββββββββ| 918M/918M [1:39:38<00:00, 154kB/s]
Downloading: 2%|β | 40.9M/2.37G [04:48<5:03:06, 128kB/s]
`
Could we just download a specific subdataset in 'wmt14', such as 'newstest14'? |
https://github.com/huggingface/datasets/issues/388 | π [Dataset] Cannot download wmt14, wmt15 and wmt17 | > The code runs but the download speed is extremely slow, the same behaviour is not observed on wmt16 and wmt18
The original source for the files may provide slow download speeds.
We can probably host these files ourselves.
> When trying to download wmt17 zh-en, I got the following error:
> ConnectionError: Couldn't reach https://storage.googleapis.com/tfdataset-data/downloadataset/uncorpus/UNv1.0.en-zh.tar.gz
Looks like the file`UNv1.0.en-zh.tar.gz` is missing, or the url changed. We need to fix that
> Could we just download a specific subdataset in 'wmt14', such as 'newstest14'?
Right now I don't think it's possible. Maybe @patrickvonplaten knows more about it
| 1. I try downloading `wmt14`, `wmt15`, `wmt17`, `wmt19` with the following code:
```
nlp.load_dataset('wmt14','de-en')
nlp.load_dataset('wmt15','de-en')
nlp.load_dataset('wmt17','de-en')
nlp.load_dataset('wmt19','de-en')
```
The code runs but the download speed is **extremely slow**, the same behaviour is not observed on `wmt16` and `wmt18`
2. When trying to download `wmt17 zh-en`, I got the following error:
> ConnectionError: Couldn't reach https://storage.googleapis.com/tfdataset-data/downloadataset/uncorpus/UNv1.0.en-zh.tar.gz | 97 | π [Dataset] Cannot download wmt14, wmt15 and wmt17
1. I try downloading `wmt14`, `wmt15`, `wmt17`, `wmt19` with the following code:
```
nlp.load_dataset('wmt14','de-en')
nlp.load_dataset('wmt15','de-en')
nlp.load_dataset('wmt17','de-en')
nlp.load_dataset('wmt19','de-en')
```
The code runs but the download speed is **extremely slow**, the same behaviour is not observed on `wmt16` and `wmt18`
2. When trying to download `wmt17 zh-en`, I got the following error:
> ConnectionError: Couldn't reach https://storage.googleapis.com/tfdataset-data/downloadataset/uncorpus/UNv1.0.en-zh.tar.gz
> The code runs but the download speed is extremely slow, the same behaviour is not observed on wmt16 and wmt18
The original source for the files may provide slow download speeds.
We can probably host these files ourselves.
> When trying to download wmt17 zh-en, I got the following error:
> ConnectionError: Couldn't reach https://storage.googleapis.com/tfdataset-data/downloadataset/uncorpus/UNv1.0.en-zh.tar.gz
Looks like the file`UNv1.0.en-zh.tar.gz` is missing, or the url changed. We need to fix that
> Could we just download a specific subdataset in 'wmt14', such as 'newstest14'?
Right now I don't think it's possible. Maybe @patrickvonplaten knows more about it
|
https://github.com/huggingface/datasets/issues/388 | π [Dataset] Cannot download wmt14, wmt15 and wmt17 | Yeah, the download speed is sadly always extremely slow :-/.
I will try to check out the `wmt17 zh-en` bug :-) | 1. I try downloading `wmt14`, `wmt15`, `wmt17`, `wmt19` with the following code:
```
nlp.load_dataset('wmt14','de-en')
nlp.load_dataset('wmt15','de-en')
nlp.load_dataset('wmt17','de-en')
nlp.load_dataset('wmt19','de-en')
```
The code runs but the download speed is **extremely slow**, the same behaviour is not observed on `wmt16` and `wmt18`
2. When trying to download `wmt17 zh-en`, I got the following error:
> ConnectionError: Couldn't reach https://storage.googleapis.com/tfdataset-data/downloadataset/uncorpus/UNv1.0.en-zh.tar.gz | 21 | π [Dataset] Cannot download wmt14, wmt15 and wmt17
1. I try downloading `wmt14`, `wmt15`, `wmt17`, `wmt19` with the following code:
```
nlp.load_dataset('wmt14','de-en')
nlp.load_dataset('wmt15','de-en')
nlp.load_dataset('wmt17','de-en')
nlp.load_dataset('wmt19','de-en')
```
The code runs but the download speed is **extremely slow**, the same behaviour is not observed on `wmt16` and `wmt18`
2. When trying to download `wmt17 zh-en`, I got the following error:
> ConnectionError: Couldn't reach https://storage.googleapis.com/tfdataset-data/downloadataset/uncorpus/UNv1.0.en-zh.tar.gz
Yeah, the download speed is sadly always extremely slow :-/.
I will try to check out the `wmt17 zh-en` bug :-) |
https://github.com/huggingface/datasets/issues/387 | Conversion through to_pandas output numpy arrays for lists instead of python objects | To convert from arrow type we have three options: to_numpy, to_pandas and to_pydict/to_pylist.
- to_numpy and to_pandas return numpy arrays instead of lists but are very fast.
- to_pydict/to_pylist can be 100x slower and become the bottleneck for reading data, but at least they return lists.
Maybe we can have to_pydict/to_pylist as the default and use to_numpy or to_pandas when the format (set by `set_format`) is 'numpy' or 'pandas' | In a related question, the conversion through to_pandas output numpy arrays for the lists instead of python objects.
Here is an example:
```python
>>> dataset._data.slice(key, 1).to_pandas().to_dict("list")
{'sentence1': ['Amrozi accused his brother , whom he called " the witness " , of deliberately distorting his evidence .'], 'sentence2': ['Referring to him as only " the witness " , Amrozi accused his brother of deliberately distorting his evidence .'], 'label': [1], 'idx': [0], 'input_ids': [array([ 101, 7277, 2180, 5303, 4806, 1117, 1711, 117, 2292,
1119, 1270, 107, 1103, 7737, 107, 117, 1104, 9938,
4267, 12223, 21811, 1117, 2554, 119, 102])], 'token_type_ids': [array([0, 0, 0, 0, 0, 0, 0, 0, 0, 0, 0, 0, 0, 0, 0, 0, 0, 0, 0, 0, 0, 0,
0, 0, 0])], 'attention_mask': [array([1, 1, 1, 1, 1, 1, 1, 1, 1, 1, 1, 1, 1, 1, 1, 1, 1, 1, 1, 1, 1, 1,
1, 1, 1])]}
>>> type(dataset._data.slice(key, 1).to_pandas().to_dict("list")['input_ids'][0])
<class 'numpy.ndarray'>
>>> dataset._data.slice(key, 1).to_pydict()
{'sentence1': ['Amrozi accused his brother , whom he called " the witness " , of deliberately distorting his evidence .'], 'sentence2': ['Referring to him as only " the witness " , Amrozi accused his brother of deliberately distorting his evidence .'], 'label': [1], 'idx': [0], 'input_ids': [[101, 7277, 2180, 5303, 4806, 1117, 1711, 117, 2292, 1119, 1270, 107, 1103, 7737, 107, 117, 1104, 9938, 4267, 12223, 21811, 1117, 2554, 119, 102]], 'token_type_ids': [[0, 0, 0, 0, 0, 0, 0, 0, 0, 0, 0, 0, 0, 0, 0, 0, 0, 0, 0, 0, 0, 0, 0, 0, 0]], 'attention_mask': [[1, 1, 1, 1, 1, 1, 1, 1, 1, 1, 1, 1, 1, 1, 1, 1, 1, 1, 1, 1, 1, 1, 1, 1, 1]]}
``` | 69 | Conversion through to_pandas output numpy arrays for lists instead of python objects
In a related question, the conversion through to_pandas output numpy arrays for the lists instead of python objects.
Here is an example:
```python
>>> dataset._data.slice(key, 1).to_pandas().to_dict("list")
{'sentence1': ['Amrozi accused his brother , whom he called " the witness " , of deliberately distorting his evidence .'], 'sentence2': ['Referring to him as only " the witness " , Amrozi accused his brother of deliberately distorting his evidence .'], 'label': [1], 'idx': [0], 'input_ids': [array([ 101, 7277, 2180, 5303, 4806, 1117, 1711, 117, 2292,
1119, 1270, 107, 1103, 7737, 107, 117, 1104, 9938,
4267, 12223, 21811, 1117, 2554, 119, 102])], 'token_type_ids': [array([0, 0, 0, 0, 0, 0, 0, 0, 0, 0, 0, 0, 0, 0, 0, 0, 0, 0, 0, 0, 0, 0,
0, 0, 0])], 'attention_mask': [array([1, 1, 1, 1, 1, 1, 1, 1, 1, 1, 1, 1, 1, 1, 1, 1, 1, 1, 1, 1, 1, 1,
1, 1, 1])]}
>>> type(dataset._data.slice(key, 1).to_pandas().to_dict("list")['input_ids'][0])
<class 'numpy.ndarray'>
>>> dataset._data.slice(key, 1).to_pydict()
{'sentence1': ['Amrozi accused his brother , whom he called " the witness " , of deliberately distorting his evidence .'], 'sentence2': ['Referring to him as only " the witness " , Amrozi accused his brother of deliberately distorting his evidence .'], 'label': [1], 'idx': [0], 'input_ids': [[101, 7277, 2180, 5303, 4806, 1117, 1711, 117, 2292, 1119, 1270, 107, 1103, 7737, 107, 117, 1104, 9938, 4267, 12223, 21811, 1117, 2554, 119, 102]], 'token_type_ids': [[0, 0, 0, 0, 0, 0, 0, 0, 0, 0, 0, 0, 0, 0, 0, 0, 0, 0, 0, 0, 0, 0, 0, 0, 0]], 'attention_mask': [[1, 1, 1, 1, 1, 1, 1, 1, 1, 1, 1, 1, 1, 1, 1, 1, 1, 1, 1, 1, 1, 1, 1, 1, 1]]}
```
To convert from arrow type we have three options: to_numpy, to_pandas and to_pydict/to_pylist.
- to_numpy and to_pandas return numpy arrays instead of lists but are very fast.
- to_pydict/to_pylist can be 100x slower and become the bottleneck for reading data, but at least they return lists.
Maybe we can have to_pydict/to_pylist as the default and use to_numpy or to_pandas when the format (set by `set_format`) is 'numpy' or 'pandas' |
https://github.com/huggingface/datasets/issues/378 | [dataset] Structure of MLQA seems unecessary nested | Same for the RACE dataset: https://github.com/huggingface/nlp/blob/master/datasets/race/race.py
Should we scan all the datasets to remove this pattern of un-necessary nesting? | The features of the MLQA dataset comprise several nested dictionaries with a single element inside (for `questions` and `ids`): https://github.com/huggingface/nlp/blob/master/datasets/mlqa/mlqa.py#L90-L97
Should we keep this @mariamabarham @patrickvonplaten? Was this added for compatibility with tfds?
```python
features=nlp.Features(
{
"context": nlp.Value("string"),
"questions": nlp.features.Sequence({"question": nlp.Value("string")}),
"answers": nlp.features.Sequence(
{"text": nlp.Value("string"), "answer_start": nlp.Value("int32"),}
),
"ids": nlp.features.Sequence({"idx": nlp.Value("string")})
``` | 19 | [dataset] Structure of MLQA seems unecessary nested
The features of the MLQA dataset comprise several nested dictionaries with a single element inside (for `questions` and `ids`): https://github.com/huggingface/nlp/blob/master/datasets/mlqa/mlqa.py#L90-L97
Should we keep this @mariamabarham @patrickvonplaten? Was this added for compatibility with tfds?
```python
features=nlp.Features(
{
"context": nlp.Value("string"),
"questions": nlp.features.Sequence({"question": nlp.Value("string")}),
"answers": nlp.features.Sequence(
{"text": nlp.Value("string"), "answer_start": nlp.Value("int32"),}
),
"ids": nlp.features.Sequence({"idx": nlp.Value("string")})
```
Same for the RACE dataset: https://github.com/huggingface/nlp/blob/master/datasets/race/race.py
Should we scan all the datasets to remove this pattern of un-necessary nesting? |
https://github.com/huggingface/datasets/issues/376 | to_pandas conversion doesn't always work | Could you try to update pyarrow to >=0.17.0 ? It should fix the `to_pandas` bug
Also I'm not sure that structures like list<struct> are fully supported in the lib (none of the datasets use that).
It can cause issues when using dataset transforms like `filter` for example | For some complex nested types, the conversion from Arrow to python dict through pandas doesn't seem to be possible.
Here is an example using the official SQUAD v2 JSON file.
This example was found while investigating #373.
```python
>>> squad = load_dataset('json', data_files={nlp.Split.TRAIN: ["./train-v2.0.json"]}, download_mode=nlp.GenerateMode.FORCE_REDOWNLOAD, version="1.0.0", field='data')
>>> squad['train']
Dataset(schema: {'title': 'string', 'paragraphs': 'list<item: struct<qas: list<item: struct<question: string, id: string, answers: list<item: struct<text: string, answer_start: int64>>, is_impossible: bool, plausible_answers: list<item: struct<text: string, answer_start: int64>>>>, context: string>>'}, num_rows: 442)
>>> squad['train'][0]
Traceback (most recent call last):
File "<stdin>", line 1, in <module>
File "/Users/thomwolf/Documents/GitHub/datasets/src/nlp/arrow_dataset.py", line 589, in __getitem__
format_kwargs=self._format_kwargs,
File "/Users/thomwolf/Documents/GitHub/datasets/src/nlp/arrow_dataset.py", line 529, in _getitem
outputs = self._unnest(self._data.slice(key, 1).to_pandas().to_dict("list"))
File "pyarrow/array.pxi", line 559, in pyarrow.lib._PandasConvertible.to_pandas
File "pyarrow/table.pxi", line 1367, in pyarrow.lib.Table._to_pandas
File "/Users/thomwolf/miniconda2/envs/datasets/lib/python3.7/site-packages/pyarrow/pandas_compat.py", line 766, in table_to_blockmanager
blocks = _table_to_blocks(options, table, categories, ext_columns_dtypes)
File "/Users/thomwolf/miniconda2/envs/datasets/lib/python3.7/site-packages/pyarrow/pandas_compat.py", line 1101, in _table_to_blocks
list(extension_columns.keys()))
File "pyarrow/table.pxi", line 881, in pyarrow.lib.table_to_blocks
File "pyarrow/error.pxi", line 105, in pyarrow.lib.check_status
pyarrow.lib.ArrowNotImplementedError: Not implemented type for Arrow list to pandas: struct<qas: list<item: struct<question: string, id: string, answers: list<item: struct<text: string, answer_start: int64>>, is_impossible: bool, plausible_answers: list<item: struct<text: string, answer_start: int64>>>>, context: string>
```
cc @lhoestq would we have a way to detect this from the schema maybe?
Here is the schema for this pretty complex JSON:
```python
>>> squad['train'].schema
title: string
paragraphs: list<item: struct<qas: list<item: struct<question: string, id: string, answers: list<item: struct<text: string, answer_start: int64>>, is_impossible: bool, plausible_answers: list<item: struct<text: string, answer_start: int64>>>>, context: string>>
child 0, item: struct<qas: list<item: struct<question: string, id: string, answers: list<item: struct<text: string, answer_start: int64>>, is_impossible: bool, plausible_answers: list<item: struct<text: string, answer_start: int64>>>>, context: string>
child 0, qas: list<item: struct<question: string, id: string, answers: list<item: struct<text: string, answer_start: int64>>, is_impossible: bool, plausible_answers: list<item: struct<text: string, answer_start: int64>>>>
child 0, item: struct<question: string, id: string, answers: list<item: struct<text: string, answer_start: int64>>, is_impossible: bool, plausible_answers: list<item: struct<text: string, answer_start: int64>>>
child 0, question: string
child 1, id: string
child 2, answers: list<item: struct<text: string, answer_start: int64>>
child 0, item: struct<text: string, answer_start: int64>
child 0, text: string
child 1, answer_start: int64
child 3, is_impossible: bool
child 4, plausible_answers: list<item: struct<text: string, answer_start: int64>>
child 0, item: struct<text: string, answer_start: int64>
child 0, text: string
child 1, answer_start: int64
child 1, context: string
``` | 47 | to_pandas conversion doesn't always work
For some complex nested types, the conversion from Arrow to python dict through pandas doesn't seem to be possible.
Here is an example using the official SQUAD v2 JSON file.
This example was found while investigating #373.
```python
>>> squad = load_dataset('json', data_files={nlp.Split.TRAIN: ["./train-v2.0.json"]}, download_mode=nlp.GenerateMode.FORCE_REDOWNLOAD, version="1.0.0", field='data')
>>> squad['train']
Dataset(schema: {'title': 'string', 'paragraphs': 'list<item: struct<qas: list<item: struct<question: string, id: string, answers: list<item: struct<text: string, answer_start: int64>>, is_impossible: bool, plausible_answers: list<item: struct<text: string, answer_start: int64>>>>, context: string>>'}, num_rows: 442)
>>> squad['train'][0]
Traceback (most recent call last):
File "<stdin>", line 1, in <module>
File "/Users/thomwolf/Documents/GitHub/datasets/src/nlp/arrow_dataset.py", line 589, in __getitem__
format_kwargs=self._format_kwargs,
File "/Users/thomwolf/Documents/GitHub/datasets/src/nlp/arrow_dataset.py", line 529, in _getitem
outputs = self._unnest(self._data.slice(key, 1).to_pandas().to_dict("list"))
File "pyarrow/array.pxi", line 559, in pyarrow.lib._PandasConvertible.to_pandas
File "pyarrow/table.pxi", line 1367, in pyarrow.lib.Table._to_pandas
File "/Users/thomwolf/miniconda2/envs/datasets/lib/python3.7/site-packages/pyarrow/pandas_compat.py", line 766, in table_to_blockmanager
blocks = _table_to_blocks(options, table, categories, ext_columns_dtypes)
File "/Users/thomwolf/miniconda2/envs/datasets/lib/python3.7/site-packages/pyarrow/pandas_compat.py", line 1101, in _table_to_blocks
list(extension_columns.keys()))
File "pyarrow/table.pxi", line 881, in pyarrow.lib.table_to_blocks
File "pyarrow/error.pxi", line 105, in pyarrow.lib.check_status
pyarrow.lib.ArrowNotImplementedError: Not implemented type for Arrow list to pandas: struct<qas: list<item: struct<question: string, id: string, answers: list<item: struct<text: string, answer_start: int64>>, is_impossible: bool, plausible_answers: list<item: struct<text: string, answer_start: int64>>>>, context: string>
```
cc @lhoestq would we have a way to detect this from the schema maybe?
Here is the schema for this pretty complex JSON:
```python
>>> squad['train'].schema
title: string
paragraphs: list<item: struct<qas: list<item: struct<question: string, id: string, answers: list<item: struct<text: string, answer_start: int64>>, is_impossible: bool, plausible_answers: list<item: struct<text: string, answer_start: int64>>>>, context: string>>
child 0, item: struct<qas: list<item: struct<question: string, id: string, answers: list<item: struct<text: string, answer_start: int64>>, is_impossible: bool, plausible_answers: list<item: struct<text: string, answer_start: int64>>>>, context: string>
child 0, qas: list<item: struct<question: string, id: string, answers: list<item: struct<text: string, answer_start: int64>>, is_impossible: bool, plausible_answers: list<item: struct<text: string, answer_start: int64>>>>
child 0, item: struct<question: string, id: string, answers: list<item: struct<text: string, answer_start: int64>>, is_impossible: bool, plausible_answers: list<item: struct<text: string, answer_start: int64>>>
child 0, question: string
child 1, id: string
child 2, answers: list<item: struct<text: string, answer_start: int64>>
child 0, item: struct<text: string, answer_start: int64>
child 0, text: string
child 1, answer_start: int64
child 3, is_impossible: bool
child 4, plausible_answers: list<item: struct<text: string, answer_start: int64>>
child 0, item: struct<text: string, answer_start: int64>
child 0, text: string
child 1, answer_start: int64
child 1, context: string
```
Could you try to update pyarrow to >=0.17.0 ? It should fix the `to_pandas` bug
Also I'm not sure that structures like list<struct> are fully supported in the lib (none of the datasets use that).
It can cause issues when using dataset transforms like `filter` for example |
https://github.com/huggingface/datasets/issues/375 | TypeError when computing bertscore | I am not able to reproduce this issue on my side.
Could you give us more details about the inputs you used ?
I do get another error though:
```
~/.virtualenvs/hf-datasets/lib/python3.7/site-packages/bert_score/utils.py in bert_cos_score_idf(model, refs, hyps, tokenizer, idf_dict, verbose, batch_size, device, all_layers)
371 return sorted(list(set(l)), key=lambda x: len(x.split(" ")))
372
--> 373 sentences = dedup_and_sort(refs + hyps)
374 embs = []
375 iter_range = range(0, len(sentences), batch_size)
ValueError: operands could not be broadcast together with shapes (0,) (2,)
```
That's because it gets numpy arrays as input and not lists. See #387 | Hi,
I installed nlp 0.3.0 via pip, and my python version is 3.7.
When I tried to compute bertscore with the code:
```
import nlp
bertscore = nlp.load_metric('bertscore')
# load hyps and refs
...
print (bertscore.compute(hyps, refs, lang='en'))
```
I got the following error.
```
Traceback (most recent call last):
File "bert_score_evaluate.py", line 16, in <module>
print (bertscore.compute(hyps, refs, lang='en'))
File "/home/willywsm/anaconda3/envs/torcher/lib/python3.7/site-packages/nlp/metric.py", line 200, in compute
output = self._compute(predictions=predictions, references=references, **metrics_kwargs)
File "/home/willywsm/anaconda3/envs/torcher/lib/python3.7/site-packages/nlp/metrics/bertscore/fb176889831bf0ce995ed197edc94b2e9a83f647a869bb8c9477dbb2d04d0f08/bertscore.py", line 105, in _compute
hashcode = bert_score.utils.get_hash(model_type, num_layers, idf, rescale_with_baseline)
TypeError: get_hash() takes 3 positional arguments but 4 were given
```
It seems like there is something wrong with get_hash() function? | 91 | TypeError when computing bertscore
Hi,
I installed nlp 0.3.0 via pip, and my python version is 3.7.
When I tried to compute bertscore with the code:
```
import nlp
bertscore = nlp.load_metric('bertscore')
# load hyps and refs
...
print (bertscore.compute(hyps, refs, lang='en'))
```
I got the following error.
```
Traceback (most recent call last):
File "bert_score_evaluate.py", line 16, in <module>
print (bertscore.compute(hyps, refs, lang='en'))
File "/home/willywsm/anaconda3/envs/torcher/lib/python3.7/site-packages/nlp/metric.py", line 200, in compute
output = self._compute(predictions=predictions, references=references, **metrics_kwargs)
File "/home/willywsm/anaconda3/envs/torcher/lib/python3.7/site-packages/nlp/metrics/bertscore/fb176889831bf0ce995ed197edc94b2e9a83f647a869bb8c9477dbb2d04d0f08/bertscore.py", line 105, in _compute
hashcode = bert_score.utils.get_hash(model_type, num_layers, idf, rescale_with_baseline)
TypeError: get_hash() takes 3 positional arguments but 4 were given
```
It seems like there is something wrong with get_hash() function?
I am not able to reproduce this issue on my side.
Could you give us more details about the inputs you used ?
I do get another error though:
```
~/.virtualenvs/hf-datasets/lib/python3.7/site-packages/bert_score/utils.py in bert_cos_score_idf(model, refs, hyps, tokenizer, idf_dict, verbose, batch_size, device, all_layers)
371 return sorted(list(set(l)), key=lambda x: len(x.split(" ")))
372
--> 373 sentences = dedup_and_sort(refs + hyps)
374 embs = []
375 iter_range = range(0, len(sentences), batch_size)
ValueError: operands could not be broadcast together with shapes (0,) (2,)
```
That's because it gets numpy arrays as input and not lists. See #387 |
https://github.com/huggingface/datasets/issues/373 | Segmentation fault when loading local JSON dataset as of #372 | I've seen this sort of thing before -- it might help to delete the directory -- I've also noticed that there is an error with the json Dataloader for any data I've tried to load. I've replaced it with this, which skips over the data feature population step:
```python
import os
import pyarrow.json as paj
import nlp as hf_nlp
from nlp import DatasetInfo, BuilderConfig, SplitGenerator, Split, utils
from nlp.arrow_writer import ArrowWriter
class JSONDatasetBuilder(hf_nlp.ArrowBasedBuilder):
BUILDER_CONFIG_CLASS = BuilderConfig
def _info(self):
return DatasetInfo()
def _split_generators(self, dl_manager):
""" We handle string, list and dicts in datafiles
"""
if isinstance(self.config.data_files, (str, list, tuple)):
files = self.config.data_files
if isinstance(files, str):
files = [files]
return [SplitGenerator(name=Split.TRAIN, gen_kwargs={"files": files})]
splits = []
for split_name in [Split.TRAIN, Split.VALIDATION, Split.TEST]:
if split_name in self.config.data_files:
files = self.config.data_files[split_name]
if isinstance(files, str):
files = [files]
splits.append(SplitGenerator(name=split_name, gen_kwargs={"files": files}))
return splits
def _prepare_split(self, split_generator):
fname = "{}-{}.arrow".format(self.name, split_generator.name)
fpath = os.path.join(self._cache_dir, fname)
writer = ArrowWriter(path=fpath)
generator = self._generate_tables(**split_generator.gen_kwargs)
for key, table in utils.tqdm(generator, unit=" tables", leave=False):
writer.write_table(table)
num_examples, num_bytes = writer.finalize()
split_generator.split_info.num_examples = num_examples
split_generator.split_info.num_bytes = num_bytes
def _generate_tables(self, files):
for i, file in enumerate(files):
pa_table = paj.read_json(
file
)
yield i, pa_table
``` | The last issue was closed (#369) once the #372 update was merged. However, I'm still not able to load a SQuAD formatted JSON file. Instead of the previously recorded pyarrow error, I now get a segmentation fault.
```
dataset = nlp.load_dataset('json', data_files={nlp.Split.TRAIN: ["./datasets/train-v2.0.json"]}, field='data')
```
causes
```
Using custom data configuration default
Downloading and preparing dataset json/default (download: Unknown size, generated: Unknown size, total: Unknown size) to /home/XXX/.cache/huggingface/datasets/json/default/0.0.0...
0 tables [00:00, ? tables/s]Segmentation fault (core dumped)
```
where `./datasets/train-v2.0.json` is downloaded directly from https://rajpurkar.github.io/SQuAD-explorer/.
This is consistent with other SQuAD-formatted JSON files.
When attempting to load the dataset again, I get the following:
```
Using custom data configuration default
Traceback (most recent call last):
File "dataloader.py", line 6, in <module>
'json', data_files={nlp.Split.TRAIN: ["./datasets/train-v2.0.json"]}, field='data')
File "/home/XXX/.conda/envs/torch/lib/python3.7/site-packages/nlp/load.py", line 524, in load_dataset
save_infos=save_infos,
File "/home/XXX/.conda/envs/torch/lib/python3.7/site-packages/nlp/builder.py", line 382, in download_and_prepare
with incomplete_dir(self._cache_dir) as tmp_data_dir:
File "/home/XXX/.conda/envs/torch/lib/python3.7/contextlib.py", line 112, in __enter__
return next(self.gen)
File "/home/XXX/.conda/envs/torch/lib/python3.7/site-packages/nlp/builder.py", line 368, in incomplete_dir
os.makedirs(tmp_dir)
File "/home/XXX/.conda/envs/torch/lib/python3.7/os.py", line 223, in makedirs
mkdir(name, mode)
FileExistsError: [Errno 17] File exists: '/home/XXX/.cache/huggingface/datasets/json/default/0.0.0.incomplete'
```
(Not sure if you wanted this in the previous issue #369 or not as it was closed.) | 191 | Segmentation fault when loading local JSON dataset as of #372
The last issue was closed (#369) once the #372 update was merged. However, I'm still not able to load a SQuAD formatted JSON file. Instead of the previously recorded pyarrow error, I now get a segmentation fault.
```
dataset = nlp.load_dataset('json', data_files={nlp.Split.TRAIN: ["./datasets/train-v2.0.json"]}, field='data')
```
causes
```
Using custom data configuration default
Downloading and preparing dataset json/default (download: Unknown size, generated: Unknown size, total: Unknown size) to /home/XXX/.cache/huggingface/datasets/json/default/0.0.0...
0 tables [00:00, ? tables/s]Segmentation fault (core dumped)
```
where `./datasets/train-v2.0.json` is downloaded directly from https://rajpurkar.github.io/SQuAD-explorer/.
This is consistent with other SQuAD-formatted JSON files.
When attempting to load the dataset again, I get the following:
```
Using custom data configuration default
Traceback (most recent call last):
File "dataloader.py", line 6, in <module>
'json', data_files={nlp.Split.TRAIN: ["./datasets/train-v2.0.json"]}, field='data')
File "/home/XXX/.conda/envs/torch/lib/python3.7/site-packages/nlp/load.py", line 524, in load_dataset
save_infos=save_infos,
File "/home/XXX/.conda/envs/torch/lib/python3.7/site-packages/nlp/builder.py", line 382, in download_and_prepare
with incomplete_dir(self._cache_dir) as tmp_data_dir:
File "/home/XXX/.conda/envs/torch/lib/python3.7/contextlib.py", line 112, in __enter__
return next(self.gen)
File "/home/XXX/.conda/envs/torch/lib/python3.7/site-packages/nlp/builder.py", line 368, in incomplete_dir
os.makedirs(tmp_dir)
File "/home/XXX/.conda/envs/torch/lib/python3.7/os.py", line 223, in makedirs
mkdir(name, mode)
FileExistsError: [Errno 17] File exists: '/home/XXX/.cache/huggingface/datasets/json/default/0.0.0.incomplete'
```
(Not sure if you wanted this in the previous issue #369 or not as it was closed.)
I've seen this sort of thing before -- it might help to delete the directory -- I've also noticed that there is an error with the json Dataloader for any data I've tried to load. I've replaced it with this, which skips over the data feature population step:
```python
import os
import pyarrow.json as paj
import nlp as hf_nlp
from nlp import DatasetInfo, BuilderConfig, SplitGenerator, Split, utils
from nlp.arrow_writer import ArrowWriter
class JSONDatasetBuilder(hf_nlp.ArrowBasedBuilder):
BUILDER_CONFIG_CLASS = BuilderConfig
def _info(self):
return DatasetInfo()
def _split_generators(self, dl_manager):
""" We handle string, list and dicts in datafiles
"""
if isinstance(self.config.data_files, (str, list, tuple)):
files = self.config.data_files
if isinstance(files, str):
files = [files]
return [SplitGenerator(name=Split.TRAIN, gen_kwargs={"files": files})]
splits = []
for split_name in [Split.TRAIN, Split.VALIDATION, Split.TEST]:
if split_name in self.config.data_files:
files = self.config.data_files[split_name]
if isinstance(files, str):
files = [files]
splits.append(SplitGenerator(name=split_name, gen_kwargs={"files": files}))
return splits
def _prepare_split(self, split_generator):
fname = "{}-{}.arrow".format(self.name, split_generator.name)
fpath = os.path.join(self._cache_dir, fname)
writer = ArrowWriter(path=fpath)
generator = self._generate_tables(**split_generator.gen_kwargs)
for key, table in utils.tqdm(generator, unit=" tables", leave=False):
writer.write_table(table)
num_examples, num_bytes = writer.finalize()
split_generator.split_info.num_examples = num_examples
split_generator.split_info.num_bytes = num_bytes
def _generate_tables(self, files):
for i, file in enumerate(files):
pa_table = paj.read_json(
file
)
yield i, pa_table
``` |
https://github.com/huggingface/datasets/issues/373 | Segmentation fault when loading local JSON dataset as of #372 | Yes, deleting the directory solves the error whenever I try to rerun.
By replacing the json-loader, you mean the cached file in my `site-packages` directory? e.g. `/home/XXX/.cache/lib/python3.7/site-packages/nlp/datasets/json/(...)/json.py`
When I was testing this out before the #372 PR was merged I had issues installing it properly locally. Since the `json.py` script was downloaded instead of actually using the one provided in the local install. Manually updating that file seemed to solve it, but it didn't seem like a proper solution. Especially when having to run this on a remote compute cluster with no access to that directory. | The last issue was closed (#369) once the #372 update was merged. However, I'm still not able to load a SQuAD formatted JSON file. Instead of the previously recorded pyarrow error, I now get a segmentation fault.
```
dataset = nlp.load_dataset('json', data_files={nlp.Split.TRAIN: ["./datasets/train-v2.0.json"]}, field='data')
```
causes
```
Using custom data configuration default
Downloading and preparing dataset json/default (download: Unknown size, generated: Unknown size, total: Unknown size) to /home/XXX/.cache/huggingface/datasets/json/default/0.0.0...
0 tables [00:00, ? tables/s]Segmentation fault (core dumped)
```
where `./datasets/train-v2.0.json` is downloaded directly from https://rajpurkar.github.io/SQuAD-explorer/.
This is consistent with other SQuAD-formatted JSON files.
When attempting to load the dataset again, I get the following:
```
Using custom data configuration default
Traceback (most recent call last):
File "dataloader.py", line 6, in <module>
'json', data_files={nlp.Split.TRAIN: ["./datasets/train-v2.0.json"]}, field='data')
File "/home/XXX/.conda/envs/torch/lib/python3.7/site-packages/nlp/load.py", line 524, in load_dataset
save_infos=save_infos,
File "/home/XXX/.conda/envs/torch/lib/python3.7/site-packages/nlp/builder.py", line 382, in download_and_prepare
with incomplete_dir(self._cache_dir) as tmp_data_dir:
File "/home/XXX/.conda/envs/torch/lib/python3.7/contextlib.py", line 112, in __enter__
return next(self.gen)
File "/home/XXX/.conda/envs/torch/lib/python3.7/site-packages/nlp/builder.py", line 368, in incomplete_dir
os.makedirs(tmp_dir)
File "/home/XXX/.conda/envs/torch/lib/python3.7/os.py", line 223, in makedirs
mkdir(name, mode)
FileExistsError: [Errno 17] File exists: '/home/XXX/.cache/huggingface/datasets/json/default/0.0.0.incomplete'
```
(Not sure if you wanted this in the previous issue #369 or not as it was closed.) | 96 | Segmentation fault when loading local JSON dataset as of #372
The last issue was closed (#369) once the #372 update was merged. However, I'm still not able to load a SQuAD formatted JSON file. Instead of the previously recorded pyarrow error, I now get a segmentation fault.
```
dataset = nlp.load_dataset('json', data_files={nlp.Split.TRAIN: ["./datasets/train-v2.0.json"]}, field='data')
```
causes
```
Using custom data configuration default
Downloading and preparing dataset json/default (download: Unknown size, generated: Unknown size, total: Unknown size) to /home/XXX/.cache/huggingface/datasets/json/default/0.0.0...
0 tables [00:00, ? tables/s]Segmentation fault (core dumped)
```
where `./datasets/train-v2.0.json` is downloaded directly from https://rajpurkar.github.io/SQuAD-explorer/.
This is consistent with other SQuAD-formatted JSON files.
When attempting to load the dataset again, I get the following:
```
Using custom data configuration default
Traceback (most recent call last):
File "dataloader.py", line 6, in <module>
'json', data_files={nlp.Split.TRAIN: ["./datasets/train-v2.0.json"]}, field='data')
File "/home/XXX/.conda/envs/torch/lib/python3.7/site-packages/nlp/load.py", line 524, in load_dataset
save_infos=save_infos,
File "/home/XXX/.conda/envs/torch/lib/python3.7/site-packages/nlp/builder.py", line 382, in download_and_prepare
with incomplete_dir(self._cache_dir) as tmp_data_dir:
File "/home/XXX/.conda/envs/torch/lib/python3.7/contextlib.py", line 112, in __enter__
return next(self.gen)
File "/home/XXX/.conda/envs/torch/lib/python3.7/site-packages/nlp/builder.py", line 368, in incomplete_dir
os.makedirs(tmp_dir)
File "/home/XXX/.conda/envs/torch/lib/python3.7/os.py", line 223, in makedirs
mkdir(name, mode)
FileExistsError: [Errno 17] File exists: '/home/XXX/.cache/huggingface/datasets/json/default/0.0.0.incomplete'
```
(Not sure if you wanted this in the previous issue #369 or not as it was closed.)
Yes, deleting the directory solves the error whenever I try to rerun.
By replacing the json-loader, you mean the cached file in my `site-packages` directory? e.g. `/home/XXX/.cache/lib/python3.7/site-packages/nlp/datasets/json/(...)/json.py`
When I was testing this out before the #372 PR was merged I had issues installing it properly locally. Since the `json.py` script was downloaded instead of actually using the one provided in the local install. Manually updating that file seemed to solve it, but it didn't seem like a proper solution. Especially when having to run this on a remote compute cluster with no access to that directory. |
https://github.com/huggingface/datasets/issues/373 | Segmentation fault when loading local JSON dataset as of #372 | I see, diving in the JSON file for SQuAD it's a pretty complex structure.
The best solution for you, if you have a dataset really similar to SQuAD would be to copy and modify the SQuAD data processing script. We will probably add soon an option to be able to specify file path to use instead of the automatic URL encoded in the script but in the meantime you can:
- copy the [squad script](https://github.com/huggingface/nlp/blob/master/datasets/squad/squad.py) in a new script for your dataset
- in the new script replace [these `urls_to_download `](https://github.com/huggingface/nlp/blob/master/datasets/squad/squad.py#L99-L102) by `urls_to_download=self.config.data_files`
- load the dataset with `dataset = load_dataset('path/to/your/new/script', data_files={nlp.Split.TRAIN: "./datasets/train-v2.0.json"})`
This way you can reuse all the processing logic of the SQuAD loading script. | The last issue was closed (#369) once the #372 update was merged. However, I'm still not able to load a SQuAD formatted JSON file. Instead of the previously recorded pyarrow error, I now get a segmentation fault.
```
dataset = nlp.load_dataset('json', data_files={nlp.Split.TRAIN: ["./datasets/train-v2.0.json"]}, field='data')
```
causes
```
Using custom data configuration default
Downloading and preparing dataset json/default (download: Unknown size, generated: Unknown size, total: Unknown size) to /home/XXX/.cache/huggingface/datasets/json/default/0.0.0...
0 tables [00:00, ? tables/s]Segmentation fault (core dumped)
```
where `./datasets/train-v2.0.json` is downloaded directly from https://rajpurkar.github.io/SQuAD-explorer/.
This is consistent with other SQuAD-formatted JSON files.
When attempting to load the dataset again, I get the following:
```
Using custom data configuration default
Traceback (most recent call last):
File "dataloader.py", line 6, in <module>
'json', data_files={nlp.Split.TRAIN: ["./datasets/train-v2.0.json"]}, field='data')
File "/home/XXX/.conda/envs/torch/lib/python3.7/site-packages/nlp/load.py", line 524, in load_dataset
save_infos=save_infos,
File "/home/XXX/.conda/envs/torch/lib/python3.7/site-packages/nlp/builder.py", line 382, in download_and_prepare
with incomplete_dir(self._cache_dir) as tmp_data_dir:
File "/home/XXX/.conda/envs/torch/lib/python3.7/contextlib.py", line 112, in __enter__
return next(self.gen)
File "/home/XXX/.conda/envs/torch/lib/python3.7/site-packages/nlp/builder.py", line 368, in incomplete_dir
os.makedirs(tmp_dir)
File "/home/XXX/.conda/envs/torch/lib/python3.7/os.py", line 223, in makedirs
mkdir(name, mode)
FileExistsError: [Errno 17] File exists: '/home/XXX/.cache/huggingface/datasets/json/default/0.0.0.incomplete'
```
(Not sure if you wanted this in the previous issue #369 or not as it was closed.) | 117 | Segmentation fault when loading local JSON dataset as of #372
The last issue was closed (#369) once the #372 update was merged. However, I'm still not able to load a SQuAD formatted JSON file. Instead of the previously recorded pyarrow error, I now get a segmentation fault.
```
dataset = nlp.load_dataset('json', data_files={nlp.Split.TRAIN: ["./datasets/train-v2.0.json"]}, field='data')
```
causes
```
Using custom data configuration default
Downloading and preparing dataset json/default (download: Unknown size, generated: Unknown size, total: Unknown size) to /home/XXX/.cache/huggingface/datasets/json/default/0.0.0...
0 tables [00:00, ? tables/s]Segmentation fault (core dumped)
```
where `./datasets/train-v2.0.json` is downloaded directly from https://rajpurkar.github.io/SQuAD-explorer/.
This is consistent with other SQuAD-formatted JSON files.
When attempting to load the dataset again, I get the following:
```
Using custom data configuration default
Traceback (most recent call last):
File "dataloader.py", line 6, in <module>
'json', data_files={nlp.Split.TRAIN: ["./datasets/train-v2.0.json"]}, field='data')
File "/home/XXX/.conda/envs/torch/lib/python3.7/site-packages/nlp/load.py", line 524, in load_dataset
save_infos=save_infos,
File "/home/XXX/.conda/envs/torch/lib/python3.7/site-packages/nlp/builder.py", line 382, in download_and_prepare
with incomplete_dir(self._cache_dir) as tmp_data_dir:
File "/home/XXX/.conda/envs/torch/lib/python3.7/contextlib.py", line 112, in __enter__
return next(self.gen)
File "/home/XXX/.conda/envs/torch/lib/python3.7/site-packages/nlp/builder.py", line 368, in incomplete_dir
os.makedirs(tmp_dir)
File "/home/XXX/.conda/envs/torch/lib/python3.7/os.py", line 223, in makedirs
mkdir(name, mode)
FileExistsError: [Errno 17] File exists: '/home/XXX/.cache/huggingface/datasets/json/default/0.0.0.incomplete'
```
(Not sure if you wanted this in the previous issue #369 or not as it was closed.)
I see, diving in the JSON file for SQuAD it's a pretty complex structure.
The best solution for you, if you have a dataset really similar to SQuAD would be to copy and modify the SQuAD data processing script. We will probably add soon an option to be able to specify file path to use instead of the automatic URL encoded in the script but in the meantime you can:
- copy the [squad script](https://github.com/huggingface/nlp/blob/master/datasets/squad/squad.py) in a new script for your dataset
- in the new script replace [these `urls_to_download `](https://github.com/huggingface/nlp/blob/master/datasets/squad/squad.py#L99-L102) by `urls_to_download=self.config.data_files`
- load the dataset with `dataset = load_dataset('path/to/your/new/script', data_files={nlp.Split.TRAIN: "./datasets/train-v2.0.json"})`
This way you can reuse all the processing logic of the SQuAD loading script. |