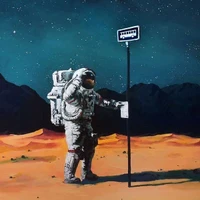
Mxode/SmolLM-Chinese-180M
Text Generation
•
Updated
•
40
max_stars_repo_path
stringlengths 3
269
| max_stars_repo_name
stringlengths 4
119
| max_stars_count
int64 0
191k
| id
stringlengths 1
7
| content
stringlengths 6
1.05M
| score
float64 0.23
5.13
| int_score
int64 0
5
|
---|---|---|---|---|---|---|
public_data/serializers.py | MTES-MCT/sparte | 0 | 0 | <reponame>MTES-MCT/sparte
from rest_framework_gis import serializers
from rest_framework import serializers as s
from .models import (
Artificialisee2015to2018,
Artificielle2018,
CommunesSybarval,
CouvertureSol,
EnveloppeUrbaine2018,
Ocsge,
Renaturee2018to2015,
Sybarval,
Voirie2018,
ZonesBaties2018,
UsageSol,
)
def get_label(code="", label=""):
if code is None:
code = "-"
if label is None:
label = "inconnu"
return f"{code} {label[:30]}"
class Artificialisee2015to2018Serializer(serializers.GeoFeatureModelSerializer):
usage_2015 = s.SerializerMethodField()
usage_2018 = s.SerializerMethodField()
couverture_2015 = s.SerializerMethodField()
couverture_2018 = s.SerializerMethodField()
def get_usage_2015(self, obj):
return get_label(code=obj.us_2015, label=obj.us_2015_label)
def get_usage_2018(self, obj):
return get_label(code=obj.us_2018, label=obj.us_2018_label)
def get_couverture_2015(self, obj):
return get_label(code=obj.cs_2015, label=obj.cs_2015_label)
def get_couverture_2018(self, obj):
return get_label(code=obj.cs_2018, label=obj.cs_2018_label)
class Meta:
fields = (
"id",
"surface",
"usage_2015",
"usage_2018",
"couverture_2015",
"couverture_2018",
)
geo_field = "mpoly"
model = Artificialisee2015to2018
class Artificielle2018Serializer(serializers.GeoFeatureModelSerializer):
couverture = s.SerializerMethodField()
def get_couverture(self, obj):
return get_label(code=obj.couverture, label=obj.couverture_label)
class Meta:
fields = (
"id",
"surface",
"couverture",
)
geo_field = "mpoly"
model = Artificielle2018
class CommunesSybarvalSerializer(serializers.GeoFeatureModelSerializer):
"""Marker GeoJSON serializer."""
class Meta:
"""Marker serializer meta class."""
fields = (
"nom",
"code_insee",
"surface",
)
geo_field = "mpoly"
model = CommunesSybarval
class EnveloppeUrbaine2018Serializer(serializers.GeoFeatureModelSerializer):
couverture = s.SerializerMethodField()
def get_couverture(self, obj):
return get_label(code=obj.couverture, label=obj.couverture_label)
class Meta:
fields = (
"id",
"couverture",
"surface",
)
geo_field = "mpoly"
model = EnveloppeUrbaine2018
class OcsgeSerializer(serializers.GeoFeatureModelSerializer):
couverture = s.SerializerMethodField()
usage = s.SerializerMethodField()
def get_couverture(self, obj):
return get_label(code=obj.couverture, label=obj.couverture_label)
def get_usage(self, obj):
return get_label(code=obj.usage, label=obj.usage_label)
class Meta:
fields = (
"id",
"couverture",
"usage",
"millesime",
"map_color",
"year",
)
geo_field = "mpoly"
model = Ocsge
class Renaturee2018to2015Serializer(serializers.GeoFeatureModelSerializer):
usage_2015 = s.SerializerMethodField()
usage_2018 = s.SerializerMethodField()
couverture_2015 = s.SerializerMethodField()
couverture_2018 = s.SerializerMethodField()
def get_usage_2015(self, obj):
return get_label(code=obj.us_2015, label=obj.us_2015_label)
def get_usage_2018(self, obj):
return get_label(code=obj.us_2018, label=obj.us_2018_label)
def get_couverture_2015(self, obj):
return get_label(code=obj.cs_2015, label=obj.cs_2015_label)
def get_couverture_2018(self, obj):
return get_label(code=obj.cs_2018, label=obj.cs_2018_label)
class Meta:
fields = (
"id",
"surface",
"usage_2015",
"usage_2018",
"couverture_2015",
"couverture_2018",
)
geo_field = "mpoly"
model = Renaturee2018to2015
class SybarvalSerializer(serializers.GeoFeatureModelSerializer):
class Meta:
fields = (
"id",
"surface",
)
geo_field = "mpoly"
model = Sybarval
class Voirie2018Serializer(serializers.GeoFeatureModelSerializer):
couverture = s.SerializerMethodField()
usage = s.SerializerMethodField()
def get_couverture(self, obj):
return get_label(code=obj.couverture, label=obj.couverture_label)
def get_usage(self, obj):
return get_label(code=obj.usage, label=obj.usage_label)
class Meta:
fields = (
"id",
"surface",
"couverture",
"usage",
)
geo_field = "mpoly"
model = Voirie2018
class ZonesBaties2018Serializer(serializers.GeoFeatureModelSerializer):
couverture = s.SerializerMethodField()
usage = s.SerializerMethodField()
def get_couverture(self, obj):
return get_label(code=obj.couverture, label=obj.couverture_label)
def get_usage(self, obj):
return get_label(code=obj.usage, label=obj.usage_label)
class Meta:
fields = (
"id",
"couverture",
"usage",
"surface",
)
geo_field = "mpoly"
model = ZonesBaties2018
class CouvertureSolSerializer(serializers.ModelSerializer):
class Meta:
fields = (
"id",
"parent",
"code",
"label",
"is_artificial",
)
model = CouvertureSol
class UsageSolSerializer(serializers.ModelSerializer):
class Meta:
fields = (
"id",
"parent",
"code",
"label",
)
model = UsageSol
| 2.015625 | 2 |
quick_search/admin.py | naman1901/django-quick-search | 0 | 1 | from django.contrib import admin
from .models import SearchResult
# Register your models here.
class SearchResultAdmin(admin.ModelAdmin):
fields = ["query", "heading", "url", "text"]
admin.site.register(SearchResult, SearchResultAdmin) | 1.640625 | 2 |
rasa/train.py | Amirali-Shirkh/rasa-for-botfront | 0 | 2 | import asyncio
import os
import tempfile
from contextlib import ExitStack
from typing import Text, Optional, List, Union, Dict
from rasa.importers.importer import TrainingDataImporter
from rasa import model
from rasa.model import FingerprintComparisonResult
from rasa.core.domain import Domain
from rasa.utils.common import TempDirectoryPath
from rasa.cli.utils import (
print_success,
print_warning,
print_error,
bcolors,
print_color,
)
from rasa.constants import DEFAULT_MODELS_PATH, DEFAULT_CORE_SUBDIRECTORY_NAME
def train(
domain: Text,
config: Text,
training_files: Union[Text, List[Text]],
output: Text = DEFAULT_MODELS_PATH,
force_training: bool = False,
fixed_model_name: Optional[Text] = None,
persist_nlu_training_data: bool = False,
additional_arguments: Optional[Dict] = None,
loop: Optional[asyncio.AbstractEventLoop] = None,
) -> Optional[Text]:
if loop is None:
try:
loop = asyncio.get_event_loop()
except RuntimeError:
loop = asyncio.new_event_loop()
asyncio.set_event_loop(loop)
return loop.run_until_complete(
train_async(
domain=domain,
config=config,
training_files=training_files,
output_path=output,
force_training=force_training,
fixed_model_name=fixed_model_name,
persist_nlu_training_data=persist_nlu_training_data,
additional_arguments=additional_arguments,
)
)
async def train_async(
domain: Union[Domain, Text],
config: Dict[Text, Text],
training_files: Optional[Union[Text, List[Text]]],
output_path: Text = DEFAULT_MODELS_PATH,
force_training: bool = False,
fixed_model_name: Optional[Text] = None,
persist_nlu_training_data: bool = False,
additional_arguments: Optional[Dict] = None,
) -> Optional[Text]:
"""Trains a Rasa model (Core and NLU).
Args:
domain: Path to the domain file.
config: Dict of paths to the config for Core and NLU. Keys are language codes
training_files: Paths to the training data for Core and NLU.
output_path: Output path.
force_training: If `True` retrain model even if data has not changed.
fixed_model_name: Name of model to be stored.
persist_nlu_training_data: `True` if the NLU training data should be persisted
with the model.
additional_arguments: Additional training parameters.
Returns:
Path of the trained model archive.
"""
# file_importer = TrainingDataImporter.load_from_config(
# config, domain, training_files
# )
with ExitStack() as stack:
train_path = stack.enter_context(TempDirectoryPath(tempfile.mkdtemp()))
# bf mod
from rasa_addons.importers import BotfrontFileImporter
file_importer = BotfrontFileImporter(config, domain, training_files)
# domain = await file_importer.get_domain()
# if domain.is_empty():
# return await handle_domain_if_not_exists(
# file_importer, output_path, fixed_model_name
# )
# /bf mod
return await _train_async_internal(
file_importer,
train_path,
output_path,
force_training,
fixed_model_name,
persist_nlu_training_data,
additional_arguments,
)
async def handle_domain_if_not_exists(
file_importer: TrainingDataImporter, output_path, fixed_model_name
):
nlu_model_only = await _train_nlu_with_validated_data(
file_importer, output=output_path, fixed_model_name=fixed_model_name
)
print_warning(
"Core training was skipped because no valid domain file was found. Only an nlu-model was created."
"Please specify a valid domain using '--domain' argument or check if the provided domain file exists."
)
return nlu_model_only
async def _train_async_internal(
file_importer: TrainingDataImporter,
train_path: Text,
output_path: Text,
force_training: bool,
fixed_model_name: Optional[Text],
persist_nlu_training_data: bool,
additional_arguments: Optional[Dict],
) -> Optional[Text]:
"""Trains a Rasa model (Core and NLU). Use only from `train_async`.
Args:
file_importer: `TrainingDataImporter` which supplies the training data.
train_path: Directory in which to train the model.
output_path: Output path.
force_training: If `True` retrain model even if data has not changed.
persist_nlu_training_data: `True` if the NLU training data should be persisted
with the model.
fixed_model_name: Name of model to be stored.
additional_arguments: Additional training parameters.
Returns:
Path of the trained model archive.
"""
stories, nlu_data = await asyncio.gather(
file_importer.get_stories(), file_importer.get_nlu_data()
)
# if stories.is_empty() and nlu_data.is_empty():
# print_error(
# "No training data given. Please provide stories and NLU data in "
# "order to train a Rasa model using the '--data' argument."
# )
# return
# if nlu_data.is_empty():
# print_warning("No NLU data present. Just a Rasa Core model will be trained.")
# return await _train_core_with_validated_data(
# file_importer,
# output=output_path,
# fixed_model_name=fixed_model_name,
# additional_arguments=additional_arguments,
# )
new_fingerprint = await model.model_fingerprint(file_importer)
old_model = model.get_latest_model(output_path)
fingerprint_comparison = FingerprintComparisonResult(force_training=force_training)
if not force_training:
fingerprint_comparison = model.should_retrain(
new_fingerprint, old_model, train_path
)
# bf mod >
if fingerprint_comparison.nlu == True: # replace True with list of all langs
fingerprint_comparison.nlu = list(new_fingerprint.get("nlu-config", {}).keys())
domain = await file_importer.get_domain()
core_untrainable = domain.is_empty() or stories.is_empty()
nlu_untrainable = [l for l, d in nlu_data.items() if d.is_empty()]
fingerprint_comparison.core = fingerprint_comparison.core and not core_untrainable
fingerprint_comparison.nlu = [l for l in fingerprint_comparison.nlu if l not in nlu_untrainable]
if core_untrainable:
print_color("Skipping Core training since domain or stories are empty.", color=bcolors.OKBLUE)
for lang in nlu_untrainable:
print_color("No NLU data found for language <{}>, skipping training...".format(lang), color=bcolors.OKBLUE)
# </ bf mod
if fingerprint_comparison.is_training_required():
await _do_training(
file_importer,
output_path=output_path,
train_path=train_path,
fingerprint_comparison_result=fingerprint_comparison,
fixed_model_name=fixed_model_name,
persist_nlu_training_data=persist_nlu_training_data,
additional_arguments=additional_arguments,
)
return model.package_model(
fingerprint=new_fingerprint,
output_directory=output_path,
train_path=train_path,
fixed_model_name=fixed_model_name,
)
print_success(
"Nothing changed. You can use the old model stored at '{}'."
"".format(os.path.abspath(old_model))
)
return old_model
async def _do_training(
file_importer: TrainingDataImporter,
output_path: Text,
train_path: Text,
fingerprint_comparison_result: Optional[FingerprintComparisonResult] = None,
fixed_model_name: Optional[Text] = None,
persist_nlu_training_data: bool = False,
additional_arguments: Optional[Dict] = None,
):
if not fingerprint_comparison_result:
fingerprint_comparison_result = FingerprintComparisonResult()
if fingerprint_comparison_result.should_retrain_core():
await _train_core_with_validated_data(
file_importer,
output=output_path,
train_path=train_path,
fixed_model_name=fixed_model_name,
additional_arguments=additional_arguments,
)
elif fingerprint_comparison_result.should_retrain_nlg():
print_color(
"Core stories/configuration did not change. "
"Only the templates section has been changed. A new model with "
"the updated templates will be created.",
color=bcolors.OKBLUE,
)
await model.update_model_with_new_domain(file_importer, train_path)
else:
print_color(
"Core stories/configuration did not change. No need to retrain Core model.",
color=bcolors.OKBLUE,
)
if fingerprint_comparison_result.should_retrain_nlu():
await _train_nlu_with_validated_data(
file_importer,
output=output_path,
train_path=train_path,
fixed_model_name=fixed_model_name,
retrain_nlu=fingerprint_comparison_result.nlu,
persist_nlu_training_data=persist_nlu_training_data,
)
else:
print_color(
"NLU data/configuration did not change. No need to retrain NLU model.",
color=bcolors.OKBLUE,
)
def train_core(
domain: Union[Domain, Text],
config: Text,
stories: Text,
output: Text,
train_path: Optional[Text] = None,
fixed_model_name: Optional[Text] = None,
additional_arguments: Optional[Dict] = None,
) -> Optional[Text]:
loop = asyncio.get_event_loop()
return loop.run_until_complete(
train_core_async(
domain=domain,
config=config,
stories=stories,
output=output,
train_path=train_path,
fixed_model_name=fixed_model_name,
additional_arguments=additional_arguments,
)
)
async def train_core_async(
domain: Union[Domain, Text],
config: Text,
stories: Text,
output: Text,
train_path: Optional[Text] = None,
fixed_model_name: Optional[Text] = None,
additional_arguments: Optional[Dict] = None,
) -> Optional[Text]:
"""Trains a Core model.
Args:
domain: Path to the domain file.
config: Path to the config file for Core.
stories: Path to the Core training data.
output: Output path.
train_path: If `None` the model will be trained in a temporary
directory, otherwise in the provided directory.
fixed_model_name: Name of model to be stored.
uncompress: If `True` the model will not be compressed.
additional_arguments: Additional training parameters.
Returns:
If `train_path` is given it returns the path to the model archive,
otherwise the path to the directory with the trained model files.
"""
file_importer = TrainingDataImporter.load_core_importer_from_config(
config, domain, [stories]
)
domain = await file_importer.get_domain()
if domain.is_empty():
print_error(
"Core training was skipped because no valid domain file was found. "
"Please specify a valid domain using '--domain' argument or check if the provided domain file exists."
)
return None
if not await file_importer.get_stories():
print_error(
"No stories given. Please provide stories in order to "
"train a Rasa Core model using the '--stories' argument."
)
return
return await _train_core_with_validated_data(
file_importer,
output=output,
train_path=train_path,
fixed_model_name=fixed_model_name,
additional_arguments=additional_arguments,
)
async def _train_core_with_validated_data(
file_importer: TrainingDataImporter,
output: Text,
train_path: Optional[Text] = None,
fixed_model_name: Optional[Text] = None,
additional_arguments: Optional[Dict] = None,
) -> Optional[Text]:
"""Train Core with validated training and config data."""
import rasa.core.train
with ExitStack() as stack:
if train_path:
# If the train path was provided, do nothing on exit.
_train_path = train_path
else:
# Otherwise, create a temp train path and clean it up on exit.
_train_path = stack.enter_context(TempDirectoryPath(tempfile.mkdtemp()))
# normal (not compare) training
print_color("Training Core model...", color=bcolors.OKBLUE)
domain, config = await asyncio.gather(
file_importer.get_domain(), file_importer.get_config()
)
await rasa.core.train(
domain_file=domain,
training_resource=file_importer,
output_path=os.path.join(_train_path, DEFAULT_CORE_SUBDIRECTORY_NAME),
policy_config=config,
additional_arguments=additional_arguments,
)
print_color("Core model training completed.", color=bcolors.OKBLUE)
if train_path is None:
# Only Core was trained.
new_fingerprint = await model.model_fingerprint(file_importer)
return model.package_model(
fingerprint=new_fingerprint,
output_directory=output,
train_path=_train_path,
fixed_model_name=fixed_model_name,
model_prefix="core-",
)
return _train_path
def train_nlu(
config: Text,
nlu_data: Text,
output: Text,
train_path: Optional[Text] = None,
fixed_model_name: Optional[Text] = None,
persist_nlu_training_data: bool = False,
) -> Optional[Text]:
"""Trains an NLU model.
Args:
config: Path to the config file for NLU.
nlu_data: Path to the NLU training data.
output: Output path.
train_path: If `None` the model will be trained in a temporary
directory, otherwise in the provided directory.
fixed_model_name: Name of the model to be stored.
persist_nlu_training_data: `True` if the NLU training data should be persisted
with the model.
Returns:
If `train_path` is given it returns the path to the model archive,
otherwise the path to the directory with the trained model files.
"""
loop = asyncio.get_event_loop()
return loop.run_until_complete(
_train_nlu_async(
config,
nlu_data,
output,
train_path,
fixed_model_name,
persist_nlu_training_data,
)
)
async def _train_nlu_async(
config: Text,
nlu_data: Text,
output: Text,
train_path: Optional[Text] = None,
fixed_model_name: Optional[Text] = None,
persist_nlu_training_data: bool = False,
):
if not nlu_data:
print_error(
"No NLU data given. Please provide NLU data in order to train "
"a Rasa NLU model using the '--nlu' argument."
)
return
# training NLU only hence the training files still have to be selected
file_importer = TrainingDataImporter.load_nlu_importer_from_config(
config, training_data_paths=[nlu_data]
)
training_datas = await file_importer.get_nlu_data()
if training_datas.is_empty():
print_error(
f"Path '{nlu_data}' doesn't contain valid NLU data in it. "
"Please verify the data format. "
"The NLU model training will be skipped now."
)
return
return await _train_nlu_with_validated_data(
file_importer,
output=output,
train_path=train_path,
fixed_model_name=fixed_model_name,
persist_nlu_training_data=persist_nlu_training_data,
)
async def _train_nlu_with_validated_data(
file_importer: TrainingDataImporter,
output: Text,
train_path: Optional[Text] = None,
fixed_model_name: Optional[Text] = None,
persist_nlu_training_data: bool = False,
retrain_nlu: Union[bool, List[Text]] = True
) -> Optional[Text]:
"""Train NLU with validated training and config data."""
import rasa.nlu.train
with ExitStack() as stack:
models = {}
from rasa.nlu import config as cfg_loader
if train_path:
# If the train path was provided, do nothing on exit.
_train_path = train_path
else:
# Otherwise, create a temp train path and clean it up on exit.
_train_path = stack.enter_context(TempDirectoryPath(tempfile.mkdtemp()))
# bf mod
config = await file_importer.get_nlu_config(retrain_nlu)
for lang in config:
if config[lang]:
print_color("Start training {} NLU model ...".format(lang), color=bcolors.OKBLUE)
_, models[lang], _ = await rasa.nlu.train(
config[lang],
file_importer,
_train_path,
fixed_model_name="nlu-{}".format(lang),
persist_nlu_training_data=persist_nlu_training_data,
)
else:
print_color("NLU data for language <{}> didn't change, skipping training...".format(lang), color=bcolors.OKBLUE)
# /bf mod
print_color("NLU model training completed.", color=bcolors.OKBLUE)
if train_path is None:
# Only NLU was trained
new_fingerprint = await model.model_fingerprint(file_importer)
return model.package_model(
fingerprint=new_fingerprint,
output_directory=output,
train_path=_train_path,
fixed_model_name=fixed_model_name,
model_prefix="nlu-",
)
return _train_path
| 2.09375 | 2 |
coding_intereview/1475. Final Prices With a Special Discount in a Shop.py | Jahidul007/Python-Bootcamp | 2 | 3 | <gh_stars>1-10
class Solution:
def finalPrices(self, prices: List[int]) -> List[int]:
res = []
for i in range(len(prices)):
for j in range(i+1,len(prices)):
if prices[j]<=prices[i]:
res.append(prices[i]-prices[j])
break
if j==len(prices)-1:
res.append(prices[i])
res.append(prices[-1])
return res | 2.765625 | 3 |
rplugin/python3/denite/ui/default.py | timgates42/denite.nvim | 0 | 4 | <gh_stars>0
# ============================================================================
# FILE: default.py
# AUTHOR: <NAME> <<EMAIL> at g<EMAIL>>
# License: MIT license
# ============================================================================
import re
import typing
from denite.util import echo, error, clearmatch, regex_convert_py_vim
from denite.util import Nvim, UserContext, Candidates, Candidate
from denite.parent import SyncParent
class Default(object):
@property
def is_async(self) -> bool:
return self._is_async
def __init__(self, vim: Nvim) -> None:
self._vim = vim
self._denite: typing.Optional[SyncParent] = None
self._selected_candidates: typing.List[int] = []
self._candidates: Candidates = []
self._cursor = 0
self._entire_len = 0
self._result: typing.List[typing.Any] = []
self._context: UserContext = {}
self._bufnr = -1
self._winid = -1
self._winrestcmd = ''
self._initialized = False
self._winheight = 0
self._winwidth = 0
self._winminheight = -1
self._is_multi = False
self._is_async = False
self._matched_pattern = ''
self._displayed_texts: typing.List[str] = []
self._statusline_sources = ''
self._titlestring = ''
self._ruler = False
self._prev_action = ''
self._prev_status: typing.Dict[str, typing.Any] = {}
self._prev_curpos: typing.List[typing.Any] = []
self._save_window_options: typing.Dict[str, typing.Any] = {}
self._sources_history: typing.List[typing.Any] = []
self._previous_text = ''
self._floating = False
self._filter_floating = False
self._updated = False
self._timers: typing.Dict[str, int] = {}
self._matched_range_id = -1
self._matched_char_id = -1
self._check_matchdelete = bool(self._vim.call(
'denite#util#check_matchdelete'))
def start(self, sources: typing.List[typing.Any],
context: UserContext) -> typing.List[typing.Any]:
if not self._denite:
# if hasattr(self._vim, 'run_coroutine'):
# self._denite = ASyncParent(self._vim)
# else:
self._denite = SyncParent(self._vim)
self._result = []
context['sources_queue'] = [sources]
self._start_sources_queue(context)
return self._result
def do_action(self, action_name: str,
command: str = '', is_manual: bool = False) -> None:
if is_manual:
candidates = self._get_selected_candidates()
elif self._get_cursor_candidate():
candidates = [self._get_cursor_candidate()]
else:
candidates = []
if not self._denite or not candidates or not action_name:
return
self._prev_action = action_name
action = self._denite.get_action(
self._context, action_name, candidates)
if not action:
return
post_action = self._context['post_action']
is_quit = action['is_quit'] or post_action == 'quit'
if is_quit:
self.quit()
self._denite.do_action(self._context, action_name, candidates)
self._result = candidates
if command != '':
self._vim.command(command)
if is_quit and post_action == 'open':
# Re-open denite buffer
prev_cursor = self._cursor
cursor_candidate = self._get_cursor_candidate()
self._init_buffer()
self.redraw(False)
if cursor_candidate == self._get_candidate(prev_cursor):
# Restore the cursor
self._move_to_pos(prev_cursor)
# Disable quit flag
is_quit = False
if not is_quit and is_manual:
self._selected_candidates = []
self.redraw(action['is_redraw'])
if is_manual and self._context['sources_queue']:
self._context['input'] = ''
self._context['quick_move'] = ''
self._start_sources_queue(self._context)
return
def redraw(self, is_force: bool = True) -> None:
self._context['is_redraw'] = is_force
if is_force:
self._gather_candidates()
if self._update_candidates():
self._update_buffer()
else:
self._update_status()
self._context['is_redraw'] = False
def quit(self) -> None:
if self._denite:
self._denite.on_close(self._context)
self._quit_buffer()
self._result = []
return
def _restart(self) -> None:
self._context['input'] = ''
self._quit_buffer()
self._init_denite()
self._gather_candidates()
self._init_buffer()
self._update_candidates()
self._update_buffer()
def _start_sources_queue(self, context: UserContext) -> None:
if not context['sources_queue']:
return
self._sources_history.append({
'sources': context['sources_queue'][0],
'path': context['path'],
})
self._start(context['sources_queue'][0], context)
if context['sources_queue']:
context['sources_queue'].pop(0)
context['path'] = self._context['path']
def _start(self, sources: typing.List[typing.Any],
context: UserContext) -> None:
from denite.ui.map import do_map
self._vim.command('silent! autocmd! denite')
if re.search(r'\[Command Line\]$', self._vim.current.buffer.name):
# Ignore command line window.
return
resume = self._initialized and context['resume']
if resume:
# Skip the initialization
update = ('immediately', 'immediately_1',
'cursor_pos', 'prev_winid',
'start_filter', 'quick_move')
for key in update:
self._context[key] = context[key]
self._check_move_option()
if self._check_do_option():
return
self._init_buffer()
if context['refresh']:
self.redraw()
self._move_to_pos(self._cursor)
else:
if self._context != context:
self._context.clear()
self._context.update(context)
self._context['sources'] = sources
self._context['is_redraw'] = False
self._is_multi = len(sources) > 1
if not sources:
# Ignore empty sources.
error(self._vim, 'Empty sources')
return
self._init_denite()
self._gather_candidates()
self._update_candidates()
self._init_cursor()
self._check_move_option()
if self._check_do_option():
return
self._init_buffer()
self._update_displayed_texts()
self._update_buffer()
self._move_to_pos(self._cursor)
if self._context['quick_move'] and do_map(self, 'quick_move', []):
return
if self._context['start_filter']:
do_map(self, 'open_filter_buffer', [])
def _init_buffer(self) -> None:
self._prev_status = dict()
self._displayed_texts = []
self._prev_bufnr = self._vim.current.buffer.number
self._prev_curpos = self._vim.call('getcurpos')
self._prev_wininfo = self._get_wininfo()
self._prev_winid = self._context['prev_winid']
self._winrestcmd = self._vim.call('winrestcmd')
self._ruler = self._vim.options['ruler']
self._switch_buffer()
self._bufnr = self._vim.current.buffer.number
self._winid = self._vim.call('win_getid')
self._resize_buffer(True)
self._winheight = self._vim.current.window.height
self._winwidth = self._vim.current.window.width
self._bufvars = self._vim.current.buffer.vars
self._bufvars['denite'] = {
'buffer_name': self._context['buffer_name'],
}
self._bufvars['denite_statusline'] = {}
self._vim.vars['denite#_previewed_buffers'] = {}
self._save_window_options = {}
window_options = {
'colorcolumn',
'concealcursor',
'conceallevel',
'cursorcolumn',
'cursorline',
'foldcolumn',
'foldenable',
'list',
'number',
'relativenumber',
'signcolumn',
'spell',
'winfixheight',
'wrap',
}
for k in window_options:
self._save_window_options[k] = self._vim.current.window.options[k]
# Note: Have to use setlocal instead of "current.window.options"
# "current.window.options" changes global value instead of local in
# neovim.
self._vim.command('setlocal colorcolumn=')
self._vim.command('setlocal conceallevel=3')
self._vim.command('setlocal concealcursor=inv')
self._vim.command('setlocal nocursorcolumn')
self._vim.command('setlocal nofoldenable')
self._vim.command('setlocal foldcolumn=0')
self._vim.command('setlocal nolist')
self._vim.command('setlocal nonumber')
self._vim.command('setlocal norelativenumber')
self._vim.command('setlocal nospell')
self._vim.command('setlocal winfixheight')
self._vim.command('setlocal nowrap')
if self._context['prompt']:
self._vim.command('setlocal signcolumn=yes')
else:
self._vim.command('setlocal signcolumn=auto')
if self._context['cursorline']:
self._vim.command('setlocal cursorline')
options = self._vim.current.buffer.options
if self._floating:
# Disable ruler
self._vim.options['ruler'] = False
options['buftype'] = 'nofile'
options['bufhidden'] = 'delete'
options['swapfile'] = False
options['buflisted'] = False
options['modeline'] = False
options['modifiable'] = False
options['filetype'] = 'denite'
if self._vim.call('exists', '#WinEnter'):
self._vim.command('doautocmd WinEnter')
if self._vim.call('exists', '#BufWinEnter'):
self._vim.command('doautocmd BufWinEnter')
if not self._vim.call('has', 'nvim'):
# In Vim8, FileType autocmd is not fired after set filetype option.
self._vim.command('silent doautocmd FileType denite')
if self._context['auto_action']:
self._vim.command('autocmd denite '
'CursorMoved <buffer> '
'call denite#call_map("auto_action")')
self._init_syntax()
def _switch_buffer(self) -> None:
split = self._context['split']
if (split != 'no' and self._winid > 0 and
self._vim.call('win_gotoid', self._winid)):
if split != 'vertical' and not self._floating:
# Move the window to bottom
self._vim.command('wincmd J')
self._winrestcmd = ''
return
self._floating = split in [
'floating',
'floating_relative_cursor',
'floating_relative_window',
]
self._filter_floating = False
if self._vim.current.buffer.options['filetype'] != 'denite':
self._titlestring = self._vim.options['titlestring']
command = 'edit'
if split == 'tab':
self._vim.command('tabnew')
elif self._floating:
self._split_floating(split)
elif self._context['filter_split_direction'] == 'floating':
self._filter_floating = True
elif split != 'no':
command = self._get_direction()
command += ' vsplit' if split == 'vertical' else ' split'
bufname = '[denite]-' + self._context['buffer_name']
if self._vim.call('exists', '*bufadd'):
bufnr = self._vim.call('bufadd', bufname)
vertical = 'vertical' if split == 'vertical' else ''
command = (
'buffer' if split
in ['no', 'tab', 'floating',
'floating_relative_window',
'floating_relative_cursor'] else 'sbuffer')
self._vim.command(
'silent keepalt %s %s %s %s' % (
self._get_direction(),
vertical,
command,
bufnr,
)
)
else:
self._vim.call(
'denite#util#execute_path',
f'silent keepalt {command}', bufname)
def _get_direction(self) -> str:
direction = str(self._context['direction'])
if direction == 'dynamictop' or direction == 'dynamicbottom':
self._update_displayed_texts()
winwidth = self._vim.call('winwidth', 0)
is_fit = not [x for x in self._displayed_texts
if self._vim.call('strwidth', x) > winwidth]
if direction == 'dynamictop':
direction = 'aboveleft' if is_fit else 'topleft'
else:
direction = 'belowright' if is_fit else 'botright'
return direction
def _get_wininfo(self) -> typing.List[typing.Any]:
return [
self._vim.options['columns'], self._vim.options['lines'],
self._vim.call('win_getid'), self._vim.call('tabpagebuflist')
]
def _switch_prev_buffer(self) -> None:
if (self._prev_bufnr == self._bufnr or
self._vim.buffers[self._prev_bufnr].name == ''):
self._vim.command('enew')
else:
self._vim.command('buffer ' + str(self._prev_bufnr))
def _init_syntax(self) -> None:
self._vim.command('syntax case ignore')
self._vim.command('highlight default link deniteInput ModeMsg')
self._vim.command('highlight link deniteMatchedRange ' +
self._context['highlight_matched_range'])
self._vim.command('highlight link deniteMatchedChar ' +
self._context['highlight_matched_char'])
self._vim.command('highlight default link ' +
'deniteStatusLinePath Comment')
self._vim.command('highlight default link ' +
'deniteStatusLineNumber LineNR')
self._vim.command('highlight default link ' +
'deniteSelectedLine Statement')
if self._floating:
self._vim.current.window.options['winhighlight'] = (
'Normal:' + self._context['highlight_window_background']
)
self._vim.command(('syntax match deniteSelectedLine /^[%s].*/' +
' contains=deniteConcealedMark') % (
self._context['selected_icon']))
self._vim.command(('syntax match deniteConcealedMark /^[ %s]/' +
' conceal contained') % (
self._context['selected_icon']))
if self._denite:
self._denite.init_syntax(self._context, self._is_multi)
def _update_candidates(self) -> bool:
if not self._denite:
return False
[self._is_async, pattern, statuses, self._entire_len,
self._candidates] = self._denite.filter_candidates(self._context)
prev_displayed_texts = self._displayed_texts
self._update_displayed_texts()
prev_matched_pattern = self._matched_pattern
self._matched_pattern = pattern
prev_statusline_sources = self._statusline_sources
self._statusline_sources = ' '.join(statuses)
if self._is_async:
self._start_timer('update_candidates')
else:
self._stop_timer('update_candidates')
updated = (self._displayed_texts != prev_displayed_texts or
self._matched_pattern != prev_matched_pattern or
self._statusline_sources != prev_statusline_sources)
if updated:
self._updated = True
self._start_timer('update_buffer')
if self._context['search'] and self._context['input']:
self._vim.call('setreg', '/', self._context['input'])
return self._updated
def _update_displayed_texts(self) -> None:
candidates_len = len(self._candidates)
if not self._is_async and self._context['auto_resize']:
winminheight = self._context['winminheight']
max_height = min(self._context['winheight'],
self._get_max_height())
if (winminheight != -1 and candidates_len < winminheight):
self._winheight = winminheight
elif candidates_len > max_height:
self._winheight = max_height
elif candidates_len != self._winheight:
self._winheight = candidates_len
max_source_name_len = 0
if self._candidates:
max_source_name_len = max([
len(self._get_display_source_name(x['source_name']))
for x in self._candidates])
self._context['max_source_name_len'] = max_source_name_len
self._context['max_source_name_format'] = (
'{:<' + str(self._context['max_source_name_len']) + '}')
self._displayed_texts = [
self._get_candidate_display_text(i)
for i in range(0, candidates_len)
]
def _update_buffer(self) -> None:
is_current_buffer = self._bufnr == self._vim.current.buffer.number
self._update_status()
if self._check_matchdelete and self._context['match_highlight']:
matches = [x['id'] for x in
self._vim.call('getmatches', self._winid)]
if self._matched_range_id in matches:
self._vim.call('matchdelete',
self._matched_range_id, self._winid)
self._matched_range_id = -1
if self._matched_char_id in matches:
self._vim.call('matchdelete',
self._matched_char_id, self._winid)
self._matched_char_id = -1
if self._matched_pattern != '':
self._matched_range_id = self._vim.call(
'matchadd', 'deniteMatchedRange',
r'\c' + regex_convert_py_vim(self._matched_pattern),
10, -1, {'window': self._winid})
matched_char_pattern = '[{}]'.format(re.sub(
r'([\[\]\\^-])',
r'\\\1',
self._context['input'].replace(' ', '')
))
self._matched_char_id = self._vim.call(
'matchadd', 'deniteMatchedChar',
matched_char_pattern,
10, -1, {'window': self._winid})
prev_linenr = self._vim.call('line', '.')
prev_candidate = self._get_cursor_candidate()
buffer = self._vim.buffers[self._bufnr]
buffer.options['modifiable'] = True
self._vim.vars['denite#_candidates'] = [
x['word'] for x in self._candidates]
buffer[:] = self._displayed_texts
buffer.options['modifiable'] = False
self._previous_text = self._context['input']
self._resize_buffer(is_current_buffer)
is_changed = (self._context['reversed'] or
(is_current_buffer and
self._previous_text != self._context['input']))
if self._updated and is_changed:
if not is_current_buffer:
save_winid = self._vim.call('win_getid')
self._vim.call('win_gotoid', self._winid)
self._init_cursor()
self._move_to_pos(self._cursor)
if not is_current_buffer:
self._vim.call('win_gotoid', save_winid)
elif is_current_buffer:
self._vim.call('cursor', [prev_linenr, 0])
if is_current_buffer:
if (self._context['auto_action'] and
prev_candidate != self._get_cursor_candidate()):
self.do_action(self._context['auto_action'])
self._updated = False
self._stop_timer('update_buffer')
def _update_status(self) -> None:
inpt = ''
if self._context['input']:
inpt = self._context['input'] + ' '
if self._context['error_messages']:
inpt = '[ERROR] ' + inpt
path = '[' + self._context['path'] + ']'
status = {
'input': inpt,
'sources': self._statusline_sources,
'path': path,
# Extra
'buffer_name': self._context['buffer_name'],
'line_total': len(self._candidates),
}
if status == self._prev_status:
return
self._bufvars['denite_statusline'] = status
self._prev_status = status
linenr = "printf('%'.(len(line('$'))+2).'d/%d',line('.'),line('$'))"
if self._context['statusline']:
if self._floating or self._filter_floating:
self._vim.options['titlestring'] = (
"%{denite#get_status('input')}%* " +
"%{denite#get_status('sources')} " +
" %{denite#get_status('path')}%*" +
"%{" + linenr + "}%*")
else:
winnr = self._vim.call('win_id2win', self._winid)
self._vim.call('setwinvar', winnr, '&statusline', (
"%#deniteInput#%{denite#get_status('input')}%* " +
"%{denite#get_status('sources')} %=" +
"%#deniteStatusLinePath# %{denite#get_status('path')}%*" +
"%#deniteStatusLineNumber#%{" + linenr + "}%*"))
def _get_display_source_name(self, name: str) -> str:
source_names = self._context['source_names']
if not self._is_multi or source_names == 'hide':
source_name = ''
else:
short_name = (re.sub(r'([a-zA-Z])[a-zA-Z]+', r'\1', name)
if re.search(r'[^a-zA-Z]', name) else name[:2])
source_name = short_name if source_names == 'short' else name
return source_name
def _get_candidate_display_text(self, index: int) -> str:
source_names = self._context['source_names']
candidate = self._candidates[index]
terms = []
if self._is_multi and source_names != 'hide':
terms.append(self._context['max_source_name_format'].format(
self._get_display_source_name(candidate['source_name'])))
encoding = self._context['encoding']
abbr = candidate.get('abbr', candidate['word']).encode(
encoding, errors='replace').decode(encoding, errors='replace')
terms.append(abbr[:int(self._context['max_candidate_width'])])
return (str(self._context['selected_icon'])
if index in self._selected_candidates
else ' ') + ' '.join(terms).replace('\n', '')
def _get_max_height(self) -> int:
return int(self._vim.options['lines']) if not self._floating else (
int(self._vim.options['lines']) -
int(self._context['winrow']) -
int(self._vim.options['cmdheight']))
def _resize_buffer(self, is_current_buffer: bool) -> None:
split = self._context['split']
if (split == 'no' or split == 'tab' or
self._vim.call('winnr', '$') == 1):
return
winheight = max(self._winheight, 1)
winwidth = max(self._winwidth, 1)
is_vertical = split == 'vertical'
if not is_current_buffer:
restore = self._vim.call('win_getid')
self._vim.call('win_gotoid', self._winid)
if not is_vertical and self._vim.current.window.height != winheight:
if self._floating:
wincol = self._context['winrow']
row = wincol
if split == 'floating':
if self._context['auto_resize'] and row > 1:
row += self._context['winheight']
row -= self._winheight
self._vim.call('nvim_win_set_config', self._winid, {
'relative': 'editor',
'row': row,
'col': self._context['wincol'],
'width': winwidth,
'height': winheight,
})
filter_row = 0 if wincol == 1 else row + winheight
filter_col = self._context['wincol']
else:
init_pos = self._vim.call('nvim_win_get_config',
self._winid)
self._vim.call('nvim_win_set_config', self._winid, {
'relative': 'win',
'win': init_pos['win'],
'row': init_pos['row'],
'col': init_pos['col'],
'width': winwidth,
'height': winheight,
})
filter_col = init_pos['col']
if init_pos['anchor'] == 'NW':
winpos = self._vim.call('nvim_win_get_position',
self._winid)
filter_row = winpos[0] + winheight
filter_winid = self._vim.vars['denite#_filter_winid']
self._context['filter_winrow'] = row
if self._vim.call('win_id2win', filter_winid) > 0:
self._vim.call('nvim_win_set_config', filter_winid, {
'relative': 'editor',
'row': filter_row,
'col': filter_col,
})
self._vim.command('resize ' + str(winheight))
if self._context['reversed']:
self._vim.command('normal! zb')
elif is_vertical and self._vim.current.window.width != winwidth:
self._vim.command('vertical resize ' + str(winwidth))
if not is_current_buffer:
self._vim.call('win_gotoid', restore)
def _check_do_option(self) -> bool:
if self._context['do'] != '':
self._do_command(self._context['do'])
return True
elif (self._candidates and self._context['immediately'] or
len(self._candidates) == 1 and self._context['immediately_1']):
self._do_immediately()
return True
return not (self._context['empty'] or
self._is_async or self._candidates)
def _check_move_option(self) -> None:
if self._context['cursor_pos'].isnumeric():
self._cursor = int(self._context['cursor_pos']) + 1
elif re.match(r'\+\d+', self._context['cursor_pos']):
for _ in range(int(self._context['cursor_pos'][1:])):
self._move_to_next_line()
elif re.match(r'-\d+', self._context['cursor_pos']):
for _ in range(int(self._context['cursor_pos'][1:])):
self._move_to_prev_line()
elif self._context['cursor_pos'] == '$':
self._move_to_last_line()
def _do_immediately(self) -> None:
goto = self._winid > 0 and self._vim.call(
'win_gotoid', self._winid)
if goto:
# Jump to denite window
self._init_buffer()
self.do_action('default')
candidate = self._get_cursor_candidate()
if not candidate:
return
echo(self._vim, 'Normal', '[{}/{}] {}'.format(
self._cursor, len(self._candidates),
candidate.get('abbr', candidate['word'])))
if goto:
# Move to the previous window
self._vim.command('wincmd p')
def _do_command(self, command: str) -> None:
self._init_cursor()
cursor = 1
while cursor < len(self._candidates):
self.do_action('default', command)
self._move_to_next_line()
self._quit_buffer()
def _cleanup(self) -> None:
self._stop_timer('update_candidates')
self._stop_timer('update_buffer')
if self._vim.current.buffer.number == self._bufnr:
self._cursor = self._vim.call('line', '.')
# Note: Close filter window before preview window
self._vim.call('denite#filter#_close_filter_window')
if not self._context['has_preview_window']:
self._vim.command('pclose!')
# Clear previewed buffers
for bufnr in self._vim.vars['denite#_previewed_buffers'].keys():
if not self._vim.call('win_findbuf', bufnr):
self._vim.command('silent bdelete ' + str(bufnr))
self._vim.vars['denite#_previewed_buffers'] = {}
self._vim.command('highlight! link CursorLine CursorLine')
if self._floating or self._filter_floating:
self._vim.options['titlestring'] = self._titlestring
self._vim.options['ruler'] = self._ruler
def _close_current_window(self) -> None:
if self._vim.call('winnr', '$') == 1:
self._vim.command('buffer #')
else:
self._vim.command('close!')
def _quit_buffer(self) -> None:
self._cleanup()
if self._vim.call('bufwinnr', self._bufnr) < 0:
# Denite buffer is already closed
return
winids = self._vim.call('win_findbuf',
self._vim.vars['denite#_filter_bufnr'])
if winids:
# Quit filter buffer
self._vim.call('win_gotoid', winids[0])
self._close_current_window()
# Move to denite window
self._vim.call('win_gotoid', self._winid)
# Restore the window
if self._context['split'] == 'no':
self._switch_prev_buffer()
for k, v in self._save_window_options.items():
self._vim.current.window.options[k] = v
else:
if self._context['split'] == 'tab':
self._vim.command('tabclose!')
if self._context['split'] != 'tab':
self._close_current_window()
self._vim.call('win_gotoid', self._prev_winid)
# Restore the position
self._vim.call('setpos', '.', self._prev_curpos)
if self._get_wininfo() and self._get_wininfo() == self._prev_wininfo:
# Note: execute restcmd twice to restore layout properly
self._vim.command(self._winrestcmd)
self._vim.command(self._winrestcmd)
clearmatch(self._vim)
def _get_cursor_candidate(self) -> Candidate:
return self._get_candidate(self._cursor)
def _get_candidate(self, pos: int) -> Candidate:
if not self._candidates or pos > len(self._candidates):
return {}
return self._candidates[pos - 1]
def _get_selected_candidates(self) -> Candidates:
if not self._selected_candidates:
return [self._get_cursor_candidate()
] if self._get_cursor_candidate() else []
return [self._candidates[x] for x in self._selected_candidates]
def _init_denite(self) -> None:
if self._denite:
self._denite.start(self._context)
self._denite.on_init(self._context)
self._initialized = True
self._winheight = self._context['winheight']
self._winwidth = self._context['winwidth']
def _gather_candidates(self) -> None:
self._selected_candidates = []
if self._denite:
self._denite.gather_candidates(self._context)
def _init_cursor(self) -> None:
if self._context['reversed']:
self._move_to_last_line()
else:
self._move_to_first_line()
def _move_to_pos(self, pos: int) -> None:
self._vim.call('cursor', pos, 0)
self._cursor = pos
if self._context['reversed']:
self._vim.command('normal! zb')
def _move_to_next_line(self) -> None:
if self._cursor < len(self._candidates):
self._cursor += 1
def _move_to_prev_line(self) -> None:
if self._cursor >= 1:
self._cursor -= 1
def _move_to_first_line(self) -> None:
self._cursor = 1
def _move_to_last_line(self) -> None:
self._cursor = len(self._candidates)
def _start_timer(self, key: str) -> None:
if key in self._timers:
return
if key == 'update_candidates':
self._timers[key] = self._vim.call(
'denite#helper#_start_update_candidates_timer', self._bufnr)
elif key == 'update_buffer':
self._timers[key] = self._vim.call(
'denite#helper#_start_update_buffer_timer', self._bufnr)
def _stop_timer(self, key: str) -> None:
if key not in self._timers:
return
self._vim.call('timer_stop', self._timers[key])
# Note: After timer_stop is called, self._timers may be removed
if key in self._timers:
self._timers.pop(key)
def _split_floating(self, split: str) -> None:
# Use floating window
if split == 'floating':
self._vim.call(
'nvim_open_win',
self._vim.call('bufnr', '%'), True, {
'relative': 'editor',
'row': self._context['winrow'],
'col': self._context['wincol'],
'width': self._context['winwidth'],
'height': self._context['winheight'],
})
elif split == 'floating_relative_cursor':
opened_pos = (self._vim.call('nvim_win_get_position', 0)[0] +
self._vim.call('winline') - 1)
if self._context['auto_resize']:
height = max(self._winheight, 1)
width = max(self._winwidth, 1)
else:
width = self._context['winwidth']
height = self._context['winheight']
if opened_pos + height + 3 > self._vim.options['lines']:
anchor = 'SW'
row = 0
self._context['filter_winrow'] = row + opened_pos
else:
anchor = 'NW'
row = 1
self._context['filter_winrow'] = row + height + opened_pos
self._vim.call(
'nvim_open_win',
self._vim.call('bufnr', '%'), True, {
'relative': 'cursor',
'row': row,
'col': 0,
'width': width,
'height': height,
'anchor': anchor,
})
elif split == 'floating_relative_window':
self._vim.call(
'nvim_open_win',
self._vim.call('bufnr', '%'), True, {
'relative': 'win',
'row': self._context['winrow'],
'col': self._context['wincol'],
'width': self._context['winwidth'],
'height': self._context['winheight'],
})
| 1.882813 | 2 |
PyDSTool/core/context_managers.py | yuanz271/PyDSTool | 0 | 5 | <filename>PyDSTool/core/context_managers.py
# -*- coding: utf-8 -*-
"""Context managers implemented for (mostly) internal use"""
import contextlib
import functools
from io import UnsupportedOperation
import os
import sys
__all__ = ["RedirectStdout", "RedirectStderr"]
@contextlib.contextmanager
def _stdchannel_redirected(stdchannel, dest_filename, mode="w"):
"""
A context manager to temporarily redirect stdout or stderr
Originally by <NAME>, 2013
(http://marc-abramowitz.com/archives/2013/07/19/python-context-manager-for-redirected-stdout-and-stderr/)
"""
oldstdchannel = None
dest_file = None
try:
if stdchannel is None:
yield iter([None])
else:
oldstdchannel = os.dup(stdchannel.fileno())
dest_file = open(dest_filename, mode)
os.dup2(dest_file.fileno(), stdchannel.fileno())
yield
except (UnsupportedOperation, AttributeError):
yield iter([None])
finally:
if oldstdchannel is not None:
os.dup2(oldstdchannel, stdchannel.fileno())
if dest_file is not None:
dest_file.close()
RedirectStdout = functools.partial(_stdchannel_redirected, sys.stdout)
RedirectStderr = functools.partial(_stdchannel_redirected, sys.stderr)
RedirectNoOp = functools.partial(_stdchannel_redirected, None, "")
| 2.359375 | 2 |
pos_kiosk/hooks.py | Muzzy73/pos_kiosk | 1 | 6 | # -*- coding: utf-8 -*-
from __future__ import unicode_literals
from . import __version__ as app_version
app_name = "pos_kiosk"
app_title = "Pos Kiosk"
app_publisher = "9t9it"
app_description = "Kiosk App"
app_icon = "octicon octicon-file-directory"
app_color = "grey"
app_email = "<EMAIL>"
app_license = "MIT"
# Includes in <head>
# ------------------
# include js, css files in header of desk.html
# app_include_css = "/assets/pos_kiosk/css/pos_kiosk.css"
# app_include_js = "/assets/pos_kiosk/js/pos_kiosk.js"
# include js, css files in header of web template
# web_include_css = "/assets/pos_kiosk/css/pos_kiosk.css"
# web_include_js = "/assets/pos_kiosk/js/pos_kiosk.js"
# include js in page
# page_js = {"page" : "public/js/file.js"}
# page_js = {
# "kiosk": ["public/js/pos_page_js.js", "public/js/includes/number_to_words.js"]
# }
# include js in doctype views
# doctype_js = {"doctype" : "public/js/doctype.js"}
# doctype_list_js = {"doctype" : "public/js/doctype_list.js"}
# doctype_tree_js = {"doctype" : "public/js/doctype_tree.js"}
# doctype_calendar_js = {"doctype" : "public/js/doctype_calendar.js"}
fixtures = [
{
"doctype": "Custom Field",
"filters": [
[
"name",
"in",
[
"Sales Invoice Item-pos_kiosk",
"Mode of Payment-logo"
]
]
]
}
]
# Home Pages
# ----------
# application home page (will override Website Settings)
# home_page = "login"
# website user home page (by Role)
# role_home_page = {
# "Role": "home_page"
# }
# Website user home page (by function)
# get_website_user_home_page = "pos_kiosk.utils.get_home_page"
# Generators
# ----------
# automatically create page for each record of this doctype
# website_generators = ["Web Page"]
# Installation
# ------------
# before_install = "pos_kiosk.install.before_install"
# after_install = "pos_kiosk.install.after_install"
# Desk Notifications
# ------------------
# See frappe.core.notifications.get_notification_config
# notification_config = "pos_kiosk.notifications.get_notification_config"
# Permissions
# -----------
# Permissions evaluated in scripted ways
# permission_query_conditions = {
# "Event": "frappe.desk.doctype.event.event.get_permission_query_conditions",
# }
#
# has_permission = {
# "Event": "frappe.desk.doctype.event.event.has_permission",
# }
# Document Events
# ---------------
# Hook on document methods and events
# doc_events = {
# "*": {
# "on_update": "method",
# "on_cancel": "method",
# "on_trash": "method"
# }
# }
# Scheduled Tasks
# ---------------
# scheduler_events = {
# "all": [
# "pos_kiosk.tasks.all"
# ],
# "daily": [
# "pos_kiosk.tasks.daily"
# ],
# "hourly": [
# "pos_kiosk.tasks.hourly"
# ],
# "weekly": [
# "pos_kiosk.tasks.weekly"
# ]
# "monthly": [
# "pos_kiosk.tasks.monthly"
# ]
# }
# Testing
# -------
# before_tests = "pos_kiosk.install.before_tests"
# Overriding Whitelisted Methods
# ------------------------------
#
# override_whitelisted_methods = {
# "pos_bahrain.api.get_item_details.get_item_details": "pos_kiosk.api.item.get_item_details" # noqa
# }
| 1.414063 | 1 |
pypagai/models/model_lstm.py | gcouti/pypagAI | 1 | 7 | <gh_stars>1-10
from keras import Model, Input
from keras.layers import Dense, concatenate, LSTM, Reshape, Permute, Embedding, Dropout, Convolution1D, Flatten
from keras.optimizers import Adam
from pypagai.models.base import KerasModel
class SimpleLSTM(KerasModel):
"""
Use a simple lstm neural network
"""
@staticmethod
def default_config():
config = KerasModel.default_config()
config['hidden'] = 32
return config
def __init__(self, cfg):
super().__init__(cfg)
self._cfg_ = cfg
def _create_network_(self):
hidden = self._cfg_['hidden']
story = Input((self._story_maxlen, ), name='story')
question = Input((self._query_maxlen, ), name='question')
conc = concatenate([story, question],)
conc = Reshape((1, int(conc.shape[1])))(conc)
conc = Permute((2, 1))(conc)
response = LSTM(hidden, dropout=0.2, recurrent_dropout=0.2)(conc)
response = Dense(self._vocab_size, activation='softmax')(response)
self._model = Model(inputs=[story, question], outputs=response)
self._model.compile(optimizer=Adam(lr=2e-4), loss='sparse_categorical_crossentropy', metrics=['accuracy'])
class EmbedLSTM(KerasModel):
"""
Use a simple lstm neural network
"""
@staticmethod
def default_config():
config = KerasModel.default_config()
config['hidden'] = 32
return config
def __init__(self, cfg):
super().__init__(cfg)
self._cfg_ = cfg
def _create_network_(self):
hidden = self._cfg_['hidden']
story = Input((self._story_maxlen, ), name='story')
question = Input((self._query_maxlen, ), name='question')
eb_story = Embedding(self._vocab_size, 64)(story)
eb_story = Dropout(0.3)(eb_story)
eb_question = Embedding(self._vocab_size, 64)(question)
eb_question = Dropout(0.3)(eb_question)
conc = concatenate([eb_story, eb_question], axis=1)
response = LSTM(hidden, dropout=0.2, recurrent_dropout=0.2)(conc)
response = Dense(self._vocab_size, activation='softmax')(response)
self._model = Model(inputs=[story, question], outputs=response)
self._model.compile(optimizer=Adam(lr=2e-4), loss='sparse_categorical_crossentropy', metrics=['accuracy'])
class ConvLSTM(KerasModel):
"""
Use a simple lstm neural network
"""
@staticmethod
def default_config():
config = KerasModel.default_config()
config['hidden'] = 32
return config
def __init__(self, model_cfg):
super().__init__(model_cfg)
self._cfg = model_cfg
def _create_network_(self):
hidden = self._cfg['hidden']
story = Input((self._story_maxlen, ), name='story')
question = Input((self._query_maxlen, ), name='question')
eb_story = Embedding(self._vocab_size, 64)(story)
eb_story = Convolution1D(64, 3, padding='same')(eb_story)
eb_story = Convolution1D(32, 3, padding='same')(eb_story)
eb_story = Convolution1D(16, 3, padding='same')(eb_story)
# eb_story = Flatten()(eb_story)
eb_question = Embedding(self._vocab_size, 64)(question)
eb_question = Convolution1D(64, 3, padding='same')(eb_question)
eb_question = Convolution1D(32, 3, padding='same')(eb_question)
eb_question = Convolution1D(16, 3, padding='same')(eb_question)
# eb_question = Flatten()(eb_question)
conc = concatenate([eb_story, eb_question], axis=1)
response = LSTM(hidden, dropout=0.2, recurrent_dropout=0.2)(conc)
response = Dense(self._vocab_size, activation='softmax')(response)
self._model = Model(inputs=[story, question], outputs=response)
self._model.compile(optimizer=Adam(lr=2e-4), loss='sparse_categorical_crossentropy', metrics=['accuracy'])
| 2.78125 | 3 |
lib/variables/latent_variables/__init__.py | joelouismarino/variational_rl | 15 | 8 | <filename>lib/variables/latent_variables/__init__.py
from .fully_connected import FullyConnectedLatentVariable
from .convolutional import ConvolutionalLatentVariable
| 1.085938 | 1 |
easyai/model/backbone/cls/pnasnet.py | lpj0822/image_point_cloud_det | 1 | 9 | #!/usr/bin/env python
# -*- coding:utf-8 -*-
# Author:
''' PNASNet in PyTorch.
Paper: Progressive Neural Architecture Search
'''
from easyai.base_name.block_name import NormalizationType, ActivationType
from easyai.base_name.backbone_name import BackboneName
from easyai.model.backbone.utility.base_backbone import *
from easyai.model.base_block.utility.utility_block import ConvBNActivationBlock
from easyai.model.base_block.cls.pnasnet_block import CellA, CellB
__all__ = ['pnasnet_A', 'pnasnet_B']
class PNASNet(BaseBackbone):
def __init__(self, data_channel=3, num_cells=6,
num_planes=44, block=CellA,
bnName=NormalizationType.BatchNormalize2d,
activationName=ActivationType.ReLU):
super().__init__()
self.set_name(BackboneName.PNASNetA)
self.data_channel = data_channel
self.num_cells = num_cells
self.block = block
self.activation_name = activationName
self.bn_name = bnName
self.first_output = num_planes
self.in_planes = self.first_output
self.create_block_list()
def create_block_list(self):
self.block_out_channels = []
self.index = 0
layer1 = ConvBNActivationBlock(in_channels=self.data_channel,
out_channels=self.first_output,
kernel_size=3,
stride=1,
padding=1,
bias=False,
bnName=self.bn_name,
activationName=self.activation_name)
self.add_block_list(layer1.get_name(), layer1, self.first_output)
self.make_layer(self.first_output, self.num_cells)
self.downsample(self.first_output * 2)
self.make_layer(self.first_output * 2, self.num_cells)
self.downsample(self.first_output * 4)
self.make_layer(self.first_output * 4, self.num_cells)
def make_layer(self, planes, num_cells):
for _ in range(num_cells):
temp_block = self.block(self.in_planes, planes, stride=1,
bn_name=self.bn_name, activation_name=self.activation_name)
self.add_block_list(temp_block.get_name(), temp_block, planes)
self.in_planes = planes
def downsample(self, planes):
down_block = self.block(self.in_planes, planes, stride=2,
bn_name=self.bn_name, activation_name=self.activation_name)
self.add_block_list(down_block.get_name(), down_block, planes)
self.in_planes = planes
def forward(self, x):
output_list = []
for block in self._modules.values():
x = block(x)
output_list.append(x)
return output_list
def pnasnet_A(data_channel):
model = PNASNet(data_channel=data_channel,
num_cells=6,
num_planes=44,
block=CellA)
model.set_name(BackboneName.PNASNetA)
return model
def pnasnet_B(data_channel):
model = PNASNet(data_channel=data_channel,
num_cells=6, num_planes=32,
block=CellB)
model.set_name(BackboneName.PNASNetB)
return model
| 2.71875 | 3 |
map_download/cmd/TerrainDownloader.py | cugxy/map_download | 27 | 10 | # -*- coding: utf-8 -*-
# coding=utf-8
import json
import os
import math
import logging
import requests
import time
from map_download.cmd.BaseDownloader import DownloadEngine, BaseDownloaderThread, latlng2tile_terrain, BoundBox
def get_access_token(token):
resp = None
request_count = 0
url = "https://api.cesium.com/v1/assets/1/endpoint"
while True:
if request_count > 4:
break
try:
request_count += 1
param = {'access_token': token}
resp = requests.get(url, params=param, timeout=2)
if resp.status_code != 200:
continue
break
except Exception as e:
resp = None
time.sleep(3)
if resp is None:
return None
resp_json = resp.json()
return resp_json.get('accessToken')
class TerrainDownloaderThread(BaseDownloaderThread):
URL = "https://assets.cesium.com/1/{z}/{x}/{y}.terrain?extensions=octvertexnormals-watermask&v=1.1.0"
def __init__(self, root_dir, bbox, token, task_q, logger=None, write_db=False):
super(TerrainDownloaderThread, self).__init__(
root_dir, bbox, task_q, logger, write_db=write_db, db_file_name='Terrain.db')
self.token = token
self._init_metadata(
format='terrain',
bounds='%f,%f,%f,%f' % (self.bbox.min_lng, self.bbox.min_lat, self.bbox.max_lng, self.bbox.max_lat))
def get_url(self, x, y, z):
return self.URL.format(x=x, y=y, z=z)
def _download(self, x, y, z):
file_path = '%s/%s/%i/%i/%i.%s' % (self.root_dir, 'Terrain', z, x, y, 'terrain')
if os.path.exists(file_path):
self._data2DB(x, y, z, file_path)
return 0
os.makedirs(os.path.dirname(file_path), exist_ok=True)
resp = None
requre_count = 0
_url = ''
access_token = get_access_token(self.token)
if access_token is None:
return -1
param = {'extensions': 'octvertexnormals-watermask', 'v': '1.1.0', 'access_token': access_token}
while True:
if requre_count > 4: break
try:
_url = self.get_url(x, y, z)
resp = requests.get(_url, params=param, stream=True, timeout=2)
break
except Exception as e:
resp = None
time.sleep(3)
requre_count += 1
if resp is None:
return -1
if resp.status_code != 200:
return -1
try:
with open(file_path, 'wb') as f:
for chunk in resp.iter_content(chunk_size=1024):
if chunk:
f.write(chunk)
except Exception as e:
return -1
self._data2DB(x, y, z, file_path)
return 1
class TerrainDownloadEngine(DownloadEngine):
root_dir = ''
def __init__(self, root_dir, bbox, token, thread_num, logger=None, write_db=False):
super(TerrainDownloadEngine, self).__init__(bbox, thread_num, logger, write_db=write_db)
self.root_dir = root_dir
self.token = token
def bbox2xyz(self, bbox, z):
min_x, min_y = latlng2tile_terrain(bbox.min_lat, bbox.min_lng, z)
max_x, max_y = latlng2tile_terrain(bbox.max_lat, bbox.max_lng, z)
return math.floor(min_x), math.floor(min_y), math.ceil(max_x) + 1, math.ceil(max_y) + 1
def generate_metadata(self):
try:
metadatas = {
"attribution": "© Analytical Graphics Inc., © CGIAR-CSI, Produced using Copernicus data and "
"information funded by the European Union - EU-DEM layers",
"available": [
[
{
"endX": 1,
"endY": 0,
"startX": 0,
"startY": 0
}
],
[
{
"endX": 3,
"endY": 1,
"startX": 0,
"startY": 0
}
],
[
{
"endX": 7,
"endY": 3,
"startX": 0,
"startY": 0
}
],
[
{
"endX": 15,
"endY": 7,
"startX": 0,
"startY": 0
}
],
[
{
"endX": 31,
"endY": 15,
"startX": 0,
"startY": 0
}
],
[
{
"endX": 63,
"endY": 31,
"startX": 0,
"startY": 0
}
],
[
{
"endX": 127,
"endY": 63,
"startX": 0,
"startY": 0
}
],
[
{
"endX": 255,
"endY": 127,
"startX": 0,
"startY": 0
}
],
[
{
"endX": 511,
"endY": 255,
"startX": 0,
"startY": 0
}
],
[
{
"endX": 1023,
"endY": 511,
"startX": 0,
"startY": 0
}
],
[
{
"endX": 2047,
"endY": 1023,
"startX": 0,
"startY": 0
}
],
[
{
"endX": 4095,
"endY": 2047,
"startX": 0,
"startY": 0
}
],
[
{
"endX": 8191,
"endY": 4095,
"startX": 0,
"startY": 0
}
],
[
{
"endX": 16383,
"endY": 8191,
"startX": 0,
"startY": 0
}
],
[
{
"endX": 32767,
"endY": 16383,
"startX": 0,
"startY": 0
}
]
],
"bounds": [-180, -90, 180, 90, ],
"description": "STK World Terrain Premium Tileset, v1.3. 10m - 30m resolution CONUS, 30m resolution "
"SRTM between 60N and 60S, 30m Europe. Minimum global coverage of 1000m.",
"extensions": ["watermask", "vertexnormals", "octvertexnormals", ],
"format": "quantized-mesh-1.0",
"maxzoom": 13,
"minzoom": 0,
"name": "world",
"projection": "EPSG:4326",
"scheme": "tms",
"tilejson": "2.1.0",
"tiles": ["{z}/{x}/{y}.terrain?v={version}", ],
"version": "1.31376.0"
}
_dir = os.path.join(self.root_dir, 'Terrain')
os.makedirs(_dir, exist_ok=True)
metadatas_path = os.path.join(_dir, 'layer.json')
with open(metadatas_path, 'w') as f:
json.dump(metadatas, f)
except Exception as e:
if self.logger is not None:
self.logger.exception(e)
def run(self):
try:
self.generate_metadata()
count = 0
bboxs = self.cut_bbox()
for bbox in bboxs:
_count = self.get_task_count(bbox)
count += _count
self.division_done_signal.emit(count)
for bbox in bboxs:
while True:
if not self.running:
time.sleep(0.01)
else:
break
task_q = self.get_task_queue(bbox)
self.threads = []
for i in range(self.thread_num):
thread = TerrainDownloaderThread(self.root_dir, self.bbox, self.token, task_q, self.logger,
write_db=self.write_db)
thread.sub_progressBar_updated_signal.connect(self.sub_update_progressBar)
self.threads.append(thread)
for thread in self.threads:
thread.start()
for thread in self.threads:
thread.wait()
for t in self.threads:
t.stop()
t.quit()
self.threads = []
self.download_done_signal.emit()
except Exception as e:
if self.logger is not None:
self.logger.error(e)
if __name__ == '__main__':
if 1:
logger = logging.getLogger('down')
try:
root = r'/Users/cugxy/Documents/data/downloader'
formatter = logging.Formatter('%(levelname)s-%(message)s')
hdlr = logging.StreamHandler()
log_file = os.path.join(root, 'down.log')
file_hdlr = logging.FileHandler(log_file)
file_hdlr.setFormatter(formatter)
logger.addHandler(file_hdlr)
logger.addHandler(hdlr)
logger.setLevel(logging.INFO)
min_lng = -180.0
max_lng = 180.0
min_lat = -90.0
max_lat = 90.0
start_zoom = 0
end_zoom = 5
bbox = BoundBox(max_lat, max_lng, min_lat, min_lng, start_zoom, end_zoom)
d = TerrainDownloadEngine(root, bbox, 8, logger)
d.start()
time.sleep(10000)
logger.error('main thread out')
except Exception as e:
logger.error(e)
if 0:
accessToken = get_access_token()
pass
| 2.453125 | 2 |
tools/utils.py | vahini01/electoral_rolls | 16 | 11 | <reponame>vahini01/electoral_rolls
#!/usr/bin/env python3
# -*- coding: utf-8 -*-
"""
Created on Fri Nov 10 23:28:58 2017
@author: dhingratul
"""
import urllib.request
import os
from selenium import webdriver
from selenium.webdriver.support.ui import Select
from bs4 import BeautifulSoup
import ssl
import requests
import wget
from PyPDF2 import PdfFileReader
def download_file(pdf_url, mdir, filename, flag=False):
if flag is True:
context = ssl._create_unverified_context()
response = urllib.request.urlopen(pdf_url, context=context)
else:
response = urllib.request.urlopen(pdf_url)
filename = mdir + filename
file = open(filename, 'wb')
file.write(response.read())
if os.stat(filename).st_size == 0:
flag = 0
else:
flag = 1
file.close()
return flag
def download_file_R(pdf_url, mdir, filename, file_out):
requests.packages.urllib3.disable_warnings()
while True: # Keep trying until the webpage successfully downloads
try:
r = requests.get(pdf_url, verify=False, timeout=10)
break # If it downloads, get out and get on with life
# If it doesn't download after the timeout period, an exceptions is thrown, and we try again
except requests.exceptions.RequestException as e:
with open(file_out, "a") as myfile:
myfile.write(pdf_url + '\n')
filename = mdir + filename
with open(filename, 'wb') as f:
f.write(r.content)
if os.stat(filename).st_size == 0:
flag = 0
else:
flag = 1
return flag
def download_file_W(pdf_url, mdir, filename, flag=False):
filename = mdir + filename
ssl._create_default_https_context = ssl._create_unverified_context
wget.download(pdf_url, filename)
if os.stat(filename).st_size == 0:
flag = 0
else:
flag = 1
return flag
def getDriver(url):
driver = webdriver.Chrome()
driver.get(url)
return driver
def is_valid_pdf(fn):
"""Check is the PDF valid """
try:
with open(fn, 'rb') as f:
pdf = PdfFileReader(f)
numpages = pdf.numPages
return (numpages > 0)
except Exception as e:
return False
| 3 | 3 |
exp/viz_raw_manhattan.py | ellencwade/coronavirus-2020 | 0 | 12 | <gh_stars>0
"""
Experiment summary
------------------
Treat each province/state in a country cases over time
as a vector, do a simple K-Nearest Neighbor between
countries. What country has the most similar trajectory
to a given country?
Plots similar countries
"""
import sys
sys.path.insert(0, '..')
from utils import data
import os
import sklearn
import numpy as np
import json
import matplotlib.pyplot as plt
plt.style.use('fivethirtyeight')
# ------------ HYPERPARAMETERS -------------
BASE_PATH = '../COVID-19/csse_covid_19_data/'
# ------------------------------------------
confirmed = os.path.join(
BASE_PATH,
'csse_covid_19_time_series',
'time_series_covid19_confirmed_global.csv')
confirmed = data.load_csv_data(confirmed)
features = []
targets = []
fig = plt.figure(figsize=(12, 12))
ax = fig.add_subplot(111)
cm = plt.get_cmap('jet')
NUM_COLORS = 0
LINE_STYLES = ['solid', 'dashed', 'dotted']
NUM_STYLES = len(LINE_STYLES)
dist_diff = os.path.join('../exp/results/', 'knn_raw.json')
f = open(dist_diff,)
dist_diff = json.load(f)
for region, dist in dist_diff.items():
plt.style.use('fivethirtyeight')
fig = plt.figure(figsize=(12, 12))
ax = fig.add_subplot(111)
cm = plt.get_cmap('jet')
other_region = dist['manhattan'][0]
regions = [region, other_region]
for val in regions:
df = data.filter_by_attribute(
confirmed, "Country/Region", val)
cases, labels = data.get_cases_chronologically(df)
cases = cases.sum(axis=0)
lines = ax.plot(cases, label=val)
ax.set_ylabel('# of confirmed cases')
ax.set_xlabel("Time (days since Jan 22, 2020)")
ax.set_yscale('log')
ax.legend()
plt.tight_layout()
region = region.replace('*', '')
other_region = other_region.replace('*', '')
plt.title(f'Comparing confirmed cases in {region} and {other_region}')
plt.savefig(f'results/raw_manhattan/{region}.png')
plt.close()
print(region) | 3.015625 | 3 |
rational/mxnet/rationals.py | steven-lang/rational_activations | 0 | 13 | <reponame>steven-lang/rational_activations
"""
Rational Activation Functions for MXNET
=======================================
This module allows you to create Rational Neural Networks using Learnable
Rational activation functions with MXNET networks.
"""
import mxnet as mx
from mxnet import initializer
from mxnet.gluon import HybridBlock
from rational.utils.get_weights import get_parameters
from rational.mxnet.versions import _version_a, _version_b, _version_c, _version_d
from rational._base.rational_base import Rational_base
class Rational(Rational_base, HybridBlock):
"""
Rational Activation Function, inheriting from ``mxnet.gluon.HybridBlock``.
Arguments:
approx_func (str):
The name of the approximated function for initialisation.
The different functions are available in `rational.rationals_config.json`.
Default: ``leaky_relu``
degrees (tuple of int):
The degrees of the numerator (P) and denominator (Q).
Default ``(5, 4)``
cuda (bool):
whether to execute on cuda device.
NOTE: THIS PARAMETER IS CURRENTLY NOT CONSIDERED.
CUDA GPUS ARE USED WHEN IT IS POSSIBLE
version (str):
Version of Rational to use. Rational(x) = P(x)/Q(x),
where
P(x) = (a_0 + a_1 * x + a_2 * x^2 + ... + a_n * x^n) and
`A`: Q(x) = (1 + |b_0 * x| + | b_1 * x^2| + ... + | b_m * x^{m+1}|)
`B`: Q(x) = (1 + |b_0 * x + b_1 * x^2 + ... + b_m * x^{m + 1}|)
`C`: Q(x) = (0.1 + |b_0 + b_1 * x + b_2 * x^2 + ... + b_m * x^m|)
`D`: like `B` with noised coefficients b_i
Default ``A``
trainable (bool):
Whether the weights are trainable, i.e, if they are updated during
backward pass.
Default ``True``
Returns:
HybridBlock:
Rational hybrid block
"""
def __init__(self, approx_func='leaky_relu', degrees=(5, 4), cuda=False,
version='A', trainable=True, **kwargs):
super(Rational, self).__init__(**kwargs)
# read initial parameter configuration from external files
w_numerator, w_denominator = get_parameters(
version, degrees, approx_func)
# convert w_numerator and w_denominator to mxnet arrays
w_numerator = mx.nd.array(w_numerator)
w_denominator = mx.nd.array(w_denominator)
# register the amount of weights in numerator and denominator, since we need them during
# symbolic execution, but are unable to retrieve them at later stages
self.numerator_length = len(w_numerator)
self.denominator_length = len(w_denominator)
self.training = trainable
self.degrees = degrees
self.version = version
self.init_approximation = approx_func
# set specified context (currently not happening, since unclear, how and why helpful)
# self.device = gpu() if cuda else cpu()
# register and configure weights (numerator and denominator coefficients)
with self.name_scope():
self.numerator = self.params.get(name='w_numerator', shape=(len(w_numerator),),
init=initializer.Constant(
w_numerator),
grad_req='write' if trainable
else 'null',
differentiable=trainable)
self.denominator = self.params.get(name='w_denominator', shape=(len(w_denominator),),
init=initializer.Constant(
w_denominator),
grad_req='write' if trainable
else 'null',
differentiable=trainable)
# register whether function is trainable, since this information needs to be passed to
# version D
self.training = trainable
self.init_approximation = approx_func
# set rational activation function version
self.rational_func = {'A': _version_a, 'B': _version_b, 'C': _version_c, 'D': _version_d} \
.get(version)
if self.rational_func is None:
raise ValueError(
"rational activation function version %s not implemented" % version)
def hybrid_forward(self, F, x, numerator, denominator):
return self.rational_func(F, x, numerator, denominator, self.training,
self.numerator_length, self.denominator_length)
def numpy(self):
"""
Returns a numpy version of this activation function.
"""
from rational.numpy import Rational as Rational_numpy
rational_n = Rational_numpy(self.init_approximation, self.degrees,
self.version)
rational_n.numerator = self.numerator.data().asnumpy().tolist()
rational_n.denominator = self.denominator.data().asnumpy().tolist()
return rational_n
| 2.75 | 3 |
torchflare/criterion/utils.py | Neklaustares-tPtwP/torchflare | 1 | 14 | <filename>torchflare/criterion/utils.py<gh_stars>1-10
"""Utils for criterion."""
import torch
import torch.nn.functional as F
def normalize(x, axis=-1):
"""Performs L2-Norm."""
num = x
denom = torch.norm(x, 2, axis, keepdim=True).expand_as(x) + 1e-12
return num / denom
# Source : https://github.com/earhian/Humpback-Whale-Identification-1st-/blob/master/models/triplet_loss.py
def euclidean_dist(x, y):
"""Computes Euclidean distance."""
m, n = x.size(0), y.size(0)
xx = torch.pow(x, 2).sum(1, keepdim=True).expand(m, n)
yy = torch.pow(x, 2).sum(1, keepdim=True).expand(m, m).t()
dist = xx + yy - 2 * torch.matmul(x, y.t())
dist = dist.clamp(min=1e-12).sqrt()
return dist
def cosine_dist(x, y):
"""Computes Cosine Distance."""
x = F.normalize(x, dim=1)
y = F.normalize(y, dim=1)
dist = 2 - 2 * torch.mm(x, y.t())
return dist
| 2.5625 | 3 |
tests/__init__.py | eloo/sensor.sbahn_munich | 0 | 15 | """Tests for the sbahn_munich integration"""
line_dict = {
"name": "S3",
"color": "#333333",
"text_color": "#444444",
}
| 1.023438 | 1 |
app/views/web/homestack.py | geudrik/hautomation | 0 | 16 | <reponame>geudrik/hautomation
#! /usr/bin/env python2.7
# -*- coding: latin-1 -*-
from flask import Blueprint
from flask import current_app
from flask import render_template
from flask_login import login_required
homestack = Blueprint("homestack", __name__, url_prefix="/homestack")
@homestack.route("/", methods=["GET"])
@login_required
def home():
return render_template("homestack/home.html")
| 2.375 | 2 |
readthedocs/donate/forms.py | gamearming/readthedocs | 0 | 17 | """Forms for RTD donations"""
import logging
from django import forms
from django.conf import settings
from django.utils.translation import ugettext_lazy as _
from readthedocs.payments.forms import StripeModelForm, StripeResourceMixin
from readthedocs.payments.utils import stripe
from .models import Supporter
log = logging.getLogger(__name__)
class SupporterForm(StripeResourceMixin, StripeModelForm):
"""Donation support sign up form
This extends the basic payment form, giving fields for credit card number,
expiry, and CVV. The proper Knockout data bindings are established on
:py:class:`StripeModelForm`
"""
class Meta:
model = Supporter
fields = (
'last_4_digits',
'name',
'email',
'dollars',
'logo_url',
'site_url',
'public',
)
labels = {
'public': _('Make this donation public'),
}
help_texts = {
'public': _('Your name and image will be displayed on the donation page'),
'email': _('Your email is used for Gravatar and so we can send you a receipt'),
'logo_url': _("URL of your company's logo, images should be 300x300 pixels or less"),
'dollars': _('Companies donating over $400 can specify a logo URL and site link'),
}
widgets = {
'dollars': forms.HiddenInput(attrs={
'data-bind': 'value: dollars'
}),
'logo_url': forms.TextInput(attrs={
'data-bind': 'value: logo_url, enable: urls_enabled'
}),
'site_url': forms.TextInput(attrs={
'data-bind': 'value: site_url, enable: urls_enabled'
}),
'last_4_digits': forms.TextInput(attrs={
'data-bind': 'valueInit: card_digits, value: card_digits'
}),
}
last_4_digits = forms.CharField(widget=forms.HiddenInput(), required=True)
name = forms.CharField(required=True)
email = forms.CharField(required=True)
def __init__(self, *args, **kwargs):
self.user = kwargs.pop('user')
super(SupporterForm, self).__init__(*args, **kwargs)
def validate_stripe(self):
"""Call stripe for payment (not ideal here) and clean up logo < $200"""
dollars = self.cleaned_data['dollars']
if dollars < 200:
self.cleaned_data['logo_url'] = None
self.cleaned_data['site_url'] = None
stripe.Charge.create(
amount=int(self.cleaned_data['dollars']) * 100,
currency='usd',
source=self.cleaned_data['stripe_token'],
description='Read the Docs Sustained Engineering',
receipt_email=self.cleaned_data['email']
)
def save(self, commit=True):
supporter = super(SupporterForm, self).save(commit)
if commit and self.user is not None and self.user.is_authenticated():
supporter.user = self.user
supporter.save()
return supporter
class EthicalAdForm(StripeResourceMixin, StripeModelForm):
"""Payment form for ethical ads
This extends the basic payment form, giving fields for credit card number,
expiry, and CVV. The proper Knockout data bindings are established on
:py:class:`StripeModelForm`
"""
class Meta:
model = Supporter
fields = (
'last_4_digits',
'name',
'email',
'dollars',
)
help_texts = {
'email': _('Your email is used so we can send you a receipt'),
}
widgets = {
'dollars': forms.HiddenInput(attrs={
'data-bind': 'value: dollars'
}),
'last_4_digits': forms.TextInput(attrs={
'data-bind': 'valueInit: card_digits, value: card_digits'
}),
}
last_4_digits = forms.CharField(widget=forms.HiddenInput(), required=True)
name = forms.CharField(required=True)
email = forms.CharField(required=True)
def validate_stripe(self):
stripe.Charge.create(
amount=int(self.cleaned_data['dollars']) * 100,
currency='usd',
source=self.cleaned_data['stripe_token'],
description='Read the Docs Sponsorship Payment',
receipt_email=self.cleaned_data['email']
)
| 2.359375 | 2 |
pandas_datareaders_unofficial/datareaders/google_finance_options.py | movermeyer/pandas_datareaders_unofficial | 18 | 18 | #!/usr/bin/env python
# -*- coding: utf-8 -*-
from .base import DataReaderBase
from ..tools import COL, _get_dates, to_float, to_int
import pandas as pd
#from pandas.tseries.frequencies import to_offset
from six.moves import cStringIO as StringIO
import logging
import traceback
import datetime
import json
import token, tokenize
def ymd_to_date(y, m, d):
"""
Returns date
>>> expiration = {u'd': 1, u'm': 12, u'y': 2014}
>>> ymd_to_date(**expiration)
datetime.date(2014, 12, 1)
>>> ymd_to_date(2014, 3, 1)
datetime.date(2014, 3, 1)
"""
return(datetime.date(year=y, month=m, day=d))
def date_to_ymd(date):
"""
Returns dict like {'y': ..., 'm': ..., 'd': ...}
>>> date_to_ymd(datetime.date(year=2010, month=1, day=3))
{'y': 2010, 'm': 1, 'd': 3}
"""
d = {
'y': date.year,
'm': date.month,
'd': date.day
}
return(d)
def fix_lazy_json(in_text):
"""
Handle lazy JSON - to fix expecting property name
this function fixes the json output from google
http://stackoverflow.com/questions/4033633/handling-lazy-json-in-python-expecting-property-name
"""
tokengen = tokenize.generate_tokens(StringIO(in_text).readline)
result = []
for tokid, tokval, _, _, _ in tokengen:
# fix unquoted strings
if (tokid == token.NAME):
if tokval not in ['true', 'false', 'null', '-Infinity', 'Infinity', 'NaN']:
tokid = token.STRING
tokval = u'"%s"' % tokval
# fix single-quoted strings
elif (tokid == token.STRING):
if tokval.startswith ("'"):
tokval = u'"%s"' % tokval[1:-1].replace ('"', '\\"')
# remove invalid commas
elif (tokid == token.OP) and ((tokval == '}') or (tokval == ']')):
if (len(result) > 0) and (result[-1][1] == ','):
result.pop()
# fix single-quoted strings
elif (tokid == token.STRING):
if tokval.startswith ("'"):
tokval = u'"%s"' % tokval[1:-1].replace ('"', '\\"')
result.append((tokid, tokval))
return tokenize.untokenize(result)
def json_decode(json_string):
try:
ret = json.loads(json_string)
except:
json_string = fix_lazy_json(json_string)
ret = json.loads(json_string)
return ret
class DataReaderGoogleFinanceOptions(DataReaderBase):
"""
DataReader to fetch data from Google Finance Options
see https://www.google.com/finance/option_chain
https://github.com/makmac213/python-google-option-chain
http://www.drtomstarke.com/index.php/option-chains-from-google-finance-api
"""
def init(self, *args, **kwargs):
self._get_multi = self._get_multi_todict
def _get_one(self, name, *args, **kwargs):
return(self._get_one_raw(name, 'All', 'json'))
def _get_one_raw(self, symbol, typ='All', output='json', y='2014', m='12', d='1'):
url = "https://www.google.com/finance/option_chain"
params = {
'q': symbol,
'type': typ,
'output': output,
}
data = self._get_content(url, params)
d = {}
lst = []
for typ in [u'puts', u'calls']:
df_typ = pd.DataFrame(data[typ])
df_typ['Type'] = typ
lst.append(df_typ)
del data[typ]
for i, expiration in enumerate(data['expirations']):
params = {
'q': symbol,
'output': output,
'expy': expiration['y'],
'expm': expiration['m'],
'expd': expiration['d'],
}
data = self._get_content(url, params)
for typ in [u'puts', u'calls']:
df_typ = pd.DataFrame(data[typ])
df_typ['Type'] = typ
lst.append(df_typ)
del data[typ]
lst.append(df_typ)
df = pd.concat(lst, axis=0, ignore_index=True)
d_cols = {
"a": "Ask",
"b": "Bid",
"p": "Last",
"strike": "Strike",
"expiry": "Expiry",
"vol": "Volume",
"name": "Name"
}
df = df.rename(columns=d_cols)
"""
d_cols = {
"a": "ask",
"b": "bid",
"c": "change",
"cid": "identity code",
"cp": "cp"
"cs": change direction. "chg" = up, "chr" = down, "chg"?
"e": # I think this tells us something about what country where the stock is traded. "OPRA" means USA.
"expiry": expiration date for this option
"name": I don't know. I have never seen a value for this
"oi": open interest. How many of these are currently being held by others.
See, http://www.investopedia.com/terms/o/openinterest.asp
"p": price, last
"s": option code.
Basically, Stock Symbol + 7 if mini option + date + "C" or "P" + price
"strike": "strike price for this option"
"vol": "the volume of options traded."
}
"""
for col in ['Ask', 'Bid', 'c', 'cp', 'Last', 'Strike']:
df[col] = df[col].map(to_float)
for col in ['Volume', 'oi', 'cid']:
df[col] = df[col].map(to_int)
df['Expiry'] = pd.to_datetime(df['Expiry'])
data['options'] = df
data['underlying_id'] = int(data['underlying_id'])
data['expiry'] = ymd_to_date(**data['expiry'])
for i, expiration in enumerate(data['expirations']):
data['expirations'][i] = ymd_to_date(**expiration)
#for col in ['Volume']:
# df[col] = df[col].fillna(0)
#d = {}
#d["options"] = df
#return(d)
return(data)
def _get_content(self, url, params):
#response = requests.get(url, params=params)
response = self.session.get(url, params=params)
if response.status_code == 200:
content_json = response.text
data = json_decode(content_json)
return(data)
if __name__ == "__main__":
import doctest
doctest.testmod()
| 2.6875 | 3 |
keras_textclassification/data_preprocess/generator_preprocess.py | Vail-qin/Keras-TextClassification | 1 | 19 | <reponame>Vail-qin/Keras-TextClassification
# !/usr/bin/python
# -*- coding: utf-8 -*-
# @time : 2019/11/2 21:08
# @author : Mo
# @function:
from keras_textclassification.data_preprocess.text_preprocess import load_json, save_json
from keras_textclassification.conf.path_config import path_model_dir
path_fast_text_model_vocab2index = path_model_dir + 'vocab2index.json'
path_fast_text_model_l2i_i2l = path_model_dir + 'l2i_i2l.json'
import numpy as np
import os
class PreprocessGenerator:
"""
数据预处理, 输入为csv格式, [label,ques]
"""
def __init__(self):
self.l2i_i2l = None
if os.path.exists(path_fast_text_model_l2i_i2l):
self.l2i_i2l = load_json(path_fast_text_model_l2i_i2l)
def prereocess_idx(self, pred):
if os.path.exists(path_fast_text_model_l2i_i2l):
pred_i2l = {}
i2l = self.l2i_i2l['i2l']
for i in range(len(pred)):
pred_i2l[i2l[str(i)]] = pred[i]
pred_i2l_rank = [sorted(pred_i2l.items(), key=lambda k: k[1], reverse=True)]
return pred_i2l_rank
else:
raise RuntimeError("path_fast_text_model_label2index is None")
def prereocess_pred_xid(self, pred):
if os.path.exists(path_fast_text_model_l2i_i2l):
pred_l2i = {}
l2i = self.l2i_i2l['l2i']
for i in range(len(pred)):
pred_l2i[pred[i]] = l2i[pred[i]]
pred_l2i_rank = [sorted(pred_l2i.items(), key=lambda k: k[1], reverse=True)]
return pred_l2i_rank
else:
raise RuntimeError("path_fast_text_model_label2index is None")
def preprocess_get_label_set(self, path):
# 首先获取label,set,即存在的具体类
label_set = set()
len_all = 0
file_csv = open(path, "r", encoding="utf-8")
for line in file_csv:
len_all += 1
if len_all > 1: # 第一条是标签'label,ques',不选择
line_sp = line.split(",")
label_org = str(line_sp[0]).strip().upper()
label_real = "NAN" if label_org=="" else label_org
label_set.add(label_real)
file_csv.close()
return label_set, len_all
def preprocess_label_ques_to_idx(self, embedding_type, batch_size, path, embed, rate=1):
label_set, len_all = self.preprocess_get_label_set(path)
# 获取label转index字典等, 如果label2index存在则不转换了, dev验证集合的时候用
if not os.path.exists(path_fast_text_model_l2i_i2l):
count = 0
label2index = {}
index2label = {}
for label_one in label_set:
label2index[label_one] = count
index2label[count] = label_one
count = count + 1
l2i_i2l = {}
l2i_i2l['l2i'] = label2index
l2i_i2l['i2l'] = index2label
save_json(l2i_i2l, path_fast_text_model_l2i_i2l)
else:
l2i_i2l = load_json(path_fast_text_model_l2i_i2l)
# 读取数据的比例
len_ql = int(rate * len_all)
if len_ql <= 500: # sample时候不生效,使得语料足够训练
len_ql = len_all
def process_line(line):
# 对每一条数据操作,获取label和问句index
line_sp = line.split(",")
ques = str(line_sp[1]).strip().upper()
label = str(line_sp[0]).strip().upper()
label = "NAN" if label == "" else label
que_embed = embed.sentence2idx(ques)
label_zeros = [0] * len(l2i_i2l['l2i'])
label_zeros[l2i_i2l['l2i'][label]] = 1
return que_embed, label_zeros
while True:
file_csv = open(path, "r", encoding="utf-8")
cout_all_line = 0
cnt = 0
x, y = [], []
# 跳出循环
if len_ql < cout_all_line:
break
for line in file_csv:
cout_all_line += 1
if cout_all_line > 1: # 第一条是标签'label,ques',不选择
x_line, y_line = process_line(line)
x.append(x_line)
y.append(y_line)
cnt += 1
if cnt == batch_size:
if embedding_type in ['bert', 'albert']:
x_, y_ = np.array(x), np.array(y)
x_1 = np.array([x[0] for x in x_])
x_2 = np.array([x[1] for x in x_])
x_all = [x_1, x_2]
elif embedding_type == 'xlnet':
x_, y_ = x, np.array(y)
x_1 = np.array([x[0][0] for x in x_])
x_2 = np.array([x[1][0] for x in x_])
x_3 = np.array([x[2][0] for x in x_])
x_all = [x_1, x_2, x_3]
else:
x_all, y_ = np.array(x), np.array(y)
cnt = 0
yield (x_all, y_)
x, y =[], []
file_csv.close()
print("preprocess_label_ques_to_idx ok")
| 2.46875 | 2 |
content/test/gpu/gpu_tests/pixel_expectations.py | metux/chromium-deb | 0 | 20 | # Copyright 2014 The Chromium Authors. All rights reserved.
# Use of this source code is governed by a BSD-style license that can be
# found in the LICENSE file.
from gpu_tests.gpu_test_expectations import GpuTestExpectations
# See the GpuTestExpectations class for documentation.
class PixelExpectations(GpuTestExpectations):
def SetExpectations(self):
# Sample Usage:
# self.Fail('Pixel_Canvas2DRedBox',
# ['mac', 'amd', ('nvidia', 0x1234)], bug=123)
# Seems to be flaky on the new AMD R7 240 drivers.
self.Flaky('Pixel_GpuRasterization_BlueBox',
['win', ('amd', 0x6613)], bug=653538)
# Software compositing is not supported on Android; so we skip these tests
# that disables gpu compositing on Android platforms.
self.Skip('Pixel_OffscreenCanvasUnaccelerated2D', ['android'])
self.Skip('Pixel_OffscreenCanvasUnaccelerated2DWorker', ['android'])
self.Skip('Pixel_OffscreenCanvasWebGLSoftwareCompositing', ['android'])
self.Skip('Pixel_OffscreenCanvasWebGLSoftwareCompositingWorker',
['android'])
self.Skip('Pixel_CanvasDisplayLinearRGBUnaccelerated2D', ['android'])
self.Fail('Pixel_ScissorTestWithPreserveDrawingBuffer',
['android'], bug=521588)
# TODO(ccameron) fix these on Mac Retina
self.Fail('Pixel_CSS3DBlueBox', ['mac'], bug=533690)
# TODO(vmiura) check / generate reference images for Android devices
self.Fail('Pixel_SolidColorBackground', ['mac', 'android'], bug=624256)
self.Fail('Pixel_OffscreenCanvasUnaccelerated2DGPUCompositingWorker',
['mac', ('nvidia', 0xfe9)], bug=706016)
self.Fail('Pixel_CSSFilterEffects',
['mac', ('nvidia', 0xfe9)], bug=690277)
# TODO(kbr): flakily timing out on this configuration.
self.Flaky('*', ['linux', 'intel', 'debug'], bug=648369)
self.Flaky('Pixel_Video_MP4', ['android', 'nvidia'], bug=716564)
# Flaky for unknown reasons only on macOS. Not planning to investigate
# further.
self.Flaky('Pixel_ScissorTestWithPreserveDrawingBuffer', ['mac'],
bug=660461)
self.Flaky('Pixel_OffscreenCanvas2DResizeOnWorker',
['win10', ('intel', 0x1912)], bug=690663)
# TODO(zakerinasab): check / generate reference images.
self.Fail('Pixel_Canvas2DUntagged', bug=713632)
self.Flaky('Pixel_OffscreenCanvasTransferBeforeStyleResize',
['mac', 'linux', 'win', 'android'], bug=735228)
self.Flaky('Pixel_OffscreenCanvasTransferAfterStyleResize',
['mac', 'linux', 'win', 'android'], bug=735171)
# TODO(junov): update reference images
self.Fail('Pixel_CSSFilterEffects', ['mac'], bug=721727)
self.Fail('Pixel_CSSFilterEffects_NoOverlays', ['mac'], bug=721727)
# TODO(dshwang): remove these after new reference images are generated.
self.Fail('Pixel_DirectComposition_Video_MP4', bug=615325)
self.Fail('Pixel_DirectComposition_Video_VP9', bug=615325)
self.Fail('Pixel_Video_MP4', bug=615325)
self.Fail('Pixel_Video_VP9', bug=615325)
| 1.90625 | 2 |
examples/p02_budgets/budget_data_ingest/migrations/0001_initial.py | 18F/data-federation-ingest | 18 | 21 | <filename>examples/p02_budgets/budget_data_ingest/migrations/0001_initial.py
# -*- coding: utf-8 -*-
# Generated by Django 1.11.13 on 2018-06-08 22:54
from __future__ import unicode_literals
from django.conf import settings
import django.contrib.postgres.fields.jsonb
from django.db import migrations, models
import django.db.models.deletion
class Migration(migrations.Migration):
initial = True
dependencies = [
migrations.swappable_dependency(settings.AUTH_USER_MODEL),
]
operations = [
migrations.CreateModel(
name='BudgetItem',
fields=[
('id', models.AutoField(auto_created=True, primary_key=True, serialize=False, verbose_name='ID')),
('year', models.IntegerField()),
('agency', models.TextField()),
('data_source', models.TextField()),
('category', models.TextField()),
('dollars_budgeted', models.DecimalField(decimal_places=2, max_digits=14)),
('dollars_spent', models.DecimalField(decimal_places=2, max_digits=14)),
('row_number', models.IntegerField()),
],
),
migrations.CreateModel(
name='Upload',
fields=[
('id', models.AutoField(auto_created=True, primary_key=True, serialize=False, verbose_name='ID')),
('created_at', models.DateTimeField(auto_now_add=True)),
('updated_at', models.DateTimeField(auto_now=True)),
('file_metadata', django.contrib.postgres.fields.jsonb.JSONField(null=True)),
('file', models.FileField(upload_to='')),
('raw', models.BinaryField(null=True)),
('validation_results', django.contrib.postgres.fields.jsonb.JSONField(null=True)),
('status', models.CharField(choices=[('LOADING', 'Loading'), ('PENDING', 'Pending'), ('STAGED', 'Staged'), ('INSERTED', 'Inserted'), ('DELETED', 'Deleted')], default='LOADING', max_length=10)),
('status_changed_at', models.DateTimeField(null=True)),
('replaces', models.ForeignKey(null=True, on_delete=django.db.models.deletion.CASCADE, related_name='replaced_by', to='budget_data_ingest.Upload')),
('status_changed_by', models.ForeignKey(null=True, on_delete=django.db.models.deletion.CASCADE, related_name='+', to=settings.AUTH_USER_MODEL)),
('submitter', models.ForeignKey(on_delete=django.db.models.deletion.CASCADE, to=settings.AUTH_USER_MODEL)),
],
options={
'abstract': False,
},
),
migrations.AddField(
model_name='budgetitem',
name='upload',
field=models.ForeignKey(on_delete=django.db.models.deletion.CASCADE, to='budget_data_ingest.Upload'),
),
]
| 1.6875 | 2 |
setup.py | Kaslanarian/PythonSVM | 2 | 22 | import setuptools #enables develop
setuptools.setup(
name='pysvm',
version='0.1',
description='PySVM : A NumPy implementation of SVM based on SMO algorithm',
author_email="<EMAIL>",
packages=['pysvm'],
license='MIT License',
long_description=open('README.md', encoding='utf-8').read(),
install_requires=[ #自动安装依赖
'numpy', 'sklearn'
],
url='https://github.com/Kaslanarian/PySVM',
)
| 0.933594 | 1 |
Object_detection_image.py | hiperus0988/pyao | 1 | 23 | <gh_stars>1-10
######## Image Object Detection Using Tensorflow-trained Classifier #########
#
# Author: <NAME>
# Date: 1/15/18
# Description:
# This program uses a TensorFlow-trained classifier to perform object detection.
# It loads the classifier uses it to perform object detection on an image.
# It draws boxes and scores around the objects of interest in the image.
## Some of the code is copied from Google's example at
## https://github.com/tensorflow/models/blob/master/research/object_detection/object_detection_tutorial.ipynb
## and some is copied from Dat Tran's example at
## https://github.com/datitran/object_detector_app/blob/master/object_detection_app.py
## but I changed it to make it more understandable to me.
# Import packages
import os
import cv2
import numpy as np
import tensorflow as tf
import sys
# This is needed since the notebook is stored in the object_detection folder.
sys.path.append("..")
# Import utilites
from utils import label_map_util
from utils import visualization_utils as vis_util
# Name of the directory containing the object detection module we're using
MODEL_NAME = 'inference_graph'
IMAGE_NAME = 'test1.jpg'
# Grab path to current working directory
CWD_PATH = os.getcwd()
# Path to frozen detection graph .pb file, which contains the model that is used
# for object detection.
PATH_TO_CKPT = os.path.join(CWD_PATH,MODEL_NAME,'frozen_inference_graph.pb')
# Path to label map file
PATH_TO_LABELS = os.path.join(CWD_PATH,'training','labelmap.pbtxt')
# Path to image
PATH_TO_IMAGE = os.path.join(CWD_PATH,IMAGE_NAME)
# Number of classes the object detector can identify
NUM_CLASSES = 6
# Load the label map.
# Label maps map indices to category names, so that when our convolution
# network predicts `5`, we know that this corresponds to `king`.
# Here we use internal utility functions, but anything that returns a
# dictionary mapping integers to appropriate string labels would be fine
label_map = label_map_util.load_labelmap(PATH_TO_LABELS)
categories = label_map_util.convert_label_map_to_categories(label_map, max_num_classes=NUM_CLASSES, use_display_name=True)
category_index = label_map_util.create_category_index(categories)
# Load the Tensorflow model into memory.
detection_graph = tf.Graph()
with detection_graph.as_default():
od_graph_def = tf.GraphDef()
with tf.gfile.GFile(PATH_TO_CKPT, 'rb') as fid:
serialized_graph = fid.read()
od_graph_def.ParseFromString(serialized_graph)
tf.import_graph_def(od_graph_def, name='')
sess = tf.Session(graph=detection_graph)
# Define input and output tensors (i.e. data) for the object detection classifier
# Input tensor is the image
image_tensor = detection_graph.get_tensor_by_name('image_tensor:0')
# Output tensors are the detection boxes, scores, and classes
# Each box represents a part of the image where a particular object was detected
detection_boxes = detection_graph.get_tensor_by_name('detection_boxes:0')
# Each score represents level of confidence for each of the objects.
# The score is shown on the result image, together with the class label.
detection_scores = detection_graph.get_tensor_by_name('detection_scores:0')
detection_classes = detection_graph.get_tensor_by_name('detection_classes:0')
# Number of objects detected
num_detections = detection_graph.get_tensor_by_name('num_detections:0')
# Load image using OpenCV and
# expand image dimensions to have shape: [1, None, None, 3]
# i.e. a single-column array, where each item in the column has the pixel RGB value
image = cv2.imread(PATH_TO_IMAGE)
image_expanded = np.expand_dims(image, axis=0)
# Perform the actual detection by running the model with the image as input
(boxes, scores, classes, num) = sess.run(
[detection_boxes, detection_scores, detection_classes, num_detections],
feed_dict={image_tensor: image_expanded})
# Draw the results of the detection (aka 'visulaize the results')
vis_util.visualize_boxes_and_labels_on_image_array(
image,
np.squeeze(boxes),
np.squeeze(classes).astype(np.int32),
np.squeeze(scores),
category_index,
use_normalized_coordinates=True,
line_thickness=8,
min_score_thresh=0.60)
# All the results have been drawn on image. Now display the image.
cv2.imshow('Object detector', image)
# Press any key to close the image
cv2.waitKey(0)
# Clean up
cv2.destroyAllWindows()
| 3 | 3 |
polling_stations/apps/data_collection/management/commands/import_torbay.py | chris48s/UK-Polling-Stations | 0 | 24 | from data_collection.management.commands import BaseXpressDemocracyClubCsvImporter
class Command(BaseXpressDemocracyClubCsvImporter):
council_id = 'E06000027'
addresses_name = 'parl.2017-06-08/Version 1/Torbay Democracy_Club__08June2017.tsv'
stations_name = 'parl.2017-06-08/Version 1/Torbay Democracy_Club__08June2017.tsv'
elections = ['parl.2017-06-08']
csv_delimiter = '\t'
| 1.71875 | 2 |
Backend/product/views.py | Bhavya0020/Readopolis | 0 | 25 | from django.db.models import Q
from django.shortcuts import render
from django.http import Http404
# Create your views here.
from rest_framework.views import APIView
from rest_framework.response import Response
from rest_framework.decorators import api_view
from .models import Product, Category
from .serializers import ProductSerializer, CategorySerializer
class LatestProductsList(APIView):
def get(self, request, format=None):
products = Product.objects.all()[0:4]
serializer = ProductSerializer(products,many=True)
return Response(serializer.data)
class ProductDetail(APIView):
def get_object(self, category_slug, product_slug):
try:
return Product.objects.filter(category__slug=category_slug).get(slug=product_slug)
except Product.DoesNotExist:
raise Http404
def get(self, request, category_slug, product_slug, format= None):
product = self.get_object(category_slug, product_slug)
serializer = ProductSerializer(product)
return Response(serializer.data)
class CategoryDetail(APIView):
def get_object(self, category_slug):
try:
return Category.objects.get(slug=category_slug)
except Category.DoesNotExist:
raise Http404
def get(self, request, category_slug, format= None):
category = self.get_object(category_slug)
serializer = CategorySerializer(category)
return Response(serializer.data)
@api_view(['POST'])
def search(request):
query = request.data.get('query', '')
if query:
products = Product.objects.filter(Q(name__icontains=query) | Q(description__icontains=query))
serializer = ProductSerializer(products, many=True)
return Response(serializer.data)
else:
return Response({"products": []}) | 2.046875 | 2 |
model/contact.py | hubogeri/python_training | 0 | 26 | from sys import maxsize
class Contact:
def __init__(self, fname=None, mname=None, lname=None, nick=None, title=None, comp=None, addr=None,
home=None, mobile=None, work=None, fax=None, email1=None, email2=None, email3=None,
homepage=None, bday=None, bmonth=None, byear=None, aday=None, amonth=None, ayear=None,
secaddr=None, secphone=None, note=None, id =None):
self.fname = fname
self.mname = mname
self.lname = lname
self.nick = nick
self.title = title
self.comp = comp
self.addr = addr
self.home = home
self.mobile = mobile
self.work = work
self.fax = fax
self.email1 = email1
self.email2 = email2
self.email3 = email3
self.homepage = homepage
self.bday = bday
self.bmonth = bmonth
self.byear = byear
self.aday = aday
self.amonth = amonth
self.ayear = ayear
self.secaddr = secaddr
self.secphone = secphone
self.note = note
self.id = id
def __repr__(self):
return "%s:%s:%s" % (self.id, self.fname, self.lname)
def __eq__(self, other):
return (self.id is None or other.id is None or self.id == other.id) and self.fname == other.fname and self.lname == other.lname
def id_or_max(self):
if self.id:
return int(self.id)
else:
return maxsize
| 3.109375 | 3 |
test/IECore/BasicPreset.py | ericmehl/cortex | 386 | 27 | ##########################################################################
#
# Copyright (c) 2010-2012, Image Engine Design Inc. All rights reserved.
#
# Redistribution and use in source and binary forms, with or without
# modification, are permitted provided that the following conditions are
# met:
#
# * Redistributions of source code must retain the above copyright
# notice, this list of conditions and the following disclaimer.
#
# * Redistributions in binary form must reproduce the above copyright
# notice, this list of conditions and the following disclaimer in the
# documentation and/or other materials provided with the distribution.
#
# * Neither the name of Image Engine Design nor the names of any
# other contributors to this software may be used to endorse or
# promote products derived from this software without specific prior
# written permission.
#
# THIS SOFTWARE IS PROVIDED BY THE COPYRIGHT HOLDERS AND CONTRIBUTORS "AS
# IS" AND ANY EXPRESS OR IMPLIED WARRANTIES, INCLUDING, BUT NOT LIMITED TO,
# THE IMPLIED WARRANTIES OF MERCHANTABILITY AND FITNESS FOR A PARTICULAR
# PURPOSE ARE DISCLAIMED. IN NO EVENT SHALL THE COPYRIGHT OWNER OR
# CONTRIBUTORS BE LIABLE FOR ANY DIRECT, INDIRECT, INCIDENTAL, SPECIAL,
# EXEMPLARY, OR CONSEQUENTIAL DAMAGES (INCLUDING, BUT NOT LIMITED TO,
# PROCUREMENT OF SUBSTITUTE GOODS OR SERVICES; LOSS OF USE, DATA, OR
# PROFITS; OR BUSINESS INTERRUPTION) HOWEVER CAUSED AND ON ANY THEORY OF
# LIABILITY, WHETHER IN CONTRACT, STRICT LIABILITY, OR TORT (INCLUDING
# NEGLIGENCE OR OTHERWISE) ARISING IN ANY WAY OUT OF THE USE OF THIS
# SOFTWARE, EVEN IF ADVISED OF THE POSSIBILITY OF SUCH DAMAGE.
#
##########################################################################
from __future__ import with_statement
import os
import sys
import shutil
import unittest
import IECore
class TestBasicPreset( unittest.TestCase ) :
def testCopy( self ) :
testObj = IECore.Parameterised( "testParameterised1" )
testObj.parameters().addParameters(
[
IECore.BoolParameter( "a", "", True ),
IECore.FloatParameter( "b", "", 1.0 ),
]
)
testObj2 = IECore.Parameterised( "testParameterised2" )
testObj2.parameters().addParameters(
[
IECore.BoolParameter( "a", "", False ),
IECore.FloatParameter( "c", "", 0.0 ),
]
)
p = IECore.BasicPreset( testObj, testObj.parameters() )
self.assertTrue( p.applicableTo( testObj, testObj.parameters() ) )
self.assertFalse( p.applicableTo( testObj2, testObj2.parameters() ) )
testObj.parameters()["a"].setTypedValue( False )
testObj.parameters()["b"].setTypedValue( 0.0 )
p( testObj, testObj.parameters() )
self.assertEqual( testObj.parameters()["a"].getTypedValue(), True )
self.assertEqual( testObj.parameters()["b"].getTypedValue(), 1.0 )
p2 = IECore.BasicPreset( testObj, testObj.parameters(), parameters=( testObj.parameters()["a"], ) )
self.assertTrue( p2.applicableTo( testObj, testObj.parameters() ) )
self.assertTrue( p2.applicableTo( testObj2, testObj.parameters() ) )
p2( testObj2, testObj2.parameters() )
self.assertEqual( testObj2.parameters()["a"].getTypedValue(), True )
self.assertEqual( testObj2.parameters()["c"].getTypedValue(), 0.0 )
def testLoad( self ) :
testObj = IECore.Parameterised( "testParameterised1" )
testObj.parameters().addParameters(
[
IECore.BoolParameter( "a", "", True ),
IECore.FloatParameter( "b", "", 1.0 ),
]
)
testObj2 = IECore.Parameterised( "testParameterised1" )
testObj2.parameters().addParameters(
[
IECore.BoolParameter( "a", "", False ),
IECore.FloatParameter( "c", "", 0.0 ),
]
)
savePath = os.path.abspath( os.path.join( os.path.dirname( __file__ ), "data", "basicPreset" ) )
messageHandler = IECore.CapturingMessageHandler()
with messageHandler :
p = IECore.BasicPreset( os.path.join( savePath, "basicPresetLoadTest", "basicPresetLoadTest-1.cob" ) )
self.assertEqual( len( messageHandler.messages ), 0 )
self.assertTrue( p.applicableTo( testObj, testObj.parameters() ) )
self.assertFalse( p.applicableTo( testObj2, testObj2.parameters() ) )
testObj.parameters()["a"].setTypedValue( False )
testObj.parameters()["b"].setTypedValue( 0.0 )
p( testObj, testObj.parameters() )
self.assertEqual( testObj.parameters()["a"].getTypedValue(), True )
self.assertEqual( testObj.parameters()["b"].getTypedValue(), 1.0 )
def testSave( self ) :
testObj = IECore.Parameterised( "testParameterised1" )
testObj.parameters().addParameters(
[
IECore.BoolParameter( "a", "", True ),
IECore.FloatParameter( "b", "", 1.0 ),
]
)
testObj2 = IECore.Parameterised( "testParameterised1" )
testObj2.parameters().addParameters(
[
IECore.BoolParameter( "a", "", False ),
IECore.FloatParameter( "c", "", 0.0 ),
]
)
savePath = os.path.abspath( os.path.join( os.path.dirname( __file__ ), "data", "basicPreset" ) )
preset = IECore.BasicPreset( testObj, testObj.parameters() )
# Save for the classLoader and check its there, we test the 'loadability' later...
preset.save( savePath, "basicPresetTest" )
self.assertTrue( os.path.isfile( os.path.join( savePath, "basicPresetTest", "basicPresetTest-1.cob" ) ) )
self.assertTrue( os.path.isfile( os.path.join( savePath, "basicPresetTest", "basicPresetTest-1.py" ) ) )
# save without the classLoader and check its there
preset.save( savePath, "basicPresetTest", classLoadable=False )
self.assertTrue( os.path.isfile( os.path.join( savePath, "basicPresetTest.cob" ) ) )
# reload
p = IECore.BasicPreset( os.path.join( savePath, "basicPresetTest.cob" ) )
self.assertTrue( p.applicableTo( testObj, testObj.parameters() ) )
self.assertFalse( p.applicableTo( testObj2, testObj2.parameters() ) )
testObj.parameters()["a"].setTypedValue( False )
testObj.parameters()["b"].setTypedValue( 0.0 )
p( testObj, testObj.parameters() )
self.assertEqual( testObj.parameters()["a"].getTypedValue(), True )
self.assertEqual( testObj.parameters()["b"].getTypedValue(), 1.0 )
preset2 = IECore.BasicPreset( testObj, testObj.parameters(), parameters=( testObj.parameters()["a"], ) )
preset2.save( savePath, "basicPresetTest2", classLoadable=False )
#reload
p2 = IECore.BasicPreset( os.path.join( savePath, "basicPresetTest2.cob" ) )
self.assertTrue( p2.applicableTo( testObj, testObj.parameters() ) )
self.assertTrue( p2.applicableTo( testObj2, testObj.parameters() ) )
p2( testObj2, testObj2.parameters() )
self.assertEqual( testObj2.parameters()["a"].getTypedValue(), True )
self.assertEqual( testObj2.parameters()["c"].getTypedValue(), 0.0 )
def testClassLoader( self ) :
testObj = IECore.Parameterised( "testParameterised1" )
testObj.parameters().addParameters(
[
IECore.BoolParameter( "a", "", True ),
IECore.FloatParameter( "b", "", 1.0 ),
]
)
savePath = os.path.abspath( os.path.join( os.path.dirname( __file__ ), "data", "basicPreset" ) )
preset = IECore.BasicPreset( testObj, testObj.parameters() )
preset.save( savePath, "basicPresetTestClassLoader" )
# make sure that no messages are emitted during loading
messageHandler = IECore.CapturingMessageHandler()
with messageHandler :
loader = IECore.ClassLoader( IECore.SearchPath( savePath ) )
p = loader.load( "basicPresetTestClassLoader" )()
self.assertEqual( len( messageHandler.messages ), 0 )
self.assertTrue( isinstance( p, IECore.BasicPreset ) )
p.metadata()
def testClasses( self ) :
testObj = IECore.Parameterised( "testParameterised1" )
testObj.parameters().addParameters(
[
IECore.BoolParameter( "a", "", True ),
IECore.ClassParameter( "b", "", "IECORE_OP_PATHS", os.path.join( "maths", "multiply" ), 2 ),
]
)
testObj2 = IECore.Parameterised( "testParameterised2" )
testObj2.parameters().addParameters(
[
IECore.ClassParameter( "c", "", "IECORE_OP_PATHS" ),
]
)
classes1 = testObj.parameters()["b"].getClass( True )
classes2 = testObj2.parameters()["c"].getClass( True )
self.assertNotEqual( classes1[1:], classes2[1:] )
p = IECore.BasicPreset( testObj, testObj.parameters()["b"] )
self.assertTrue( p.applicableTo( testObj, testObj.parameters()["b"] ) )
self.assertFalse( p.applicableTo( testObj, testObj.parameters() ) )
self.assertTrue( p.applicableTo( testObj2, testObj2.parameters()["c"] ) )
p( testObj2, testObj2.parameters()["c"] )
classes1 = testObj.parameters()["b"].getClass( True )
classes2 = testObj2.parameters()["c"].getClass( True )
self.assertEqual( classes1[1:], classes2[1:] )
def testClassVectors( self ) :
testObj = IECore.Parameterised( "testParameterised1" )
testObj.parameters().addParameters(
[
IECore.BoolParameter( "a", "", True ),
IECore.ClassVectorParameter( "b", "", "IECORE_OP_PATHS" ),
]
)
testObj.parameters()["b"].setClasses(
[
( "mult", os.path.join( "maths", "multiply" ), 2 ),
( "coIO", "compoundObjectInOut", 1 ),
]
)
testObj2 = IECore.Parameterised( "testParameterised2" )
testObj2.parameters().addParameters(
[
IECore.ClassVectorParameter( "c", "", "IECORE_OP_PATHS" ),
]
)
classes1 = [ c[1:] for c in testObj.parameters()["b"].getClasses( True ) ]
classes2 = [ c[1:] for c in testObj2.parameters()["c"].getClasses( True ) ]
self.assertNotEqual( classes1, classes2 )
p = IECore.BasicPreset( testObj, testObj.parameters()["b"] )
self.assertTrue( p.applicableTo( testObj, testObj.parameters()["b"] ) )
self.assertFalse( p.applicableTo( testObj, testObj.parameters() ) )
self.assertTrue( p.applicableTo( testObj2, testObj2.parameters()["c"] ) )
p( testObj2, testObj2.parameters()["c"] )
classes1 = [ c[1:] for c in testObj.parameters()["b"].getClasses( True ) ]
classes2 = [ c[1:] for c in testObj2.parameters()["c"].getClasses( True ) ]
self.assertEqual( classes1, classes2 )
def testCompoundVectorParameter( self ) :
p = IECore.Parameterised( "test" )
p.parameters().addParameters(
[
IECore.BoolParameter( "a", "", False ),
IECore.CompoundVectorParameter(
"c",
"",
members = [
IECore.StringVectorParameter( "s", "", IECore.StringVectorData() ),
IECore.BoolVectorParameter( "b", "", IECore.BoolVectorData() ),
]
)
]
)
p["c"]["s"].setValue( IECore.StringVectorData( [ "1", "2", "3" ] ) )
p["c"]["b"].setValue( IECore.BoolVectorData( [ True, False, True ] ) )
v = p.parameters().getValue().copy()
preset = IECore.BasicPreset( p, p.parameters() )
self.assertTrue( preset.applicableTo( p, p.parameters() ) )
p.parameters().setValue( p.parameters().defaultValue )
self.assertNotEqual( p.parameters().getValue(), v )
preset( p, p.parameters() )
self.assertEqual( p.parameters().getValue(), v )
def tearDown( self ) :
savePath = os.path.abspath( os.path.join( os.path.dirname( __file__ ), "data", "basicPreset" ) )
paths = (
os.path.join( savePath, "basicPresetTest" ),
os.path.join( savePath, "basicPresetTest.cob" ),
os.path.join( savePath, "basicPresetTest2.cob" ),
os.path.join( savePath, "basicPresetTestClassLoader" ),
)
for p in paths :
if os.path.isdir( p ) :
shutil.rmtree( p )
elif os.path.isfile( p ) :
os.remove( p )
if __name__ == "__main__":
unittest.main()
| 1.210938 | 1 |
rlpy/Domains/Pacman.py | imanolarrieta/RL | 1 | 28 | <filename>rlpy/Domains/Pacman.py
"""Pacman game domain."""
from rlpy.Tools import __rlpy_location__
from .Domain import Domain
from .PacmanPackage import layout, pacman, game, ghostAgents
from .PacmanPackage import graphicsDisplay
import numpy as np
from copy import deepcopy
import os
import time
__copyright__ = "Copyright 2013, RLPy http://acl.mit.edu/RLPy"
__credits__ = ["<NAME>", "<NAME>", "<NAME>",
"<NAME>", "<NAME>"]
__license__ = "BSD 3-Clause"
__author__ = "<NAME>"
class Pacman(Domain):
"""
Pacman domain, which acts as a wrapper for the Pacman implementation
from the BerkeleyX/CS188.1x course project 3.
**STATE:** The state vector has a series of dimensions:
* [2] The x and y coordinates of pacman
* [3 * ng] the x and y coordinates as well as the scare time of each ghost
("scare time" is how long the ghost remains scared after consuming a capsule.)
* [nf] binary variables indicating if a food is still on the board or not
* [nc] binary variables for each capsule indicating if it is still on the board or not
*nf* and *nc* are map-dependent, and *ng* can be set as a parameter.
Based on above, total dimensionality of state vector is map-dependent,
and given by (2 + 3*ng + nf + nc).
**ACTIONS:** Move Pacman [up, down, left, right, stay]
**REWARD:** See the Berkeley project website below for more info.
.. note::
The visualization runs as fast as your CPU will permit; to slow things
down so gameplay is actually visible, de-comment time.sleep()
in the showDomain() method.
**REFERENCE:** This domain is an RLPy wrapper for the implementation
from the `BerkeleyX/CS188.1x course project 3 <https://courses.edx.org/courses/BerkeleyX/CS188.1x/2013_Spring/courseware/Week_9/Project_3_Reinforcement/>`_
See the original `source code (zipped) <https://courses.edx.org/static/content-berkeley-cs188x~2013_Spring/projects/reinforcement/reinforcement.zip>`_
For more details of the domain see the original package in the `Domains/PacmanPackage` folder.
"""
_max_scared_time = 39
actions = ["Stop", "North", "East", "South", "West"]
actions_num = 5
episodeCap = 1000
#: location of layouts shipped with rlpy
default_layout_dir = os.path.join(
__rlpy_location__, "Domains", "PacmanPackage",
"layouts")
def __init__(self, noise=.1, timeout=30,
layoutFile=os.path.join(
default_layout_dir, 'trickyClassic.lay'),
numGhostAgents=1000):
"""
layoutFile:
filename of the map file
noise:
with this probability pacman makes a random move instead the one
specified by the action
"""
self.noise = noise
# Specifies which Pacman world you want
self.layoutFile = layoutFile
# Puts the file in line stripped format
layout_file_content = self._tryToLoad(self.layoutFile)
self.layout = layout.Layout(layout_file_content)
# Number of ghosts
self.numGhostAgents = numGhostAgents
# Intitializes Pacman game
self.game_state = pacman.GameState()
self.game_rules = pacman.ClassicGameRules(timeout)
self.layout_copy = deepcopy(self.layout)
self.game_state.data.initialize(self.layout_copy, self.numGhostAgents)
self.num_total_food = len(self.layout_copy.food.asList())
self.num_total_capsules = len(self.layout_copy.capsules)
self._defaultSettings()
self.restartGraphics = None
self.timerswitch = False
self.savedtimer = None
self.gameDisplay = None
self._set_statespace_limits()
super(Pacman, self).__init__()
def _set_statespace_limits(self):
# Makes an array of limits for each dimension in the state vector.
statespace_limits = []
# adds pacman x, y locations
statespace_limits.append([1, self.layout.width - 2])
statespace_limits.append([1, self.layout.height - 2])
# adds ghost x, y locations and scaredTimer (how long they can be
# eaten)
for ghost in self.game_state.data.agentStates[1:]:
statespace_limits.append([1, self.layout.width - 2])
statespace_limits.append([1, self.layout.height - 2])
statespace_limits.append([0, self._max_scared_time])
statespace_limits += [[0, 1]] * (
self.num_total_food + self.num_total_capsules)
self.statespace_limits = np.array(statespace_limits, dtype="float")
def _set_state(self, s):
"""
Takes a vector s and sets the internal game state used by the original
pacman package.
"""
# copies most recent state
data = self.game_state.data
agent_states = data.agentStates
# set pacman position
agent_states.configuration.pos = (s[0], s[1])
# set ghost position
num_ghosts = len(agent_states) - 1
for i in range(1, num_ghosts + 1):
part_s = s[(3 * i) - 1:3 * i]
agent_states[i].configuration.pos = (part_s[0], part_s[1])
agent_states[i].scaredTimer = part_s[2]
# set food and capsules locations
s_food = s[(num_ghosts + 1) * 3:]
x = 0
y = 0
i = 0
data.capsules = []
for char in str(self.layout_copy):
if char == ".":
data.food[x][y] = bool(s_food[i])
i += 1
elif char == "o":
coord = (x, self.layout_copy.height - y)
if s_food[i]:
data.capsules.append(coord)
i += 1
elif char == "\n":
y += 1
x = -1
x += 1
def _get_state(self):
"""
get the internal game state represented as a numpy array
"""
data = self.game_state.data
agent_states = self.game_state.data.agentStates
num_ghosts = len(agent_states) - 1
s = np.zeros(
2 + num_ghosts * 3 + self.num_total_food + self.num_total_capsules)
# get pacman position
s[:2] = agent_states[0].configuration.pos
# import ipdb; ipdb.set_trace()
# get ghost info
for i in range(num_ghosts):
s[2 + i * 3: 2 + i * 3 + 2] = agent_states[i + 1].configuration.pos
s[2 + i * 3 + 2] = agent_states[i + 1].scaredTimer
# get food and capsules status
i = 2 + num_ghosts * 3
x = 0
y = 0
for char in str(self.layout_copy):
if char == ".":
s[i] = data.food[x][y]
i += 1
elif char == "\n":
y += 1
x = -1
elif char == "o":
coord = (x, self.layout_copy.height - y)
if coord in data.capsules:
s[i] = 1.
i += 1
x += 1
return s
state = property(_get_state, _set_state)
def showDomain(self, a, s=None):
if s is not None:
errStr = 'ERROR: In Pacman.py, attempted to pass a state (s)'\
'to showDomain(); Pacman only supports internal states.'\
'If you do pass a state parameter, ensure it is set to None.'
raise Exception(errStr)
s = self.game_state
if self.gameDisplay is None:
self.gameDisplay = graphicsDisplay.PacmanGraphics()
self.gameDisplay.startGraphics(self)
self.gameDisplay.drawStaticObjects(s.data)
self.gameDisplay.drawAgentObjects(s.data)
elif self._cleanup_graphics:
self._cleanup_graphics = False
self.gameDisplay.removeAllFood()
self.gameDisplay.removeAllCapsules()
self.gameDisplay.food = self.gameDisplay.drawFood(
self.gameDisplay.layout.food)
self.gameDisplay.capsules = self.gameDisplay.drawCapsules(
self.gameDisplay.layout.capsules)
# converts s vector in pacman gamestate instance and updates
# the display every time pacman or a ghost moves.
# s.data.food is the correct food matrix
s.data.layout.food = s.data.food
for agent in range(len(s.data.agentStates)):
s.data._agentMoved = agent
self.gameDisplay.update(s.data)
s._foodEaten = None
s._capsuleEaten = None
# time.sleep(0.1) # Sleep for 0.1 sec
def step(self, a):
"""
Applies actions from outside the Pacman domain to the given state.
Internal states accounted for along with scoring and terminal checking.
Returns a tuple of form (reward, new state vector, terminal)
"""
if self.random_state.random_sample() < self.noise:
# Random Move
a = self.random_state.choice(self.possibleActions())
a = self.actions[a]
next_state_p = self.game_state.generateSuccessor(0, a)
next_state = next_state_p
# pacman performs action "a" in current state object
# pacman.PacmanRules.applyAction(self.game_state, a)
# pacman.GhostRules.checkDeath(self.game_state, 0)
# the ghosts move randomly
for i in range(1, len(self.game_state.data.agentStates)):
if next_state.isWin() or next_state.isLose():
break
ghostOptions = pacman.GhostRules.getLegalActions(next_state, i)
# TODO: use domain random stream
randomAction_ind = self.random_state.randint(len(ghostOptions))
randomAction = ghostOptions[randomAction_ind]
next_state = next_state.generateSuccessor(i, randomAction)
# keep track of eaten stuff for graphics (original code assumes
# graphics are updated after every agent's move)
next_state.data._foodEaten = next_state_p.data._foodEaten
next_state.data._capsuleEaten = next_state_p.data._capsuleEaten
# scoring in pacman
r = next_state.data.score - self.game_state.data.score
self.game_state = next_state
terminal = self.isTerminal()
return r, self._get_state(), terminal, self.possibleActions()
def s0(self):
"""
re-initializes internal states when an episode starts, returns a s vector
"""
self.game_state = pacman.GameState()
self.game_rules = pacman.ClassicGameRules(timeout=30)
self.layout_copy = deepcopy(self.layout)
self.game = self.game_rules.newGame(
self.layout_copy, pacman, self.ghosts, DummyGraphics(), self.beQuiet, catchExceptions=False)
self.game_state.data.initialize(self.layout_copy, self.numGhostAgents)
self._cleanup_graphics = True
return self.state, self.isTerminal(), self.possibleActions()
def possibleActions(self):
if self.isTerminal():
# somewhat hacky, but should not matter anyway, maybe clean up in
# the future
return np.array([0])
# makes an array of possible actions pacman can perform at any given
# state
possibleActions = []
possibleMoves = pacman.GameState.getLegalActions(
self.game_state, agentIndex=0)
for a in possibleMoves:
possibleActions.append(self.actions.index(a))
return np.array(possibleActions)
def isTerminal(self):
"""
Checks whether the game should terminate at the given state.
(Terminate for failure, ie eaten by ghost or out of time, and for
success, all food on map eaten.)
If game should terminate, returns the proper indication to step function.
Accounts for scoring changes in terminal states.
"""
return self.game_state.data._lose or self.game_state.data._win
def _defaultSettings(self):
self.ghostNum = 2
self.ghosts = [ghostAgents.RandomGhost(
game.Agent) for i in range(self.ghostNum)]
self.beQuiet = False
def _tryToLoad(self, fullname):
# used in getLayout function
f = open(fullname)
grid = [line.strip() for line in f]
f.close()
return grid
class DummyGraphics(object):
def initialize(self, *arg, **kwargs):
pass
def update(self, *arg, **kwargs):
pass
def finalize(self, *arg, **kwargs):
pass
| 2.6875 | 3 |
core/src/zeit/cms/settings/interfaces.py | rickdg/vivi | 5 | 29 | from zeit.cms.i18n import MessageFactory as _
import zope.interface
import zope.schema
class IGlobalSettings(zope.interface.Interface):
"""Global CMS settings."""
default_year = zope.schema.Int(
title=_("Default year"),
min=1900,
max=2100)
default_volume = zope.schema.Int(
title=_("Default volume"),
min=1,
max=54)
def get_working_directory(template):
"""Return the collection which is the main working directory.
template:
Template which will be filled with year and volume. In
``template`` the placeholders $year and $volume will be replaced.
Example: 'online/$year/$volume/foo'
If the respective collection does not exist, it will be created before
returning it.
"""
| 2.15625 | 2 |
abc/abc165/abc165e.py | c-yan/atcoder | 1 | 30 | <filename>abc/abc165/abc165e.py
N, M = map(int, input().split())
for i in range(1, M + 1):
if i % 2 == 1:
j = (i - 1) // 2
print(1 + j, M + 1 - j)
else:
j = (i - 2) // 2
print(M + 2 + j, 2 * M + 1 - j)
| 2.953125 | 3 |
setup.py | giggslam/python-messengerbot-sdk | 23 | 31 | <reponame>giggslam/python-messengerbot-sdk<filename>setup.py
#!/usr/bin/env python
# -*- coding: utf-8 -*-
# Licensed under the Apache License, Version 2.0 (the "License"); you may
# not use this file except in compliance with the License. You may obtain
# a copy of the License at
#
# http://www.apache.org/licenses/LICENSE-2.0
#
# Unless required by applicable law or agreed to in writing, software
# distributed under the License is distributed on an "AS IS" BASIS, WITHOUT
# WARRANTIES OR CONDITIONS OF ANY KIND, either express or implied. See the
# License for the specific language governing permissions and limitations
# under the License.
import re
import sys
from setuptools import setup
from setuptools.command.test import test as TestCommand
__version__ = ''
with open('facebookbot/__about__.py', 'r') as fd:
reg = re.compile(r'__version__ = [\'"]([^\'"]*)[\'"]')
for line in fd:
m = reg.match(line)
if m:
__version__ = m.group(1)
break
def _requirements():
with open('requirements.txt', 'r') as fd:
return [name.strip() for name in fd.readlines()]
with open('README.rst', 'r') as fd:
long_description = fd.read()
setup(
name="fbsdk",
version=__version__,
author="<NAME>",
author_email="<EMAIL>",
maintainer="<NAME>",
maintainer_email="<EMAIL>",
url="https://github.com/boompieman/fbsdk",
description="Facebook Messaging API SDK for Python",
long_description=long_description,
license='Apache License 2.0',
packages=[
"facebookbot", "facebookbot.models"
],
install_requires=_requirements(),
classifiers=[
"Development Status :: 5 - Production/Stable",
"License :: OSI Approved :: Apache Software License",
"Intended Audience :: Developers",
"Programming Language :: Python :: 3",
"Topic :: Software Development"
]
)
| 1.6875 | 2 |
src/transformers/models/mmbt/modeling_mmbt.py | MaximovaIrina/transformers | 1 | 32 | <gh_stars>1-10
# coding=utf-8
# Copyright (c) Facebook, Inc. and its affiliates.
# Copyright (c) HuggingFace Inc. team.
#
# Licensed under the Apache License, Version 2.0 (the "License");
# you may not use this file except in compliance with the License.
# You may obtain a copy of the License at
#
# http://www.apache.org/licenses/LICENSE-2.0
#
# Unless required by applicable law or agreed to in writing, software
# distributed under the License is distributed on an "AS IS" BASIS,
# WITHOUT WARRANTIES OR CONDITIONS OF ANY KIND, either express or implied.
# See the License for the specific language governing permissions and
# limitations under the License.
"""PyTorch MMBT model. """
import torch
from torch import nn
from torch.nn import CrossEntropyLoss, MSELoss
from ...file_utils import add_start_docstrings, add_start_docstrings_to_model_forward, replace_return_docstrings
from ...modeling_outputs import BaseModelOutputWithPooling, SequenceClassifierOutput
from ...modeling_utils import ModuleUtilsMixin
from ...utils import logging
logger = logging.get_logger(__name__)
_CONFIG_FOR_DOC = "MMBTConfig"
class ModalEmbeddings(nn.Module):
"""Generic Modal Embeddings which takes in an encoder, and a transformer embedding."""
def __init__(self, config, encoder, embeddings):
super().__init__()
self.config = config
self.encoder = encoder
self.proj_embeddings = nn.Linear(config.modal_hidden_size, config.hidden_size)
self.position_embeddings = embeddings.position_embeddings
self.token_type_embeddings = embeddings.token_type_embeddings
self.word_embeddings = embeddings.word_embeddings
self.LayerNorm = embeddings.LayerNorm
self.dropout = nn.Dropout(p=config.hidden_dropout_prob)
def forward(self, input_modal, start_token=None, end_token=None, position_ids=None, token_type_ids=None):
token_embeddings = self.proj_embeddings(self.encoder(input_modal))
seq_length = token_embeddings.size(1)
if start_token is not None:
start_token_embeds = self.word_embeddings(start_token)
seq_length += 1
token_embeddings = torch.cat([start_token_embeds.unsqueeze(1), token_embeddings], dim=1)
if end_token is not None:
end_token_embeds = self.word_embeddings(end_token)
seq_length += 1
token_embeddings = torch.cat([token_embeddings, end_token_embeds.unsqueeze(1)], dim=1)
if position_ids is None:
position_ids = torch.arange(seq_length, dtype=torch.long, device=input_modal.device)
position_ids = position_ids.unsqueeze(0).expand(input_modal.size(0), seq_length)
if token_type_ids is None:
token_type_ids = torch.zeros(
(input_modal.size(0), seq_length), dtype=torch.long, device=input_modal.device
)
position_embeddings = self.position_embeddings(position_ids)
token_type_embeddings = self.token_type_embeddings(token_type_ids)
embeddings = token_embeddings + position_embeddings + token_type_embeddings
embeddings = self.LayerNorm(embeddings)
embeddings = self.dropout(embeddings)
return embeddings
MMBT_START_DOCSTRING = r"""
MMBT model was proposed in [Supervised Multimodal Bitransformers for Classifying Images and Text](https://github.com/facebookresearch/mmbt) by <NAME>, <NAME>, <NAME>, <NAME>.
It's a supervised multimodal bitransformer model that fuses information from text and other image encoders, and
obtain state-of-the-art performance on various multimodal classification benchmark tasks.
This model inherits from [`PreTrainedModel`]. Check the superclass documentation for the generic
methods the library implements for all its model (such as downloading or saving, resizing the input embeddings,
pruning heads etc.)
This model is also a PyTorch [torch.nn.Module](https://pytorch.org/docs/stable/nn.html#torch.nn.Module)
subclass. Use it as a regular PyTorch Module and refer to the PyTorch documentation for all matter related to
general usage and behavior.
Parameters:
config ([`MMBTConfig`]): Model configuration class with all the parameters of the model.
Initializing with a config file does not load the weights associated with the model, only the
configuration.
transformer (:class: *~nn.Module*): A text transformer that is used by MMBT.
It should have embeddings, encoder, and pooler attributes.
encoder (:class: *~nn.Module*): Encoder for the second modality.
It should take in a batch of modal inputs and return k, n dimension embeddings.
"""
MMBT_INPUTS_DOCSTRING = r"""
Args:
input_modal (`torch.FloatTensor` of shape `(batch_size, ***)`):
The other modality data. It will be the shape that the encoder for that type expects. e.g. With an Image
Encoder, the shape would be (batch_size, channels, height, width)
input_ids (`torch.LongTensor` of shape `(batch_size, sequence_length)`):
Indices of input sequence tokens in the vocabulary. It does not expect [CLS] token to be added as it's
appended to the end of other modality embeddings. Indices can be obtained using
[`BertTokenizer`]. See [`PreTrainedTokenizer.encode`] and
[`PreTrainedTokenizer.__call__`] for details.
[What are input IDs?](../glossary#input-ids)
modal_start_tokens (`torch.LongTensor` of shape `(batch_size,)`, *optional*):
Optional start token to be added to Other Modality Embedding. [CLS] Most commonly used for classification
tasks.
modal_end_tokens (`torch.LongTensor` of shape `(batch_size,)`, *optional*):
Optional end token to be added to Other Modality Embedding. [SEP] Most commonly used.
attention_mask (*optional*) `torch.FloatTensor` of shape `(batch_size, sequence_length)`:
Mask to avoid performing attention on padding token indices. Mask values selected in `[0, 1]`:
- 1 for tokens that are **not masked**,
- 0 for tokens that are **masked**.
[What are attention masks?](../glossary#attention-mask)
token_type_ids (*optional*) `torch.LongTensor` of shape `(batch_size, sequence_length)`:
Segment token indices to indicate first and second portions of the inputs. Indices are selected in `[0, 1]`:
- 0 corresponds to a *sentence A* token,
- 1 corresponds to a *sentence B* token.
[What are token type IDs?](../glossary#token-type-ids)
modal_token_type_ids (*optional*) `torch.LongTensor` of shape `(batch_size, modal_sequence_length)`:
Segment token indices to indicate different portions of the non-text modality. The embeddings from these
tokens will be summed with the respective token embeddings for the non-text modality.
position_ids (`torch.LongTensor` of shape `(batch_size, sequence_length)`, *optional*):
Indices of positions of each input sequence tokens in the position embeddings. Selected in the range `[0, config.max_position_embeddings - 1]`.
[What are position IDs?](../glossary#position-ids)
modal_position_ids (`torch.LongTensor` of shape `(batch_size, modal_sequence_length)`, *optional*):
Indices of positions of each input sequence tokens in the position embeddings for the non-text modality.
Selected in the range `[0, config.max_position_embeddings - 1]`.
[What are position IDs?](../glossary#position-ids)
head_mask (`torch.FloatTensor` of shape `(num_heads,)` or `(num_layers, num_heads)`, *optional*):
Mask to nullify selected heads of the self-attention modules. Mask values selected in `[0, 1]`:
- 1 indicates the head is **not masked**,
- 0 indicates the head is **masked**.
inputs_embeds (`torch.FloatTensor` of shape `(batch_size, sequence_length, embedding_dim)`, *optional*):
Optionally, instead of passing `input_ids` you can choose to directly pass an embedded representation.
This is useful if you want more control over how to convert `input_ids` indices into associated
vectors than the model's internal embedding lookup matrix.
encoder_hidden_states (`torch.FloatTensor` of shape `(batch_size, sequence_length, hidden_size)`, *optional*):
Sequence of hidden-states at the output of the last layer of the encoder. Used in the cross-attention if
the model is configured as a decoder.
encoder_attention_mask (`torch.FloatTensor` of shape `(batch_size, sequence_length)`, *optional*):
Mask to avoid performing attention on the padding token indices of the encoder input. This mask is used in
the cross-attention if the model is configured as a decoder. Mask values selected in `[0, 1]`:
- 1 for tokens that are **not masked**,
- 0 for tokens that are **masked**.
output_attentions (`bool`, *optional*):
Whether or not to return the attentions tensors of all attention layers. See `attentions` under returned
tensors for more detail.
output_hidden_states (`bool`, *optional*):
Whether or not to return the hidden states of all layers. See `hidden_states` under returned tensors for
more detail.
return_dict (`bool`, *optional*):
Whether or not to return a [`~file_utils.ModelOutput`] instead of a plain tuple.
"""
@add_start_docstrings(
"The bare MMBT Model outputting raw hidden-states without any specific head on top.",
MMBT_START_DOCSTRING,
)
class MMBTModel(nn.Module, ModuleUtilsMixin):
def __init__(self, config, transformer, encoder):
super().__init__()
self.config = config
self.transformer = transformer
self.modal_encoder = ModalEmbeddings(config, encoder, transformer.embeddings)
@add_start_docstrings_to_model_forward(MMBT_INPUTS_DOCSTRING)
@replace_return_docstrings(output_type=BaseModelOutputWithPooling, config_class=_CONFIG_FOR_DOC)
def forward(
self,
input_modal,
input_ids=None,
modal_start_tokens=None,
modal_end_tokens=None,
attention_mask=None,
token_type_ids=None,
modal_token_type_ids=None,
position_ids=None,
modal_position_ids=None,
head_mask=None,
inputs_embeds=None,
encoder_hidden_states=None,
encoder_attention_mask=None,
output_attentions=None,
output_hidden_states=None,
return_dict=None,
):
r"""
Returns:
Examples::
# For example purposes. Not runnable.
transformer = BertModel.from_pretrained('bert-base-uncased')
encoder = ImageEncoder(args)
mmbt = MMBTModel(config, transformer, encoder)
"""
output_attentions = output_attentions if output_attentions is not None else self.config.output_attentions
output_hidden_states = (
output_hidden_states if output_hidden_states is not None else self.config.output_hidden_states
)
return_dict = return_dict if return_dict is not None else self.config.use_return_dict
if input_ids is not None and inputs_embeds is not None:
raise ValueError("You cannot specify both input_ids and inputs_embeds at the same time")
elif input_ids is not None:
input_txt_shape = input_ids.size()
elif inputs_embeds is not None:
input_txt_shape = inputs_embeds.size()[:-1]
else:
raise ValueError("You have to specify either input_ids or inputs_embeds")
device = input_ids.device if input_ids is not None else inputs_embeds.device
modal_embeddings = self.modal_encoder(
input_modal,
start_token=modal_start_tokens,
end_token=modal_end_tokens,
position_ids=modal_position_ids,
token_type_ids=modal_token_type_ids,
)
input_modal_shape = modal_embeddings.size()[:-1]
if token_type_ids is None:
token_type_ids = torch.ones(input_txt_shape, dtype=torch.long, device=device)
txt_embeddings = self.transformer.embeddings(
input_ids=input_ids, position_ids=position_ids, token_type_ids=token_type_ids, inputs_embeds=inputs_embeds
)
embedding_output = torch.cat([modal_embeddings, txt_embeddings], 1)
input_shape = embedding_output.size()[:-1]
if attention_mask is None:
attention_mask = torch.ones(input_shape, device=device)
else:
attention_mask = torch.cat(
[torch.ones(input_modal_shape, device=device, dtype=torch.long), attention_mask], dim=1
)
if encoder_attention_mask is None:
encoder_attention_mask = torch.ones(input_shape, device=device)
else:
encoder_attention_mask = torch.cat(
[torch.ones(input_modal_shape, device=device), encoder_attention_mask], dim=1
)
extended_attention_mask = self.get_extended_attention_mask(attention_mask, input_shape, self.device)
encoder_extended_attention_mask = self.invert_attention_mask(encoder_attention_mask)
head_mask = self.get_head_mask(head_mask, self.config.num_hidden_layers)
encoder_outputs = self.transformer.encoder(
embedding_output,
attention_mask=extended_attention_mask,
head_mask=head_mask,
encoder_hidden_states=encoder_hidden_states,
encoder_attention_mask=encoder_extended_attention_mask,
output_attentions=output_attentions,
output_hidden_states=output_hidden_states,
return_dict=return_dict,
)
sequence_output = encoder_outputs[0]
pooled_output = self.transformer.pooler(sequence_output)
if not return_dict:
return (sequence_output, pooled_output) + encoder_outputs[1:]
return BaseModelOutputWithPooling(
last_hidden_state=sequence_output,
pooler_output=pooled_output,
hidden_states=encoder_outputs.hidden_states,
attentions=encoder_outputs.attentions,
)
def get_input_embeddings(self):
return self.embeddings.word_embeddings
def set_input_embeddings(self, value):
self.embeddings.word_embeddings = value
@add_start_docstrings(
"""
MMBT Model with a sequence classification/regression head on top (a linear layer on top of the pooled output)
""",
MMBT_START_DOCSTRING,
MMBT_INPUTS_DOCSTRING,
)
class MMBTForClassification(nn.Module):
r"""
**labels**: (*optional*) `torch.LongTensor` of shape `(batch_size,)`:
Labels for computing the sequence classification/regression loss. Indices should be in `[0, ..., config.num_labels - 1]`. If `config.num_labels == 1` a regression loss is computed (Mean-Square loss),
If `config.num_labels > 1` a classification loss is computed (Cross-Entropy).
Returns: *Tuple* comprising various elements depending on the configuration (config) and inputs: **loss**:
(*optional*, returned when `labels` is provided) `torch.FloatTensor` of shape `(1,)`: Classification (or
regression if config.num_labels==1) loss. **logits**: `torch.FloatTensor` of shape `(batch_size, config.num_labels)` Classification (or regression if config.num_labels==1) scores (before SoftMax).
**hidden_states**: (*optional*, returned when `output_hidden_states=True`) list of `torch.FloatTensor` (one for
the output of each layer + the output of the embeddings) of shape `(batch_size, sequence_length, hidden_size)`:
Hidden-states of the model at the output of each layer plus the initial embedding outputs. **attentions**:
(*optional*, returned when `output_attentions=True`) list of `torch.FloatTensor` (one for each layer) of shape
`(batch_size, num_heads, sequence_length, sequence_length)`: Attentions weights after the attention softmax, used
to compute the weighted average in the self-attention heads.
Examples:
```python
# For example purposes. Not runnable.
transformer = BertModel.from_pretrained('bert-base-uncased')
encoder = ImageEncoder(args)
model = MMBTForClassification(config, transformer, encoder)
outputs = model(input_modal, input_ids, labels=labels)
loss, logits = outputs[:2]
```"""
def __init__(self, config, transformer, encoder):
super().__init__()
self.num_labels = config.num_labels
self.mmbt = MMBTModel(config, transformer, encoder)
self.dropout = nn.Dropout(config.hidden_dropout_prob)
self.classifier = nn.Linear(config.hidden_size, config.num_labels)
def forward(
self,
input_modal,
input_ids=None,
modal_start_tokens=None,
modal_end_tokens=None,
attention_mask=None,
token_type_ids=None,
modal_token_type_ids=None,
position_ids=None,
modal_position_ids=None,
head_mask=None,
inputs_embeds=None,
labels=None,
return_dict=None,
):
return_dict = return_dict if return_dict is not None else self.config.use_return_dict
outputs = self.mmbt(
input_modal=input_modal,
input_ids=input_ids,
modal_start_tokens=modal_start_tokens,
modal_end_tokens=modal_end_tokens,
attention_mask=attention_mask,
token_type_ids=token_type_ids,
modal_token_type_ids=modal_token_type_ids,
position_ids=position_ids,
modal_position_ids=modal_position_ids,
head_mask=head_mask,
inputs_embeds=inputs_embeds,
return_dict=return_dict,
)
pooled_output = outputs[1]
pooled_output = self.dropout(pooled_output)
logits = self.classifier(pooled_output)
loss = None
if labels is not None:
if self.num_labels == 1:
# We are doing regression
loss_fct = MSELoss()
loss = loss_fct(logits.view(-1), labels.view(-1))
else:
loss_fct = CrossEntropyLoss()
loss = loss_fct(logits.view(-1, self.num_labels), labels.view(-1))
if not return_dict:
output = (logits,) + outputs[2:]
return ((loss,) + output) if loss is not None else output
return SequenceClassifierOutput(
loss=loss,
logits=logits,
hidden_states=outputs.hidden_states,
attentions=outputs.attentions,
)
| 1.820313 | 2 |
eth2/beacon/chains/base.py | mhchia/trinity | 0 | 33 | <filename>eth2/beacon/chains/base.py
from abc import (
ABC,
abstractmethod,
)
import logging
from typing import (
TYPE_CHECKING,
Tuple,
Type,
)
from eth._utils.datatypes import (
Configurable,
)
from eth.db.backends.base import (
BaseAtomicDB,
)
from eth.exceptions import (
BlockNotFound,
)
from eth.validation import (
validate_word,
)
from eth_typing import (
Hash32,
)
from eth_utils import (
ValidationError,
encode_hex,
)
from eth2._utils.ssz import (
validate_imported_block_unchanged,
)
from eth2.beacon.db.chain import (
BaseBeaconChainDB,
BeaconChainDB,
)
from eth2.beacon.exceptions import (
BlockClassError,
StateMachineNotFound,
)
from eth2.beacon.types.blocks import (
BaseBeaconBlock,
)
from eth2.beacon.types.states import (
BeaconState,
)
from eth2.beacon.typing import (
FromBlockParams,
Slot,
)
from eth2.beacon.validation import (
validate_slot,
)
if TYPE_CHECKING:
from eth2.beacon.state_machines.base import ( # noqa: F401
BaseBeaconStateMachine,
)
class BaseBeaconChain(Configurable, ABC):
"""
The base class for all BeaconChain objects
"""
chaindb = None # type: BaseBeaconChainDB
chaindb_class = None # type: Type[BaseBeaconChainDB]
sm_configuration = None # type: Tuple[Tuple[Slot, Type[BaseBeaconStateMachine]], ...]
chain_id = None # type: int
#
# Helpers
#
@classmethod
@abstractmethod
def get_chaindb_class(cls) -> Type[BaseBeaconChainDB]:
pass
#
# Chain API
#
@classmethod
@abstractmethod
def from_genesis(cls,
base_db: BaseAtomicDB,
genesis_state: BeaconState,
genesis_block: BaseBeaconBlock) -> 'BaseBeaconChain':
pass
#
# State Machine API
#
@classmethod
@abstractmethod
def get_state_machine_class(
cls,
block: BaseBeaconBlock) -> Type['BaseBeaconStateMachine']:
pass
@abstractmethod
def get_state_machine(self, at_block: BaseBeaconBlock=None) -> 'BaseBeaconStateMachine':
pass
@classmethod
@abstractmethod
def get_state_machine_class_for_block_slot(
cls,
slot: Slot) -> Type['BaseBeaconStateMachine']:
pass
#
# Block API
#
@abstractmethod
def get_block_class(self, block_root: Hash32) -> Type[BaseBeaconBlock]:
pass
@abstractmethod
def create_block_from_parent(self,
parent_block: BaseBeaconBlock,
block_params: FromBlockParams) -> BaseBeaconBlock:
pass
@abstractmethod
def get_block_by_root(self, block_root: Hash32) -> BaseBeaconBlock:
pass
@abstractmethod
def get_canonical_head(self) -> BaseBeaconBlock:
pass
@abstractmethod
def get_score(self, block_root: Hash32) -> int:
pass
@abstractmethod
def ensure_block(self, block: BaseBeaconBlock=None) -> BaseBeaconBlock:
pass
@abstractmethod
def get_block(self) -> BaseBeaconBlock:
pass
@abstractmethod
def get_canonical_block_by_slot(self, slot: Slot) -> BaseBeaconBlock:
pass
@abstractmethod
def get_canonical_block_root(self, slot: Slot) -> Hash32:
pass
@abstractmethod
def import_block(
self,
block: BaseBeaconBlock,
perform_validation: bool=True
) -> Tuple[BaseBeaconBlock, Tuple[BaseBeaconBlock, ...], Tuple[BaseBeaconBlock, ...]]:
pass
class BeaconChain(BaseBeaconChain):
"""
A Chain is a combination of one or more ``StateMachine`` classes. Each ``StateMachine``
is associated with a range of slots. The Chain class acts as a wrapper around these other
StateMachine classes, delegating operations to the appropriate StateMachine depending on the
current block slot number.
"""
logger = logging.getLogger("eth2.beacon.chains.BeaconChain")
chaindb_class = BeaconChainDB # type: Type[BaseBeaconChainDB]
def __init__(self, base_db: BaseAtomicDB) -> None:
if not self.sm_configuration:
raise ValueError(
"The Chain class cannot be instantiated with an empty `sm_configuration`"
)
else:
# TODO implment validate_sm_configuration(self.sm_configuration)
# validate_sm_configuration(self.sm_configuration)
pass
self.chaindb = self.get_chaindb_class()(base_db)
#
# Helpers
#
@classmethod
def get_chaindb_class(cls) -> Type['BaseBeaconChainDB']:
if cls.chaindb_class is None:
raise AttributeError("`chaindb_class` not set")
return cls.chaindb_class
#
# Chain API
#
@classmethod
def from_genesis(cls,
base_db: BaseAtomicDB,
genesis_state: BeaconState,
genesis_block: BaseBeaconBlock) -> 'BaseBeaconChain':
"""
Initialize the ``BeaconChain`` from a genesis state.
"""
sm_class = cls.get_state_machine_class_for_block_slot(genesis_block.slot)
if type(genesis_block) != sm_class.block_class:
raise BlockClassError(
"Given genesis block class: {}, StateMachine.block_class: {}".format(
type(genesis_block),
sm_class.block_class
)
)
chaindb = cls.get_chaindb_class()(db=base_db)
chaindb.persist_state(genesis_state)
return cls._from_genesis_block(base_db, genesis_block)
@classmethod
def _from_genesis_block(cls,
base_db: BaseAtomicDB,
genesis_block: BaseBeaconBlock) -> 'BaseBeaconChain':
"""
Initialize the ``BeaconChain`` from the genesis block.
"""
chaindb = cls.get_chaindb_class()(db=base_db)
chaindb.persist_block(genesis_block, genesis_block.__class__)
return cls(base_db)
#
# StateMachine API
#
@classmethod
def get_state_machine_class(cls, block: BaseBeaconBlock) -> Type['BaseBeaconStateMachine']:
"""
Returns the ``StateMachine`` instance for the given block slot number.
"""
return cls.get_state_machine_class_for_block_slot(block.slot)
@classmethod
def get_state_machine_class_for_block_slot(
cls,
slot: Slot) -> Type['BaseBeaconStateMachine']:
"""
Return the ``StateMachine`` class for the given block slot number.
"""
if cls.sm_configuration is None:
raise AttributeError("Chain classes must define the StateMachines in sm_configuration")
validate_slot(slot)
for start_slot, sm_class in reversed(cls.sm_configuration):
if slot >= start_slot:
return sm_class
raise StateMachineNotFound("No StateMachine available for block slot: #{0}".format(slot))
def get_state_machine(self, at_block: BaseBeaconBlock=None) -> 'BaseBeaconStateMachine':
"""
Return the ``StateMachine`` instance for the given block number.
"""
block = self.ensure_block(at_block)
sm_class = self.get_state_machine_class_for_block_slot(block.slot)
return sm_class(
chaindb=self.chaindb,
block=block,
)
#
# Block API
#
def get_block_class(self, block_root: Hash32) -> Type[BaseBeaconBlock]:
slot = self.chaindb.get_slot_by_root(block_root)
sm_class = self.get_state_machine_class_for_block_slot(slot)
block_class = sm_class.block_class
return block_class
def create_block_from_parent(self,
parent_block: BaseBeaconBlock,
block_params: FromBlockParams) -> BaseBeaconBlock:
"""
Passthrough helper to the ``StateMachine`` class of the block descending from the
given block.
"""
return self.get_state_machine_class_for_block_slot(
slot=parent_block.slot + 1 if block_params.slot is None else block_params.slot,
).create_block_from_parent(parent_block, block_params)
def get_block_by_root(self, block_root: Hash32) -> BaseBeaconBlock:
"""
Return the requested block as specified by block hash.
Raise ``BlockNotFound`` if there's no block with the given hash in the db.
"""
validate_word(block_root, title="Block Hash")
block_class = self.get_block_class(block_root)
return self.chaindb.get_block_by_root(block_root, block_class)
def get_canonical_head(self) -> BaseBeaconBlock:
"""
Return the block at the canonical chain head.
Raise ``CanonicalHeadNotFound`` if there's no head defined for the canonical chain.
"""
block_root = self.chaindb.get_canonical_head_root()
block_class = self.get_block_class(block_root)
return self.chaindb.get_block_by_root(block_root, block_class)
def get_score(self, block_root: Hash32) -> int:
"""
Return the score of the block with the given hash.
Raise ``BlockNotFound`` if there is no matching black hash.
"""
return self.chaindb.get_score(block_root)
def ensure_block(self, block: BaseBeaconBlock=None) -> BaseBeaconBlock:
"""
Return ``block`` if it is not ``None``, otherwise return the block
of the canonical head.
"""
if block is None:
head = self.get_canonical_head()
return self.create_block_from_parent(head, FromBlockParams())
else:
return block
def get_block(self) -> BaseBeaconBlock:
"""
Return the current TIP block.
"""
return self.get_state_machine().block
def get_canonical_block_by_slot(self, slot: Slot) -> BaseBeaconBlock:
"""
Return the block with the given number in the canonical chain.
Raise ``BlockNotFound`` if there's no block with the given number in the
canonical chain.
"""
validate_slot(slot)
return self.get_block_by_root(self.chaindb.get_canonical_block_root(slot))
def get_canonical_block_root(self, slot: Slot) -> Hash32:
"""
Return the block hash with the given number in the canonical chain.
Raise ``BlockNotFound`` if there's no block with the given number in the
canonical chain.
"""
return self.chaindb.get_canonical_block_root(slot)
def import_block(
self,
block: BaseBeaconBlock,
perform_validation: bool=True
) -> Tuple[BaseBeaconBlock, Tuple[BaseBeaconBlock, ...], Tuple[BaseBeaconBlock, ...]]:
"""
Import a complete block and returns a 3-tuple
- the imported block
- a tuple of blocks which are now part of the canonical chain.
- a tuple of blocks which were canonical and now are no longer canonical.
"""
try:
parent_block = self.get_block_by_root(block.previous_block_root)
except BlockNotFound:
raise ValidationError(
"Attempt to import block #{}. Cannot import block {} before importing "
"its parent block at {}".format(
block.slot,
block.signed_root,
block.previous_block_root,
)
)
base_block_for_import = self.create_block_from_parent(
parent_block,
FromBlockParams(),
)
state, imported_block = self.get_state_machine(base_block_for_import).import_block(block)
# Validate the imported block.
if perform_validation:
validate_imported_block_unchanged(imported_block, block)
# TODO: Now it just persists all state. Should design how to clean up the old state.
self.chaindb.persist_state(state)
(
new_canonical_blocks,
old_canonical_blocks,
) = self.chaindb.persist_block(imported_block, imported_block.__class__)
self.logger.debug(
'IMPORTED_BLOCK: slot %s | signed root %s',
imported_block.slot,
encode_hex(imported_block.signed_root),
)
return imported_block, new_canonical_blocks, old_canonical_blocks
| 2.1875 | 2 |
StarCoder Training Dataset Cleaned and Scored
This dataset is a filtered version of StarCoder Training Dataset
that has been scored with the python-edu-scorer
.
@misc{allal2024SmolLM,
title={SmolLM - blazingly fast and remarkably powerful},
author={Loubna Ben Allal and Anton Lozhkov and Elie Bakouch and Leandro von Werra and Thomas Wolf},
year={2024},
}
@article{li2023starcoder,
title={StarCoder: may the source be with you!},
author={Raymond Li and Loubna Ben Allal and Yangtian Zi and Niklas Muennighoff and Denis Kocetkov and Chenghao Mou and Marc Marone and Christopher Akiki and Jia Li and Jenny Chim and Qian Liu and Evgenii Zheltonozhskii and Terry Yue Zhuo and Thomas Wang and Olivier Dehaene and Mishig Davaadorj and Joel Lamy-Poirier and João Monteiro and Oleh Shliazhko and Nicolas Gontier and Nicholas Meade and Armel Zebaze and Ming-Ho Yee and Logesh Kumar Umapathi and Jian Zhu and Benjamin Lipkin and Muhtasham Oblokulov and Zhiruo Wang and Rudra Murthy and Jason Stillerman and Siva Sankalp Patel and Dmitry Abulkhanov and Marco Zocca and Manan Dey and Zhihan Zhang and Nour Fahmy and Urvashi Bhattacharyya and Wenhao Yu and Swayam Singh and Sasha Luccioni and Paulo Villegas and Maxim Kunakov and Fedor Zhdanov and Manuel Romero and Tony Lee and Nadav Timor and Jennifer Ding and Claire Schlesinger and Hailey Schoelkopf and Jan Ebert and Tri Dao and Mayank Mishra and Alex Gu and Jennifer Robinson and Carolyn Jane Anderson and Brendan Dolan-Gavitt and Danish Contractor and Siva Reddy and Daniel Fried and Dzmitry Bahdanau and Yacine Jernite and Carlos Muñoz Ferrandis and Sean Hughes and Thomas Wolf and Arjun Guha and Leandro von Werra and Harm de Vries},
year={2023},
eprint={2305.06161},
archivePrefix={arXiv},
primaryClass={cs.CL}
}