|
--- |
|
language: en |
|
tags: |
|
- exbert |
|
license: mit |
|
datasets: |
|
- bookcorpus |
|
- wikipedia |
|
--- |
|
|
|
# Data2Vec-Text base model |
|
|
|
Pretrained model on English language using the *data2vec* objective. It was introduced in |
|
[this paper](https://arxiv.org/abs/2202.03555) and first released in |
|
[this repository](https://github.com/pytorch/fairseq/tree/main/examples/data2vec). This model is case-sensitive: it |
|
makes a difference between english and English. |
|
|
|
Disclaimer: The team releasing Data2Vec-Text did not write a model card for this model so this model card has been written by |
|
the Hugging Face team. |
|
|
|
## Pre-Training method |
|
|
|
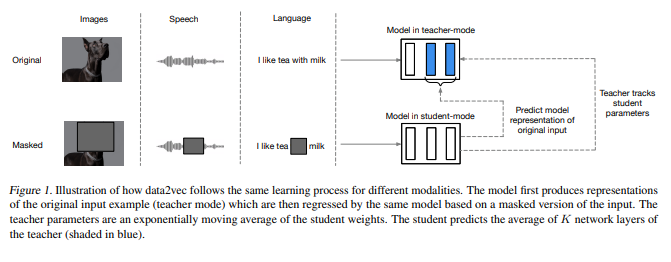 |
|
|
|
For more information, please take a look at the [official paper](https://arxiv.org/abs/2202.03555). |
|
|
|
## Abstract |
|
|
|
*While the general idea of self-supervised learning is identical across modalities, the actual algorithms and objectives differ widely because |
|
they were developed with a single modality in |
|
mind. To get us closer to general self-supervised |
|
learning, we present data2vec, a framework that |
|
uses the same learning method for either speech, |
|
NLP or computer vision. The core idea is to predict latent representations of the full input data |
|
based on a masked view of the input in a selfdistillation setup using a standard Transformer architecture. Instead of predicting modality-specific |
|
targets such as words, visual tokens or units of |
|
human speech which are local in nature, data2vec |
|
predicts contextualized latent representations that |
|
contain information from the entire input. Experiments on the major benchmarks of speech |
|
recognition, image classification, and natural language understanding demonstrate a new state of |
|
the art or competitive performance to predominant approaches.* |
|
|
|
## Intended uses & limitations |
|
|
|
The model is intended to be fine-tuned on a downstream task. |
|
See the [model hub](https://huggingface.co/models?filter=data2vec-text) to look for fine-tuned versions on a task that |
|
interests you. |
|
|
|
Note that this model is primarily aimed at being fine-tuned on tasks that use the whole sentence (potentially masked) |
|
to make decisions, such as sequence classification, token classification or question answering. For tasks such as text |
|
generation you should look at model like GPT2. |
|
|
|
## Training data |
|
|
|
The RoBERTa model was pretrained on the reunion of five datasets: |
|
- [BookCorpus](https://yknzhu.wixsite.com/mbweb), a dataset consisting of 11,038 unpublished books; |
|
- [English Wikipedia](https://en.wikipedia.org/wiki/English_Wikipedia) (excluding lists, tables and headers) ; |
|
- [CC-News](https://commoncrawl.org/2016/10/news-dataset-available/), a dataset containing 63 millions English news |
|
articles crawled between September 2016 and February 2019. |
|
- [OpenWebText](https://github.com/jcpeterson/openwebtext), an opensource recreation of the WebText dataset used to |
|
train GPT-2, |
|
- [Stories](https://arxiv.org/abs/1806.02847) a dataset containing a subset of CommonCrawl data filtered to match the |
|
story-like style of Winograd schemas. |
|
|
|
Together theses datasets weight 160GB of text. |
|
|
|
### BibTeX entry and citation info |
|
|
|
```bibtex |
|
@misc{https://doi.org/10.48550/arxiv.2202.03555, |
|
doi = {10.48550/ARXIV.2202.03555}, |
|
url = {https://arxiv.org/abs/2202.03555}, |
|
author = {Baevski, Alexei and Hsu, Wei-Ning and Xu, Qiantong and Babu, Arun and Gu, Jiatao and Auli, Michael}, |
|
keywords = {Machine Learning (cs.LG), FOS: Computer and information sciences, FOS: Computer and information sciences}, |
|
title = {data2vec: A General Framework for Self-supervised Learning in Speech, Vision and Language}, |
|
publisher = {arXiv}, |
|
year = {2022}, |
|
copyright = {arXiv.org perpetual, non-exclusive license} |
|
} |
|
``` |