ml4floods trained models
This repository contains the trained models of the publication:
E. Portalés-Julià, G. Mateo-García, C. Purcell, and L. Gómez-Chova Global flood extent segmentation in optical satellite images. Scientific Reports 13, 20316 (2023). DOI: 10.1038/s41598-023-47595-7.
We include the trained models:
- Unet multioutput in folder
models/WF2_unetv2_all
- Unet multioutput S2-to-L8 in folder
models/WF2_unetv2_bgriswirs
- Unet multioutput RGBNIR in folder
models/WF2_unetv2_rgbi
The following table shows the performance of the models in the test dataset:
In order to run any of these models in a Landsat or Sentinel-2 scene see the tutorial Inference with clouds aware floods segmentation model in the ml4floods docs.
If you find this work useful please cite:
@article{portales-julia_global_2023,
title = {Global flood extent segmentation in optical satellite images},
volume = {13},
issn = {2045-2322},
doi = {10.1038/s41598-023-47595-7},
number = {1},
urldate = {2023-11-30},
journal = {Scientific Reports},
author = {Portalés-Julià, Enrique and Mateo-García, Gonzalo and Purcell, Cormac and Gómez-Chova, Luis},
month = nov,
year = {2023},
pages = {20316},
}
Licence
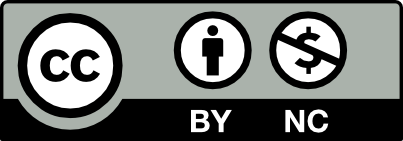
All pre-trained models in this repository are released under a Creative Commons non-commercial licence
The ML4Floods python package is published under a GNU Lesser GPL v3 licence
Acknowledgments
This research has been supported by the DEEPCLOUD project (PID2019-109026RB-I00) funded by the Spanish Ministry of Science and Innovation (MCIN/AEI/10.13039/501100011033) and the European Union (NextGenerationEU).