You can also experiment with these models using my Monte Carlo Tree Search generation pipeline:
https://github.com/mkurman/mcts-pytorch
Mariusz Kurman PRO
mkurman
AI & ML interests
AI Tech Lead | MD
Recent Activity
replied to
their
post
about 5 hours ago
ReasonFlow ๐ง
Are you fascinated by reasoning models? If so, you won't want to miss my latest project! I've implemented multiple path generations to supercharge the reasoning capabilities of O1-like models. Explore how this work can elevate your model in complex reasoning tasks!
https://github.com/mkurman/ReasonFlow
Use it with:
https://huggingface.co/mkurman/phi4-MedIT-10B-o1
- or -
https://huggingface.co/mkurman/llama-3.2-MEDIT-3B-o1
posted
an
update
about 6 hours ago
ReasonFlow ๐ง
Are you fascinated by reasoning models? If so, you won't want to miss my latest project! I've implemented multiple path generations to supercharge the reasoning capabilities of O1-like models. Explore how this work can elevate your model in complex reasoning tasks!
https://github.com/mkurman/ReasonFlow
Use it with:
https://huggingface.co/mkurman/phi4-MedIT-10B-o1
- or -
https://huggingface.co/mkurman/llama-3.2-MEDIT-3B-o1
updated
a model
about 6 hours ago
mkurman/phi4-MedIT-10B-o1
Organizations
mkurman's activity
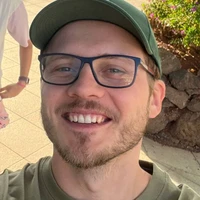
replied to
their
post
about 5 hours ago
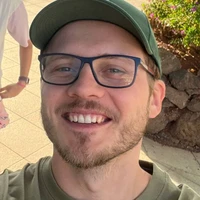
posted
an
update
about 6 hours ago
Post
128
ReasonFlow ๐ง
Are you fascinated by reasoning models? If so, you won't want to miss my latest project! I've implemented multiple path generations to supercharge the reasoning capabilities of O1-like models. Explore how this work can elevate your model in complex reasoning tasks!
https://github.com/mkurman/ReasonFlow
Use it with:
mkurman/phi4-MedIT-10B-o1
- or -
mkurman/llama-3.2-MEDIT-3B-o1
Are you fascinated by reasoning models? If so, you won't want to miss my latest project! I've implemented multiple path generations to supercharge the reasoning capabilities of O1-like models. Explore how this work can elevate your model in complex reasoning tasks!
https://github.com/mkurman/ReasonFlow
Use it with:
mkurman/phi4-MedIT-10B-o1
- or -
mkurman/llama-3.2-MEDIT-3B-o1
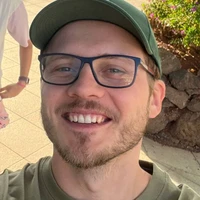
reacted to
Jaward's
post with ๐๐ฅ
7 days ago
Post
1336
Huge AI win in medicine๐
"Large language of life model" just dropped!!
Full paper: https://www.nature.com/articles/s41586-024-08391-z
"Large language of life model" just dropped!!
Full paper: https://www.nature.com/articles/s41586-024-08391-z
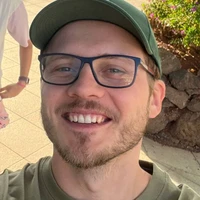
reacted to
prithivMLmods's
post with ๐๐ฅ
12 days ago
Post
5851
Reasoning SmolLM2 ๐
๐ฏFine-tuning SmolLM2 on a lightweight synthetic reasoning dataset for reasoning-specific tasks. Future updates will focus on lightweight, blazing-fast reasoning models. Until then, check out the blog for fine-tuning details.
๐ฅBlog : https://huggingface.co/blog/prithivMLmods/smollm2-ft
๐ผ Models :
+ SmolLM2-CoT-360M : prithivMLmods/SmolLM2-CoT-360M
+ Reasoning-SmolLM2-135M : prithivMLmods/Reasoning-SmolLM2-135M
+ SmolLM2-CoT-360M-GGUF : prithivMLmods/SmolLM2-CoT-360M-GGUF
๐ค Other Details :
+ Demo : prithivMLmods/SmolLM2-CoT-360M
+ Fine-tune nB : prithivMLmods/SmolLM2-CoT-360M
๐ฏFine-tuning SmolLM2 on a lightweight synthetic reasoning dataset for reasoning-specific tasks. Future updates will focus on lightweight, blazing-fast reasoning models. Until then, check out the blog for fine-tuning details.
๐ฅBlog : https://huggingface.co/blog/prithivMLmods/smollm2-ft
๐ผ Models :
+ SmolLM2-CoT-360M : prithivMLmods/SmolLM2-CoT-360M
+ Reasoning-SmolLM2-135M : prithivMLmods/Reasoning-SmolLM2-135M
+ SmolLM2-CoT-360M-GGUF : prithivMLmods/SmolLM2-CoT-360M-GGUF
๐ค Other Details :
+ Demo : prithivMLmods/SmolLM2-CoT-360M
+ Fine-tune nB : prithivMLmods/SmolLM2-CoT-360M
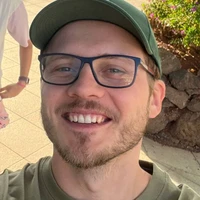
reacted to
openfree's
post with ๐ฅ
12 days ago
Post
5162
# ๐งฌ Protein Genesis AI: Design Proteins with Just a Prompt
## ๐ค Current Challenges in Protein Design
Traditional protein design faces critical barriers:
- ๐ฐ High costs ($1M - $10M+) & long development cycles (2-3 years)
- ๐ฌ Complex equipment and expert knowledge required
- ๐ Low success rates (<10%)
- โฐ Time-consuming experimental validation
## โจ Our Solution: Protein Genesis AI
Transform protein design through simple natural language input:
### Key Features
- ๐ค AI-powered automated design
- ๐ Real-time analysis & optimization
- ๐ฌ Instant 3D visualization
- ๐พ Immediate PDB file generation
## ๐ฏ Applications
### Medical & Industrial
- ๐ฅ Drug development
- ๐ Antibody design
- ๐ญ Industrial enzymes
- โป๏ธ Environmental solutions
### Research & Education
- ๐ฌ Basic research
- ๐ Educational tools
- ๐งซ Experimental design
- ๐ Data analysis
## ๐ซ Key Advantages
- ๐จโ๐ป No coding or technical expertise needed
- โก Results in minutes (vs. years)
- ๐ฐ 90% cost reduction
- ๐ Accessible anywhere
## ๐ Who Needs This?
- ๐ข Biotech companies
- ๐ฅ Pharmaceutical research
- ๐ Academic institutions
- ๐งช Research laboratories
## ๐ Why It Matters
Protein Genesis AI democratizes protein design by transforming complex processes into simple text prompts. This breakthrough accelerates scientific discovery, potentially leading to faster drug development and innovative biotechnology solutions. The future of protein design starts with a simple prompt! ๐
openfree/ProteinGenesis
## ๐ค Current Challenges in Protein Design
Traditional protein design faces critical barriers:
- ๐ฐ High costs ($1M - $10M+) & long development cycles (2-3 years)
- ๐ฌ Complex equipment and expert knowledge required
- ๐ Low success rates (<10%)
- โฐ Time-consuming experimental validation
## โจ Our Solution: Protein Genesis AI
Transform protein design through simple natural language input:
"Design a protein that targets cancer cells"
"Create an enzyme that breaks down plastic"
### Key Features
- ๐ค AI-powered automated design
- ๐ Real-time analysis & optimization
- ๐ฌ Instant 3D visualization
- ๐พ Immediate PDB file generation
## ๐ฏ Applications
### Medical & Industrial
- ๐ฅ Drug development
- ๐ Antibody design
- ๐ญ Industrial enzymes
- โป๏ธ Environmental solutions
### Research & Education
- ๐ฌ Basic research
- ๐ Educational tools
- ๐งซ Experimental design
- ๐ Data analysis
## ๐ซ Key Advantages
- ๐จโ๐ป No coding or technical expertise needed
- โก Results in minutes (vs. years)
- ๐ฐ 90% cost reduction
- ๐ Accessible anywhere
## ๐ Who Needs This?
- ๐ข Biotech companies
- ๐ฅ Pharmaceutical research
- ๐ Academic institutions
- ๐งช Research laboratories
## ๐ Why It Matters
Protein Genesis AI democratizes protein design by transforming complex processes into simple text prompts. This breakthrough accelerates scientific discovery, potentially leading to faster drug development and innovative biotechnology solutions. The future of protein design starts with a simple prompt! ๐
openfree/ProteinGenesis
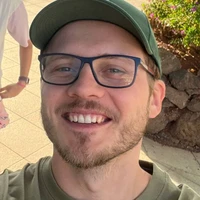
reacted to
singhsidhukuldeep's
post with ๐
12 days ago
Post
3390
Exciting breakthrough in e-commerce recommendation systems!
Walmart Global Tech researchers have developed a novel Triple Modality Fusion (TMF) framework that revolutionizes how we make product recommendations.
>> Key Innovation
The framework ingeniously combines three distinct data types:
- Visual data to capture product aesthetics and context
- Textual information for detailed product features
- Graph data to understand complex user-item relationships
>> Technical Architecture
The system leverages a Large Language Model (Llama2-7B) as its backbone and introduces several sophisticated components:
Modality Fusion Module
- All-Modality Self-Attention (AMSA) for unified representation
- Cross-Modality Attention (CMA) mechanism for deep feature integration
- Custom FFN adapters to align different modality embeddings
Advanced Training Strategy
- Curriculum learning approach with three complexity levels
- Parameter-Efficient Fine-Tuning using LoRA
- Special token system for behavior and item representation
>> Real-World Impact
The results are remarkable:
- 38.25% improvement in Electronics recommendations
- 43.09% boost in Sports category accuracy
- Significantly higher human evaluation scores compared to traditional methods
Currently deployed in Walmart's production environment, this research demonstrates how combining multiple data modalities with advanced LLM architectures can dramatically improve recommendation accuracy and user satisfaction.
Walmart Global Tech researchers have developed a novel Triple Modality Fusion (TMF) framework that revolutionizes how we make product recommendations.
>> Key Innovation
The framework ingeniously combines three distinct data types:
- Visual data to capture product aesthetics and context
- Textual information for detailed product features
- Graph data to understand complex user-item relationships
>> Technical Architecture
The system leverages a Large Language Model (Llama2-7B) as its backbone and introduces several sophisticated components:
Modality Fusion Module
- All-Modality Self-Attention (AMSA) for unified representation
- Cross-Modality Attention (CMA) mechanism for deep feature integration
- Custom FFN adapters to align different modality embeddings
Advanced Training Strategy
- Curriculum learning approach with three complexity levels
- Parameter-Efficient Fine-Tuning using LoRA
- Special token system for behavior and item representation
>> Real-World Impact
The results are remarkable:
- 38.25% improvement in Electronics recommendations
- 43.09% boost in Sports category accuracy
- Significantly higher human evaluation scores compared to traditional methods
Currently deployed in Walmart's production environment, this research demonstrates how combining multiple data modalities with advanced LLM architectures can dramatically improve recommendation accuracy and user satisfaction.
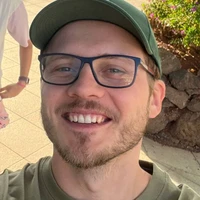
reacted to
Sri-Vigneshwar-DJ's
post with ๐ฅ
13 days ago
Post
2331
Combining smolagents with Anthropicโs best practices simplifies building powerful AI agents:
1. Code-Based Agents: Write actions as Python code, reducing steps by 30%.
2. Prompt Chaining: Break tasks into sequential subtasks with validation gates.
3. Routing: Classify inputs and direct them to specialized handlers.
4. Fallback: Handle tasks even if classification fails.
https://huggingface.co/blog/Sri-Vigneshwar-DJ/building-effective-agents-with-anthropics-best-pra
1. Code-Based Agents: Write actions as Python code, reducing steps by 30%.
2. Prompt Chaining: Break tasks into sequential subtasks with validation gates.
3. Routing: Classify inputs and direct them to specialized handlers.
4. Fallback: Handle tasks even if classification fails.
https://huggingface.co/blog/Sri-Vigneshwar-DJ/building-effective-agents-with-anthropics-best-pra
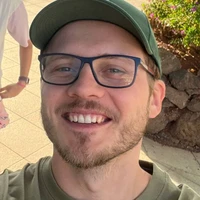
reacted to
ezgikorkmaz's
post with ๐ฅ
13 days ago
Post
1893
If you are interested in adversarial deep reinforcement learning find the compact reading list below:
https://github.com/EzgiKorkmaz/adversarial-reinforcement-learning
https://github.com/EzgiKorkmaz/adversarial-reinforcement-learning
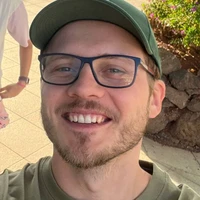
posted
an
update
13 days ago
Post
1882
I kindly invite you to try my experimental Llama 3.2 3B with o1-like thinking.
It utilizes Thoughts when needed, so don't be surprised when it's not. It also has a minor bug that requires further fine-tuning (sometimes it starts with the <|python_tag|> instead of <Thought>).
Enjoy!
Give some likes and whatever to make me feel better and motivated to keep going ๐
mkurman/llama-3.2-MEDIT-3B-o1
It utilizes Thoughts when needed, so don't be surprised when it's not. It also has a minor bug that requires further fine-tuning (sometimes it starts with the <|python_tag|> instead of <Thought>).
Enjoy!
Give some likes and whatever to make me feel better and motivated to keep going ๐
mkurman/llama-3.2-MEDIT-3B-o1
a runnable script to download all the dataset to colab or kaggle notebook
1
#1 opened 4 months ago
by
actualbrain
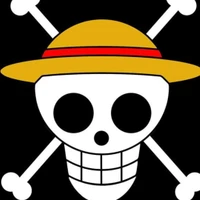
Add generated example
1
#3 opened about 1 month ago
by
mkurman
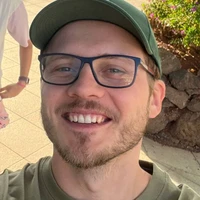