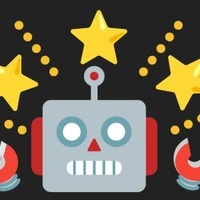
autoevaluator
HF staff
Add evaluation results on the 3.0.0 config and test split of cnn_dailymail
78d4ed5
language: | |
- en | |
license: | |
- apache-2.0 | |
- bsd-3-clause | |
tags: | |
- summarization | |
- led | |
- summary | |
- longformer | |
- booksum | |
- long-document | |
- long-form | |
datasets: | |
- kmfoda/booksum | |
metrics: | |
- rouge | |
widget: | |
- text: large earthquakes along a given fault segment do not occur at random intervals | |
because it takes time to accumulate the strain energy for the rupture. The rates | |
at which tectonic plates move and accumulate strain at their boundaries are approximately | |
uniform. Therefore, in first approximation, one may expect that large ruptures | |
of the same fault segment will occur at approximately constant time intervals. | |
If subsequent main shocks have different amounts of slip across the fault, then | |
the recurrence time may vary, and the basic idea of periodic mainshocks must be | |
modified. For great plate boundary ruptures the length and slip often vary by | |
a factor of 2. Along the southern segment of the San Andreas fault the recurrence | |
interval is 145 years with variations of several decades. The smaller the standard | |
deviation of the average recurrence interval, the more specific could be the long | |
term prediction of a future mainshock. | |
example_title: earthquakes | |
- text: ' A typical feed-forward neural field algorithm. Spatiotemporal coordinates | |
are fed into a neural network that predicts values in the reconstructed domain. | |
Then, this domain is mapped to the sensor domain where sensor measurements are | |
available as supervision. Class and Section Problems Addressed Generalization | |
(Section 2) Inverse problems, ill-posed problems, editability; symmetries. Hybrid | |
Representations (Section 3) Computation & memory efficiency, representation capacity, | |
editability: Forward Maps (Section 4) Inverse problems Network Architecture (Section | |
5) Spectral bias, integration & derivatives. Manipulating Neural Fields (Section | |
6) Edit ability, constraints, regularization. Table 2: The five classes of techniques | |
in the neural field toolbox each addresses problems that arise in learning, inference, | |
and control. (Section 3). We can supervise reconstruction via differentiable forward | |
maps that transform Or project our domain (e.g, 3D reconstruction via 2D images; | |
Section 4) With appropriate network architecture choices, we can overcome neural | |
network spectral biases (blurriness) and efficiently compute derivatives and integrals | |
(Section 5). Finally, we can manipulate neural fields to add constraints and regularizations, | |
and to achieve editable representations (Section 6). Collectively, these classes | |
constitute a ''toolbox'' of techniques to help solve problems with neural fields | |
There are three components in a conditional neural field: (1) An encoder or inference | |
function € that outputs the conditioning latent variable 2 given an observation | |
0 E(0) =2. 2 is typically a low-dimensional vector, and is often referred to aS | |
a latent code Or feature code_ (2) A mapping function 4 between Z and neural field | |
parameters O: Y(z) = O; (3) The neural field itself $. The encoder € finds the | |
most probable z given the observations O: argmaxz P(2/0). The decoder maximizes | |
the inverse conditional probability to find the most probable 0 given Z: arg- | |
max P(Olz). We discuss different encoding schemes with different optimality guarantees | |
(Section 2.1.1), both global and local conditioning (Section 2.1.2), and different | |
mapping functions Y (Section 2.1.3) 2. Generalization Suppose we wish to estimate | |
a plausible 3D surface shape given a partial or noisy point cloud. We need a suitable | |
prior over the sur- face in its reconstruction domain to generalize to the partial | |
observations. A neural network expresses a prior via the function space of its | |
architecture and parameters 0, and generalization is influenced by the inductive | |
bias of this function space (Section 5).' | |
example_title: scientific paper | |
- text: ' the big variety of data coming from diverse sources is one of the key properties | |
of the big data phenomenon. It is, therefore, beneficial to understand how data | |
is generated in various environments and scenarios, before looking at what should | |
be done with this data and how to design the best possible architecture to accomplish | |
this The evolution of IT architectures, described in Chapter 2, means that the | |
data is no longer processed by a few big monolith systems, but rather by a group | |
of services In parallel to the processing layer, the underlying data storage has | |
also changed and became more distributed This, in turn, required a significant | |
paradigm shift as the traditional approach to transactions (ACID) could no longer | |
be supported. On top of this, cloud computing is becoming a major approach with | |
the benefits of reducing costs and providing on-demand scalability but at the | |
same time introducing concerns about privacy, data ownership, etc In the meantime | |
the Internet continues its exponential growth: Every day both structured and unstructured | |
data is published and available for processing: To achieve competitive advantage | |
companies have to relate their corporate resources to external services, e.g. | |
financial markets, weather forecasts, social media, etc While several of the sites | |
provide some sort of API to access the data in a more orderly fashion; countless | |
sources require advanced web mining and Natural Language Processing (NLP) processing | |
techniques: Advances in science push researchers to construct new instruments | |
for observing the universe O conducting experiments to understand even better | |
the laws of physics and other domains. Every year humans have at their disposal | |
new telescopes, space probes, particle accelerators, etc These instruments generate | |
huge streams of data, which need to be stored and analyzed. The constant drive | |
for efficiency in the industry motivates the introduction of new automation techniques | |
and process optimization: This could not be done without analyzing the precise | |
data that describe these processes. As more and more human tasks are automated, | |
machines provide rich data sets, which can be analyzed in real-time to drive efficiency | |
to new levels. Finally, it is now evident that the growth of the Internet of Things | |
is becoming a major source of data. More and more of the devices are equipped | |
with significant computational power and can generate a continuous data stream | |
from their sensors. In the subsequent sections of this chapter, we will look at | |
the domains described above to see what they generate in terms of data sets. We | |
will compare the volumes but will also look at what is characteristic and important | |
from their respective points of view. 3.1 The Internet is undoubtedly the largest | |
database ever created by humans. While several well described; cleaned, and structured | |
data sets have been made available through this medium, most of the resources | |
are of an ambiguous, unstructured, incomplete or even erroneous nature. Still, | |
several examples in the areas such as opinion mining, social media analysis, e-governance, | |
etc, clearly show the potential lying in these resources. Those who can successfully | |
mine and interpret the Internet data can gain unique insight and competitive advantage | |
in their business An important area of data analytics on the edge of corporate | |
IT and the Internet is Web Analytics.' | |
example_title: data science textbook | |
- text: 'Transformer-based models have shown to be very useful for many NLP tasks. | |
However, a major limitation of transformers-based models is its O(n^2)O(n 2) time | |
& memory complexity (where nn is sequence length). Hence, it''s computationally | |
very expensive to apply transformer-based models on long sequences n > 512n>512. | |
Several recent papers, e.g. Longformer, Performer, Reformer, Clustered attention | |
try to remedy this problem by approximating the full attention matrix. You can | |
checkout 🤗''s recent blog post in case you are unfamiliar with these models. | |
BigBird (introduced in paper) is one of such recent models to address this issue. | |
BigBird relies on block sparse attention instead of normal attention (i.e. BERT''s | |
attention) and can handle sequences up to a length of 4096 at a much lower computational | |
cost compared to BERT. It has achieved SOTA on various tasks involving very long | |
sequences such as long documents summarization, question-answering with long contexts. | |
BigBird RoBERTa-like model is now available in 🤗Transformers. The goal of this | |
post is to give the reader an in-depth understanding of big bird implementation | |
& ease one''s life in using BigBird with 🤗Transformers. But, before going into | |
more depth, it is important to remember that the BigBird''s attention is an approximation | |
of BERT''s full attention and therefore does not strive to be better than BERT''s | |
full attention, but rather to be more efficient. It simply allows to apply transformer-based | |
models to much longer sequences since BERT''s quadratic memory requirement quickly | |
becomes unbearable. Simply put, if we would have ∞ compute & ∞ time, BERT''s attention | |
would be preferred over block sparse attention (which we are going to discuss | |
in this post). | |
If you wonder why we need more compute when working with longer sequences, this | |
blog post is just right for you! | |
Some of the main questions one might have when working with standard BERT-like | |
attention include: | |
Do all tokens really have to attend to all other tokens? Why not compute attention | |
only over important tokens? How to decide what tokens are important? How to attend | |
to just a few tokens in a very efficient way? In this blog post, we will try to | |
answer those questions. | |
What tokens should be attended to? We will give a practical example of how attention | |
works by considering the sentence ''BigBird is now available in HuggingFace for | |
extractive question answering''. In BERT-like attention, every word would simply | |
attend to all other tokens. | |
Let''s think about a sensible choice of key tokens that a queried token actually | |
only should attend to by writing some pseudo-code. Will will assume that the token | |
available is queried and build a sensible list of key tokens to attend to. | |
>>> # let''s consider following sentence as an example >>> example = [''BigBird'', | |
''is'', ''now'', ''available'', ''in'', ''HuggingFace'', ''for'', ''extractive'', | |
''question'', ''answering''] | |
>>> # further let''s assume, we''re trying to understand the representation of | |
''available'' i.e. >>> query_token = ''available'' >>> # We will initialize an | |
empty `set` and fill up the tokens of our interest as we proceed in this section. | |
>>> key_tokens = [] # => currently ''available'' token doesn''t have anything | |
to attend Nearby tokens should be important because, in a sentence (sequence of | |
words), the current word is highly dependent on neighboring past & future tokens. | |
This intuition is the idea behind the concept of sliding attention.' | |
example_title: bigbird blog intro | |
- text: 'The majority of available text summarization datasets include short-form | |
source documents that lack long-range causal and temporal dependencies, and often | |
contain strong layout and stylistic biases. While relevant, such datasets will | |
offer limited challenges for future generations of text summarization systems. | |
We address these issues by introducing BookSum, a collection of datasets for long-form | |
narrative summarization. Our dataset covers source documents from the literature | |
domain, such as novels, plays and stories, and includes highly abstractive, human | |
written summaries on three levels of granularity of increasing difficulty: paragraph-, | |
chapter-, and book-level. The domain and structure of our dataset poses a unique | |
set of challenges for summarization systems, which include: processing very long | |
documents, non-trivial causal and temporal dependencies, and rich discourse structures. | |
To facilitate future work, we trained and evaluated multiple extractive and abstractive | |
summarization models as baselines for our dataset.' | |
example_title: BookSum Abstract | |
inference: | |
parameters: | |
max_length: 64 | |
min_length: 8 | |
no_repeat_ngram_size: 3 | |
early_stopping: true | |
repetition_penalty: 3.5 | |
length_penalty: 0.3 | |
encoder_no_repeat_ngram_size: 3 | |
num_beams: 4 | |
model-index: | |
- name: pszemraj/led-large-book-summary | |
results: | |
- task: | |
type: summarization | |
name: Summarization | |
dataset: | |
name: kmfoda/booksum | |
type: kmfoda/booksum | |
config: kmfoda--booksum | |
split: test | |
metrics: | |
- type: rouge | |
value: 31.7308 | |
name: ROUGE-1 | |
verified: true | |
verifyToken: eyJhbGciOiJFZERTQSIsInR5cCI6IkpXVCJ9.eyJoYXNoIjoiNjJmZjMxYTY0OGU3MzNjNmIzNmYyODNlNDg2ZGRhZDAzNTMwMDM5YWMxODc1OTc1ZWE3MzM2OTg1ODFhZDBkNCIsInZlcnNpb24iOjF9.B8BCKgySYVZW910_1zP0LfCpQYJbAe6loyWut76JlgZb2kV1_x9ybqtNESX0ka-lNqhYyXUNDpuS-7pTmsJVDg | |
- type: rouge | |
value: 5.3311 | |
name: ROUGE-2 | |
verified: true | |
verifyToken: eyJhbGciOiJFZERTQSIsInR5cCI6IkpXVCJ9.eyJoYXNoIjoiYzViMmY4ODFjYTc5ODk5MmRhMDQ3ZDRiYWQwMDg0OTk3ZTA4NDAxYTNiNDgyMmI4NDA3ZDMwYWViOTBkODBjNyIsInZlcnNpb24iOjF9.MOhJLDcgvv93mVFL1igIgIiTAH3b2Xa4gmBObq7RF44Mmu8Kxtd1KP7rOlDVFOrtrsooGPGsyE1GMCQ2kqeMDg | |
- type: rouge | |
value: 16.1465 | |
name: ROUGE-L | |
verified: true | |
verifyToken: eyJhbGciOiJFZERTQSIsInR5cCI6IkpXVCJ9.eyJoYXNoIjoiNzNjMzEwMTliZGE3ZmQ4M2UxMDAyMTY3YzJjZmMyMDYyN2YyNDM0N2VhNzI1MDc1YTg4MTRjMmEzNjVkNTk1NCIsInZlcnNpb24iOjF9.XLJ-DVKiYLlbw5E5rWADKbzUzf5fNHhlTCWPCC5dU4NI9Yeh76aR7TPt36ZzLDwTBknnR8KHqlaF8F8YAvBUAg | |
- type: rouge | |
value: 29.0883 | |
name: ROUGE-LSUM | |
verified: true | |
verifyToken: eyJhbGciOiJFZERTQSIsInR5cCI6IkpXVCJ9.eyJoYXNoIjoiMTcwNzEwMmE5NjQxZTkzYmQyZDZmNzllYzYyNGI5OTMyNWMwNjdiM2I2YmM5YjdmY2E5OWQ3OTk3ZDA1MTc3YyIsInZlcnNpb24iOjF9.d6rFxjCB6RJNI_pn2DNNSjuZe4rdvj0RatkaTJRp5lP0F_AFfU5Zn9zRWzZJV7V-xMauIc4UhfdoLp9r_-CABA | |
- type: loss | |
value: 4.815707206726074 | |
name: loss | |
verified: true | |
verifyToken: eyJhbGciOiJFZERTQSIsInR5cCI6IkpXVCJ9.eyJoYXNoIjoiNTMwMTgxMmJkODY3MjkzOWJhMzJhOTIxMWVkODhjZmM0MWUzMWQ1N2JkZjRhOTQxNmU1YWVjYzQ0MDNlZWI3OSIsInZlcnNpb24iOjF9.mkBQHYhYFfDV6F4klXGJ1dSsF-pbCs-6F9zcw6IYznwmXUjtk7m5J4Zt4JAju5LKz4YizvEcUCl_L0WddnfvDA | |
- type: gen_len | |
value: 154.9036 | |
name: gen_len | |
verified: true | |
verifyToken: eyJhbGciOiJFZERTQSIsInR5cCI6IkpXVCJ9.eyJoYXNoIjoiMTc0ZmM1ZDM4MDE0MzY3MDM3OWJhNDkzZjJkZDdkMjU5M2JmMDJjYTIxODA1OTllNmY5ZWQzZDlmNWFiYzk4NiIsInZlcnNpb24iOjF9.VQ_O_xSTz870tnM08PJXQOwg9OsNNwI_HVX4S7AuW57_FzGGyRaWSuGE5SWzRS4Tur9YP0QxV4VV0Yoaoi3IAA | |
- task: | |
type: summarization | |
name: Summarization | |
dataset: | |
name: samsum | |
type: samsum | |
config: samsum | |
split: test | |
metrics: | |
- type: rouge | |
value: 33.4484 | |
name: ROUGE-1 | |
verified: true | |
verifyToken: eyJhbGciOiJFZERTQSIsInR5cCI6IkpXVCJ9.eyJoYXNoIjoiNTk4Yjg1YTc4YmY0MzBiZDU4ZjFhNzI4MjZkMWU1MzBlOWNlMjQ5ODMzY2YzYzRhYjJkMGUzNmI3ZjdkMzIzZSIsInZlcnNpb24iOjF9.AqS8A1OUiM0IZFBEGirv5F3Novk8lSUYSfPc3bYWLA6t-W7wgup3qA207eGbE5j9CkDWZ7QrSG1U6Z9A0sOqAA | |
- type: rouge | |
value: 10.4249 | |
name: ROUGE-2 | |
verified: true | |
verifyToken: eyJhbGciOiJFZERTQSIsInR5cCI6IkpXVCJ9.eyJoYXNoIjoiN2U4NjUyNTFmOGM5OTlhZDMyMTlmM2E4OWI2NGFiMDAyMGJjMzRjNWNlMGEyYWFmNTE5ZWMxM2I0ZGZmNWNmOCIsInZlcnNpb24iOjF9.SgJcHJ4qoRWXFvFiwv1PUutWktvsxQNynVPEv-GtBgxd6WI7o561ONyco5U-5tcyE_1SbSCJzz-L-R-q3cvoDA | |
- type: rouge | |
value: 24.5802 | |
name: ROUGE-L | |
verified: true | |
verifyToken: eyJhbGciOiJFZERTQSIsInR5cCI6IkpXVCJ9.eyJoYXNoIjoiZmQ5MDI5MzdiNGE5NDM0MmU5OThmZTBkNjkxMzg5N2IxNGVlODdhZTZhNjg3NzFjYWEyMzA3MTQxNjMyMjRkOCIsInZlcnNpb24iOjF9.Bg5dHqCcJjmxa-xGWNR5lD9g3quX7lKkH0pjiTd2xE5WiPoLLN2c0mYa2GovdW7__WnYwhhHC7es03jmvyZbCw | |
- type: rouge | |
value: 29.8226 | |
name: ROUGE-LSUM | |
verified: true | |
verifyToken: eyJhbGciOiJFZERTQSIsInR5cCI6IkpXVCJ9.eyJoYXNoIjoiNGFhOTEwNGM1MmZkNDk2ZjQ1Y2MyNjM3MGI5MGY3MWVkM2I0MjU2NWFiYmEwMjE4MTJlZWIwOGQ2MjQ3YjgzYSIsInZlcnNpb24iOjF9.W_aQKs10oXQdKEczJBGM3iiwJgb-VaXTpyA3sGof5WbhHf9vITAQA-xvynh5LgKtXQ1zjx737hnHgjEsu_Y0Cw | |
- type: loss | |
value: 4.176078796386719 | |
name: loss | |
verified: true | |
verifyToken: eyJhbGciOiJFZERTQSIsInR5cCI6IkpXVCJ9.eyJoYXNoIjoiN2JhODQ5YTZkNDZkZGYyNGU2MzkxMWU5MTEwMGM2YmVjZTA5YzI5NTMxMDNhYjhlOTAxMzFiMDYwYmM0MjEzZCIsInZlcnNpb24iOjF9.OvZrPBOR5jhkoTGBgsInkH7j3_xpacXHDoT7UIXEnyXzadfBO-O-K6fjalLNZw8wSkbjHIFcL_6S_qTTxPsNAQ | |
- type: gen_len | |
value: 65.4005 | |
name: gen_len | |
verified: true | |
verifyToken: eyJhbGciOiJFZERTQSIsInR5cCI6IkpXVCJ9.eyJoYXNoIjoiM2NhYjc3ZjQzNDEwYmMzOTM0ODkyZTJhZWNhNzZhYmEyZTYxMzA2YTYzMWFjOTA5ZjlhYWMzODg3NzY1ZTUwYSIsInZlcnNpb24iOjF9.vk9bgmtQFeRwdY3VXjtrJr_5wUCIeoAkI3kO0cHxhxmJo6RvUnyXiut72FuB-mlLZvqgiNkaZ-u_bh0Z3DjuCw | |
- task: | |
type: summarization | |
name: Summarization | |
dataset: | |
name: billsum | |
type: billsum | |
config: default | |
split: test | |
metrics: | |
- type: rouge | |
value: 40.5843 | |
name: ROUGE-1 | |
verified: true | |
verifyToken: eyJhbGciOiJFZERTQSIsInR5cCI6IkpXVCJ9.eyJoYXNoIjoiNTVjMDkyMWZjYTQ0NzgzNGUxZjNiMTg3NjU1MWJlNTQ2MWQ1NjE1MDk1OTU4ZjJiNGQ5ODg3Y2VlMWUyMzllNyIsInZlcnNpb24iOjF9.OhqBcVIuHk7fzmdrsWMvUe1bLeVMZVstZUoZpP7C1vR-3aIDl7r6eBmPrt5w-KcNq5p4teNPBsq7oKzbd5ZgDQ | |
- type: rouge | |
value: 17.3401 | |
name: ROUGE-2 | |
verified: true | |
verifyToken: eyJhbGciOiJFZERTQSIsInR5cCI6IkpXVCJ9.eyJoYXNoIjoiNGQxYmQzMmE0OTcyNTM5NmMwNjIxNzYxZDcwMDFkYzJkOWY4YWY3NTdhZGRhZDdlMDAxNzcwODQ5OGM3Mzc1MCIsInZlcnNpb24iOjF9.Pksn25EEqvmx757N7Swrd4yXc_xU7-AMN9yNe8lrbBa-l1LoI_2PUASvnjML4f705cfuyMAfb0FkFp5WfER2AA | |
- type: rouge | |
value: 25.1256 | |
name: ROUGE-L | |
verified: true | |
verifyToken: eyJhbGciOiJFZERTQSIsInR5cCI6IkpXVCJ9.eyJoYXNoIjoiMjhjYzI5MDBiMjk2NTY3MDNmZTdiOGYwMTRlYjIwZjAwMjdlNTAyYzdhYTJlODQ4MjYzYmQ3MjRlYTA2YzhhZSIsInZlcnNpb24iOjF9.1jPepsweS2bzIqDverQzzhmhFGch7gpoEGFGqQ8zW7K10aUKWFX8lt-uZAmTa1Z5ZhzyXGBzc3dReFPhWRRJBg | |
- type: rouge | |
value: 34.6619 | |
name: ROUGE-LSUM | |
verified: true | |
verifyToken: eyJhbGciOiJFZERTQSIsInR5cCI6IkpXVCJ9.eyJoYXNoIjoiM2VkZDIxNWJjOTA0NzFjOTIwOTdjYjc1M2EyNDVjZjY2ZjY3MjIxNDk3YTc5YWExNzAwN2FhOTc1NjVhYjBkYiIsInZlcnNpb24iOjF9.8opqHSUckPohoSF9jfPTpXDz2AtDwvdMqOdIXx2kE1tkOcbLPbOBfcc8RhRR98y8S26yC6EYFhFnf03CV2ejAQ | |
- type: loss | |
value: 4.792657375335693 | |
name: loss | |
verified: true | |
verifyToken: eyJhbGciOiJFZERTQSIsInR5cCI6IkpXVCJ9.eyJoYXNoIjoiYTY5ZTRkMGU3OGVkODMzMDU5OWE1NTM5YjA4NDliZDlmNzc2NzZjNjFmNTA3M2EwY2NmN2E0MWJmZjQ5ZDliMiIsInZlcnNpb24iOjF9.KCKdk8xt2NWcMmYKV3-9eVEsFm9MqGllSMu9QCFJFIQlnyNXllHKdBLouoaGQz8IRYXvZKH8_TLDPIQx-31jAg | |
- type: gen_len | |
value: 163.9394 | |
name: gen_len | |
verified: true | |
verifyToken: eyJhbGciOiJFZERTQSIsInR5cCI6IkpXVCJ9.eyJoYXNoIjoiYzdkZDYyZGUzYmFkZmI2NjUwYmQ0MzZjMmIyZjI1YTFiMzM4OThiZjBiMzljOTVkZTgwMjA0NTE5OGM2YmFjMiIsInZlcnNpb24iOjF9.XyMZLUdkUIF32KTJMuv_bJswQCx_Tfg4Fx823cURUixSeoIKps8_a634AreZ3Z8kb7bfE_sFGh3rM9KWsMxlDw | |
- task: | |
type: summarization | |
name: Summarization | |
dataset: | |
name: multi_news | |
type: multi_news | |
config: default | |
split: test | |
metrics: | |
- type: rouge | |
value: 39.0834 | |
name: ROUGE-1 | |
verified: true | |
verifyToken: eyJhbGciOiJFZERTQSIsInR5cCI6IkpXVCJ9.eyJoYXNoIjoiNjYzMmVlMDM4MTNkMTI4MjAyMTU2YTg1ZWQwNTI1MmJlNGUwZmE1NTRmYTljZTQwY2RlMjcxOTgyZGMyYTc0ZiIsInZlcnNpb24iOjF9.6yuSr7UmsFatwqQ-mEO4gmsEtWI05kGB5Ib2pnl05H1OiPT2uUwmqdUytUw8KTx9u1jv9q0cTF1cL-n2kPEJAA | |
- type: rouge | |
value: 11.4043 | |
name: ROUGE-2 | |
verified: true | |
verifyToken: eyJhbGciOiJFZERTQSIsInR5cCI6IkpXVCJ9.eyJoYXNoIjoiMWI5N2U2ZWI1ODM2MWUwOTIzYTAzNmRhNDA2OWEzZWRjMGEzMjBmY2EwN2YyYzU1NWE0YjIyZDE3MWE0MmMxZCIsInZlcnNpb24iOjF9.wonuxbBl25TzEaHUH_E816nHJ1OSXKfkaq7eJzbLpsfeGwcDklxUSxZxRO7VBiBMaY3Qttf9ywmEIPp40HnpBA | |
- type: rouge | |
value: 19.1813 | |
name: ROUGE-L | |
verified: true | |
verifyToken: eyJhbGciOiJFZERTQSIsInR5cCI6IkpXVCJ9.eyJoYXNoIjoiZjU1NDZhN2NkMzZiZGJkODE4NDZiYjViOTZkNGMyNDlkNjBlZmFjYzU1N2IzMjFjYjY1MDU1Zjk2MzA0M2U4NyIsInZlcnNpb24iOjF9.bTCRzv3J9NiCh4aV23tAWGTvrdQCv_RS40zGwC4AJXtGS40cY7tJHYwBf9U9_rCetDBxqfjJpdaUbCAOglxLAA | |
- type: rouge | |
value: 35.1581 | |
name: ROUGE-LSUM | |
verified: true | |
verifyToken: eyJhbGciOiJFZERTQSIsInR5cCI6IkpXVCJ9.eyJoYXNoIjoiMDNhNTUyZjE4NjYxYjIzYThmMDM2YWNhM2QwYzY1ODI2ZTE3NmNjMmVhOTAzZjZlOWQwYzc1NzU2NDNjNzIxMyIsInZlcnNpb24iOjF9.cWlSbEBgrMN5D-fV_yL9geNMyMkIItcVO3wehNJPzFi3E0v1-4q8pnX-UgjLzto8X7JLi6as2V_HtZE4-C-CDw | |
- type: loss | |
value: 4.654905319213867 | |
name: loss | |
verified: true | |
verifyToken: eyJhbGciOiJFZERTQSIsInR5cCI6IkpXVCJ9.eyJoYXNoIjoiYTc5Nzk0ODhiNWUzNTAxNzk2YzZmMjU2NDliY2UzOTYyYTdmZGEyYjI5NDNhOTE0MGUxOTgxMGVjMmNhM2UyMSIsInZlcnNpb24iOjF9.eBBAebcl3AwkrjR6a8BvoSjDfpw8LWTRFjyIFHVzspvoOKVfnO8_NB_UeR_K127OwXyoZ70Z7X_aKJOe-2kTDA | |
- type: gen_len | |
value: 186.2494 | |
name: gen_len | |
verified: true | |
verifyToken: eyJhbGciOiJFZERTQSIsInR5cCI6IkpXVCJ9.eyJoYXNoIjoiOWI2NjVlYjgwYWJiMjcyMDUzMzEwNDNjZTMxMDM0MjAzMzk1ZmIwY2Q1ZDQ2Y2M5NDBlMDEzYzFkNWEyNzJmNiIsInZlcnNpb24iOjF9.iZ1Iy7FuWL4GH7LS5EylVj5eZRC3L2ZsbYQapAkMNzR_VXPoMGvoM69Hp-kU7gW55tmz2V4Qxhvoz9cM8fciBA | |
- task: | |
type: summarization | |
name: Summarization | |
dataset: | |
name: cnn_dailymail | |
type: cnn_dailymail | |
config: 3.0.0 | |
split: test | |
metrics: | |
- type: rouge | |
value: 32.8774 | |
name: ROUGE-1 | |
verified: true | |
verifyToken: eyJhbGciOiJFZERTQSIsInR5cCI6IkpXVCJ9.eyJoYXNoIjoiYWVlNjQzNWU1NTgyNTk2MzdhMDkyM2U3N2UzYzQ3ODJmOTJiMGViZDc0NzNiNDlmZGZmNTQzZmNjYTFjMzJmMCIsInZlcnNpb24iOjF9.qA54KJrGf79XCLnDrAMPp0saErVL_zKicLso9ZX2xxNdCANGExal5PFmmTT7aw7TUdkmUsNhmIRI9cBZ8J_1BA | |
- type: rouge | |
value: 13.3706 | |
name: ROUGE-2 | |
verified: true | |
verifyToken: eyJhbGciOiJFZERTQSIsInR5cCI6IkpXVCJ9.eyJoYXNoIjoiZDMzZWVjZmQ4ZWI2MWZmMGEzNjJhY2JmZjJhZTYwMTk2OTM2ODhlMmFmYmMxZGUyZWQzMmUxYzA0ZjJiMjcwYiIsInZlcnNpb24iOjF9.03Di-BfbZoWAVqRJc3x37Tn1Ae6vtZWymZL2w1ob8OQ8iOggYwmDmNQwv-bCXjT7fLjXYvh9uTndYsL05nj_Ag | |
- type: rouge | |
value: 20.4365 | |
name: ROUGE-L | |
verified: true | |
verifyToken: eyJhbGciOiJFZERTQSIsInR5cCI6IkpXVCJ9.eyJoYXNoIjoiYjI5YzdjZmM0YmZjYTU0OTg3ZTRjZWZkYTU2NzhlZjkwNGE2YmUzYzI1OThjMDUxOTcyNzk3ZTUyNmIzMWYzZCIsInZlcnNpb24iOjF9.LDg9lCKTh74kilxRBpunGSeOXJohaICXWjNf525ck-1h21AtjIQB8U7BTm80eyNRe7yIQpAlgOruCAxRqpTHDw | |
- type: rouge | |
value: 30.4408 | |
name: ROUGE-LSUM | |
verified: true | |
verifyToken: eyJhbGciOiJFZERTQSIsInR5cCI6IkpXVCJ9.eyJoYXNoIjoiNTZhMGJjMzg0MzQxY2U2ZTIzYTYzOGRhMGEyYjY1ZjQyZjNmNGIwMzFjOWJjNzU2NWQzMzc1Y2IxYWZkZGY5YyIsInZlcnNpb24iOjF9.LkvaIEsw0U-osBR--46f7rsF-s1fcu19Z22DkvwiMwWJj9AnsUwDWNcCecIyi5tziQpUx0PpZEKyXAhCrVx1Bw | |
- type: loss | |
value: 5.3488945960998535 | |
name: loss | |
verified: true | |
verifyToken: eyJhbGciOiJFZERTQSIsInR5cCI6IkpXVCJ9.eyJoYXNoIjoiNTc4Y2JlZWRlNDRkOTI4ODQyZjBlMjU5NmUyZTZmNzJjYTg0NjM1YzI4NzUzYjhmODBkY2U4NGJiMTlhYTc2ZiIsInZlcnNpb24iOjF9.CB6oO5j3cKJPOelM8pwT2lTenp5bZTkBFC5MPYW_nus-O5F1s4DaY-gdSUK3baTkMXbQ2yqaI_g_QAfNVmqhDQ | |
- type: gen_len | |
value: 181.8326 | |
name: gen_len | |
verified: true | |
verifyToken: eyJhbGciOiJFZERTQSIsInR5cCI6IkpXVCJ9.eyJoYXNoIjoiOThmMGNlMGEwYjljMmNiZjdkMjc5NzZhNTYwMzAzOWFkYzA1NzZiNTIyN2IxNDJmOTk4MDliYzY2YjdjNGY4MSIsInZlcnNpb24iOjF9._buvRpxKLuKNNtOmALbFm3-nWCs2NCLh1l8gfVqDmKmv8JqJHQ27cdgZ4mklPLYOUhf6YWjby5_lp3ZGEctkCQ | |
# led-large-book-summary | |
<a href="https://colab.research.google.com/gist/pszemraj/3eba944ddc9fc9a4a1bfb21e83b57620/summarization-token-batching.ipynb"> | |
<img src="https://colab.research.google.com/assets/colab-badge.svg" alt="Open In Colab"/> | |
</a> | |
This model is a fine-tuned version of [allenai/led-large-16384](https://huggingface.co/allenai/led-large-16384) on the `BookSum` dataset (`kmfoda/booksum`). It aims to generalize well and be useful in summarizing lengthy text for both academic and everyday purposes. | |
- Handles up to 16,384 tokens input | |
- See the Colab demo linked above or try the [demo on Spaces](https://huggingface.co/spaces/pszemraj/summarize-long-text) | |
> **Note:** Due to inference API timeout constraints, outputs may be truncated before the fully summary is returned (try python or the demo) | |
--- | |
## Basic Usage | |
To improve summary quality, use `encoder_no_repeat_ngram_size=3` when calling the pipeline object. This setting encourages the model to utilize new vocabulary and construct an abstractive summary. | |
Load the model into a pipeline object: | |
```python | |
import torch | |
from transformers import pipeline | |
hf_name = 'pszemraj/led-large-book-summary' | |
summarizer = pipeline( | |
"summarization", | |
hf_name, | |
device=0 if torch.cuda.is_available() else -1, | |
) | |
``` | |
Feed the text into the pipeline object: | |
```python | |
wall_of_text = "your words here" | |
result = summarizer( | |
wall_of_text, | |
min_length=16, | |
max_length=256, | |
no_repeat_ngram_size=3, | |
encoder_no_repeat_ngram_size=3, | |
repetition_penalty=3.5, | |
num_beams=4, | |
early_stopping=True, | |
) | |
``` | |
**Important:** For optimal summary quality, use the global attention mask when decoding, as demonstrated in [this community notebook](https://colab.research.google.com/drive/12INTTR6n64TzS4RrXZxMSXfrOd9Xzamo?usp=sharing), see the definition of `generate_answer(batch)`. | |
If you're facing computing constraints, consider using the base version [`pszemraj/led-base-book-summary`](https://huggingface.co/pszemraj/led-base-book-summary). | |
--- | |
## Training Information | |
### Data | |
The model was fine-tuned on the [booksum](https://arxiv.org/abs/2105.08209) dataset. During training, the `chapter`was the input col, while the `summary_text` was the output. | |
### Procedure | |
Fine-tuning was run on the BookSum dataset across 13+ epochs. Notably, the final four epochs combined the training and validation sets as 'train' to enhance generalization. | |
### Hyperparameters | |
The training process involved different settings across stages: | |
- **Initial Three Epochs:** Low learning rate (5e-05), batch size of 1, 4 gradient accumulation steps, and a linear learning rate scheduler. | |
- **In-between Epochs:** Learning rate reduced to 4e-05, increased batch size to 2, 16 gradient accumulation steps, and switched to a cosine learning rate scheduler with a 0.05 warmup ratio. | |
- **Final Two Epochs:** Further reduced learning rate (2e-05), batch size reverted to 1, maintained gradient accumulation steps at 16, and continued with a cosine learning rate scheduler, albeit with a lower warmup ratio (0.03). | |
### Versions | |
- Transformers 4.19.2 | |
- Pytorch 1.11.0+cu113 | |
- Datasets 2.2.2 | |
- Tokenizers 0.12.1 | |
--- | |
## Simplified Usage with TextSum | |
To streamline the process of using this and other models, I've developed [a Python package utility](https://github.com/pszemraj/textsum) named `textsum`. This package offers simple interfaces for applying summarization models to text documents of arbitrary length. | |
Install TextSum: | |
```bash | |
pip install textsum | |
``` | |
Then use it in Python with this model: | |
```python | |
from textsum.summarize import Summarizer | |
model_name = "pszemraj/led-large-book-summary" | |
summarizer = Summarizer( | |
model_name_or_path=model_name, # you can use any Seq2Seq model on the Hub | |
token_batch_length=4096, # tokens to batch summarize at a time, up to 16384 | |
) | |
long_string = "This is a long string of text that will be summarized." | |
out_str = summarizer.summarize_string(long_string) | |
print(f"summary: {out_str}") | |
``` | |
Currently implemented interfaces include a Python API, a Command-Line Interface (CLI), and a demo/web UI. | |
For detailed explanations and documentation, check the [README](https://github.com/pszemraj/textsum) or the [wiki](https://github.com/pszemraj/textsum/wiki) | |
--- | |
## Related Models | |
Check out these other related models, also trained on the BookSum dataset: | |
- [LED-large continued](https://huggingface.co/pszemraj/led-large-book-summary-continued) - experiment with further fine-tuning | |
- [Long-T5-tglobal-base](https://huggingface.co/pszemraj/long-t5-tglobal-base-16384-book-summary) | |
- [BigBird-Pegasus-Large-K](https://huggingface.co/pszemraj/bigbird-pegasus-large-K-booksum) | |
- [Pegasus-X-Large](https://huggingface.co/pszemraj/pegasus-x-large-book-summary) | |
- [Long-T5-tglobal-XL](https://huggingface.co/pszemraj/long-t5-tglobal-xl-16384-book-summary) | |
There are also other variants on other datasets etc on my hf profile, feel free to try them out :) | |
--- | |