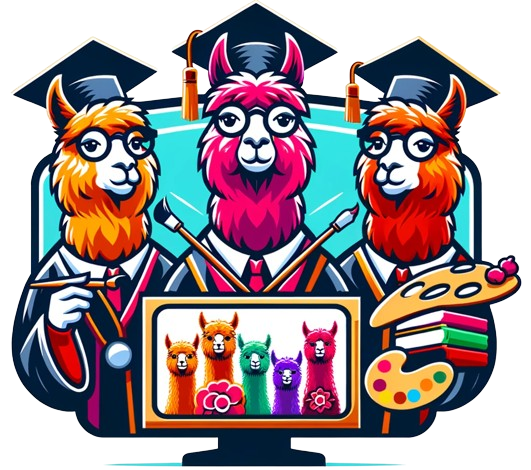
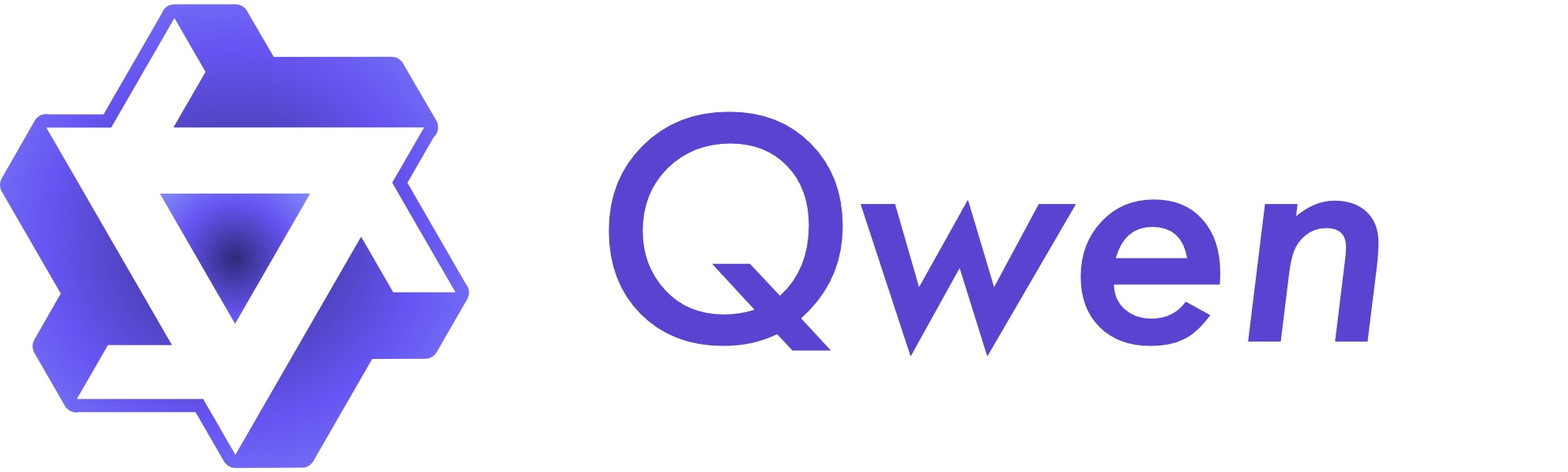
MoE-LLaVA-Qwen1.5-1.8B×4-Top2: When Vision meet Small-scaled Language Model and Vietnamese Synthetic Dataset
Introducing MoE-LLaVA-Qwen1.5-1.8B×4-Top2 for Vietnamese
We are excited to present MoE-LLaVA-Qwen1.5-1.8B×4-Top2, tailored for the Vietnamese language. This model is part of our ongoing efforts to develop Vision Language Models (VLM) for Vietnamese, a domain that is currently limited and predominantly features larger models (~7B parameters). Our model activates approximately 2.2B 🤗😎 parameters per call, significantly reducing the memory footprint, and it can be quantized for local execution.
Bias, Risks, and Limitations
The dataset may contain biases originating from its sources. Users should remain aware of these potential biases when utilizing the dataset.
More Information
This dataset represents the first stage of a two-stage development process for a larger model. Stay tuned for future developments by subscribing to our updates.
Training and evaluation data
Training Dataset
Our model is trained on the comprehensive Vi-VLM/Vista dataset, which includes around 700,000 Vietnamese vision-language samples curated by Gemini Pro. We employed various prompt engineering techniques, including:
- Few-shot Learning
- Caption-based Prompting
- Image-based Prompting
Techniques Used
- MoE-LLaVA: MoE-LLaVA
Evaluation
- Comming soon 🫡
Training procedure
Training hyperparameters
The following hyperparameters were used during training:
- learning_rate: 2e-05
- train_batch_size: 4
- eval_batch_size: 4
- seed: 42
- distributed_type: multi-GPU
- num_devices: 4
- gradient_accumulation_steps: 8
- total_train_batch_size: 128
- total_eval_batch_size: 16
- optimizer: Adam with betas=(0.9,0.999) and epsilon=1e-08
- lr_scheduler_type: cosine
- lr_scheduler_warmup_ratio: 0.03
- num_epochs: 1.0
Training results
Framework versions
- Transformers 4.37.0
- Pytorch 2.0.1+cu117
- Datasets 2.20.0
- Tokenizers 0.15.1
- Downloads last month
- 10