html_url
stringlengths 47
49
| title
stringlengths 4
111
| comments
stringlengths 71
20.4k
| body
stringlengths 0
12.9k
β | comment_length_in_words
int64 16
1.61k
| text
stringlengths 100
20.5k
|
---|---|---|---|---|---|
https://github.com/huggingface/datasets/pull/2819 | Added XL-Sum dataset | Hi ! Ideally everything should be in the same place, so feel free to create a community dataset on the Hub and upload your data files as well as you dataset script (and also the readme.md and dataset_infos.json).
The only change you have to do in your dataset script is use a relative path to your data files instead of urls.
For example if your repository looks like this:
```
xlsum/
βββ data/
β βββ amharic_XLSum_v2.0.tar.bz2
β βββ ...
β βββ yoruba_XLSum_v2.0.tar.bz2
βββ xlsum.py
βββ README.md
βββ dataset_infos.json
```
Then you just need to pass `"data/amharic_XLSum_v2.0.tar.bz2"` to `dl_manager.download_and_extract(...)`, instead of an url.
Locally you can test that it's working as expected with
```python
load_dataset("path/to/my/directory/named/xlsum")
```
Then once it's on the Hub, you can load it with
```python
load_dataset("username/xlsum")
```
Let me know if you have questions :) | Added XL-Sum dataset published in ACL-IJCNLP 2021. (https://aclanthology.org/2021.findings-acl.413/). The default timeout values in `src/datasets/utils/file_utls.py` were increased to enable downloading from the original google drive links. | 137 | text: Added XL-Sum dataset
Added XL-Sum dataset published in ACL-IJCNLP 2021. (https://aclanthology.org/2021.findings-acl.413/). The default timeout values in `src/datasets/utils/file_utls.py` were increased to enable downloading from the original google drive links.
Hi ! Ideally everything should be in the same place, so feel free to create a community dataset on the Hub and upload your data files as well as you dataset script (and also the readme.md and dataset_infos.json).
The only change you have to do in your dataset script is use a relative path to your data files instead of urls.
For example if your repository looks like this:
```
xlsum/
βββ data/
β βββ amharic_XLSum_v2.0.tar.bz2
β βββ ...
β βββ yoruba_XLSum_v2.0.tar.bz2
βββ xlsum.py
βββ README.md
βββ dataset_infos.json
```
Then you just need to pass `"data/amharic_XLSum_v2.0.tar.bz2"` to `dl_manager.download_and_extract(...)`, instead of an url.
Locally you can test that it's working as expected with
```python
load_dataset("path/to/my/directory/named/xlsum")
```
Then once it's on the Hub, you can load it with
```python
load_dataset("username/xlsum")
```
Let me know if you have questions :) |
https://github.com/huggingface/datasets/pull/2819 | Added XL-Sum dataset | Thank you for your detailed response regarding the community dataset building process. However, will this pull request be merged into the main branch? | Added XL-Sum dataset published in ACL-IJCNLP 2021. (https://aclanthology.org/2021.findings-acl.413/). The default timeout values in `src/datasets/utils/file_utls.py` were increased to enable downloading from the original google drive links. | 23 | text: Added XL-Sum dataset
Added XL-Sum dataset published in ACL-IJCNLP 2021. (https://aclanthology.org/2021.findings-acl.413/). The default timeout values in `src/datasets/utils/file_utls.py` were increased to enable downloading from the original google drive links.
Thank you for your detailed response regarding the community dataset building process. However, will this pull request be merged into the main branch? |
https://github.com/huggingface/datasets/pull/2819 | Added XL-Sum dataset | If XL-sum is available via the Hub we don't need to add it again in the `datasets` github repo ;) | Added XL-Sum dataset published in ACL-IJCNLP 2021. (https://aclanthology.org/2021.findings-acl.413/). The default timeout values in `src/datasets/utils/file_utls.py` were increased to enable downloading from the original google drive links. | 20 | text: Added XL-Sum dataset
Added XL-Sum dataset published in ACL-IJCNLP 2021. (https://aclanthology.org/2021.findings-acl.413/). The default timeout values in `src/datasets/utils/file_utls.py` were increased to enable downloading from the original google drive links.
If XL-sum is available via the Hub we don't need to add it again in the `datasets` github repo ;) |
https://github.com/huggingface/datasets/pull/2817 | Rename The Pile subsets | I think the main `the_pile` datasets will be the one that is the mix of all the subsets: https://the-eye.eu/public/AI/pile/
We can also add configurations for each subset, and even allow users to specify the subsets they want:
```python
from datasets import load_dataset
load_dataset("the_pile", subsets=["openwebtext2", "books3", "hn"])
```
We're alrady doing something similar for mC4, where users can specify the list of languages they want to load. | After discussing with @yjernite we think it's better to have the subsets of The Pile explicitly have "the_pile" in their names.
I'm doing the changes for the subsets that @richarddwang added:
- [x] books3 -> the_pile_books3 https://github.com/huggingface/datasets/pull/2801
- [x] stack_exchange -> the_pile_stack_exchange https://github.com/huggingface/datasets/pull/2803
- [x] openwebtext2 -> the_pile_openwebtext2 https://github.com/huggingface/datasets/pull/2802
For consistency we should also rename `bookcorpusopen` to `the_pile_bookcorpus` IMO, but let me know what you think.
(we can just add a deprecation message to `bookcorpusopen` for now and add `the_pile_bookcorpus`) | 66 | text: Rename The Pile subsets
After discussing with @yjernite we think it's better to have the subsets of The Pile explicitly have "the_pile" in their names.
I'm doing the changes for the subsets that @richarddwang added:
- [x] books3 -> the_pile_books3 https://github.com/huggingface/datasets/pull/2801
- [x] stack_exchange -> the_pile_stack_exchange https://github.com/huggingface/datasets/pull/2803
- [x] openwebtext2 -> the_pile_openwebtext2 https://github.com/huggingface/datasets/pull/2802
For consistency we should also rename `bookcorpusopen` to `the_pile_bookcorpus` IMO, but let me know what you think.
(we can just add a deprecation message to `bookcorpusopen` for now and add `the_pile_bookcorpus`)
I think the main `the_pile` datasets will be the one that is the mix of all the subsets: https://the-eye.eu/public/AI/pile/
We can also add configurations for each subset, and even allow users to specify the subsets they want:
```python
from datasets import load_dataset
load_dataset("the_pile", subsets=["openwebtext2", "books3", "hn"])
```
We're alrady doing something similar for mC4, where users can specify the list of languages they want to load. |
https://github.com/huggingface/datasets/pull/2811 | Fix stream oscar | One additional note: if we can try to not change the code of oscar.py too often, I'm sure users that have it in their cache directory will be happy to not have to redownload it every time they update the library ;)
(since changing the code changes the cache directory of the dataset) | Previously, an additional `open` was added to oscar to make it stream-compatible: 587bbb94e891b22863b312b99696e32708c379f4.
This was argued that might be problematic: https://github.com/huggingface/datasets/pull/2786#discussion_r690045921
This PR:
- removes that additional `open`
- patches `gzip.open` with `xopen` + `compression="gzip"` | 53 | text: Fix stream oscar
Previously, an additional `open` was added to oscar to make it stream-compatible: 587bbb94e891b22863b312b99696e32708c379f4.
This was argued that might be problematic: https://github.com/huggingface/datasets/pull/2786#discussion_r690045921
This PR:
- removes that additional `open`
- patches `gzip.open` with `xopen` + `compression="gzip"`
One additional note: if we can try to not change the code of oscar.py too often, I'm sure users that have it in their cache directory will be happy to not have to redownload it every time they update the library ;)
(since changing the code changes the cache directory of the dataset) |
https://github.com/huggingface/datasets/pull/2811 | Fix stream oscar | I don't think this is confusing for users because users don't even know we have patched `open`. The only thing users care is that if the pass `streaming=True`, they want to be able to load the dataset in streaming mode.
I don't see any other dataset where patching `open` with `fsspec.open`+`compression` is an "underlying issue". Are there other datasets where this is an issue?
The only dataset where this was an issue is in oscar and the issue is indeed due to the additional `open` you added inside `zip.open`. | Previously, an additional `open` was added to oscar to make it stream-compatible: 587bbb94e891b22863b312b99696e32708c379f4.
This was argued that might be problematic: https://github.com/huggingface/datasets/pull/2786#discussion_r690045921
This PR:
- removes that additional `open`
- patches `gzip.open` with `xopen` + `compression="gzip"` | 89 | text: Fix stream oscar
Previously, an additional `open` was added to oscar to make it stream-compatible: 587bbb94e891b22863b312b99696e32708c379f4.
This was argued that might be problematic: https://github.com/huggingface/datasets/pull/2786#discussion_r690045921
This PR:
- removes that additional `open`
- patches `gzip.open` with `xopen` + `compression="gzip"`
I don't think this is confusing for users because users don't even know we have patched `open`. The only thing users care is that if the pass `streaming=True`, they want to be able to load the dataset in streaming mode.
I don't see any other dataset where patching `open` with `fsspec.open`+`compression` is an "underlying issue". Are there other datasets where this is an issue?
The only dataset where this was an issue is in oscar and the issue is indeed due to the additional `open` you added inside `zip.open`. |
https://github.com/huggingface/datasets/pull/2806 | Fix streaming tar files from canonical datasets | In case it's relevant for this PR, I'm finding that I cannot stream the `bookcorpus` dataset (using the `master` branch of `datasets`), which is a `.tar.bz2` file:
```python
from datasets import load_dataset
books_dataset_streamed = load_dataset("bookcorpus", split="train", streaming=True)
# Throws a 404 HTTP error
next(iter(books_dataset_streamed))
```
The full stack trace is:
```
---------------------------------------------------------------------------
ClientResponseError Traceback (most recent call last)
<ipython-input-11-5ebbbe110b13> in <module>()
----> 1 next(iter(books_dataset_streamed))
11 frames
/usr/local/lib/python3.7/dist-packages/datasets/iterable_dataset.py in __iter__(self)
339
340 def __iter__(self):
--> 341 for key, example in self._iter():
342 if self.features:
343 # we encode the example for ClassLabel feature types for example
/usr/local/lib/python3.7/dist-packages/datasets/iterable_dataset.py in _iter(self)
336 else:
337 ex_iterable = self._ex_iterable
--> 338 yield from ex_iterable
339
340 def __iter__(self):
/usr/local/lib/python3.7/dist-packages/datasets/iterable_dataset.py in __iter__(self)
76
77 def __iter__(self):
---> 78 for key, example in self.generate_examples_fn(**self.kwargs):
79 yield key, example
80
/root/.cache/huggingface/modules/datasets_modules/datasets/bookcorpus/44662c4a114441c35200992bea923b170e6f13f2f0beb7c14e43759cec498700/bookcorpus.py in _generate_examples(self, directory)
98 for txt_file in files:
99 with open(txt_file, mode="r", encoding="utf-8") as f:
--> 100 for line in f:
101 yield _id, {"text": line.strip()}
102 _id += 1
/usr/local/lib/python3.7/dist-packages/fsspec/implementations/http.py in read(self, length)
496 else:
497 length = min(self.size - self.loc, length)
--> 498 return super().read(length)
499
500 async def async_fetch_all(self):
/usr/local/lib/python3.7/dist-packages/fsspec/spec.py in read(self, length)
1481 # don't even bother calling fetch
1482 return b""
-> 1483 out = self.cache._fetch(self.loc, self.loc + length)
1484 self.loc += len(out)
1485 return out
/usr/local/lib/python3.7/dist-packages/fsspec/caching.py in _fetch(self, start, end)
374 ):
375 # First read, or extending both before and after
--> 376 self.cache = self.fetcher(start, bend)
377 self.start = start
378 elif start < self.start:
/usr/local/lib/python3.7/dist-packages/fsspec/asyn.py in wrapper(*args, **kwargs)
86 def wrapper(*args, **kwargs):
87 self = obj or args[0]
---> 88 return sync(self.loop, func, *args, **kwargs)
89
90 return wrapper
/usr/local/lib/python3.7/dist-packages/fsspec/asyn.py in sync(loop, func, timeout, *args, **kwargs)
67 raise FSTimeoutError
68 if isinstance(result[0], BaseException):
---> 69 raise result[0]
70 return result[0]
71
/usr/local/lib/python3.7/dist-packages/fsspec/asyn.py in _runner(event, coro, result, timeout)
23 coro = asyncio.wait_for(coro, timeout=timeout)
24 try:
---> 25 result[0] = await coro
26 except Exception as ex:
27 result[0] = ex
/usr/local/lib/python3.7/dist-packages/fsspec/implementations/http.py in async_fetch_range(self, start, end)
535 # range request outside file
536 return b""
--> 537 r.raise_for_status()
538 if r.status == 206:
539 # partial content, as expected
/usr/local/lib/python3.7/dist-packages/aiohttp/client_reqrep.py in raise_for_status(self)
1003 status=self.status,
1004 message=self.reason,
-> 1005 headers=self.headers,
1006 )
1007
ClientResponseError: 404, message='Not Found', url=URL('https://storage.googleapis.com/huggingface-nlp/datasets/bookcorpus/bookcorpus.tar.bz2/books_large_p1.txt')
```
Let me know if this is unrelated and I'll open a separate issue :)
Environment info:
```
- `datasets` version: 1.11.1.dev0
- Platform: Linux-5.4.104+-x86_64-with-Ubuntu-18.04-bionic
- Python version: 3.7.11
- PyArrow version: 3.0.0
``` | Previous PR #2800 implemented support to stream remote tar files when passing the parameter `data_files`: they required a glob string `"*"`.
However, this glob string creates an error when streaming canonical datasets (with a `join` after the `open`).
This PR fixes this issue and allows streaming tar files both from:
- canonical datasets scripts and
- data files.
This PR also adds support for compressed tar files: `.tar.gz`, `.tar.bz2`,...
| 401 | text: Fix streaming tar files from canonical datasets
Previous PR #2800 implemented support to stream remote tar files when passing the parameter `data_files`: they required a glob string `"*"`.
However, this glob string creates an error when streaming canonical datasets (with a `join` after the `open`).
This PR fixes this issue and allows streaming tar files both from:
- canonical datasets scripts and
- data files.
This PR also adds support for compressed tar files: `.tar.gz`, `.tar.bz2`,...
In case it's relevant for this PR, I'm finding that I cannot stream the `bookcorpus` dataset (using the `master` branch of `datasets`), which is a `.tar.bz2` file:
```python
from datasets import load_dataset
books_dataset_streamed = load_dataset("bookcorpus", split="train", streaming=True)
# Throws a 404 HTTP error
next(iter(books_dataset_streamed))
```
The full stack trace is:
```
---------------------------------------------------------------------------
ClientResponseError Traceback (most recent call last)
<ipython-input-11-5ebbbe110b13> in <module>()
----> 1 next(iter(books_dataset_streamed))
11 frames
/usr/local/lib/python3.7/dist-packages/datasets/iterable_dataset.py in __iter__(self)
339
340 def __iter__(self):
--> 341 for key, example in self._iter():
342 if self.features:
343 # we encode the example for ClassLabel feature types for example
/usr/local/lib/python3.7/dist-packages/datasets/iterable_dataset.py in _iter(self)
336 else:
337 ex_iterable = self._ex_iterable
--> 338 yield from ex_iterable
339
340 def __iter__(self):
/usr/local/lib/python3.7/dist-packages/datasets/iterable_dataset.py in __iter__(self)
76
77 def __iter__(self):
---> 78 for key, example in self.generate_examples_fn(**self.kwargs):
79 yield key, example
80
/root/.cache/huggingface/modules/datasets_modules/datasets/bookcorpus/44662c4a114441c35200992bea923b170e6f13f2f0beb7c14e43759cec498700/bookcorpus.py in _generate_examples(self, directory)
98 for txt_file in files:
99 with open(txt_file, mode="r", encoding="utf-8") as f:
--> 100 for line in f:
101 yield _id, {"text": line.strip()}
102 _id += 1
/usr/local/lib/python3.7/dist-packages/fsspec/implementations/http.py in read(self, length)
496 else:
497 length = min(self.size - self.loc, length)
--> 498 return super().read(length)
499
500 async def async_fetch_all(self):
/usr/local/lib/python3.7/dist-packages/fsspec/spec.py in read(self, length)
1481 # don't even bother calling fetch
1482 return b""
-> 1483 out = self.cache._fetch(self.loc, self.loc + length)
1484 self.loc += len(out)
1485 return out
/usr/local/lib/python3.7/dist-packages/fsspec/caching.py in _fetch(self, start, end)
374 ):
375 # First read, or extending both before and after
--> 376 self.cache = self.fetcher(start, bend)
377 self.start = start
378 elif start < self.start:
/usr/local/lib/python3.7/dist-packages/fsspec/asyn.py in wrapper(*args, **kwargs)
86 def wrapper(*args, **kwargs):
87 self = obj or args[0]
---> 88 return sync(self.loop, func, *args, **kwargs)
89
90 return wrapper
/usr/local/lib/python3.7/dist-packages/fsspec/asyn.py in sync(loop, func, timeout, *args, **kwargs)
67 raise FSTimeoutError
68 if isinstance(result[0], BaseException):
---> 69 raise result[0]
70 return result[0]
71
/usr/local/lib/python3.7/dist-packages/fsspec/asyn.py in _runner(event, coro, result, timeout)
23 coro = asyncio.wait_for(coro, timeout=timeout)
24 try:
---> 25 result[0] = await coro
26 except Exception as ex:
27 result[0] = ex
/usr/local/lib/python3.7/dist-packages/fsspec/implementations/http.py in async_fetch_range(self, start, end)
535 # range request outside file
536 return b""
--> 537 r.raise_for_status()
538 if r.status == 206:
539 # partial content, as expected
/usr/local/lib/python3.7/dist-packages/aiohttp/client_reqrep.py in raise_for_status(self)
1003 status=self.status,
1004 message=self.reason,
-> 1005 headers=self.headers,
1006 )
1007
ClientResponseError: 404, message='Not Found', url=URL('https://storage.googleapis.com/huggingface-nlp/datasets/bookcorpus/bookcorpus.tar.bz2/books_large_p1.txt')
```
Let me know if this is unrelated and I'll open a separate issue :)
Environment info:
```
- `datasets` version: 1.11.1.dev0
- Platform: Linux-5.4.104+-x86_64-with-Ubuntu-18.04-bionic
- Python version: 3.7.11
- PyArrow version: 3.0.0
``` |
https://github.com/huggingface/datasets/pull/2806 | Fix streaming tar files from canonical datasets | > @lewtun: `.tar.compression-extension` files are not supported yet. That is the objective of this PR.
thanks for the context and the great work on the streaming features (right now i'm writing the streaming section of the HF course, so am acting like a beta tester π) | Previous PR #2800 implemented support to stream remote tar files when passing the parameter `data_files`: they required a glob string `"*"`.
However, this glob string creates an error when streaming canonical datasets (with a `join` after the `open`).
This PR fixes this issue and allows streaming tar files both from:
- canonical datasets scripts and
- data files.
This PR also adds support for compressed tar files: `.tar.gz`, `.tar.bz2`,...
| 46 | text: Fix streaming tar files from canonical datasets
Previous PR #2800 implemented support to stream remote tar files when passing the parameter `data_files`: they required a glob string `"*"`.
However, this glob string creates an error when streaming canonical datasets (with a `join` after the `open`).
This PR fixes this issue and allows streaming tar files both from:
- canonical datasets scripts and
- data files.
This PR also adds support for compressed tar files: `.tar.gz`, `.tar.bz2`,...
> @lewtun: `.tar.compression-extension` files are not supported yet. That is the objective of this PR.
thanks for the context and the great work on the streaming features (right now i'm writing the streaming section of the HF course, so am acting like a beta tester π) |
https://github.com/huggingface/datasets/pull/2806 | Fix streaming tar files from canonical datasets | @lewtun this PR fixes previous issue with xjoin:
Given:
```python
xjoin(
"https://storage.googleapis.com/huggingface-nlp/datasets/bookcorpus/bookcorpus.tar.bz2",
"books_large_p1.txt"
)
```
- Before it gave:
`"https://storage.googleapis.com/huggingface-nlp/datasets/bookcorpus/bookcorpus.tar.bz2/books_large_p1.txt"`
thus raising the 404 error
- Now it gives:
`tar://books_large_p1.txt::https://storage.googleapis.com/huggingface-nlp/datasets/bookcorpus/bookcorpus.tar.bz2`
(this is the expected format for `fsspec`) and additionally passes the parameter `compression="bz2"`.
See: https://github.com/huggingface/datasets/pull/2806/files#diff-97bb2d08db65ce3b679aefc43cadad76d053c1e58ecc315e49b80873d0fbdabeR15 | Previous PR #2800 implemented support to stream remote tar files when passing the parameter `data_files`: they required a glob string `"*"`.
However, this glob string creates an error when streaming canonical datasets (with a `join` after the `open`).
This PR fixes this issue and allows streaming tar files both from:
- canonical datasets scripts and
- data files.
This PR also adds support for compressed tar files: `.tar.gz`, `.tar.bz2`,...
| 45 | text: Fix streaming tar files from canonical datasets
Previous PR #2800 implemented support to stream remote tar files when passing the parameter `data_files`: they required a glob string `"*"`.
However, this glob string creates an error when streaming canonical datasets (with a `join` after the `open`).
This PR fixes this issue and allows streaming tar files both from:
- canonical datasets scripts and
- data files.
This PR also adds support for compressed tar files: `.tar.gz`, `.tar.bz2`,...
@lewtun this PR fixes previous issue with xjoin:
Given:
```python
xjoin(
"https://storage.googleapis.com/huggingface-nlp/datasets/bookcorpus/bookcorpus.tar.bz2",
"books_large_p1.txt"
)
```
- Before it gave:
`"https://storage.googleapis.com/huggingface-nlp/datasets/bookcorpus/bookcorpus.tar.bz2/books_large_p1.txt"`
thus raising the 404 error
- Now it gives:
`tar://books_large_p1.txt::https://storage.googleapis.com/huggingface-nlp/datasets/bookcorpus/bookcorpus.tar.bz2`
(this is the expected format for `fsspec`) and additionally passes the parameter `compression="bz2"`.
See: https://github.com/huggingface/datasets/pull/2806/files#diff-97bb2d08db65ce3b679aefc43cadad76d053c1e58ecc315e49b80873d0fbdabeR15 |
https://github.com/huggingface/datasets/pull/2803 | add stack exchange | Hi ! Merging this one since it's all good :)
However I think it would also be better to actually rename it `the_pile_stack_exchange` to make things clearer and to avoid name collisions in the future. I would like to do the same for `books3` as well.
If you don't mind I'll open a PR to do the renaming | stack exchange is part of EleutherAI/The Pile, but AFAIK, The Pile dataset blend all sub datasets together thus we are not able to use just one of its sub dataset from The Pile data. So I create an independent dataset using The Pile preliminary components.
I also change default `timeout` to 100 seconds instead of 10 seconds, otherwise I keep getting read time out when downloading source data of stack exchange and cc100 dataset.
When I was creating dataset card. I found there is room for creating / editing dataset card. I've made it an issue. #2797
Also I am wondering whether the import of The Pile dataset is actively undertaken (because I may need it recently)? #1675 | 58 | text: add stack exchange
stack exchange is part of EleutherAI/The Pile, but AFAIK, The Pile dataset blend all sub datasets together thus we are not able to use just one of its sub dataset from The Pile data. So I create an independent dataset using The Pile preliminary components.
I also change default `timeout` to 100 seconds instead of 10 seconds, otherwise I keep getting read time out when downloading source data of stack exchange and cc100 dataset.
When I was creating dataset card. I found there is room for creating / editing dataset card. I've made it an issue. #2797
Also I am wondering whether the import of The Pile dataset is actively undertaken (because I may need it recently)? #1675
Hi ! Merging this one since it's all good :)
However I think it would also be better to actually rename it `the_pile_stack_exchange` to make things clearer and to avoid name collisions in the future. I would like to do the same for `books3` as well.
If you don't mind I'll open a PR to do the renaming |
https://github.com/huggingface/datasets/pull/2803 | add stack exchange |
> If you don't mind I'll open a PR to do the renaming
@lhoestq That will be nice !!
| stack exchange is part of EleutherAI/The Pile, but AFAIK, The Pile dataset blend all sub datasets together thus we are not able to use just one of its sub dataset from The Pile data. So I create an independent dataset using The Pile preliminary components.
I also change default `timeout` to 100 seconds instead of 10 seconds, otherwise I keep getting read time out when downloading source data of stack exchange and cc100 dataset.
When I was creating dataset card. I found there is room for creating / editing dataset card. I've made it an issue. #2797
Also I am wondering whether the import of The Pile dataset is actively undertaken (because I may need it recently)? #1675 | 19 | text: add stack exchange
stack exchange is part of EleutherAI/The Pile, but AFAIK, The Pile dataset blend all sub datasets together thus we are not able to use just one of its sub dataset from The Pile data. So I create an independent dataset using The Pile preliminary components.
I also change default `timeout` to 100 seconds instead of 10 seconds, otherwise I keep getting read time out when downloading source data of stack exchange and cc100 dataset.
When I was creating dataset card. I found there is room for creating / editing dataset card. I've made it an issue. #2797
Also I am wondering whether the import of The Pile dataset is actively undertaken (because I may need it recently)? #1675
> If you don't mind I'll open a PR to do the renaming
@lhoestq That will be nice !!
|
https://github.com/huggingface/datasets/pull/2802 | add openwebtext2 | Hi ! Do you really need `jsonlines` ? I think it simply uses `json.loads` under the hood.
Currently the test are failing because `jsonlines` is not part of the extra requirements `TESTS_REQUIRE` in setup.py
So either you can replace `jsonlines` with a simple for loop on the lines of the files and use `json.loads`, or you can add `TESTS_REQUIRE` to the test requirements (but in this case users will have to install it as well). | openwebtext2 is part of EleutherAI/The Pile, but AFAIK, The Pile dataset blend all sub datasets together thus we are not able to use just one of its sub dataset from The Pile data. So I create an independent dataset using The Pile preliminary components.
When I was creating dataset card. I found there is room for creating / editing dataset card. I've made it an issue. #2797
Also I am wondering whether the import of The Pile dataset is actively undertaken (because I may need it recently)? #1675 | 75 | text: add openwebtext2
openwebtext2 is part of EleutherAI/The Pile, but AFAIK, The Pile dataset blend all sub datasets together thus we are not able to use just one of its sub dataset from The Pile data. So I create an independent dataset using The Pile preliminary components.
When I was creating dataset card. I found there is room for creating / editing dataset card. I've made it an issue. #2797
Also I am wondering whether the import of The Pile dataset is actively undertaken (because I may need it recently)? #1675
Hi ! Do you really need `jsonlines` ? I think it simply uses `json.loads` under the hood.
Currently the test are failing because `jsonlines` is not part of the extra requirements `TESTS_REQUIRE` in setup.py
So either you can replace `jsonlines` with a simple for loop on the lines of the files and use `json.loads`, or you can add `TESTS_REQUIRE` to the test requirements (but in this case users will have to install it as well). |
https://github.com/huggingface/datasets/pull/2802 | add openwebtext2 | Thanks for your suggestion. I now know `io` and json lines format better and has changed `jsonlines` to just `readlines`. | openwebtext2 is part of EleutherAI/The Pile, but AFAIK, The Pile dataset blend all sub datasets together thus we are not able to use just one of its sub dataset from The Pile data. So I create an independent dataset using The Pile preliminary components.
When I was creating dataset card. I found there is room for creating / editing dataset card. I've made it an issue. #2797
Also I am wondering whether the import of The Pile dataset is actively undertaken (because I may need it recently)? #1675 | 20 | text: add openwebtext2
openwebtext2 is part of EleutherAI/The Pile, but AFAIK, The Pile dataset blend all sub datasets together thus we are not able to use just one of its sub dataset from The Pile data. So I create an independent dataset using The Pile preliminary components.
When I was creating dataset card. I found there is room for creating / editing dataset card. I've made it an issue. #2797
Also I am wondering whether the import of The Pile dataset is actively undertaken (because I may need it recently)? #1675
Thanks for your suggestion. I now know `io` and json lines format better and has changed `jsonlines` to just `readlines`. |
https://github.com/huggingface/datasets/pull/2801 | add books3 | > When I was creating dataset card. I found there is room for creating / editing dataset card. I've made it an issue. #2797
Thanks for the message, we'll definitely improve this
> Also I am wondering whether the import of The Pile dataset is actively undertaken (because I may need it recently)? #1675
Well currently no, but I think @lewtun was about to do it (though he's currently on vacations) | books3 is part of EleutherAI/The Pile, but AFAIK, The Pile dataset blend all sub datasets together thus we are not able to use just one of its sub dataset from The Pile data. So I create an independent dataset using The Pile preliminary components.
When I was creating dataset card. I found there is room for creating / editing dataset card. I've made it an issue. #2797
Also I am wondering whether the import of The Pile dataset is actively undertaken (because I may need it recently)? #1675 | 71 | text: add books3
books3 is part of EleutherAI/The Pile, but AFAIK, The Pile dataset blend all sub datasets together thus we are not able to use just one of its sub dataset from The Pile data. So I create an independent dataset using The Pile preliminary components.
When I was creating dataset card. I found there is room for creating / editing dataset card. I've made it an issue. #2797
Also I am wondering whether the import of The Pile dataset is actively undertaken (because I may need it recently)? #1675
> When I was creating dataset card. I found there is room for creating / editing dataset card. I've made it an issue. #2797
Thanks for the message, we'll definitely improve this
> Also I am wondering whether the import of The Pile dataset is actively undertaken (because I may need it recently)? #1675
Well currently no, but I think @lewtun was about to do it (though he's currently on vacations) |
https://github.com/huggingface/datasets/pull/2801 | add books3 | > > Also I am wondering whether the import of The Pile dataset is actively undertaken (because I may need it recently)? #1675
>
> Well currently no, but I think @lewtun was about to do it (though he's currently on vacations)
yes i plan to start working on this next week #2185
one question for @richarddwang - do you know if eleutherai happened to also release the "existing" datasets like enron emails and opensubtitles?
in appendix c of their paper, they provide details on how they extracted these datasets, but it would be nice if we could just point to a url so we can be as close as possible to original implementation. | books3 is part of EleutherAI/The Pile, but AFAIK, The Pile dataset blend all sub datasets together thus we are not able to use just one of its sub dataset from The Pile data. So I create an independent dataset using The Pile preliminary components.
When I was creating dataset card. I found there is room for creating / editing dataset card. I've made it an issue. #2797
Also I am wondering whether the import of The Pile dataset is actively undertaken (because I may need it recently)? #1675 | 114 | text: add books3
books3 is part of EleutherAI/The Pile, but AFAIK, The Pile dataset blend all sub datasets together thus we are not able to use just one of its sub dataset from The Pile data. So I create an independent dataset using The Pile preliminary components.
When I was creating dataset card. I found there is room for creating / editing dataset card. I've made it an issue. #2797
Also I am wondering whether the import of The Pile dataset is actively undertaken (because I may need it recently)? #1675
> > Also I am wondering whether the import of The Pile dataset is actively undertaken (because I may need it recently)? #1675
>
> Well currently no, but I think @lewtun was about to do it (though he's currently on vacations)
yes i plan to start working on this next week #2185
one question for @richarddwang - do you know if eleutherai happened to also release the "existing" datasets like enron emails and opensubtitles?
in appendix c of their paper, they provide details on how they extracted these datasets, but it would be nice if we could just point to a url so we can be as close as possible to original implementation. |
https://github.com/huggingface/datasets/pull/2801 | add books3 | @lewtun
> yes i plan to start working on this next week
Nice! Looking forward to it.
> one question for @richarddwang - do you know if eleutherai happened to also release the "existing" datasets like enron emails and opensubtitles?
Sadly, I don't know any existing dataset of enron emails, but I believe opensubtitles dataset is hosted at here. https://the-eye.eu/public/AI/pile_preliminary_components/
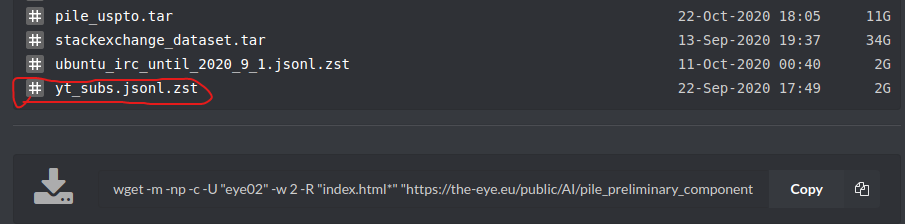
| books3 is part of EleutherAI/The Pile, but AFAIK, The Pile dataset blend all sub datasets together thus we are not able to use just one of its sub dataset from The Pile data. So I create an independent dataset using The Pile preliminary components.
When I was creating dataset card. I found there is room for creating / editing dataset card. I've made it an issue. #2797
Also I am wondering whether the import of The Pile dataset is actively undertaken (because I may need it recently)? #1675 | 61 | text: add books3
books3 is part of EleutherAI/The Pile, but AFAIK, The Pile dataset blend all sub datasets together thus we are not able to use just one of its sub dataset from The Pile data. So I create an independent dataset using The Pile preliminary components.
When I was creating dataset card. I found there is room for creating / editing dataset card. I've made it an issue. #2797
Also I am wondering whether the import of The Pile dataset is actively undertaken (because I may need it recently)? #1675
@lewtun
> yes i plan to start working on this next week
Nice! Looking forward to it.
> one question for @richarddwang - do you know if eleutherai happened to also release the "existing" datasets like enron emails and opensubtitles?
Sadly, I don't know any existing dataset of enron emails, but I believe opensubtitles dataset is hosted at here. https://the-eye.eu/public/AI/pile_preliminary_components/
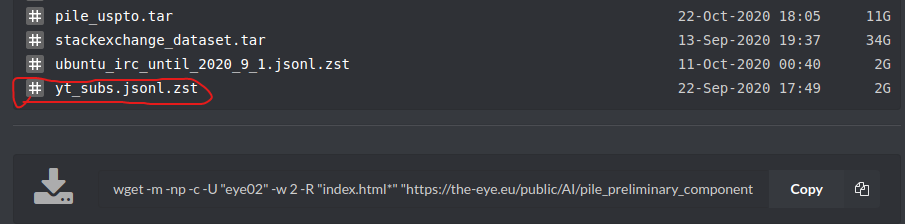
|
https://github.com/huggingface/datasets/pull/2801 | add books3 | thanks for the link @richarddwang! i think that corpus is actually the youtube subtitles one and my impression is that eleutherai have only uploaded the 14 new datasets they created. i've contacted one of the authors so hopefully they can share some additional info for us :)
btw it might take a while to put together all the corpora if i also need to preprocess them (e.g. the open subtitles / enron email etc), but i expect no longer than a few weeks. | books3 is part of EleutherAI/The Pile, but AFAIK, The Pile dataset blend all sub datasets together thus we are not able to use just one of its sub dataset from The Pile data. So I create an independent dataset using The Pile preliminary components.
When I was creating dataset card. I found there is room for creating / editing dataset card. I've made it an issue. #2797
Also I am wondering whether the import of The Pile dataset is actively undertaken (because I may need it recently)? #1675 | 83 | text: add books3
books3 is part of EleutherAI/The Pile, but AFAIK, The Pile dataset blend all sub datasets together thus we are not able to use just one of its sub dataset from The Pile data. So I create an independent dataset using The Pile preliminary components.
When I was creating dataset card. I found there is room for creating / editing dataset card. I've made it an issue. #2797
Also I am wondering whether the import of The Pile dataset is actively undertaken (because I may need it recently)? #1675
thanks for the link @richarddwang! i think that corpus is actually the youtube subtitles one and my impression is that eleutherai have only uploaded the 14 new datasets they created. i've contacted one of the authors so hopefully they can share some additional info for us :)
btw it might take a while to put together all the corpora if i also need to preprocess them (e.g. the open subtitles / enron email etc), but i expect no longer than a few weeks. |
https://github.com/huggingface/datasets/pull/2800 | Support streaming tar files | Hi ! Why do we need the custom `readline` for exactly ? feel free to add a comment to say why it's needed | This PR adds support to stream tar files by using the `fsspec` tar protocol.
It also uses the custom `readline` implemented in PR #2786.
The corresponding test is implemented in PR #2786. | 23 | text: Support streaming tar files
This PR adds support to stream tar files by using the `fsspec` tar protocol.
It also uses the custom `readline` implemented in PR #2786.
The corresponding test is implemented in PR #2786.
Hi ! Why do we need the custom `readline` for exactly ? feel free to add a comment to say why it's needed |
https://github.com/huggingface/datasets/pull/2798 | Fix streaming zip files | Hi ! I don't fully understand this change @albertvillanova
The `_extract` method used to return the compound URL that points to the root of the inside of the archive.
This way users can use the usual os.path.join or other functions to point to the relevant files. I don't see why you're using a glob pattern ? | Currently, streaming remote zip data files gives `FileNotFoundError` message:
```python
data_files = f"https://huggingface.co/datasets/albertvillanova/datasets-tests-compression/resolve/main/sample.zip"
ds = load_dataset("json", split="train", data_files=data_files, streaming=True)
next(iter(ds))
```
This PR fixes it by adding a glob string.
The corresponding test is implemented in PR #2786. | 56 | text: Fix streaming zip files
Currently, streaming remote zip data files gives `FileNotFoundError` message:
```python
data_files = f"https://huggingface.co/datasets/albertvillanova/datasets-tests-compression/resolve/main/sample.zip"
ds = load_dataset("json", split="train", data_files=data_files, streaming=True)
next(iter(ds))
```
This PR fixes it by adding a glob string.
The corresponding test is implemented in PR #2786.
Hi ! I don't fully understand this change @albertvillanova
The `_extract` method used to return the compound URL that points to the root of the inside of the archive.
This way users can use the usual os.path.join or other functions to point to the relevant files. I don't see why you're using a glob pattern ? |
https://github.com/huggingface/datasets/pull/2798 | Fix streaming zip files | This change is to allow this:
```python
data_files = f"https://huggingface.co/datasets/albertvillanova/datasets-tests-compression/resolve/main/sample.zip"
ds = load_dataset("json", split="train", data_files=data_files, streaming=True)
assert isinstance(ds, IterableDataset)
```
Note that in this case the user will not call os.path.join.
Before this PR it gave error because pointing to the root, without any subsequent join, gives error:
```python
fsspec.open("zip://::https://huggingface.co/datasets/albertvillanova/datasets-tests-compression/resolve/main/sample.zip")
``` | Currently, streaming remote zip data files gives `FileNotFoundError` message:
```python
data_files = f"https://huggingface.co/datasets/albertvillanova/datasets-tests-compression/resolve/main/sample.zip"
ds = load_dataset("json", split="train", data_files=data_files, streaming=True)
next(iter(ds))
```
This PR fixes it by adding a glob string.
The corresponding test is implemented in PR #2786. | 51 | text: Fix streaming zip files
Currently, streaming remote zip data files gives `FileNotFoundError` message:
```python
data_files = f"https://huggingface.co/datasets/albertvillanova/datasets-tests-compression/resolve/main/sample.zip"
ds = load_dataset("json", split="train", data_files=data_files, streaming=True)
next(iter(ds))
```
This PR fixes it by adding a glob string.
The corresponding test is implemented in PR #2786.
This change is to allow this:
```python
data_files = f"https://huggingface.co/datasets/albertvillanova/datasets-tests-compression/resolve/main/sample.zip"
ds = load_dataset("json", split="train", data_files=data_files, streaming=True)
assert isinstance(ds, IterableDataset)
```
Note that in this case the user will not call os.path.join.
Before this PR it gave error because pointing to the root, without any subsequent join, gives error:
```python
fsspec.open("zip://::https://huggingface.co/datasets/albertvillanova/datasets-tests-compression/resolve/main/sample.zip")
``` |
https://github.com/huggingface/datasets/pull/2796 | add cedr dataset | > Hi ! Thanks a lot for adding this one :)
>
> Good job with the dataset card and the dataset script !
>
> I left a few suggestions
Thank you very much for your helpful suggestions. I have tried to carry them all out. | null | 47 | text: add cedr dataset
> Hi ! Thanks a lot for adding this one :)
>
> Good job with the dataset card and the dataset script !
>
> I left a few suggestions
Thank you very much for your helpful suggestions. I have tried to carry them all out. |
https://github.com/huggingface/datasets/pull/2792 | Update: GooAQ - add train/val/test splits | @albertvillanova my tests are failing here:
```
dataset_name = 'gooaq'
def test_load_dataset(self, dataset_name):
configs = self.dataset_tester.load_all_configs(dataset_name, is_local=True)[:1]
> self.dataset_tester.check_load_dataset(dataset_name, configs, is_local=True, use_local_dummy_data=True)
tests/test_dataset_common.py:234:
_ _ _ _ _ _ _ _ _ _ _ _ _ _ _ _ _ _ _ _ _ _ _ _ _ _ _ _ _ _ _ _ _ _ _ _ _ _ _ _
tests/test_dataset_common.py:187: in check_load_dataset
self.parent.assertTrue(len(dataset[split]) > 0)
E AssertionError: False is not true
```
When I try loading dataset on local machine it works fine. Any suggestions on how can I avoid this error? | [GooAQ](https://github.com/allenai/gooaq) dataset was recently updated after splits were added for the same. This PR contains new updated GooAQ with train/val/test splits and updated README as well. | 96 | text: Update: GooAQ - add train/val/test splits
[GooAQ](https://github.com/allenai/gooaq) dataset was recently updated after splits were added for the same. This PR contains new updated GooAQ with train/val/test splits and updated README as well.
@albertvillanova my tests are failing here:
```
dataset_name = 'gooaq'
def test_load_dataset(self, dataset_name):
configs = self.dataset_tester.load_all_configs(dataset_name, is_local=True)[:1]
> self.dataset_tester.check_load_dataset(dataset_name, configs, is_local=True, use_local_dummy_data=True)
tests/test_dataset_common.py:234:
_ _ _ _ _ _ _ _ _ _ _ _ _ _ _ _ _ _ _ _ _ _ _ _ _ _ _ _ _ _ _ _ _ _ _ _ _ _ _ _
tests/test_dataset_common.py:187: in check_load_dataset
self.parent.assertTrue(len(dataset[split]) > 0)
E AssertionError: False is not true
```
When I try loading dataset on local machine it works fine. Any suggestions on how can I avoid this error? |
https://github.com/huggingface/datasets/pull/2783 | Add KS task to SUPERB | thanks a lot for implementing this @anton-l !!
i won't have time to review this while i'm away, so happy for @albertvillanova and @patrickvonplaten to decide when to merge :) | Add the KS (keyword spotting) task as described in the [SUPERB paper](https://arxiv.org/abs/2105.01051).
- [s3prl instructions](https://github.com/s3prl/s3prl/blob/master/s3prl/downstream/README.md#ks-keyword-spotting)
- [s3prl implementation](https://github.com/s3prl/s3prl/blob/master/s3prl/downstream/speech_commands/dataset.py)
- [TFDS implementation](https://github.com/tensorflow/datasets/blob/master/tensorflow_datasets/audio/speech_commands.py)
Some notable quirks:
- The dataset is originally single-archive (train+val+test all in one), but the test set has a "canonical" distribution in a separate archive, which is also used here (see `_split_ks_files()`).
- The `_background_noise_`/`_silence_` audio files are much longer than others, so they require some sort of slicing for downstream training. I decided to leave the implementation of that up to the users, since TFDS and s3prl take different approaches (either slicing wavs deterministically, or subsampling randomly at runtime)
Related to #2619. | 30 | text: Add KS task to SUPERB
Add the KS (keyword spotting) task as described in the [SUPERB paper](https://arxiv.org/abs/2105.01051).
- [s3prl instructions](https://github.com/s3prl/s3prl/blob/master/s3prl/downstream/README.md#ks-keyword-spotting)
- [s3prl implementation](https://github.com/s3prl/s3prl/blob/master/s3prl/downstream/speech_commands/dataset.py)
- [TFDS implementation](https://github.com/tensorflow/datasets/blob/master/tensorflow_datasets/audio/speech_commands.py)
Some notable quirks:
- The dataset is originally single-archive (train+val+test all in one), but the test set has a "canonical" distribution in a separate archive, which is also used here (see `_split_ks_files()`).
- The `_background_noise_`/`_silence_` audio files are much longer than others, so they require some sort of slicing for downstream training. I decided to leave the implementation of that up to the users, since TFDS and s3prl take different approaches (either slicing wavs deterministically, or subsampling randomly at runtime)
Related to #2619.
thanks a lot for implementing this @anton-l !!
i won't have time to review this while i'm away, so happy for @albertvillanova and @patrickvonplaten to decide when to merge :) |
https://github.com/huggingface/datasets/pull/2783 | Add KS task to SUPERB | > The _background_noise_/_silence_ audio files are much longer than others, so they require some sort of slicing for downstream training. I decided to leave the implementation of that up to the users, since TFDS and s3prl take different approaches (either slicing wavs deterministically, or subsampling randomly at runtime)
@anton-l I was thinking that maybe we could give some hints in the dataset card (in a Usage section); something similar as for diarization: https://github.com/huggingface/datasets/blob/master/datasets/superb/README.md#example-of-usage
Note that for diarization it is not yet finished: we have to test it and then provide an end-to-end example: https://github.com/huggingface/datasets/pull/2661/files#r680224909 | Add the KS (keyword spotting) task as described in the [SUPERB paper](https://arxiv.org/abs/2105.01051).
- [s3prl instructions](https://github.com/s3prl/s3prl/blob/master/s3prl/downstream/README.md#ks-keyword-spotting)
- [s3prl implementation](https://github.com/s3prl/s3prl/blob/master/s3prl/downstream/speech_commands/dataset.py)
- [TFDS implementation](https://github.com/tensorflow/datasets/blob/master/tensorflow_datasets/audio/speech_commands.py)
Some notable quirks:
- The dataset is originally single-archive (train+val+test all in one), but the test set has a "canonical" distribution in a separate archive, which is also used here (see `_split_ks_files()`).
- The `_background_noise_`/`_silence_` audio files are much longer than others, so they require some sort of slicing for downstream training. I decided to leave the implementation of that up to the users, since TFDS and s3prl take different approaches (either slicing wavs deterministically, or subsampling randomly at runtime)
Related to #2619. | 94 | text: Add KS task to SUPERB
Add the KS (keyword spotting) task as described in the [SUPERB paper](https://arxiv.org/abs/2105.01051).
- [s3prl instructions](https://github.com/s3prl/s3prl/blob/master/s3prl/downstream/README.md#ks-keyword-spotting)
- [s3prl implementation](https://github.com/s3prl/s3prl/blob/master/s3prl/downstream/speech_commands/dataset.py)
- [TFDS implementation](https://github.com/tensorflow/datasets/blob/master/tensorflow_datasets/audio/speech_commands.py)
Some notable quirks:
- The dataset is originally single-archive (train+val+test all in one), but the test set has a "canonical" distribution in a separate archive, which is also used here (see `_split_ks_files()`).
- The `_background_noise_`/`_silence_` audio files are much longer than others, so they require some sort of slicing for downstream training. I decided to leave the implementation of that up to the users, since TFDS and s3prl take different approaches (either slicing wavs deterministically, or subsampling randomly at runtime)
Related to #2619.
> The _background_noise_/_silence_ audio files are much longer than others, so they require some sort of slicing for downstream training. I decided to leave the implementation of that up to the users, since TFDS and s3prl take different approaches (either slicing wavs deterministically, or subsampling randomly at runtime)
@anton-l I was thinking that maybe we could give some hints in the dataset card (in a Usage section); something similar as for diarization: https://github.com/huggingface/datasets/blob/master/datasets/superb/README.md#example-of-usage
Note that for diarization it is not yet finished: we have to test it and then provide an end-to-end example: https://github.com/huggingface/datasets/pull/2661/files#r680224909 |
https://github.com/huggingface/datasets/pull/2783 | Add KS task to SUPERB | @albertvillanova yeah, I'm not sure how to best implement it in pure `datasets` yet. It's something like this, where `sample_noise()` needs to be called from a pytorch batch collator or other framework-specific variant:
```python
def map_to_array(example):
import soundfile as sf
speech_array, sample_rate = sf.read(example["file"])
example["speech"] = speech_array
example["sample_rate"] = sample_rate
return example
def sample_noise(example):
# Use a version of this function in a stateless way to extract random 1 sec slices of background noise
# on each epoch
from random import randint
# _silence_ audios are longer than 1 sec
if example["label"] == "_silence_":
random_offset = randint(0, len(example["speech"]) - example["sample_rate"] - 1)
example["speech"] = example["speech"][random_offset : random_offset + example["sample_rate"]]
return example
``` | Add the KS (keyword spotting) task as described in the [SUPERB paper](https://arxiv.org/abs/2105.01051).
- [s3prl instructions](https://github.com/s3prl/s3prl/blob/master/s3prl/downstream/README.md#ks-keyword-spotting)
- [s3prl implementation](https://github.com/s3prl/s3prl/blob/master/s3prl/downstream/speech_commands/dataset.py)
- [TFDS implementation](https://github.com/tensorflow/datasets/blob/master/tensorflow_datasets/audio/speech_commands.py)
Some notable quirks:
- The dataset is originally single-archive (train+val+test all in one), but the test set has a "canonical" distribution in a separate archive, which is also used here (see `_split_ks_files()`).
- The `_background_noise_`/`_silence_` audio files are much longer than others, so they require some sort of slicing for downstream training. I decided to leave the implementation of that up to the users, since TFDS and s3prl take different approaches (either slicing wavs deterministically, or subsampling randomly at runtime)
Related to #2619. | 112 | text: Add KS task to SUPERB
Add the KS (keyword spotting) task as described in the [SUPERB paper](https://arxiv.org/abs/2105.01051).
- [s3prl instructions](https://github.com/s3prl/s3prl/blob/master/s3prl/downstream/README.md#ks-keyword-spotting)
- [s3prl implementation](https://github.com/s3prl/s3prl/blob/master/s3prl/downstream/speech_commands/dataset.py)
- [TFDS implementation](https://github.com/tensorflow/datasets/blob/master/tensorflow_datasets/audio/speech_commands.py)
Some notable quirks:
- The dataset is originally single-archive (train+val+test all in one), but the test set has a "canonical" distribution in a separate archive, which is also used here (see `_split_ks_files()`).
- The `_background_noise_`/`_silence_` audio files are much longer than others, so they require some sort of slicing for downstream training. I decided to leave the implementation of that up to the users, since TFDS and s3prl take different approaches (either slicing wavs deterministically, or subsampling randomly at runtime)
Related to #2619.
@albertvillanova yeah, I'm not sure how to best implement it in pure `datasets` yet. It's something like this, where `sample_noise()` needs to be called from a pytorch batch collator or other framework-specific variant:
```python
def map_to_array(example):
import soundfile as sf
speech_array, sample_rate = sf.read(example["file"])
example["speech"] = speech_array
example["sample_rate"] = sample_rate
return example
def sample_noise(example):
# Use a version of this function in a stateless way to extract random 1 sec slices of background noise
# on each epoch
from random import randint
# _silence_ audios are longer than 1 sec
if example["label"] == "_silence_":
random_offset = randint(0, len(example["speech"]) - example["sample_rate"] - 1)
example["speech"] = example["speech"][random_offset : random_offset + example["sample_rate"]]
return example
``` |
https://github.com/huggingface/datasets/pull/2783 | Add KS task to SUPERB | I see... Yes, not trivial indeed. Maybe for the moment you could add those functions above to the README (as it is the case for now in diarization)? What do you think? | Add the KS (keyword spotting) task as described in the [SUPERB paper](https://arxiv.org/abs/2105.01051).
- [s3prl instructions](https://github.com/s3prl/s3prl/blob/master/s3prl/downstream/README.md#ks-keyword-spotting)
- [s3prl implementation](https://github.com/s3prl/s3prl/blob/master/s3prl/downstream/speech_commands/dataset.py)
- [TFDS implementation](https://github.com/tensorflow/datasets/blob/master/tensorflow_datasets/audio/speech_commands.py)
Some notable quirks:
- The dataset is originally single-archive (train+val+test all in one), but the test set has a "canonical" distribution in a separate archive, which is also used here (see `_split_ks_files()`).
- The `_background_noise_`/`_silence_` audio files are much longer than others, so they require some sort of slicing for downstream training. I decided to leave the implementation of that up to the users, since TFDS and s3prl take different approaches (either slicing wavs deterministically, or subsampling randomly at runtime)
Related to #2619. | 32 | text: Add KS task to SUPERB
Add the KS (keyword spotting) task as described in the [SUPERB paper](https://arxiv.org/abs/2105.01051).
- [s3prl instructions](https://github.com/s3prl/s3prl/blob/master/s3prl/downstream/README.md#ks-keyword-spotting)
- [s3prl implementation](https://github.com/s3prl/s3prl/blob/master/s3prl/downstream/speech_commands/dataset.py)
- [TFDS implementation](https://github.com/tensorflow/datasets/blob/master/tensorflow_datasets/audio/speech_commands.py)
Some notable quirks:
- The dataset is originally single-archive (train+val+test all in one), but the test set has a "canonical" distribution in a separate archive, which is also used here (see `_split_ks_files()`).
- The `_background_noise_`/`_silence_` audio files are much longer than others, so they require some sort of slicing for downstream training. I decided to leave the implementation of that up to the users, since TFDS and s3prl take different approaches (either slicing wavs deterministically, or subsampling randomly at runtime)
Related to #2619.
I see... Yes, not trivial indeed. Maybe for the moment you could add those functions above to the README (as it is the case for now in diarization)? What do you think? |
https://github.com/huggingface/datasets/pull/2774 | Prevent .map from using multiprocessing when loading from cache | Hi @thomasw21, yes you are right: those failing tests were fixed with #2779.
Would you mind to merge current upstream master branch and push again?
```
git checkout sequential_map_when_cached
git fetch upstream master
git merge upstream/master
git push -u origin sequential_map_when_cached
``` | ## Context
On our setup, we use different setup to train vs proprocessing datasets. Usually we are able to obtain a high number of cpus to preprocess, which allows us to use `num_proc` however we can't use as many during training phase. Currently if we use `num_proc={whatever the preprocessing value was}` we load from cache, but we get:
```
Traceback (most recent call last):
File "lib/python3.8/site-packages/multiprocess/pool.py", line 131, in worker
put((job, i, result))
File "lib/python3.8/site-packages/multiprocess/queues.py", line 371, in put
self._writer.send_bytes(obj)
File "lib/python3.8/site-packages/multiprocess/connection.py", line 203, in send_bytes
self._send_bytes(m[offset:offset + size])
File "lib/python3.8/site-packages/multiprocess/connection.py", line 414, in _send_bytes
self._send(header + buf)
File "lib/python3.8/site-packages/multiprocess/connection.py", line 371, in _send
n = write(self._handle, buf)
BrokenPipeError: [Errno 32] Broken pipe
```
Our current guess, is that we're spawning too many processes compared to the number of cpus available, and it's running OOM. Also we're loading this in DDP setting which means that for each gpu, I need to spawn a high number of processes to match the preprocessing fingerprint.
Instead what we suggest:
- Allow loading shard sequentially, sharing the same fingerprint as the multiprocessed one, in order to leverage multiprocessing when we actually generate the cache, and remove it when loading from cache.
## Current issues
~I'm having a hard time making fingerprints match. For some reason, the multiprocessing and the sequential version generate two different hash.~
**EDIT**: Turns out multiprocessing and sequential have different `transform` value for fingerprinting (check `fingerprint_transform`) when running `_map_single`:
- sequential : `datasets.arrow_dataset.Dataset._map_single`
- multiprocessing: `datasets.arrow_dataset._map_single`
This discrepancy is caused by multiprocessing pickling the transformer function, it doesn't seem to keep the `Dataset` hierarchy. I'm still unclear on why `func.__qual_name__` isn't handled correctly in multiprocessing. But replacing `__qualname__` by `__name__` fixes the issue.
## What was done
~We try to prevent the usage of multiprocessing when loading a dataset. Instead we load all cached shards sequentially.~
I couldn't find a nice way to obtain the cached_file_name and check they all exist before deciding to use the multiprocessing flow or not. Instead I expose an optional boolean `sequential` in `map` method.
## TODO
- [x] Check that the multiprocessed version and the sequential version output the same output
- [x] Check that sequential can load multiprocessed
- [x] Check that multiprocessed can load sequential
## Test
```python
from datasets import load_dataset
from multiprocessing import Pool
import random
def process(batch, rng):
length = len(batch["text"])
return {**batch, "processed_text": [f"PROCESSED {rng.random()}" for _ in range(length)]}
dataset = load_dataset("stas/openwebtext-10k", split="train")
print(dataset.column_names)
print(type(dataset))
rng = random.Random(42)
dataset1 = dataset.map(process, batched=True, batch_size=50, num_proc=4, fn_kwargs={"rng": rng})
# This one should be loaded from cache
rng = random.Random(42)
dataset2 = dataset.map(process, batched=True, batch_size=50, num_proc=4, fn_kwargs={"rng": rng}, sequential=True)
# Just to check that the random generator was correct
print(dataset1[-1]["processed_text"])
print(dataset2[-1]["processed_text"])
```
## Other solutions
I chose to load everything sequentially, but we can probably find a way to load shards in parallel using another number of workers (essentially this would be an argument not used for fingerprinting, allowing to allow `m` shards using `n` processes, which would be very useful when same dataset have to be loaded on two different setup, and we still want to leverage cache).
Also we can use a env variable similarly to `TOKENIZERS_PARALLELISM` as this seems generally setup related (though this changes slightly if we use multiprocessing).
cc @lhoestq (since I had asked you previously on `num_proc` being used for fingerprinting). Don't know if this is acceptable. | 42 | text: Prevent .map from using multiprocessing when loading from cache
## Context
On our setup, we use different setup to train vs proprocessing datasets. Usually we are able to obtain a high number of cpus to preprocess, which allows us to use `num_proc` however we can't use as many during training phase. Currently if we use `num_proc={whatever the preprocessing value was}` we load from cache, but we get:
```
Traceback (most recent call last):
File "lib/python3.8/site-packages/multiprocess/pool.py", line 131, in worker
put((job, i, result))
File "lib/python3.8/site-packages/multiprocess/queues.py", line 371, in put
self._writer.send_bytes(obj)
File "lib/python3.8/site-packages/multiprocess/connection.py", line 203, in send_bytes
self._send_bytes(m[offset:offset + size])
File "lib/python3.8/site-packages/multiprocess/connection.py", line 414, in _send_bytes
self._send(header + buf)
File "lib/python3.8/site-packages/multiprocess/connection.py", line 371, in _send
n = write(self._handle, buf)
BrokenPipeError: [Errno 32] Broken pipe
```
Our current guess, is that we're spawning too many processes compared to the number of cpus available, and it's running OOM. Also we're loading this in DDP setting which means that for each gpu, I need to spawn a high number of processes to match the preprocessing fingerprint.
Instead what we suggest:
- Allow loading shard sequentially, sharing the same fingerprint as the multiprocessed one, in order to leverage multiprocessing when we actually generate the cache, and remove it when loading from cache.
## Current issues
~I'm having a hard time making fingerprints match. For some reason, the multiprocessing and the sequential version generate two different hash.~
**EDIT**: Turns out multiprocessing and sequential have different `transform` value for fingerprinting (check `fingerprint_transform`) when running `_map_single`:
- sequential : `datasets.arrow_dataset.Dataset._map_single`
- multiprocessing: `datasets.arrow_dataset._map_single`
This discrepancy is caused by multiprocessing pickling the transformer function, it doesn't seem to keep the `Dataset` hierarchy. I'm still unclear on why `func.__qual_name__` isn't handled correctly in multiprocessing. But replacing `__qualname__` by `__name__` fixes the issue.
## What was done
~We try to prevent the usage of multiprocessing when loading a dataset. Instead we load all cached shards sequentially.~
I couldn't find a nice way to obtain the cached_file_name and check they all exist before deciding to use the multiprocessing flow or not. Instead I expose an optional boolean `sequential` in `map` method.
## TODO
- [x] Check that the multiprocessed version and the sequential version output the same output
- [x] Check that sequential can load multiprocessed
- [x] Check that multiprocessed can load sequential
## Test
```python
from datasets import load_dataset
from multiprocessing import Pool
import random
def process(batch, rng):
length = len(batch["text"])
return {**batch, "processed_text": [f"PROCESSED {rng.random()}" for _ in range(length)]}
dataset = load_dataset("stas/openwebtext-10k", split="train")
print(dataset.column_names)
print(type(dataset))
rng = random.Random(42)
dataset1 = dataset.map(process, batched=True, batch_size=50, num_proc=4, fn_kwargs={"rng": rng})
# This one should be loaded from cache
rng = random.Random(42)
dataset2 = dataset.map(process, batched=True, batch_size=50, num_proc=4, fn_kwargs={"rng": rng}, sequential=True)
# Just to check that the random generator was correct
print(dataset1[-1]["processed_text"])
print(dataset2[-1]["processed_text"])
```
## Other solutions
I chose to load everything sequentially, but we can probably find a way to load shards in parallel using another number of workers (essentially this would be an argument not used for fingerprinting, allowing to allow `m` shards using `n` processes, which would be very useful when same dataset have to be loaded on two different setup, and we still want to leverage cache).
Also we can use a env variable similarly to `TOKENIZERS_PARALLELISM` as this seems generally setup related (though this changes slightly if we use multiprocessing).
cc @lhoestq (since I had asked you previously on `num_proc` being used for fingerprinting). Don't know if this is acceptable.
Hi @thomasw21, yes you are right: those failing tests were fixed with #2779.
Would you mind to merge current upstream master branch and push again?
```
git checkout sequential_map_when_cached
git fetch upstream master
git merge upstream/master
git push -u origin sequential_map_when_cached
``` |
https://github.com/huggingface/datasets/pull/2774 | Prevent .map from using multiprocessing when loading from cache | Thanks for working on this ! I'm sure we can figure something out ;)
Currently `map` starts a process to apply the map function on each shard. If the shard has already been processed, then the process that has been spawned loads the processed shard from the cache and returns it.
I think we should be able to simply not start a process if a shard is already processed and cached.
This way:
- you won't need to specify `sequential=True`
- it won't create new processes if the dataset is already processed and cached
- it will properly reload each processed shard that is cached
To know if we have to start a new process for a shard you can use the function `update_fingerprint` from fingerprint.py to know the expected fingerprint of the processed shard.
Then, if the shard has already been processed, there will be a cache file named `cached-<new_fingerprint>.arrow` and you can load it with
```
Dataset.from_file(path_to_cache_file, info=self.info, split=self.split)
```
Let me know if that makes sense ! | ## Context
On our setup, we use different setup to train vs proprocessing datasets. Usually we are able to obtain a high number of cpus to preprocess, which allows us to use `num_proc` however we can't use as many during training phase. Currently if we use `num_proc={whatever the preprocessing value was}` we load from cache, but we get:
```
Traceback (most recent call last):
File "lib/python3.8/site-packages/multiprocess/pool.py", line 131, in worker
put((job, i, result))
File "lib/python3.8/site-packages/multiprocess/queues.py", line 371, in put
self._writer.send_bytes(obj)
File "lib/python3.8/site-packages/multiprocess/connection.py", line 203, in send_bytes
self._send_bytes(m[offset:offset + size])
File "lib/python3.8/site-packages/multiprocess/connection.py", line 414, in _send_bytes
self._send(header + buf)
File "lib/python3.8/site-packages/multiprocess/connection.py", line 371, in _send
n = write(self._handle, buf)
BrokenPipeError: [Errno 32] Broken pipe
```
Our current guess, is that we're spawning too many processes compared to the number of cpus available, and it's running OOM. Also we're loading this in DDP setting which means that for each gpu, I need to spawn a high number of processes to match the preprocessing fingerprint.
Instead what we suggest:
- Allow loading shard sequentially, sharing the same fingerprint as the multiprocessed one, in order to leverage multiprocessing when we actually generate the cache, and remove it when loading from cache.
## Current issues
~I'm having a hard time making fingerprints match. For some reason, the multiprocessing and the sequential version generate two different hash.~
**EDIT**: Turns out multiprocessing and sequential have different `transform` value for fingerprinting (check `fingerprint_transform`) when running `_map_single`:
- sequential : `datasets.arrow_dataset.Dataset._map_single`
- multiprocessing: `datasets.arrow_dataset._map_single`
This discrepancy is caused by multiprocessing pickling the transformer function, it doesn't seem to keep the `Dataset` hierarchy. I'm still unclear on why `func.__qual_name__` isn't handled correctly in multiprocessing. But replacing `__qualname__` by `__name__` fixes the issue.
## What was done
~We try to prevent the usage of multiprocessing when loading a dataset. Instead we load all cached shards sequentially.~
I couldn't find a nice way to obtain the cached_file_name and check they all exist before deciding to use the multiprocessing flow or not. Instead I expose an optional boolean `sequential` in `map` method.
## TODO
- [x] Check that the multiprocessed version and the sequential version output the same output
- [x] Check that sequential can load multiprocessed
- [x] Check that multiprocessed can load sequential
## Test
```python
from datasets import load_dataset
from multiprocessing import Pool
import random
def process(batch, rng):
length = len(batch["text"])
return {**batch, "processed_text": [f"PROCESSED {rng.random()}" for _ in range(length)]}
dataset = load_dataset("stas/openwebtext-10k", split="train")
print(dataset.column_names)
print(type(dataset))
rng = random.Random(42)
dataset1 = dataset.map(process, batched=True, batch_size=50, num_proc=4, fn_kwargs={"rng": rng})
# This one should be loaded from cache
rng = random.Random(42)
dataset2 = dataset.map(process, batched=True, batch_size=50, num_proc=4, fn_kwargs={"rng": rng}, sequential=True)
# Just to check that the random generator was correct
print(dataset1[-1]["processed_text"])
print(dataset2[-1]["processed_text"])
```
## Other solutions
I chose to load everything sequentially, but we can probably find a way to load shards in parallel using another number of workers (essentially this would be an argument not used for fingerprinting, allowing to allow `m` shards using `n` processes, which would be very useful when same dataset have to be loaded on two different setup, and we still want to leverage cache).
Also we can use a env variable similarly to `TOKENIZERS_PARALLELISM` as this seems generally setup related (though this changes slightly if we use multiprocessing).
cc @lhoestq (since I had asked you previously on `num_proc` being used for fingerprinting). Don't know if this is acceptable. | 170 | text: Prevent .map from using multiprocessing when loading from cache
## Context
On our setup, we use different setup to train vs proprocessing datasets. Usually we are able to obtain a high number of cpus to preprocess, which allows us to use `num_proc` however we can't use as many during training phase. Currently if we use `num_proc={whatever the preprocessing value was}` we load from cache, but we get:
```
Traceback (most recent call last):
File "lib/python3.8/site-packages/multiprocess/pool.py", line 131, in worker
put((job, i, result))
File "lib/python3.8/site-packages/multiprocess/queues.py", line 371, in put
self._writer.send_bytes(obj)
File "lib/python3.8/site-packages/multiprocess/connection.py", line 203, in send_bytes
self._send_bytes(m[offset:offset + size])
File "lib/python3.8/site-packages/multiprocess/connection.py", line 414, in _send_bytes
self._send(header + buf)
File "lib/python3.8/site-packages/multiprocess/connection.py", line 371, in _send
n = write(self._handle, buf)
BrokenPipeError: [Errno 32] Broken pipe
```
Our current guess, is that we're spawning too many processes compared to the number of cpus available, and it's running OOM. Also we're loading this in DDP setting which means that for each gpu, I need to spawn a high number of processes to match the preprocessing fingerprint.
Instead what we suggest:
- Allow loading shard sequentially, sharing the same fingerprint as the multiprocessed one, in order to leverage multiprocessing when we actually generate the cache, and remove it when loading from cache.
## Current issues
~I'm having a hard time making fingerprints match. For some reason, the multiprocessing and the sequential version generate two different hash.~
**EDIT**: Turns out multiprocessing and sequential have different `transform` value for fingerprinting (check `fingerprint_transform`) when running `_map_single`:
- sequential : `datasets.arrow_dataset.Dataset._map_single`
- multiprocessing: `datasets.arrow_dataset._map_single`
This discrepancy is caused by multiprocessing pickling the transformer function, it doesn't seem to keep the `Dataset` hierarchy. I'm still unclear on why `func.__qual_name__` isn't handled correctly in multiprocessing. But replacing `__qualname__` by `__name__` fixes the issue.
## What was done
~We try to prevent the usage of multiprocessing when loading a dataset. Instead we load all cached shards sequentially.~
I couldn't find a nice way to obtain the cached_file_name and check they all exist before deciding to use the multiprocessing flow or not. Instead I expose an optional boolean `sequential` in `map` method.
## TODO
- [x] Check that the multiprocessed version and the sequential version output the same output
- [x] Check that sequential can load multiprocessed
- [x] Check that multiprocessed can load sequential
## Test
```python
from datasets import load_dataset
from multiprocessing import Pool
import random
def process(batch, rng):
length = len(batch["text"])
return {**batch, "processed_text": [f"PROCESSED {rng.random()}" for _ in range(length)]}
dataset = load_dataset("stas/openwebtext-10k", split="train")
print(dataset.column_names)
print(type(dataset))
rng = random.Random(42)
dataset1 = dataset.map(process, batched=True, batch_size=50, num_proc=4, fn_kwargs={"rng": rng})
# This one should be loaded from cache
rng = random.Random(42)
dataset2 = dataset.map(process, batched=True, batch_size=50, num_proc=4, fn_kwargs={"rng": rng}, sequential=True)
# Just to check that the random generator was correct
print(dataset1[-1]["processed_text"])
print(dataset2[-1]["processed_text"])
```
## Other solutions
I chose to load everything sequentially, but we can probably find a way to load shards in parallel using another number of workers (essentially this would be an argument not used for fingerprinting, allowing to allow `m` shards using `n` processes, which would be very useful when same dataset have to be loaded on two different setup, and we still want to leverage cache).
Also we can use a env variable similarly to `TOKENIZERS_PARALLELISM` as this seems generally setup related (though this changes slightly if we use multiprocessing).
cc @lhoestq (since I had asked you previously on `num_proc` being used for fingerprinting). Don't know if this is acceptable.
Thanks for working on this ! I'm sure we can figure something out ;)
Currently `map` starts a process to apply the map function on each shard. If the shard has already been processed, then the process that has been spawned loads the processed shard from the cache and returns it.
I think we should be able to simply not start a process if a shard is already processed and cached.
This way:
- you won't need to specify `sequential=True`
- it won't create new processes if the dataset is already processed and cached
- it will properly reload each processed shard that is cached
To know if we have to start a new process for a shard you can use the function `update_fingerprint` from fingerprint.py to know the expected fingerprint of the processed shard.
Then, if the shard has already been processed, there will be a cache file named `cached-<new_fingerprint>.arrow` and you can load it with
```
Dataset.from_file(path_to_cache_file, info=self.info, split=self.split)
```
Let me know if that makes sense ! |
https://github.com/huggingface/datasets/pull/2774 | Prevent .map from using multiprocessing when loading from cache | Yes that makes total sense, I tried to initially do that, except the way fingerprint is handled doesn't allow to easily manipulate such a field. Typically the fingerprinting is handled in `@fingerprint_transform` which has a bunch of params that aren't quite easy to extract. Those params are used to manipulate args, kwargs in fancy ways in order to finally obtain a dictionary used for fingerprint. I could duplicate everything, but this look like a very risky thing to do. I'll take a look if I can make something work with `inspect` if I can make a very simple wrapper.
A much more simpler solution I think is adding an optional `shard: Optional[int] = None` parameter. If None, we use the number of proc as the number of shards, otherwise we pass down the expected number of shards and use either sequential/multiprocessing (with arbitrary number of workers) to load the shards? This would allow the weird case where one wants a large number of shards with a limited amount of processes. Not the smartest thing to do, but it's not an absurd behaviour. Would this be acceptable? | ## Context
On our setup, we use different setup to train vs proprocessing datasets. Usually we are able to obtain a high number of cpus to preprocess, which allows us to use `num_proc` however we can't use as many during training phase. Currently if we use `num_proc={whatever the preprocessing value was}` we load from cache, but we get:
```
Traceback (most recent call last):
File "lib/python3.8/site-packages/multiprocess/pool.py", line 131, in worker
put((job, i, result))
File "lib/python3.8/site-packages/multiprocess/queues.py", line 371, in put
self._writer.send_bytes(obj)
File "lib/python3.8/site-packages/multiprocess/connection.py", line 203, in send_bytes
self._send_bytes(m[offset:offset + size])
File "lib/python3.8/site-packages/multiprocess/connection.py", line 414, in _send_bytes
self._send(header + buf)
File "lib/python3.8/site-packages/multiprocess/connection.py", line 371, in _send
n = write(self._handle, buf)
BrokenPipeError: [Errno 32] Broken pipe
```
Our current guess, is that we're spawning too many processes compared to the number of cpus available, and it's running OOM. Also we're loading this in DDP setting which means that for each gpu, I need to spawn a high number of processes to match the preprocessing fingerprint.
Instead what we suggest:
- Allow loading shard sequentially, sharing the same fingerprint as the multiprocessed one, in order to leverage multiprocessing when we actually generate the cache, and remove it when loading from cache.
## Current issues
~I'm having a hard time making fingerprints match. For some reason, the multiprocessing and the sequential version generate two different hash.~
**EDIT**: Turns out multiprocessing and sequential have different `transform` value for fingerprinting (check `fingerprint_transform`) when running `_map_single`:
- sequential : `datasets.arrow_dataset.Dataset._map_single`
- multiprocessing: `datasets.arrow_dataset._map_single`
This discrepancy is caused by multiprocessing pickling the transformer function, it doesn't seem to keep the `Dataset` hierarchy. I'm still unclear on why `func.__qual_name__` isn't handled correctly in multiprocessing. But replacing `__qualname__` by `__name__` fixes the issue.
## What was done
~We try to prevent the usage of multiprocessing when loading a dataset. Instead we load all cached shards sequentially.~
I couldn't find a nice way to obtain the cached_file_name and check they all exist before deciding to use the multiprocessing flow or not. Instead I expose an optional boolean `sequential` in `map` method.
## TODO
- [x] Check that the multiprocessed version and the sequential version output the same output
- [x] Check that sequential can load multiprocessed
- [x] Check that multiprocessed can load sequential
## Test
```python
from datasets import load_dataset
from multiprocessing import Pool
import random
def process(batch, rng):
length = len(batch["text"])
return {**batch, "processed_text": [f"PROCESSED {rng.random()}" for _ in range(length)]}
dataset = load_dataset("stas/openwebtext-10k", split="train")
print(dataset.column_names)
print(type(dataset))
rng = random.Random(42)
dataset1 = dataset.map(process, batched=True, batch_size=50, num_proc=4, fn_kwargs={"rng": rng})
# This one should be loaded from cache
rng = random.Random(42)
dataset2 = dataset.map(process, batched=True, batch_size=50, num_proc=4, fn_kwargs={"rng": rng}, sequential=True)
# Just to check that the random generator was correct
print(dataset1[-1]["processed_text"])
print(dataset2[-1]["processed_text"])
```
## Other solutions
I chose to load everything sequentially, but we can probably find a way to load shards in parallel using another number of workers (essentially this would be an argument not used for fingerprinting, allowing to allow `m` shards using `n` processes, which would be very useful when same dataset have to be loaded on two different setup, and we still want to leverage cache).
Also we can use a env variable similarly to `TOKENIZERS_PARALLELISM` as this seems generally setup related (though this changes slightly if we use multiprocessing).
cc @lhoestq (since I had asked you previously on `num_proc` being used for fingerprinting). Don't know if this is acceptable. | 186 | text: Prevent .map from using multiprocessing when loading from cache
## Context
On our setup, we use different setup to train vs proprocessing datasets. Usually we are able to obtain a high number of cpus to preprocess, which allows us to use `num_proc` however we can't use as many during training phase. Currently if we use `num_proc={whatever the preprocessing value was}` we load from cache, but we get:
```
Traceback (most recent call last):
File "lib/python3.8/site-packages/multiprocess/pool.py", line 131, in worker
put((job, i, result))
File "lib/python3.8/site-packages/multiprocess/queues.py", line 371, in put
self._writer.send_bytes(obj)
File "lib/python3.8/site-packages/multiprocess/connection.py", line 203, in send_bytes
self._send_bytes(m[offset:offset + size])
File "lib/python3.8/site-packages/multiprocess/connection.py", line 414, in _send_bytes
self._send(header + buf)
File "lib/python3.8/site-packages/multiprocess/connection.py", line 371, in _send
n = write(self._handle, buf)
BrokenPipeError: [Errno 32] Broken pipe
```
Our current guess, is that we're spawning too many processes compared to the number of cpus available, and it's running OOM. Also we're loading this in DDP setting which means that for each gpu, I need to spawn a high number of processes to match the preprocessing fingerprint.
Instead what we suggest:
- Allow loading shard sequentially, sharing the same fingerprint as the multiprocessed one, in order to leverage multiprocessing when we actually generate the cache, and remove it when loading from cache.
## Current issues
~I'm having a hard time making fingerprints match. For some reason, the multiprocessing and the sequential version generate two different hash.~
**EDIT**: Turns out multiprocessing and sequential have different `transform` value for fingerprinting (check `fingerprint_transform`) when running `_map_single`:
- sequential : `datasets.arrow_dataset.Dataset._map_single`
- multiprocessing: `datasets.arrow_dataset._map_single`
This discrepancy is caused by multiprocessing pickling the transformer function, it doesn't seem to keep the `Dataset` hierarchy. I'm still unclear on why `func.__qual_name__` isn't handled correctly in multiprocessing. But replacing `__qualname__` by `__name__` fixes the issue.
## What was done
~We try to prevent the usage of multiprocessing when loading a dataset. Instead we load all cached shards sequentially.~
I couldn't find a nice way to obtain the cached_file_name and check they all exist before deciding to use the multiprocessing flow or not. Instead I expose an optional boolean `sequential` in `map` method.
## TODO
- [x] Check that the multiprocessed version and the sequential version output the same output
- [x] Check that sequential can load multiprocessed
- [x] Check that multiprocessed can load sequential
## Test
```python
from datasets import load_dataset
from multiprocessing import Pool
import random
def process(batch, rng):
length = len(batch["text"])
return {**batch, "processed_text": [f"PROCESSED {rng.random()}" for _ in range(length)]}
dataset = load_dataset("stas/openwebtext-10k", split="train")
print(dataset.column_names)
print(type(dataset))
rng = random.Random(42)
dataset1 = dataset.map(process, batched=True, batch_size=50, num_proc=4, fn_kwargs={"rng": rng})
# This one should be loaded from cache
rng = random.Random(42)
dataset2 = dataset.map(process, batched=True, batch_size=50, num_proc=4, fn_kwargs={"rng": rng}, sequential=True)
# Just to check that the random generator was correct
print(dataset1[-1]["processed_text"])
print(dataset2[-1]["processed_text"])
```
## Other solutions
I chose to load everything sequentially, but we can probably find a way to load shards in parallel using another number of workers (essentially this would be an argument not used for fingerprinting, allowing to allow `m` shards using `n` processes, which would be very useful when same dataset have to be loaded on two different setup, and we still want to leverage cache).
Also we can use a env variable similarly to `TOKENIZERS_PARALLELISM` as this seems generally setup related (though this changes slightly if we use multiprocessing).
cc @lhoestq (since I had asked you previously on `num_proc` being used for fingerprinting). Don't know if this is acceptable.
Yes that makes total sense, I tried to initially do that, except the way fingerprint is handled doesn't allow to easily manipulate such a field. Typically the fingerprinting is handled in `@fingerprint_transform` which has a bunch of params that aren't quite easy to extract. Those params are used to manipulate args, kwargs in fancy ways in order to finally obtain a dictionary used for fingerprint. I could duplicate everything, but this look like a very risky thing to do. I'll take a look if I can make something work with `inspect` if I can make a very simple wrapper.
A much more simpler solution I think is adding an optional `shard: Optional[int] = None` parameter. If None, we use the number of proc as the number of shards, otherwise we pass down the expected number of shards and use either sequential/multiprocessing (with arbitrary number of workers) to load the shards? This would allow the weird case where one wants a large number of shards with a limited amount of processes. Not the smartest thing to do, but it's not an absurd behaviour. Would this be acceptable? |
https://github.com/huggingface/datasets/pull/2774 | Prevent .map from using multiprocessing when loading from cache | The CI error is unrelated to the changes of this PR - it looks like an SSL issue with conda | ## Context
On our setup, we use different setup to train vs proprocessing datasets. Usually we are able to obtain a high number of cpus to preprocess, which allows us to use `num_proc` however we can't use as many during training phase. Currently if we use `num_proc={whatever the preprocessing value was}` we load from cache, but we get:
```
Traceback (most recent call last):
File "lib/python3.8/site-packages/multiprocess/pool.py", line 131, in worker
put((job, i, result))
File "lib/python3.8/site-packages/multiprocess/queues.py", line 371, in put
self._writer.send_bytes(obj)
File "lib/python3.8/site-packages/multiprocess/connection.py", line 203, in send_bytes
self._send_bytes(m[offset:offset + size])
File "lib/python3.8/site-packages/multiprocess/connection.py", line 414, in _send_bytes
self._send(header + buf)
File "lib/python3.8/site-packages/multiprocess/connection.py", line 371, in _send
n = write(self._handle, buf)
BrokenPipeError: [Errno 32] Broken pipe
```
Our current guess, is that we're spawning too many processes compared to the number of cpus available, and it's running OOM. Also we're loading this in DDP setting which means that for each gpu, I need to spawn a high number of processes to match the preprocessing fingerprint.
Instead what we suggest:
- Allow loading shard sequentially, sharing the same fingerprint as the multiprocessed one, in order to leverage multiprocessing when we actually generate the cache, and remove it when loading from cache.
## Current issues
~I'm having a hard time making fingerprints match. For some reason, the multiprocessing and the sequential version generate two different hash.~
**EDIT**: Turns out multiprocessing and sequential have different `transform` value for fingerprinting (check `fingerprint_transform`) when running `_map_single`:
- sequential : `datasets.arrow_dataset.Dataset._map_single`
- multiprocessing: `datasets.arrow_dataset._map_single`
This discrepancy is caused by multiprocessing pickling the transformer function, it doesn't seem to keep the `Dataset` hierarchy. I'm still unclear on why `func.__qual_name__` isn't handled correctly in multiprocessing. But replacing `__qualname__` by `__name__` fixes the issue.
## What was done
~We try to prevent the usage of multiprocessing when loading a dataset. Instead we load all cached shards sequentially.~
I couldn't find a nice way to obtain the cached_file_name and check they all exist before deciding to use the multiprocessing flow or not. Instead I expose an optional boolean `sequential` in `map` method.
## TODO
- [x] Check that the multiprocessed version and the sequential version output the same output
- [x] Check that sequential can load multiprocessed
- [x] Check that multiprocessed can load sequential
## Test
```python
from datasets import load_dataset
from multiprocessing import Pool
import random
def process(batch, rng):
length = len(batch["text"])
return {**batch, "processed_text": [f"PROCESSED {rng.random()}" for _ in range(length)]}
dataset = load_dataset("stas/openwebtext-10k", split="train")
print(dataset.column_names)
print(type(dataset))
rng = random.Random(42)
dataset1 = dataset.map(process, batched=True, batch_size=50, num_proc=4, fn_kwargs={"rng": rng})
# This one should be loaded from cache
rng = random.Random(42)
dataset2 = dataset.map(process, batched=True, batch_size=50, num_proc=4, fn_kwargs={"rng": rng}, sequential=True)
# Just to check that the random generator was correct
print(dataset1[-1]["processed_text"])
print(dataset2[-1]["processed_text"])
```
## Other solutions
I chose to load everything sequentially, but we can probably find a way to load shards in parallel using another number of workers (essentially this would be an argument not used for fingerprinting, allowing to allow `m` shards using `n` processes, which would be very useful when same dataset have to be loaded on two different setup, and we still want to leverage cache).
Also we can use a env variable similarly to `TOKENIZERS_PARALLELISM` as this seems generally setup related (though this changes slightly if we use multiprocessing).
cc @lhoestq (since I had asked you previously on `num_proc` being used for fingerprinting). Don't know if this is acceptable. | 20 | text: Prevent .map from using multiprocessing when loading from cache
## Context
On our setup, we use different setup to train vs proprocessing datasets. Usually we are able to obtain a high number of cpus to preprocess, which allows us to use `num_proc` however we can't use as many during training phase. Currently if we use `num_proc={whatever the preprocessing value was}` we load from cache, but we get:
```
Traceback (most recent call last):
File "lib/python3.8/site-packages/multiprocess/pool.py", line 131, in worker
put((job, i, result))
File "lib/python3.8/site-packages/multiprocess/queues.py", line 371, in put
self._writer.send_bytes(obj)
File "lib/python3.8/site-packages/multiprocess/connection.py", line 203, in send_bytes
self._send_bytes(m[offset:offset + size])
File "lib/python3.8/site-packages/multiprocess/connection.py", line 414, in _send_bytes
self._send(header + buf)
File "lib/python3.8/site-packages/multiprocess/connection.py", line 371, in _send
n = write(self._handle, buf)
BrokenPipeError: [Errno 32] Broken pipe
```
Our current guess, is that we're spawning too many processes compared to the number of cpus available, and it's running OOM. Also we're loading this in DDP setting which means that for each gpu, I need to spawn a high number of processes to match the preprocessing fingerprint.
Instead what we suggest:
- Allow loading shard sequentially, sharing the same fingerprint as the multiprocessed one, in order to leverage multiprocessing when we actually generate the cache, and remove it when loading from cache.
## Current issues
~I'm having a hard time making fingerprints match. For some reason, the multiprocessing and the sequential version generate two different hash.~
**EDIT**: Turns out multiprocessing and sequential have different `transform` value for fingerprinting (check `fingerprint_transform`) when running `_map_single`:
- sequential : `datasets.arrow_dataset.Dataset._map_single`
- multiprocessing: `datasets.arrow_dataset._map_single`
This discrepancy is caused by multiprocessing pickling the transformer function, it doesn't seem to keep the `Dataset` hierarchy. I'm still unclear on why `func.__qual_name__` isn't handled correctly in multiprocessing. But replacing `__qualname__` by `__name__` fixes the issue.
## What was done
~We try to prevent the usage of multiprocessing when loading a dataset. Instead we load all cached shards sequentially.~
I couldn't find a nice way to obtain the cached_file_name and check they all exist before deciding to use the multiprocessing flow or not. Instead I expose an optional boolean `sequential` in `map` method.
## TODO
- [x] Check that the multiprocessed version and the sequential version output the same output
- [x] Check that sequential can load multiprocessed
- [x] Check that multiprocessed can load sequential
## Test
```python
from datasets import load_dataset
from multiprocessing import Pool
import random
def process(batch, rng):
length = len(batch["text"])
return {**batch, "processed_text": [f"PROCESSED {rng.random()}" for _ in range(length)]}
dataset = load_dataset("stas/openwebtext-10k", split="train")
print(dataset.column_names)
print(type(dataset))
rng = random.Random(42)
dataset1 = dataset.map(process, batched=True, batch_size=50, num_proc=4, fn_kwargs={"rng": rng})
# This one should be loaded from cache
rng = random.Random(42)
dataset2 = dataset.map(process, batched=True, batch_size=50, num_proc=4, fn_kwargs={"rng": rng}, sequential=True)
# Just to check that the random generator was correct
print(dataset1[-1]["processed_text"])
print(dataset2[-1]["processed_text"])
```
## Other solutions
I chose to load everything sequentially, but we can probably find a way to load shards in parallel using another number of workers (essentially this would be an argument not used for fingerprinting, allowing to allow `m` shards using `n` processes, which would be very useful when same dataset have to be loaded on two different setup, and we still want to leverage cache).
Also we can use a env variable similarly to `TOKENIZERS_PARALLELISM` as this seems generally setup related (though this changes slightly if we use multiprocessing).
cc @lhoestq (since I had asked you previously on `num_proc` being used for fingerprinting). Don't know if this is acceptable.
The CI error is unrelated to the changes of this PR - it looks like an SSL issue with conda |
https://github.com/huggingface/datasets/pull/2771 | [WIP][Common Voice 7] Add common voice 7.0 | Hi ! I think the name `common_voice_7` is fine :)
Moreover if the dataset_infos.json is missing I'm pretty sure you don't need to specify `ignore_verifications=True` | This PR allows to load the new common voice dataset manually as explained when doing:
```python
from datasets import load_dataset
ds = load_dataset("./datasets/datasets/common_voice_7", "ab")
```
=>
```
Please follow the manual download instructions:
You need to manually the dataset from `https://commonvoice.mozilla.org/en/datasets`.
Make sure you choose the version `Common Voice Corpus 7.0`.
Choose a language of your choice and find the corresponding language-id, *e.g.*, `Abkhaz` with language-id `ab`. The following language-ids are available:
['ab', 'ar', 'as', 'az', 'ba', 'bas', 'be', 'bg', 'br', 'ca', 'cnh', 'cs', 'cv', 'cy', 'de', 'dv', 'el', 'en', 'eo', 'es', 'et', 'eu', 'fa', 'fi', 'fr', 'fy-NL', 'ga-IE', 'gl', 'gn', 'ha', 'hi', 'hsb', 'hu', 'hy-AM', 'ia', 'id', 'it', 'ja', 'ka', 'kab', 'kk', 'kmr', 'ky', 'lg', 'lt', 'lv', 'mn', 'mt', 'nl', 'or', 'pa-IN', 'pl', 'pt', 'rm-sursilv', 'rm-vallader', 'ro', 'ru', 'rw', 'sah', 'sk', 'sl', 'sr', 'sv-SE', 'ta', 'th', 'tr', 'tt', 'ug', 'uk', 'ur', 'uz', 'vi', 'vot', 'zh-CN', 'zh-HK', 'zh-TW']
Next, you will have to enter your email address to download the dataset in the `tar.gz` format. Save the file under <path-to-file>.
The file should then be extracted with: ``tar -xvzf <path-to-file>`` which will extract a folder called ``cv-corpus-7.0-2021-07-21``.
The dataset can then be loaded with `datasets.load_dataset("common_voice", <language-id>, data_dir="<path-to-'cv-corpus-7.0-2021-07-21'-folder>", ignore_verifications=True).
```
Having followed those instructions one can then download the data as follows:
```python
from datasets import load_dataset
ds = load_dataset("./datasets/datasets/common_voice_7", "ab", data_dir="./cv-corpus-7.0-2021-07-21/", ignore_verifications=True)
```
## TODO
- [ ] Discuss naming. Is the name ok here "common_voice_7"? The dataset script differs only really in one point from `common_voice.py` in that all the metadata is different (more hours etc...) and that it has to use manual data dir for now
- [ ] Ideally we should get a bundled download link. For `common_voice.py` there is a bundled download link: `https://voice-prod-bundler-ee1969a6ce8178826482b88e843c335139bd3fb4.s3.amazonaws.com/cv-corpus-6.1-2020-12-11/{}.tar.gz` that allows one to directly download the data. However such a link is missing for Common Voice 7. I guess we should try to contact common voice about it and ask whether we could host the data or help otherwise somehow. See: https://github.com/common-voice/common-voice-bundler/issues/15 cc @yjernite
- [ ] I did not compute the dataset.json and it would mean that I'd have to download 76 datasets totalling around 1TB manually before running the checksum command. This just takes too much time. For now the user will have to add a `ignore_verifications=True` to download the data. This step would also be much easier if we could get a bundled link
- [ ] Add dummy data | 25 | text: [WIP][Common Voice 7] Add common voice 7.0
This PR allows to load the new common voice dataset manually as explained when doing:
```python
from datasets import load_dataset
ds = load_dataset("./datasets/datasets/common_voice_7", "ab")
```
=>
```
Please follow the manual download instructions:
You need to manually the dataset from `https://commonvoice.mozilla.org/en/datasets`.
Make sure you choose the version `Common Voice Corpus 7.0`.
Choose a language of your choice and find the corresponding language-id, *e.g.*, `Abkhaz` with language-id `ab`. The following language-ids are available:
['ab', 'ar', 'as', 'az', 'ba', 'bas', 'be', 'bg', 'br', 'ca', 'cnh', 'cs', 'cv', 'cy', 'de', 'dv', 'el', 'en', 'eo', 'es', 'et', 'eu', 'fa', 'fi', 'fr', 'fy-NL', 'ga-IE', 'gl', 'gn', 'ha', 'hi', 'hsb', 'hu', 'hy-AM', 'ia', 'id', 'it', 'ja', 'ka', 'kab', 'kk', 'kmr', 'ky', 'lg', 'lt', 'lv', 'mn', 'mt', 'nl', 'or', 'pa-IN', 'pl', 'pt', 'rm-sursilv', 'rm-vallader', 'ro', 'ru', 'rw', 'sah', 'sk', 'sl', 'sr', 'sv-SE', 'ta', 'th', 'tr', 'tt', 'ug', 'uk', 'ur', 'uz', 'vi', 'vot', 'zh-CN', 'zh-HK', 'zh-TW']
Next, you will have to enter your email address to download the dataset in the `tar.gz` format. Save the file under <path-to-file>.
The file should then be extracted with: ``tar -xvzf <path-to-file>`` which will extract a folder called ``cv-corpus-7.0-2021-07-21``.
The dataset can then be loaded with `datasets.load_dataset("common_voice", <language-id>, data_dir="<path-to-'cv-corpus-7.0-2021-07-21'-folder>", ignore_verifications=True).
```
Having followed those instructions one can then download the data as follows:
```python
from datasets import load_dataset
ds = load_dataset("./datasets/datasets/common_voice_7", "ab", data_dir="./cv-corpus-7.0-2021-07-21/", ignore_verifications=True)
```
## TODO
- [ ] Discuss naming. Is the name ok here "common_voice_7"? The dataset script differs only really in one point from `common_voice.py` in that all the metadata is different (more hours etc...) and that it has to use manual data dir for now
- [ ] Ideally we should get a bundled download link. For `common_voice.py` there is a bundled download link: `https://voice-prod-bundler-ee1969a6ce8178826482b88e843c335139bd3fb4.s3.amazonaws.com/cv-corpus-6.1-2020-12-11/{}.tar.gz` that allows one to directly download the data. However such a link is missing for Common Voice 7. I guess we should try to contact common voice about it and ask whether we could host the data or help otherwise somehow. See: https://github.com/common-voice/common-voice-bundler/issues/15 cc @yjernite
- [ ] I did not compute the dataset.json and it would mean that I'd have to download 76 datasets totalling around 1TB manually before running the checksum command. This just takes too much time. For now the user will have to add a `ignore_verifications=True` to download the data. This step would also be much easier if we could get a bundled link
- [ ] Add dummy data
Hi ! I think the name `common_voice_7` is fine :)
Moreover if the dataset_infos.json is missing I'm pretty sure you don't need to specify `ignore_verifications=True` |
https://github.com/huggingface/datasets/pull/2747 | add multi-proc in `to_json` | Thank you for working on this, @bhavitvyamalik
10% is not solving the issue, we want 5-10x faster on a machine that has lots of resources, but limited processing time.
So let's benchmark it on an instance with many more cores, I can test with 12 on my dev box and 40 on JZ.
Could you please share the test I could run with both versions?
Should we also test the sharded version I shared in https://github.com/huggingface/datasets/issues/2663#issue-946552273 so optionally 3 versions to test. | Closes #2663. I've tried adding multiprocessing in `to_json`. Here's some benchmarking I did to compare the timings of current version (say v1) and multi-proc version (say v2). I did this with `cpu_count` 4 (2015 Macbook Air)
1. Dataset name: `ascent_kb` - 8.9M samples (all samples were used, reporting this for a single run)
v1- ~225 seconds for converting whole dataset to json
v2- ~200 seconds for converting whole dataset to json
2. Dataset name: `lama` - 1.3M samples (all samples were used, reporting this for 2 runs)
v1- ~26 seconds for converting whole dataset to json
v2- ~23.6 seconds for converting whole dataset to json
I think it's safe to say that v2 is 10% faster as compared to v1. Timings may improve further with better configuration.
The only bottleneck I feel is writing to file from the output list. If we can improve that aspect then timings may improve further.
Let me know if any changes/improvements can be done in this @stas00, @lhoestq, @albertvillanova. @lhoestq even suggested to extend this work with other export methods as well like `csv` or `parquet`. | 82 | text: add multi-proc in `to_json`
Closes #2663. I've tried adding multiprocessing in `to_json`. Here's some benchmarking I did to compare the timings of current version (say v1) and multi-proc version (say v2). I did this with `cpu_count` 4 (2015 Macbook Air)
1. Dataset name: `ascent_kb` - 8.9M samples (all samples were used, reporting this for a single run)
v1- ~225 seconds for converting whole dataset to json
v2- ~200 seconds for converting whole dataset to json
2. Dataset name: `lama` - 1.3M samples (all samples were used, reporting this for 2 runs)
v1- ~26 seconds for converting whole dataset to json
v2- ~23.6 seconds for converting whole dataset to json
I think it's safe to say that v2 is 10% faster as compared to v1. Timings may improve further with better configuration.
The only bottleneck I feel is writing to file from the output list. If we can improve that aspect then timings may improve further.
Let me know if any changes/improvements can be done in this @stas00, @lhoestq, @albertvillanova. @lhoestq even suggested to extend this work with other export methods as well like `csv` or `parquet`.
Thank you for working on this, @bhavitvyamalik
10% is not solving the issue, we want 5-10x faster on a machine that has lots of resources, but limited processing time.
So let's benchmark it on an instance with many more cores, I can test with 12 on my dev box and 40 on JZ.
Could you please share the test I could run with both versions?
Should we also test the sharded version I shared in https://github.com/huggingface/datasets/issues/2663#issue-946552273 so optionally 3 versions to test. |
https://github.com/huggingface/datasets/pull/2747 | add multi-proc in `to_json` | Since I was facing `OSError: [Errno 12] Cannot allocate memory` in CircleCI tests, I've added `num_proc` option instead of always using full `cpu_count`. You can test both v1 and v2 through this branch (some redundancy needs to be removed).
Update: I was able to convert into json which took 50% less time as compared to v1 on `ascent_kb` dataset. Will post the benchmarking script with results here. | Closes #2663. I've tried adding multiprocessing in `to_json`. Here's some benchmarking I did to compare the timings of current version (say v1) and multi-proc version (say v2). I did this with `cpu_count` 4 (2015 Macbook Air)
1. Dataset name: `ascent_kb` - 8.9M samples (all samples were used, reporting this for a single run)
v1- ~225 seconds for converting whole dataset to json
v2- ~200 seconds for converting whole dataset to json
2. Dataset name: `lama` - 1.3M samples (all samples were used, reporting this for 2 runs)
v1- ~26 seconds for converting whole dataset to json
v2- ~23.6 seconds for converting whole dataset to json
I think it's safe to say that v2 is 10% faster as compared to v1. Timings may improve further with better configuration.
The only bottleneck I feel is writing to file from the output list. If we can improve that aspect then timings may improve further.
Let me know if any changes/improvements can be done in this @stas00, @lhoestq, @albertvillanova. @lhoestq even suggested to extend this work with other export methods as well like `csv` or `parquet`. | 67 | text: add multi-proc in `to_json`
Closes #2663. I've tried adding multiprocessing in `to_json`. Here's some benchmarking I did to compare the timings of current version (say v1) and multi-proc version (say v2). I did this with `cpu_count` 4 (2015 Macbook Air)
1. Dataset name: `ascent_kb` - 8.9M samples (all samples were used, reporting this for a single run)
v1- ~225 seconds for converting whole dataset to json
v2- ~200 seconds for converting whole dataset to json
2. Dataset name: `lama` - 1.3M samples (all samples were used, reporting this for 2 runs)
v1- ~26 seconds for converting whole dataset to json
v2- ~23.6 seconds for converting whole dataset to json
I think it's safe to say that v2 is 10% faster as compared to v1. Timings may improve further with better configuration.
The only bottleneck I feel is writing to file from the output list. If we can improve that aspect then timings may improve further.
Let me know if any changes/improvements can be done in this @stas00, @lhoestq, @albertvillanova. @lhoestq even suggested to extend this work with other export methods as well like `csv` or `parquet`.
Since I was facing `OSError: [Errno 12] Cannot allocate memory` in CircleCI tests, I've added `num_proc` option instead of always using full `cpu_count`. You can test both v1 and v2 through this branch (some redundancy needs to be removed).
Update: I was able to convert into json which took 50% less time as compared to v1 on `ascent_kb` dataset. Will post the benchmarking script with results here. |
https://github.com/huggingface/datasets/pull/2747 | add multi-proc in `to_json` | Here are the benchmarks with the current branch for both v1 and v2 (dataset: `ascent_kb`, 8.9M samples):
| batch_size | time (in sec) | time (in sec) |
|------------|---------------|---------------|
| | num_proc = 1 | num_proc = 4 |
| 10k | 185.56 | 170.11 |
| 50k | 175.79 | 86.84 |
| **100k** | 191.09 | **78.35** |
| 125k | 198.28 | 90.89 |
Increasing the batch size on my machine helped in making v2 around 50% faster as compared to v1. Timings may vary depending on the machine. I'm including the benchmarking script as well. CircleCI errors are unrelated (something related to `bertscore`)
```
import time
from datasets import load_dataset
import pathlib
import os
from pathlib import Path
import shutil
import gc
batch_sizes = [10_000, 50_000, 100_000, 125_000]
num_procs = [1, 4] # change this according to your machine
SAVE_LOC = "./new_dataset.json"
for batch in batch_sizes:
for num in num_procs:
dataset = load_dataset("ascent_kb")
local_start = time.time()
ans = dataset['train'].to_json(SAVE_LOC, batch_size=batch, num_proc=num)
local_end = time.time() - local_start
print(f"Time taken on {num} num_proc and {batch} batch_size: ", local_end)
# remove that dataset and its contents from cache and newly generated json
new_json = pathlib.Path(SAVE_LOC)
new_json.unlink()
try:
shutil.rmtree(os.path.join(str(Path.home()), ".cache", "huggingface"))
except OSError as e:
print("Error: %s - %s." % (e.filename, e.strerror))
gc.collect()
```
This will download the dataset in every iteration and run `to_json`. I didn't do multiple iterations here for `to_json` (for a specific batch_size and num_proc) and took average time as I found that v1 got faster after 1st iteration (maybe it's caching somewhere). Since you'll be doing this operation only once, I thought it'll be better to report how both v1 and v2 performed in single iteration only.
Important: Benchmarking script will delete the newly generated json and `~/.cache/huggingface/` after every iteration so that it doesn't end up using any cached data (just to be on a safe side) | Closes #2663. I've tried adding multiprocessing in `to_json`. Here's some benchmarking I did to compare the timings of current version (say v1) and multi-proc version (say v2). I did this with `cpu_count` 4 (2015 Macbook Air)
1. Dataset name: `ascent_kb` - 8.9M samples (all samples were used, reporting this for a single run)
v1- ~225 seconds for converting whole dataset to json
v2- ~200 seconds for converting whole dataset to json
2. Dataset name: `lama` - 1.3M samples (all samples were used, reporting this for 2 runs)
v1- ~26 seconds for converting whole dataset to json
v2- ~23.6 seconds for converting whole dataset to json
I think it's safe to say that v2 is 10% faster as compared to v1. Timings may improve further with better configuration.
The only bottleneck I feel is writing to file from the output list. If we can improve that aspect then timings may improve further.
Let me know if any changes/improvements can be done in this @stas00, @lhoestq, @albertvillanova. @lhoestq even suggested to extend this work with other export methods as well like `csv` or `parquet`. | 313 | text: add multi-proc in `to_json`
Closes #2663. I've tried adding multiprocessing in `to_json`. Here's some benchmarking I did to compare the timings of current version (say v1) and multi-proc version (say v2). I did this with `cpu_count` 4 (2015 Macbook Air)
1. Dataset name: `ascent_kb` - 8.9M samples (all samples were used, reporting this for a single run)
v1- ~225 seconds for converting whole dataset to json
v2- ~200 seconds for converting whole dataset to json
2. Dataset name: `lama` - 1.3M samples (all samples were used, reporting this for 2 runs)
v1- ~26 seconds for converting whole dataset to json
v2- ~23.6 seconds for converting whole dataset to json
I think it's safe to say that v2 is 10% faster as compared to v1. Timings may improve further with better configuration.
The only bottleneck I feel is writing to file from the output list. If we can improve that aspect then timings may improve further.
Let me know if any changes/improvements can be done in this @stas00, @lhoestq, @albertvillanova. @lhoestq even suggested to extend this work with other export methods as well like `csv` or `parquet`.
Here are the benchmarks with the current branch for both v1 and v2 (dataset: `ascent_kb`, 8.9M samples):
| batch_size | time (in sec) | time (in sec) |
|------------|---------------|---------------|
| | num_proc = 1 | num_proc = 4 |
| 10k | 185.56 | 170.11 |
| 50k | 175.79 | 86.84 |
| **100k** | 191.09 | **78.35** |
| 125k | 198.28 | 90.89 |
Increasing the batch size on my machine helped in making v2 around 50% faster as compared to v1. Timings may vary depending on the machine. I'm including the benchmarking script as well. CircleCI errors are unrelated (something related to `bertscore`)
```
import time
from datasets import load_dataset
import pathlib
import os
from pathlib import Path
import shutil
import gc
batch_sizes = [10_000, 50_000, 100_000, 125_000]
num_procs = [1, 4] # change this according to your machine
SAVE_LOC = "./new_dataset.json"
for batch in batch_sizes:
for num in num_procs:
dataset = load_dataset("ascent_kb")
local_start = time.time()
ans = dataset['train'].to_json(SAVE_LOC, batch_size=batch, num_proc=num)
local_end = time.time() - local_start
print(f"Time taken on {num} num_proc and {batch} batch_size: ", local_end)
# remove that dataset and its contents from cache and newly generated json
new_json = pathlib.Path(SAVE_LOC)
new_json.unlink()
try:
shutil.rmtree(os.path.join(str(Path.home()), ".cache", "huggingface"))
except OSError as e:
print("Error: %s - %s." % (e.filename, e.strerror))
gc.collect()
```
This will download the dataset in every iteration and run `to_json`. I didn't do multiple iterations here for `to_json` (for a specific batch_size and num_proc) and took average time as I found that v1 got faster after 1st iteration (maybe it's caching somewhere). Since you'll be doing this operation only once, I thought it'll be better to report how both v1 and v2 performed in single iteration only.
Important: Benchmarking script will delete the newly generated json and `~/.cache/huggingface/` after every iteration so that it doesn't end up using any cached data (just to be on a safe side) |
https://github.com/huggingface/datasets/pull/2747 | add multi-proc in `to_json` | Thank you for sharing the benchmark, @bhavitvyamalik. Your results look promising.
But if I remember correctly the sharded version at https://github.com/huggingface/datasets/issues/2663#issue-946552273 was much faster. So we probably should compare to it as well? And if it's faster than at least document that manual sharding version?
-------
That's a dangerous benchmark as it'd wipe out many other HF things. Why not wipe out:
```
~/.cache/huggingface/datasets/ascent_kb/
```
Running the benchmark now. | Closes #2663. I've tried adding multiprocessing in `to_json`. Here's some benchmarking I did to compare the timings of current version (say v1) and multi-proc version (say v2). I did this with `cpu_count` 4 (2015 Macbook Air)
1. Dataset name: `ascent_kb` - 8.9M samples (all samples were used, reporting this for a single run)
v1- ~225 seconds for converting whole dataset to json
v2- ~200 seconds for converting whole dataset to json
2. Dataset name: `lama` - 1.3M samples (all samples were used, reporting this for 2 runs)
v1- ~26 seconds for converting whole dataset to json
v2- ~23.6 seconds for converting whole dataset to json
I think it's safe to say that v2 is 10% faster as compared to v1. Timings may improve further with better configuration.
The only bottleneck I feel is writing to file from the output list. If we can improve that aspect then timings may improve further.
Let me know if any changes/improvements can be done in this @stas00, @lhoestq, @albertvillanova. @lhoestq even suggested to extend this work with other export methods as well like `csv` or `parquet`. | 69 | text: add multi-proc in `to_json`
Closes #2663. I've tried adding multiprocessing in `to_json`. Here's some benchmarking I did to compare the timings of current version (say v1) and multi-proc version (say v2). I did this with `cpu_count` 4 (2015 Macbook Air)
1. Dataset name: `ascent_kb` - 8.9M samples (all samples were used, reporting this for a single run)
v1- ~225 seconds for converting whole dataset to json
v2- ~200 seconds for converting whole dataset to json
2. Dataset name: `lama` - 1.3M samples (all samples were used, reporting this for 2 runs)
v1- ~26 seconds for converting whole dataset to json
v2- ~23.6 seconds for converting whole dataset to json
I think it's safe to say that v2 is 10% faster as compared to v1. Timings may improve further with better configuration.
The only bottleneck I feel is writing to file from the output list. If we can improve that aspect then timings may improve further.
Let me know if any changes/improvements can be done in this @stas00, @lhoestq, @albertvillanova. @lhoestq even suggested to extend this work with other export methods as well like `csv` or `parquet`.
Thank you for sharing the benchmark, @bhavitvyamalik. Your results look promising.
But if I remember correctly the sharded version at https://github.com/huggingface/datasets/issues/2663#issue-946552273 was much faster. So we probably should compare to it as well? And if it's faster than at least document that manual sharding version?
-------
That's a dangerous benchmark as it'd wipe out many other HF things. Why not wipe out:
```
~/.cache/huggingface/datasets/ascent_kb/
```
Running the benchmark now. |
https://github.com/huggingface/datasets/pull/2747 | add multi-proc in `to_json` | Weird, I tried to adapt your benchmark to using shards and the program no longer works. It instead quickly uses up all available RAM and hangs. Has something changed recently in `datasets`? You can try:
```
import time
from datasets import load_dataset
import pathlib
import os
from pathlib import Path
import shutil
import gc
from multiprocessing import cpu_count, Process, Queue
batch_sizes = [10_000, 50_000, 100_000, 125_000]
num_procs = [1, 8] # change this according to your machine
DATASET_NAME = ("ascent_kb")
num_shards = [1, 8]
for batch in batch_sizes:
for shards in num_shards:
dataset = load_dataset(DATASET_NAME)["train"]
#print(dataset)
def process_shard(idx):
print(f"Sharding {idx}")
ds_shard = dataset.shard(shards, idx, contiguous=True)
# ds_shard = ds_shard.shuffle() # remove contiguous=True above if shuffling
print(f"Saving {DATASET_NAME}-{idx}.jsonl")
ds_shard.to_json(f"{DATASET_NAME}-{idx}.jsonl", orient="records", lines=True, force_ascii=False)
local_start = time.time()
queue = Queue()
processes = [Process(target=process_shard, args=(idx,)) for idx in range(shards)]
for p in processes:
p.start()
for p in processes:
p.join()
local_end = time.time() - local_start
print(f"Time taken on {shards} shards and {batch} batch_size: ", local_end)
```
Just careful, so that it won't crash your compute environment. As it almost crashed mine. | Closes #2663. I've tried adding multiprocessing in `to_json`. Here's some benchmarking I did to compare the timings of current version (say v1) and multi-proc version (say v2). I did this with `cpu_count` 4 (2015 Macbook Air)
1. Dataset name: `ascent_kb` - 8.9M samples (all samples were used, reporting this for a single run)
v1- ~225 seconds for converting whole dataset to json
v2- ~200 seconds for converting whole dataset to json
2. Dataset name: `lama` - 1.3M samples (all samples were used, reporting this for 2 runs)
v1- ~26 seconds for converting whole dataset to json
v2- ~23.6 seconds for converting whole dataset to json
I think it's safe to say that v2 is 10% faster as compared to v1. Timings may improve further with better configuration.
The only bottleneck I feel is writing to file from the output list. If we can improve that aspect then timings may improve further.
Let me know if any changes/improvements can be done in this @stas00, @lhoestq, @albertvillanova. @lhoestq even suggested to extend this work with other export methods as well like `csv` or `parquet`. | 176 | text: add multi-proc in `to_json`
Closes #2663. I've tried adding multiprocessing in `to_json`. Here's some benchmarking I did to compare the timings of current version (say v1) and multi-proc version (say v2). I did this with `cpu_count` 4 (2015 Macbook Air)
1. Dataset name: `ascent_kb` - 8.9M samples (all samples were used, reporting this for a single run)
v1- ~225 seconds for converting whole dataset to json
v2- ~200 seconds for converting whole dataset to json
2. Dataset name: `lama` - 1.3M samples (all samples were used, reporting this for 2 runs)
v1- ~26 seconds for converting whole dataset to json
v2- ~23.6 seconds for converting whole dataset to json
I think it's safe to say that v2 is 10% faster as compared to v1. Timings may improve further with better configuration.
The only bottleneck I feel is writing to file from the output list. If we can improve that aspect then timings may improve further.
Let me know if any changes/improvements can be done in this @stas00, @lhoestq, @albertvillanova. @lhoestq even suggested to extend this work with other export methods as well like `csv` or `parquet`.
Weird, I tried to adapt your benchmark to using shards and the program no longer works. It instead quickly uses up all available RAM and hangs. Has something changed recently in `datasets`? You can try:
```
import time
from datasets import load_dataset
import pathlib
import os
from pathlib import Path
import shutil
import gc
from multiprocessing import cpu_count, Process, Queue
batch_sizes = [10_000, 50_000, 100_000, 125_000]
num_procs = [1, 8] # change this according to your machine
DATASET_NAME = ("ascent_kb")
num_shards = [1, 8]
for batch in batch_sizes:
for shards in num_shards:
dataset = load_dataset(DATASET_NAME)["train"]
#print(dataset)
def process_shard(idx):
print(f"Sharding {idx}")
ds_shard = dataset.shard(shards, idx, contiguous=True)
# ds_shard = ds_shard.shuffle() # remove contiguous=True above if shuffling
print(f"Saving {DATASET_NAME}-{idx}.jsonl")
ds_shard.to_json(f"{DATASET_NAME}-{idx}.jsonl", orient="records", lines=True, force_ascii=False)
local_start = time.time()
queue = Queue()
processes = [Process(target=process_shard, args=(idx,)) for idx in range(shards)]
for p in processes:
p.start()
for p in processes:
p.join()
local_end = time.time() - local_start
print(f"Time taken on {shards} shards and {batch} batch_size: ", local_end)
```
Just careful, so that it won't crash your compute environment. As it almost crashed mine. |
https://github.com/huggingface/datasets/pull/2747 | add multi-proc in `to_json` | So this part seems to no longer work:
```
dataset = load_dataset("ascent_kb")["train"]
ds_shard = dataset.shard(1, 0, contiguous=True)
ds_shard.to_json("ascent_kb-0.jsonl", orient="records", lines=True, force_ascii=False)
``` | Closes #2663. I've tried adding multiprocessing in `to_json`. Here's some benchmarking I did to compare the timings of current version (say v1) and multi-proc version (say v2). I did this with `cpu_count` 4 (2015 Macbook Air)
1. Dataset name: `ascent_kb` - 8.9M samples (all samples were used, reporting this for a single run)
v1- ~225 seconds for converting whole dataset to json
v2- ~200 seconds for converting whole dataset to json
2. Dataset name: `lama` - 1.3M samples (all samples were used, reporting this for 2 runs)
v1- ~26 seconds for converting whole dataset to json
v2- ~23.6 seconds for converting whole dataset to json
I think it's safe to say that v2 is 10% faster as compared to v1. Timings may improve further with better configuration.
The only bottleneck I feel is writing to file from the output list. If we can improve that aspect then timings may improve further.
Let me know if any changes/improvements can be done in this @stas00, @lhoestq, @albertvillanova. @lhoestq even suggested to extend this work with other export methods as well like `csv` or `parquet`. | 22 | text: add multi-proc in `to_json`
Closes #2663. I've tried adding multiprocessing in `to_json`. Here's some benchmarking I did to compare the timings of current version (say v1) and multi-proc version (say v2). I did this with `cpu_count` 4 (2015 Macbook Air)
1. Dataset name: `ascent_kb` - 8.9M samples (all samples were used, reporting this for a single run)
v1- ~225 seconds for converting whole dataset to json
v2- ~200 seconds for converting whole dataset to json
2. Dataset name: `lama` - 1.3M samples (all samples were used, reporting this for 2 runs)
v1- ~26 seconds for converting whole dataset to json
v2- ~23.6 seconds for converting whole dataset to json
I think it's safe to say that v2 is 10% faster as compared to v1. Timings may improve further with better configuration.
The only bottleneck I feel is writing to file from the output list. If we can improve that aspect then timings may improve further.
Let me know if any changes/improvements can be done in this @stas00, @lhoestq, @albertvillanova. @lhoestq even suggested to extend this work with other export methods as well like `csv` or `parquet`.
So this part seems to no longer work:
```
dataset = load_dataset("ascent_kb")["train"]
ds_shard = dataset.shard(1, 0, contiguous=True)
ds_shard.to_json("ascent_kb-0.jsonl", orient="records", lines=True, force_ascii=False)
``` |
https://github.com/huggingface/datasets/pull/2747 | add multi-proc in `to_json` | If you are using `to_json` without any `num_proc`or `num_proc=1` then essentially it'll fall back to v1 only and I've kept it as it is (the tests were passing as well)
> That's a dangerous benchmark as it'd wipe out many other HF things. Why not wipe out:
That's because some dataset related files were still left inside `~/.cache/huggingface/datasets` folder. You can wipe off datasets folder inside your cache maybe
> dataset = load_dataset("ascent_kb")["train"]
> ds_shard = dataset.shard(1, 0, contiguous=True)
> ds_shard.to_json("ascent_kb-0.jsonl", orient="records", lines=True, force_ascii=False)
I tried this `lama` dataset (1.3M) and it worked fine. Trying it with `ascent_kb` currently, will update it here. | Closes #2663. I've tried adding multiprocessing in `to_json`. Here's some benchmarking I did to compare the timings of current version (say v1) and multi-proc version (say v2). I did this with `cpu_count` 4 (2015 Macbook Air)
1. Dataset name: `ascent_kb` - 8.9M samples (all samples were used, reporting this for a single run)
v1- ~225 seconds for converting whole dataset to json
v2- ~200 seconds for converting whole dataset to json
2. Dataset name: `lama` - 1.3M samples (all samples were used, reporting this for 2 runs)
v1- ~26 seconds for converting whole dataset to json
v2- ~23.6 seconds for converting whole dataset to json
I think it's safe to say that v2 is 10% faster as compared to v1. Timings may improve further with better configuration.
The only bottleneck I feel is writing to file from the output list. If we can improve that aspect then timings may improve further.
Let me know if any changes/improvements can be done in this @stas00, @lhoestq, @albertvillanova. @lhoestq even suggested to extend this work with other export methods as well like `csv` or `parquet`. | 103 | text: add multi-proc in `to_json`
Closes #2663. I've tried adding multiprocessing in `to_json`. Here's some benchmarking I did to compare the timings of current version (say v1) and multi-proc version (say v2). I did this with `cpu_count` 4 (2015 Macbook Air)
1. Dataset name: `ascent_kb` - 8.9M samples (all samples were used, reporting this for a single run)
v1- ~225 seconds for converting whole dataset to json
v2- ~200 seconds for converting whole dataset to json
2. Dataset name: `lama` - 1.3M samples (all samples were used, reporting this for 2 runs)
v1- ~26 seconds for converting whole dataset to json
v2- ~23.6 seconds for converting whole dataset to json
I think it's safe to say that v2 is 10% faster as compared to v1. Timings may improve further with better configuration.
The only bottleneck I feel is writing to file from the output list. If we can improve that aspect then timings may improve further.
Let me know if any changes/improvements can be done in this @stas00, @lhoestq, @albertvillanova. @lhoestq even suggested to extend this work with other export methods as well like `csv` or `parquet`.
If you are using `to_json` without any `num_proc`or `num_proc=1` then essentially it'll fall back to v1 only and I've kept it as it is (the tests were passing as well)
> That's a dangerous benchmark as it'd wipe out many other HF things. Why not wipe out:
That's because some dataset related files were still left inside `~/.cache/huggingface/datasets` folder. You can wipe off datasets folder inside your cache maybe
> dataset = load_dataset("ascent_kb")["train"]
> ds_shard = dataset.shard(1, 0, contiguous=True)
> ds_shard.to_json("ascent_kb-0.jsonl", orient="records", lines=True, force_ascii=False)
I tried this `lama` dataset (1.3M) and it worked fine. Trying it with `ascent_kb` currently, will update it here. |
https://github.com/huggingface/datasets/pull/2747 | add multi-proc in `to_json` | I don't think the issue has anything to do with your work, @bhavitvyamalik. I forgot to mention I tested to see the same problem with the latest datasets release.
Interesting, I tried your suggestion. This:
```
python -c 'import datasets; ds="lama"; dataset = datasets.load_dataset(ds)["train"]; \
dataset.shard(1, 0, contiguous=True).to_json(f"{ds}-0.jsonl", orient="records", lines=True, force_ascii=False)'
```
works fine and takes just a few GBs to complete.
this on the other hand blows up memory-wise:
```
python -c 'import datasets; ds="ascent_kb"; dataset = datasets.load_dataset(ds)["train"]; \
dataset.shard(1, 0, contiguous=True).to_json(f"{ds}-0.jsonl", orient="records", lines=True, force_ascii=False)'
```
and I have to kill it before it uses up all RAM. (I have 128GB of it, so it should be more than enough) | Closes #2663. I've tried adding multiprocessing in `to_json`. Here's some benchmarking I did to compare the timings of current version (say v1) and multi-proc version (say v2). I did this with `cpu_count` 4 (2015 Macbook Air)
1. Dataset name: `ascent_kb` - 8.9M samples (all samples were used, reporting this for a single run)
v1- ~225 seconds for converting whole dataset to json
v2- ~200 seconds for converting whole dataset to json
2. Dataset name: `lama` - 1.3M samples (all samples were used, reporting this for 2 runs)
v1- ~26 seconds for converting whole dataset to json
v2- ~23.6 seconds for converting whole dataset to json
I think it's safe to say that v2 is 10% faster as compared to v1. Timings may improve further with better configuration.
The only bottleneck I feel is writing to file from the output list. If we can improve that aspect then timings may improve further.
Let me know if any changes/improvements can be done in this @stas00, @lhoestq, @albertvillanova. @lhoestq even suggested to extend this work with other export methods as well like `csv` or `parquet`. | 111 | text: add multi-proc in `to_json`
Closes #2663. I've tried adding multiprocessing in `to_json`. Here's some benchmarking I did to compare the timings of current version (say v1) and multi-proc version (say v2). I did this with `cpu_count` 4 (2015 Macbook Air)
1. Dataset name: `ascent_kb` - 8.9M samples (all samples were used, reporting this for a single run)
v1- ~225 seconds for converting whole dataset to json
v2- ~200 seconds for converting whole dataset to json
2. Dataset name: `lama` - 1.3M samples (all samples were used, reporting this for 2 runs)
v1- ~26 seconds for converting whole dataset to json
v2- ~23.6 seconds for converting whole dataset to json
I think it's safe to say that v2 is 10% faster as compared to v1. Timings may improve further with better configuration.
The only bottleneck I feel is writing to file from the output list. If we can improve that aspect then timings may improve further.
Let me know if any changes/improvements can be done in this @stas00, @lhoestq, @albertvillanova. @lhoestq even suggested to extend this work with other export methods as well like `csv` or `parquet`.
I don't think the issue has anything to do with your work, @bhavitvyamalik. I forgot to mention I tested to see the same problem with the latest datasets release.
Interesting, I tried your suggestion. This:
```
python -c 'import datasets; ds="lama"; dataset = datasets.load_dataset(ds)["train"]; \
dataset.shard(1, 0, contiguous=True).to_json(f"{ds}-0.jsonl", orient="records", lines=True, force_ascii=False)'
```
works fine and takes just a few GBs to complete.
this on the other hand blows up memory-wise:
```
python -c 'import datasets; ds="ascent_kb"; dataset = datasets.load_dataset(ds)["train"]; \
dataset.shard(1, 0, contiguous=True).to_json(f"{ds}-0.jsonl", orient="records", lines=True, force_ascii=False)'
```
and I have to kill it before it uses up all RAM. (I have 128GB of it, so it should be more than enough) |
https://github.com/huggingface/datasets/pull/2747 | add multi-proc in `to_json` | > That's because some dataset related files were still left inside ~/.cache/huggingface/datasets folder. You can wipe off datasets folder inside your cache maybe
I think recent datasets added a method that will print out the path for all the different components for a given dataset, I can't recall the name though. It was when we were discussing a janitor program to clear up space selectively. | Closes #2663. I've tried adding multiprocessing in `to_json`. Here's some benchmarking I did to compare the timings of current version (say v1) and multi-proc version (say v2). I did this with `cpu_count` 4 (2015 Macbook Air)
1. Dataset name: `ascent_kb` - 8.9M samples (all samples were used, reporting this for a single run)
v1- ~225 seconds for converting whole dataset to json
v2- ~200 seconds for converting whole dataset to json
2. Dataset name: `lama` - 1.3M samples (all samples were used, reporting this for 2 runs)
v1- ~26 seconds for converting whole dataset to json
v2- ~23.6 seconds for converting whole dataset to json
I think it's safe to say that v2 is 10% faster as compared to v1. Timings may improve further with better configuration.
The only bottleneck I feel is writing to file from the output list. If we can improve that aspect then timings may improve further.
Let me know if any changes/improvements can be done in this @stas00, @lhoestq, @albertvillanova. @lhoestq even suggested to extend this work with other export methods as well like `csv` or `parquet`. | 65 | text: add multi-proc in `to_json`
Closes #2663. I've tried adding multiprocessing in `to_json`. Here's some benchmarking I did to compare the timings of current version (say v1) and multi-proc version (say v2). I did this with `cpu_count` 4 (2015 Macbook Air)
1. Dataset name: `ascent_kb` - 8.9M samples (all samples were used, reporting this for a single run)
v1- ~225 seconds for converting whole dataset to json
v2- ~200 seconds for converting whole dataset to json
2. Dataset name: `lama` - 1.3M samples (all samples were used, reporting this for 2 runs)
v1- ~26 seconds for converting whole dataset to json
v2- ~23.6 seconds for converting whole dataset to json
I think it's safe to say that v2 is 10% faster as compared to v1. Timings may improve further with better configuration.
The only bottleneck I feel is writing to file from the output list. If we can improve that aspect then timings may improve further.
Let me know if any changes/improvements can be done in this @stas00, @lhoestq, @albertvillanova. @lhoestq even suggested to extend this work with other export methods as well like `csv` or `parquet`.
> That's because some dataset related files were still left inside ~/.cache/huggingface/datasets folder. You can wipe off datasets folder inside your cache maybe
I think recent datasets added a method that will print out the path for all the different components for a given dataset, I can't recall the name though. It was when we were discussing a janitor program to clear up space selectively. |
https://github.com/huggingface/datasets/pull/2747 | add multi-proc in `to_json` | > and I have to kill it before it uses up all RAM. (I have 128GB of it, so it should be more than enough)
Same thing just happened on my machine too. Memory leak somewhere maybe? Even if you were to load this dataset in your memory it shouldn't take more than 4GB. You were earlier doing this for `oscar` dataset. Is it working fine for that? | Closes #2663. I've tried adding multiprocessing in `to_json`. Here's some benchmarking I did to compare the timings of current version (say v1) and multi-proc version (say v2). I did this with `cpu_count` 4 (2015 Macbook Air)
1. Dataset name: `ascent_kb` - 8.9M samples (all samples were used, reporting this for a single run)
v1- ~225 seconds for converting whole dataset to json
v2- ~200 seconds for converting whole dataset to json
2. Dataset name: `lama` - 1.3M samples (all samples were used, reporting this for 2 runs)
v1- ~26 seconds for converting whole dataset to json
v2- ~23.6 seconds for converting whole dataset to json
I think it's safe to say that v2 is 10% faster as compared to v1. Timings may improve further with better configuration.
The only bottleneck I feel is writing to file from the output list. If we can improve that aspect then timings may improve further.
Let me know if any changes/improvements can be done in this @stas00, @lhoestq, @albertvillanova. @lhoestq even suggested to extend this work with other export methods as well like `csv` or `parquet`. | 68 | text: add multi-proc in `to_json`
Closes #2663. I've tried adding multiprocessing in `to_json`. Here's some benchmarking I did to compare the timings of current version (say v1) and multi-proc version (say v2). I did this with `cpu_count` 4 (2015 Macbook Air)
1. Dataset name: `ascent_kb` - 8.9M samples (all samples were used, reporting this for a single run)
v1- ~225 seconds for converting whole dataset to json
v2- ~200 seconds for converting whole dataset to json
2. Dataset name: `lama` - 1.3M samples (all samples were used, reporting this for 2 runs)
v1- ~26 seconds for converting whole dataset to json
v2- ~23.6 seconds for converting whole dataset to json
I think it's safe to say that v2 is 10% faster as compared to v1. Timings may improve further with better configuration.
The only bottleneck I feel is writing to file from the output list. If we can improve that aspect then timings may improve further.
Let me know if any changes/improvements can be done in this @stas00, @lhoestq, @albertvillanova. @lhoestq even suggested to extend this work with other export methods as well like `csv` or `parquet`.
> and I have to kill it before it uses up all RAM. (I have 128GB of it, so it should be more than enough)
Same thing just happened on my machine too. Memory leak somewhere maybe? Even if you were to load this dataset in your memory it shouldn't take more than 4GB. You were earlier doing this for `oscar` dataset. Is it working fine for that? |
https://github.com/huggingface/datasets/pull/2747 | add multi-proc in `to_json` | Hmm, looks like `datasets` has changed and won't accept my currently cached oscar-en (crashes), so I'd rather not download 0.5TB again.
Were you able to reproduce the memory blow up with `ascent_kb`? It's should be a much quicker task to verify.
But yes, oscar worked just fine with `.shard()` which is what I used to process it fast. | Closes #2663. I've tried adding multiprocessing in `to_json`. Here's some benchmarking I did to compare the timings of current version (say v1) and multi-proc version (say v2). I did this with `cpu_count` 4 (2015 Macbook Air)
1. Dataset name: `ascent_kb` - 8.9M samples (all samples were used, reporting this for a single run)
v1- ~225 seconds for converting whole dataset to json
v2- ~200 seconds for converting whole dataset to json
2. Dataset name: `lama` - 1.3M samples (all samples were used, reporting this for 2 runs)
v1- ~26 seconds for converting whole dataset to json
v2- ~23.6 seconds for converting whole dataset to json
I think it's safe to say that v2 is 10% faster as compared to v1. Timings may improve further with better configuration.
The only bottleneck I feel is writing to file from the output list. If we can improve that aspect then timings may improve further.
Let me know if any changes/improvements can be done in this @stas00, @lhoestq, @albertvillanova. @lhoestq even suggested to extend this work with other export methods as well like `csv` or `parquet`. | 58 | text: add multi-proc in `to_json`
Closes #2663. I've tried adding multiprocessing in `to_json`. Here's some benchmarking I did to compare the timings of current version (say v1) and multi-proc version (say v2). I did this with `cpu_count` 4 (2015 Macbook Air)
1. Dataset name: `ascent_kb` - 8.9M samples (all samples were used, reporting this for a single run)
v1- ~225 seconds for converting whole dataset to json
v2- ~200 seconds for converting whole dataset to json
2. Dataset name: `lama` - 1.3M samples (all samples were used, reporting this for 2 runs)
v1- ~26 seconds for converting whole dataset to json
v2- ~23.6 seconds for converting whole dataset to json
I think it's safe to say that v2 is 10% faster as compared to v1. Timings may improve further with better configuration.
The only bottleneck I feel is writing to file from the output list. If we can improve that aspect then timings may improve further.
Let me know if any changes/improvements can be done in this @stas00, @lhoestq, @albertvillanova. @lhoestq even suggested to extend this work with other export methods as well like `csv` or `parquet`.
Hmm, looks like `datasets` has changed and won't accept my currently cached oscar-en (crashes), so I'd rather not download 0.5TB again.
Were you able to reproduce the memory blow up with `ascent_kb`? It's should be a much quicker task to verify.
But yes, oscar worked just fine with `.shard()` which is what I used to process it fast. |
https://github.com/huggingface/datasets/pull/2747 | add multi-proc in `to_json` | What I tried is:
```
HF_DATASETS_OFFLINE=1 HF_DATASETS_CACHE=cache python -c 'import datasets; ds="oscar"; \
dataset = datasets.load_dataset(ds, "unshuffled_deduplicated_en")["train"]; \
dataset.shard(1000000, 0, contiguous=True).to_json(f"{ds}-0.jsonl", orient="records", lines=True, force_ascii=False)'
```
and got:
```
Using the latest cached version of the module from /gpfswork/rech/six/commun/modules/datasets_modules/datasets/oscar/e4f06cecc7ae02f7adf85640b4019bf476d44453f251a1d84aebae28b0f8d51d (last modified on Fri Aug 6 01:52:35 2021) since it couldn't be found locally at oscar/oscar.py or remotely (OfflineModeIsEnabled).
Reusing dataset oscar (cache/oscar/unshuffled_deduplicated_en/1.0.0/e4f06cecc7ae02f7adf85640b4019bf476d44453f251a1d84aebae28b0f8d51d)
Traceback (most recent call last):
File "<string>", line 1, in <module>
File "/gpfswork/rech/six/commun/conda/stas/lib/python3.8/site-packages/datasets/load.py", line 755, in load_dataset
ds = builder_instance.as_dataset(split=split, ignore_verifications=ignore_verifications, in_memory=keep_in_memory)
File "/gpfswork/rech/six/commun/conda/stas/lib/python3.8/site-packages/datasets/builder.py", line 737, in as_dataset
datasets = utils.map_nested(
File "/gpfswork/rech/six/commun/conda/stas/lib/python3.8/site-packages/datasets/utils/py_utils.py", line 203, in map_nested
mapped = [
File "/gpfswork/rech/six/commun/conda/stas/lib/python3.8/site-packages/datasets/utils/py_utils.py", line 204, in <listcomp>
_single_map_nested((function, obj, types, None, True)) for obj in tqdm(iterable, disable=disable_tqdm)
File "/gpfswork/rech/six/commun/conda/stas/lib/python3.8/site-packages/datasets/utils/py_utils.py", line 142, in _single_map_nested
return function(data_struct)
File "/gpfswork/rech/six/commun/conda/stas/lib/python3.8/site-packages/datasets/builder.py", line 764, in _build_single_dataset
ds = self._as_dataset(
File "/gpfswork/rech/six/commun/conda/stas/lib/python3.8/site-packages/datasets/builder.py", line 834, in _as_dataset
dataset_kwargs = ArrowReader(self._cache_dir, self.info).read(
File "/gpfswork/rech/six/commun/conda/stas/lib/python3.8/site-packages/datasets/arrow_reader.py", line 217, in read
return self.read_files(files=files, original_instructions=instructions, in_memory=in_memory)
File "/gpfswork/rech/six/commun/conda/stas/lib/python3.8/site-packages/datasets/arrow_reader.py", line 238, in read_files
pa_table = self._read_files(files, in_memory=in_memory)
File "/gpfswork/rech/six/commun/conda/stas/lib/python3.8/site-packages/datasets/arrow_reader.py", line 173, in _read_files
pa_table: Table = self._get_table_from_filename(f_dict, in_memory=in_memory)
File "/gpfswork/rech/six/commun/conda/stas/lib/python3.8/site-packages/datasets/arrow_reader.py", line 308, in _get_table_from_filename
table = ArrowReader.read_table(filename, in_memory=in_memory)
File "/gpfswork/rech/six/commun/conda/stas/lib/python3.8/site-packages/datasets/arrow_reader.py", line 327, in read_table
return table_cls.from_file(filename)
File "/gpfswork/rech/six/commun/conda/stas/lib/python3.8/site-packages/datasets/table.py", line 450, in from_file
table = _memory_mapped_arrow_table_from_file(filename)
File "/gpfswork/rech/six/commun/conda/stas/lib/python3.8/site-packages/datasets/table.py", line 43, in _memory_mapped_arrow_table_from_file
memory_mapped_stream = pa.memory_map(filename)
File "pyarrow/io.pxi", line 782, in pyarrow.lib.memory_map
File "pyarrow/io.pxi", line 743, in pyarrow.lib.MemoryMappedFile._open
File "pyarrow/error.pxi", line 122, in pyarrow.lib.pyarrow_internal_check_status
File "pyarrow/error.pxi", line 99, in pyarrow.lib.check_status
OSError: Memory mapping file failed: Cannot allocate memory
``` | Closes #2663. I've tried adding multiprocessing in `to_json`. Here's some benchmarking I did to compare the timings of current version (say v1) and multi-proc version (say v2). I did this with `cpu_count` 4 (2015 Macbook Air)
1. Dataset name: `ascent_kb` - 8.9M samples (all samples were used, reporting this for a single run)
v1- ~225 seconds for converting whole dataset to json
v2- ~200 seconds for converting whole dataset to json
2. Dataset name: `lama` - 1.3M samples (all samples were used, reporting this for 2 runs)
v1- ~26 seconds for converting whole dataset to json
v2- ~23.6 seconds for converting whole dataset to json
I think it's safe to say that v2 is 10% faster as compared to v1. Timings may improve further with better configuration.
The only bottleneck I feel is writing to file from the output list. If we can improve that aspect then timings may improve further.
Let me know if any changes/improvements can be done in this @stas00, @lhoestq, @albertvillanova. @lhoestq even suggested to extend this work with other export methods as well like `csv` or `parquet`. | 244 | text: add multi-proc in `to_json`
Closes #2663. I've tried adding multiprocessing in `to_json`. Here's some benchmarking I did to compare the timings of current version (say v1) and multi-proc version (say v2). I did this with `cpu_count` 4 (2015 Macbook Air)
1. Dataset name: `ascent_kb` - 8.9M samples (all samples were used, reporting this for a single run)
v1- ~225 seconds for converting whole dataset to json
v2- ~200 seconds for converting whole dataset to json
2. Dataset name: `lama` - 1.3M samples (all samples were used, reporting this for 2 runs)
v1- ~26 seconds for converting whole dataset to json
v2- ~23.6 seconds for converting whole dataset to json
I think it's safe to say that v2 is 10% faster as compared to v1. Timings may improve further with better configuration.
The only bottleneck I feel is writing to file from the output list. If we can improve that aspect then timings may improve further.
Let me know if any changes/improvements can be done in this @stas00, @lhoestq, @albertvillanova. @lhoestq even suggested to extend this work with other export methods as well like `csv` or `parquet`.
What I tried is:
```
HF_DATASETS_OFFLINE=1 HF_DATASETS_CACHE=cache python -c 'import datasets; ds="oscar"; \
dataset = datasets.load_dataset(ds, "unshuffled_deduplicated_en")["train"]; \
dataset.shard(1000000, 0, contiguous=True).to_json(f"{ds}-0.jsonl", orient="records", lines=True, force_ascii=False)'
```
and got:
```
Using the latest cached version of the module from /gpfswork/rech/six/commun/modules/datasets_modules/datasets/oscar/e4f06cecc7ae02f7adf85640b4019bf476d44453f251a1d84aebae28b0f8d51d (last modified on Fri Aug 6 01:52:35 2021) since it couldn't be found locally at oscar/oscar.py or remotely (OfflineModeIsEnabled).
Reusing dataset oscar (cache/oscar/unshuffled_deduplicated_en/1.0.0/e4f06cecc7ae02f7adf85640b4019bf476d44453f251a1d84aebae28b0f8d51d)
Traceback (most recent call last):
File "<string>", line 1, in <module>
File "/gpfswork/rech/six/commun/conda/stas/lib/python3.8/site-packages/datasets/load.py", line 755, in load_dataset
ds = builder_instance.as_dataset(split=split, ignore_verifications=ignore_verifications, in_memory=keep_in_memory)
File "/gpfswork/rech/six/commun/conda/stas/lib/python3.8/site-packages/datasets/builder.py", line 737, in as_dataset
datasets = utils.map_nested(
File "/gpfswork/rech/six/commun/conda/stas/lib/python3.8/site-packages/datasets/utils/py_utils.py", line 203, in map_nested
mapped = [
File "/gpfswork/rech/six/commun/conda/stas/lib/python3.8/site-packages/datasets/utils/py_utils.py", line 204, in <listcomp>
_single_map_nested((function, obj, types, None, True)) for obj in tqdm(iterable, disable=disable_tqdm)
File "/gpfswork/rech/six/commun/conda/stas/lib/python3.8/site-packages/datasets/utils/py_utils.py", line 142, in _single_map_nested
return function(data_struct)
File "/gpfswork/rech/six/commun/conda/stas/lib/python3.8/site-packages/datasets/builder.py", line 764, in _build_single_dataset
ds = self._as_dataset(
File "/gpfswork/rech/six/commun/conda/stas/lib/python3.8/site-packages/datasets/builder.py", line 834, in _as_dataset
dataset_kwargs = ArrowReader(self._cache_dir, self.info).read(
File "/gpfswork/rech/six/commun/conda/stas/lib/python3.8/site-packages/datasets/arrow_reader.py", line 217, in read
return self.read_files(files=files, original_instructions=instructions, in_memory=in_memory)
File "/gpfswork/rech/six/commun/conda/stas/lib/python3.8/site-packages/datasets/arrow_reader.py", line 238, in read_files
pa_table = self._read_files(files, in_memory=in_memory)
File "/gpfswork/rech/six/commun/conda/stas/lib/python3.8/site-packages/datasets/arrow_reader.py", line 173, in _read_files
pa_table: Table = self._get_table_from_filename(f_dict, in_memory=in_memory)
File "/gpfswork/rech/six/commun/conda/stas/lib/python3.8/site-packages/datasets/arrow_reader.py", line 308, in _get_table_from_filename
table = ArrowReader.read_table(filename, in_memory=in_memory)
File "/gpfswork/rech/six/commun/conda/stas/lib/python3.8/site-packages/datasets/arrow_reader.py", line 327, in read_table
return table_cls.from_file(filename)
File "/gpfswork/rech/six/commun/conda/stas/lib/python3.8/site-packages/datasets/table.py", line 450, in from_file
table = _memory_mapped_arrow_table_from_file(filename)
File "/gpfswork/rech/six/commun/conda/stas/lib/python3.8/site-packages/datasets/table.py", line 43, in _memory_mapped_arrow_table_from_file
memory_mapped_stream = pa.memory_map(filename)
File "pyarrow/io.pxi", line 782, in pyarrow.lib.memory_map
File "pyarrow/io.pxi", line 743, in pyarrow.lib.MemoryMappedFile._open
File "pyarrow/error.pxi", line 122, in pyarrow.lib.pyarrow_internal_check_status
File "pyarrow/error.pxi", line 99, in pyarrow.lib.check_status
OSError: Memory mapping file failed: Cannot allocate memory
``` |
https://github.com/huggingface/datasets/pull/2747 | add multi-proc in `to_json` | > Were you able to reproduce the memory blow up with ascent_kb? It's should be a much quicker task to verify.
Yes, this blows up memory-wise on my machine too.
I found that a [similar error](https://discuss.huggingface.co/t/saving-memory-with-run-mlm-py-with-wikipedia-datasets/4160) was posted on the forum on 5th March. Since you already knew how much time [#2663 comment](https://github.com/huggingface/datasets/issues/2663#issue-946552273) took, can you try benchmarking v1 and v2 for now maybe until we have a fix for this memory blow up? | Closes #2663. I've tried adding multiprocessing in `to_json`. Here's some benchmarking I did to compare the timings of current version (say v1) and multi-proc version (say v2). I did this with `cpu_count` 4 (2015 Macbook Air)
1. Dataset name: `ascent_kb` - 8.9M samples (all samples were used, reporting this for a single run)
v1- ~225 seconds for converting whole dataset to json
v2- ~200 seconds for converting whole dataset to json
2. Dataset name: `lama` - 1.3M samples (all samples were used, reporting this for 2 runs)
v1- ~26 seconds for converting whole dataset to json
v2- ~23.6 seconds for converting whole dataset to json
I think it's safe to say that v2 is 10% faster as compared to v1. Timings may improve further with better configuration.
The only bottleneck I feel is writing to file from the output list. If we can improve that aspect then timings may improve further.
Let me know if any changes/improvements can be done in this @stas00, @lhoestq, @albertvillanova. @lhoestq even suggested to extend this work with other export methods as well like `csv` or `parquet`. | 74 | text: add multi-proc in `to_json`
Closes #2663. I've tried adding multiprocessing in `to_json`. Here's some benchmarking I did to compare the timings of current version (say v1) and multi-proc version (say v2). I did this with `cpu_count` 4 (2015 Macbook Air)
1. Dataset name: `ascent_kb` - 8.9M samples (all samples were used, reporting this for a single run)
v1- ~225 seconds for converting whole dataset to json
v2- ~200 seconds for converting whole dataset to json
2. Dataset name: `lama` - 1.3M samples (all samples were used, reporting this for 2 runs)
v1- ~26 seconds for converting whole dataset to json
v2- ~23.6 seconds for converting whole dataset to json
I think it's safe to say that v2 is 10% faster as compared to v1. Timings may improve further with better configuration.
The only bottleneck I feel is writing to file from the output list. If we can improve that aspect then timings may improve further.
Let me know if any changes/improvements can be done in this @stas00, @lhoestq, @albertvillanova. @lhoestq even suggested to extend this work with other export methods as well like `csv` or `parquet`.
> Were you able to reproduce the memory blow up with ascent_kb? It's should be a much quicker task to verify.
Yes, this blows up memory-wise on my machine too.
I found that a [similar error](https://discuss.huggingface.co/t/saving-memory-with-run-mlm-py-with-wikipedia-datasets/4160) was posted on the forum on 5th March. Since you already knew how much time [#2663 comment](https://github.com/huggingface/datasets/issues/2663#issue-946552273) took, can you try benchmarking v1 and v2 for now maybe until we have a fix for this memory blow up? |
https://github.com/huggingface/datasets/pull/2747 | add multi-proc in `to_json` | OK, so I benchmarked using "lama" though it's too small for this kind of test, since the sharding is much slower than one thread here.
Results: https://gist.github.com/stas00/dc1597a1e245c5915cfeefa0eee6902c
So sharding does really bad there, and your json over procs is doing great!
Any suggestions to a somewhat bigger dataset, but not too big? say 10 times of lama? | Closes #2663. I've tried adding multiprocessing in `to_json`. Here's some benchmarking I did to compare the timings of current version (say v1) and multi-proc version (say v2). I did this with `cpu_count` 4 (2015 Macbook Air)
1. Dataset name: `ascent_kb` - 8.9M samples (all samples were used, reporting this for a single run)
v1- ~225 seconds for converting whole dataset to json
v2- ~200 seconds for converting whole dataset to json
2. Dataset name: `lama` - 1.3M samples (all samples were used, reporting this for 2 runs)
v1- ~26 seconds for converting whole dataset to json
v2- ~23.6 seconds for converting whole dataset to json
I think it's safe to say that v2 is 10% faster as compared to v1. Timings may improve further with better configuration.
The only bottleneck I feel is writing to file from the output list. If we can improve that aspect then timings may improve further.
Let me know if any changes/improvements can be done in this @stas00, @lhoestq, @albertvillanova. @lhoestq even suggested to extend this work with other export methods as well like `csv` or `parquet`. | 57 | text: add multi-proc in `to_json`
Closes #2663. I've tried adding multiprocessing in `to_json`. Here's some benchmarking I did to compare the timings of current version (say v1) and multi-proc version (say v2). I did this with `cpu_count` 4 (2015 Macbook Air)
1. Dataset name: `ascent_kb` - 8.9M samples (all samples were used, reporting this for a single run)
v1- ~225 seconds for converting whole dataset to json
v2- ~200 seconds for converting whole dataset to json
2. Dataset name: `lama` - 1.3M samples (all samples were used, reporting this for 2 runs)
v1- ~26 seconds for converting whole dataset to json
v2- ~23.6 seconds for converting whole dataset to json
I think it's safe to say that v2 is 10% faster as compared to v1. Timings may improve further with better configuration.
The only bottleneck I feel is writing to file from the output list. If we can improve that aspect then timings may improve further.
Let me know if any changes/improvements can be done in this @stas00, @lhoestq, @albertvillanova. @lhoestq even suggested to extend this work with other export methods as well like `csv` or `parquet`.
OK, so I benchmarked using "lama" though it's too small for this kind of test, since the sharding is much slower than one thread here.
Results: https://gist.github.com/stas00/dc1597a1e245c5915cfeefa0eee6902c
So sharding does really bad there, and your json over procs is doing great!
Any suggestions to a somewhat bigger dataset, but not too big? say 10 times of lama? |
https://github.com/huggingface/datasets/pull/2747 | add multi-proc in `to_json` | Looks great! I had a few questions/suggestions related to `benchmark-datasets-to_json.py`:
1. You have used only 10_000 and 100_000 batch size. Including more batch sizes may help you find the perfect batch size for your machine and even give you some extra speed-up.
For eg, I found `load_dataset("cc100", lang="eu")` with batch size 125_000 took less time as compared to batch size 100_000 (71.16 sec v/s 67.26 sec) since this dataset has 2 fields only `['id', 'text']`, so that's why we can go for higher batch size here.
2. Why have you used `num_procs` 1 and 4 only?
You can use:
1. `dataset = load_dataset("cc100", lang="af")`. Even though it has only 2 fields but there are around 9.9 mil samples. (lama had around 1.3 mil samples)
2. `dataset = load_dataset("cc100", lang="eu")` -> 16 mil samples. (if you want something more than 9.9 mil)
3. `dataset = load_dataset("neural_code_search", 'search_corpus')` -> 4.7 mil samples | Closes #2663. I've tried adding multiprocessing in `to_json`. Here's some benchmarking I did to compare the timings of current version (say v1) and multi-proc version (say v2). I did this with `cpu_count` 4 (2015 Macbook Air)
1. Dataset name: `ascent_kb` - 8.9M samples (all samples were used, reporting this for a single run)
v1- ~225 seconds for converting whole dataset to json
v2- ~200 seconds for converting whole dataset to json
2. Dataset name: `lama` - 1.3M samples (all samples were used, reporting this for 2 runs)
v1- ~26 seconds for converting whole dataset to json
v2- ~23.6 seconds for converting whole dataset to json
I think it's safe to say that v2 is 10% faster as compared to v1. Timings may improve further with better configuration.
The only bottleneck I feel is writing to file from the output list. If we can improve that aspect then timings may improve further.
Let me know if any changes/improvements can be done in this @stas00, @lhoestq, @albertvillanova. @lhoestq even suggested to extend this work with other export methods as well like `csv` or `parquet`. | 150 | text: add multi-proc in `to_json`
Closes #2663. I've tried adding multiprocessing in `to_json`. Here's some benchmarking I did to compare the timings of current version (say v1) and multi-proc version (say v2). I did this with `cpu_count` 4 (2015 Macbook Air)
1. Dataset name: `ascent_kb` - 8.9M samples (all samples were used, reporting this for a single run)
v1- ~225 seconds for converting whole dataset to json
v2- ~200 seconds for converting whole dataset to json
2. Dataset name: `lama` - 1.3M samples (all samples were used, reporting this for 2 runs)
v1- ~26 seconds for converting whole dataset to json
v2- ~23.6 seconds for converting whole dataset to json
I think it's safe to say that v2 is 10% faster as compared to v1. Timings may improve further with better configuration.
The only bottleneck I feel is writing to file from the output list. If we can improve that aspect then timings may improve further.
Let me know if any changes/improvements can be done in this @stas00, @lhoestq, @albertvillanova. @lhoestq even suggested to extend this work with other export methods as well like `csv` or `parquet`.
Looks great! I had a few questions/suggestions related to `benchmark-datasets-to_json.py`:
1. You have used only 10_000 and 100_000 batch size. Including more batch sizes may help you find the perfect batch size for your machine and even give you some extra speed-up.
For eg, I found `load_dataset("cc100", lang="eu")` with batch size 125_000 took less time as compared to batch size 100_000 (71.16 sec v/s 67.26 sec) since this dataset has 2 fields only `['id', 'text']`, so that's why we can go for higher batch size here.
2. Why have you used `num_procs` 1 and 4 only?
You can use:
1. `dataset = load_dataset("cc100", lang="af")`. Even though it has only 2 fields but there are around 9.9 mil samples. (lama had around 1.3 mil samples)
2. `dataset = load_dataset("cc100", lang="eu")` -> 16 mil samples. (if you want something more than 9.9 mil)
3. `dataset = load_dataset("neural_code_search", 'search_corpus')` -> 4.7 mil samples |
https://github.com/huggingface/datasets/pull/2747 | add multi-proc in `to_json` | Thank you, @bhavitvyamalik
My apologies, at the moment I have not found time to do more benchmark with the proposed other datasets. I will try to do it later, but I don't want it to hold your PR, it's definitely a great improvement based on the benchmarks I did run! And the comparison to sharded is really just of interest to me to see if it's on par or slower.
So if other reviewers are happy, this definitely looks like a great improvement to me and addresses the request I made in the first place.
> Why have you used num_procs 1 and 4 only?
Oh, no particular reason, I was just comparing to 4 shards on my desktop. Typically it's sufficient to go from 1 to 2-4 to see whether the distributed approach is faster or not. Once hit larger numbers you often run into bottlenecks like IO, and then numbers can be less representative. I hope it makes sense. | Closes #2663. I've tried adding multiprocessing in `to_json`. Here's some benchmarking I did to compare the timings of current version (say v1) and multi-proc version (say v2). I did this with `cpu_count` 4 (2015 Macbook Air)
1. Dataset name: `ascent_kb` - 8.9M samples (all samples were used, reporting this for a single run)
v1- ~225 seconds for converting whole dataset to json
v2- ~200 seconds for converting whole dataset to json
2. Dataset name: `lama` - 1.3M samples (all samples were used, reporting this for 2 runs)
v1- ~26 seconds for converting whole dataset to json
v2- ~23.6 seconds for converting whole dataset to json
I think it's safe to say that v2 is 10% faster as compared to v1. Timings may improve further with better configuration.
The only bottleneck I feel is writing to file from the output list. If we can improve that aspect then timings may improve further.
Let me know if any changes/improvements can be done in this @stas00, @lhoestq, @albertvillanova. @lhoestq even suggested to extend this work with other export methods as well like `csv` or `parquet`. | 161 | text: add multi-proc in `to_json`
Closes #2663. I've tried adding multiprocessing in `to_json`. Here's some benchmarking I did to compare the timings of current version (say v1) and multi-proc version (say v2). I did this with `cpu_count` 4 (2015 Macbook Air)
1. Dataset name: `ascent_kb` - 8.9M samples (all samples were used, reporting this for a single run)
v1- ~225 seconds for converting whole dataset to json
v2- ~200 seconds for converting whole dataset to json
2. Dataset name: `lama` - 1.3M samples (all samples were used, reporting this for 2 runs)
v1- ~26 seconds for converting whole dataset to json
v2- ~23.6 seconds for converting whole dataset to json
I think it's safe to say that v2 is 10% faster as compared to v1. Timings may improve further with better configuration.
The only bottleneck I feel is writing to file from the output list. If we can improve that aspect then timings may improve further.
Let me know if any changes/improvements can be done in this @stas00, @lhoestq, @albertvillanova. @lhoestq even suggested to extend this work with other export methods as well like `csv` or `parquet`.
Thank you, @bhavitvyamalik
My apologies, at the moment I have not found time to do more benchmark with the proposed other datasets. I will try to do it later, but I don't want it to hold your PR, it's definitely a great improvement based on the benchmarks I did run! And the comparison to sharded is really just of interest to me to see if it's on par or slower.
So if other reviewers are happy, this definitely looks like a great improvement to me and addresses the request I made in the first place.
> Why have you used num_procs 1 and 4 only?
Oh, no particular reason, I was just comparing to 4 shards on my desktop. Typically it's sufficient to go from 1 to 2-4 to see whether the distributed approach is faster or not. Once hit larger numbers you often run into bottlenecks like IO, and then numbers can be less representative. I hope it makes sense. |
https://github.com/huggingface/datasets/pull/2747 | add multi-proc in `to_json` | Tested it with a larger dataset (`srwac`) and memory utilisation remained constant with no swap memory used. @lhoestq should I also add test for the same? Last time I tried this, I got `OSError: [Errno 12] Cannot allocate memory` in CircleCI tests | Closes #2663. I've tried adding multiprocessing in `to_json`. Here's some benchmarking I did to compare the timings of current version (say v1) and multi-proc version (say v2). I did this with `cpu_count` 4 (2015 Macbook Air)
1. Dataset name: `ascent_kb` - 8.9M samples (all samples were used, reporting this for a single run)
v1- ~225 seconds for converting whole dataset to json
v2- ~200 seconds for converting whole dataset to json
2. Dataset name: `lama` - 1.3M samples (all samples were used, reporting this for 2 runs)
v1- ~26 seconds for converting whole dataset to json
v2- ~23.6 seconds for converting whole dataset to json
I think it's safe to say that v2 is 10% faster as compared to v1. Timings may improve further with better configuration.
The only bottleneck I feel is writing to file from the output list. If we can improve that aspect then timings may improve further.
Let me know if any changes/improvements can be done in this @stas00, @lhoestq, @albertvillanova. @lhoestq even suggested to extend this work with other export methods as well like `csv` or `parquet`. | 42 | text: add multi-proc in `to_json`
Closes #2663. I've tried adding multiprocessing in `to_json`. Here's some benchmarking I did to compare the timings of current version (say v1) and multi-proc version (say v2). I did this with `cpu_count` 4 (2015 Macbook Air)
1. Dataset name: `ascent_kb` - 8.9M samples (all samples were used, reporting this for a single run)
v1- ~225 seconds for converting whole dataset to json
v2- ~200 seconds for converting whole dataset to json
2. Dataset name: `lama` - 1.3M samples (all samples were used, reporting this for 2 runs)
v1- ~26 seconds for converting whole dataset to json
v2- ~23.6 seconds for converting whole dataset to json
I think it's safe to say that v2 is 10% faster as compared to v1. Timings may improve further with better configuration.
The only bottleneck I feel is writing to file from the output list. If we can improve that aspect then timings may improve further.
Let me know if any changes/improvements can be done in this @stas00, @lhoestq, @albertvillanova. @lhoestq even suggested to extend this work with other export methods as well like `csv` or `parquet`.
Tested it with a larger dataset (`srwac`) and memory utilisation remained constant with no swap memory used. @lhoestq should I also add test for the same? Last time I tried this, I got `OSError: [Errno 12] Cannot allocate memory` in CircleCI tests |
https://github.com/huggingface/datasets/pull/2745 | added semeval18_emotion_classification dataset | For training the multilabel classifier, I would combine the labels into a list, for example for the English dataset:
```
dfpre=pd.read_csv(path+"2018-E-c-En-train.txt",sep="\t")
dfpre['list'] = dfpre[dfpre.columns[2:]].values.tolist()
df = dfpre[['Tweet', 'list']].copy()
df.rename(columns={'list': 'labels'}, inplace=True)
``` | I added the data set of SemEval 2018 Task 1 (Subtask 5) for emotion detection in three languages.
```
datasets-cli test datasets/semeval18_emotion_classification/ --save_infos --all_configs
RUN_SLOW=1 pytest tests/test_dataset_common.py::LocalDatasetTest::test_load_real_dataset_semeval18_emotion_classification
```
Both commands ran successfully.
I couldn't create the dummy data (the files are tsvs but have .txt ending, maybe that's the problem?) and therefore the test on the dummy data fails, maybe someone can help here.
I also formatted the code:
```
black --line-length 119 --target-version py36 datasets/semeval18_emotion_classification/
isort datasets/semeval18_emotion_classification/
flake8 datasets/semeval18_emotion_classification/
```
That's the publication for reference:
Mohammad, S., Bravo-Marquez, F., Salameh, M., & Kiritchenko, S. (2018). SemEval-2018 task 1: Affect in tweets. Proceedings of the 12th International Workshop on Semantic Evaluation, 1β17. https://doi.org/10.18653/v1/S18-1001 | 32 | text: added semeval18_emotion_classification dataset
I added the data set of SemEval 2018 Task 1 (Subtask 5) for emotion detection in three languages.
```
datasets-cli test datasets/semeval18_emotion_classification/ --save_infos --all_configs
RUN_SLOW=1 pytest tests/test_dataset_common.py::LocalDatasetTest::test_load_real_dataset_semeval18_emotion_classification
```
Both commands ran successfully.
I couldn't create the dummy data (the files are tsvs but have .txt ending, maybe that's the problem?) and therefore the test on the dummy data fails, maybe someone can help here.
I also formatted the code:
```
black --line-length 119 --target-version py36 datasets/semeval18_emotion_classification/
isort datasets/semeval18_emotion_classification/
flake8 datasets/semeval18_emotion_classification/
```
That's the publication for reference:
Mohammad, S., Bravo-Marquez, F., Salameh, M., & Kiritchenko, S. (2018). SemEval-2018 task 1: Affect in tweets. Proceedings of the 12th International Workshop on Semantic Evaluation, 1β17. https://doi.org/10.18653/v1/S18-1001
For training the multilabel classifier, I would combine the labels into a list, for example for the English dataset:
```
dfpre=pd.read_csv(path+"2018-E-c-En-train.txt",sep="\t")
dfpre['list'] = dfpre[dfpre.columns[2:]].values.tolist()
df = dfpre[['Tweet', 'list']].copy()
df.rename(columns={'list': 'labels'}, inplace=True)
``` |
https://github.com/huggingface/datasets/pull/2745 | added semeval18_emotion_classification dataset | Hi @maxpel , have you had a chance to take my comments into account ?
Let me know if you have questions or if I can help :) | I added the data set of SemEval 2018 Task 1 (Subtask 5) for emotion detection in three languages.
```
datasets-cli test datasets/semeval18_emotion_classification/ --save_infos --all_configs
RUN_SLOW=1 pytest tests/test_dataset_common.py::LocalDatasetTest::test_load_real_dataset_semeval18_emotion_classification
```
Both commands ran successfully.
I couldn't create the dummy data (the files are tsvs but have .txt ending, maybe that's the problem?) and therefore the test on the dummy data fails, maybe someone can help here.
I also formatted the code:
```
black --line-length 119 --target-version py36 datasets/semeval18_emotion_classification/
isort datasets/semeval18_emotion_classification/
flake8 datasets/semeval18_emotion_classification/
```
That's the publication for reference:
Mohammad, S., Bravo-Marquez, F., Salameh, M., & Kiritchenko, S. (2018). SemEval-2018 task 1: Affect in tweets. Proceedings of the 12th International Workshop on Semantic Evaluation, 1β17. https://doi.org/10.18653/v1/S18-1001 | 28 | text: added semeval18_emotion_classification dataset
I added the data set of SemEval 2018 Task 1 (Subtask 5) for emotion detection in three languages.
```
datasets-cli test datasets/semeval18_emotion_classification/ --save_infos --all_configs
RUN_SLOW=1 pytest tests/test_dataset_common.py::LocalDatasetTest::test_load_real_dataset_semeval18_emotion_classification
```
Both commands ran successfully.
I couldn't create the dummy data (the files are tsvs but have .txt ending, maybe that's the problem?) and therefore the test on the dummy data fails, maybe someone can help here.
I also formatted the code:
```
black --line-length 119 --target-version py36 datasets/semeval18_emotion_classification/
isort datasets/semeval18_emotion_classification/
flake8 datasets/semeval18_emotion_classification/
```
That's the publication for reference:
Mohammad, S., Bravo-Marquez, F., Salameh, M., & Kiritchenko, S. (2018). SemEval-2018 task 1: Affect in tweets. Proceedings of the 12th International Workshop on Semantic Evaluation, 1β17. https://doi.org/10.18653/v1/S18-1001
Hi @maxpel , have you had a chance to take my comments into account ?
Let me know if you have questions or if I can help :) |
https://github.com/huggingface/datasets/pull/2745 | added semeval18_emotion_classification dataset | Hi @lhoestq ! I did take your comments into account, changed the naming and tried to add dummy data (manually). I am not sure if the dummy data is correct, maybe you can take a look at that.
The model card is still missing as I am currently very busy. | I added the data set of SemEval 2018 Task 1 (Subtask 5) for emotion detection in three languages.
```
datasets-cli test datasets/semeval18_emotion_classification/ --save_infos --all_configs
RUN_SLOW=1 pytest tests/test_dataset_common.py::LocalDatasetTest::test_load_real_dataset_semeval18_emotion_classification
```
Both commands ran successfully.
I couldn't create the dummy data (the files are tsvs but have .txt ending, maybe that's the problem?) and therefore the test on the dummy data fails, maybe someone can help here.
I also formatted the code:
```
black --line-length 119 --target-version py36 datasets/semeval18_emotion_classification/
isort datasets/semeval18_emotion_classification/
flake8 datasets/semeval18_emotion_classification/
```
That's the publication for reference:
Mohammad, S., Bravo-Marquez, F., Salameh, M., & Kiritchenko, S. (2018). SemEval-2018 task 1: Affect in tweets. Proceedings of the 12th International Workshop on Semantic Evaluation, 1β17. https://doi.org/10.18653/v1/S18-1001 | 50 | text: added semeval18_emotion_classification dataset
I added the data set of SemEval 2018 Task 1 (Subtask 5) for emotion detection in three languages.
```
datasets-cli test datasets/semeval18_emotion_classification/ --save_infos --all_configs
RUN_SLOW=1 pytest tests/test_dataset_common.py::LocalDatasetTest::test_load_real_dataset_semeval18_emotion_classification
```
Both commands ran successfully.
I couldn't create the dummy data (the files are tsvs but have .txt ending, maybe that's the problem?) and therefore the test on the dummy data fails, maybe someone can help here.
I also formatted the code:
```
black --line-length 119 --target-version py36 datasets/semeval18_emotion_classification/
isort datasets/semeval18_emotion_classification/
flake8 datasets/semeval18_emotion_classification/
```
That's the publication for reference:
Mohammad, S., Bravo-Marquez, F., Salameh, M., & Kiritchenko, S. (2018). SemEval-2018 task 1: Affect in tweets. Proceedings of the 12th International Workshop on Semantic Evaluation, 1β17. https://doi.org/10.18653/v1/S18-1001
Hi @lhoestq ! I did take your comments into account, changed the naming and tried to add dummy data (manually). I am not sure if the dummy data is correct, maybe you can take a look at that.
The model card is still missing as I am currently very busy. |
https://github.com/huggingface/datasets/pull/2745 | added semeval18_emotion_classification dataset | Thanks ! The dummy data looks all good, good job :)
The CI error can be fixed by merging `master` into your branch
```bash
git fetch upstream
git merge upstream/master
``` | I added the data set of SemEval 2018 Task 1 (Subtask 5) for emotion detection in three languages.
```
datasets-cli test datasets/semeval18_emotion_classification/ --save_infos --all_configs
RUN_SLOW=1 pytest tests/test_dataset_common.py::LocalDatasetTest::test_load_real_dataset_semeval18_emotion_classification
```
Both commands ran successfully.
I couldn't create the dummy data (the files are tsvs but have .txt ending, maybe that's the problem?) and therefore the test on the dummy data fails, maybe someone can help here.
I also formatted the code:
```
black --line-length 119 --target-version py36 datasets/semeval18_emotion_classification/
isort datasets/semeval18_emotion_classification/
flake8 datasets/semeval18_emotion_classification/
```
That's the publication for reference:
Mohammad, S., Bravo-Marquez, F., Salameh, M., & Kiritchenko, S. (2018). SemEval-2018 task 1: Affect in tweets. Proceedings of the 12th International Workshop on Semantic Evaluation, 1β17. https://doi.org/10.18653/v1/S18-1001 | 31 | text: added semeval18_emotion_classification dataset
I added the data set of SemEval 2018 Task 1 (Subtask 5) for emotion detection in three languages.
```
datasets-cli test datasets/semeval18_emotion_classification/ --save_infos --all_configs
RUN_SLOW=1 pytest tests/test_dataset_common.py::LocalDatasetTest::test_load_real_dataset_semeval18_emotion_classification
```
Both commands ran successfully.
I couldn't create the dummy data (the files are tsvs but have .txt ending, maybe that's the problem?) and therefore the test on the dummy data fails, maybe someone can help here.
I also formatted the code:
```
black --line-length 119 --target-version py36 datasets/semeval18_emotion_classification/
isort datasets/semeval18_emotion_classification/
flake8 datasets/semeval18_emotion_classification/
```
That's the publication for reference:
Mohammad, S., Bravo-Marquez, F., Salameh, M., & Kiritchenko, S. (2018). SemEval-2018 task 1: Affect in tweets. Proceedings of the 12th International Workshop on Semantic Evaluation, 1β17. https://doi.org/10.18653/v1/S18-1001
Thanks ! The dummy data looks all good, good job :)
The CI error can be fixed by merging `master` into your branch
```bash
git fetch upstream
git merge upstream/master
``` |
https://github.com/huggingface/datasets/pull/2745 | added semeval18_emotion_classification dataset | Hi! I just added the model card and I did the merge you showed above. Should I then add and commit again? The CI error is still there right now. | I added the data set of SemEval 2018 Task 1 (Subtask 5) for emotion detection in three languages.
```
datasets-cli test datasets/semeval18_emotion_classification/ --save_infos --all_configs
RUN_SLOW=1 pytest tests/test_dataset_common.py::LocalDatasetTest::test_load_real_dataset_semeval18_emotion_classification
```
Both commands ran successfully.
I couldn't create the dummy data (the files are tsvs but have .txt ending, maybe that's the problem?) and therefore the test on the dummy data fails, maybe someone can help here.
I also formatted the code:
```
black --line-length 119 --target-version py36 datasets/semeval18_emotion_classification/
isort datasets/semeval18_emotion_classification/
flake8 datasets/semeval18_emotion_classification/
```
That's the publication for reference:
Mohammad, S., Bravo-Marquez, F., Salameh, M., & Kiritchenko, S. (2018). SemEval-2018 task 1: Affect in tweets. Proceedings of the 12th International Workshop on Semantic Evaluation, 1β17. https://doi.org/10.18653/v1/S18-1001 | 30 | text: added semeval18_emotion_classification dataset
I added the data set of SemEval 2018 Task 1 (Subtask 5) for emotion detection in three languages.
```
datasets-cli test datasets/semeval18_emotion_classification/ --save_infos --all_configs
RUN_SLOW=1 pytest tests/test_dataset_common.py::LocalDatasetTest::test_load_real_dataset_semeval18_emotion_classification
```
Both commands ran successfully.
I couldn't create the dummy data (the files are tsvs but have .txt ending, maybe that's the problem?) and therefore the test on the dummy data fails, maybe someone can help here.
I also formatted the code:
```
black --line-length 119 --target-version py36 datasets/semeval18_emotion_classification/
isort datasets/semeval18_emotion_classification/
flake8 datasets/semeval18_emotion_classification/
```
That's the publication for reference:
Mohammad, S., Bravo-Marquez, F., Salameh, M., & Kiritchenko, S. (2018). SemEval-2018 task 1: Affect in tweets. Proceedings of the 12th International Workshop on Semantic Evaluation, 1β17. https://doi.org/10.18653/v1/S18-1001
Hi! I just added the model card and I did the merge you showed above. Should I then add and commit again? The CI error is still there right now. |
https://github.com/huggingface/datasets/pull/2738 | Sunbird AI Ugandan low resource language dataset | Hi @ak3ra , have you had a chance to take my comments into account ?
Let me know if you have questions or if I can help :) | Multi-way parallel text corpus of 5 key Ugandan languages for the task of machine translation. | 28 | text: Sunbird AI Ugandan low resource language dataset
Multi-way parallel text corpus of 5 key Ugandan languages for the task of machine translation.
Hi @ak3ra , have you had a chance to take my comments into account ?
Let me know if you have questions or if I can help :) |
https://github.com/huggingface/datasets/pull/2731 | Adding to_tf_dataset method | This seems to be working reasonably well in testing, and performance is way better. `tf.py_function` has been dropped for an input generator, but I moved as much of the code as possible outside the generator to allow TF to compile it correctly. I also avoid `tf.RaggedTensor` at all costs, and do the shuffle in the dataset followed by accessing sequential chunks, instead of shuffling an index tensor. The combination of all of these gives us a more flexible data loader as well as a ~20X boost in performance compared to the first solution. | Oh my **god** do not merge this yet, it's just a draft.
I've added a method (via a mixin) to the `arrow_dataset.Dataset` class that automatically converts our Dataset classes to TF Dataset classes ready for training. It hopefully has most of the features we want, including streaming from disk (no need to load the whole dataset in memory!), correct shuffling, variable-length batches to reduce compute, and correct support for unusual padding. It achieves that by calling the tokenizer `pad` method in the middle of a TF compute graph via a very hacky call to `tf.py_function`, which is heretical but seems to work.
A number of issues need to be resolved before it's ready to merge, though:
1) Is a MixIn the right way to do this? Do other classes besides `arrow_dataset.Dataset` need this method too?
2) Needs an argument to support constant-length batches for TPU training - this is easy to add and I'll do it soon.
3) Needs the user to supply the list of columns to drop from the arrow `Dataset`. Is there some automatic way to get the columns we want, or see which columns were added by the tokenizer?
4) Assumes the label column is always present and always called "label" - this is probably not great, but I'm not sure what the 'correct' thing to do here is. | 93 | text: Adding to_tf_dataset method
Oh my **god** do not merge this yet, it's just a draft.
I've added a method (via a mixin) to the `arrow_dataset.Dataset` class that automatically converts our Dataset classes to TF Dataset classes ready for training. It hopefully has most of the features we want, including streaming from disk (no need to load the whole dataset in memory!), correct shuffling, variable-length batches to reduce compute, and correct support for unusual padding. It achieves that by calling the tokenizer `pad` method in the middle of a TF compute graph via a very hacky call to `tf.py_function`, which is heretical but seems to work.
A number of issues need to be resolved before it's ready to merge, though:
1) Is a MixIn the right way to do this? Do other classes besides `arrow_dataset.Dataset` need this method too?
2) Needs an argument to support constant-length batches for TPU training - this is easy to add and I'll do it soon.
3) Needs the user to supply the list of columns to drop from the arrow `Dataset`. Is there some automatic way to get the columns we want, or see which columns were added by the tokenizer?
4) Assumes the label column is always present and always called "label" - this is probably not great, but I'm not sure what the 'correct' thing to do here is.
This seems to be working reasonably well in testing, and performance is way better. `tf.py_function` has been dropped for an input generator, but I moved as much of the code as possible outside the generator to allow TF to compile it correctly. I also avoid `tf.RaggedTensor` at all costs, and do the shuffle in the dataset followed by accessing sequential chunks, instead of shuffling an index tensor. The combination of all of these gives us a more flexible data loader as well as a ~20X boost in performance compared to the first solution. |
https://github.com/huggingface/datasets/pull/2731 | Adding to_tf_dataset method | I made a change to the `TFFormatter` in this PR that will need some changes to the tests, so I wanted to ping @lhoestq and anyone else before I made those changes.
The key problem is that up until now the `TFFormatter` always returns `RaggedTensor`, created using the very slow `tf.ragged.constant` function. This is a big performance penalty, but it's also (imo) surprising for users - `RaggedTensor` handles tensors where one dimension has variable length. This is a good choice for tokenized datasets with variable sequence length, but it's an odd choice when the non-batch dimensions are constant, such as in image datasets, or in datasets where all samples are padded to the same length (e.g. for TPU training).
The change I made was to try to return standard `Tensor` objects instead of `RaggedTensor` when all the samples in the batch had the same shape, and if that was not the case to fall back to fast `RaggedTensor` creation with `tf.ragged.stack`, and only falling back to the very slow `tf.ragged.constant` function as a last resort. I think this will match user expectations in most cases and greatly improve performance, but it's a (very slightly) breaking change, so any feedback is welcome! | Oh my **god** do not merge this yet, it's just a draft.
I've added a method (via a mixin) to the `arrow_dataset.Dataset` class that automatically converts our Dataset classes to TF Dataset classes ready for training. It hopefully has most of the features we want, including streaming from disk (no need to load the whole dataset in memory!), correct shuffling, variable-length batches to reduce compute, and correct support for unusual padding. It achieves that by calling the tokenizer `pad` method in the middle of a TF compute graph via a very hacky call to `tf.py_function`, which is heretical but seems to work.
A number of issues need to be resolved before it's ready to merge, though:
1) Is a MixIn the right way to do this? Do other classes besides `arrow_dataset.Dataset` need this method too?
2) Needs an argument to support constant-length batches for TPU training - this is easy to add and I'll do it soon.
3) Needs the user to supply the list of columns to drop from the arrow `Dataset`. Is there some automatic way to get the columns we want, or see which columns were added by the tokenizer?
4) Assumes the label column is always present and always called "label" - this is probably not great, but I'm not sure what the 'correct' thing to do here is. | 201 | text: Adding to_tf_dataset method
Oh my **god** do not merge this yet, it's just a draft.
I've added a method (via a mixin) to the `arrow_dataset.Dataset` class that automatically converts our Dataset classes to TF Dataset classes ready for training. It hopefully has most of the features we want, including streaming from disk (no need to load the whole dataset in memory!), correct shuffling, variable-length batches to reduce compute, and correct support for unusual padding. It achieves that by calling the tokenizer `pad` method in the middle of a TF compute graph via a very hacky call to `tf.py_function`, which is heretical but seems to work.
A number of issues need to be resolved before it's ready to merge, though:
1) Is a MixIn the right way to do this? Do other classes besides `arrow_dataset.Dataset` need this method too?
2) Needs an argument to support constant-length batches for TPU training - this is easy to add and I'll do it soon.
3) Needs the user to supply the list of columns to drop from the arrow `Dataset`. Is there some automatic way to get the columns we want, or see which columns were added by the tokenizer?
4) Assumes the label column is always present and always called "label" - this is probably not great, but I'm not sure what the 'correct' thing to do here is.
I made a change to the `TFFormatter` in this PR that will need some changes to the tests, so I wanted to ping @lhoestq and anyone else before I made those changes.
The key problem is that up until now the `TFFormatter` always returns `RaggedTensor`, created using the very slow `tf.ragged.constant` function. This is a big performance penalty, but it's also (imo) surprising for users - `RaggedTensor` handles tensors where one dimension has variable length. This is a good choice for tokenized datasets with variable sequence length, but it's an odd choice when the non-batch dimensions are constant, such as in image datasets, or in datasets where all samples are padded to the same length (e.g. for TPU training).
The change I made was to try to return standard `Tensor` objects instead of `RaggedTensor` when all the samples in the batch had the same shape, and if that was not the case to fall back to fast `RaggedTensor` creation with `tf.ragged.stack`, and only falling back to the very slow `tf.ragged.constant` function as a last resort. I think this will match user expectations in most cases and greatly improve performance, but it's a (very slightly) breaking change, so any feedback is welcome! |
https://github.com/huggingface/datasets/pull/2731 | Adding to_tf_dataset method | Also I really can't emphasize enough how slow `tf.ragged.constant` is, it's bad enough to create a data pipeline bottleneck in more or less any training setup:
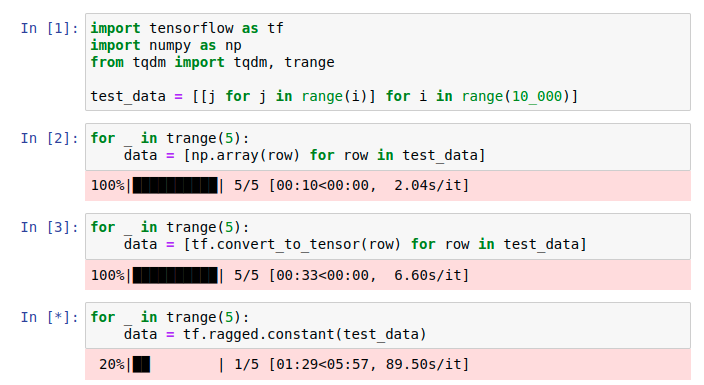
| Oh my **god** do not merge this yet, it's just a draft.
I've added a method (via a mixin) to the `arrow_dataset.Dataset` class that automatically converts our Dataset classes to TF Dataset classes ready for training. It hopefully has most of the features we want, including streaming from disk (no need to load the whole dataset in memory!), correct shuffling, variable-length batches to reduce compute, and correct support for unusual padding. It achieves that by calling the tokenizer `pad` method in the middle of a TF compute graph via a very hacky call to `tf.py_function`, which is heretical but seems to work.
A number of issues need to be resolved before it's ready to merge, though:
1) Is a MixIn the right way to do this? Do other classes besides `arrow_dataset.Dataset` need this method too?
2) Needs an argument to support constant-length batches for TPU training - this is easy to add and I'll do it soon.
3) Needs the user to supply the list of columns to drop from the arrow `Dataset`. Is there some automatic way to get the columns we want, or see which columns were added by the tokenizer?
4) Assumes the label column is always present and always called "label" - this is probably not great, but I'm not sure what the 'correct' thing to do here is. | 27 | text: Adding to_tf_dataset method
Oh my **god** do not merge this yet, it's just a draft.
I've added a method (via a mixin) to the `arrow_dataset.Dataset` class that automatically converts our Dataset classes to TF Dataset classes ready for training. It hopefully has most of the features we want, including streaming from disk (no need to load the whole dataset in memory!), correct shuffling, variable-length batches to reduce compute, and correct support for unusual padding. It achieves that by calling the tokenizer `pad` method in the middle of a TF compute graph via a very hacky call to `tf.py_function`, which is heretical but seems to work.
A number of issues need to be resolved before it's ready to merge, though:
1) Is a MixIn the right way to do this? Do other classes besides `arrow_dataset.Dataset` need this method too?
2) Needs an argument to support constant-length batches for TPU training - this is easy to add and I'll do it soon.
3) Needs the user to supply the list of columns to drop from the arrow `Dataset`. Is there some automatic way to get the columns we want, or see which columns were added by the tokenizer?
4) Assumes the label column is always present and always called "label" - this is probably not great, but I'm not sure what the 'correct' thing to do here is.
Also I really can't emphasize enough how slow `tf.ragged.constant` is, it's bad enough to create a data pipeline bottleneck in more or less any training setup:
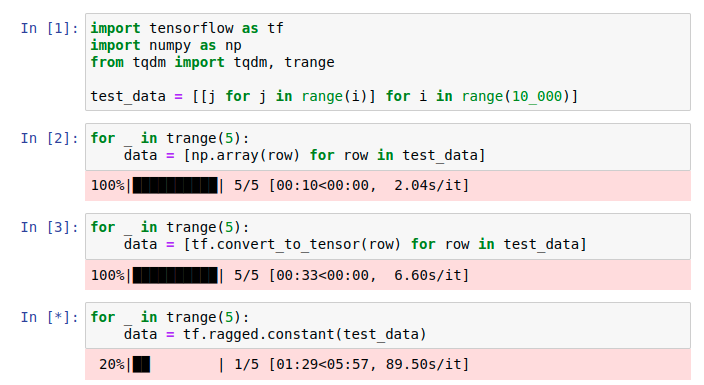
|
https://github.com/huggingface/datasets/pull/2731 | Adding to_tf_dataset method | Hi @lhoestq, the tests have been modified and everything is passing. The Windows tests look to be failing for an unrelated reason, but other than that I'm ready to merge if you are! | Oh my **god** do not merge this yet, it's just a draft.
I've added a method (via a mixin) to the `arrow_dataset.Dataset` class that automatically converts our Dataset classes to TF Dataset classes ready for training. It hopefully has most of the features we want, including streaming from disk (no need to load the whole dataset in memory!), correct shuffling, variable-length batches to reduce compute, and correct support for unusual padding. It achieves that by calling the tokenizer `pad` method in the middle of a TF compute graph via a very hacky call to `tf.py_function`, which is heretical but seems to work.
A number of issues need to be resolved before it's ready to merge, though:
1) Is a MixIn the right way to do this? Do other classes besides `arrow_dataset.Dataset` need this method too?
2) Needs an argument to support constant-length batches for TPU training - this is easy to add and I'll do it soon.
3) Needs the user to supply the list of columns to drop from the arrow `Dataset`. Is there some automatic way to get the columns we want, or see which columns were added by the tokenizer?
4) Assumes the label column is always present and always called "label" - this is probably not great, but I'm not sure what the 'correct' thing to do here is. | 33 | text: Adding to_tf_dataset method
Oh my **god** do not merge this yet, it's just a draft.
I've added a method (via a mixin) to the `arrow_dataset.Dataset` class that automatically converts our Dataset classes to TF Dataset classes ready for training. It hopefully has most of the features we want, including streaming from disk (no need to load the whole dataset in memory!), correct shuffling, variable-length batches to reduce compute, and correct support for unusual padding. It achieves that by calling the tokenizer `pad` method in the middle of a TF compute graph via a very hacky call to `tf.py_function`, which is heretical but seems to work.
A number of issues need to be resolved before it's ready to merge, though:
1) Is a MixIn the right way to do this? Do other classes besides `arrow_dataset.Dataset` need this method too?
2) Needs an argument to support constant-length batches for TPU training - this is easy to add and I'll do it soon.
3) Needs the user to supply the list of columns to drop from the arrow `Dataset`. Is there some automatic way to get the columns we want, or see which columns were added by the tokenizer?
4) Assumes the label column is always present and always called "label" - this is probably not great, but I'm not sure what the 'correct' thing to do here is.
Hi @lhoestq, the tests have been modified and everything is passing. The Windows tests look to be failing for an unrelated reason, but other than that I'm ready to merge if you are! |
https://github.com/huggingface/datasets/pull/2731 | Adding to_tf_dataset method | Hi @Rocketknight1 ! Feel free to merge `master` into this branch to fix and run the full CI :) | Oh my **god** do not merge this yet, it's just a draft.
I've added a method (via a mixin) to the `arrow_dataset.Dataset` class that automatically converts our Dataset classes to TF Dataset classes ready for training. It hopefully has most of the features we want, including streaming from disk (no need to load the whole dataset in memory!), correct shuffling, variable-length batches to reduce compute, and correct support for unusual padding. It achieves that by calling the tokenizer `pad` method in the middle of a TF compute graph via a very hacky call to `tf.py_function`, which is heretical but seems to work.
A number of issues need to be resolved before it's ready to merge, though:
1) Is a MixIn the right way to do this? Do other classes besides `arrow_dataset.Dataset` need this method too?
2) Needs an argument to support constant-length batches for TPU training - this is easy to add and I'll do it soon.
3) Needs the user to supply the list of columns to drop from the arrow `Dataset`. Is there some automatic way to get the columns we want, or see which columns were added by the tokenizer?
4) Assumes the label column is always present and always called "label" - this is probably not great, but I'm not sure what the 'correct' thing to do here is. | 19 | text: Adding to_tf_dataset method
Oh my **god** do not merge this yet, it's just a draft.
I've added a method (via a mixin) to the `arrow_dataset.Dataset` class that automatically converts our Dataset classes to TF Dataset classes ready for training. It hopefully has most of the features we want, including streaming from disk (no need to load the whole dataset in memory!), correct shuffling, variable-length batches to reduce compute, and correct support for unusual padding. It achieves that by calling the tokenizer `pad` method in the middle of a TF compute graph via a very hacky call to `tf.py_function`, which is heretical but seems to work.
A number of issues need to be resolved before it's ready to merge, though:
1) Is a MixIn the right way to do this? Do other classes besides `arrow_dataset.Dataset` need this method too?
2) Needs an argument to support constant-length batches for TPU training - this is easy to add and I'll do it soon.
3) Needs the user to supply the list of columns to drop from the arrow `Dataset`. Is there some automatic way to get the columns we want, or see which columns were added by the tokenizer?
4) Assumes the label column is always present and always called "label" - this is probably not great, but I'm not sure what the 'correct' thing to do here is.
Hi @Rocketknight1 ! Feel free to merge `master` into this branch to fix and run the full CI :) |
https://github.com/huggingface/datasets/pull/2731 | Adding to_tf_dataset method | @lhoestq rebased onto master and it looks good! I'm doing some testing with new notebook examples, but are you happy to merge if that looks good? | Oh my **god** do not merge this yet, it's just a draft.
I've added a method (via a mixin) to the `arrow_dataset.Dataset` class that automatically converts our Dataset classes to TF Dataset classes ready for training. It hopefully has most of the features we want, including streaming from disk (no need to load the whole dataset in memory!), correct shuffling, variable-length batches to reduce compute, and correct support for unusual padding. It achieves that by calling the tokenizer `pad` method in the middle of a TF compute graph via a very hacky call to `tf.py_function`, which is heretical but seems to work.
A number of issues need to be resolved before it's ready to merge, though:
1) Is a MixIn the right way to do this? Do other classes besides `arrow_dataset.Dataset` need this method too?
2) Needs an argument to support constant-length batches for TPU training - this is easy to add and I'll do it soon.
3) Needs the user to supply the list of columns to drop from the arrow `Dataset`. Is there some automatic way to get the columns we want, or see which columns were added by the tokenizer?
4) Assumes the label column is always present and always called "label" - this is probably not great, but I'm not sure what the 'correct' thing to do here is. | 26 | text: Adding to_tf_dataset method
Oh my **god** do not merge this yet, it's just a draft.
I've added a method (via a mixin) to the `arrow_dataset.Dataset` class that automatically converts our Dataset classes to TF Dataset classes ready for training. It hopefully has most of the features we want, including streaming from disk (no need to load the whole dataset in memory!), correct shuffling, variable-length batches to reduce compute, and correct support for unusual padding. It achieves that by calling the tokenizer `pad` method in the middle of a TF compute graph via a very hacky call to `tf.py_function`, which is heretical but seems to work.
A number of issues need to be resolved before it's ready to merge, though:
1) Is a MixIn the right way to do this? Do other classes besides `arrow_dataset.Dataset` need this method too?
2) Needs an argument to support constant-length batches for TPU training - this is easy to add and I'll do it soon.
3) Needs the user to supply the list of columns to drop from the arrow `Dataset`. Is there some automatic way to get the columns we want, or see which columns were added by the tokenizer?
4) Assumes the label column is always present and always called "label" - this is probably not great, but I'm not sure what the 'correct' thing to do here is.
@lhoestq rebased onto master and it looks good! I'm doing some testing with new notebook examples, but are you happy to merge if that looks good? |
https://github.com/huggingface/datasets/pull/2721 | Deal with the bad check in test_load.py | Hi ! I did a change for this test already in #2662 :
https://github.com/huggingface/datasets/blob/00686c46b7aaf6bfcd4102cec300a3c031284a5a/tests/test_load.py#L312-L316
(though I have to change the variable name `m_combined_path` to `m_url` or something)
I guess it's ok to remove this check for now :) | This PR removes a check that's been added in #2684. My intention with this check was to capture an URL in the error message, but instead, it captures a substring of the previous regex match in the test function. Another option would be to replace this check with:
```python
m_paths = re.findall(r"\S*_dummy/_dummy.py\b", str(exc_info.value)) # on Linux this will match an URL as well as a local_path due to different os.sep, so take the last element (an URL always comes last in the list)
assert len(m_paths) > 0 and is_remote_url(m_paths[-1]) # is_remote_url comes from datasets.utils.file_utils
```
@lhoestq Let me know which one of these two approaches (delete or replace) do you prefer? | 38 | text: Deal with the bad check in test_load.py
This PR removes a check that's been added in #2684. My intention with this check was to capture an URL in the error message, but instead, it captures a substring of the previous regex match in the test function. Another option would be to replace this check with:
```python
m_paths = re.findall(r"\S*_dummy/_dummy.py\b", str(exc_info.value)) # on Linux this will match an URL as well as a local_path due to different os.sep, so take the last element (an URL always comes last in the list)
assert len(m_paths) > 0 and is_remote_url(m_paths[-1]) # is_remote_url comes from datasets.utils.file_utils
```
@lhoestq Let me know which one of these two approaches (delete or replace) do you prefer?
Hi ! I did a change for this test already in #2662 :
https://github.com/huggingface/datasets/blob/00686c46b7aaf6bfcd4102cec300a3c031284a5a/tests/test_load.py#L312-L316
(though I have to change the variable name `m_combined_path` to `m_url` or something)
I guess it's ok to remove this check for now :) |
https://github.com/huggingface/datasets/pull/2718 | New documentation structure | I just did some minor changes + added some content in these sections: share, about arrow, about cache
Feel free to mark this PR as ready for review ! :) | Organize Datasets documentation into four documentation types to improve clarity and discoverability of content.
**Content to add in the very short term (feel free to add anything I'm missing):**
- A discussion on why Datasets uses Arrow that includes some context and background about why we use Arrow. Would also be great to talk about Datasets speed and performance here, and if you can share any benchmarking/tests you did, that would be awesome! Finally, a discussion about how memory-mapping frees the user from RAM constraints would be very helpful.
- Explain why you would want to disable or override verifications when loading a dataset.
- If possible, include a code sample of when the number of elements in the field of an output dictionary arenβt the same as the other fields in the output dictionary (taken from the [note](https://huggingface.co/docs/datasets/processing.html#augmenting-the-dataset) here). | 30 | text: New documentation structure
Organize Datasets documentation into four documentation types to improve clarity and discoverability of content.
**Content to add in the very short term (feel free to add anything I'm missing):**
- A discussion on why Datasets uses Arrow that includes some context and background about why we use Arrow. Would also be great to talk about Datasets speed and performance here, and if you can share any benchmarking/tests you did, that would be awesome! Finally, a discussion about how memory-mapping frees the user from RAM constraints would be very helpful.
- Explain why you would want to disable or override verifications when loading a dataset.
- If possible, include a code sample of when the number of elements in the field of an output dictionary arenβt the same as the other fields in the output dictionary (taken from the [note](https://huggingface.co/docs/datasets/processing.html#augmenting-the-dataset) here).
I just did some minor changes + added some content in these sections: share, about arrow, about cache
Feel free to mark this PR as ready for review ! :) |
https://github.com/huggingface/datasets/pull/2718 | New documentation structure | I just separated the `Share` How-to page into three pages: share, dataset_script and dataset_card.
This way in the share page we can explain in more details how to share a community or a canonical dataset - focus in their differences and the steps to upload them.
Also given that making a dataset script or a dataset card both require several steps, I feel like it's better to have dedicated pages for them.
Let me know what you think @stevhliu and others. We can still revert this change if you feel like it was better with everything in the same place. | Organize Datasets documentation into four documentation types to improve clarity and discoverability of content.
**Content to add in the very short term (feel free to add anything I'm missing):**
- A discussion on why Datasets uses Arrow that includes some context and background about why we use Arrow. Would also be great to talk about Datasets speed and performance here, and if you can share any benchmarking/tests you did, that would be awesome! Finally, a discussion about how memory-mapping frees the user from RAM constraints would be very helpful.
- Explain why you would want to disable or override verifications when loading a dataset.
- If possible, include a code sample of when the number of elements in the field of an output dictionary arenβt the same as the other fields in the output dictionary (taken from the [note](https://huggingface.co/docs/datasets/processing.html#augmenting-the-dataset) here). | 100 | text: New documentation structure
Organize Datasets documentation into four documentation types to improve clarity and discoverability of content.
**Content to add in the very short term (feel free to add anything I'm missing):**
- A discussion on why Datasets uses Arrow that includes some context and background about why we use Arrow. Would also be great to talk about Datasets speed and performance here, and if you can share any benchmarking/tests you did, that would be awesome! Finally, a discussion about how memory-mapping frees the user from RAM constraints would be very helpful.
- Explain why you would want to disable or override verifications when loading a dataset.
- If possible, include a code sample of when the number of elements in the field of an output dictionary arenβt the same as the other fields in the output dictionary (taken from the [note](https://huggingface.co/docs/datasets/processing.html#augmenting-the-dataset) here).
I just separated the `Share` How-to page into three pages: share, dataset_script and dataset_card.
This way in the share page we can explain in more details how to share a community or a canonical dataset - focus in their differences and the steps to upload them.
Also given that making a dataset script or a dataset card both require several steps, I feel like it's better to have dedicated pages for them.
Let me know what you think @stevhliu and others. We can still revert this change if you feel like it was better with everything in the same place. |
https://github.com/huggingface/datasets/pull/2718 | New documentation structure | I just added some minor changes to match the style, fix typos, etc. Great work on the conceptual guides, I learned a lot from them and I'm sure they will help a lot of other people too!
I am fine with splitting `Share` into three separate pages. I think this probably makes it easier for users to navigate, instead of having to scroll up and down on a really long single page. | Organize Datasets documentation into four documentation types to improve clarity and discoverability of content.
**Content to add in the very short term (feel free to add anything I'm missing):**
- A discussion on why Datasets uses Arrow that includes some context and background about why we use Arrow. Would also be great to talk about Datasets speed and performance here, and if you can share any benchmarking/tests you did, that would be awesome! Finally, a discussion about how memory-mapping frees the user from RAM constraints would be very helpful.
- Explain why you would want to disable or override verifications when loading a dataset.
- If possible, include a code sample of when the number of elements in the field of an output dictionary arenβt the same as the other fields in the output dictionary (taken from the [note](https://huggingface.co/docs/datasets/processing.html#augmenting-the-dataset) here). | 72 | text: New documentation structure
Organize Datasets documentation into four documentation types to improve clarity and discoverability of content.
**Content to add in the very short term (feel free to add anything I'm missing):**
- A discussion on why Datasets uses Arrow that includes some context and background about why we use Arrow. Would also be great to talk about Datasets speed and performance here, and if you can share any benchmarking/tests you did, that would be awesome! Finally, a discussion about how memory-mapping frees the user from RAM constraints would be very helpful.
- Explain why you would want to disable or override verifications when loading a dataset.
- If possible, include a code sample of when the number of elements in the field of an output dictionary arenβt the same as the other fields in the output dictionary (taken from the [note](https://huggingface.co/docs/datasets/processing.html#augmenting-the-dataset) here).
I just added some minor changes to match the style, fix typos, etc. Great work on the conceptual guides, I learned a lot from them and I'm sure they will help a lot of other people too!
I am fine with splitting `Share` into three separate pages. I think this probably makes it easier for users to navigate, instead of having to scroll up and down on a really long single page. |
https://github.com/huggingface/datasets/pull/2718 | New documentation structure | Thanks a lot for all the suggestions ! I'm doing the final changes based on the remaining comments, then we can merge and release v1.12 of `datasets` and the new documentation ^^ | Organize Datasets documentation into four documentation types to improve clarity and discoverability of content.
**Content to add in the very short term (feel free to add anything I'm missing):**
- A discussion on why Datasets uses Arrow that includes some context and background about why we use Arrow. Would also be great to talk about Datasets speed and performance here, and if you can share any benchmarking/tests you did, that would be awesome! Finally, a discussion about how memory-mapping frees the user from RAM constraints would be very helpful.
- Explain why you would want to disable or override verifications when loading a dataset.
- If possible, include a code sample of when the number of elements in the field of an output dictionary arenβt the same as the other fields in the output dictionary (taken from the [note](https://huggingface.co/docs/datasets/processing.html#augmenting-the-dataset) here). | 32 | text: New documentation structure
Organize Datasets documentation into four documentation types to improve clarity and discoverability of content.
**Content to add in the very short term (feel free to add anything I'm missing):**
- A discussion on why Datasets uses Arrow that includes some context and background about why we use Arrow. Would also be great to talk about Datasets speed and performance here, and if you can share any benchmarking/tests you did, that would be awesome! Finally, a discussion about how memory-mapping frees the user from RAM constraints would be very helpful.
- Explain why you would want to disable or override verifications when loading a dataset.
- If possible, include a code sample of when the number of elements in the field of an output dictionary arenβt the same as the other fields in the output dictionary (taken from the [note](https://huggingface.co/docs/datasets/processing.html#augmenting-the-dataset) here).
Thanks a lot for all the suggestions ! I'm doing the final changes based on the remaining comments, then we can merge and release v1.12 of `datasets` and the new documentation ^^ |
https://github.com/huggingface/datasets/pull/2718 | New documentation structure | Alright I think I took all the suggestions and comments into account :)
Thanks everyone for the help ! | Organize Datasets documentation into four documentation types to improve clarity and discoverability of content.
**Content to add in the very short term (feel free to add anything I'm missing):**
- A discussion on why Datasets uses Arrow that includes some context and background about why we use Arrow. Would also be great to talk about Datasets speed and performance here, and if you can share any benchmarking/tests you did, that would be awesome! Finally, a discussion about how memory-mapping frees the user from RAM constraints would be very helpful.
- Explain why you would want to disable or override verifications when loading a dataset.
- If possible, include a code sample of when the number of elements in the field of an output dictionary arenβt the same as the other fields in the output dictionary (taken from the [note](https://huggingface.co/docs/datasets/processing.html#augmenting-the-dataset) here). | 19 | text: New documentation structure
Organize Datasets documentation into four documentation types to improve clarity and discoverability of content.
**Content to add in the very short term (feel free to add anything I'm missing):**
- A discussion on why Datasets uses Arrow that includes some context and background about why we use Arrow. Would also be great to talk about Datasets speed and performance here, and if you can share any benchmarking/tests you did, that would be awesome! Finally, a discussion about how memory-mapping frees the user from RAM constraints would be very helpful.
- Explain why you would want to disable or override verifications when loading a dataset.
- If possible, include a code sample of when the number of elements in the field of an output dictionary arenβt the same as the other fields in the output dictionary (taken from the [note](https://huggingface.co/docs/datasets/processing.html#augmenting-the-dataset) here).
Alright I think I took all the suggestions and comments into account :)
Thanks everyone for the help ! |
https://github.com/huggingface/datasets/pull/2697 | Fix import on Colab | @lhoestq @albertvillanova - It might be a good idea to have a patch release after this gets merged (presumably tomorrow morning when you're around). The Colab issue linked to this PR is a pretty big blocker. | Fix #2695, fix #2700. | 36 | text: Fix import on Colab
Fix #2695, fix #2700.
@lhoestq @albertvillanova - It might be a good idea to have a patch release after this gets merged (presumably tomorrow morning when you're around). The Colab issue linked to this PR is a pretty big blocker. |
https://github.com/huggingface/datasets/pull/2690 | Docs details | Thanks for all the comments and for the corrections in the docs !
About all the points you mentioned:
> * the code samples assume the expected libraries have already been installed. Maybe add a section at start, or add it to every code sample. Something like `pip install datasets transformers torch 'datasets[streaming]'` (maybe just link to https://huggingface.co/docs/datasets/installation.html + a one-liner that installs all the requirements / alternatively a requirements.txt file)
Yes good idea
> * "If youβd like to play with the examples, you must install it from source." in https://huggingface.co/docs/datasets/installation.html: it's not clear to me what this means (what are these "examples"?)
It refers to examples scripts inside the git repository of the library, see the `examples` folder in the `transformers` repo.
We don't have examples yet in the git repo of `datasets` as in transformers. So currently there are no examples. Maybe we can just remove this sentence from the docs for now
> * in https://huggingface.co/docs/datasets/loading_datasets.html: "or AWS bucket if itβs not already stored in the library". It's the only place in the doc (aside from the docstring https://huggingface.co/docs/datasets/package_reference/loading_methods.html?highlight=aws bucket#datasets.list_datasets) where the "AWS bucket" is mentioned. It's not easy to understand what this means. Maybe explain more, and link to https://s3.amazonaws.com/datasets.huggingface.co and/or https://huggingface.co/docs/datasets/filesystems.html.
This is outdated and must be replaced by
```
or from the Hugging Face Hub if itβs not already stored in the library
```
> * example in https://huggingface.co/docs/datasets/loading_datasets.html#manually-downloading-files is obsoleted by [Enable auto-download for PAN-X / Wikiann domain in XTREMEΒ #2326](https://github.com/huggingface/datasets/pull/2326). Also: see [xtreme / pan-x cannot be downloadedΒ #2691](https://github.com/huggingface/datasets/issues/2691) for a bug on this specific dataset.
We can replace the `XTREME` `PANX` dataste by `matinf` instead for example
> * in https://huggingface.co/docs/datasets/loading_datasets.html#manually-downloading-files the doc says "After youβve downloaded the files, you can point to the folder hosting them locally with the data_dir argument as follows:", but the following example does not show how to use `data_dir`
Let's add `data_dir="path/to/your/downloaded/data"` for example
> * in https://huggingface.co/docs/datasets/loading_datasets.html#csv-files, it would be nice to have an URL to the csv loader reference (but I'm not sure there is one in the API reference). This comment applies in many places in the doc: I would want the API reference to contain doc for all the code/functions/classes... and I would want a lot more links inside the doc pointing to the API entries.
Currently there's no documentation for the CSV loader config. Maybe we can add the docstrings to the `CsvConfig` class to explain the parameters and how it works, and then redirect to the doc of this class in this section of the documentation.
> * in the API reference (docstrings) I would prefer "SOURCE" to link to github instead of a copy of the code inside the docs site (eg. https://github.com/huggingface/datasets/blob/master/src/datasets/load.py#L711 instead of https://huggingface.co/docs/datasets/_modules/datasets/load.html#load_dataset)
This is the same as in `transformers`, not sure if this is a big issue
> * it seems like not all the API is exposed in the doc. For example, there is no doc for [`disable_progress_bar`](https://github.com/huggingface/datasets/search?q=disable_progress_bar), see https://huggingface.co/docs/datasets/search.html?q=disable_progress_bar, even if the code contains docstrings. Does it mean that the function is not officially supported? (otherwise, maybe it also deserves a mention in https://huggingface.co/docs/datasets/package_reference/logging_methods.html)
The function `disable_progress_bar` should definitely be in the docs, thanks. We can add it to the logging methods
> * in https://huggingface.co/docs/datasets/loading_datasets.html?highlight=most%20efficient%20format%20have%20json%20files%20consisting%20multiple%20json%20objects#json-files, "The most efficient format is to have JSON files consisting of multiple JSON objects, one per line, representing individual data rows:", maybe link to https://en.wikipedia.org/wiki/JSON_streaming#Line-delimited_JSON and give it a name ("line-delimited JSON"? "JSON Lines" as in https://huggingface.co/docs/datasets/processing.html#exporting-a-dataset-to-csv-json-parquet-or-to-python-objects ?)
Yes good idea !
> * in https://huggingface.co/docs/datasets/loading_datasets.html, for the local files sections, it would be nice to provide sample csv / json / text files to download, so that it's easier for the reader to try to load them (instead: they won't try)
Sure why not. Moreover the csv loader now supports remote files so you could just run the code pass an an URL to the sample csv file.
> * the doc explains how to shard a dataset, but does not explain why and when a dataset should be sharded (I have no idea... for [parallelizing](https://huggingface.co/docs/datasets/processing.html#multiprocessing)?). It does neither give an idea of the number of shards a dataset typically should have and why.
This can be used for distributed processing or just to use a percentage of the data. We can definitely give example of use cases
> * the code example in https://huggingface.co/docs/datasets/processing.html#mapping-in-a-distributed-setting does not work, because `training_args` has not been defined before in the doc.
`training_args` comes from `transformers`, it's a practical way to define all your arguments to train a model. Maybe we can just import it from `transformers` and use it with the default values
| Some comments here:
- the code samples assume the expected libraries have already been installed. Maybe add a section at start, or add it to every code sample. Something like `pip install datasets transformers torch 'datasets[streaming]'` (maybe just link to https://huggingface.co/docs/datasets/installation.html + a one-liner that installs all the requirements / alternatively a requirements.txt file)
- "If youβd like to play with the examples, you must install it from source." in https://huggingface.co/docs/datasets/installation.html: it's not clear to me what this means (what are these "examples"?)
- in https://huggingface.co/docs/datasets/loading_datasets.html: "or AWS bucket if itβs not already stored in the library". It's the only place in the doc (aside from the docstring https://huggingface.co/docs/datasets/package_reference/loading_methods.html?highlight=aws bucket#datasets.list_datasets) where the "AWS bucket" is mentioned. It's not easy to understand what this means. Maybe explain more, and link to https://s3.amazonaws.com/datasets.huggingface.co and/or https://huggingface.co/docs/datasets/filesystems.html.
- example in https://huggingface.co/docs/datasets/loading_datasets.html#manually-downloading-files is obsoleted by https://github.com/huggingface/datasets/pull/2326. Also: see https://github.com/huggingface/datasets/issues/2691 for a bug on this specific dataset.
- in https://huggingface.co/docs/datasets/loading_datasets.html#manually-downloading-files the doc says "After youβve downloaded the files, you can point to the folder hosting them locally with the data_dir argument as follows:", but the following example does not show how to use `data_dir`
- in https://huggingface.co/docs/datasets/loading_datasets.html#csv-files, it would be nice to have an URL to the csv loader reference (but I'm not sure there is one in the API reference). This comment applies in many places in the doc: I would want the API reference to contain doc for all the code/functions/classes... and I would want a lot more links inside the doc pointing to the API entries.
- in the API reference (docstrings) I would prefer "SOURCE" to link to github instead of a copy of the code inside the docs site (eg. https://github.com/huggingface/datasets/blob/master/src/datasets/load.py#L711 instead of https://huggingface.co/docs/datasets/_modules/datasets/load.html#load_dataset)
- it seems like not all the API is exposed in the doc. For example, there is no doc for [`disable_progress_bar`](https://github.com/huggingface/datasets/search?q=disable_progress_bar), see https://huggingface.co/docs/datasets/search.html?q=disable_progress_bar, even if the code contains docstrings. Does it mean that the function is not officially supported? (otherwise, maybe it also deserves a mention in https://huggingface.co/docs/datasets/package_reference/logging_methods.html)
- in https://huggingface.co/docs/datasets/loading_datasets.html?highlight=most%20efficient%20format%20have%20json%20files%20consisting%20multiple%20json%20objects#json-files, "The most efficient format is to have JSON files consisting of multiple JSON objects, one per line, representing individual data rows:", maybe link to https://en.wikipedia.org/wiki/JSON_streaming#Line-delimited_JSON and give it a name ("line-delimited JSON"? "JSON Lines" as in https://huggingface.co/docs/datasets/processing.html#exporting-a-dataset-to-csv-json-parquet-or-to-python-objects ?)
- in https://huggingface.co/docs/datasets/loading_datasets.html, for the local files sections, it would be nice to provide sample csv / json / text files to download, so that it's easier for the reader to try to load them (instead: they won't try)
- the doc explains how to shard a dataset, but does not explain why and when a dataset should be sharded (I have no idea... for [parallelizing](https://huggingface.co/docs/datasets/processing.html#multiprocessing)?). It does neither give an idea of the number of shards a dataset typically should have and why.
- the code example in https://huggingface.co/docs/datasets/processing.html#mapping-in-a-distributed-setting does not work, because `training_args` has not been defined before in the doc. | 776 | text: Docs details
Some comments here:
- the code samples assume the expected libraries have already been installed. Maybe add a section at start, or add it to every code sample. Something like `pip install datasets transformers torch 'datasets[streaming]'` (maybe just link to https://huggingface.co/docs/datasets/installation.html + a one-liner that installs all the requirements / alternatively a requirements.txt file)
- "If youβd like to play with the examples, you must install it from source." in https://huggingface.co/docs/datasets/installation.html: it's not clear to me what this means (what are these "examples"?)
- in https://huggingface.co/docs/datasets/loading_datasets.html: "or AWS bucket if itβs not already stored in the library". It's the only place in the doc (aside from the docstring https://huggingface.co/docs/datasets/package_reference/loading_methods.html?highlight=aws bucket#datasets.list_datasets) where the "AWS bucket" is mentioned. It's not easy to understand what this means. Maybe explain more, and link to https://s3.amazonaws.com/datasets.huggingface.co and/or https://huggingface.co/docs/datasets/filesystems.html.
- example in https://huggingface.co/docs/datasets/loading_datasets.html#manually-downloading-files is obsoleted by https://github.com/huggingface/datasets/pull/2326. Also: see https://github.com/huggingface/datasets/issues/2691 for a bug on this specific dataset.
- in https://huggingface.co/docs/datasets/loading_datasets.html#manually-downloading-files the doc says "After youβve downloaded the files, you can point to the folder hosting them locally with the data_dir argument as follows:", but the following example does not show how to use `data_dir`
- in https://huggingface.co/docs/datasets/loading_datasets.html#csv-files, it would be nice to have an URL to the csv loader reference (but I'm not sure there is one in the API reference). This comment applies in many places in the doc: I would want the API reference to contain doc for all the code/functions/classes... and I would want a lot more links inside the doc pointing to the API entries.
- in the API reference (docstrings) I would prefer "SOURCE" to link to github instead of a copy of the code inside the docs site (eg. https://github.com/huggingface/datasets/blob/master/src/datasets/load.py#L711 instead of https://huggingface.co/docs/datasets/_modules/datasets/load.html#load_dataset)
- it seems like not all the API is exposed in the doc. For example, there is no doc for [`disable_progress_bar`](https://github.com/huggingface/datasets/search?q=disable_progress_bar), see https://huggingface.co/docs/datasets/search.html?q=disable_progress_bar, even if the code contains docstrings. Does it mean that the function is not officially supported? (otherwise, maybe it also deserves a mention in https://huggingface.co/docs/datasets/package_reference/logging_methods.html)
- in https://huggingface.co/docs/datasets/loading_datasets.html?highlight=most%20efficient%20format%20have%20json%20files%20consisting%20multiple%20json%20objects#json-files, "The most efficient format is to have JSON files consisting of multiple JSON objects, one per line, representing individual data rows:", maybe link to https://en.wikipedia.org/wiki/JSON_streaming#Line-delimited_JSON and give it a name ("line-delimited JSON"? "JSON Lines" as in https://huggingface.co/docs/datasets/processing.html#exporting-a-dataset-to-csv-json-parquet-or-to-python-objects ?)
- in https://huggingface.co/docs/datasets/loading_datasets.html, for the local files sections, it would be nice to provide sample csv / json / text files to download, so that it's easier for the reader to try to load them (instead: they won't try)
- the doc explains how to shard a dataset, but does not explain why and when a dataset should be sharded (I have no idea... for [parallelizing](https://huggingface.co/docs/datasets/processing.html#multiprocessing)?). It does neither give an idea of the number of shards a dataset typically should have and why.
- the code example in https://huggingface.co/docs/datasets/processing.html#mapping-in-a-distributed-setting does not work, because `training_args` has not been defined before in the doc.
Thanks for all the comments and for the corrections in the docs !
About all the points you mentioned:
> * the code samples assume the expected libraries have already been installed. Maybe add a section at start, or add it to every code sample. Something like `pip install datasets transformers torch 'datasets[streaming]'` (maybe just link to https://huggingface.co/docs/datasets/installation.html + a one-liner that installs all the requirements / alternatively a requirements.txt file)
Yes good idea
> * "If youβd like to play with the examples, you must install it from source." in https://huggingface.co/docs/datasets/installation.html: it's not clear to me what this means (what are these "examples"?)
It refers to examples scripts inside the git repository of the library, see the `examples` folder in the `transformers` repo.
We don't have examples yet in the git repo of `datasets` as in transformers. So currently there are no examples. Maybe we can just remove this sentence from the docs for now
> * in https://huggingface.co/docs/datasets/loading_datasets.html: "or AWS bucket if itβs not already stored in the library". It's the only place in the doc (aside from the docstring https://huggingface.co/docs/datasets/package_reference/loading_methods.html?highlight=aws bucket#datasets.list_datasets) where the "AWS bucket" is mentioned. It's not easy to understand what this means. Maybe explain more, and link to https://s3.amazonaws.com/datasets.huggingface.co and/or https://huggingface.co/docs/datasets/filesystems.html.
This is outdated and must be replaced by
```
or from the Hugging Face Hub if itβs not already stored in the library
```
> * example in https://huggingface.co/docs/datasets/loading_datasets.html#manually-downloading-files is obsoleted by [Enable auto-download for PAN-X / Wikiann domain in XTREMEΒ #2326](https://github.com/huggingface/datasets/pull/2326). Also: see [xtreme / pan-x cannot be downloadedΒ #2691](https://github.com/huggingface/datasets/issues/2691) for a bug on this specific dataset.
We can replace the `XTREME` `PANX` dataste by `matinf` instead for example
> * in https://huggingface.co/docs/datasets/loading_datasets.html#manually-downloading-files the doc says "After youβve downloaded the files, you can point to the folder hosting them locally with the data_dir argument as follows:", but the following example does not show how to use `data_dir`
Let's add `data_dir="path/to/your/downloaded/data"` for example
> * in https://huggingface.co/docs/datasets/loading_datasets.html#csv-files, it would be nice to have an URL to the csv loader reference (but I'm not sure there is one in the API reference). This comment applies in many places in the doc: I would want the API reference to contain doc for all the code/functions/classes... and I would want a lot more links inside the doc pointing to the API entries.
Currently there's no documentation for the CSV loader config. Maybe we can add the docstrings to the `CsvConfig` class to explain the parameters and how it works, and then redirect to the doc of this class in this section of the documentation.
> * in the API reference (docstrings) I would prefer "SOURCE" to link to github instead of a copy of the code inside the docs site (eg. https://github.com/huggingface/datasets/blob/master/src/datasets/load.py#L711 instead of https://huggingface.co/docs/datasets/_modules/datasets/load.html#load_dataset)
This is the same as in `transformers`, not sure if this is a big issue
> * it seems like not all the API is exposed in the doc. For example, there is no doc for [`disable_progress_bar`](https://github.com/huggingface/datasets/search?q=disable_progress_bar), see https://huggingface.co/docs/datasets/search.html?q=disable_progress_bar, even if the code contains docstrings. Does it mean that the function is not officially supported? (otherwise, maybe it also deserves a mention in https://huggingface.co/docs/datasets/package_reference/logging_methods.html)
The function `disable_progress_bar` should definitely be in the docs, thanks. We can add it to the logging methods
> * in https://huggingface.co/docs/datasets/loading_datasets.html?highlight=most%20efficient%20format%20have%20json%20files%20consisting%20multiple%20json%20objects#json-files, "The most efficient format is to have JSON files consisting of multiple JSON objects, one per line, representing individual data rows:", maybe link to https://en.wikipedia.org/wiki/JSON_streaming#Line-delimited_JSON and give it a name ("line-delimited JSON"? "JSON Lines" as in https://huggingface.co/docs/datasets/processing.html#exporting-a-dataset-to-csv-json-parquet-or-to-python-objects ?)
Yes good idea !
> * in https://huggingface.co/docs/datasets/loading_datasets.html, for the local files sections, it would be nice to provide sample csv / json / text files to download, so that it's easier for the reader to try to load them (instead: they won't try)
Sure why not. Moreover the csv loader now supports remote files so you could just run the code pass an an URL to the sample csv file.
> * the doc explains how to shard a dataset, but does not explain why and when a dataset should be sharded (I have no idea... for [parallelizing](https://huggingface.co/docs/datasets/processing.html#multiprocessing)?). It does neither give an idea of the number of shards a dataset typically should have and why.
This can be used for distributed processing or just to use a percentage of the data. We can definitely give example of use cases
> * the code example in https://huggingface.co/docs/datasets/processing.html#mapping-in-a-distributed-setting does not work, because `training_args` has not been defined before in the doc.
`training_args` comes from `transformers`, it's a practical way to define all your arguments to train a model. Maybe we can just import it from `transformers` and use it with the default values
|
https://github.com/huggingface/datasets/pull/2685 | Fix Blog Authorship Corpus dataset | That is:
```
=========================== short test summary info ============================
FAILED tests/test_dataset_cards.py::test_changed_dataset_card[blog_authorship_corpus]
==== 1 failed, 3182 passed, 2763 skipped, 16 warnings in 201.23s (0:03:21) =====
``` | This PR:
- Update the JSON metadata file, which previously was raising a `NonMatchingSplitsSizesError`
- Fix the codec of the data files (`latin_1` instead of `utf-8`), which previously was raising ` UnicodeDecodeError` for some files
Close #2679. | 25 | text: Fix Blog Authorship Corpus dataset
This PR:
- Update the JSON metadata file, which previously was raising a `NonMatchingSplitsSizesError`
- Fix the codec of the data files (`latin_1` instead of `utf-8`), which previously was raising ` UnicodeDecodeError` for some files
Close #2679.
That is:
```
=========================== short test summary info ============================
FAILED tests/test_dataset_cards.py::test_changed_dataset_card[blog_authorship_corpus]
==== 1 failed, 3182 passed, 2763 skipped, 16 warnings in 201.23s (0:03:21) =====
``` |
https://github.com/huggingface/datasets/pull/2685 | Fix Blog Authorship Corpus dataset | @lhoestq, apart from the dataset card, everything is OK with this PR: I tested it locally. | This PR:
- Update the JSON metadata file, which previously was raising a `NonMatchingSplitsSizesError`
- Fix the codec of the data files (`latin_1` instead of `utf-8`), which previously was raising ` UnicodeDecodeError` for some files
Close #2679. | 16 | text: Fix Blog Authorship Corpus dataset
This PR:
- Update the JSON metadata file, which previously was raising a `NonMatchingSplitsSizesError`
- Fix the codec of the data files (`latin_1` instead of `utf-8`), which previously was raising ` UnicodeDecodeError` for some files
Close #2679.
@lhoestq, apart from the dataset card, everything is OK with this PR: I tested it locally. |
https://github.com/huggingface/datasets/pull/2668 | Add Russian SuperGLUE | Thanks a lot ! Once the last comment about the label names is addressed we can merge :) | Hi,
This adds the [Russian SuperGLUE](https://russiansuperglue.com/) dataset. For the most part I reused the code for the original SuperGLUE, although there are some relatively minor differences in the structure that I accounted for. | 18 | text: Add Russian SuperGLUE
Hi,
This adds the [Russian SuperGLUE](https://russiansuperglue.com/) dataset. For the most part I reused the code for the original SuperGLUE, although there are some relatively minor differences in the structure that I accounted for.
Thanks a lot ! Once the last comment about the label names is addressed we can merge :) |
https://github.com/huggingface/datasets/pull/2667 | Use tqdm from tqdm_utils | Merging since the CI is only failing because of dataset card issues, which is unrelated to this PR | This PR replaces `tqdm` from the `tqdm` lib with `tqdm` from `datasets.utils.tqdm_utils`. With this change, it's possible to disable progress bars just by calling `disable_progress_bar`. Note this doesn't work on Windows when using multiprocessing due to how global variables are shared between processes. Currently, there is no easy way to disable progress bars in a multiprocess setting on Windows (patching logging with `datasets.utils.logging.get_verbosity = lambda: datasets.utils.logging.NOTSET` doesn't seem to work as well), so adding support for this is a future goal. Additionally, this PR adds a unit ("ba" for batches) to the bar printed by `Dataset.to_json` (this change is motivated by https://github.com/huggingface/datasets/issues/2657). | 18 | text: Use tqdm from tqdm_utils
This PR replaces `tqdm` from the `tqdm` lib with `tqdm` from `datasets.utils.tqdm_utils`. With this change, it's possible to disable progress bars just by calling `disable_progress_bar`. Note this doesn't work on Windows when using multiprocessing due to how global variables are shared between processes. Currently, there is no easy way to disable progress bars in a multiprocess setting on Windows (patching logging with `datasets.utils.logging.get_verbosity = lambda: datasets.utils.logging.NOTSET` doesn't seem to work as well), so adding support for this is a future goal. Additionally, this PR adds a unit ("ba" for batches) to the bar printed by `Dataset.to_json` (this change is motivated by https://github.com/huggingface/datasets/issues/2657).
Merging since the CI is only failing because of dataset card issues, which is unrelated to this PR |
https://github.com/huggingface/datasets/pull/2662 | Load Dataset from the Hub (NO DATASET SCRIPT) | This is ready for review now :)
I would love to have some feedback on the changes in load.py @albertvillanova. There are many changes so if you have questions let me know, especially on the `resolve_data_files` functions and on the changes in `prepare_module`.
And @thomwolf if you want to take a look at the documentation, feel free to share your suggestions :) | ## Load the data from any Dataset repository on the Hub
This PR adds support for loading datasets from any dataset repository on the hub, without requiring any dataset script.
As a user it's now possible to create a repo and upload some csv/json/text/parquet files, and then be able to load the data in one line. Here is an example with the `allenai/c4` repository that contains a lot of compressed json lines files:
```python
from datasets import load_dataset
data_files = {"train": "en/c4-train.*.json.gz"}
c4 = load_dataset("allenai/c4", data_files=data_files, split="train", streaming=True)
print(c4.n_shards)
# 1024
print(next(iter(c4)))
# {'text': 'Beginners BBQ Class Takin...'}
```
By default it loads all the files, but as shown in the example you can choose the ones you want with unix style patterns.
Of course it's still possible to use dataset scripts since they offer the most flexibility.
## Implementation details
It uses `huggingface_hub` to list the files in a dataset repository.
If you provide a path to a local directory instead of a repository name, it works the same way but it uses `glob`.
Depending on the data files available, or passed in the `data_files` parameter, one of the available builders will be used among the csv, json, text and parquet builders.
Because of this, it's not possible to load both csv and json files at once. In this case you have to load them separately and then concatenate the two datasets for example.
## TODO
- [x] tests
- [x] docs
- [x] when huggingface_hub gets a new release, update the CI and the setup.py
Close https://github.com/huggingface/datasets/issues/2629 | 62 | text: Load Dataset from the Hub (NO DATASET SCRIPT)
## Load the data from any Dataset repository on the Hub
This PR adds support for loading datasets from any dataset repository on the hub, without requiring any dataset script.
As a user it's now possible to create a repo and upload some csv/json/text/parquet files, and then be able to load the data in one line. Here is an example with the `allenai/c4` repository that contains a lot of compressed json lines files:
```python
from datasets import load_dataset
data_files = {"train": "en/c4-train.*.json.gz"}
c4 = load_dataset("allenai/c4", data_files=data_files, split="train", streaming=True)
print(c4.n_shards)
# 1024
print(next(iter(c4)))
# {'text': 'Beginners BBQ Class Takin...'}
```
By default it loads all the files, but as shown in the example you can choose the ones you want with unix style patterns.
Of course it's still possible to use dataset scripts since they offer the most flexibility.
## Implementation details
It uses `huggingface_hub` to list the files in a dataset repository.
If you provide a path to a local directory instead of a repository name, it works the same way but it uses `glob`.
Depending on the data files available, or passed in the `data_files` parameter, one of the available builders will be used among the csv, json, text and parquet builders.
Because of this, it's not possible to load both csv and json files at once. In this case you have to load them separately and then concatenate the two datasets for example.
## TODO
- [x] tests
- [x] docs
- [x] when huggingface_hub gets a new release, update the CI and the setup.py
Close https://github.com/huggingface/datasets/issues/2629
This is ready for review now :)
I would love to have some feedback on the changes in load.py @albertvillanova. There are many changes so if you have questions let me know, especially on the `resolve_data_files` functions and on the changes in `prepare_module`.
And @thomwolf if you want to take a look at the documentation, feel free to share your suggestions :) |
https://github.com/huggingface/datasets/pull/2662 | Load Dataset from the Hub (NO DATASET SCRIPT) | I took your comments into account thanks !
And I made `aiohttp` a required dependency :) | ## Load the data from any Dataset repository on the Hub
This PR adds support for loading datasets from any dataset repository on the hub, without requiring any dataset script.
As a user it's now possible to create a repo and upload some csv/json/text/parquet files, and then be able to load the data in one line. Here is an example with the `allenai/c4` repository that contains a lot of compressed json lines files:
```python
from datasets import load_dataset
data_files = {"train": "en/c4-train.*.json.gz"}
c4 = load_dataset("allenai/c4", data_files=data_files, split="train", streaming=True)
print(c4.n_shards)
# 1024
print(next(iter(c4)))
# {'text': 'Beginners BBQ Class Takin...'}
```
By default it loads all the files, but as shown in the example you can choose the ones you want with unix style patterns.
Of course it's still possible to use dataset scripts since they offer the most flexibility.
## Implementation details
It uses `huggingface_hub` to list the files in a dataset repository.
If you provide a path to a local directory instead of a repository name, it works the same way but it uses `glob`.
Depending on the data files available, or passed in the `data_files` parameter, one of the available builders will be used among the csv, json, text and parquet builders.
Because of this, it's not possible to load both csv and json files at once. In this case you have to load them separately and then concatenate the two datasets for example.
## TODO
- [x] tests
- [x] docs
- [x] when huggingface_hub gets a new release, update the CI and the setup.py
Close https://github.com/huggingface/datasets/issues/2629 | 16 | text: Load Dataset from the Hub (NO DATASET SCRIPT)
## Load the data from any Dataset repository on the Hub
This PR adds support for loading datasets from any dataset repository on the hub, without requiring any dataset script.
As a user it's now possible to create a repo and upload some csv/json/text/parquet files, and then be able to load the data in one line. Here is an example with the `allenai/c4` repository that contains a lot of compressed json lines files:
```python
from datasets import load_dataset
data_files = {"train": "en/c4-train.*.json.gz"}
c4 = load_dataset("allenai/c4", data_files=data_files, split="train", streaming=True)
print(c4.n_shards)
# 1024
print(next(iter(c4)))
# {'text': 'Beginners BBQ Class Takin...'}
```
By default it loads all the files, but as shown in the example you can choose the ones you want with unix style patterns.
Of course it's still possible to use dataset scripts since they offer the most flexibility.
## Implementation details
It uses `huggingface_hub` to list the files in a dataset repository.
If you provide a path to a local directory instead of a repository name, it works the same way but it uses `glob`.
Depending on the data files available, or passed in the `data_files` parameter, one of the available builders will be used among the csv, json, text and parquet builders.
Because of this, it's not possible to load both csv and json files at once. In this case you have to load them separately and then concatenate the two datasets for example.
## TODO
- [x] tests
- [x] docs
- [x] when huggingface_hub gets a new release, update the CI and the setup.py
Close https://github.com/huggingface/datasets/issues/2629
I took your comments into account thanks !
And I made `aiohttp` a required dependency :) |
https://github.com/huggingface/datasets/pull/2662 | Load Dataset from the Hub (NO DATASET SCRIPT) | Merging this one :)
We can try to integrate the changes in the docs to #2718 @stevhliu ! | ## Load the data from any Dataset repository on the Hub
This PR adds support for loading datasets from any dataset repository on the hub, without requiring any dataset script.
As a user it's now possible to create a repo and upload some csv/json/text/parquet files, and then be able to load the data in one line. Here is an example with the `allenai/c4` repository that contains a lot of compressed json lines files:
```python
from datasets import load_dataset
data_files = {"train": "en/c4-train.*.json.gz"}
c4 = load_dataset("allenai/c4", data_files=data_files, split="train", streaming=True)
print(c4.n_shards)
# 1024
print(next(iter(c4)))
# {'text': 'Beginners BBQ Class Takin...'}
```
By default it loads all the files, but as shown in the example you can choose the ones you want with unix style patterns.
Of course it's still possible to use dataset scripts since they offer the most flexibility.
## Implementation details
It uses `huggingface_hub` to list the files in a dataset repository.
If you provide a path to a local directory instead of a repository name, it works the same way but it uses `glob`.
Depending on the data files available, or passed in the `data_files` parameter, one of the available builders will be used among the csv, json, text and parquet builders.
Because of this, it's not possible to load both csv and json files at once. In this case you have to load them separately and then concatenate the two datasets for example.
## TODO
- [x] tests
- [x] docs
- [x] when huggingface_hub gets a new release, update the CI and the setup.py
Close https://github.com/huggingface/datasets/issues/2629 | 18 | text: Load Dataset from the Hub (NO DATASET SCRIPT)
## Load the data from any Dataset repository on the Hub
This PR adds support for loading datasets from any dataset repository on the hub, without requiring any dataset script.
As a user it's now possible to create a repo and upload some csv/json/text/parquet files, and then be able to load the data in one line. Here is an example with the `allenai/c4` repository that contains a lot of compressed json lines files:
```python
from datasets import load_dataset
data_files = {"train": "en/c4-train.*.json.gz"}
c4 = load_dataset("allenai/c4", data_files=data_files, split="train", streaming=True)
print(c4.n_shards)
# 1024
print(next(iter(c4)))
# {'text': 'Beginners BBQ Class Takin...'}
```
By default it loads all the files, but as shown in the example you can choose the ones you want with unix style patterns.
Of course it's still possible to use dataset scripts since they offer the most flexibility.
## Implementation details
It uses `huggingface_hub` to list the files in a dataset repository.
If you provide a path to a local directory instead of a repository name, it works the same way but it uses `glob`.
Depending on the data files available, or passed in the `data_files` parameter, one of the available builders will be used among the csv, json, text and parquet builders.
Because of this, it's not possible to load both csv and json files at once. In this case you have to load them separately and then concatenate the two datasets for example.
## TODO
- [x] tests
- [x] docs
- [x] when huggingface_hub gets a new release, update the CI and the setup.py
Close https://github.com/huggingface/datasets/issues/2629
Merging this one :)
We can try to integrate the changes in the docs to #2718 @stevhliu ! |
https://github.com/huggingface/datasets/pull/2662 | Load Dataset from the Hub (NO DATASET SCRIPT) | Baked this into the [docs](https://44335-250213286-gh.circle-artifacts.com/0/docs/_build/html/loading.html#hugging-face-hub) already, let me know if there is anything else I should add! :) | ## Load the data from any Dataset repository on the Hub
This PR adds support for loading datasets from any dataset repository on the hub, without requiring any dataset script.
As a user it's now possible to create a repo and upload some csv/json/text/parquet files, and then be able to load the data in one line. Here is an example with the `allenai/c4` repository that contains a lot of compressed json lines files:
```python
from datasets import load_dataset
data_files = {"train": "en/c4-train.*.json.gz"}
c4 = load_dataset("allenai/c4", data_files=data_files, split="train", streaming=True)
print(c4.n_shards)
# 1024
print(next(iter(c4)))
# {'text': 'Beginners BBQ Class Takin...'}
```
By default it loads all the files, but as shown in the example you can choose the ones you want with unix style patterns.
Of course it's still possible to use dataset scripts since they offer the most flexibility.
## Implementation details
It uses `huggingface_hub` to list the files in a dataset repository.
If you provide a path to a local directory instead of a repository name, it works the same way but it uses `glob`.
Depending on the data files available, or passed in the `data_files` parameter, one of the available builders will be used among the csv, json, text and parquet builders.
Because of this, it's not possible to load both csv and json files at once. In this case you have to load them separately and then concatenate the two datasets for example.
## TODO
- [x] tests
- [x] docs
- [x] when huggingface_hub gets a new release, update the CI and the setup.py
Close https://github.com/huggingface/datasets/issues/2629 | 18 | text: Load Dataset from the Hub (NO DATASET SCRIPT)
## Load the data from any Dataset repository on the Hub
This PR adds support for loading datasets from any dataset repository on the hub, without requiring any dataset script.
As a user it's now possible to create a repo and upload some csv/json/text/parquet files, and then be able to load the data in one line. Here is an example with the `allenai/c4` repository that contains a lot of compressed json lines files:
```python
from datasets import load_dataset
data_files = {"train": "en/c4-train.*.json.gz"}
c4 = load_dataset("allenai/c4", data_files=data_files, split="train", streaming=True)
print(c4.n_shards)
# 1024
print(next(iter(c4)))
# {'text': 'Beginners BBQ Class Takin...'}
```
By default it loads all the files, but as shown in the example you can choose the ones you want with unix style patterns.
Of course it's still possible to use dataset scripts since they offer the most flexibility.
## Implementation details
It uses `huggingface_hub` to list the files in a dataset repository.
If you provide a path to a local directory instead of a repository name, it works the same way but it uses `glob`.
Depending on the data files available, or passed in the `data_files` parameter, one of the available builders will be used among the csv, json, text and parquet builders.
Because of this, it's not possible to load both csv and json files at once. In this case you have to load them separately and then concatenate the two datasets for example.
## TODO
- [x] tests
- [x] docs
- [x] when huggingface_hub gets a new release, update the CI and the setup.py
Close https://github.com/huggingface/datasets/issues/2629
Baked this into the [docs](https://44335-250213286-gh.circle-artifacts.com/0/docs/_build/html/loading.html#hugging-face-hub) already, let me know if there is anything else I should add! :) |
https://github.com/huggingface/datasets/pull/2661 | Add SD task for SUPERB | I make a summary about our discussion with @lewtun and @Narsil on the agreed schema for this dataset and the additional steps required to generate the 2D array labels:
- The labels for this dataset are a 2D array:
Given an example:
```python
{"record_id": record_id, "file": file, "start": start, "end": end, "speakers": [...]}
```
the labels are a 2D array of shape `(num_frames, num_speakers)` where `num_frames = end - start` and `num_speakers = 2`.
- In order to avoid a too large dataset (too large disk space), `datasets` does not store the 2D array label. Instead, we store a compact form:
```
"speakers": [
{"speaker_id": speaker_0_id, "start": start_0_speaker_0, "end": end_0_speaker_0},
{"speaker_id": speaker_0_id, "start": start_1_speaker_0, "end": end_1_speaker_0},
{"speaker_id": speaker_1_id, "start": start_0_speaker_1, "end": end_0_speaker_1},
],
```
- Once loaded the dataset, an additional step is required to generate the 2D array label from this compact form
- This additional step should be a modified version of the s3prl method `_get_labeled_speech`:
- Original s3prl `_get_labeled_speech` includes 2 functionalities: reading the audio file and transforming it into an array, and generating the label 2D array; I think we should separate these 2 functionalities
- Original s3prl `_get_labeled_speech` performs 2 steps to generate the labels:
- Transform start/end seconds (float) into frame numbers (int): I have already done this step to generate the dataset
- Generate the 2D array label from the frame numbers
I also ping @osanseviero and @lhoestq to include them in the loop. | Include the SD (Speaker Diarization) task as described in the [SUPERB paper](https://arxiv.org/abs/2105.01051) and `s3prl` [instructions](https://github.com/s3prl/s3prl/tree/master/s3prl/downstream#sd-speaker-diarization).
TODO:
- [x] Generate the LibriMix corpus
- [x] Prepare the corpus for diarization
- [x] Upload these files to the superb-data repo
- [x] Transcribe the corresponding s3prl processing of these files into our superb loading script
- [x] README: tags + description sections
- ~~Add DER metric~~ (we leave the DER metric for a follow-up PR)
Related to #2619.
Close #2653.
cc: @lewtun | 241 | text: Add SD task for SUPERB
Include the SD (Speaker Diarization) task as described in the [SUPERB paper](https://arxiv.org/abs/2105.01051) and `s3prl` [instructions](https://github.com/s3prl/s3prl/tree/master/s3prl/downstream#sd-speaker-diarization).
TODO:
- [x] Generate the LibriMix corpus
- [x] Prepare the corpus for diarization
- [x] Upload these files to the superb-data repo
- [x] Transcribe the corresponding s3prl processing of these files into our superb loading script
- [x] README: tags + description sections
- ~~Add DER metric~~ (we leave the DER metric for a follow-up PR)
Related to #2619.
Close #2653.
cc: @lewtun
I make a summary about our discussion with @lewtun and @Narsil on the agreed schema for this dataset and the additional steps required to generate the 2D array labels:
- The labels for this dataset are a 2D array:
Given an example:
```python
{"record_id": record_id, "file": file, "start": start, "end": end, "speakers": [...]}
```
the labels are a 2D array of shape `(num_frames, num_speakers)` where `num_frames = end - start` and `num_speakers = 2`.
- In order to avoid a too large dataset (too large disk space), `datasets` does not store the 2D array label. Instead, we store a compact form:
```
"speakers": [
{"speaker_id": speaker_0_id, "start": start_0_speaker_0, "end": end_0_speaker_0},
{"speaker_id": speaker_0_id, "start": start_1_speaker_0, "end": end_1_speaker_0},
{"speaker_id": speaker_1_id, "start": start_0_speaker_1, "end": end_0_speaker_1},
],
```
- Once loaded the dataset, an additional step is required to generate the 2D array label from this compact form
- This additional step should be a modified version of the s3prl method `_get_labeled_speech`:
- Original s3prl `_get_labeled_speech` includes 2 functionalities: reading the audio file and transforming it into an array, and generating the label 2D array; I think we should separate these 2 functionalities
- Original s3prl `_get_labeled_speech` performs 2 steps to generate the labels:
- Transform start/end seconds (float) into frame numbers (int): I have already done this step to generate the dataset
- Generate the 2D array label from the frame numbers
I also ping @osanseviero and @lhoestq to include them in the loop. |
https://github.com/huggingface/datasets/pull/2661 | Add SD task for SUPERB | Here I would like to discuss (and agree) one of the decisions I made, as I'm not completely satisfied with it: to transform the seconds (float) into frame numbers (int) to generate this dataset.
- A priori, the most natural and general choice would be to preserve the seconds (float), because:
- this is the way the raw data comes from
- the transformation into frame numbers depends on the sample rate, frame_shift and subsampling
However, I finally decided to transform seconds into frame numbers because:
- for SUPERB, sampling rate, frame_shift and subsampling are fixed (`rate = 16_000`, `frame_shift = 160`, `subsampling = 1`)
- it makes easier the post-processing, as labels are generated from sample numbers: labels are a 2D array of shape `(num_frames, num_speakers)`
- the number of examples depends on the number of frames:
- if an example has more than 2_000 frames, then it is split into 2 examples. This is the case for `record_id = "7859-102521-0017_3983-5371-0014"`, which has 2_452 frames and it is split into 2 examples:
```
{"record_id": "7859-102521-0017_3983-5371-0014", "start"= 0, "end": 2_000,...},
{"record_id": "7859-102521-0017_3983-5371-0014", "start"= 2_000, "end": 2_452,...},
```
As I told you, I'm not totally convinced of this decision, and I would really appreciate your opinion.
cc: @lewtun @Narsil @osanseviero @lhoestq | Include the SD (Speaker Diarization) task as described in the [SUPERB paper](https://arxiv.org/abs/2105.01051) and `s3prl` [instructions](https://github.com/s3prl/s3prl/tree/master/s3prl/downstream#sd-speaker-diarization).
TODO:
- [x] Generate the LibriMix corpus
- [x] Prepare the corpus for diarization
- [x] Upload these files to the superb-data repo
- [x] Transcribe the corresponding s3prl processing of these files into our superb loading script
- [x] README: tags + description sections
- ~~Add DER metric~~ (we leave the DER metric for a follow-up PR)
Related to #2619.
Close #2653.
cc: @lewtun | 210 | text: Add SD task for SUPERB
Include the SD (Speaker Diarization) task as described in the [SUPERB paper](https://arxiv.org/abs/2105.01051) and `s3prl` [instructions](https://github.com/s3prl/s3prl/tree/master/s3prl/downstream#sd-speaker-diarization).
TODO:
- [x] Generate the LibriMix corpus
- [x] Prepare the corpus for diarization
- [x] Upload these files to the superb-data repo
- [x] Transcribe the corresponding s3prl processing of these files into our superb loading script
- [x] README: tags + description sections
- ~~Add DER metric~~ (we leave the DER metric for a follow-up PR)
Related to #2619.
Close #2653.
cc: @lewtun
Here I would like to discuss (and agree) one of the decisions I made, as I'm not completely satisfied with it: to transform the seconds (float) into frame numbers (int) to generate this dataset.
- A priori, the most natural and general choice would be to preserve the seconds (float), because:
- this is the way the raw data comes from
- the transformation into frame numbers depends on the sample rate, frame_shift and subsampling
However, I finally decided to transform seconds into frame numbers because:
- for SUPERB, sampling rate, frame_shift and subsampling are fixed (`rate = 16_000`, `frame_shift = 160`, `subsampling = 1`)
- it makes easier the post-processing, as labels are generated from sample numbers: labels are a 2D array of shape `(num_frames, num_speakers)`
- the number of examples depends on the number of frames:
- if an example has more than 2_000 frames, then it is split into 2 examples. This is the case for `record_id = "7859-102521-0017_3983-5371-0014"`, which has 2_452 frames and it is split into 2 examples:
```
{"record_id": "7859-102521-0017_3983-5371-0014", "start"= 0, "end": 2_000,...},
{"record_id": "7859-102521-0017_3983-5371-0014", "start"= 2_000, "end": 2_452,...},
```
As I told you, I'm not totally convinced of this decision, and I would really appreciate your opinion.
cc: @lewtun @Narsil @osanseviero @lhoestq |
https://github.com/huggingface/datasets/pull/2661 | Add SD task for SUPERB | It makes total sense to prepare the data to be in a format that can actually be used for model training and evaluation. That's one of the roles of this lib :)
So for me it's ok to use frames as a unit instead of seconds. Just pinging @patrickvonplaten in case he has ever played with such audio tasks and has some advice. For the context: the task is to classify which speaker is speaking, let us know if you are aware of any convenient/standard format for this.
Also I'm not sure why you have to split an example if it's longer that 2,000 frames ? | Include the SD (Speaker Diarization) task as described in the [SUPERB paper](https://arxiv.org/abs/2105.01051) and `s3prl` [instructions](https://github.com/s3prl/s3prl/tree/master/s3prl/downstream#sd-speaker-diarization).
TODO:
- [x] Generate the LibriMix corpus
- [x] Prepare the corpus for diarization
- [x] Upload these files to the superb-data repo
- [x] Transcribe the corresponding s3prl processing of these files into our superb loading script
- [x] README: tags + description sections
- ~~Add DER metric~~ (we leave the DER metric for a follow-up PR)
Related to #2619.
Close #2653.
cc: @lewtun | 106 | text: Add SD task for SUPERB
Include the SD (Speaker Diarization) task as described in the [SUPERB paper](https://arxiv.org/abs/2105.01051) and `s3prl` [instructions](https://github.com/s3prl/s3prl/tree/master/s3prl/downstream#sd-speaker-diarization).
TODO:
- [x] Generate the LibriMix corpus
- [x] Prepare the corpus for diarization
- [x] Upload these files to the superb-data repo
- [x] Transcribe the corresponding s3prl processing of these files into our superb loading script
- [x] README: tags + description sections
- ~~Add DER metric~~ (we leave the DER metric for a follow-up PR)
Related to #2619.
Close #2653.
cc: @lewtun
It makes total sense to prepare the data to be in a format that can actually be used for model training and evaluation. That's one of the roles of this lib :)
So for me it's ok to use frames as a unit instead of seconds. Just pinging @patrickvonplaten in case he has ever played with such audio tasks and has some advice. For the context: the task is to classify which speaker is speaking, let us know if you are aware of any convenient/standard format for this.
Also I'm not sure why you have to split an example if it's longer that 2,000 frames ? |
https://github.com/huggingface/datasets/pull/2661 | Add SD task for SUPERB | > Also I'm not sure why you have to split an example if it's longer that 2,000 frames ?
It is a convention in SUPERB benchmark. | Include the SD (Speaker Diarization) task as described in the [SUPERB paper](https://arxiv.org/abs/2105.01051) and `s3prl` [instructions](https://github.com/s3prl/s3prl/tree/master/s3prl/downstream#sd-speaker-diarization).
TODO:
- [x] Generate the LibriMix corpus
- [x] Prepare the corpus for diarization
- [x] Upload these files to the superb-data repo
- [x] Transcribe the corresponding s3prl processing of these files into our superb loading script
- [x] README: tags + description sections
- ~~Add DER metric~~ (we leave the DER metric for a follow-up PR)
Related to #2619.
Close #2653.
cc: @lewtun | 26 | text: Add SD task for SUPERB
Include the SD (Speaker Diarization) task as described in the [SUPERB paper](https://arxiv.org/abs/2105.01051) and `s3prl` [instructions](https://github.com/s3prl/s3prl/tree/master/s3prl/downstream#sd-speaker-diarization).
TODO:
- [x] Generate the LibriMix corpus
- [x] Prepare the corpus for diarization
- [x] Upload these files to the superb-data repo
- [x] Transcribe the corresponding s3prl processing of these files into our superb loading script
- [x] README: tags + description sections
- ~~Add DER metric~~ (we leave the DER metric for a follow-up PR)
Related to #2619.
Close #2653.
cc: @lewtun
> Also I'm not sure why you have to split an example if it's longer that 2,000 frames ?
It is a convention in SUPERB benchmark. |
https://github.com/huggingface/datasets/pull/2661 | Add SD task for SUPERB | Note that if we agree to leave the dataset as it is now, 2 additional custom functions must be used:
- one to generate the 2D array labels
- one to load the audio file into an array, but taking into account start/end to cut the audio
Is there a way we can give these functions ready to be used? Or should we leave this entirely to the end user? This is not trivial... | Include the SD (Speaker Diarization) task as described in the [SUPERB paper](https://arxiv.org/abs/2105.01051) and `s3prl` [instructions](https://github.com/s3prl/s3prl/tree/master/s3prl/downstream#sd-speaker-diarization).
TODO:
- [x] Generate the LibriMix corpus
- [x] Prepare the corpus for diarization
- [x] Upload these files to the superb-data repo
- [x] Transcribe the corresponding s3prl processing of these files into our superb loading script
- [x] README: tags + description sections
- ~~Add DER metric~~ (we leave the DER metric for a follow-up PR)
Related to #2619.
Close #2653.
cc: @lewtun | 74 | text: Add SD task for SUPERB
Include the SD (Speaker Diarization) task as described in the [SUPERB paper](https://arxiv.org/abs/2105.01051) and `s3prl` [instructions](https://github.com/s3prl/s3prl/tree/master/s3prl/downstream#sd-speaker-diarization).
TODO:
- [x] Generate the LibriMix corpus
- [x] Prepare the corpus for diarization
- [x] Upload these files to the superb-data repo
- [x] Transcribe the corresponding s3prl processing of these files into our superb loading script
- [x] README: tags + description sections
- ~~Add DER metric~~ (we leave the DER metric for a follow-up PR)
Related to #2619.
Close #2653.
cc: @lewtun
Note that if we agree to leave the dataset as it is now, 2 additional custom functions must be used:
- one to generate the 2D array labels
- one to load the audio file into an array, but taking into account start/end to cut the audio
Is there a way we can give these functions ready to be used? Or should we leave this entirely to the end user? This is not trivial... |
https://github.com/huggingface/datasets/pull/2661 | Add SD task for SUPERB | You could add an example of usage in the dataset card, as it is done for other audio datasets | Include the SD (Speaker Diarization) task as described in the [SUPERB paper](https://arxiv.org/abs/2105.01051) and `s3prl` [instructions](https://github.com/s3prl/s3prl/tree/master/s3prl/downstream#sd-speaker-diarization).
TODO:
- [x] Generate the LibriMix corpus
- [x] Prepare the corpus for diarization
- [x] Upload these files to the superb-data repo
- [x] Transcribe the corresponding s3prl processing of these files into our superb loading script
- [x] README: tags + description sections
- ~~Add DER metric~~ (we leave the DER metric for a follow-up PR)
Related to #2619.
Close #2653.
cc: @lewtun | 19 | text: Add SD task for SUPERB
Include the SD (Speaker Diarization) task as described in the [SUPERB paper](https://arxiv.org/abs/2105.01051) and `s3prl` [instructions](https://github.com/s3prl/s3prl/tree/master/s3prl/downstream#sd-speaker-diarization).
TODO:
- [x] Generate the LibriMix corpus
- [x] Prepare the corpus for diarization
- [x] Upload these files to the superb-data repo
- [x] Transcribe the corresponding s3prl processing of these files into our superb loading script
- [x] README: tags + description sections
- ~~Add DER metric~~ (we leave the DER metric for a follow-up PR)
Related to #2619.
Close #2653.
cc: @lewtun
You could add an example of usage in the dataset card, as it is done for other audio datasets |
https://github.com/huggingface/datasets/pull/2661 | Add SD task for SUPERB | Windows ? What is it ? (Not sure not able to test, it's directly calling ffmpeg binary, so depending on the setup it could but can't say for sure without testing)
| Include the SD (Speaker Diarization) task as described in the [SUPERB paper](https://arxiv.org/abs/2105.01051) and `s3prl` [instructions](https://github.com/s3prl/s3prl/tree/master/s3prl/downstream#sd-speaker-diarization).
TODO:
- [x] Generate the LibriMix corpus
- [x] Prepare the corpus for diarization
- [x] Upload these files to the superb-data repo
- [x] Transcribe the corresponding s3prl processing of these files into our superb loading script
- [x] README: tags + description sections
- ~~Add DER metric~~ (we leave the DER metric for a follow-up PR)
Related to #2619.
Close #2653.
cc: @lewtun | 31 | text: Add SD task for SUPERB
Include the SD (Speaker Diarization) task as described in the [SUPERB paper](https://arxiv.org/abs/2105.01051) and `s3prl` [instructions](https://github.com/s3prl/s3prl/tree/master/s3prl/downstream#sd-speaker-diarization).
TODO:
- [x] Generate the LibriMix corpus
- [x] Prepare the corpus for diarization
- [x] Upload these files to the superb-data repo
- [x] Transcribe the corresponding s3prl processing of these files into our superb loading script
- [x] README: tags + description sections
- ~~Add DER metric~~ (we leave the DER metric for a follow-up PR)
Related to #2619.
Close #2653.
cc: @lewtun
Windows ? What is it ? (Not sure not able to test, it's directly calling ffmpeg binary, so depending on the setup it could but can't say for sure without testing)
|
https://github.com/huggingface/datasets/pull/2661 | Add SD task for SUPERB | It's one of the OS we're supposed to support :P (for the better and for the worse) | Include the SD (Speaker Diarization) task as described in the [SUPERB paper](https://arxiv.org/abs/2105.01051) and `s3prl` [instructions](https://github.com/s3prl/s3prl/tree/master/s3prl/downstream#sd-speaker-diarization).
TODO:
- [x] Generate the LibriMix corpus
- [x] Prepare the corpus for diarization
- [x] Upload these files to the superb-data repo
- [x] Transcribe the corresponding s3prl processing of these files into our superb loading script
- [x] README: tags + description sections
- ~~Add DER metric~~ (we leave the DER metric for a follow-up PR)
Related to #2619.
Close #2653.
cc: @lewtun | 17 | text: Add SD task for SUPERB
Include the SD (Speaker Diarization) task as described in the [SUPERB paper](https://arxiv.org/abs/2105.01051) and `s3prl` [instructions](https://github.com/s3prl/s3prl/tree/master/s3prl/downstream#sd-speaker-diarization).
TODO:
- [x] Generate the LibriMix corpus
- [x] Prepare the corpus for diarization
- [x] Upload these files to the superb-data repo
- [x] Transcribe the corresponding s3prl processing of these files into our superb loading script
- [x] README: tags + description sections
- ~~Add DER metric~~ (we leave the DER metric for a follow-up PR)
Related to #2619.
Close #2653.
cc: @lewtun
It's one of the OS we're supposed to support :P (for the better and for the worse) |
https://github.com/huggingface/datasets/pull/2661 | Add SD task for SUPERB | > Note that if we agree to leave the dataset as it is now, 2 additional custom functions must be used:
>
> * one to generate the 2D array labels
> * one to load the audio file into an array, but taking into account start/end to cut the audio
>
> Is there a way we can give these functions ready to be used? Or should we leave this entirely to the end user? This is not trivial...
+1 on providing the necessary functions on the dataset card. aside from that, the current implementation looks great from my perspective! | Include the SD (Speaker Diarization) task as described in the [SUPERB paper](https://arxiv.org/abs/2105.01051) and `s3prl` [instructions](https://github.com/s3prl/s3prl/tree/master/s3prl/downstream#sd-speaker-diarization).
TODO:
- [x] Generate the LibriMix corpus
- [x] Prepare the corpus for diarization
- [x] Upload these files to the superb-data repo
- [x] Transcribe the corresponding s3prl processing of these files into our superb loading script
- [x] README: tags + description sections
- ~~Add DER metric~~ (we leave the DER metric for a follow-up PR)
Related to #2619.
Close #2653.
cc: @lewtun | 101 | text: Add SD task for SUPERB
Include the SD (Speaker Diarization) task as described in the [SUPERB paper](https://arxiv.org/abs/2105.01051) and `s3prl` [instructions](https://github.com/s3prl/s3prl/tree/master/s3prl/downstream#sd-speaker-diarization).
TODO:
- [x] Generate the LibriMix corpus
- [x] Prepare the corpus for diarization
- [x] Upload these files to the superb-data repo
- [x] Transcribe the corresponding s3prl processing of these files into our superb loading script
- [x] README: tags + description sections
- ~~Add DER metric~~ (we leave the DER metric for a follow-up PR)
Related to #2619.
Close #2653.
cc: @lewtun
> Note that if we agree to leave the dataset as it is now, 2 additional custom functions must be used:
>
> * one to generate the 2D array labels
> * one to load the audio file into an array, but taking into account start/end to cut the audio
>
> Is there a way we can give these functions ready to be used? Or should we leave this entirely to the end user? This is not trivial...
+1 on providing the necessary functions on the dataset card. aside from that, the current implementation looks great from my perspective! |
https://github.com/huggingface/datasets/pull/2656 | Change `from_csv` default arguments | This is not the default in pandas right ?
We try to align our CSV loader with the pandas API.
Moreover according to their documentation, the python parser is used when sep is None, which might not be the fastest one.
Maybe users could just specify `sep=None` themselves ?
In this case we should add some documentation about this | Passing `sep=None` to pandas's `read_csv` lets pandas guess the CSV file's separator
This PR allows users to use this pandas's feature by passing `sep=None` to `Dataset.from_csv`:
```python
Dataset.from_csv(
...,
sep=None
)
``` | 59 | text: Change `from_csv` default arguments
Passing `sep=None` to pandas's `read_csv` lets pandas guess the CSV file's separator
This PR allows users to use this pandas's feature by passing `sep=None` to `Dataset.from_csv`:
```python
Dataset.from_csv(
...,
sep=None
)
```
This is not the default in pandas right ?
We try to align our CSV loader with the pandas API.
Moreover according to their documentation, the python parser is used when sep is None, which might not be the fastest one.
Maybe users could just specify `sep=None` themselves ?
In this case we should add some documentation about this |
https://github.com/huggingface/datasets/pull/2638 | Streaming for the Json loader | A note is that I think we should add a few indicator of status (as mentioned by @stas00 in #2649), probably at the (1) downloading, (2) extracting and (3) reading steps. In particular when loading many very large files it's interesting to know a bit where we are in the process. | It was not using `open` in the builder. Therefore `pyarrow.json.read_json` was downloading the full file to start yielding rows.
Moreover, it appeared that `pyarrow.json.read_json` was not really suited for streaming as it was downloading too much data and failing if `block_size` was not properly configured (related to #2573).
So I switched to using `open` which is extended to support reading from remote file progressively, and I removed the pyarrow json reader which was not practical.
Instead, I'm using the classical `json.loads` from the standard library. | 51 | text: Streaming for the Json loader
It was not using `open` in the builder. Therefore `pyarrow.json.read_json` was downloading the full file to start yielding rows.
Moreover, it appeared that `pyarrow.json.read_json` was not really suited for streaming as it was downloading too much data and failing if `block_size` was not properly configured (related to #2573).
So I switched to using `open` which is extended to support reading from remote file progressively, and I removed the pyarrow json reader which was not practical.
Instead, I'm using the classical `json.loads` from the standard library.
A note is that I think we should add a few indicator of status (as mentioned by @stas00 in #2649), probably at the (1) downloading, (2) extracting and (3) reading steps. In particular when loading many very large files it's interesting to know a bit where we are in the process. |
https://github.com/huggingface/datasets/pull/2638 | Streaming for the Json loader | I tested locally, and the builtin `json` loader is 4x slower than `pyarrow.json`. Thanks for the comment @albertvillanova !
Therefore I switched back to using `pyarrow.json`, but only on the batch that is read. This way we don't have to deal with its `block_size`, and it only loads in memory one batch at a time. | It was not using `open` in the builder. Therefore `pyarrow.json.read_json` was downloading the full file to start yielding rows.
Moreover, it appeared that `pyarrow.json.read_json` was not really suited for streaming as it was downloading too much data and failing if `block_size` was not properly configured (related to #2573).
So I switched to using `open` which is extended to support reading from remote file progressively, and I removed the pyarrow json reader which was not practical.
Instead, I'm using the classical `json.loads` from the standard library. | 55 | text: Streaming for the Json loader
It was not using `open` in the builder. Therefore `pyarrow.json.read_json` was downloading the full file to start yielding rows.
Moreover, it appeared that `pyarrow.json.read_json` was not really suited for streaming as it was downloading too much data and failing if `block_size` was not properly configured (related to #2573).
So I switched to using `open` which is extended to support reading from remote file progressively, and I removed the pyarrow json reader which was not practical.
Instead, I'm using the classical `json.loads` from the standard library.
I tested locally, and the builtin `json` loader is 4x slower than `pyarrow.json`. Thanks for the comment @albertvillanova !
Therefore I switched back to using `pyarrow.json`, but only on the batch that is read. This way we don't have to deal with its `block_size`, and it only loads in memory one batch at a time. |
https://github.com/huggingface/datasets/pull/2631 | Delete extracted files when loading dataset | The problem with this approach is that it also deletes the downloaded files (if they need not be extracted). π | Close #2481, close #2604, close #2591.
cc: @stas00, @thomwolf, @BirgerMoell | 20 | text: Delete extracted files when loading dataset
Close #2481, close #2604, close #2591.
cc: @stas00, @thomwolf, @BirgerMoell
The problem with this approach is that it also deletes the downloaded files (if they need not be extracted). π |
https://github.com/huggingface/datasets/pull/2631 | Delete extracted files when loading dataset | > The problem with this approach is that it also deletes the downloaded files (if they need not be extracted). worried
Right! These probably should not be deleted by default, but having an option for those users who are tight on disc space? | Close #2481, close #2604, close #2591.
cc: @stas00, @thomwolf, @BirgerMoell | 43 | text: Delete extracted files when loading dataset
Close #2481, close #2604, close #2591.
cc: @stas00, @thomwolf, @BirgerMoell
> The problem with this approach is that it also deletes the downloaded files (if they need not be extracted). worried
Right! These probably should not be deleted by default, but having an option for those users who are tight on disc space? |
https://github.com/huggingface/datasets/pull/2631 | Delete extracted files when loading dataset | > Right! These probably should not be deleted by default, but having an option for those users who are tight on disc space?
I propose leaving that for another PR, and leave this one handling only with "extracted" files. Is it OK for you? :) | Close #2481, close #2604, close #2591.
cc: @stas00, @thomwolf, @BirgerMoell | 45 | text: Delete extracted files when loading dataset
Close #2481, close #2604, close #2591.
cc: @stas00, @thomwolf, @BirgerMoell
> Right! These probably should not be deleted by default, but having an option for those users who are tight on disc space?
I propose leaving that for another PR, and leave this one handling only with "extracted" files. Is it OK for you? :) |
https://github.com/huggingface/datasets/pull/2631 | Delete extracted files when loading dataset | Awesome thanks !
I just have one question: what about image/audio datasets for which we store the path to the extracted file on the arrow data ?
In this case the default should be to keep the extracted files.
So for now I would just make `keep_extracted=True` by default until we have a way to separate extracted files that can be deleted and extracted files that are actual resources of the dataset. | Close #2481, close #2604, close #2591.
cc: @stas00, @thomwolf, @BirgerMoell | 72 | text: Delete extracted files when loading dataset
Close #2481, close #2604, close #2591.
cc: @stas00, @thomwolf, @BirgerMoell
Awesome thanks !
I just have one question: what about image/audio datasets for which we store the path to the extracted file on the arrow data ?
In this case the default should be to keep the extracted files.
So for now I would just make `keep_extracted=True` by default until we have a way to separate extracted files that can be deleted and extracted files that are actual resources of the dataset. |
https://github.com/huggingface/datasets/pull/2631 | Delete extracted files when loading dataset | @lhoestq, current implementation only deletes extracted "files", not extracted "directories", as it uses: `os.remove(path)`. I'm going to add a filter on files, so that this line does not throw an exception when passed a directory.
For audio datasets, the audio files are inside the extracted "directory", so they are not deleted. | Close #2481, close #2604, close #2591.
cc: @stas00, @thomwolf, @BirgerMoell | 51 | text: Delete extracted files when loading dataset
Close #2481, close #2604, close #2591.
cc: @stas00, @thomwolf, @BirgerMoell
@lhoestq, current implementation only deletes extracted "files", not extracted "directories", as it uses: `os.remove(path)`. I'm going to add a filter on files, so that this line does not throw an exception when passed a directory.
For audio datasets, the audio files are inside the extracted "directory", so they are not deleted. |
https://github.com/huggingface/datasets/pull/2631 | Delete extracted files when loading dataset | I'm still more in favor of having `keep_extracted=True` by default:
- When working with a dataset, you call `load_dataset` many times. By default we want to keep objects extracted to not extract them over and over again (it can take a long time). Then once you know what you're doing and you want to optimize disk space, you can do `keep_extracted=False`. Deleting the extracted files by default is a regression that can lead to slow downs for people calling `load_dataset` many times, which is common when experimenting
- This behavior doesn't sound natural as a default behavior. In the rest of the library, things are cached and not removed unless you explicitly say do (`map` caching for example). Moreover the function in the download manager is called `download_and_extract`, not `download_and_extract_and_remove_extracted_files`
Let me know what you think ! | Close #2481, close #2604, close #2591.
cc: @stas00, @thomwolf, @BirgerMoell | 137 | text: Delete extracted files when loading dataset
Close #2481, close #2604, close #2591.
cc: @stas00, @thomwolf, @BirgerMoell
I'm still more in favor of having `keep_extracted=True` by default:
- When working with a dataset, you call `load_dataset` many times. By default we want to keep objects extracted to not extract them over and over again (it can take a long time). Then once you know what you're doing and you want to optimize disk space, you can do `keep_extracted=False`. Deleting the extracted files by default is a regression that can lead to slow downs for people calling `load_dataset` many times, which is common when experimenting
- This behavior doesn't sound natural as a default behavior. In the rest of the library, things are cached and not removed unless you explicitly say do (`map` caching for example). Moreover the function in the download manager is called `download_and_extract`, not `download_and_extract_and_remove_extracted_files`
Let me know what you think ! |
https://github.com/huggingface/datasets/pull/2631 | Delete extracted files when loading dataset | I think the main issue is that after doing some work users typically move on to other datasets and the amount of disc space used keeps on growing. So your logic is very sound and perhaps what's really needed is a cleansweep function that can go through **all** datasets and clean them up to the desired degree:
- delete all extracted files
- delete all sources
- delete all caches
- delete all caches that haven't been accessed in 6 months
- delete completely old datasets that haven't been accessed in 6 months
- more?
So a user can launch a little application, choose what they want to clean up and voila they have just freed up a huge amount of disc space. Makes me think of Ubuntu Tweak's Janitor app - very useful.
At the moment, this process of linting is very daunting and error-prone, especially due to all those dirs/files with hash names. | Close #2481, close #2604, close #2591.
cc: @stas00, @thomwolf, @BirgerMoell | 155 | text: Delete extracted files when loading dataset
Close #2481, close #2604, close #2591.
cc: @stas00, @thomwolf, @BirgerMoell
I think the main issue is that after doing some work users typically move on to other datasets and the amount of disc space used keeps on growing. So your logic is very sound and perhaps what's really needed is a cleansweep function that can go through **all** datasets and clean them up to the desired degree:
- delete all extracted files
- delete all sources
- delete all caches
- delete all caches that haven't been accessed in 6 months
- delete completely old datasets that haven't been accessed in 6 months
- more?
So a user can launch a little application, choose what they want to clean up and voila they have just freed up a huge amount of disc space. Makes me think of Ubuntu Tweak's Janitor app - very useful.
At the moment, this process of linting is very daunting and error-prone, especially due to all those dirs/files with hash names. |
https://github.com/huggingface/datasets/pull/2631 | Delete extracted files when loading dataset | @stas00 I've had the same idea. Instead of the full-fledged app, a simpler approach would be to add a new command to the CLI. | Close #2481, close #2604, close #2591.
cc: @stas00, @thomwolf, @BirgerMoell | 24 | text: Delete extracted files when loading dataset
Close #2481, close #2604, close #2591.
cc: @stas00, @thomwolf, @BirgerMoell
@stas00 I've had the same idea. Instead of the full-fledged app, a simpler approach would be to add a new command to the CLI. |
https://github.com/huggingface/datasets/pull/2631 | Delete extracted files when loading dataset | oh, CLI would be perfect. I didn't mean to request a GUI-one specifically, was just using it as an example.
One could even do a crontab to delete old datasets that haven't been accesses in X months. | Close #2481, close #2604, close #2591.
cc: @stas00, @thomwolf, @BirgerMoell | 37 | text: Delete extracted files when loading dataset
Close #2481, close #2604, close #2591.
cc: @stas00, @thomwolf, @BirgerMoell
oh, CLI would be perfect. I didn't mean to request a GUI-one specifically, was just using it as an example.
One could even do a crontab to delete old datasets that haven't been accesses in X months. |
https://github.com/huggingface/datasets/pull/2631 | Delete extracted files when loading dataset | @lhoestq I totally agree with you. I'm addressing that change.
@stas00, @mariosasko, that could eventually be addressed in another pull request. The objective of this PR is:
- add an option to pass to `load_dataset`, so that extracted files are deleted
- do this deletion file per file, once the file has been already used to generate the cache Arrow file | Close #2481, close #2604, close #2591.
cc: @stas00, @thomwolf, @BirgerMoell | 61 | text: Delete extracted files when loading dataset
Close #2481, close #2604, close #2591.
cc: @stas00, @thomwolf, @BirgerMoell
@lhoestq I totally agree with you. I'm addressing that change.
@stas00, @mariosasko, that could eventually be addressed in another pull request. The objective of this PR is:
- add an option to pass to `load_dataset`, so that extracted files are deleted
- do this deletion file per file, once the file has been already used to generate the cache Arrow file |
https://github.com/huggingface/datasets/pull/2631 | Delete extracted files when loading dataset | I also like the idea of having a CLI tool to help users clean their cache and save disk space, good idea ! | Close #2481, close #2604, close #2591.
cc: @stas00, @thomwolf, @BirgerMoell | 23 | text: Delete extracted files when loading dataset
Close #2481, close #2604, close #2591.
cc: @stas00, @thomwolf, @BirgerMoell
I also like the idea of having a CLI tool to help users clean their cache and save disk space, good idea ! |
https://github.com/huggingface/datasets/pull/2621 | Use prefix to allow exceed Windows MAX_PATH | > Or maybe we could leave it in case a relative path exceeds the MAX_PATH limit?
What about converting relative paths to absolute? | By using this prefix, you can exceed the Windows MAX_PATH limit.
See: https://docs.microsoft.com/en-us/windows/win32/fileio/naming-a-file?redirectedfrom=MSDN#win32-file-namespaces
Related to #2524, #2220. | 23 | text: Use prefix to allow exceed Windows MAX_PATH
By using this prefix, you can exceed the Windows MAX_PATH limit.
See: https://docs.microsoft.com/en-us/windows/win32/fileio/naming-a-file?redirectedfrom=MSDN#win32-file-namespaces
Related to #2524, #2220.
> Or maybe we could leave it in case a relative path exceeds the MAX_PATH limit?
What about converting relative paths to absolute? |