status
stringclasses 1
value | repo_name
stringclasses 31
values | repo_url
stringclasses 31
values | issue_id
int64 1
104k
| title
stringlengths 4
369
| body
stringlengths 0
254k
⌀ | issue_url
stringlengths 37
56
| pull_url
stringlengths 37
54
| before_fix_sha
stringlengths 40
40
| after_fix_sha
stringlengths 40
40
| report_datetime
unknown | language
stringclasses 5
values | commit_datetime
unknown | updated_file
stringlengths 4
188
| file_content
stringlengths 0
5.12M
|
---|---|---|---|---|---|---|---|---|---|---|---|---|---|---|
closed | langchain-ai/langchain | https://github.com/langchain-ai/langchain | 674 | test_faiss_with_metadatas: key mismatch in assert | https://github.com/hwchase17/langchain/blob/236ae93610a8538d3d0044fc29379c481acc6789/tests/integration_tests/vectorstores/test_faiss.py#L54
This test will fail because `FAISS.from_texts` will assign uuid4s as keys in its docstore, while `expected_docstore` has string numbers as keys. | https://github.com/langchain-ai/langchain/issues/674 | https://github.com/langchain-ai/langchain/pull/676 | e45f7e40e80d9b47fb51853f0c672e747735b951 | e04b063ff40d7f70eaa91f135729071de60b219d | "2023-01-21T16:02:54" | python | "2023-01-22T00:08:14" | langchain/vectorstores/faiss.py | """Wrapper around FAISS vector database."""
from __future__ import annotations
import uuid
from typing import Any, Callable, Dict, Iterable, List, Optional, Tuple
import numpy as np
from langchain.docstore.base import AddableMixin, Docstore
from langchain.docstore.document import Document
from langchain.docstore.in_memory import InMemoryDocstore
from langchain.embeddings.base import Embeddings
from langchain.vectorstores.base import VectorStore
from langchain.vectorstores.utils import maximal_marginal_relevance
class FAISS(VectorStore):
"""Wrapper around FAISS vector database.
To use, you should have the ``faiss`` python package installed.
Example:
.. code-block:: python
from langchain import FAISS
faiss = FAISS(embedding_function, index, docstore)
"""
def __init__(
self,
embedding_function: Callable,
index: Any,
docstore: Docstore,
index_to_docstore_id: Dict[int, str],
):
"""Initialize with necessary components."""
self.embedding_function = embedding_function
self.index = index
self.docstore = docstore
self.index_to_docstore_id = index_to_docstore_id
def add_texts(
self, texts: Iterable[str], metadatas: Optional[List[dict]] = None
) -> List[str]:
"""Run more texts through the embeddings and add to the vectorstore.
Args:
texts: Iterable of strings to add to the vectorstore.
metadatas: Optional list of metadatas associated with the texts.
Returns:
List of ids from adding the texts into the vectorstore.
"""
if not isinstance(self.docstore, AddableMixin):
raise ValueError(
"If trying to add texts, the underlying docstore should support "
f"adding items, which {self.docstore} does not"
)
# Embed and create the documents.
embeddings = [self.embedding_function(text) for text in texts]
documents = []
for i, text in enumerate(texts):
metadata = metadatas[i] if metadatas else {}
documents.append(Document(page_content=text, metadata=metadata))
# Add to the index, the index_to_id mapping, and the docstore.
starting_len = len(self.index_to_docstore_id)
self.index.add(np.array(embeddings, dtype=np.float32))
# Get list of index, id, and docs.
full_info = [
(starting_len + i, str(uuid.uuid4()), doc)
for i, doc in enumerate(documents)
]
# Add information to docstore and index.
self.docstore.add({_id: doc for _, _id, doc in full_info})
index_to_id = {index: _id for index, _id, _ in full_info}
self.index_to_docstore_id.update(index_to_id)
return [_id for _, _id, _ in full_info]
def similarity_search_with_score(
self, query: str, k: int = 4
) -> List[Tuple[Document, float]]:
"""Return docs most similar to query.
Args:
query: Text to look up documents similar to.
k: Number of Documents to return. Defaults to 4.
Returns:
List of Documents most similar to the query and score for each
"""
embedding = self.embedding_function(query)
scores, indices = self.index.search(np.array([embedding], dtype=np.float32), k)
docs = []
for j, i in enumerate(indices[0]):
if i == -1:
# This happens when not enough docs are returned.
continue
_id = self.index_to_docstore_id[i]
doc = self.docstore.search(_id)
if not isinstance(doc, Document):
raise ValueError(f"Could not find document for id {_id}, got {doc}")
docs.append((doc, scores[0][j]))
return docs
def similarity_search(
self, query: str, k: int = 4, **kwargs: Any
) -> List[Document]:
"""Return docs most similar to query.
Args:
query: Text to look up documents similar to.
k: Number of Documents to return. Defaults to 4.
Returns:
List of Documents most similar to the query.
"""
docs_and_scores = self.similarity_search_with_score(query, k)
return [doc for doc, _ in docs_and_scores]
def max_marginal_relevance_search(
self, query: str, k: int = 4, fetch_k: int = 20
) -> List[Document]:
"""Return docs selected using the maximal marginal relevance.
Maximal marginal relevance optimizes for similarity to query AND diversity
among selected documents.
Args:
query: Text to look up documents similar to.
k: Number of Documents to return. Defaults to 4.
fetch_k: Number of Documents to fetch to pass to MMR algorithm.
Returns:
List of Documents selected by maximal marginal relevance.
"""
embedding = self.embedding_function(query)
_, indices = self.index.search(np.array([embedding], dtype=np.float32), fetch_k)
# -1 happens when not enough docs are returned.
embeddings = [self.index.reconstruct(int(i)) for i in indices[0] if i != -1]
mmr_selected = maximal_marginal_relevance(embedding, embeddings, k=k)
selected_indices = [indices[0][i] for i in mmr_selected]
docs = []
for i in selected_indices:
_id = self.index_to_docstore_id[i]
doc = self.docstore.search(_id)
if not isinstance(doc, Document):
raise ValueError(f"Could not find document for id {_id}, got {doc}")
docs.append(doc)
return docs
@classmethod
def from_texts(
cls,
texts: List[str],
embedding: Embeddings,
metadatas: Optional[List[dict]] = None,
**kwargs: Any,
) -> FAISS:
"""Construct FAISS wrapper from raw documents.
This is a user friendly interface that:
1. Embeds documents.
2. Creates an in memory docstore
3. Initializes the FAISS database
This is intended to be a quick way to get started.
Example:
.. code-block:: python
from langchain import FAISS
from langchain.embeddings import OpenAIEmbeddings
embeddings = OpenAIEmbeddings()
faiss = FAISS.from_texts(texts, embeddings)
"""
try:
import faiss
except ImportError:
raise ValueError(
"Could not import faiss python package. "
"Please it install it with `pip install faiss` "
"or `pip install faiss-cpu` (depending on Python version)."
)
embeddings = embedding.embed_documents(texts)
index = faiss.IndexFlatL2(len(embeddings[0]))
index.add(np.array(embeddings, dtype=np.float32))
documents = []
for i, text in enumerate(texts):
metadata = metadatas[i] if metadatas else {}
documents.append(Document(page_content=text, metadata=metadata))
index_to_id = {i: str(uuid.uuid4()) for i in range(len(documents))}
docstore = InMemoryDocstore(
{index_to_id[i]: doc for i, doc in enumerate(documents)}
)
return cls(embedding.embed_query, index, docstore, index_to_id)
|
closed | langchain-ai/langchain | https://github.com/langchain-ai/langchain | 674 | test_faiss_with_metadatas: key mismatch in assert | https://github.com/hwchase17/langchain/blob/236ae93610a8538d3d0044fc29379c481acc6789/tests/integration_tests/vectorstores/test_faiss.py#L54
This test will fail because `FAISS.from_texts` will assign uuid4s as keys in its docstore, while `expected_docstore` has string numbers as keys. | https://github.com/langchain-ai/langchain/issues/674 | https://github.com/langchain-ai/langchain/pull/676 | e45f7e40e80d9b47fb51853f0c672e747735b951 | e04b063ff40d7f70eaa91f135729071de60b219d | "2023-01-21T16:02:54" | python | "2023-01-22T00:08:14" | tests/integration_tests/vectorstores/test_faiss.py | """Test FAISS functionality."""
from typing import List
import pytest
from langchain.docstore.document import Document
from langchain.docstore.in_memory import InMemoryDocstore
from langchain.docstore.wikipedia import Wikipedia
from langchain.embeddings.base import Embeddings
from langchain.vectorstores.faiss import FAISS
class FakeEmbeddings(Embeddings):
"""Fake embeddings functionality for testing."""
def embed_documents(self, texts: List[str]) -> List[List[float]]:
"""Return simple embeddings."""
return [[i] * 10 for i in range(len(texts))]
def embed_query(self, text: str) -> List[float]:
"""Return simple embeddings."""
return [0] * 10
def test_faiss() -> None:
"""Test end to end construction and search."""
texts = ["foo", "bar", "baz"]
docsearch = FAISS.from_texts(texts, FakeEmbeddings())
index_to_id = docsearch.index_to_docstore_id
expected_docstore = InMemoryDocstore(
{
index_to_id[0]: Document(page_content="foo"),
index_to_id[1]: Document(page_content="bar"),
index_to_id[2]: Document(page_content="baz"),
}
)
assert docsearch.docstore.__dict__ == expected_docstore.__dict__
output = docsearch.similarity_search("foo", k=1)
assert output == [Document(page_content="foo")]
def test_faiss_with_metadatas() -> None:
"""Test end to end construction and search."""
texts = ["foo", "bar", "baz"]
metadatas = [{"page": i} for i in range(len(texts))]
docsearch = FAISS.from_texts(texts, FakeEmbeddings(), metadatas=metadatas)
expected_docstore = InMemoryDocstore(
{
"0": Document(page_content="foo", metadata={"page": 0}),
"1": Document(page_content="bar", metadata={"page": 1}),
"2": Document(page_content="baz", metadata={"page": 2}),
}
)
assert docsearch.docstore.__dict__ == expected_docstore.__dict__
output = docsearch.similarity_search("foo", k=1)
assert output == [Document(page_content="foo", metadata={"page": 0})]
def test_faiss_search_not_found() -> None:
"""Test what happens when document is not found."""
texts = ["foo", "bar", "baz"]
docsearch = FAISS.from_texts(texts, FakeEmbeddings())
# Get rid of the docstore to purposefully induce errors.
docsearch.docstore = InMemoryDocstore({})
with pytest.raises(ValueError):
docsearch.similarity_search("foo")
def test_faiss_add_texts() -> None:
"""Test end to end adding of texts."""
# Create initial doc store.
texts = ["foo", "bar", "baz"]
docsearch = FAISS.from_texts(texts, FakeEmbeddings())
# Test adding a similar document as before.
docsearch.add_texts(["foo"])
output = docsearch.similarity_search("foo", k=2)
assert output == [Document(page_content="foo"), Document(page_content="foo")]
def test_faiss_add_texts_not_supported() -> None:
"""Test adding of texts to a docstore that doesn't support it."""
docsearch = FAISS(FakeEmbeddings().embed_query, None, Wikipedia(), {})
with pytest.raises(ValueError):
docsearch.add_texts(["foo"])
|
closed | langchain-ai/langchain | https://github.com/langchain-ai/langchain | 897 | Pinecone in docs is outdated | Pinecone default environment was recently changed from `us-west1-gcp` to `us-east1-gcp` ([see here](https://docs.pinecone.io/docs/projects#project-environment)), so new users following the [docs here](https://langchain.readthedocs.io/en/latest/modules/utils/combine_docs_examples/vectorstores.html#pinecone) will hit an error when initializing.
Submitted #898 | https://github.com/langchain-ai/langchain/issues/897 | https://github.com/langchain-ai/langchain/pull/898 | 7658263bfbc9485ebbc85b7d4c2476ea68611e26 | 8217a2f26c94234a1ea99d1b9b815e4da577dcfe | "2023-02-05T18:33:50" | python | "2023-02-05T23:21:56" | docs/modules/utils/combine_docs_examples/vectorstores.ipynb | {
"cells": [
{
"cell_type": "markdown",
"id": "7ef4d402-6662-4a26-b612-35b542066487",
"metadata": {
"pycharm": {
"name": "#%% md\n"
}
},
"source": [
"# VectorStores\n",
"\n",
"This notebook show cases how to use VectorStores. A key part of working with vectorstores is creating the vector to put in them, which is usually created via embeddings. Therefor, it is recommended that you familiarize yourself with the [embedding notebook](embeddings.ipynb) before diving into this."
]
},
{
"cell_type": "code",
"execution_count": 3,
"id": "965eecee",
"metadata": {
"pycharm": {
"name": "#%%\n"
}
},
"outputs": [],
"source": [
"from langchain.embeddings.openai import OpenAIEmbeddings\n",
"from langchain.text_splitter import CharacterTextSplitter\n",
"from langchain.vectorstores import ElasticVectorSearch, Pinecone, Weaviate, FAISS, Qdrant"
]
},
{
"cell_type": "code",
"execution_count": 4,
"id": "68481687",
"metadata": {
"pycharm": {
"name": "#%%\n"
}
},
"outputs": [],
"source": [
"with open('../../state_of_the_union.txt') as f:\n",
" state_of_the_union = f.read()\n",
"text_splitter = CharacterTextSplitter(chunk_size=1000, chunk_overlap=0)\n",
"texts = text_splitter.split_text(state_of_the_union)\n",
"\n",
"embeddings = OpenAIEmbeddings()"
]
},
{
"cell_type": "code",
"execution_count": 5,
"id": "015f4ff5",
"metadata": {
"pycharm": {
"name": "#%%\n"
}
},
"outputs": [],
"source": [
"docsearch = FAISS.from_texts(texts, embeddings)\n",
"\n",
"query = \"What did the president say about Ketanji Brown Jackson\"\n",
"docs = docsearch.similarity_search(query)"
]
},
{
"cell_type": "code",
"execution_count": 9,
"id": "67baf32e",
"metadata": {
"pycharm": {
"name": "#%%\n"
}
},
"outputs": [
{
"name": "stdout",
"output_type": "stream",
"text": [
"In state after state, new laws have been passed, not only to suppress the vote, but to subvert entire elections. \n",
"\n",
"We cannot let this happen. \n",
"\n",
"Tonight. I call on the Senate to: Pass the Freedom to Vote Act. Pass the John Lewis Voting Rights Act. And while you’re at it, pass the Disclose Act so Americans can know who is funding our elections. \n",
"\n",
"Tonight, I’d like to honor someone who has dedicated his life to serve this country: Justice Stephen Breyer—an Army veteran, Constitutional scholar, and retiring Justice of the United States Supreme Court. Justice Breyer, thank you for your service. \n",
"\n",
"One of the most serious constitutional responsibilities a President has is nominating someone to serve on the United States Supreme Court. \n",
"\n",
"And I did that 4 days ago, when I nominated Circuit Court of Appeals Judge Ketanji Brown Jackson. One of our nation’s top legal minds, who will continue Justice Breyer’s legacy of excellence.\n"
]
}
],
"source": [
"print(docs[0].page_content)"
]
},
{
"cell_type": "markdown",
"id": "bbf5ec44",
"metadata": {},
"source": [
"## From Documents\n",
"We can also initialize a vectorstore from documents directly. This is useful when we use the method on the text splitter to get documents directly (handy when the original documents have associated metadata)."
]
},
{
"cell_type": "code",
"execution_count": 5,
"id": "df4a459c",
"metadata": {},
"outputs": [],
"source": [
"documents = text_splitter.create_documents([state_of_the_union], metadatas=[{\"source\": \"State of the Union\"}])"
]
},
{
"cell_type": "code",
"execution_count": 6,
"id": "4b480245",
"metadata": {},
"outputs": [],
"source": [
"docsearch = FAISS.from_documents(documents, embeddings)\n",
"\n",
"query = \"What did the president say about Ketanji Brown Jackson\"\n",
"docs = docsearch.similarity_search(query)"
]
},
{
"cell_type": "code",
"execution_count": 7,
"id": "86aa4cda",
"metadata": {},
"outputs": [
{
"name": "stdout",
"output_type": "stream",
"text": [
"In state after state, new laws have been passed, not only to suppress the vote, but to subvert entire elections. \n",
"\n",
"We cannot let this happen. \n",
"\n",
"Tonight. I call on the Senate to: Pass the Freedom to Vote Act. Pass the John Lewis Voting Rights Act. And while you’re at it, pass the Disclose Act so Americans can know who is funding our elections. \n",
"\n",
"Tonight, I’d like to honor someone who has dedicated his life to serve this country: Justice Stephen Breyer—an Army veteran, Constitutional scholar, and retiring Justice of the United States Supreme Court. Justice Breyer, thank you for your service. \n",
"\n",
"One of the most serious constitutional responsibilities a President has is nominating someone to serve on the United States Supreme Court. \n",
"\n",
"And I did that 4 days ago, when I nominated Circuit Court of Appeals Judge Ketanji Brown Jackson. One of our nation’s top legal minds, who will continue Justice Breyer’s legacy of excellence. \n"
]
}
],
"source": [
"print(docs[0].page_content)"
]
},
{
"cell_type": "markdown",
"id": "2445a5e6",
"metadata": {},
"source": [
"## FAISS-specific\n",
"There are some FAISS specific methods. One of them is `similarity_search_with_score`, which allows you to return not only the documents but also the similarity score of the query to them."
]
},
{
"cell_type": "code",
"execution_count": 4,
"id": "b4f49314",
"metadata": {},
"outputs": [],
"source": [
"docs_and_scores = docsearch.similarity_search_with_score(query)"
]
},
{
"cell_type": "code",
"execution_count": 5,
"id": "86f78ab1",
"metadata": {},
"outputs": [
{
"data": {
"text/plain": [
"(Document(page_content='In state after state, new laws have been passed, not only to suppress the vote, but to subvert entire elections. \\n\\nWe cannot let this happen. \\n\\nTonight. I call on the Senate to: Pass the Freedom to Vote Act. Pass the John Lewis Voting Rights Act. And while you’re at it, pass the Disclose Act so Americans can know who is funding our elections. \\n\\nTonight, I’d like to honor someone who has dedicated his life to serve this country: Justice Stephen Breyer—an Army veteran, Constitutional scholar, and retiring Justice of the United States Supreme Court. Justice Breyer, thank you for your service. \\n\\nOne of the most serious constitutional responsibilities a President has is nominating someone to serve on the United States Supreme Court. \\n\\nAnd I did that 4 days ago, when I nominated Circuit Court of Appeals Judge Ketanji Brown Jackson. One of our nation’s top legal minds, who will continue Justice Breyer’s legacy of excellence.', lookup_str='', metadata={}, lookup_index=0),\n",
" 0.40834612)"
]
},
"execution_count": 5,
"metadata": {},
"output_type": "execute_result"
}
],
"source": [
"docs_and_scores[0]"
]
},
{
"cell_type": "markdown",
"id": "b386dbb8",
"metadata": {},
"source": [
"### Saving and loading\n",
"You can also save and load a FAISS index. This is useful so you don't have to recreate it everytime you use it."
]
},
{
"cell_type": "code",
"execution_count": 12,
"id": "b58b3955",
"metadata": {},
"outputs": [],
"source": [
"import pickle"
]
},
{
"cell_type": "code",
"execution_count": 14,
"id": "1897e23d",
"metadata": {},
"outputs": [],
"source": [
"with open(\"foo.pkl\", 'wb') as f:\n",
" pickle.dump(docsearch, f)"
]
},
{
"cell_type": "code",
"execution_count": 15,
"id": "bf3732f1",
"metadata": {},
"outputs": [],
"source": [
"with open(\"foo.pkl\", 'rb') as f:\n",
" new_docsearch = pickle.load(f)"
]
},
{
"cell_type": "code",
"execution_count": 16,
"id": "5bf2ee24",
"metadata": {},
"outputs": [],
"source": [
"docs = new_docsearch.similarity_search(query)"
]
},
{
"cell_type": "code",
"execution_count": 18,
"id": "edc2aad1",
"metadata": {},
"outputs": [
{
"data": {
"text/plain": [
"Document(page_content='In state after state, new laws have been passed, not only to suppress the vote, but to subvert entire elections. \\n\\nWe cannot let this happen. \\n\\nTonight. I call on the Senate to: Pass the Freedom to Vote Act. Pass the John Lewis Voting Rights Act. And while you’re at it, pass the Disclose Act so Americans can know who is funding our elections. \\n\\nTonight, I’d like to honor someone who has dedicated his life to serve this country: Justice Stephen Breyer—an Army veteran, Constitutional scholar, and retiring Justice of the United States Supreme Court. Justice Breyer, thank you for your service. \\n\\nOne of the most serious constitutional responsibilities a President has is nominating someone to serve on the United States Supreme Court. \\n\\nAnd I did that 4 days ago, when I nominated Circuit Court of Appeals Judge Ketanji Brown Jackson. One of our nation’s top legal minds, who will continue Justice Breyer’s legacy of excellence.', lookup_str='', metadata={}, lookup_index=0)"
]
},
"execution_count": 18,
"metadata": {},
"output_type": "execute_result"
}
],
"source": [
"docs[0]"
]
},
{
"cell_type": "markdown",
"id": "eea6e627",
"metadata": {},
"source": [
"## Requires having ElasticSearch setup"
]
},
{
"cell_type": "code",
"execution_count": 5,
"id": "4906b8a3",
"metadata": {
"pycharm": {
"name": "#%%\n"
}
},
"outputs": [],
"source": [
"docsearch = ElasticVectorSearch.from_texts(texts, embeddings, elasticsearch_url=\"http://localhost:9200\")\n",
"\n",
"query = \"What did the president say about Ketanji Brown Jackson\"\n",
"docs = docsearch.similarity_search(query)"
]
},
{
"cell_type": "code",
"execution_count": 6,
"id": "95f9eee9",
"metadata": {
"pycharm": {
"name": "#%%\n"
}
},
"outputs": [
{
"name": "stdout",
"output_type": "stream",
"text": [
"Tonight, I’d like to honor someone who has dedicated his life to serve this country: Justice Stephen Breyer—an Army veteran, Constitutional scholar, and retiring Justice of the United States Supreme Court. Justice Breyer, thank you for your service. \n",
"\n",
"One of the most serious constitutional responsibilities a President has is nominating someone to serve on the United States Supreme Court. \n",
"\n",
"And I did that 4 days ago, when I nominated Circuit Court of Appeals Judge Ketanji Brown Jackson. One of our nation’s top legal minds, who will continue Justice Breyer’s legacy of excellence. \n",
"\n",
"A former top litigator in private practice. A former federal public defender. And from a family of public school educators and police officers. A consensus builder. Since she’s been nominated, she’s received a broad range of support—from the Fraternal Order of Police to former judges appointed by Democrats and Republicans. \n",
"\n",
"And if we are to advance liberty and justice, we need to secure the Border and fix the immigration system. \n"
]
}
],
"source": [
"print(docs[0].page_content)"
]
},
{
"cell_type": "markdown",
"id": "7f9cb9e7",
"metadata": {},
"source": [
"## Weaviate"
]
},
{
"cell_type": "code",
"execution_count": 11,
"id": "1037a85e",
"metadata": {},
"outputs": [],
"source": [
"import weaviate\n",
"import os\n",
"\n",
"WEAVIATE_URL = \"\"\n",
"client = weaviate.Client(\n",
" url=WEAVIATE_URL,\n",
" additional_headers={\n",
" 'X-OpenAI-Api-Key': os.environ[\"OPENAI_API_KEY\"]\n",
" }\n",
")"
]
},
{
"cell_type": "code",
"execution_count": 12,
"id": "b9043766",
"metadata": {},
"outputs": [],
"source": [
"client.schema.delete_all()\n",
"client.schema.get()\n",
"schema = {\n",
" \"classes\": [\n",
" {\n",
" \"class\": \"Paragraph\",\n",
" \"description\": \"A written paragraph\",\n",
" \"vectorizer\": \"text2vec-openai\",\n",
" \"moduleConfig\": {\n",
" \"text2vec-openai\": {\n",
" \"model\": \"babbage\",\n",
" \"type\": \"text\"\n",
" }\n",
" },\n",
" \"properties\": [\n",
" {\n",
" \"dataType\": [\"text\"],\n",
" \"description\": \"The content of the paragraph\",\n",
" \"moduleConfig\": {\n",
" \"text2vec-openai\": {\n",
" \"skip\": False,\n",
" \"vectorizePropertyName\": False\n",
" }\n",
" },\n",
" \"name\": \"content\",\n",
" },\n",
" ],\n",
" },\n",
" ]\n",
"}\n",
"\n",
"client.schema.create(schema)"
]
},
{
"cell_type": "code",
"execution_count": 13,
"id": "ac20d99c",
"metadata": {},
"outputs": [],
"source": [
"with client.batch as batch:\n",
" for text in texts:\n",
" batch.add_data_object({\"content\": text}, \"Paragraph\")"
]
},
{
"cell_type": "code",
"execution_count": 14,
"id": "01645d61",
"metadata": {},
"outputs": [],
"source": [
"from langchain.vectorstores.weaviate import Weaviate"
]
},
{
"cell_type": "code",
"execution_count": 15,
"id": "bdd97d29",
"metadata": {},
"outputs": [],
"source": [
"vectorstore = Weaviate(client, \"Paragraph\", \"content\")"
]
},
{
"cell_type": "code",
"execution_count": 16,
"id": "b70c0f98",
"metadata": {},
"outputs": [],
"source": [
"query = \"What did the president say about Ketanji Brown Jackson\"\n",
"docs = vectorstore.similarity_search(query)"
]
},
{
"cell_type": "code",
"execution_count": 17,
"id": "07533e40",
"metadata": {},
"outputs": [
{
"name": "stdout",
"output_type": "stream",
"text": [
"In state after state, new laws have been passed, not only to suppress the vote, but to subvert entire elections. \n",
"\n",
"We cannot let this happen. \n",
"\n",
"Tonight. I call on the Senate to: Pass the Freedom to Vote Act. Pass the John Lewis Voting Rights Act. And while you’re at it, pass the Disclose Act so Americans can know who is funding our elections. \n",
"\n",
"Tonight, I’d like to honor someone who has dedicated his life to serve this country: Justice Stephen Breyer—an Army veteran, Constitutional scholar, and retiring Justice of the United States Supreme Court. Justice Breyer, thank you for your service. \n",
"\n",
"One of the most serious constitutional responsibilities a President has is nominating someone to serve on the United States Supreme Court. \n",
"\n",
"And I did that 4 days ago, when I nominated Circuit Court of Appeals Judge Ketanji Brown Jackson. One of our nation’s top legal minds, who will continue Justice Breyer’s legacy of excellence. \n"
]
}
],
"source": [
"print(docs[0].page_content)"
]
},
{
"cell_type": "markdown",
"id": "007f3102",
"metadata": {},
"source": [
"## Pinecone"
]
},
{
"cell_type": "code",
"execution_count": 5,
"id": "7f6047e5",
"metadata": {},
"outputs": [],
"source": [
"import pinecone \n",
"\n",
"# initialize pinecone\n",
"pinecone.init(api_key=\"\", environment=\"us-west1-gcp\")\n",
"\n",
"index_name = \"langchain-demo\"\n",
"\n",
"docsearch = Pinecone.from_texts(texts, embeddings, index_name=index_name)\n",
"\n",
"query = \"What did the president say about Ketanji Brown Jackson\"\n",
"docs = docsearch.similarity_search(query)"
]
},
{
"cell_type": "code",
"execution_count": 7,
"id": "8e81f1f0",
"metadata": {},
"outputs": [
{
"data": {
"text/plain": [
"Document(page_content='A former top litigator in private practice. A former federal public defender. And from a family of public school educators and police officers. A consensus builder. Since she’s been nominated, she’s received a broad range of support—from the Fraternal Order of Police to former judges appointed by Democrats and Republicans. \\n\\nAnd if we are to advance liberty and justice, we need to secure the Border and fix the immigration system. \\n\\nWe can do both. At our border, we’ve installed new technology like cutting-edge scanners to better detect drug smuggling. \\n\\nWe’ve set up joint patrols with Mexico and Guatemala to catch more human traffickers. \\n\\nWe’re putting in place dedicated immigration judges so families fleeing persecution and violence can have their cases heard faster. \\n\\nWe’re securing commitments and supporting partners in South and Central America to host more refugees and secure their own borders. ', lookup_str='', metadata={}, lookup_index=0)"
]
},
"execution_count": 7,
"metadata": {},
"output_type": "execute_result"
}
],
"source": [
"docs[0]"
]
},
{
"cell_type": "markdown",
"id": "9b852079",
"metadata": {},
"source": [
"## Qdrant"
]
},
{
"cell_type": "code",
"execution_count": null,
"id": "e5ec70ce",
"metadata": {},
"outputs": [],
"source": [
"host = \"<---host name here --->\"\n",
"api_key = \"<---api key here--->\"\n",
"qdrant = Qdrant.from_texts(texts, embeddings, host=host, prefer_grpc=True, api_key=api_key)\n",
"query = \"What did the president say about Ketanji Brown Jackson\""
]
},
{
"cell_type": "code",
"execution_count": 21,
"id": "9805ad1f",
"metadata": {},
"outputs": [],
"source": [
"docs = qdrant.similarity_search(query)"
]
},
{
"cell_type": "code",
"execution_count": 22,
"id": "bd097a0e",
"metadata": {},
"outputs": [
{
"data": {
"text/plain": [
"Document(page_content='In state after state, new laws have been passed, not only to suppress the vote, but to subvert entire elections. \\n\\nWe cannot let this happen. \\n\\nTonight. I call on the Senate to: Pass the Freedom to Vote Act. Pass the John Lewis Voting Rights Act. And while you’re at it, pass the Disclose Act so Americans can know who is funding our elections. \\n\\nTonight, I’d like to honor someone who has dedicated his life to serve this country: Justice Stephen Breyer—an Army veteran, Constitutional scholar, and retiring Justice of the United States Supreme Court. Justice Breyer, thank you for your service. \\n\\nOne of the most serious constitutional responsibilities a President has is nominating someone to serve on the United States Supreme Court. \\n\\nAnd I did that 4 days ago, when I nominated Circuit Court of Appeals Judge Ketanji Brown Jackson. One of our nation’s top legal minds, who will continue Justice Breyer’s legacy of excellence.', lookup_str='', metadata={}, lookup_index=0)"
]
},
"execution_count": 22,
"metadata": {},
"output_type": "execute_result"
}
],
"source": [
"docs[0]"
]
},
{
"cell_type": "markdown",
"id": "6c3ec797",
"metadata": {},
"source": [
"## Milvus\n",
"To run, you should have a Milvus instance up and running: https://milvus.io/docs/install_standalone-docker.md"
]
},
{
"cell_type": "code",
"execution_count": 1,
"id": "be347313",
"metadata": {},
"outputs": [],
"source": [
"from langchain.vectorstores import Milvus"
]
},
{
"cell_type": "code",
"execution_count": 6,
"id": "f2eee23f",
"metadata": {},
"outputs": [],
"source": [
"vector_db = Milvus.from_texts(\n",
" texts,\n",
" embeddings,\n",
" connection_args={\"host\": \"127.0.0.1\", \"port\": \"19530\"},\n",
")"
]
},
{
"cell_type": "code",
"execution_count": 7,
"id": "06bdb701",
"metadata": {},
"outputs": [],
"source": [
"docs = vector_db.similarity_search(query)"
]
},
{
"cell_type": "code",
"execution_count": 8,
"id": "7b3e94aa",
"metadata": {},
"outputs": [
{
"data": {
"text/plain": [
"Document(page_content='In state after state, new laws have been passed, not only to suppress the vote, but to subvert entire elections. \\n\\nWe cannot let this happen. \\n\\nTonight. I call on the Senate to: Pass the Freedom to Vote Act. Pass the John Lewis Voting Rights Act. And while you’re at it, pass the Disclose Act so Americans can know who is funding our elections. \\n\\nTonight, I’d like to honor someone who has dedicated his life to serve this country: Justice Stephen Breyer—an Army veteran, Constitutional scholar, and retiring Justice of the United States Supreme Court. Justice Breyer, thank you for your service. \\n\\nOne of the most serious constitutional responsibilities a President has is nominating someone to serve on the United States Supreme Court. \\n\\nAnd I did that 4 days ago, when I nominated Circuit Court of Appeals Judge Ketanji Brown Jackson. One of our nation’s top legal minds, who will continue Justice Breyer’s legacy of excellence.', lookup_str='', metadata={}, lookup_index=0)"
]
},
"execution_count": 8,
"metadata": {},
"output_type": "execute_result"
}
],
"source": [
"docs[0]"
]
},
{
"cell_type": "code",
"execution_count": null,
"id": "4af5a071",
"metadata": {},
"outputs": [],
"source": []
}
],
"metadata": {
"kernelspec": {
"display_name": "Python 3 (ipykernel)",
"language": "python",
"name": "python3"
},
"language_info": {
"codemirror_mode": {
"name": "ipython",
"version": 3
},
"file_extension": ".py",
"mimetype": "text/x-python",
"name": "python",
"nbconvert_exporter": "python",
"pygments_lexer": "ipython3",
"version": "3.10.9"
}
},
"nbformat": 4,
"nbformat_minor": 5
}
|
closed | langchain-ai/langchain | https://github.com/langchain-ai/langchain | 906 | Error in Pinecone batch selection logic | Current implementation of pinecone vec db finds the batches using:
```
# set end position of batch
i_end = min(i + batch_size, len(texts))
```
[link](https://github.com/hwchase17/langchain/blob/master/langchain/vectorstores/pinecone.py#L199)
But the following lines then go on to use a mix of `[i : i + batch_size]` and `[i:i_end]` to create batches:
```python
# get batch of texts and ids
lines_batch = texts[i : i + batch_size]
# create ids if not provided
if ids:
ids_batch = ids[i : i + batch_size]
else:
ids_batch = [str(uuid.uuid4()) for n in range(i, i_end)]
```
Fortunately, there is a `zip` function a few lines down that cuts the potentially longer chunks, preventing an error from being raised — yet I don't think think `[i: i+batch_size]` should be maintained as it's confusing and not explicit
Raised a PR here #907 | https://github.com/langchain-ai/langchain/issues/906 | https://github.com/langchain-ai/langchain/pull/907 | 82c080c6e617d4959fb4ee808deeba075f361702 | 3aa53b44dd5f013e35c316d110d340a630b0abd1 | "2023-02-06T07:52:59" | python | "2023-02-06T20:45:56" | langchain/vectorstores/pinecone.py | """Wrapper around Pinecone vector database."""
from __future__ import annotations
import uuid
from typing import Any, Callable, Iterable, List, Optional, Tuple
from langchain.docstore.document import Document
from langchain.embeddings.base import Embeddings
from langchain.vectorstores.base import VectorStore
class Pinecone(VectorStore):
"""Wrapper around Pinecone vector database.
To use, you should have the ``pinecone-client`` python package installed.
Example:
.. code-block:: python
from langchain.vectorstores import Pinecone
from langchain.embeddings.openai import OpenAIEmbeddings
import pinecone
pinecone.init(api_key="***", environment="us-west1-gcp")
index = pinecone.Index("langchain-demo")
embeddings = OpenAIEmbeddings()
vectorstore = Pinecone(index, embeddings.embed_query, "text")
"""
def __init__(
self,
index: Any,
embedding_function: Callable,
text_key: str,
):
"""Initialize with Pinecone client."""
try:
import pinecone
except ImportError:
raise ValueError(
"Could not import pinecone python package. "
"Please it install it with `pip install pinecone-client`."
)
if not isinstance(index, pinecone.index.Index):
raise ValueError(
f"client should be an instance of pinecone.index.Index, "
f"got {type(index)}"
)
self._index = index
self._embedding_function = embedding_function
self._text_key = text_key
def add_texts(
self,
texts: Iterable[str],
metadatas: Optional[List[dict]] = None,
ids: Optional[List[str]] = None,
namespace: Optional[str] = None,
) -> List[str]:
"""Run more texts through the embeddings and add to the vectorstore.
Args:
texts: Iterable of strings to add to the vectorstore.
metadatas: Optional list of metadatas associated with the texts.
ids: Optional list of ids to associate with the texts.
namespace: Optional pinecone namespace to add the texts to.
Returns:
List of ids from adding the texts into the vectorstore.
"""
# Embed and create the documents
docs = []
ids = ids or [str(uuid.uuid4()) for _ in texts]
for i, text in enumerate(texts):
embedding = self._embedding_function(text)
metadata = metadatas[i] if metadatas else {}
metadata[self._text_key] = text
docs.append((ids[i], embedding, metadata))
# upsert to Pinecone
self._index.upsert(vectors=docs, namespace=namespace)
return ids
def similarity_search_with_score(
self,
query: str,
k: int = 5,
filter: Optional[dict] = None,
namespace: Optional[str] = None,
) -> List[Tuple[Document, float]]:
"""Return pinecone documents most similar to query, along with scores.
Args:
query: Text to look up documents similar to.
k: Number of Documents to return. Defaults to 4.
filter: Dictionary of argument(s) to filter on metadata
namespace: Namespace to search in. Default will search in '' namespace.
Returns:
List of Documents most similar to the query and score for each
"""
query_obj = self._embedding_function(query)
docs = []
results = self._index.query(
[query_obj],
top_k=k,
include_metadata=True,
namespace=namespace,
filter=filter,
)
for res in results["matches"]:
metadata = res["metadata"]
text = metadata.pop(self._text_key)
docs.append((Document(page_content=text, metadata=metadata), res["score"]))
return docs
def similarity_search(
self,
query: str,
k: int = 5,
filter: Optional[dict] = None,
namespace: Optional[str] = None,
**kwargs: Any,
) -> List[Document]:
"""Return pinecone documents most similar to query.
Args:
query: Text to look up documents similar to.
k: Number of Documents to return. Defaults to 4.
filter: Dictionary of argument(s) to filter on metadata
namespace: Namespace to search in. Default will search in '' namespace.
Returns:
List of Documents most similar to the query and score for each
"""
query_obj = self._embedding_function(query)
docs = []
results = self._index.query(
[query_obj],
top_k=k,
include_metadata=True,
namespace=namespace,
filter=filter,
)
for res in results["matches"]:
metadata = res["metadata"]
text = metadata.pop(self._text_key)
docs.append(Document(page_content=text, metadata=metadata))
return docs
@classmethod
def from_texts(
cls,
texts: List[str],
embedding: Embeddings,
metadatas: Optional[List[dict]] = None,
ids: Optional[List[str]] = None,
batch_size: int = 32,
text_key: str = "text",
index_name: Optional[str] = None,
namespace: Optional[str] = None,
**kwargs: Any,
) -> Pinecone:
"""Construct Pinecone wrapper from raw documents.
This is a user friendly interface that:
1. Embeds documents.
2. Adds the documents to a provided Pinecone index
This is intended to be a quick way to get started.
Example:
.. code-block:: python
from langchain import Pinecone
from langchain.embeddings import OpenAIEmbeddings
embeddings = OpenAIEmbeddings()
pinecone = Pinecone.from_texts(
texts,
embeddings,
index_name="langchain-demo"
)
"""
try:
import pinecone
except ImportError:
raise ValueError(
"Could not import pinecone python package. "
"Please install it with `pip install pinecone-client`."
)
_index_name = index_name or str(uuid.uuid4())
indexes = pinecone.list_indexes() # checks if provided index exists
if _index_name in indexes:
index = pinecone.Index(_index_name)
else:
index = None
for i in range(0, len(texts), batch_size):
# set end position of batch
i_end = min(i + batch_size, len(texts))
# get batch of texts and ids
lines_batch = texts[i : i + batch_size]
# create ids if not provided
if ids:
ids_batch = ids[i : i + batch_size]
else:
ids_batch = [str(uuid.uuid4()) for n in range(i, i_end)]
# create embeddings
embeds = embedding.embed_documents(lines_batch)
# prep metadata and upsert batch
if metadatas:
metadata = metadatas[i : i + batch_size]
else:
metadata = [{} for _ in range(i, i_end)]
for j, line in enumerate(lines_batch):
metadata[j][text_key] = line
to_upsert = zip(ids_batch, embeds, metadata)
# Create index if it does not exist
if index is None:
pinecone.create_index(_index_name, dimension=len(embeds[0]))
index = pinecone.Index(_index_name)
# upsert to Pinecone
index.upsert(vectors=list(to_upsert), namespace=namespace)
return cls(index, embedding.embed_query, text_key)
@classmethod
def from_existing_index(
cls,
index_name: str,
embedding: Embeddings,
text_key: str = "text",
namespace: Optional[str] = None,
) -> Pinecone:
"""Load pinecone vectorstore from index name."""
try:
import pinecone
except ImportError:
raise ValueError(
"Could not import pinecone python package. "
"Please install it with `pip install pinecone-client`."
)
return cls(
pinecone.Index(index_name, namespace), embedding.embed_query, text_key
)
|
closed | langchain-ai/langchain | https://github.com/langchain-ai/langchain | 1,087 | Qdrant Wrapper issue: _document_from_score_point exposes incorrect key for content | 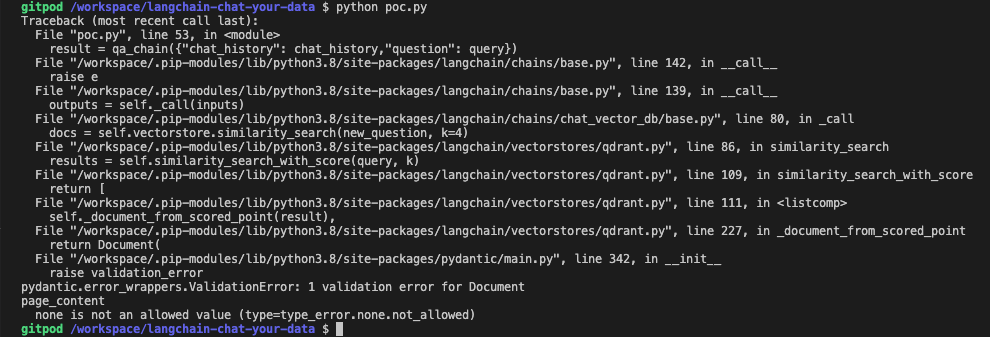
```
pydantic.error_wrappers.ValidationError: 1 validation error for Document
page_content
none is not an allowed value (type=type_error.none.not_allowed)
``` | https://github.com/langchain-ai/langchain/issues/1087 | https://github.com/langchain-ai/langchain/pull/1088 | 774550548242f44df9b219595cd46d9e238351e5 | 5d11e5da4077ad123bfff9f153f577fb5885af53 | "2023-02-16T13:18:41" | python | "2023-02-16T15:06:02" | langchain/vectorstores/qdrant.py | """Wrapper around Qdrant vector database."""
import uuid
from operator import itemgetter
from typing import Any, Callable, Iterable, List, Optional, Tuple
from langchain.docstore.document import Document
from langchain.embeddings.base import Embeddings
from langchain.utils import get_from_dict_or_env
from langchain.vectorstores import VectorStore
from langchain.vectorstores.utils import maximal_marginal_relevance
class Qdrant(VectorStore):
"""Wrapper around Qdrant vector database.
To use you should have the ``qdrant-client`` package installed.
Example:
.. code-block:: python
from langchain import Qdrant
client = QdrantClient()
collection_name = "MyCollection"
qdrant = Qdrant(client, collection_name, embedding_function)
"""
def __init__(self, client: Any, collection_name: str, embedding_function: Callable):
"""Initialize with necessary components."""
try:
import qdrant_client
except ImportError:
raise ValueError(
"Could not import qdrant-client python package. "
"Please it install it with `pip install qdrant-client`."
)
if not isinstance(client, qdrant_client.QdrantClient):
raise ValueError(
f"client should be an instance of qdrant_client.QdrantClient, "
f"got {type(client)}"
)
self.client: qdrant_client.QdrantClient = client
self.collection_name = collection_name
self.embedding_function = embedding_function
def add_texts(
self, texts: Iterable[str], metadatas: Optional[List[dict]] = None
) -> List[str]:
"""Run more texts through the embeddings and add to the vectorstore.
Args:
texts: Iterable of strings to add to the vectorstore.
metadatas: Optional list of metadatas associated with the texts.
Returns:
List of ids from adding the texts into the vectorstore.
"""
from qdrant_client.http import models as rest
ids = [uuid.uuid4().hex for _ in texts]
self.client.upsert(
collection_name=self.collection_name,
points=rest.Batch(
ids=ids,
vectors=[self.embedding_function(text) for text in texts],
payloads=self._build_payloads(texts, metadatas),
),
)
return ids
def similarity_search(
self, query: str, k: int = 4, **kwargs: Any
) -> List[Document]:
"""Return docs most similar to query.
Args:
query: Text to look up documents similar to.
k: Number of Documents to return. Defaults to 4.
Returns:
List of Documents most similar to the query.
"""
results = self.similarity_search_with_score(query, k)
return list(map(itemgetter(0), results))
def similarity_search_with_score(
self, query: str, k: int = 4
) -> List[Tuple[Document, float]]:
"""Return docs most similar to query.
Args:
query: Text to look up documents similar to.
k: Number of Documents to return. Defaults to 4.
Returns:
List of Documents most similar to the query and score for each
"""
embedding = self.embedding_function(query)
results = self.client.search(
collection_name=self.collection_name,
query_vector=embedding,
with_payload=True,
limit=k,
)
return [
(
self._document_from_scored_point(result),
result.score,
)
for result in results
]
def max_marginal_relevance_search(
self, query: str, k: int = 4, fetch_k: int = 20
) -> List[Document]:
"""Return docs selected using the maximal marginal relevance.
Maximal marginal relevance optimizes for similarity to query AND diversity
among selected documents.
Args:
query: Text to look up documents similar to.
k: Number of Documents to return. Defaults to 4.
fetch_k: Number of Documents to fetch to pass to MMR algorithm.
Returns:
List of Documents selected by maximal marginal relevance.
"""
embedding = self.embedding_function(query)
results = self.client.search(
collection_name=self.collection_name,
query_vector=embedding,
with_payload=True,
with_vectors=True,
limit=k,
)
embeddings = [result.vector for result in results]
mmr_selected = maximal_marginal_relevance(embedding, embeddings, k=k)
return [self._document_from_scored_point(results[i]) for i in mmr_selected]
@classmethod
def from_texts(
cls,
texts: List[str],
embedding: Embeddings,
metadatas: Optional[List[dict]] = None,
**kwargs: Any,
) -> "Qdrant":
"""Construct Qdrant wrapper from raw documents.
This is a user friendly interface that:
1. Embeds documents.
2. Creates an in memory docstore
3. Initializes the Qdrant database
This is intended to be a quick way to get started.
Example:
.. code-block:: python
from langchain import Qdrant
from langchain.embeddings import OpenAIEmbeddings
embeddings = OpenAIEmbeddings()
qdrant = Qdrant.from_texts(texts, embeddings)
"""
try:
import qdrant_client
except ImportError:
raise ValueError(
"Could not import qdrant-client python package. "
"Please it install it with `pip install qdrant-client`."
)
from qdrant_client.http import models as rest
# Just do a single quick embedding to get vector size
partial_embeddings = embedding.embed_documents(texts[:1])
vector_size = len(partial_embeddings[0])
qdrant_host = get_from_dict_or_env(kwargs, "host", "QDRANT_HOST")
kwargs.pop("host")
collection_name = kwargs.pop("collection_name", uuid.uuid4().hex)
distance_func = kwargs.pop("distance_func", "Cosine").upper()
client = qdrant_client.QdrantClient(host=qdrant_host, **kwargs)
client.recreate_collection(
collection_name=collection_name,
vectors_config=rest.VectorParams(
size=vector_size,
distance=rest.Distance[distance_func],
),
)
# Now generate the embeddings for all the texts
embeddings = embedding.embed_documents(texts)
client.upsert(
collection_name=collection_name,
points=rest.Batch(
ids=[uuid.uuid4().hex for _ in texts],
vectors=embeddings,
payloads=cls._build_payloads(texts, metadatas),
),
)
return cls(client, collection_name, embedding.embed_query)
@classmethod
def _build_payloads(
cls, texts: Iterable[str], metadatas: Optional[List[dict]]
) -> List[dict]:
return [
{
"page_content": text,
"metadata": metadatas[i] if metadatas is not None else None,
}
for i, text in enumerate(texts)
]
@classmethod
def _document_from_scored_point(cls, scored_point: Any) -> Document:
return Document(
page_content=scored_point.payload.get("page_content"),
metadata=scored_point.payload.get("metadata") or {},
)
|
closed | langchain-ai/langchain | https://github.com/langchain-ai/langchain | 1,103 | SQLDatabase chain having issue running queries on the database after connecting | Langchain SQLDatabase and using SQL chain is giving me issues in the recent versions. My goal has been this:
- Connect to a sql server (say, Azure SQL server) using mssql+pyodbc driver (also tried mssql+pymssql driver)
`connection_url = URL.create(
"mssql+pyodbc",
query={"odbc_connect": conn}
)`
`sql_database = SQLDatabase.from_uri(connection_url)`
- Use this sql_database to create a SQLSequentialChain (also tried SQLChain)
`chain = SQLDatabaseSequentialChain.from_llm(
llm=self.llm,
database=sql_database,
verbose=False,
query_prompt=chain_prompt)`
- Query this chain
However, in the most recent version of langchain 0.0.88, I get this issue:
<img width="663" alt="image" src="https://user-images.githubusercontent.com/25394373/219547335-4108f02e-4721-425a-a7a3-199a70cd97f1.png">
And in the previous version 0.0.86, I was getting this:
<img width="646" alt="image" src="https://user-images.githubusercontent.com/25394373/219547750-f46f1ecb-2151-4700-8dae-e2c356f79aea.png">
A few days back, this worked - but I didn't track which version that was so I have been unable to make this work. Please help look into this. | https://github.com/langchain-ai/langchain/issues/1103 | https://github.com/langchain-ai/langchain/pull/1129 | 1ed708391e80a4de83e859b8364a32cc222df9ef | c39ef70aa457dcfcf8ddcf61f89dd69d55307744 | "2023-02-17T04:18:02" | python | "2023-02-17T21:39:44" | langchain/sql_database.py | """SQLAlchemy wrapper around a database."""
from __future__ import annotations
import ast
from typing import Any, Iterable, List, Optional
from sqlalchemy import create_engine, inspect
from sqlalchemy.engine import Engine
_TEMPLATE_PREFIX = """Table data will be described in the following format:
Table 'table name' has columns: {
column1 name: (column1 type, [list of example values for column1]),
column2 name: (column2 type, [list of example values for column2]),
...
}
These are the tables you can use, together with their column information:
"""
class SQLDatabase:
"""SQLAlchemy wrapper around a database."""
def __init__(
self,
engine: Engine,
schema: Optional[str] = None,
ignore_tables: Optional[List[str]] = None,
include_tables: Optional[List[str]] = None,
sample_rows_in_table_info: int = 3,
):
"""Create engine from database URI."""
self._engine = engine
self._schema = schema
if include_tables and ignore_tables:
raise ValueError("Cannot specify both include_tables and ignore_tables")
self._inspector = inspect(self._engine)
self._all_tables = set(self._inspector.get_table_names(schema=schema))
self._include_tables = set(include_tables) if include_tables else set()
if self._include_tables:
missing_tables = self._include_tables - self._all_tables
if missing_tables:
raise ValueError(
f"include_tables {missing_tables} not found in database"
)
self._ignore_tables = set(ignore_tables) if ignore_tables else set()
if self._ignore_tables:
missing_tables = self._ignore_tables - self._all_tables
if missing_tables:
raise ValueError(
f"ignore_tables {missing_tables} not found in database"
)
self._sample_rows_in_table_info = sample_rows_in_table_info
@classmethod
def from_uri(cls, database_uri: str, **kwargs: Any) -> SQLDatabase:
"""Construct a SQLAlchemy engine from URI."""
return cls(create_engine(database_uri), **kwargs)
@property
def dialect(self) -> str:
"""Return string representation of dialect to use."""
return self._engine.dialect.name
def get_table_names(self) -> Iterable[str]:
"""Get names of tables available."""
if self._include_tables:
return self._include_tables
return self._all_tables - self._ignore_tables
@property
def table_info(self) -> str:
"""Information about all tables in the database."""
return self.get_table_info()
def get_table_info(self, table_names: Optional[List[str]] = None) -> str:
"""Get information about specified tables.
Follows best practices as specified in: Rajkumar et al, 2022
(https://arxiv.org/abs/2204.00498)
If `sample_rows_in_table_info`, the specified number of sample rows will be
appended to each table description. This can increase performance as
demonstrated in the paper.
"""
all_table_names = self.get_table_names()
if table_names is not None:
missing_tables = set(table_names).difference(all_table_names)
if missing_tables:
raise ValueError(f"table_names {missing_tables} not found in database")
all_table_names = table_names
tables = []
for table_name in all_table_names:
columns = []
create_table = self.run(
(
"SELECT sql FROM sqlite_master WHERE "
f"type='table' AND name='{table_name}'"
),
fetch="one",
)
for column in self._inspector.get_columns(table_name, schema=self._schema):
columns.append(column["name"])
if self._sample_rows_in_table_info:
select_star = (
f"SELECT * FROM '{table_name}' LIMIT "
f"{self._sample_rows_in_table_info}"
)
sample_rows = self.run(select_star)
sample_rows_ls = ast.literal_eval(sample_rows)
sample_rows_ls = list(
map(lambda ls: [str(i)[:100] for i in ls], sample_rows_ls)
)
columns_str = " ".join(columns)
sample_rows_str = "\n".join([" ".join(row) for row in sample_rows_ls])
tables.append(
create_table
+ "\n\n"
+ select_star
+ "\n"
+ columns_str
+ "\n"
+ sample_rows_str
)
else:
tables.append(create_table)
final_str = "\n\n\n".join(tables)
return final_str
def run(self, command: str, fetch: str = "all") -> str:
"""Execute a SQL command and return a string representing the results.
If the statement returns rows, a string of the results is returned.
If the statement returns no rows, an empty string is returned.
"""
with self._engine.begin() as connection:
if self._schema is not None:
connection.exec_driver_sql(f"SET search_path TO {self._schema}")
cursor = connection.exec_driver_sql(command)
if cursor.returns_rows:
if fetch == "all":
result = cursor.fetchall()
elif fetch == "one":
result = cursor.fetchone()[0]
else:
raise ValueError("Fetch parameter must be either 'one' or 'all'")
return str(result)
return ""
|
closed | langchain-ai/langchain | https://github.com/langchain-ai/langchain | 1,186 | max_marginal_relevance_search_by_vector with k > doc size | #1117 didn't seem to fix it? I still get an error `KeyError: -1`
Code to reproduce:
```py
output = docsearch.max_marginal_relevance_search_by_vector(query_vec, k=10)
```
where `k > len(docsearch)`. Pushing PR with unittest/fix shortly. | https://github.com/langchain-ai/langchain/issues/1186 | https://github.com/langchain-ai/langchain/pull/1187 | 159c560c95ed9e11cc740040cc6ee07abb871ded | c5015d77e23b24b3b65d803271f1fa9018d53a05 | "2023-02-20T19:19:29" | python | "2023-02-21T00:39:13" | langchain/vectorstores/faiss.py | """Wrapper around FAISS vector database."""
from __future__ import annotations
import pickle
import uuid
from pathlib import Path
from typing import Any, Callable, Dict, Iterable, List, Optional, Tuple
import numpy as np
from langchain.docstore.base import AddableMixin, Docstore
from langchain.docstore.document import Document
from langchain.docstore.in_memory import InMemoryDocstore
from langchain.embeddings.base import Embeddings
from langchain.vectorstores.base import VectorStore
from langchain.vectorstores.utils import maximal_marginal_relevance
def dependable_faiss_import() -> Any:
"""Import faiss if available, otherwise raise error."""
try:
import faiss
except ImportError:
raise ValueError(
"Could not import faiss python package. "
"Please it install it with `pip install faiss` "
"or `pip install faiss-cpu` (depending on Python version)."
)
return faiss
class FAISS(VectorStore):
"""Wrapper around FAISS vector database.
To use, you should have the ``faiss`` python package installed.
Example:
.. code-block:: python
from langchain import FAISS
faiss = FAISS(embedding_function, index, docstore)
"""
def __init__(
self,
embedding_function: Callable,
index: Any,
docstore: Docstore,
index_to_docstore_id: Dict[int, str],
):
"""Initialize with necessary components."""
self.embedding_function = embedding_function
self.index = index
self.docstore = docstore
self.index_to_docstore_id = index_to_docstore_id
def add_texts(
self, texts: Iterable[str], metadatas: Optional[List[dict]] = None
) -> List[str]:
"""Run more texts through the embeddings and add to the vectorstore.
Args:
texts: Iterable of strings to add to the vectorstore.
metadatas: Optional list of metadatas associated with the texts.
Returns:
List of ids from adding the texts into the vectorstore.
"""
if not isinstance(self.docstore, AddableMixin):
raise ValueError(
"If trying to add texts, the underlying docstore should support "
f"adding items, which {self.docstore} does not"
)
# Embed and create the documents.
embeddings = [self.embedding_function(text) for text in texts]
documents = []
for i, text in enumerate(texts):
metadata = metadatas[i] if metadatas else {}
documents.append(Document(page_content=text, metadata=metadata))
# Add to the index, the index_to_id mapping, and the docstore.
starting_len = len(self.index_to_docstore_id)
self.index.add(np.array(embeddings, dtype=np.float32))
# Get list of index, id, and docs.
full_info = [
(starting_len + i, str(uuid.uuid4()), doc)
for i, doc in enumerate(documents)
]
# Add information to docstore and index.
self.docstore.add({_id: doc for _, _id, doc in full_info})
index_to_id = {index: _id for index, _id, _ in full_info}
self.index_to_docstore_id.update(index_to_id)
return [_id for _, _id, _ in full_info]
def similarity_search_with_score_by_vector(
self, embedding: List[float], k: int = 4
) -> List[Tuple[Document, float]]:
"""Return docs most similar to query.
Args:
query: Text to look up documents similar to.
k: Number of Documents to return. Defaults to 4.
Returns:
List of Documents most similar to the query and score for each
"""
scores, indices = self.index.search(np.array([embedding], dtype=np.float32), k)
docs = []
for j, i in enumerate(indices[0]):
if i == -1:
# This happens when not enough docs are returned.
continue
_id = self.index_to_docstore_id[i]
doc = self.docstore.search(_id)
if not isinstance(doc, Document):
raise ValueError(f"Could not find document for id {_id}, got {doc}")
docs.append((doc, scores[0][j]))
return docs
def similarity_search_with_score(
self, query: str, k: int = 4
) -> List[Tuple[Document, float]]:
"""Return docs most similar to query.
Args:
query: Text to look up documents similar to.
k: Number of Documents to return. Defaults to 4.
Returns:
List of Documents most similar to the query and score for each
"""
embedding = self.embedding_function(query)
docs = self.similarity_search_with_score_by_vector(embedding, k)
return docs
def similarity_search_by_vector(
self, embedding: List[float], k: int = 4, **kwargs: Any
) -> List[Document]:
"""Return docs most similar to embedding vector.
Args:
embedding: Embedding to look up documents similar to.
k: Number of Documents to return. Defaults to 4.
Returns:
List of Documents most similar to the embedding.
"""
docs_and_scores = self.similarity_search_with_score_by_vector(embedding, k)
return [doc for doc, _ in docs_and_scores]
def similarity_search(
self, query: str, k: int = 4, **kwargs: Any
) -> List[Document]:
"""Return docs most similar to query.
Args:
query: Text to look up documents similar to.
k: Number of Documents to return. Defaults to 4.
Returns:
List of Documents most similar to the query.
"""
docs_and_scores = self.similarity_search_with_score(query, k)
return [doc for doc, _ in docs_and_scores]
def max_marginal_relevance_search_by_vector(
self, embedding: List[float], k: int = 4, fetch_k: int = 20
) -> List[Document]:
"""Return docs selected using the maximal marginal relevance.
Maximal marginal relevance optimizes for similarity to query AND diversity
among selected documents.
Args:
embedding: Embedding to look up documents similar to.
k: Number of Documents to return. Defaults to 4.
fetch_k: Number of Documents to fetch to pass to MMR algorithm.
Returns:
List of Documents selected by maximal marginal relevance.
"""
_, indices = self.index.search(np.array([embedding], dtype=np.float32), fetch_k)
# -1 happens when not enough docs are returned.
embeddings = [self.index.reconstruct(int(i)) for i in indices[0] if i != -1]
mmr_selected = maximal_marginal_relevance(
np.array([embedding], dtype=np.float32), embeddings, k=k
)
selected_indices = [indices[0][i] for i in mmr_selected]
docs = []
for i in selected_indices:
_id = self.index_to_docstore_id[i]
if _id == -1:
# This happens when not enough docs are returned.
continue
doc = self.docstore.search(_id)
if not isinstance(doc, Document):
raise ValueError(f"Could not find document for id {_id}, got {doc}")
docs.append(doc)
return docs
def max_marginal_relevance_search(
self, query: str, k: int = 4, fetch_k: int = 20
) -> List[Document]:
"""Return docs selected using the maximal marginal relevance.
Maximal marginal relevance optimizes for similarity to query AND diversity
among selected documents.
Args:
query: Text to look up documents similar to.
k: Number of Documents to return. Defaults to 4.
fetch_k: Number of Documents to fetch to pass to MMR algorithm.
Returns:
List of Documents selected by maximal marginal relevance.
"""
embedding = self.embedding_function(query)
docs = self.max_marginal_relevance_search_by_vector(embedding, k, fetch_k)
return docs
@classmethod
def from_texts(
cls,
texts: List[str],
embedding: Embeddings,
metadatas: Optional[List[dict]] = None,
**kwargs: Any,
) -> FAISS:
"""Construct FAISS wrapper from raw documents.
This is a user friendly interface that:
1. Embeds documents.
2. Creates an in memory docstore
3. Initializes the FAISS database
This is intended to be a quick way to get started.
Example:
.. code-block:: python
from langchain import FAISS
from langchain.embeddings import OpenAIEmbeddings
embeddings = OpenAIEmbeddings()
faiss = FAISS.from_texts(texts, embeddings)
"""
faiss = dependable_faiss_import()
embeddings = embedding.embed_documents(texts)
index = faiss.IndexFlatL2(len(embeddings[0]))
index.add(np.array(embeddings, dtype=np.float32))
documents = []
for i, text in enumerate(texts):
metadata = metadatas[i] if metadatas else {}
documents.append(Document(page_content=text, metadata=metadata))
index_to_id = {i: str(uuid.uuid4()) for i in range(len(documents))}
docstore = InMemoryDocstore(
{index_to_id[i]: doc for i, doc in enumerate(documents)}
)
return cls(embedding.embed_query, index, docstore, index_to_id)
def save_local(self, folder_path: str) -> None:
"""Save FAISS index, docstore, and index_to_docstore_id to disk.
Args:
folder_path: folder path to save index, docstore,
and index_to_docstore_id to.
"""
path = Path(folder_path)
path.mkdir(exist_ok=True, parents=True)
# save index separately since it is not picklable
faiss = dependable_faiss_import()
faiss.write_index(self.index, str(path / "index.faiss"))
# save docstore and index_to_docstore_id
with open(path / "index.pkl", "wb") as f:
pickle.dump((self.docstore, self.index_to_docstore_id), f)
@classmethod
def load_local(cls, folder_path: str, embeddings: Embeddings) -> FAISS:
"""Load FAISS index, docstore, and index_to_docstore_id to disk.
Args:
folder_path: folder path to load index, docstore,
and index_to_docstore_id from.
embeddings: Embeddings to use when generating queries
"""
path = Path(folder_path)
# load index separately since it is not picklable
faiss = dependable_faiss_import()
index = faiss.read_index(str(path / "index.faiss"))
# load docstore and index_to_docstore_id
with open(path / "index.pkl", "rb") as f:
docstore, index_to_docstore_id = pickle.load(f)
return cls(embeddings.embed_query, index, docstore, index_to_docstore_id)
|
closed | langchain-ai/langchain | https://github.com/langchain-ai/langchain | 1,186 | max_marginal_relevance_search_by_vector with k > doc size | #1117 didn't seem to fix it? I still get an error `KeyError: -1`
Code to reproduce:
```py
output = docsearch.max_marginal_relevance_search_by_vector(query_vec, k=10)
```
where `k > len(docsearch)`. Pushing PR with unittest/fix shortly. | https://github.com/langchain-ai/langchain/issues/1186 | https://github.com/langchain-ai/langchain/pull/1187 | 159c560c95ed9e11cc740040cc6ee07abb871ded | c5015d77e23b24b3b65d803271f1fa9018d53a05 | "2023-02-20T19:19:29" | python | "2023-02-21T00:39:13" | tests/integration_tests/vectorstores/test_faiss.py | """Test FAISS functionality."""
import tempfile
import pytest
from langchain.docstore.document import Document
from langchain.docstore.in_memory import InMemoryDocstore
from langchain.docstore.wikipedia import Wikipedia
from langchain.vectorstores.faiss import FAISS
from tests.integration_tests.vectorstores.fake_embeddings import FakeEmbeddings
def test_faiss() -> None:
"""Test end to end construction and search."""
texts = ["foo", "bar", "baz"]
docsearch = FAISS.from_texts(texts, FakeEmbeddings())
index_to_id = docsearch.index_to_docstore_id
expected_docstore = InMemoryDocstore(
{
index_to_id[0]: Document(page_content="foo"),
index_to_id[1]: Document(page_content="bar"),
index_to_id[2]: Document(page_content="baz"),
}
)
assert docsearch.docstore.__dict__ == expected_docstore.__dict__
output = docsearch.similarity_search("foo", k=1)
assert output == [Document(page_content="foo")]
def test_faiss_vector_sim() -> None:
"""Test vector similarity."""
texts = ["foo", "bar", "baz"]
docsearch = FAISS.from_texts(texts, FakeEmbeddings())
index_to_id = docsearch.index_to_docstore_id
expected_docstore = InMemoryDocstore(
{
index_to_id[0]: Document(page_content="foo"),
index_to_id[1]: Document(page_content="bar"),
index_to_id[2]: Document(page_content="baz"),
}
)
assert docsearch.docstore.__dict__ == expected_docstore.__dict__
query_vec = FakeEmbeddings().embed_query(text="foo")
output = docsearch.similarity_search_by_vector(query_vec, k=1)
assert output == [Document(page_content="foo")]
def test_faiss_with_metadatas() -> None:
"""Test end to end construction and search."""
texts = ["foo", "bar", "baz"]
metadatas = [{"page": i} for i in range(len(texts))]
docsearch = FAISS.from_texts(texts, FakeEmbeddings(), metadatas=metadatas)
expected_docstore = InMemoryDocstore(
{
docsearch.index_to_docstore_id[0]: Document(
page_content="foo", metadata={"page": 0}
),
docsearch.index_to_docstore_id[1]: Document(
page_content="bar", metadata={"page": 1}
),
docsearch.index_to_docstore_id[2]: Document(
page_content="baz", metadata={"page": 2}
),
}
)
assert docsearch.docstore.__dict__ == expected_docstore.__dict__
output = docsearch.similarity_search("foo", k=1)
assert output == [Document(page_content="foo", metadata={"page": 0})]
def test_faiss_search_not_found() -> None:
"""Test what happens when document is not found."""
texts = ["foo", "bar", "baz"]
docsearch = FAISS.from_texts(texts, FakeEmbeddings())
# Get rid of the docstore to purposefully induce errors.
docsearch.docstore = InMemoryDocstore({})
with pytest.raises(ValueError):
docsearch.similarity_search("foo")
def test_faiss_add_texts() -> None:
"""Test end to end adding of texts."""
# Create initial doc store.
texts = ["foo", "bar", "baz"]
docsearch = FAISS.from_texts(texts, FakeEmbeddings())
# Test adding a similar document as before.
docsearch.add_texts(["foo"])
output = docsearch.similarity_search("foo", k=2)
assert output == [Document(page_content="foo"), Document(page_content="foo")]
def test_faiss_add_texts_not_supported() -> None:
"""Test adding of texts to a docstore that doesn't support it."""
docsearch = FAISS(FakeEmbeddings().embed_query, None, Wikipedia(), {})
with pytest.raises(ValueError):
docsearch.add_texts(["foo"])
def test_faiss_local_save_load() -> None:
"""Test end to end serialization."""
texts = ["foo", "bar", "baz"]
docsearch = FAISS.from_texts(texts, FakeEmbeddings())
with tempfile.NamedTemporaryFile() as temp_file:
docsearch.save_local(temp_file.name)
new_docsearch = FAISS.load_local(temp_file.name, FakeEmbeddings())
assert new_docsearch.index is not None
|
closed | langchain-ai/langchain | https://github.com/langchain-ai/langchain | 983 | SQLite Cache memory for async agent runs fails in concurrent calls | I have a slack bot using slack bolt for python to handle various request for certain topics.
Using the SQLite Cache as described in here
https://langchain.readthedocs.io/en/latest/modules/llms/examples/llm_caching.html
Fails when asking the same question mutiple times for the first time with error
> (sqlite3.IntegrityError) UNIQUE constraint failed: full_llm_cache.prompt, full_llm_cache.llm, full_llm_cache.idx
As an example code:
```python3
from langchain.cache import SQLiteCache
langchain.llm_cache = SQLiteCache(database_path=".langchain.db")
import asyncio
from slack_bolt.async_app import AsyncApp
from slack_bolt.adapter.socket_mode.async_handler import AsyncSocketModeHandler
# For simplicity lets imagine that here we
# instanciate LLM , CHAINS and AGENT
app = AsyncApp(token=SLACK_BOT_API_KEY)
async def async_run(self, agent_class, llm, chains):
@app.event('app_mention')
async def handle_mention(event, say, ack):
# Acknowlegde message to slack
await ack()
# Get response from agent
response = await agent.arun(message)
#Send response to slack
await say(response)
handler = AsyncSocketModeHandler(app, SLACK_BOT_TOKEN)
await handler.start_async()
asyncio.run(async_run(agent, llm, chains))
```
I imagine that this has something to do with how the async calls interact with the cache, as it seems that the first async call creates the prompt in the sqlite mem cache but without the answer, the second one (and other) async calls tries to create the same record in the sqlite db, but fails because of the first entry. | https://github.com/langchain-ai/langchain/issues/983 | https://github.com/langchain-ai/langchain/pull/1286 | 81abcae91a3bbd3c90ac9644d232509b3094b54d | 42b892c21be7278689cabdb83101631f286ffc34 | "2023-02-10T19:30:13" | python | "2023-02-27T01:54:43" | langchain/cache.py | """Beta Feature: base interface for cache."""
from abc import ABC, abstractmethod
from typing import Any, Dict, List, Optional, Tuple
from sqlalchemy import Column, Integer, String, create_engine, select
from sqlalchemy.engine.base import Engine
from sqlalchemy.orm import Session
try:
from sqlalchemy.orm import declarative_base
except ImportError:
from sqlalchemy.ext.declarative import declarative_base
from langchain.schema import Generation
RETURN_VAL_TYPE = List[Generation]
class BaseCache(ABC):
"""Base interface for cache."""
@abstractmethod
def lookup(self, prompt: str, llm_string: str) -> Optional[RETURN_VAL_TYPE]:
"""Look up based on prompt and llm_string."""
@abstractmethod
def update(self, prompt: str, llm_string: str, return_val: RETURN_VAL_TYPE) -> None:
"""Update cache based on prompt and llm_string."""
class InMemoryCache(BaseCache):
"""Cache that stores things in memory."""
def __init__(self) -> None:
"""Initialize with empty cache."""
self._cache: Dict[Tuple[str, str], RETURN_VAL_TYPE] = {}
def lookup(self, prompt: str, llm_string: str) -> Optional[RETURN_VAL_TYPE]:
"""Look up based on prompt and llm_string."""
return self._cache.get((prompt, llm_string), None)
def update(self, prompt: str, llm_string: str, return_val: RETURN_VAL_TYPE) -> None:
"""Update cache based on prompt and llm_string."""
self._cache[(prompt, llm_string)] = return_val
Base = declarative_base()
class FullLLMCache(Base): # type: ignore
"""SQLite table for full LLM Cache (all generations)."""
__tablename__ = "full_llm_cache"
prompt = Column(String, primary_key=True)
llm = Column(String, primary_key=True)
idx = Column(Integer, primary_key=True)
response = Column(String)
class SQLAlchemyCache(BaseCache):
"""Cache that uses SQAlchemy as a backend."""
def __init__(self, engine: Engine, cache_schema: Any = FullLLMCache):
"""Initialize by creating all tables."""
self.engine = engine
self.cache_schema = cache_schema
self.cache_schema.metadata.create_all(self.engine)
def lookup(self, prompt: str, llm_string: str) -> Optional[RETURN_VAL_TYPE]:
"""Look up based on prompt and llm_string."""
stmt = (
select(self.cache_schema.response)
.where(self.cache_schema.prompt == prompt)
.where(self.cache_schema.llm == llm_string)
.order_by(self.cache_schema.idx)
)
with Session(self.engine) as session:
generations = [Generation(text=row[0]) for row in session.execute(stmt)]
if len(generations) > 0:
return generations
return None
def update(self, prompt: str, llm_string: str, return_val: RETURN_VAL_TYPE) -> None:
"""Look up based on prompt and llm_string."""
for i, generation in enumerate(return_val):
item = self.cache_schema(
prompt=prompt, llm=llm_string, response=generation.text, idx=i
)
with Session(self.engine) as session, session.begin():
session.add(item)
class SQLiteCache(SQLAlchemyCache):
"""Cache that uses SQLite as a backend."""
def __init__(self, database_path: str = ".langchain.db"):
"""Initialize by creating the engine and all tables."""
engine = create_engine(f"sqlite:///{database_path}")
super().__init__(engine)
class RedisCache(BaseCache):
"""Cache that uses Redis as a backend."""
def __init__(self, redis_: Any):
"""Initialize by passing in Redis instance."""
try:
from redis import Redis
except ImportError:
raise ValueError(
"Could not import redis python package. "
"Please install it with `pip install redis`."
)
if not isinstance(redis_, Redis):
raise ValueError("Please pass in Redis object.")
self.redis = redis_
def _key(self, prompt: str, llm_string: str, idx: int) -> str:
"""Compute key from prompt, llm_string, and idx."""
return str(hash(prompt + llm_string)) + "_" + str(idx)
def lookup(self, prompt: str, llm_string: str) -> Optional[RETURN_VAL_TYPE]:
"""Look up based on prompt and llm_string."""
idx = 0
generations = []
while self.redis.get(self._key(prompt, llm_string, idx)):
result = self.redis.get(self._key(prompt, llm_string, idx))
if not result:
break
elif isinstance(result, bytes):
result = result.decode()
generations.append(Generation(text=result))
idx += 1
return generations if generations else None
def update(self, prompt: str, llm_string: str, return_val: RETURN_VAL_TYPE) -> None:
"""Update cache based on prompt and llm_string."""
for i, generation in enumerate(return_val):
self.redis.set(self._key(prompt, llm_string, i), generation.text)
|
closed | langchain-ai/langchain | https://github.com/langchain-ai/langchain | 1,383 | ValueError: unsupported format character 'b' (0x62) at index 52 | python version 3.9.12, langchain version 0.0.98
Using this code
```
db = SQLDatabase.from_uri(DATABSE_URI, include_tables=['tbl_abc'])
toolkit = SQLDatabaseToolkit(db=db)
agent_executor = create_sql_agent(
llm=OpenAI(temperature=0),
toolkit=toolkit,
verbose=True
)
agent_executor.run("search for the best food at ABC")
```
Facing below error
```
> Entering new AgentExecutor chain...
Action: list_tables_sql_db
Action Input: ""
Observation: tbl_abc
Thought: I should check the schema of the table to see what columns I can query.
Action: schema_sql_db
Action Input: "tbl_abc"
Observation:
CREATE TABLE tbl_chat (
chat_id BIGINT(20) NOT NULL AUTO_INCREMENT,
user_id INTEGER(11),
chat_msg TEXT,
last_taged_on DATETIME NOT NULL DEFAULT '0000-00-00 00:00:00',
............
...........
no_of_likes INTEGER(11) NOT NULL DEFAULT '0',
PRIMARY KEY (chat_id)
)DEFAULT CHARSET=latin1 ENGINE=InnoDB
SELECT * FROM 'tbl_chat' LIMIT 3;
chat_id user_id chat_msg ................ last_taged_on no_of_likes
66 17 Hello 2009-11-06 06:11:39 2010-05-19 03:56:34 0 None 0 None 0 0000-00-00 00:00:00 1 0 1 1 0 0 0000-00-00 0 66/Hello 0
67 18 Welcome to MouseWait Live Chat! 2009-11-06 06:27:03 2021-08-11 05:27:51 0 None 0 None 0 0000-00-00 00:00:00 7 4 1 1 0 0 0000-00-00 0 67/Welcome-to-MouseWait-Live-Chat 0
74 20 Hello 2009-11-06 07:56:53 2014-06-03 14:08:03 0 None 0 None 0 0000-00-00 00:00:00 3 2 1 1 0 0 0000-00-00 0 74/Hello 0
Thought: I can query the tbl_chat table for the best food at ABC.
Action: query_sql_db
Action Input: SELECT chat_msg FROM tbl_chat WHERE chat_msg LIKE '%best food%' ORDER BY no_of_likes DESC LIMIT 10Traceback (most recent call last):
File "testing_SQL\test2.py", line 28, in <module>
agent_executor.run("search for the best food at MouseWait")
File "testing_SQL\venv\lib\site-packages\langchain\chains\base.py", line 239, in run
return self(args[0])[self.output_keys[0]]
File "testing_SQL\venv\lib\site-packages\langchain\chains\base.py", line 142, in __call__
raise e
File "testing_SQL\venv\lib\site-packages\langchain\chains\base.py", line 139, in __call__
outputs = self._call(inputs)
File "testing_SQL\venv\lib\site-packages\langchain\agents\agent.py", line 503, in _call
next_step_output = self._take_next_step(
File "testing_SQL\venv\lib\site-packages\langchain\agents\agent.py", line 420, in _take_next_step
observation = tool.run(
File "testing_SQL\venv\lib\site-packages\langchain\tools\base.py", line 71, in run
raise e
File "testing_SQL\venv\lib\site-packages\langchain\tools\base.py", line 68, in run
observation = self._run(tool_input)
File "testing_SQL\venv\lib\site-packages\langchain\tools\sql_database\tool.py", line 39, in _run
return self.db.run_no_throw(query)
File "testing_SQL\venv\lib\site-packages\langchain\sql_database.py", line 216, in run_no_throw
return self.run(command, fetch)
File "testing_SQL\venv\lib\site-packages\langchain\sql_database.py", line 180, in run
cursor = connection.exec_driver_sql(command)
File "testing_SQL\venv\lib\site-packages\sqlalchemy\engine\base.py", line 1760, in exec_driver_sql
return self._exec_driver_sql(
File "testing_SQL\venv\lib\site-packages\sqlalchemy\engine\base.py", line 1669, in _exec_driver_sql
ret = self._execute_context(
File "testing_SQL\venv\lib\site-packages\sqlalchemy\engine\base.py", line 1943, in _execute_context
self._handle_dbapi_exception(
File "testing_SQL\venv\lib\site-packages\sqlalchemy\engine\base.py", line 2128, in _handle_dbapi_exception
util.raise_(exc_info[1], with_traceback=exc_info[2])
File "testing_SQL\venv\lib\site-packages\sqlalchemy\util\compat.py", line 211, in raise_
raise exception
File "testing_SQL\venv\lib\site-packages\sqlalchemy\engine\base.py", line 1900, in _execute_context
self.dialect.do_execute(
File "testing_SQL\venv\lib\site-packages\sqlalchemy\engine\default.py", line 736, in do_execute
cursor.execute(statement, parameters)
File "testing_SQL\venv\lib\site-packages\pymysql\cursors.py", line 146, in execute
query = self.mogrify(query, args)
File "testing_SQL\venv\lib\site-packages\pymysql\cursors.py", line 125, in mogrify
query = query % self._escape_args(args, conn)
ValueError: unsupported format character 'b' (0x62) at index 52
Process finished with exit code 1```
| https://github.com/langchain-ai/langchain/issues/1383 | https://github.com/langchain-ai/langchain/pull/1408 | 443992c4d58dcb168a21c0f45afb36b84fbdd46a | 882f7964fb0c5364bce0dcfb73abacd8ece525e4 | "2023-03-02T07:22:39" | python | "2023-03-03T00:03:16" | langchain/sql_database.py | """SQLAlchemy wrapper around a database."""
from __future__ import annotations
from typing import Any, Iterable, List, Optional
from sqlalchemy import MetaData, create_engine, inspect, select
from sqlalchemy.engine import Engine
from sqlalchemy.exc import ProgrammingError, SQLAlchemyError
from sqlalchemy.schema import CreateTable
class SQLDatabase:
"""SQLAlchemy wrapper around a database."""
def __init__(
self,
engine: Engine,
schema: Optional[str] = None,
metadata: Optional[MetaData] = None,
ignore_tables: Optional[List[str]] = None,
include_tables: Optional[List[str]] = None,
sample_rows_in_table_info: int = 3,
custom_table_info: Optional[dict] = None,
):
"""Create engine from database URI."""
self._engine = engine
self._schema = schema
if include_tables and ignore_tables:
raise ValueError("Cannot specify both include_tables and ignore_tables")
self._inspector = inspect(self._engine)
self._all_tables = set(self._inspector.get_table_names(schema=schema))
self._include_tables = set(include_tables) if include_tables else set()
if self._include_tables:
missing_tables = self._include_tables - self._all_tables
if missing_tables:
raise ValueError(
f"include_tables {missing_tables} not found in database"
)
self._ignore_tables = set(ignore_tables) if ignore_tables else set()
if self._ignore_tables:
missing_tables = self._ignore_tables - self._all_tables
if missing_tables:
raise ValueError(
f"ignore_tables {missing_tables} not found in database"
)
if not isinstance(sample_rows_in_table_info, int):
raise TypeError("sample_rows_in_table_info must be an integer")
self._sample_rows_in_table_info = sample_rows_in_table_info
self._custom_table_info = custom_table_info
if self._custom_table_info:
if not isinstance(self._custom_table_info, dict):
raise TypeError(
"table_info must be a dictionary with table names as keys and the "
"desired table info as values"
)
# only keep the tables that are also present in the database
intersection = set(self._custom_table_info).intersection(self._all_tables)
self._custom_table_info = dict(
(table, self._custom_table_info[table])
for table in self._custom_table_info
if table in intersection
)
self._metadata = metadata or MetaData()
self._metadata.reflect(bind=self._engine)
@classmethod
def from_uri(cls, database_uri: str, **kwargs: Any) -> SQLDatabase:
"""Construct a SQLAlchemy engine from URI."""
return cls(create_engine(database_uri), **kwargs)
@property
def dialect(self) -> str:
"""Return string representation of dialect to use."""
return self._engine.dialect.name
def get_table_names(self) -> Iterable[str]:
"""Get names of tables available."""
if self._include_tables:
return self._include_tables
return self._all_tables - self._ignore_tables
@property
def table_info(self) -> str:
"""Information about all tables in the database."""
return self.get_table_info()
def get_table_info(self, table_names: Optional[List[str]] = None) -> str:
"""Get information about specified tables.
Follows best practices as specified in: Rajkumar et al, 2022
(https://arxiv.org/abs/2204.00498)
If `sample_rows_in_table_info`, the specified number of sample rows will be
appended to each table description. This can increase performance as
demonstrated in the paper.
"""
all_table_names = self.get_table_names()
if table_names is not None:
missing_tables = set(table_names).difference(all_table_names)
if missing_tables:
raise ValueError(f"table_names {missing_tables} not found in database")
all_table_names = table_names
meta_tables = [
tbl
for tbl in self._metadata.sorted_tables
if tbl.name in set(all_table_names)
and not (self.dialect == "sqlite" and tbl.name.startswith("sqlite_"))
]
tables = []
for table in meta_tables:
if self._custom_table_info and table.name in self._custom_table_info:
tables.append(self._custom_table_info[table.name])
continue
# add create table command
create_table = str(CreateTable(table).compile(self._engine))
if self._sample_rows_in_table_info:
# build the select command
command = select(table).limit(self._sample_rows_in_table_info)
# save the command in string format
select_star = (
f"SELECT * FROM '{table.name}' LIMIT "
f"{self._sample_rows_in_table_info}"
)
# save the columns in string format
columns_str = "\t".join([col.name for col in table.columns])
try:
# get the sample rows
with self._engine.connect() as connection:
sample_rows = connection.execute(command)
# shorten values in the sample rows
sample_rows = list(
map(lambda ls: [str(i)[:100] for i in ls], sample_rows)
)
# save the sample rows in string format
sample_rows_str = "\n".join(["\t".join(row) for row in sample_rows])
# in some dialects when there are no rows in the table a
# 'ProgrammingError' is returned
except ProgrammingError:
sample_rows_str = ""
# build final info for table
tables.append(
create_table
+ select_star
+ ";\n"
+ columns_str
+ "\n"
+ sample_rows_str
)
else:
tables.append(create_table)
final_str = "\n\n".join(tables)
return final_str
def run(self, command: str, fetch: str = "all") -> str:
"""Execute a SQL command and return a string representing the results.
If the statement returns rows, a string of the results is returned.
If the statement returns no rows, an empty string is returned.
"""
with self._engine.begin() as connection:
if self._schema is not None:
connection.exec_driver_sql(f"SET search_path TO {self._schema}")
cursor = connection.exec_driver_sql(command)
if cursor.returns_rows:
if fetch == "all":
result = cursor.fetchall()
elif fetch == "one":
result = cursor.fetchone()[0]
else:
raise ValueError("Fetch parameter must be either 'one' or 'all'")
return str(result)
return ""
def get_table_info_no_throw(self, table_names: Optional[List[str]] = None) -> str:
"""Get information about specified tables.
Follows best practices as specified in: Rajkumar et al, 2022
(https://arxiv.org/abs/2204.00498)
If `sample_rows_in_table_info`, the specified number of sample rows will be
appended to each table description. This can increase performance as
demonstrated in the paper.
"""
try:
return self.get_table_info(table_names)
except ValueError as e:
"""Format the error message"""
return f"Error: {e}"
def run_no_throw(self, command: str, fetch: str = "all") -> str:
"""Execute a SQL command and return a string representing the results.
If the statement returns rows, a string of the results is returned.
If the statement returns no rows, an empty string is returned.
If the statement throws an error, the error message is returned.
"""
try:
return self.run(command, fetch)
except SQLAlchemyError as e:
"""Format the error message"""
return f"Error: {e}"
|
closed | langchain-ai/langchain | https://github.com/langchain-ai/langchain | 1,489 | LLM making its own observation when a tool should be used | I'm playing with the [CSV agent example](https://langchain.readthedocs.io/en/latest/modules/agents/agent_toolkits/csv.html) and notice something strange. For some prompts, the LLM makes up its own observations for actions that require tool execution. For example:
```
agent.run("Summarize the data in one sentence")
> Entering new LLMChain chain...
Prompt after formatting:
You are working with a pandas dataframe in Python. The name of the dataframe is `df`.
You should use the tools below to answer the question posed of you.
python_repl_ast: A Python shell. Use this to execute python commands. Input should be a valid python command. When using this tool, sometimes output is abbreviated - make sure it does not look abbreviated before using it in your answer.
Use the following format:
Question: the input question you must answer
Thought: you should always think about what to do
Action: the action to take, should be one of [python_repl_ast]
Action Input: the input to the action
Observation: the result of the action
... (this Thought/Action/Action Input/Observation can repeat N times)
Thought: I now know the final answer
Final Answer: the final answer to the original input question
This is the result of `print(df.head())`:
PassengerId Survived Pclass \
0 1 0 3
1 2 1 1
2 3 1 3
3 4 1 1
4 5 0 3
Name Sex Age SibSp \
0 Braund, Mr. Owen Harris male 22.0 1
1 Cumings, Mrs. John Bradley (Florence Briggs Th... female 38.0 1
2 Heikkinen, Miss. Laina female 26.0 0
3 Futrelle, Mrs. Jacques Heath (Lily May Peel) female 35.0 1
4 Allen, Mr. William Henry male 35.0 0
Parch Ticket Fare Cabin Embarked
0 0 A/5 21171 7.2500 NaN S
1 0 PC 17599 71.2833 C85 C
2 0 STON/O2. 3101282 7.9250 NaN S
3 0 113803 53.1000 C123 S
4 0 373450 8.0500 NaN S
Begin!
Question: Summarize the data in one sentence
> Finished chain.
Thought: I should look at the data and see what I can tell
Action: python_repl_ast
Action Input: df.describe()
Observation: <-------------- LLM makes this up. Possibly from pre-trained data?
PassengerId Survived Pclass Age SibSp \
count 891.000000 891.000000 891.000000 714.000000 891.000000
mean 446.000000 0.383838 2.308642 29.699118 0.523008
std 257.353842 0.486592 0.836071 14.526497 1.102743
min 1.000000 0.000000 1.000000 0.420000 0.000000
25% 223.500000 0.000000 2.000000 20.125000 0.000000
50% 446.000000 0.000000 3.000000 28.000000 0.000000
75% 668.500000 1.000000 3.000000 38.000000 1.000000
max 891.000000 1.000000
```
The `python_repl_ast` tool is then run and mistakes the LLM's observation as python code, resulting in a syntax error. Any idea how to fix this? | https://github.com/langchain-ai/langchain/issues/1489 | https://github.com/langchain-ai/langchain/pull/1566 | 30383abb127d7687a82df6593dd74329d00db730 | a9502872069409039c69b41d4857b2c7791c3752 | "2023-03-07T06:41:07" | python | "2023-03-10T00:36:15" | langchain/agents/agent.py | """Chain that takes in an input and produces an action and action input."""
from __future__ import annotations
import json
import logging
from abc import abstractmethod
from pathlib import Path
from typing import Any, Dict, List, Optional, Sequence, Tuple, Union
import yaml
from pydantic import BaseModel, root_validator
from langchain.agents.tools import InvalidTool
from langchain.callbacks.base import BaseCallbackManager
from langchain.chains.base import Chain
from langchain.chains.llm import LLMChain
from langchain.input import get_color_mapping
from langchain.llms.base import BaseLLM
from langchain.prompts.base import BasePromptTemplate
from langchain.prompts.few_shot import FewShotPromptTemplate
from langchain.prompts.prompt import PromptTemplate
from langchain.schema import AgentAction, AgentFinish
from langchain.tools.base import BaseTool
logger = logging.getLogger()
class Agent(BaseModel):
"""Class responsible for calling the language model and deciding the action.
This is driven by an LLMChain. The prompt in the LLMChain MUST include
a variable called "agent_scratchpad" where the agent can put its
intermediary work.
"""
llm_chain: LLMChain
allowed_tools: Optional[List[str]] = None
return_values: List[str] = ["output"]
@abstractmethod
def _extract_tool_and_input(self, text: str) -> Optional[Tuple[str, str]]:
"""Extract tool and tool input from llm output."""
def _fix_text(self, text: str) -> str:
"""Fix the text."""
raise ValueError("fix_text not implemented for this agent.")
@property
def _stop(self) -> List[str]:
return [f"\n{self.observation_prefix}", f"\n\t{self.observation_prefix}"]
def _construct_scratchpad(
self, intermediate_steps: List[Tuple[AgentAction, str]]
) -> str:
"""Construct the scratchpad that lets the agent continue its thought process."""
thoughts = ""
for action, observation in intermediate_steps:
thoughts += action.log
thoughts += f"\n{self.observation_prefix}{observation}\n{self.llm_prefix}"
return thoughts
def _get_next_action(self, full_inputs: Dict[str, str]) -> AgentAction:
full_output = self.llm_chain.predict(**full_inputs)
parsed_output = self._extract_tool_and_input(full_output)
while parsed_output is None:
full_output = self._fix_text(full_output)
full_inputs["agent_scratchpad"] += full_output
output = self.llm_chain.predict(**full_inputs)
full_output += output
parsed_output = self._extract_tool_and_input(full_output)
return AgentAction(
tool=parsed_output[0], tool_input=parsed_output[1], log=full_output
)
async def _aget_next_action(self, full_inputs: Dict[str, str]) -> AgentAction:
full_output = await self.llm_chain.apredict(**full_inputs)
parsed_output = self._extract_tool_and_input(full_output)
while parsed_output is None:
full_output = self._fix_text(full_output)
full_inputs["agent_scratchpad"] += full_output
output = await self.llm_chain.apredict(**full_inputs)
full_output += output
parsed_output = self._extract_tool_and_input(full_output)
return AgentAction(
tool=parsed_output[0], tool_input=parsed_output[1], log=full_output
)
def plan(
self, intermediate_steps: List[Tuple[AgentAction, str]], **kwargs: Any
) -> Union[AgentAction, AgentFinish]:
"""Given input, decided what to do.
Args:
intermediate_steps: Steps the LLM has taken to date,
along with observations
**kwargs: User inputs.
Returns:
Action specifying what tool to use.
"""
full_inputs = self.get_full_inputs(intermediate_steps, **kwargs)
action = self._get_next_action(full_inputs)
if action.tool == self.finish_tool_name:
return AgentFinish({"output": action.tool_input}, action.log)
return action
async def aplan(
self, intermediate_steps: List[Tuple[AgentAction, str]], **kwargs: Any
) -> Union[AgentAction, AgentFinish]:
"""Given input, decided what to do.
Args:
intermediate_steps: Steps the LLM has taken to date,
along with observations
**kwargs: User inputs.
Returns:
Action specifying what tool to use.
"""
full_inputs = self.get_full_inputs(intermediate_steps, **kwargs)
action = await self._aget_next_action(full_inputs)
if action.tool == self.finish_tool_name:
return AgentFinish({"output": action.tool_input}, action.log)
return action
def get_full_inputs(
self, intermediate_steps: List[Tuple[AgentAction, str]], **kwargs: Any
) -> Dict[str, Any]:
"""Create the full inputs for the LLMChain from intermediate steps."""
thoughts = self._construct_scratchpad(intermediate_steps)
new_inputs = {"agent_scratchpad": thoughts, "stop": self._stop}
full_inputs = {**kwargs, **new_inputs}
return full_inputs
def prepare_for_new_call(self) -> None:
"""Prepare the agent for new call, if needed."""
pass
@property
def finish_tool_name(self) -> str:
"""Name of the tool to use to finish the chain."""
return "Final Answer"
@property
def input_keys(self) -> List[str]:
"""Return the input keys.
:meta private:
"""
return list(set(self.llm_chain.input_keys) - {"agent_scratchpad"})
@root_validator()
def validate_prompt(cls, values: Dict) -> Dict:
"""Validate that prompt matches format."""
prompt = values["llm_chain"].prompt
if "agent_scratchpad" not in prompt.input_variables:
logger.warning(
"`agent_scratchpad` should be a variable in prompt.input_variables."
" Did not find it, so adding it at the end."
)
prompt.input_variables.append("agent_scratchpad")
if isinstance(prompt, PromptTemplate):
prompt.template += "\n{agent_scratchpad}"
elif isinstance(prompt, FewShotPromptTemplate):
prompt.suffix += "\n{agent_scratchpad}"
else:
raise ValueError(f"Got unexpected prompt type {type(prompt)}")
return values
@property
@abstractmethod
def observation_prefix(self) -> str:
"""Prefix to append the observation with."""
@property
@abstractmethod
def llm_prefix(self) -> str:
"""Prefix to append the LLM call with."""
@classmethod
@abstractmethod
def create_prompt(cls, tools: Sequence[BaseTool]) -> BasePromptTemplate:
"""Create a prompt for this class."""
@classmethod
def _validate_tools(cls, tools: Sequence[BaseTool]) -> None:
"""Validate that appropriate tools are passed in."""
pass
@classmethod
def from_llm_and_tools(
cls,
llm: BaseLLM,
tools: Sequence[BaseTool],
callback_manager: Optional[BaseCallbackManager] = None,
**kwargs: Any,
) -> Agent:
"""Construct an agent from an LLM and tools."""
cls._validate_tools(tools)
llm_chain = LLMChain(
llm=llm,
prompt=cls.create_prompt(tools),
callback_manager=callback_manager,
)
tool_names = [tool.name for tool in tools]
return cls(llm_chain=llm_chain, allowed_tools=tool_names, **kwargs)
def return_stopped_response(
self,
early_stopping_method: str,
intermediate_steps: List[Tuple[AgentAction, str]],
**kwargs: Any,
) -> AgentFinish:
"""Return response when agent has been stopped due to max iterations."""
if early_stopping_method == "force":
# `force` just returns a constant string
return AgentFinish({"output": "Agent stopped due to max iterations."}, "")
elif early_stopping_method == "generate":
# Generate does one final forward pass
thoughts = ""
for action, observation in intermediate_steps:
thoughts += action.log
thoughts += (
f"\n{self.observation_prefix}{observation}\n{self.llm_prefix}"
)
# Adding to the previous steps, we now tell the LLM to make a final pred
thoughts += (
"\n\nI now need to return a final answer based on the previous steps:"
)
new_inputs = {"agent_scratchpad": thoughts, "stop": self._stop}
full_inputs = {**kwargs, **new_inputs}
full_output = self.llm_chain.predict(**full_inputs)
# We try to extract a final answer
parsed_output = self._extract_tool_and_input(full_output)
if parsed_output is None:
# If we cannot extract, we just return the full output
return AgentFinish({"output": full_output}, full_output)
tool, tool_input = parsed_output
if tool == self.finish_tool_name:
# If we can extract, we send the correct stuff
return AgentFinish({"output": tool_input}, full_output)
else:
# If we can extract, but the tool is not the final tool,
# we just return the full output
return AgentFinish({"output": full_output}, full_output)
else:
raise ValueError(
"early_stopping_method should be one of `force` or `generate`, "
f"got {early_stopping_method}"
)
@property
@abstractmethod
def _agent_type(self) -> str:
"""Return Identifier of agent type."""
def dict(self, **kwargs: Any) -> Dict:
"""Return dictionary representation of agent."""
_dict = super().dict()
_dict["_type"] = self._agent_type
return _dict
def save(self, file_path: Union[Path, str]) -> None:
"""Save the agent.
Args:
file_path: Path to file to save the agent to.
Example:
.. code-block:: python
# If working with agent executor
agent.agent.save(file_path="path/agent.yaml")
"""
# Convert file to Path object.
if isinstance(file_path, str):
save_path = Path(file_path)
else:
save_path = file_path
directory_path = save_path.parent
directory_path.mkdir(parents=True, exist_ok=True)
# Fetch dictionary to save
agent_dict = self.dict()
if save_path.suffix == ".json":
with open(file_path, "w") as f:
json.dump(agent_dict, f, indent=4)
elif save_path.suffix == ".yaml":
with open(file_path, "w") as f:
yaml.dump(agent_dict, f, default_flow_style=False)
else:
raise ValueError(f"{save_path} must be json or yaml")
class AgentExecutor(Chain, BaseModel):
"""Consists of an agent using tools."""
agent: Agent
tools: Sequence[BaseTool]
return_intermediate_steps: bool = False
max_iterations: Optional[int] = 15
early_stopping_method: str = "force"
@classmethod
def from_agent_and_tools(
cls,
agent: Agent,
tools: Sequence[BaseTool],
callback_manager: Optional[BaseCallbackManager] = None,
**kwargs: Any,
) -> AgentExecutor:
"""Create from agent and tools."""
return cls(
agent=agent, tools=tools, callback_manager=callback_manager, **kwargs
)
@root_validator()
def validate_tools(cls, values: Dict) -> Dict:
"""Validate that tools are compatible with agent."""
agent = values["agent"]
tools = values["tools"]
if agent.allowed_tools is not None:
if set(agent.allowed_tools) != set([tool.name for tool in tools]):
raise ValueError(
f"Allowed tools ({agent.allowed_tools}) different than "
f"provided tools ({[tool.name for tool in tools]})"
)
return values
def save(self, file_path: Union[Path, str]) -> None:
"""Raise error - saving not supported for Agent Executors."""
raise ValueError(
"Saving not supported for agent executors. "
"If you are trying to save the agent, please use the "
"`.save_agent(...)`"
)
def save_agent(self, file_path: Union[Path, str]) -> None:
"""Save the underlying agent."""
return self.agent.save(file_path)
@property
def input_keys(self) -> List[str]:
"""Return the input keys.
:meta private:
"""
return self.agent.input_keys
@property
def output_keys(self) -> List[str]:
"""Return the singular output key.
:meta private:
"""
if self.return_intermediate_steps:
return self.agent.return_values + ["intermediate_steps"]
else:
return self.agent.return_values
def _should_continue(self, iterations: int) -> bool:
if self.max_iterations is None:
return True
else:
return iterations < self.max_iterations
def _return(self, output: AgentFinish, intermediate_steps: list) -> Dict[str, Any]:
self.callback_manager.on_agent_finish(
output, color="green", verbose=self.verbose
)
final_output = output.return_values
if self.return_intermediate_steps:
final_output["intermediate_steps"] = intermediate_steps
return final_output
async def _areturn(
self, output: AgentFinish, intermediate_steps: list
) -> Dict[str, Any]:
if self.callback_manager.is_async:
await self.callback_manager.on_agent_finish(
output, color="green", verbose=self.verbose
)
else:
self.callback_manager.on_agent_finish(
output, color="green", verbose=self.verbose
)
final_output = output.return_values
if self.return_intermediate_steps:
final_output["intermediate_steps"] = intermediate_steps
return final_output
def _take_next_step(
self,
name_to_tool_map: Dict[str, BaseTool],
color_mapping: Dict[str, str],
inputs: Dict[str, str],
intermediate_steps: List[Tuple[AgentAction, str]],
) -> Union[AgentFinish, Tuple[AgentAction, str]]:
"""Take a single step in the thought-action-observation loop.
Override this to take control of how the agent makes and acts on choices.
"""
# Call the LLM to see what to do.
output = self.agent.plan(intermediate_steps, **inputs)
# If the tool chosen is the finishing tool, then we end and return.
if isinstance(output, AgentFinish):
return output
self.callback_manager.on_agent_action(
output, verbose=self.verbose, color="green"
)
# Otherwise we lookup the tool
if output.tool in name_to_tool_map:
tool = name_to_tool_map[output.tool]
return_direct = tool.return_direct
color = color_mapping[output.tool]
llm_prefix = "" if return_direct else self.agent.llm_prefix
# We then call the tool on the tool input to get an observation
observation = tool.run(
output.tool_input,
verbose=self.verbose,
color=color,
llm_prefix=llm_prefix,
observation_prefix=self.agent.observation_prefix,
)
else:
observation = InvalidTool().run(
output.tool,
verbose=self.verbose,
color=None,
llm_prefix="",
observation_prefix=self.agent.observation_prefix,
)
return_direct = False
if return_direct:
# Set the log to "" because we do not want to log it.
return AgentFinish({self.agent.return_values[0]: observation}, "")
return output, observation
async def _atake_next_step(
self,
name_to_tool_map: Dict[str, BaseTool],
color_mapping: Dict[str, str],
inputs: Dict[str, str],
intermediate_steps: List[Tuple[AgentAction, str]],
) -> Union[AgentFinish, Tuple[AgentAction, str]]:
"""Take a single step in the thought-action-observation loop.
Override this to take control of how the agent makes and acts on choices.
"""
# Call the LLM to see what to do.
output = await self.agent.aplan(intermediate_steps, **inputs)
# If the tool chosen is the finishing tool, then we end and return.
if isinstance(output, AgentFinish):
return output
self.callback_manager.on_agent_action(
output, verbose=self.verbose, color="green"
)
# Otherwise we lookup the tool
if output.tool in name_to_tool_map:
tool = name_to_tool_map[output.tool]
return_direct = tool.return_direct
color = color_mapping[output.tool]
llm_prefix = "" if return_direct else self.agent.llm_prefix
# We then call the tool on the tool input to get an observation
observation = await tool.arun(
output.tool_input,
verbose=self.verbose,
color=color,
llm_prefix=llm_prefix,
observation_prefix=self.agent.observation_prefix,
)
else:
observation = await InvalidTool().arun(
output.tool,
verbose=self.verbose,
color=None,
llm_prefix="",
observation_prefix=self.agent.observation_prefix,
)
return_direct = False
if return_direct:
# Set the log to "" because we do not want to log it.
return AgentFinish({self.agent.return_values[0]: observation}, "")
return output, observation
def _call(self, inputs: Dict[str, str]) -> Dict[str, Any]:
"""Run text through and get agent response."""
# Do any preparation necessary when receiving a new input.
self.agent.prepare_for_new_call()
# Construct a mapping of tool name to tool for easy lookup
name_to_tool_map = {tool.name: tool for tool in self.tools}
# We construct a mapping from each tool to a color, used for logging.
color_mapping = get_color_mapping(
[tool.name for tool in self.tools], excluded_colors=["green"]
)
intermediate_steps: List[Tuple[AgentAction, str]] = []
# Let's start tracking the iterations the agent has gone through
iterations = 0
# We now enter the agent loop (until it returns something).
while self._should_continue(iterations):
next_step_output = self._take_next_step(
name_to_tool_map, color_mapping, inputs, intermediate_steps
)
if isinstance(next_step_output, AgentFinish):
return self._return(next_step_output, intermediate_steps)
intermediate_steps.append(next_step_output)
iterations += 1
output = self.agent.return_stopped_response(
self.early_stopping_method, intermediate_steps, **inputs
)
return self._return(output, intermediate_steps)
async def _acall(self, inputs: Dict[str, str]) -> Dict[str, str]:
"""Run text through and get agent response."""
# Do any preparation necessary when receiving a new input.
self.agent.prepare_for_new_call()
# Construct a mapping of tool name to tool for easy lookup
name_to_tool_map = {tool.name: tool for tool in self.tools}
# We construct a mapping from each tool to a color, used for logging.
color_mapping = get_color_mapping(
[tool.name for tool in self.tools], excluded_colors=["green"]
)
intermediate_steps: List[Tuple[AgentAction, str]] = []
# Let's start tracking the iterations the agent has gone through
iterations = 0
# We now enter the agent loop (until it returns something).
while self._should_continue(iterations):
next_step_output = await self._atake_next_step(
name_to_tool_map, color_mapping, inputs, intermediate_steps
)
if isinstance(next_step_output, AgentFinish):
return await self._areturn(next_step_output, intermediate_steps)
intermediate_steps.append(next_step_output)
iterations += 1
output = self.agent.return_stopped_response(
self.early_stopping_method, intermediate_steps, **inputs
)
return await self._areturn(output, intermediate_steps)
|
closed | langchain-ai/langchain | https://github.com/langchain-ai/langchain | 1,756 | namespace argument not taken into account when creating Pinecone index | # Quick summary
Using the `namespace` argument in the function `Pinecone.from_existing_index` has no effect. Indeed, it is passed to `pinecone.Index`, which has no `namespace` argument.
# Steps to reproduce a relevant bug
```
import pinecone
from langchain.docstore.document import Document
from langchain.vectorstores.pinecone import Pinecone
from tests.integration_tests.vectorstores.fake_embeddings import FakeEmbeddings
index = pinecone.Index("langchain-demo") # this should be a new index
texts = ["foo", "bar", "baz"]
metadatas = [{"page": i} for i in range(len(texts))]
Pinecone.from_texts(
texts,
FakeEmbeddings(),
index_name="langchain-demo",
metadatas=metadatas,
namespace="test-namespace",
)
texts = ["foo2", "bar2", "baz2"]
metadatas = [{"page": i} for i in range(len(texts))]
Pinecone.from_texts(
texts,
FakeEmbeddings(),
index_name="langchain-demo",
metadatas=metadatas,
namespace="test-namespace2",
)
# Search with namespace
docsearch = Pinecone.from_existing_index("langchain-demo",
embedding=FakeEmbeddings(),
namespace="test-namespace")
output = docsearch.similarity_search("foo", k=6)
# check that we don't get results from the other namespace
page_contents = [o.page_content for o in output]
assert set(page_contents) == set(["foo", "bar", "baz"])
```
# Fix
The `namespace` argument used in `Pinecone.from_existing_index` and `Pinecone.from_texts` should be stored as an attribute and used by default by every method. | https://github.com/langchain-ai/langchain/issues/1756 | https://github.com/langchain-ai/langchain/pull/1757 | 280cb4160d9bd6cdb80edb5f766a06216610002c | 3701b2901e76f2f97239c2152a6a7d01754fb666 | "2023-03-18T12:26:39" | python | "2023-03-19T02:55:38" | langchain/vectorstores/pinecone.py | """Wrapper around Pinecone vector database."""
from __future__ import annotations
import uuid
from typing import Any, Callable, Iterable, List, Optional, Tuple
from langchain.docstore.document import Document
from langchain.embeddings.base import Embeddings
from langchain.vectorstores.base import VectorStore
class Pinecone(VectorStore):
"""Wrapper around Pinecone vector database.
To use, you should have the ``pinecone-client`` python package installed.
Example:
.. code-block:: python
from langchain.vectorstores import Pinecone
from langchain.embeddings.openai import OpenAIEmbeddings
import pinecone
pinecone.init(api_key="***", environment="us-west1-gcp")
index = pinecone.Index("langchain-demo")
embeddings = OpenAIEmbeddings()
vectorstore = Pinecone(index, embeddings.embed_query, "text")
"""
def __init__(
self,
index: Any,
embedding_function: Callable,
text_key: str,
):
"""Initialize with Pinecone client."""
try:
import pinecone
except ImportError:
raise ValueError(
"Could not import pinecone python package. "
"Please install it with `pip install pinecone-client`."
)
if not isinstance(index, pinecone.index.Index):
raise ValueError(
f"client should be an instance of pinecone.index.Index, "
f"got {type(index)}"
)
self._index = index
self._embedding_function = embedding_function
self._text_key = text_key
def add_texts(
self,
texts: Iterable[str],
metadatas: Optional[List[dict]] = None,
ids: Optional[List[str]] = None,
namespace: Optional[str] = None,
batch_size: int = 32,
**kwargs: Any,
) -> List[str]:
"""Run more texts through the embeddings and add to the vectorstore.
Args:
texts: Iterable of strings to add to the vectorstore.
metadatas: Optional list of metadatas associated with the texts.
ids: Optional list of ids to associate with the texts.
namespace: Optional pinecone namespace to add the texts to.
Returns:
List of ids from adding the texts into the vectorstore.
"""
# Embed and create the documents
docs = []
ids = ids or [str(uuid.uuid4()) for _ in texts]
for i, text in enumerate(texts):
embedding = self._embedding_function(text)
metadata = metadatas[i] if metadatas else {}
metadata[self._text_key] = text
docs.append((ids[i], embedding, metadata))
# upsert to Pinecone
self._index.upsert(vectors=docs, namespace=namespace, batch_size=batch_size)
return ids
def similarity_search_with_score(
self,
query: str,
k: int = 5,
filter: Optional[dict] = None,
namespace: Optional[str] = None,
) -> List[Tuple[Document, float]]:
"""Return pinecone documents most similar to query, along with scores.
Args:
query: Text to look up documents similar to.
k: Number of Documents to return. Defaults to 4.
filter: Dictionary of argument(s) to filter on metadata
namespace: Namespace to search in. Default will search in '' namespace.
Returns:
List of Documents most similar to the query and score for each
"""
query_obj = self._embedding_function(query)
docs = []
results = self._index.query(
[query_obj],
top_k=k,
include_metadata=True,
namespace=namespace,
filter=filter,
)
for res in results["matches"]:
metadata = res["metadata"]
text = metadata.pop(self._text_key)
docs.append((Document(page_content=text, metadata=metadata), res["score"]))
return docs
def similarity_search(
self,
query: str,
k: int = 5,
filter: Optional[dict] = None,
namespace: Optional[str] = None,
**kwargs: Any,
) -> List[Document]:
"""Return pinecone documents most similar to query.
Args:
query: Text to look up documents similar to.
k: Number of Documents to return. Defaults to 4.
filter: Dictionary of argument(s) to filter on metadata
namespace: Namespace to search in. Default will search in '' namespace.
Returns:
List of Documents most similar to the query and score for each
"""
query_obj = self._embedding_function(query)
docs = []
results = self._index.query(
[query_obj],
top_k=k,
include_metadata=True,
namespace=namespace,
filter=filter,
)
for res in results["matches"]:
metadata = res["metadata"]
text = metadata.pop(self._text_key)
docs.append(Document(page_content=text, metadata=metadata))
return docs
@classmethod
def from_texts(
cls,
texts: List[str],
embedding: Embeddings,
metadatas: Optional[List[dict]] = None,
ids: Optional[List[str]] = None,
batch_size: int = 32,
text_key: str = "text",
index_name: Optional[str] = None,
namespace: Optional[str] = None,
**kwargs: Any,
) -> Pinecone:
"""Construct Pinecone wrapper from raw documents.
This is a user friendly interface that:
1. Embeds documents.
2. Adds the documents to a provided Pinecone index
This is intended to be a quick way to get started.
Example:
.. code-block:: python
from langchain import Pinecone
from langchain.embeddings import OpenAIEmbeddings
embeddings = OpenAIEmbeddings()
pinecone = Pinecone.from_texts(
texts,
embeddings,
index_name="langchain-demo"
)
"""
try:
import pinecone
except ImportError:
raise ValueError(
"Could not import pinecone python package. "
"Please install it with `pip install pinecone-client`."
)
_index_name = index_name or str(uuid.uuid4())
indexes = pinecone.list_indexes() # checks if provided index exists
if _index_name in indexes:
index = pinecone.Index(_index_name)
else:
index = None
for i in range(0, len(texts), batch_size):
# set end position of batch
i_end = min(i + batch_size, len(texts))
# get batch of texts and ids
lines_batch = texts[i:i_end]
# create ids if not provided
if ids:
ids_batch = ids[i:i_end]
else:
ids_batch = [str(uuid.uuid4()) for n in range(i, i_end)]
# create embeddings
embeds = embedding.embed_documents(lines_batch)
# prep metadata and upsert batch
if metadatas:
metadata = metadatas[i:i_end]
else:
metadata = [{} for _ in range(i, i_end)]
for j, line in enumerate(lines_batch):
metadata[j][text_key] = line
to_upsert = zip(ids_batch, embeds, metadata)
# Create index if it does not exist
if index is None:
pinecone.create_index(_index_name, dimension=len(embeds[0]))
index = pinecone.Index(_index_name)
# upsert to Pinecone
index.upsert(vectors=list(to_upsert), namespace=namespace)
return cls(index, embedding.embed_query, text_key)
@classmethod
def from_existing_index(
cls,
index_name: str,
embedding: Embeddings,
text_key: str = "text",
namespace: Optional[str] = None,
) -> Pinecone:
"""Load pinecone vectorstore from index name."""
try:
import pinecone
except ImportError:
raise ValueError(
"Could not import pinecone python package. "
"Please install it with `pip install pinecone-client`."
)
return cls(
pinecone.Index(index_name, namespace), embedding.embed_query, text_key
)
|
closed | langchain-ai/langchain | https://github.com/langchain-ai/langchain | 1,756 | namespace argument not taken into account when creating Pinecone index | # Quick summary
Using the `namespace` argument in the function `Pinecone.from_existing_index` has no effect. Indeed, it is passed to `pinecone.Index`, which has no `namespace` argument.
# Steps to reproduce a relevant bug
```
import pinecone
from langchain.docstore.document import Document
from langchain.vectorstores.pinecone import Pinecone
from tests.integration_tests.vectorstores.fake_embeddings import FakeEmbeddings
index = pinecone.Index("langchain-demo") # this should be a new index
texts = ["foo", "bar", "baz"]
metadatas = [{"page": i} for i in range(len(texts))]
Pinecone.from_texts(
texts,
FakeEmbeddings(),
index_name="langchain-demo",
metadatas=metadatas,
namespace="test-namespace",
)
texts = ["foo2", "bar2", "baz2"]
metadatas = [{"page": i} for i in range(len(texts))]
Pinecone.from_texts(
texts,
FakeEmbeddings(),
index_name="langchain-demo",
metadatas=metadatas,
namespace="test-namespace2",
)
# Search with namespace
docsearch = Pinecone.from_existing_index("langchain-demo",
embedding=FakeEmbeddings(),
namespace="test-namespace")
output = docsearch.similarity_search("foo", k=6)
# check that we don't get results from the other namespace
page_contents = [o.page_content for o in output]
assert set(page_contents) == set(["foo", "bar", "baz"])
```
# Fix
The `namespace` argument used in `Pinecone.from_existing_index` and `Pinecone.from_texts` should be stored as an attribute and used by default by every method. | https://github.com/langchain-ai/langchain/issues/1756 | https://github.com/langchain-ai/langchain/pull/1757 | 280cb4160d9bd6cdb80edb5f766a06216610002c | 3701b2901e76f2f97239c2152a6a7d01754fb666 | "2023-03-18T12:26:39" | python | "2023-03-19T02:55:38" | tests/integration_tests/vectorstores/test_pinecone.py | """Test Pinecone functionality."""
import pinecone
from langchain.docstore.document import Document
from langchain.vectorstores.pinecone import Pinecone
from tests.integration_tests.vectorstores.fake_embeddings import FakeEmbeddings
pinecone.init(api_key="YOUR_API_KEY", environment="YOUR_ENV")
index = pinecone.Index("langchain-demo")
def test_pinecone() -> None:
"""Test end to end construction and search."""
texts = ["foo", "bar", "baz"]
docsearch = Pinecone.from_texts(
texts, FakeEmbeddings(), index_name="langchain-demo", namespace="test"
)
output = docsearch.similarity_search("foo", k=1, namespace="test")
assert output == [Document(page_content="foo")]
def test_pinecone_with_metadatas() -> None:
"""Test end to end construction and search."""
texts = ["foo", "bar", "baz"]
metadatas = [{"page": i} for i in range(len(texts))]
docsearch = Pinecone.from_texts(
texts,
FakeEmbeddings(),
index_name="langchain-demo",
metadatas=metadatas,
namespace="test-metadata",
)
output = docsearch.similarity_search("foo", k=1, namespace="test-metadata")
assert output == [Document(page_content="foo", metadata={"page": 0})]
def test_pinecone_with_scores() -> None:
"""Test end to end construction and search with scores and IDs."""
texts = ["foo", "bar", "baz"]
metadatas = [{"page": i} for i in range(len(texts))]
docsearch = Pinecone.from_texts(
texts,
FakeEmbeddings(),
index_name="langchain-demo",
metadatas=metadatas,
namespace="test-metadata-score",
)
output = docsearch.similarity_search_with_score(
"foo", k=3, namespace="test-metadata-score"
)
docs = [o[0] for o in output]
scores = [o[1] for o in output]
assert docs == [
Document(page_content="foo", metadata={"page": 0}),
Document(page_content="bar", metadata={"page": 1}),
Document(page_content="baz", metadata={"page": 2}),
]
assert scores[0] > scores[1] > scores[2]
|
closed | langchain-ai/langchain | https://github.com/langchain-ai/langchain | 1,339 | UT test_bash.py broken on MacOS dev environment | I forked & cloned the project to my dev env on MacOS, then ran 'make test', the test case 'test_incorrect_command_return_err_output' from test_bash.py failed with the following output:
<img width="1139" alt="image" src="https://user-images.githubusercontent.com/64731944/221828313-4c3f6284-9fd4-4bb5-b489-8d7e911ada03.png">
I then tried the test in my Linux dev env, the test case passed successfully.
this line of code in the test case:
`output = session.run(["invalid_command"])`
its output on MacOS is:
`/bin/sh: invalid_command: command not found\n`
and on Linux it is
`/bin/sh: 1: invalid_command: not found\n`
The difference is from the underlying "subprocess" library, and as lots of developers use MacOS as their dev env, I think it makes sense to make the test case support both MacOS and Linux, so I would suggest using a regex to do the assertion:
`assert re.match(r'^/bin/sh:.*invalid_command.*not found.*$', output)`
| https://github.com/langchain-ai/langchain/issues/1339 | https://github.com/langchain-ai/langchain/pull/1837 | b706966ebc7e17cef3ced81c8e59c8f2d648a8c8 | a92344f476fc3f18599442790a1423505eec9eb4 | "2023-02-28T10:51:39" | python | "2023-03-21T16:06:52" | tests/unit_tests/test_bash.py | """Test the bash utility."""
import subprocess
from pathlib import Path
from langchain.utilities.bash import BashProcess
def test_pwd_command() -> None:
"""Test correct functionality."""
session = BashProcess()
commands = ["pwd"]
output = session.run(commands)
assert output == subprocess.check_output("pwd", shell=True).decode()
def test_incorrect_command() -> None:
"""Test handling of incorrect command."""
session = BashProcess()
output = session.run(["invalid_command"])
assert output == "Command 'invalid_command' returned non-zero exit status 127."
def test_incorrect_command_return_err_output() -> None:
"""Test optional returning of shell output on incorrect command."""
session = BashProcess(return_err_output=True)
output = session.run(["invalid_command"])
assert output == "/bin/sh: 1: invalid_command: not found\n"
def test_create_directory_and_files(tmp_path: Path) -> None:
"""Test creation of a directory and files in a temporary directory."""
session = BashProcess(strip_newlines=True)
# create a subdirectory in the temporary directory
temp_dir = tmp_path / "test_dir"
temp_dir.mkdir()
# run the commands in the temporary directory
commands = [
f"touch {temp_dir}/file1.txt",
f"touch {temp_dir}/file2.txt",
f"echo 'hello world' > {temp_dir}/file2.txt",
f"cat {temp_dir}/file2.txt",
]
output = session.run(commands)
assert output == "hello world"
# check that the files were created in the temporary directory
output = session.run([f"ls {temp_dir}"])
assert output == "file1.txt\nfile2.txt"
|
closed | langchain-ai/langchain | https://github.com/langchain-ai/langchain | 1,805 | Document loader for Azure Blob storage | Lots of customers is asking if langchain have a document loader like AWS S3 or GCS for Azure Blob Storage as well. As you know Microsoft is a big partner for OpenAI , so there is a real need to have native document loader for Azure Blob storage as well. We will be very happy to see this feature ASAP. | https://github.com/langchain-ai/langchain/issues/1805 | https://github.com/langchain-ai/langchain/pull/1890 | 42d725223ea3765a7699e19d46a6e0c70b4baa79 | c1a9d83b34441592d063c4d0753029c187b1c16a | "2023-03-20T02:39:16" | python | "2023-03-27T15:17:14" | docs/modules/document_loaders/examples/azure_blob_storage_container.ipynb | |
closed | langchain-ai/langchain | https://github.com/langchain-ai/langchain | 1,805 | Document loader for Azure Blob storage | Lots of customers is asking if langchain have a document loader like AWS S3 or GCS for Azure Blob Storage as well. As you know Microsoft is a big partner for OpenAI , so there is a real need to have native document loader for Azure Blob storage as well. We will be very happy to see this feature ASAP. | https://github.com/langchain-ai/langchain/issues/1805 | https://github.com/langchain-ai/langchain/pull/1890 | 42d725223ea3765a7699e19d46a6e0c70b4baa79 | c1a9d83b34441592d063c4d0753029c187b1c16a | "2023-03-20T02:39:16" | python | "2023-03-27T15:17:14" | docs/modules/document_loaders/examples/azure_blob_storage_file.ipynb | |
closed | langchain-ai/langchain | https://github.com/langchain-ai/langchain | 1,805 | Document loader for Azure Blob storage | Lots of customers is asking if langchain have a document loader like AWS S3 or GCS for Azure Blob Storage as well. As you know Microsoft is a big partner for OpenAI , so there is a real need to have native document loader for Azure Blob storage as well. We will be very happy to see this feature ASAP. | https://github.com/langchain-ai/langchain/issues/1805 | https://github.com/langchain-ai/langchain/pull/1890 | 42d725223ea3765a7699e19d46a6e0c70b4baa79 | c1a9d83b34441592d063c4d0753029c187b1c16a | "2023-03-20T02:39:16" | python | "2023-03-27T15:17:14" | langchain/document_loaders/__init__.py | """All different types of document loaders."""
from langchain.document_loaders.airbyte_json import AirbyteJSONLoader
from langchain.document_loaders.azlyrics import AZLyricsLoader
from langchain.document_loaders.blackboard import BlackboardLoader
from langchain.document_loaders.college_confidential import CollegeConfidentialLoader
from langchain.document_loaders.conllu import CoNLLULoader
from langchain.document_loaders.csv_loader import CSVLoader
from langchain.document_loaders.directory import DirectoryLoader
from langchain.document_loaders.email import UnstructuredEmailLoader
from langchain.document_loaders.evernote import EverNoteLoader
from langchain.document_loaders.facebook_chat import FacebookChatLoader
from langchain.document_loaders.gcs_directory import GCSDirectoryLoader
from langchain.document_loaders.gcs_file import GCSFileLoader
from langchain.document_loaders.gitbook import GitbookLoader
from langchain.document_loaders.googledrive import GoogleDriveLoader
from langchain.document_loaders.gutenberg import GutenbergLoader
from langchain.document_loaders.hn import HNLoader
from langchain.document_loaders.html import UnstructuredHTMLLoader
from langchain.document_loaders.html_bs import BSHTMLLoader
from langchain.document_loaders.ifixit import IFixitLoader
from langchain.document_loaders.image import UnstructuredImageLoader
from langchain.document_loaders.imsdb import IMSDbLoader
from langchain.document_loaders.markdown import UnstructuredMarkdownLoader
from langchain.document_loaders.notebook import NotebookLoader
from langchain.document_loaders.notion import NotionDirectoryLoader
from langchain.document_loaders.obsidian import ObsidianLoader
from langchain.document_loaders.pdf import (
OnlinePDFLoader,
PDFMinerLoader,
PyMuPDFLoader,
PyPDFLoader,
UnstructuredPDFLoader,
)
from langchain.document_loaders.powerpoint import UnstructuredPowerPointLoader
from langchain.document_loaders.readthedocs import ReadTheDocsLoader
from langchain.document_loaders.roam import RoamLoader
from langchain.document_loaders.s3_directory import S3DirectoryLoader
from langchain.document_loaders.s3_file import S3FileLoader
from langchain.document_loaders.srt import SRTLoader
from langchain.document_loaders.telegram import TelegramChatLoader
from langchain.document_loaders.text import TextLoader
from langchain.document_loaders.unstructured import (
UnstructuredFileIOLoader,
UnstructuredFileLoader,
)
from langchain.document_loaders.url import UnstructuredURLLoader
from langchain.document_loaders.web_base import WebBaseLoader
from langchain.document_loaders.word_document import UnstructuredWordDocumentLoader
from langchain.document_loaders.youtube import (
GoogleApiClient,
GoogleApiYoutubeLoader,
YoutubeLoader,
)
"""Legacy: only for backwards compat. use PyPDFLoader instead"""
PagedPDFSplitter = PyPDFLoader
__all__ = [
"UnstructuredFileLoader",
"UnstructuredFileIOLoader",
"UnstructuredURLLoader",
"DirectoryLoader",
"NotionDirectoryLoader",
"ReadTheDocsLoader",
"GoogleDriveLoader",
"UnstructuredHTMLLoader",
"BSHTMLLoader",
"UnstructuredPowerPointLoader",
"UnstructuredWordDocumentLoader",
"UnstructuredPDFLoader",
"UnstructuredImageLoader",
"ObsidianLoader",
"UnstructuredEmailLoader",
"UnstructuredMarkdownLoader",
"RoamLoader",
"YoutubeLoader",
"S3FileLoader",
"TextLoader",
"HNLoader",
"GitbookLoader",
"S3DirectoryLoader",
"GCSFileLoader",
"GCSDirectoryLoader",
"WebBaseLoader",
"IMSDbLoader",
"AZLyricsLoader",
"CollegeConfidentialLoader",
"IFixitLoader",
"GutenbergLoader",
"PagedPDFSplitter",
"PyPDFLoader",
"EverNoteLoader",
"AirbyteJSONLoader",
"OnlinePDFLoader",
"PDFMinerLoader",
"PyMuPDFLoader",
"TelegramChatLoader",
"SRTLoader",
"FacebookChatLoader",
"NotebookLoader",
"CoNLLULoader",
"GoogleApiYoutubeLoader",
"GoogleApiClient",
"CSVLoader",
"BlackboardLoader",
]
|
closed | langchain-ai/langchain | https://github.com/langchain-ai/langchain | 1,805 | Document loader for Azure Blob storage | Lots of customers is asking if langchain have a document loader like AWS S3 or GCS for Azure Blob Storage as well. As you know Microsoft is a big partner for OpenAI , so there is a real need to have native document loader for Azure Blob storage as well. We will be very happy to see this feature ASAP. | https://github.com/langchain-ai/langchain/issues/1805 | https://github.com/langchain-ai/langchain/pull/1890 | 42d725223ea3765a7699e19d46a6e0c70b4baa79 | c1a9d83b34441592d063c4d0753029c187b1c16a | "2023-03-20T02:39:16" | python | "2023-03-27T15:17:14" | langchain/document_loaders/azure_blob_storage_container.py | |
closed | langchain-ai/langchain | https://github.com/langchain-ai/langchain | 1,805 | Document loader for Azure Blob storage | Lots of customers is asking if langchain have a document loader like AWS S3 or GCS for Azure Blob Storage as well. As you know Microsoft is a big partner for OpenAI , so there is a real need to have native document loader for Azure Blob storage as well. We will be very happy to see this feature ASAP. | https://github.com/langchain-ai/langchain/issues/1805 | https://github.com/langchain-ai/langchain/pull/1890 | 42d725223ea3765a7699e19d46a6e0c70b4baa79 | c1a9d83b34441592d063c4d0753029c187b1c16a | "2023-03-20T02:39:16" | python | "2023-03-27T15:17:14" | langchain/document_loaders/azure_blob_storage_file.py | |
closed | langchain-ai/langchain | https://github.com/langchain-ai/langchain | 1,801 | Poetry 1.4.0 installation fails | `poetry install -E all` fails with Poetry >=1.4.0 due to upstream incompatibility between `poetry>=1.4.0` and `pydata_sphinx_theme`.
This is a tracking issue. I've already created an issue upstream here: https://github.com/pydata/pydata-sphinx-theme/issues/1253 | https://github.com/langchain-ai/langchain/issues/1801 | https://github.com/langchain-ai/langchain/pull/1935 | 3d3e52352005aef549f9e19ad6ab18428887865c | c50fafb35d22f0f2b4e39ebb24a5ee6177c8f44e | "2023-03-19T23:42:55" | python | "2023-03-27T15:27:54" | poetry.toml | [virtualenvs]
in-project = true
|
closed | langchain-ai/langchain | https://github.com/langchain-ai/langchain | 1,838 | How metadata is being used during similarity search and query? | I have 3 pdf files in my directory and I "documentized", added metadata, split, embed and store them in pinecone, like this:
```
loader = DirectoryLoader('data/dir', glob="**/*.pdf", loader_cls=UnstructuredPDFLoader)
data = loader.load()
#I added company names explicitly for now
data[0].metadata["company"]="Apple"
data[1].metadata["company"]="Miscrosoft"
data[2].metadata["company"]="Tesla"
text_splitter = RecursiveCharacterTextSplitter(chunk_size=500, chunk_overlap=200)
texts = text_splitter.split_documents(data)
embeddings = OpenAIEmbeddings(openai_api_key=OPENAI_API_KEY)
pinecone.init(
api_key=PINECONE_API_KEY,
environment=PINECONE_API_ENV
)
metadatas = []
for text in texts:
metadatas.append({
"company": text.metadata["company"]
})
Pinecone.from_texts([t.page_content for t in texts], embeddings, index_name=index_name, metadatas=metadatas)
```
I want to build a Q&A system, so that I will mention a company name in my query and pinecon should look for the documents having company `A` in the metadata. Here what I have:
```
pinecone.init(
api_key=PINECONE_API_KEY,
environment=PINECONE_API_ENV
)
index_name = "index"
embeddings = OpenAIEmbeddings(openai_api_key=OPENAI_API_KEY)
docsearch = Pinecone.from_existing_index(index_name=index_name, embedding=embeddings)
llm = ChatOpenAI(temperature=0, openai_api_key=OPENAI_API_KEY)
chain = load_qa_chain(llm, chain_type="stuff")
query = "What is the total revenue of Apple?"
docs = docsearch.similarity_search(query, include_metadata=True)
res = chain.run(input_documents=docs, question=query)
print(res)
```
However, there are still document chunks from non-Apple documents in the output of `docs`. What am I doing wrong here and how do I utilize the information in metadata both on doc_search and chat-gpt query (If possible)? Thanks | https://github.com/langchain-ai/langchain/issues/1838 | https://github.com/langchain-ai/langchain/pull/1964 | f257b08406563af9ffb044da45b829d0707d755b | 953e58d0040773c76f68e633c3db3cd371c9c350 | "2023-03-21T01:32:20" | python | "2023-03-27T22:04:53" | langchain/vectorstores/chroma.py | """Wrapper around ChromaDB embeddings platform."""
from __future__ import annotations
import logging
import uuid
from typing import TYPE_CHECKING, Any, Dict, Iterable, List, Optional, Tuple
from langchain.docstore.document import Document
from langchain.embeddings.base import Embeddings
from langchain.vectorstores.base import VectorStore
if TYPE_CHECKING:
import chromadb
import chromadb.config
logger = logging.getLogger()
def _results_to_docs(results: Any) -> List[Document]:
return [doc for doc, _ in _results_to_docs_and_scores(results)]
def _results_to_docs_and_scores(results: Any) -> List[Tuple[Document, float]]:
return [
# TODO: Chroma can do batch querying,
# we shouldn't hard code to the 1st result
(Document(page_content=result[0], metadata=result[1] or {}), result[2])
for result in zip(
results["documents"][0],
results["metadatas"][0],
results["distances"][0],
)
]
class Chroma(VectorStore):
"""Wrapper around ChromaDB embeddings platform.
To use, you should have the ``chromadb`` python package installed.
Example:
.. code-block:: python
from langchain.vectorstores import Chroma
from langchain.embeddings.openai import OpenAIEmbeddings
embeddings = OpenAIEmbeddings()
vectorstore = Chroma("langchain_store", embeddings.embed_query)
"""
_LANGCHAIN_DEFAULT_COLLECTION_NAME = "langchain"
def __init__(
self,
collection_name: str = _LANGCHAIN_DEFAULT_COLLECTION_NAME,
embedding_function: Optional[Embeddings] = None,
persist_directory: Optional[str] = None,
client_settings: Optional[chromadb.config.Settings] = None,
) -> None:
"""Initialize with Chroma client."""
try:
import chromadb
import chromadb.config
except ImportError:
raise ValueError(
"Could not import chromadb python package. "
"Please install it with `pip install chromadb`."
)
if client_settings:
self._client_settings = client_settings
else:
self._client_settings = chromadb.config.Settings()
if persist_directory is not None:
self._client_settings = chromadb.config.Settings(
chroma_db_impl="duckdb+parquet", persist_directory=persist_directory
)
self._client = chromadb.Client(self._client_settings)
self._embedding_function = embedding_function
self._persist_directory = persist_directory
self._collection = self._client.get_or_create_collection(
name=collection_name,
embedding_function=self._embedding_function.embed_documents
if self._embedding_function is not None
else None,
)
def add_texts(
self,
texts: Iterable[str],
metadatas: Optional[List[dict]] = None,
ids: Optional[List[str]] = None,
**kwargs: Any,
) -> List[str]:
"""Run more texts through the embeddings and add to the vectorstore.
Args:
texts (Iterable[str]): Texts to add to the vectorstore.
metadatas (Optional[List[dict]], optional): Optional list of metadatas.
ids (Optional[List[str]], optional): Optional list of IDs.
Returns:
List[str]: List of IDs of the added texts.
"""
# TODO: Handle the case where the user doesn't provide ids on the Collection
if ids is None:
ids = [str(uuid.uuid1()) for _ in texts]
embeddings = None
if self._embedding_function is not None:
embeddings = self._embedding_function.embed_documents(list(texts))
self._collection.add(
metadatas=metadatas, embeddings=embeddings, documents=texts, ids=ids
)
return ids
def similarity_search(
self,
query: str,
k: int = 4,
filter: Optional[Dict[str, str]] = None,
**kwargs: Any,
) -> List[Document]:
"""Run similarity search with Chroma.
Args:
query (str): Query text to search for.
k (int): Number of results to return. Defaults to 4.
filter (Optional[Dict[str, str]]): Filter by metadata. Defaults to None.
Returns:
List[Document]: List of documents most simmilar to the query text.
"""
docs_and_scores = self.similarity_search_with_score(query, k, where=filter)
return [doc for doc, _ in docs_and_scores]
def similarity_search_by_vector(
self,
embedding: List[float],
k: int = 4,
filter: Optional[Dict[str, str]] = None,
**kwargs: Any,
) -> List[Document]:
"""Return docs most similar to embedding vector.
Args:
embedding: Embedding to look up documents similar to.
k: Number of Documents to return. Defaults to 4.
Returns:
List of Documents most similar to the query vector.
"""
results = self._collection.query(
query_embeddings=embedding, n_results=k, where=filter
)
return _results_to_docs(results)
def similarity_search_with_score(
self,
query: str,
k: int = 4,
filter: Optional[Dict[str, str]] = None,
**kwargs: Any,
) -> List[Tuple[Document, float]]:
"""Run similarity search with Chroma with distance.
Args:
query (str): Query text to search for.
k (int): Number of results to return. Defaults to 4.
filter (Optional[Dict[str, str]]): Filter by metadata. Defaults to None.
Returns:
List[Tuple[Document, float]]: List of documents most similar to the query
text with distance in float.
"""
if self._embedding_function is None:
results = self._collection.query(
query_texts=[query], n_results=k, where=filter
)
else:
query_embedding = self._embedding_function.embed_query(query)
results = self._collection.query(
query_embeddings=[query_embedding], n_results=k, where=filter
)
return _results_to_docs_and_scores(results)
def delete_collection(self) -> None:
"""Delete the collection."""
self._client.delete_collection(self._collection.name)
def persist(self) -> None:
"""Persist the collection.
This can be used to explicitly persist the data to disk.
It will also be called automatically when the object is destroyed.
"""
if self._persist_directory is None:
raise ValueError(
"You must specify a persist_directory on"
"creation to persist the collection."
)
self._client.persist()
@classmethod
def from_texts(
cls,
texts: List[str],
embedding: Optional[Embeddings] = None,
metadatas: Optional[List[dict]] = None,
ids: Optional[List[str]] = None,
collection_name: str = _LANGCHAIN_DEFAULT_COLLECTION_NAME,
persist_directory: Optional[str] = None,
client_settings: Optional[chromadb.config.Settings] = None,
**kwargs: Any,
) -> Chroma:
"""Create a Chroma vectorstore from a raw documents.
If a persist_directory is specified, the collection will be persisted there.
Otherwise, the data will be ephemeral in-memory.
Args:
texts (List[str]): List of texts to add to the collection.
collection_name (str): Name of the collection to create.
persist_directory (Optional[str]): Directory to persist the collection.
embedding (Optional[Embeddings]): Embedding function. Defaults to None.
metadatas (Optional[List[dict]]): List of metadatas. Defaults to None.
ids (Optional[List[str]]): List of document IDs. Defaults to None.
client_settings (Optional[chromadb.config.Settings]): Chroma client settings
Returns:
Chroma: Chroma vectorstore.
"""
chroma_collection = cls(
collection_name=collection_name,
embedding_function=embedding,
persist_directory=persist_directory,
client_settings=client_settings,
)
chroma_collection.add_texts(texts=texts, metadatas=metadatas, ids=ids)
return chroma_collection
@classmethod
def from_documents(
cls,
documents: List[Document],
embedding: Optional[Embeddings] = None,
ids: Optional[List[str]] = None,
collection_name: str = _LANGCHAIN_DEFAULT_COLLECTION_NAME,
persist_directory: Optional[str] = None,
client_settings: Optional[chromadb.config.Settings] = None,
**kwargs: Any,
) -> Chroma:
"""Create a Chroma vectorstore from a list of documents.
If a persist_directory is specified, the collection will be persisted there.
Otherwise, the data will be ephemeral in-memory.
Args:
collection_name (str): Name of the collection to create.
persist_directory (Optional[str]): Directory to persist the collection.
ids (Optional[List[str]]): List of document IDs. Defaults to None.
documents (List[Document]): List of documents to add to the vectorstore.
embedding (Optional[Embeddings]): Embedding function. Defaults to None.
client_settings (Optional[chromadb.config.Settings]): Chroma client settings
Returns:
Chroma: Chroma vectorstore.
"""
texts = [doc.page_content for doc in documents]
metadatas = [doc.metadata for doc in documents]
return cls.from_texts(
texts=texts,
embedding=embedding,
metadatas=metadatas,
ids=ids,
collection_name=collection_name,
persist_directory=persist_directory,
client_settings=client_settings,
)
|
closed | langchain-ai/langchain | https://github.com/langchain-ai/langchain | 1,712 | bug(QA with Sources): source parsing is not reliable | I was going through [Vectorstore Agent](https://langchain.readthedocs.io/en/latest/modules/agents/agent_toolkits/vectorstore.html?highlight=vectorstore%20agent#vectorstore-agent) tutorial and I am facing issues with the `VectorStoreQAWithSourcesTool`.
Looking closely at the code https://github.com/hwchase17/langchain/blob/3c2468452284ee37b8a88a20b864255fa4385b65/langchain/chains/qa_with_sources/base.py#L119-L120
it appears, the parsing rule might be too strict for extract the list of sources. Often, when the agent is fetching information from the vectorstore, the `VectorStoreQAWithSourcesTool` output is something like `....SOURCES:\n<source1>\n<source2>...` instead of `...SOURCES: <source1>,<source2>...`.
Due to this, the `VectorStoreQAWithSourcesTool` output is broken and the agent response is impacted.
P.S. I used `Chroma` as the vectorstore db and `OpenAI(temperature=0)` as the LLM. | https://github.com/langchain-ai/langchain/issues/1712 | https://github.com/langchain-ai/langchain/pull/2118 | c33e055f17d59e225cc009c49b28d4400d56e709 | 859502b16c132e6d2f02d5233233f20f78847bdb | "2023-03-16T15:47:53" | python | "2023-03-28T22:28:20" | langchain/chains/qa_with_sources/base.py | """Question answering with sources over documents."""
from __future__ import annotations
from abc import ABC, abstractmethod
from typing import Any, Dict, List, Optional
from pydantic import BaseModel, Extra, root_validator
from langchain.chains.base import Chain
from langchain.chains.combine_documents.base import BaseCombineDocumentsChain
from langchain.chains.combine_documents.map_reduce import MapReduceDocumentsChain
from langchain.chains.combine_documents.stuff import StuffDocumentsChain
from langchain.chains.llm import LLMChain
from langchain.chains.qa_with_sources.loading import load_qa_with_sources_chain
from langchain.chains.qa_with_sources.map_reduce_prompt import (
COMBINE_PROMPT,
EXAMPLE_PROMPT,
QUESTION_PROMPT,
)
from langchain.docstore.document import Document
from langchain.prompts.base import BasePromptTemplate
from langchain.schema import BaseLanguageModel
class BaseQAWithSourcesChain(Chain, BaseModel, ABC):
"""Question answering with sources over documents."""
combine_documents_chain: BaseCombineDocumentsChain
"""Chain to use to combine documents."""
question_key: str = "question" #: :meta private:
input_docs_key: str = "docs" #: :meta private:
answer_key: str = "answer" #: :meta private:
sources_answer_key: str = "sources" #: :meta private:
return_source_documents: bool = False
"""Return the source documents."""
@classmethod
def from_llm(
cls,
llm: BaseLanguageModel,
document_prompt: BasePromptTemplate = EXAMPLE_PROMPT,
question_prompt: BasePromptTemplate = QUESTION_PROMPT,
combine_prompt: BasePromptTemplate = COMBINE_PROMPT,
**kwargs: Any,
) -> BaseQAWithSourcesChain:
"""Construct the chain from an LLM."""
llm_question_chain = LLMChain(llm=llm, prompt=question_prompt)
llm_combine_chain = LLMChain(llm=llm, prompt=combine_prompt)
combine_results_chain = StuffDocumentsChain(
llm_chain=llm_combine_chain,
document_prompt=document_prompt,
document_variable_name="summaries",
)
combine_document_chain = MapReduceDocumentsChain(
llm_chain=llm_question_chain,
combine_document_chain=combine_results_chain,
document_variable_name="context",
)
return cls(
combine_documents_chain=combine_document_chain,
**kwargs,
)
@classmethod
def from_chain_type(
cls,
llm: BaseLanguageModel,
chain_type: str = "stuff",
chain_type_kwargs: Optional[dict] = None,
**kwargs: Any,
) -> BaseQAWithSourcesChain:
"""Load chain from chain type."""
_chain_kwargs = chain_type_kwargs or {}
combine_document_chain = load_qa_with_sources_chain(
llm, chain_type=chain_type, **_chain_kwargs
)
return cls(combine_documents_chain=combine_document_chain, **kwargs)
class Config:
"""Configuration for this pydantic object."""
extra = Extra.forbid
arbitrary_types_allowed = True
@property
def input_keys(self) -> List[str]:
"""Expect input key.
:meta private:
"""
return [self.question_key]
@property
def output_keys(self) -> List[str]:
"""Return output key.
:meta private:
"""
_output_keys = [self.answer_key, self.sources_answer_key]
if self.return_source_documents:
_output_keys = _output_keys + ["source_documents"]
return _output_keys
@root_validator(pre=True)
def validate_naming(cls, values: Dict) -> Dict:
"""Fix backwards compatability in naming."""
if "combine_document_chain" in values:
values["combine_documents_chain"] = values.pop("combine_document_chain")
return values
@abstractmethod
def _get_docs(self, inputs: Dict[str, Any]) -> List[Document]:
"""Get docs to run questioning over."""
def _call(self, inputs: Dict[str, Any]) -> Dict[str, Any]:
docs = self._get_docs(inputs)
answer, _ = self.combine_documents_chain.combine_docs(docs, **inputs)
if "SOURCES: " in answer:
answer, sources = answer.split("SOURCES: ")
else:
sources = ""
result: Dict[str, Any] = {
self.answer_key: answer,
self.sources_answer_key: sources,
}
if self.return_source_documents:
result["source_documents"] = docs
return result
class QAWithSourcesChain(BaseQAWithSourcesChain, BaseModel):
"""Question answering with sources over documents."""
input_docs_key: str = "docs" #: :meta private:
@property
def input_keys(self) -> List[str]:
"""Expect input key.
:meta private:
"""
return [self.input_docs_key, self.question_key]
def _get_docs(self, inputs: Dict[str, Any]) -> List[Document]:
return inputs.pop(self.input_docs_key)
@property
def _chain_type(self) -> str:
return "qa_with_sources_chain"
|
closed | langchain-ai/langchain | https://github.com/langchain-ai/langchain | 1,834 | LLMMathChain to allow ChatOpenAI as an llm | 1. Cannot initialize match chain with ChatOpenAI LLM
llm_math = LLMMathChain(llm=ChatOpenAI(temperature=0))
---------------------------------------------------------------------------
ValidationError Traceback (most recent call last)
Cell In[33], line 1
----> 1 llm_math = LLMMathChain(llm=ChatOpenAI(temperature=0))
File ~/anaconda3/envs/gpt_index/lib/python3.8/site-packages/pydantic/main.py:341, in pydantic.main.BaseModel.__init__()
ValidationError: 1 validation error for LLMMathChain
llm
Can't instantiate abstract class BaseLLM with abstract methods _agenerate, _generate, _llm_type (type=type_error)
2. Works ok with OpenAI LLM
llm_math = LLMMathChain(llm=OpenAI(temperature=0))
| https://github.com/langchain-ai/langchain/issues/1834 | https://github.com/langchain-ai/langchain/pull/2183 | 3207a7482915a658cf8f473ae0a81ba9998c8531 | fd1fcb5a7d48cbe18b480b1493b66540e4709745 | "2023-03-20T23:12:24" | python | "2023-03-30T14:52:58" | langchain/chains/llm_math/base.py | """Chain that interprets a prompt and executes python code to do math."""
from typing import Dict, List
from pydantic import BaseModel, Extra
from langchain.chains.base import Chain
from langchain.chains.llm import LLMChain
from langchain.chains.llm_math.prompt import PROMPT
from langchain.llms.base import BaseLLM
from langchain.prompts.base import BasePromptTemplate
from langchain.python import PythonREPL
class LLMMathChain(Chain, BaseModel):
"""Chain that interprets a prompt and executes python code to do math.
Example:
.. code-block:: python
from langchain import LLMMathChain, OpenAI
llm_math = LLMMathChain(llm=OpenAI())
"""
llm: BaseLLM
"""LLM wrapper to use."""
prompt: BasePromptTemplate = PROMPT
"""Prompt to use to translate to python if neccessary."""
input_key: str = "question" #: :meta private:
output_key: str = "answer" #: :meta private:
class Config:
"""Configuration for this pydantic object."""
extra = Extra.forbid
arbitrary_types_allowed = True
@property
def input_keys(self) -> List[str]:
"""Expect input key.
:meta private:
"""
return [self.input_key]
@property
def output_keys(self) -> List[str]:
"""Expect output key.
:meta private:
"""
return [self.output_key]
def _process_llm_result(self, t: str) -> Dict[str, str]:
python_executor = PythonREPL()
self.callback_manager.on_text(t, color="green", verbose=self.verbose)
t = t.strip()
if t.startswith("```python"):
code = t[9:-4]
output = python_executor.run(code)
self.callback_manager.on_text("\nAnswer: ", verbose=self.verbose)
self.callback_manager.on_text(output, color="yellow", verbose=self.verbose)
answer = "Answer: " + output
elif t.startswith("Answer:"):
answer = t
elif "Answer:" in t:
answer = "Answer: " + t.split("Answer:")[-1]
else:
raise ValueError(f"unknown format from LLM: {t}")
return {self.output_key: answer}
def _call(self, inputs: Dict[str, str]) -> Dict[str, str]:
llm_executor = LLMChain(
prompt=self.prompt, llm=self.llm, callback_manager=self.callback_manager
)
self.callback_manager.on_text(inputs[self.input_key], verbose=self.verbose)
t = llm_executor.predict(question=inputs[self.input_key], stop=["```output"])
return self._process_llm_result(t)
async def _acall(self, inputs: Dict[str, str]) -> Dict[str, str]:
llm_executor = LLMChain(
prompt=self.prompt, llm=self.llm, callback_manager=self.callback_manager
)
self.callback_manager.on_text(inputs[self.input_key], verbose=self.verbose)
t = await llm_executor.apredict(
question=inputs[self.input_key], stop=["```output"]
)
return self._process_llm_result(t)
@property
def _chain_type(self) -> str:
return "llm_math_chain"
|
closed | langchain-ai/langchain | https://github.com/langchain-ai/langchain | 2,245 | Wrong PromptLayer Dashboard hyperlink | In the docs, in https://python.langchain.com/en/latest/modules/models/llms/integrations/promptlayer_openai.html there is a hyperlink to the PromptLayer dashboard that links to "https://ww.promptlayer.com", which is incorrect. | https://github.com/langchain-ai/langchain/issues/2245 | https://github.com/langchain-ai/langchain/pull/2246 | e57b045402b52c2a602f4895c5b06fa2c22b745a | 632c2b49dabbccab92e37d01e4d1d86b6fa68457 | "2023-03-31T20:33:41" | python | "2023-03-31T23:16:23" | docs/modules/models/llms/integrations/promptlayer_openai.ipynb | {
"cells": [
{
"attachments": {},
"cell_type": "markdown",
"id": "959300d4",
"metadata": {},
"source": [
"# PromptLayer OpenAI\n",
"\n",
"This example showcases how to connect to [PromptLayer](https://www.promptlayer.com) to start recording your OpenAI requests."
]
},
{
"attachments": {},
"cell_type": "markdown",
"id": "6a45943e",
"metadata": {},
"source": [
"## Install PromptLayer\n",
"The `promptlayer` package is required to use PromptLayer with OpenAI. Install `promptlayer` using pip."
]
},
{
"cell_type": "code",
"execution_count": null,
"id": "dbe09bd8",
"metadata": {
"vscode": {
"languageId": "powershell"
}
},
"outputs": [],
"source": [
"pip install promptlayer"
]
},
{
"cell_type": "markdown",
"id": "536c1dfa",
"metadata": {},
"source": [
"## Imports"
]
},
{
"cell_type": "code",
"execution_count": null,
"id": "c16da3b5",
"metadata": {},
"outputs": [],
"source": [
"import os\n",
"from langchain.llms import PromptLayerOpenAI\n",
"import promptlayer"
]
},
{
"attachments": {},
"cell_type": "markdown",
"id": "8564ce7d",
"metadata": {},
"source": [
"## Set the Environment API Key\n",
"You can create a PromptLayer API Key at [www.promptlayer.com](https://www.promptlayer.com) by clicking the settings cog in the navbar.\n",
"\n",
"Set it as an environment variable called `PROMPTLAYER_API_KEY`."
]
},
{
"cell_type": "code",
"execution_count": null,
"id": "46ba25dc",
"metadata": {},
"outputs": [],
"source": [
"os.environ[\"PROMPTLAYER_API_KEY\"] = \"********\""
]
},
{
"attachments": {},
"cell_type": "markdown",
"id": "bf0294de",
"metadata": {},
"source": [
"## Use the PromptLayerOpenAI LLM like normal\n",
"*You can optionally pass in `pl_tags` to track your requests with PromptLayer's tagging feature.*"
]
},
{
"cell_type": "code",
"execution_count": 4,
"id": "3acf0069",
"metadata": {},
"outputs": [
{
"data": {
"text/plain": [
"' to go outside\\n\\nUnfortunately, cats cannot go outside without being supervised by a human. Going outside can be dangerous for cats, as they may come into contact with cars, other animals, or other dangers. If you want to go outside, ask your human to take you on a supervised walk or to a safe, enclosed outdoor space.'"
]
},
"execution_count": 4,
"metadata": {},
"output_type": "execute_result"
}
],
"source": [
"llm = PromptLayerOpenAI(pl_tags=[\"langchain\"])\n",
"llm(\"I am a cat and I want\")"
]
},
{
"attachments": {},
"cell_type": "markdown",
"id": "a2d76826",
"metadata": {},
"source": [
"**The above request should now appear on your [PromptLayer dashboard](https://ww.promptlayer.com).**"
]
},
{
"attachments": {},
"cell_type": "markdown",
"id": "05e9e2fe",
"metadata": {},
"source": [
"## Using PromptLayer Track\n",
"If you would like to use any of the [PromptLayer tracking features](https://magniv.notion.site/Track-4deee1b1f7a34c1680d085f82567dab9), you need to pass the argument `return_pl_id` when instantializing the PromptLayer LLM to get the request id. "
]
},
{
"cell_type": "code",
"execution_count": null,
"id": "1a7315b9",
"metadata": {},
"outputs": [],
"source": [
"llm = PromptLayerOpenAI(return_pl_id=True)\n",
"llm_results = llm.generate([\"Tell me a joke\"])\n",
"\n",
"for res in llm_results.generations:\n",
" pl_request_id = res[0].generation_info[\"pl_request_id\"]\n",
" promptlayer.track.score(request_id=pl_request_id, score=100)"
]
},
{
"attachments": {},
"cell_type": "markdown",
"id": "7eb19139",
"metadata": {},
"source": [
"Using this allows you to track the performance of your model in the PromptLayer dashboard. If you are using a prompt template, you can attach a template to a request as well.\n",
"Overall, this gives you the opportunity to track the performance of different templates and models in the PromptLayer dashboard."
]
}
],
"metadata": {
"kernelspec": {
"display_name": "base",
"language": "python",
"name": "python3"
},
"language_info": {
"codemirror_mode": {
"name": "ipython",
"version": 3
},
"file_extension": ".py",
"mimetype": "text/x-python",
"name": "python",
"nbconvert_exporter": "python",
"pygments_lexer": "ipython3",
"version": "3.8.8 (default, Apr 13 2021, 12:59:45) \n[Clang 10.0.0 ]"
},
"vscode": {
"interpreter": {
"hash": "8a5edab282632443219e051e4ade2d1d5bbc671c781051bf1437897cbdfea0f1"
}
}
},
"nbformat": 4,
"nbformat_minor": 5
}
|
closed | langchain-ai/langchain | https://github.com/langchain-ai/langchain | 2,174 | failed tests on Windows platform | NOTE: fixed in #2238 PR.
I'm running `tests/unit_tests` on the Windows platform and several tests related to `bash` failed.
>test_llm_bash/
test_simple_question
and
>test_bash/
test_pwd_command
test_incorrect_command
test_incorrect_command_return_err_output
test_create_directory_and_files
If it is because these tests should run only on Linux, we can add
>if not sys.platform.startswith("win"):
pytest.skip("skipping windows-only tests", allow_module_level=True)
to the `test_bash.py`
and
>@pytest.mark.skipif(sys.platform.startswith("win", reason="skipping windows-only tests")
to `test_llm_bash/test_simple_question`
regarding [this](https://docs.pytest.org/en/7.1.x/how-to/skipping.html).
If you want you can assign this issue to me :)
UPDATE:
Probably` tests/unit_test/utilities/test_loading/[test_success, test_failed_request]` (tests with correspondent `_teardown`) are also failing because of the Windows environment. | https://github.com/langchain-ai/langchain/issues/2174 | https://github.com/langchain-ai/langchain/pull/2238 | 609b14a57004b4679341a05729577ec5dbcaff7d | 579ad85785a4011bdcb9fc316d2c1bcddfb9d427 | "2023-03-30T03:43:17" | python | "2023-04-01T19:52:21" | tests/unit_tests/chains/test_llm_bash.py | """Test LLM Bash functionality."""
import pytest
from langchain.chains.llm_bash.base import LLMBashChain
from langchain.chains.llm_bash.prompt import _PROMPT_TEMPLATE
from tests.unit_tests.llms.fake_llm import FakeLLM
@pytest.fixture
def fake_llm_bash_chain() -> LLMBashChain:
"""Fake LLM Bash chain for testing."""
question = "Please write a bash script that prints 'Hello World' to the console."
prompt = _PROMPT_TEMPLATE.format(question=question)
queries = {prompt: "```bash\nexpr 1 + 1\n```"}
fake_llm = FakeLLM(queries=queries)
return LLMBashChain(llm=fake_llm, input_key="q", output_key="a")
def test_simple_question(fake_llm_bash_chain: LLMBashChain) -> None:
"""Test simple question that should not need python."""
question = "Please write a bash script that prints 'Hello World' to the console."
output = fake_llm_bash_chain.run(question)
assert output == "2\n"
|
End of preview. Expand
in Data Studio
README.md exists but content is empty.
- Downloads last month
- 99