Spaces:
Sleeping
Sleeping
Gaussian Head Avatar: Ultra High-fidelity Head Avatar via Dynamic Gaussians
Paper | Project Page
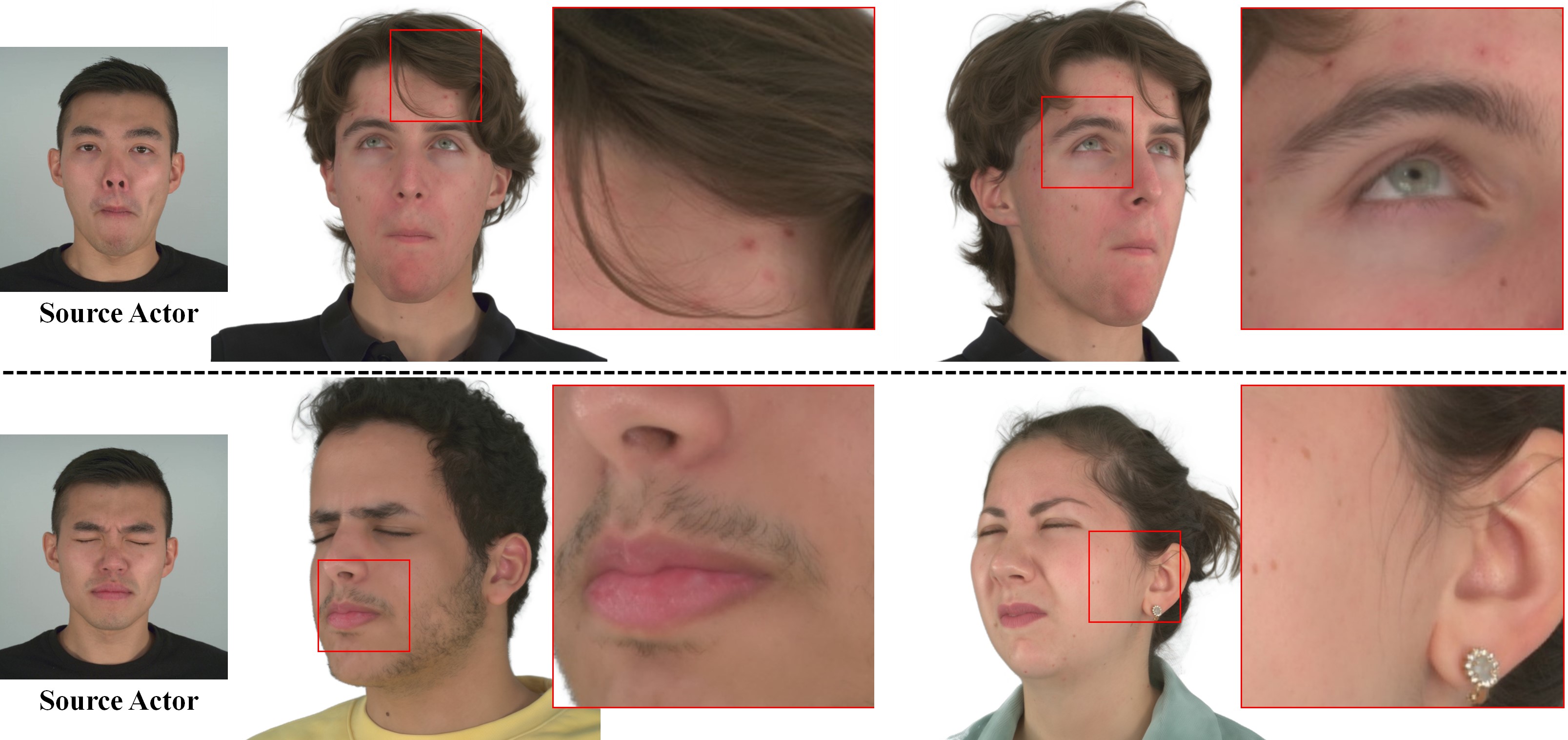
Requirements
- Create a conda environment.
conda env create -f environment.yaml
- Install Pytorch3d.
pip install --no-index --no-cache-dir pytorch3d -f https://dl.fbaipublicfiles.com/pytorch3d/packaging/wheels/py38_cu113_pyt1120/download.html
- Install kaolin.
pip install kaolin==0.13.0 -f https://nvidia-kaolin.s3.us-east-2.amazonaws.com/torch-1.12.0_cu113.html
- Install diff-gaussian-rasterization and simple_knn from gaussian-splatting. Note, for rendering 32-channel images, please modify "NUM_CHANNELS 3" to "NUM_CHANNELS 32" in "diff-gaussian-rasterization/cuda_rasterizer/config.h".
cd path/to/gaussian-splatting
# Modify "submodules/diff-gaussian-rasterization/cuda_rasterizer/config.h"
pip install submodules/diff-gaussian-rasterization
pip install submodules/simple-knn
- Download "tets_data.npz" and put it into "assets/".
Datasets
We provide instructions for preprocessing NeRSemble dataset:
- Apply to download NeRSemble dataset and unzip it into "path/to/raw_NeRSemble/".
- Extract the images, cameras and background for specific identities into a structured dataset "NeRSemble/{id}".
cd preprocess
python preprocess_nersemble.py
- Remove background using BackgroundMattingV2. Please git clone the code. Download pytorch_resnet101.pth and put it into "path/to/BackgroundMattingV2/assets/". Then run the script we provide "preprocess/remove_background_nersemble.py".
cp preprocess/remove_background_nersemble.py path/to/BackgroundMattingV2/
cd path/to/BackgroundMattingV2
python remove_background_nersemble.py
- Fit BFM model for head pose and expression coefficients using Multiview-3DMM-Fitting. Please follow the instructions.
We provide a mini demo dataset for checking whether the code is runnable. Note, before downloading it, you must first sign the NeRSemble Terms of Use.
Training
First, edit the config file, for example "config/train_meshhead_N031", and train the geometry guidance model.
python train_meshhead.py --config config/train_meshhead_N031.yaml
Second, edit the config file "config/train_gaussianhead_N031", and train the gaussian head avatar.
python train_gaussianhead.py --config config/train_gaussianhead_N031.yaml
Reenactment
Once the two-stage training is completed, the trained avatar can be reenacted by a sequence of expression coefficients. Please specify the avatar checkpoints and the source data in the config file "config/reenactment_N031.py" and run the reenactment application.
python reenactment.py --config config/reenactment_N031.yaml
Acknowledgement
Part of the code is borrowed from gaussian-splatting.
Citation
@inproceedings{xu2023gaussianheadavatar,
title={Gaussian Head Avatar: Ultra High-fidelity Head Avatar via Dynamic Gaussians},
author={Xu, Yuelang and Chen, Benwang and Li, Zhe and Zhang, Hongwen and Wang, Lizhen and Zheng, Zerong and Liu, Yebin},
booktitle={Proceedings of the IEEE/CVF Conference on Computer Vision and Pattern Recognition (CVPR)},
year={2024}
}