metadata
base_model: sentence-transformers/all-mpnet-base-v2
library_name: setfit
metrics:
- accuracy
pipeline_tag: text-classification
tags:
- setfit
- sentence-transformers
- text-classification
- generated_from_setfit_trainer
widget:
- text: "How to get single UnidirectionalSequenceRnnOp in tflite model ### Issue Type\r\n\r\nSupport\r\n\r\n### Source\r\n\r\nsource\r\n\r\n### Tensorflow Version\r\n\r\n2.8\r\n\r\n### Custom Code\r\n\r\nYes\r\n\r\n### OS Platform and Distribution\r\n\r\nUbuntu 18.04\r\n\r\nAccording to https://github.com/tensorflow/tensorflow/blob/master/tensorflow/compiler/mlir/lite/python/tf_tfl_flatbuffer_helpers.cc there is `kUnidirectionalSequenceRnnOp` as a single operation in tflite, could you give a python code example - how can I get this? For example - this code for LSTM gives tflite with one UnidirectionalSequenceLSTM Op.\r\n```py\r\n# NOTE tested with TF 2.8.0\r\nimport tensorflow as tf\r\nimport numpy as np\r\n\r\nfrom tensorflow import keras\r\n\r\n\r\nmodel = keras.Sequential()\r\nshape = (4, 4)\r\n\r\nmodel.add(keras.layers.InputLayer(input_shape=shape, batch_size=1))\r\nmodel.add(keras.layers.LSTM(2, input_shape=shape))\r\n```\r\n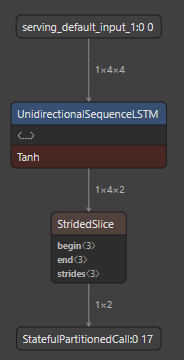\r\nHow can I do same for UnidirectionalSequenceRnn?"
- text: "[Feature Request] GELU activation with the Hexagon delegate **System information**\r\n- OS Platform and Distribution (e.g., Linux Ubuntu 16.04): Ubuntu 20.04\r\n- TensorFlow installed from (source or binary): binary\r\n- TensorFlow version (or github SHA if from source): 2.9.1\r\n\r\nI think I'd be able to implement this myself, but wanted to see if there was any interest in including this upstream. Most of this I'm writing out to make sure my own understanding is correct.\r\n\r\n### The problem\r\n\r\nI'd like to add support for the GELU op to the Hexagon Delegate. The motivation for this is mostly for use with [DistilBERT](https://huggingface.co/distilbert-base-multilingual-cased), which uses this activation function in its feedforward network layers. (Also used by BERT, GPT-3, RoBERTa, etc.)\r\n\r\nAdding this as a supported op for the Hexagon delegate would avoid creating a graph partition/transferring between DSP<-->CPU each time the GELU activation function is used.\r\n\r\n### How I'd implement this\r\n\r\nGELU in TF Lite is implemented as a lookup table when there are integer inputs ([here](https://github.com/tensorflow/tensorflow/blob/master/tensorflow/lite/kernels/activations.cc#L120-L140) and [here](https://github.com/tensorflow/tensorflow/blob/master/tensorflow/lite/kernels/internal/reference/gelu.h#L37-L53)).\r\n\r\nThis same approach could be used for the Hexagon delegate, as it has int8/uint8 data types and also supports lookup tables.\r\n\r\nI'd plan to do this by adding a new op builder in the delegate, populating a lookup table for each node as is currently done for the CPU version of the op, and then using the [Gather_8](https://source.codeaurora.org/quic/hexagon_nn/nnlib/tree/hexagon/ops/src/op_gather.c) nnlib library function to do the lookup.\r\n\r\n### Possible workaround\r\n\r\nA workaround I thought of:\r\n\r\nI'm going to try removing the [pattern matching](https://github.com/tensorflow/tensorflow/blob/master/tensorflow/compiler/mlir/lite/transforms/optimize_patterns.td#L1034-L1095) for approximate GELU in MLIR, and then using the approximate version of GELU (so that using tanh and not Erf). This will probably be slower, but should let me keep execution on the DSP.\r\n\r\nSince this will then be tanh, addition, multiplication ops instead of GELU they should all be runnable by the DSP."
- text: >-
Data init API for TFLite Swift <details><summary>Click to
expand!</summary>
### Issue Type
Feature Request
### Source
source
### Tensorflow Version
2.8+
### Custom Code
No
### OS Platform and Distribution
_No response_
### Mobile device
_No response_
### Python version
_No response_
### Bazel version
_No response_
### GCC/Compiler version
_No response_
### CUDA/cuDNN version
_No response_
### GPU model and memory
_No response_
### Current Behaviour?
```shell
The current Swift API only has `init` functions from files on disk unlike
the Java (Android) API which has a byte buffer initializer. It'd be
convenient if the Swift API could initialize `Interpreters` from `Data`.
```
### Standalone code to reproduce the issue
```shell
No code. This is a feature request
```
### Relevant log output
_No response_</details>
- text: "tf.distribute.MirroredStrategy for asynchronous training <details><summary>Click to expand!</summary> \r\n \r\n ### Issue Type\r\n\r\nFeature Request\r\n\r\n### Tensorflow Version\r\n\r\n2.8.1\r\n\r\n### Python version\r\n\r\n3.8.13\r\n\r\n### CUDA/cuDNN version\r\n\r\n11.8\r\n\r\n### Use Case\r\n\r\nI need to run multiple asynchronous copies of the same model on different slices of the dataset (e.g. with bootstrap sampling). There's no *good* way to do this in keras api that I'm aware of, although a couple of hacks exist. Would this use case be feasible with tf.distribute?\r\n\r\n### Feature Request\r\n\r\n`tf.distribute.MirroredStrategy` is a synchronous, data parallel strategy for distributed training across multiple devices on a single host worker.\r\n\r\nWould it be possible to modify this strategy to allow for asynchronous training of all model replicas, without computing the average gradient over all replicas to update weights? In this case each replica would need its own un-mirrored copy of model weights, and the update rule would depend only on the loss and gradients of each replica.\r\n\r\nThanks"
- text: "Build TensorFlow Lite for iOS failed!!!! Please go to Stack Overflow for help and support:\r\n\r\nhttps://stackoverflow.com/questions/tagged/tensorflow\r\n\r\nIf you open a GitHub issue, here is our policy:\r\n\r\n1. `bazel build --config=ios_arm64 -c opt --cxxopt=--std=c++17 \\\\\r\n //tensorflow/lite/ios:TensorFlowLiteC_framework\r\n❯ bazel build --incompatible_run_shell_command_string=false --verbose_failures --config=ios_arm64 -c opt //tensorflow/lite/ios:TensorFlowLiteCMetal_framework\r\nINFO: Options provided by the client:\r\n Inherited 'common' options: --isatty=1 --terminal_columns=170\r\nINFO: Reading rc options for 'build' from /Users/thao/Desktop/tensorflow/.bazelrc:\r\n Inherited 'common' options: --experimental_repo_remote_exec\r\nINFO: Reading rc options for 'build' from /Users/thao/Desktop/tensorflow/.bazelrc:\r\n 'build' options: --define framework_shared_object=true --define tsl_protobuf_header_only=true --define=use_fast_cpp_protos=true --define=allow_oversize_protos=true --spawn_strategy=standalone -c opt --announce_rc --define=grpc_no_ares=true --noincompatible_remove_legacy_whole_archive --enable_platform_specific_config --define=with_xla_support=true --config=short_logs --config=v2 --define=no_aws_support=true --define=no_hdfs_support=true --experimental_cc_shared_library --experimental_link_static_libraries_once=false\r\nINFO: Reading rc options for 'build' from /Users/thao/Desktop/tensorflow/.tf_configure.bazelrc:\r\n 'build' options: --action_env PYTHON_BIN_PATH=/Users/thao/miniforge3/bin/python --action_env PYTHON_LIB_PATH=/Users/thao/miniforge3/lib/python3.10/site-packages --python_path=/Users/thao/miniforge3/bin/python\r\nINFO: Reading rc options for 'build' from /Users/thao/Desktop/tensorflow/.bazelrc:\r\n 'build' options: --deleted_packages=tensorflow/compiler/mlir/tfrt,tensorflow/compiler/mlir/tfrt/benchmarks,tensorflow/compiler/mlir/tfrt/jit/python_binding,tensorflow/compiler/mlir/tfrt/jit/transforms,tensorflow/compiler/mlir/tfrt/python_tests,tensorflow/compiler/mlir/tfrt/tests,tensorflow/compiler/mlir/tfrt/tests/ir,tensorflow/compiler/mlir/tfrt/tests/analysis,tensorflow/compiler/mlir/tfrt/tests/jit,tensorflow/compiler/mlir/tfrt/tests/lhlo_to_tfrt,tensorflow/compiler/mlir/tfrt/tests/lhlo_to_jitrt,tensorflow/compiler/mlir/tfrt/tests/tf_to_corert,tensorflow/compiler/mlir/tfrt/tests/tf_to_tfrt_data,tensorflow/compiler/mlir/tfrt/tests/saved_model,tensorflow/compiler/mlir/tfrt/transforms/lhlo_gpu_to_tfrt_gpu,tensorflow/core/runtime_fallback,tensorflow/core/runtime_fallback/conversion,tensorflow/core/runtime_fallback/kernel,tensorflow/core/runtime_fallback/opdefs,tensorflow/core/runtime_fallback/runtime,tensorflow/core/runtime_fallback/util,tensorflow/core/tfrt/common,tensorflow/core/tfrt/eager,tensorflow/core/tfrt/eager/backends/cpu,tensorflow/core/tfrt/eager/backends/gpu,tensorflow/core/tfrt/eager/core_runtime,tensorflow/core/tfrt/eager/cpp_tests/core_runtime,tensorflow/core/tfrt/gpu,tensorflow/core/tfrt/run_handler_thread_pool,tensorflow/core/tfrt/runtime,tensorflow/core/tfrt/saved_model,tensorflow/core/tfrt/graph_executor,tensorflow/core/tfrt/saved_model/tests,tensorflow/core/tfrt/tpu,tensorflow/core/tfrt/utils\r\nINFO: Found applicable config definition build:short_logs in file /Users/thao/Desktop/tensorflow/.bazelrc: --output_filter=DONT_MATCH_ANYTHING\r\nINFO: Found applicable config definition build:v2 in file /Users/thao/Desktop/tensorflow/.bazelrc: --define=tf_api_version=2 --action_env=TF2_BEHAVIOR=1\r\nINFO: Found applicable config definition build:ios_arm64 in file /Users/thao/Desktop/tensorflow/.bazelrc: --config=ios --cpu=ios_arm64\r\nINFO: Found applicable config definition build:ios in file /Users/thao/Desktop/tensorflow/.bazelrc: --apple_platform_type=ios --apple_bitcode=embedded --copt=-fembed-bitcode --copt=-Wno-c++11-narrowing --noenable_platform_specific_config --copt=-w --cxxopt=-std=c++17 --host_cxxopt=-std=c++17 --define=with_xla_support=false\r\nINFO: Build option --cxxopt has changed, discarding analysis cache.\r\nERROR: /private/var/tmp/_bazel_thao/26d40dc75f2c247e7283b353a9ab184f/external/local_config_cc/BUILD:48:19: in cc_toolchain_suite rule @local_config_cc//:toolchain: cc_toolchain_suite '@local_config_cc//:toolchain' does not contain a toolchain for cpu 'ios_arm64'\r\nERROR: /private/var/tmp/_bazel_thao/26d40dc75f2c247e7283b353a9ab184f/external/local_config_cc/BUILD:48:19: Analysis of target '@local_config_cc//:toolchain' failed\r\nERROR: Analysis of target '//tensorflow/lite/ios:TensorFlowLiteCMetal_framework' failed; build aborted: \r\nINFO: Elapsed time: 45.455s\r\nINFO: 0 processes.\r\nFAILED: Build did NOT complete successfully (66 packages loaded, 1118 targets configured)`\r\n\r\n**Here's why we have that policy**: TensorFlow developers respond to issues. We want to focus on work that benefits the whole community, e.g., fixing bugs and adding features. Support only helps individuals. GitHub also notifies thousands of people when issues are filed. We want them to see you communicating an interesting problem, rather than being redirected to Stack Overflow.\r\n\r\n------------------------\r\n\r\n### System information\r\nMacOS-M1Max : 13.3\r\nTensorflow:2.9.2\r\nPython: 3.10.0\r\n\r\n\r\n\r\n### Describe the problem\r\nDescribe the problem clearly here. Be sure to convey here why it's a bug in TensorFlow or a feature request.\r\n\r\n### Source code / logs\r\nInclude any logs or source code that would be helpful to diagnose the problem. If including tracebacks, please include the full traceback. Large logs and files should be attached. Try to provide a reproducible test case that is the bare minimum necessary to generate the problem.\r\n"
inference: true
SetFit with sentence-transformers/all-mpnet-base-v2
This is a SetFit model that can be used for Text Classification. This SetFit model uses sentence-transformers/all-mpnet-base-v2 as the Sentence Transformer embedding model. A LogisticRegression instance is used for classification.
The model has been trained using an efficient few-shot learning technique that involves:
- Fine-tuning a Sentence Transformer with contrastive learning.
- Training a classification head with features from the fine-tuned Sentence Transformer.
Model Details
Model Description
- Model Type: SetFit
- Sentence Transformer body: sentence-transformers/all-mpnet-base-v2
- Classification head: a LogisticRegression instance
- Maximum Sequence Length: 384 tokens
- Number of Classes: 3 classes
Model Sources
- Repository: SetFit on GitHub
- Paper: Efficient Few-Shot Learning Without Prompts
- Blogpost: SetFit: Efficient Few-Shot Learning Without Prompts
Model Labels
Label | Examples |
---|---|
question |
|
feature |
|
bug |
|
Uses
Direct Use for Inference
First install the SetFit library:
pip install setfit
Then you can load this model and run inference.
from setfit import SetFitModel
# Download from the 🤗 Hub
model = SetFitModel.from_pretrained("setfit_model_id")
# Run inference
preds = model("Data init API for TFLite Swift <details><summary>Click to expand!</summary>
### Issue Type
Feature Request
### Source
source
### Tensorflow Version
2.8+
### Custom Code
No
### OS Platform and Distribution
_No response_
### Mobile device
_No response_
### Python version
_No response_
### Bazel version
_No response_
### GCC/Compiler version
_No response_
### CUDA/cuDNN version
_No response_
### GPU model and memory
_No response_
### Current Behaviour?
```shell
The current Swift API only has `init` functions from files on disk unlike the Java (Android) API which has a byte buffer initializer. It'd be convenient if the Swift API could initialize `Interpreters` from `Data`.
Standalone code to reproduce the issue
No code. This is a feature request
Relevant log output
No response")
<!--
### Downstream Use
*List how someone could finetune this model on their own dataset.*
-->
<!--
### Out-of-Scope Use
*List how the model may foreseeably be misused and address what users ought not to do with the model.*
-->
<!--
## Bias, Risks and Limitations
*What are the known or foreseeable issues stemming from this model? You could also flag here known failure cases or weaknesses of the model.*
-->
<!--
### Recommendations
*What are recommendations with respect to the foreseeable issues? For example, filtering explicit content.*
-->
## Training Details
### Training Set Metrics
| Training set | Min | Median | Max |
|:-------------|:----|:---------|:-----|
| Word count | 5 | 353.7433 | 6124 |
| Label | Training Sample Count |
|:---------|:----------------------|
| bug | 200 |
| feature | 200 |
| question | 200 |
### Training Hyperparameters
- batch_size: (16, 2)
- num_epochs: (1, 1)
- max_steps: -1
- sampling_strategy: oversampling
- num_iterations: 20
- body_learning_rate: (2e-05, 1e-05)
- head_learning_rate: 0.01
- loss: CosineSimilarityLoss
- distance_metric: cosine_distance
- margin: 0.25
- end_to_end: False
- use_amp: False
- warmup_proportion: 0.1
- seed: 42
- eval_max_steps: -1
- load_best_model_at_end: False
### Training Results
| Epoch | Step | Training Loss | Validation Loss |
|:------:|:----:|:-------------:|:---------------:|
| 0.0007 | 1 | 0.1719 | - |
| 0.0067 | 10 | 0.2869 | - |
| 0.0133 | 20 | 0.2513 | - |
| 0.02 | 30 | 0.1871 | - |
| 0.0267 | 40 | 0.2065 | - |
| 0.0333 | 50 | 0.2302 | - |
| 0.04 | 60 | 0.1645 | - |
| 0.0467 | 70 | 0.1887 | - |
| 0.0533 | 80 | 0.1376 | - |
| 0.06 | 90 | 0.1171 | - |
| 0.0667 | 100 | 0.1303 | - |
| 0.0733 | 110 | 0.121 | - |
| 0.08 | 120 | 0.1126 | - |
| 0.0867 | 130 | 0.1247 | - |
| 0.0933 | 140 | 0.1764 | - |
| 0.1 | 150 | 0.0401 | - |
| 0.1067 | 160 | 0.1571 | - |
| 0.1133 | 170 | 0.0186 | - |
| 0.12 | 180 | 0.0501 | - |
| 0.1267 | 190 | 0.1003 | - |
| 0.1333 | 200 | 0.0152 | - |
| 0.14 | 210 | 0.0784 | - |
| 0.1467 | 220 | 0.1423 | - |
| 0.1533 | 230 | 0.1313 | - |
| 0.16 | 240 | 0.0799 | - |
| 0.1667 | 250 | 0.0542 | - |
| 0.1733 | 260 | 0.0426 | - |
| 0.18 | 270 | 0.047 | - |
| 0.1867 | 280 | 0.0062 | - |
| 0.1933 | 290 | 0.0085 | - |
| 0.2 | 300 | 0.0625 | - |
| 0.2067 | 310 | 0.095 | - |
| 0.2133 | 320 | 0.0262 | - |
| 0.22 | 330 | 0.0029 | - |
| 0.2267 | 340 | 0.0097 | - |
| 0.2333 | 350 | 0.063 | - |
| 0.24 | 360 | 0.0059 | - |
| 0.2467 | 370 | 0.0016 | - |
| 0.2533 | 380 | 0.0025 | - |
| 0.26 | 390 | 0.0033 | - |
| 0.2667 | 400 | 0.0006 | - |
| 0.2733 | 410 | 0.0032 | - |
| 0.28 | 420 | 0.0045 | - |
| 0.2867 | 430 | 0.0013 | - |
| 0.2933 | 440 | 0.0011 | - |
| 0.3 | 450 | 0.001 | - |
| 0.3067 | 460 | 0.0044 | - |
| 0.3133 | 470 | 0.001 | - |
| 0.32 | 480 | 0.0009 | - |
| 0.3267 | 490 | 0.0004 | - |
| 0.3333 | 500 | 0.0006 | - |
| 0.34 | 510 | 0.001 | - |
| 0.3467 | 520 | 0.0003 | - |
| 0.3533 | 530 | 0.0008 | - |
| 0.36 | 540 | 0.0003 | - |
| 0.3667 | 550 | 0.0023 | - |
| 0.3733 | 560 | 0.0336 | - |
| 0.38 | 570 | 0.0004 | - |
| 0.3867 | 580 | 0.0003 | - |
| 0.3933 | 590 | 0.0006 | - |
| 0.4 | 600 | 0.0008 | - |
| 0.4067 | 610 | 0.0011 | - |
| 0.4133 | 620 | 0.0002 | - |
| 0.42 | 630 | 0.0004 | - |
| 0.4267 | 640 | 0.0005 | - |
| 0.4333 | 650 | 0.0601 | - |
| 0.44 | 660 | 0.0003 | - |
| 0.4467 | 670 | 0.0003 | - |
| 0.4533 | 680 | 0.0006 | - |
| 0.46 | 690 | 0.0005 | - |
| 0.4667 | 700 | 0.0003 | - |
| 0.4733 | 710 | 0.0006 | - |
| 0.48 | 720 | 0.0001 | - |
| 0.4867 | 730 | 0.0002 | - |
| 0.4933 | 740 | 0.0002 | - |
| 0.5 | 750 | 0.0002 | - |
| 0.5067 | 760 | 0.0002 | - |
| 0.5133 | 770 | 0.0016 | - |
| 0.52 | 780 | 0.0001 | - |
| 0.5267 | 790 | 0.0005 | - |
| 0.5333 | 800 | 0.0004 | - |
| 0.54 | 810 | 0.0039 | - |
| 0.5467 | 820 | 0.0031 | - |
| 0.5533 | 830 | 0.0008 | - |
| 0.56 | 840 | 0.0003 | - |
| 0.5667 | 850 | 0.0002 | - |
| 0.5733 | 860 | 0.0002 | - |
| 0.58 | 870 | 0.0002 | - |
| 0.5867 | 880 | 0.0001 | - |
| 0.5933 | 890 | 0.0004 | - |
| 0.6 | 900 | 0.0002 | - |
| 0.6067 | 910 | 0.0008 | - |
| 0.6133 | 920 | 0.0005 | - |
| 0.62 | 930 | 0.0005 | - |
| 0.6267 | 940 | 0.0002 | - |
| 0.6333 | 950 | 0.0001 | - |
| 0.64 | 960 | 0.0002 | - |
| 0.6467 | 970 | 0.0007 | - |
| 0.6533 | 980 | 0.0002 | - |
| 0.66 | 990 | 0.0002 | - |
| 0.6667 | 1000 | 0.0002 | - |
| 0.6733 | 1010 | 0.0002 | - |
| 0.68 | 1020 | 0.0002 | - |
| 0.6867 | 1030 | 0.0002 | - |
| 0.6933 | 1040 | 0.0004 | - |
| 0.7 | 1050 | 0.0076 | - |
| 0.7067 | 1060 | 0.0002 | - |
| 0.7133 | 1070 | 0.0002 | - |
| 0.72 | 1080 | 0.0001 | - |
| 0.7267 | 1090 | 0.0002 | - |
| 0.7333 | 1100 | 0.0001 | - |
| 0.74 | 1110 | 0.0365 | - |
| 0.7467 | 1120 | 0.0002 | - |
| 0.7533 | 1130 | 0.0002 | - |
| 0.76 | 1140 | 0.0003 | - |
| 0.7667 | 1150 | 0.0002 | - |
| 0.7733 | 1160 | 0.0002 | - |
| 0.78 | 1170 | 0.0004 | - |
| 0.7867 | 1180 | 0.0001 | - |
| 0.7933 | 1190 | 0.0001 | - |
| 0.8 | 1200 | 0.0001 | - |
| 0.8067 | 1210 | 0.0001 | - |
| 0.8133 | 1220 | 0.0002 | - |
| 0.82 | 1230 | 0.0002 | - |
| 0.8267 | 1240 | 0.0001 | - |
| 0.8333 | 1250 | 0.0001 | - |
| 0.84 | 1260 | 0.0002 | - |
| 0.8467 | 1270 | 0.0002 | - |
| 0.8533 | 1280 | 0.0 | - |
| 0.86 | 1290 | 0.0002 | - |
| 0.8667 | 1300 | 0.032 | - |
| 0.8733 | 1310 | 0.0001 | - |
| 0.88 | 1320 | 0.0001 | - |
| 0.8867 | 1330 | 0.0001 | - |
| 0.8933 | 1340 | 0.0003 | - |
| 0.9 | 1350 | 0.0001 | - |
| 0.9067 | 1360 | 0.0001 | - |
| 0.9133 | 1370 | 0.0001 | - |
| 0.92 | 1380 | 0.0001 | - |
| 0.9267 | 1390 | 0.0001 | - |
| 0.9333 | 1400 | 0.0001 | - |
| 0.94 | 1410 | 0.0001 | - |
| 0.9467 | 1420 | 0.0001 | - |
| 0.9533 | 1430 | 0.031 | - |
| 0.96 | 1440 | 0.0001 | - |
| 0.9667 | 1450 | 0.0003 | - |
| 0.9733 | 1460 | 0.0001 | - |
| 0.98 | 1470 | 0.0001 | - |
| 0.9867 | 1480 | 0.0001 | - |
| 0.9933 | 1490 | 0.0001 | - |
| 1.0 | 1500 | 0.0001 | - |
### Framework Versions
- Python: 3.10.12
- SetFit: 1.0.3
- Sentence Transformers: 3.0.1
- Transformers: 4.39.0
- PyTorch: 2.3.0+cu121
- Datasets: 2.20.0
- Tokenizers: 0.15.2
## Citation
### BibTeX
```bibtex
@article{https://doi.org/10.48550/arxiv.2209.11055,
doi = {10.48550/ARXIV.2209.11055},
url = {https://arxiv.org/abs/2209.11055},
author = {Tunstall, Lewis and Reimers, Nils and Jo, Unso Eun Seo and Bates, Luke and Korat, Daniel and Wasserblat, Moshe and Pereg, Oren},
keywords = {Computation and Language (cs.CL), FOS: Computer and information sciences, FOS: Computer and information sciences},
title = {Efficient Few-Shot Learning Without Prompts},
publisher = {arXiv},
year = {2022},
copyright = {Creative Commons Attribution 4.0 International}
}